- Centre for Positive Health Sciences, Royal College of Surgeons in Ireland, Dublin, Ireland
Introduction: Digital Health Interventions (DHIs) have been identified as a solution to the United Nations Sustainable Development Goals (SDG3) for health promotion and prevention. However, DHIs face criticism for shallow and transactional engagement and retention challenges. Integrating DHIs with health coaching represents a promising solution that might address these issues by combining the scalable and accessible nature of DHIs with the meaningful and engaging nature of health coaching. This systematic review aims to synthesise existing peer-reviewed research on coach-facilitated DHIs to understand how digital health coaching is being used in DHIs and the impact it has on engagement and lifestyle outcomes.
Methods: Studies examining DHIs with a coaching component addressing lifestyle outcomes were included. A search of APA PsychINFO, Medline, Web of Science, and Scopus was performed from inception to February 2025. Three authors conducted the study selection, quality appraisal using the Mixed Methods Appraisal Tool (MMAT), and data extraction. Data extraction captured study characteristics, coaching features, participant engagement, and lifestyle outcomes.
Results: Thirty-five studies were identified and synthesised using a narrative synthesis approach. This review highlights three coaching modalities in DHIs: digital human coaching, Artificial Intelligence (AI) coaching, and hybrid (human-AI) coaching. All coaching modalities demonstrated feasibility and acceptability.
Discussion: While both human and AI coaching have shown a positive impact on both engagement and lifestyle outcomes, hybrid approaches need further refinement to harness AI's scalability and the depth of human coaching. However, the variability of engagement metrics and coaching protocols limited study comparability. Standardising how engagement and coaching delivery are measured and contextualised is crucial for advancing evidence-based digital health coaching. This review followed PRISMA guidelines and was registered in PROSPERO (Registration number: CRD42022363279). The Irish Research Council supported this work.
Systematic Review Registration: https://www.crd.york.ac.uk/PROSPERO/view/CRD42022363279, identifier: CRD42022363279.
1 Introduction
The United Nations (UN) has developed a universal agenda for sustainable development, with the overarching vision for all human beings to thrive and to reach their full potential in dignity and equality in a healthy environment (1). The third goal (SDG3) of this agenda is to “ensure healthy lives and promote wellbeing for all at all ages” (1). Under this goal, the UN emphasised reducing noncommunicable disease (NCD)-related mortality rates by one-third through prevention and treatment and promoting mental health and wellbeing (1). Digital health has been identified as a feasible, accessible and affordable solution to the UN goals of preventing NCDs and promoting health and wellbeing (2). Digital health is a complex and multifaceted field of both knowledge and practice, focused on the development and use of various technologies (i.e., smart watches, digital tracking tools, robotics, artificial intelligence and machine learning) for improving health (2–4). There has been substantial growth in the field of digital health research, especially in terms of evidence-based digital health interventions (DHIs) (5).
DHIs create new opportunities for medical doctors, health professionals and other caregivers to scale and tailor health and lifestyle interventions at a lower cost (5). DHIs are particularly effective for promoting healthy lifestyle behaviours like healthy eating, physical activity, stress reduction and psychological wellbeing (6–10). These behaviours, in turn, can promote healthier living and aid in the prevention and management of lifestyle-related NCDs such as cardiovascular disease, diabetes, certain cancers and chronic respiratory diseases (4, 5, 11–13). However, challenges exist in DHI implementation, particularly with self-guided or automated interventions. Despite their low cost and scalability, these interventions are often perceived as shallow, impersonal, and transactional, with participants preferring human support for more meaningful engagement (4, 14–17). Retention and engagement also pose significant issues, with a pooled estimated dropout rate of 43% across DHIs (18–20).
Integrating health coaching with DHIs may offer a solution to the limitation of passive or automated interventions by providing live and engaging support to participants. Health coaching is a non-clinical, evidence-based health promotion intervention that promotes sustainable health behaviours and improves lifestyle outcomes through personalised, solution-focused, person-centred support. By tailoring interventions to individual needs and fostering a collaborative relationship, health coaching empowers clients to make informed decisions about their health and facilitates behaviour change (21–23). Health coaching is already recognised as a valuable asset to healthcare systems. Specifically, for the health promotion and prevention of NCDs (24, 25). As such, health coach-facilitated DHIs could create meaningful yet scalable and accessible digital health solutions for health promotion and NCD prevention (26–28).
This integration paves the way for a new field of research relating to digital health coaching. There has already been a substantial surge of professional coaches transitioning to digital spaces, with 93.3% of coaches globally transitioning to online coaching (29). In the digital space, health coaching is mediated through technology, where coaches interact with coachees via digital platforms to guide behaviour change (30). This allows coaches and clients to interact regardless of geographical location and through different modalities (i.e., through text, video call or phone call) (31). While the conversation between coaches and clients remains central to digital health coaching, it can be supported by a variety of technologies, including digital tracking tools (i.e., smart watches, glucose monitors), self-guided educational modules, habit tracking and automated reminders. Additionally, recent advancements in artificial intelligence (AI) have spurred research into conversational agent coaching or chatbot coaches as flexible, accessible and cost-efficient alternatives to human-facilitated digital health coaching (32, 33). Chatbots are AI-driven programmes designed to interact with individuals using natural language that mimics human dialogue through algorithm-generated responses (34). However, the active engagement between the coach (AI or human) and coachee through personalised and real-time interaction is central to digital health coaching.
While there has been increasing interest in the integration of coaching within DHIs, a clear gap remains in understanding the impact of different coaching modalities (e.g., human, AI, and hybrid) on participant engagement and health outcomes. Although some studies have investigated coach-facilitated DHIs, comprehensive analyses and synthesis regarding the effectiveness of these modalities, particularly their role in enhancing lifestyle behaviours and preventing NCDs, are limited. This systematic review aims to synthesise existing research on coach-facilitated DHIs to understand how digital health coaching is being used in DHIs and the impact it has on lifestyle outcomes and engagement. Ultimately, this review seeks to provide evidence to inform the development of future digital health interventions and research.
2 Materials and methods
The methodology for this systematic review complies with the Preferred Reporting Items for Systematic Reviews and Meta-Analyses (PRISMA) guidelines for systematic reviews (35) (Supplementary File S1). This protocol has been registered on PROSPERO (Registration number: CRD42022363279). After registration, amendments were made to enhance the comprehensiveness of the review. The review timeline was updated to incorporate a more recent search date. Additionally, further details were added to the inclusion criteria to clarify modifications. Lastly, the search strategy was revised to reflect the updated search parameters.
2.1 Inclusion and exclusion criteria
The PICO framework guided the definition of eligibility for this review (Table 1). However, in this review, the traditional “Comparator” element in PICO was replaced with “Context”, as this review focuses on the integration of coaching within DHIs without the need for a direct comparator.
This review seeks to gather evidence of coach-facilitated DHIs implemented to increase the health and wellbeing of populations. While this largely falls into preventative interventions to help reduce the risk of NCDs, substantial studies are using coach-facilitated DHIs to help increase the health and wellbeing of cohorts living with NCDs (predominantly cancers and diabetes). It would be remiss to exclude these cohorts because of their disease status, despite the intervention aligning with inclusion criteria. As a result, we included adults living with NCDs once the primary outcomes were focused on lifestyle outcomes and not clinical or medical outcomes or markers (i.e., HbA1c, Cholesterol, BMI, weight reduction or waist circumference). This review concentrated on the adult population (18 years and older). As such, studies involving adolescents or children were excluded.
Peer-reviewed studies were considered if they explored digital coaching in DHIs and addressed at least one of the lifestyle medicine pillars: sleep, physical activity, psychological wellbeing and stress management, substance use, or healthy eating. Studies were excluded if they focused on medical interventions or used coaching as a part of medical or mental health treatments (i.e., coaching for patient education, trust building for treatments, pain management, medication adherence) that were not directly aimed at increasing lifestyle or wellbeing outcomes. Similarly, studies with clinical primary outcomes or biological markers as the primary outcome were excluded.
Studies that incorporated human, AI or hybrid (AI and human) coaching components alongside DHIs were included. For the inclusion criteria, digital health coaching was defined as a coach-coachee partnership facilitated online with the purpose of providing tailored support to enhance the DHI and promote behaviour change and health outcomes among participants. The coaching component of the DHI must align with this definition, meaning that coaching through ad hoc non-interactive messages or notifications was excluded. Studies that explored in-person or face-to-face interventions only, as well as career, executive or athletic coaching, were excluded. Finally, all peer-reviewed studies from any country were included, and no publication date limit was applied, given the limited literature on coaching, especially digital coaching. Studies that were not available in English were excluded.
2.2 Search strategy
The search strategy used keywords identified through an initial review of the literature. Keywords were grouped using Boolean operators and truncations. The PICO Framework also guided the formation of the final search strategy (Table 2).
2.3 Informational sources
We conducted searches in electronic databases, including APA PsychINFO, Medline, Web of Science, and Scopus, on February 1, 2025. In addition to the electronic database searches, backward and forward citation searching of included studies was conducted to identify any additional relevant studies. Furthermore, forward searching of protocols deemed relevant during screening was carried out to ensure the inclusion of studies that may have been missed in the initial search.
2.4 Study screening
Covidence, an online specialised systematic review website, was used to screen studies. One reviewer (CL) screened the titles and abstracts of the identified studies based on the eligibility criteria. Three reviewers (CL, ROD, and JL) then independently reviewed the identified full-text studies. Reviewers met to discuss and resolve any conflicts or disagreements. If consensus could not be reached, a fourth reviewer (PJD) was designated to assess the relevant records. Three reviewers are qualified health coaches accredited by the European Mentoring and Coaching Council (EMCC). Two reviewers hold psychology degrees (CL, ROD), and the fourth reviewer holds a PhD in immunology and a degree in counselling and psychotherapy (PJD). The third reviewer (JL) has a biomedical engineering degree with specific expertise in digital health research.
2.5 Data extraction process
Two reviewers (CL & ROD) independently extracted the data using the Covidence Data Extraction template. To resolve discrepancies in the data extracted, reviewers came together to review data extraction and any existing conflicts. Conflicts that couldn't be resolved were referred to a third reviewer for resolution (PJD). However, this was not needed. The following characteristics were recorded: author, year, study aim, participant description and inclusion and exclusion status, total participant number, study design, lifestyle focus, measures taken, key outcomes, coaching delivery and intensity, coaching theory, role of coaching, length of intervention, and limitations.
2.6 Quality assessment
The Mixed Methods Appraisal Tool assessed the quality of all studies included in the systematic review. This was conducted independently by two reviewers (CL and ROD), who then jointly reviewed independent quality appraisal for any conflicts. Unresolved conflicts were referred to a third reviewer (PJD), though this step was not needed. The final MMAT results are included in the supplementary files (Supplementary File S2).
2.7 Study synthesis
The identified studies were synthesised using a narrative synthesis in relation to the study question: How is digital health coaching used in DHIs, and what is the impact of lifestyle outcomes and engagement? The synthesis followed the steps outlined by Popay (36), which included: becoming familiar with the studies, organising them into logical categories, comparing and synthesising the studies, exploring the relationships within and between the studies and synthesising the data under the relevant themes. Studies included in this review were first grouped by coaching modality (i.e., human, AI, or hybrid coaching) and then further categorised by targeted lifestyle outcomes (physical activity, psychological wellbeing, stress management, healthy eating, sleep, and substance use) and engagement outcomes. We evaluated the consistency of the findings across studies to assess the certainty of evidence. Missing or inconsistent data that was not a criterion for inclusion (i.e., engagement statistics, coaching theories, and length of intervention) was noted during synthesis and presented in the results. Studies were not excluded based on these missing data, but it was considered as a limitation during synthesis. A meta-analysis could not be performed due to significant variations in sample populations, outcome measures, and study designs across the included studies.
3 Results
The database search yielded a total of 4,894 studies. After removing 1,748 duplicates, a further 2,936 studies were removed after title and abstract screening. One hundred and seventy-six studies were removed during the full-text review. In total, 35 studies were included in this review. The complete screening process is illustrated through the PRISMA diagram (Figure 1).
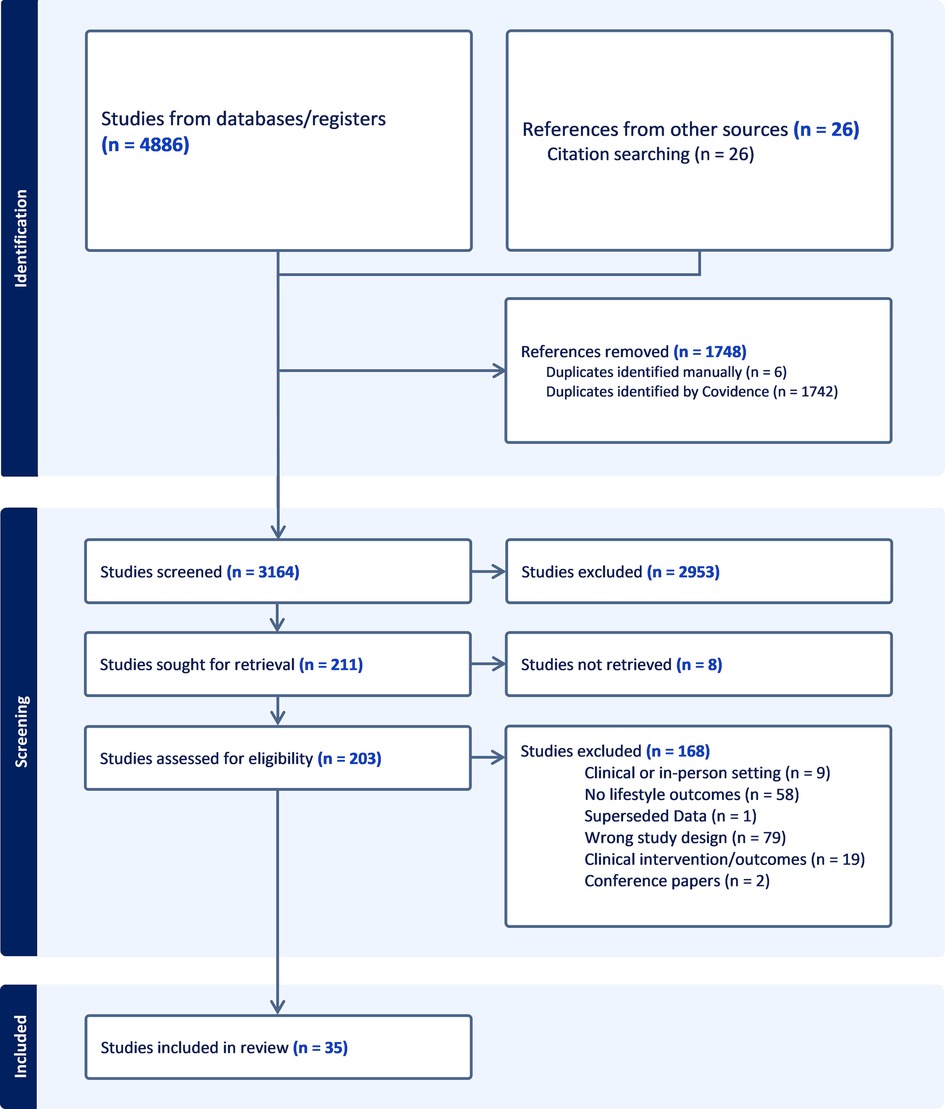
Figure 1. PRISMA flow diagram for systematic reviews illustrating the study selection process. Adapted with permission from “PRISMA 2020 flow diagram template for systematic reviews”, by Page et al., licensed under CC BY 4.0.
3.1 Quality assessment
No articles were excluded from the review based on the MMAT quality appraisal score (Supplementary File S2). Regarding the methodological rigour of the included articles, 11 studies scored 100% (5/5), and 23 scored 80% (4/5), indicating high quality. Only one study scored 60% (3/5), indicating medium quality. This demonstrates that the majority of studies included in the review met a high standard of methodological rigor.
3.2 Description of the included studies
Thirty-five studies were included for full review and data extraction. Table 3 summarises the study characteristics, including year of publication, country, length of intervention, number of participants, study design, intervention description, lifestyle areas addressed, measures used, and key outcomes. Most of the studies were pilot, feasibility, or early-stage studies, with only seven studies (20.6%) being full-scale randomised controlled trials (37–43). Intervention lengths ranged from 1 week to 12 months, and sample sizes ranged from 7 to 3,629 participants (Table 3).
To assess the heterogeneity of the participants, demographic information, including sex, age, race, and education, was collected and is presented in Table 4. Participants were predominantly Caucasian, though African American/Black, Asian, multiracial, and other racial groups were represented in smaller proportions. Notably, four studies had a larger representation of African American/Black (47) and Asian participants (39, 48, 51). The mean age of participants varied widely. Participants were largely aged between 40 and 60 years, with the exception of outlying studies focusing on younger adults and students (18–34 years) (47–49, 51, 52, 55, 61, 65, 67, 69) and older populations (60 + years) (38, 43, 45, 46, 50). Women were more frequently recruited for digital health interventions, with female participation ranging from 25.2% to 100% across studies. Education levels were inconsistently reported, but in studies that provided this information, the percentage of participants with a college degree ranged from 20.7% to 95%.
3.3 Presence of coaching in DHIs
This review focused on the role and impact of health coaching in DHIs. Table 5 summarises the key characteristics of coaching that are evidenced across included studies. Coaching was exhibited through two crucial elements: (1) an active coaching component and (2) evidence-based coaching theories. Theories not only guided the coaching process (27, 40–42, 45, 46, 50, 53, 57, 58, 61, 62, 68) but also influenced the overall design of DHIs and AI chatbots (17, 38, 48, 49, 51, 52, 55, 56, 60, 65–67, 69). The active coaching component of DHIs was evident through three different delivery modes: (1) digital human coaching, (2) AI-powered coaching, or (3) a mix of both, which we refer to as hybrid coaching (Table 5). Using the three modes of coaching identified, this review presents the features of coaching, followed by the trends of lifestyle outcomes and engagement that emerged from the included studies.
3.3.1 Digital human coaching
Digital human coaching refers to any study that used a human coach alongside DHIs. Eighteen of the included studies (51%) employed human coaching as a part of their interventions (27, 39–43, 45–47, 50, 53, 54, 57, 58, 61, 62, 64, 68). Coaching was often enhanced with digital tracking tools (e.g., smart scales, watches, accelerometers, and breath sensors) (41–43, 46, 53, 57, 58, 62, 64) and educational resources or modules (27, 39–41, 47, 50, 54, 58, 61, 62, 68). One study used a telepresence robot controlled remotely by practitioners to facilitate communication with participants (45). A consistent finding emerged from these studies: the human coach played a crucial role in engaging participants and enhancing motivation, adherence, behaviour change, and personalisation (39, 46, 58, 61, 62). However, the intensity of human coaching delivered varied. In most cases (67%), the coach was central to the intervention, interacting regularly with participants. In contrast, six studies assigned coaches a more supportive role, primarily supplementing educational modules, training, and activity monitors (27, 39, 40, 46, 54, 58). In these instances, participants primarily engaged with the digital components and the coaches provided additional support to reinforce engagement and adherence, focusing only on issues related to the main intervention (27, 39, 40, 46, 54, 58).
3.3.2 AI-powered coaching
Thirteen included studies (37%) analysed the use of AI-powered coaching using conversational agents (i.e., chatbots) to deliver DHIs (17, 38, 48, 49, 51, 52, 55, 56, 60, 65–67, 69). The development of conversational agents and chatbots in these studies was primarily based on Natural Language Processing (NLP) models (17, 49, 51, 56) or rule-based approaches, including decision-tree algorithms (38, 51). Most AI coaching interventions were delivered remotely through a digital platform, available 24/7 (38, 45, 48, 49, 51, 52, 55, 56, 60, 65, 66, 69). However, one study used on-site AI coaching in a controlled environment (17). Eleven studies mimicked human coaching sessions through quick text-based sessions with the AI chatbot. One chatbot, however, was primarily voice-based, with text-based options for certain activities. This chatbot could interpret speech and synthesise voices to respond to participants (17). Three studies provided unlimited access to the AI chatbots, enabling ad hoc questions, as well as guidance, monitoring and feedback outside of structured coaching sessions (56, 65, 66). Unlike human coaches, AI coaches were central to all DHIs. Given the artificial nature of the coaching design, no coaching qualifications or accreditations were reported in AI coaching studies.
3.3.3 Hybrid coaching
Four studies (11%) investigated hybrid coaching in DHIs (37, 44, 59, 63). Hybrid coaching refers to any study that integrates human coaches with AI-powered features within a DHI. Three studies investigated the impact of combining human coaching (delivered via video or text) with automated personalisation, support, advice, and motivation delivered by in-app messages, nudges or knowledge pills (short tips or pieces of advice) (37, 44, 59). The AI in these studies typically performed administrative tasks such as (a) sending personalised interventions and educational content (44, 59, 63), (b) monitoring activities, progress, engagement and adherence (37, 63), (c) sending motivational messages or nudges (37, 63), and (d) administering questionnaires (59). While two studies used AI and human coaching in parallel, the third study employed a “human-in-the-loop” approach for delivering hybrid coaching, where the human coach monitored, modified, and validated the AI coach's recommendations (59). The fourth study combined human coaching with a digital coach (similar to AI-powered coaching). The digital coach was an avatar that participants could interact with in-app through text-based prompts (63). This resulted in the AI-powered coach taking on more interactive and meaningful tasks like goal-setting with participants (63).
3.4 Lifestyle outcomes across coaching modalities
The reviewed studies assessed changes in physical activity, psychological wellbeing, stress management, healthy eating, sleep, and substance use across human-delivered, AI, and hybrid coaching interventions (Table 3). Among these lifestyle domains, physical activity was the most commonly addressed, covered by 55.8% of studies (n = 19), followed by psychological wellbeing (n = 18; 52.9%). Stress management was examined in nine studies and was closely associated with psychological wellbeing, with many studies addressing both constructs together. Given its interrelated role, stress management was discussed as a subset of psychological wellbeing in this review. Healthy eating was explored in 13 studies, while sleep (n = 4) and substance use (n = 2) were less commonly examined.
3.4.1 Physical activity
Physical activity was assessed through a variety measures, including active minutes, Metabolic Equivalent Tasks (METs), daily steps, or sedentary time measured through biometric feedback (37, 42–44, 59, 64, 66) and self-reported physical activity assessments (41, 45–48, 51, 56, 58, 61, 63, 66), and qualitative interviews (54). Studies examining human coaching reported mixed findings regarding its impact on physical activity. Several studies found improvements in physical activity measured through changes in METs (41, 47) and moderate-to-vigorous activity (MVPA) minutes (42, 43). However, other studies showed no meaningful changes or a decline in physical activity (45, 46, 64). Some studies also found that human-coach-facilitated DHIs promoted shifts in participants' awareness, motivation, and readiness to change, leading to active change in physical activity (46, 47), along with an increase in purposeful movement, like taking the stairs or walking instead of driving (54).
AI coaching generally showed consistent positive effects on physical activity. Studies reported improvements in physical activity measures, including METs (48), step count (66), active minutes (56, 66), MVPA minutes, and reduced sitting time (51). AI coaching was also associated with improved adherence and increased motivation (56). All four hybrid studies investigated the impact of this approach on physical activity, reporting variable results. Two studies found significant to moderate improvements in physical activity through active minutes (44, 63). However, a third study found decreased active minutes and steps among both intervention and control groups (37). The fourth study did not assess changes in physical activity from baseline but did report high rates of adherence and goal completion (i.e., achieving step targets and weekly active minute goals) (59).
3.4.2 Psychological wellbeing
Psychological wellbeing was primarily assessed through the reduction of depression and anxiety symptoms (27, 40, 45, 49, 52, 60, 62, 67) and general psychological wellbeing using a variety of wellbeing and mental health scales (50, 53, 62, 63, 65, 69). Other aspects of psychological wellbeing, such as worry, emotional control, mindfulness, personal growth, and life satisfaction, were also assessed to a lesser extent (17, 27, 47, 52, 55). Human coaching interventions were associated with reductions in depressive and anxiety symptoms (27, 40, 45, 62), along with reduced worry (40) and increased emotional self-efficacy and emotional control (47, 62). However, this was not reflected in one study among coached and non-coached groups (50) and another which aimed to improve health and wellbeing but did not use scales to assess wellbeing changes (53). AI coaching generally reported positive improvements in psychological wellbeing, including reductions in depressive symptoms (49, 60, 67) and anxiety (49, 52, 60), as well as improvement in general psychological wellbeing (69). AI coaching interventions also improved other wellbeing constructs like mindfulness (52), personal growth (17, 55), and life satisfaction (55). However, two AI-coaching interventions failed to report differences in psychological wellbeing (65, 67). Only one hybrid coaching study assessed psychological wellbeing (63]. While psychological wellbeing improved from baselines, this was not sustained after the human-coaching element of the intervention ended (63).
3.4.2.1 Stress management
All human coaching interventions examining perceived stress reported positive outcomes (40, 47, 68), with some showing greater improvement compared to control groups (40, 68). However, one study observed only minimal reductions in stress levels from baseline (47). Likewise, AI coaching interventions generally led to reductions in perceived stress, ranging from significant (52, 55, 67, 69) to minor improvements (49). One AI intervention reported no positive changes in stress levels (65). Hybrid coaching interventions did not assess stress management outcomes.
3.4.3 Healthy eating
Healthy eating was examined in AI and human coaching interventions only. The most common measure used to assess dietary improvements was daily fruit and vegetable intake (41, 51, 58, 61). Other measures included overall diet quality assessed via questionnaires (39, 42), adherence to the Mediterranean diet (56), protein intake (50), behaviours related to overeating and self-regulation (48). Intervention utilising human coaching demonstrated significant improvements in protein intake (50), fruit and vegetable consumption (41, 58, 61), and overall diet quality (39, 42). Two studies explored behavioural factors influencing healthy eating, reporting increased autonomy and competence (61) and improved control over eating habits (62). AI coaching interventions also yielded positive effects on healthy eating, though to varying degrees. While all three studies showed positive changes (48, 51, 56), only one found significant improvement in their diet scores (56). The latter study focused on eating habits rather than food quality, reporting significant reduction in overeating and snacking habits as well as self-regulation in eating behaviour (48).
3.4.4 Sleep
Sleep was assessed through self-reported sleep quality (27, 51, 53), and sleep duration measured via wearable devices (59). Studies examining human coaching generally reported improvements in sleep quality. One study found that most participants either improved or maintained their sleep quality (53), while another reduced sleep disturbances, with improvements sustained at follow-up (27). In contrast, AI coaching showed minimal impact, with no significant improvements in sleep quality or sleep scores (51). Hybrid coaching interventions provided limited findings on sleep. While one hybrid study assessed sleep outcomes, baseline comparisons were not available. However, results indicated that participants met the recommended seven to eight hours of sleep on nearly half of the recorded days (59).
3.4.5 Substance use
Substance use was assessed by two studies using human coaching. Both studies showed that coaching interventions had a positive impact on decreasing alcohol consumption (58), tobacco use, and smoking quit rates (57). The human-coach-led smoking cessation intervention also enhanced participants' confidence to quit smoking and reduced perceived difficulty in maintaining abstinence (57).
3.5 Engagement and satisfaction
Engagement was measured inconsistently across studies, with definitions and measures varying widely (Table 6). The most commonly reported metric was retention or intervention completion rate. For human coaching interventions, completion and retention rates varied from 80% to 100% (27, 39–41, 46, 47, 53, 57). AI coaching interventions reported similar completion and retention rates of 90% to 93%, with three outlying studies reporting significantly lower completion rates of 58% (52), 20.3%–45.4% (49), and 9.8% (60). Hybrid coaching interventions had retention rates of 55%–56.5% (37, 44). However, one hybrid study found that the human coaching component improved adherence to syncing data from wearable devices (37).
Several studies reported correlations between engagement levels and outcomes. Studies investigating the addition of a human-coaching component in DHIs found that participants who completed coaching spent more time on the DHI, completed more educational modules (44), had better adherence to the intervention (37, 58), improved retention (61) and increased wellbeing (63). Additionally, higher engagement with AI chatbots correlated with lower anxiety and depressive symptoms (49), improved wellbeing (38), increased physical activity (66), and a higher increase in goal attainment (65). Finally, one study found that early engagement (within the first week) predicted sustained engagement throughout the intervention (56), while another reported that participants who completed the full intervention had lower stress levels (40).
3.5.1 Satisfaction
Satisfaction with the DHI interventions was reported in 37% of the included studies (n = 13) (Table 6). Among hybrid studies, 75% (3 out of 4) reported satisfaction outcomes. However, AI coaching interventions had the highest proportion of studies reporting satisfaction, accounting for 46% (6/13), compared to 22% (4/18) of human coaching studies. Overall satisfaction rates were high across studies using human coaching in their DHIs, with 81.9% to 92.5% of participants reporting satisfaction for coaching interventions (40, 62). Additional studies reporting satisfaction through mean scores reported that 85% of participants rated a full score for satisfaction (5/5) (53) and a mean reporting score of 5.19/7 (27). AI coaching interventions showed mixed satisfaction outcomes, generally ranging from moderate to high satisfaction rates (17, 38, 51, 69). Three studies reported on the likelihood of participants recommending the intervention. One study found a significant change in the Net Promoter Score (60), while two studies reported that 43.4% and 54% of participants would recommend the AI intervention. Additionally, 57% of participants indicated they would use it again (51, 66). Hybrid studies reported varied satisfaction rates varying from 67% to 81.5% (44, 59), with one study reporting usability below the average threshold, indicating low satisfaction (63). Interestingly, one hybrid study found that satisfaction rates were higher among participants who completed the coaching component of the intervention (88% compared to 64%) (44).
3.6 Working alliance
Seven studies (20.6%) examined working alliance and connection between participants and coaches. While one study used a working alliance scale, most explored working alliance through qualitative feedback from participants. Human coaching showed a high working alliance. Participants experienced authentic and strong connections, social support, and accountability (64). They also described a sense of investment and warmth from their coach (61), even in text-based coaching (64). This allowed participants to feel comfortable, motivated, and honest about their progress (61). Participants perceived AI coaches as engaging and lifelike, often viewing their interactions as relational (55, 60, 69). Participants viewed chatbots as a positive addition, appreciating its non-judgemental nature, finding it easier to share information (17) and feeling validated in their experiences (69). On a 7-point working alliance scale, the overall alliance with AI and human coaches was rated 4.23, with the bond component scoring 4.20 (67). Another study using the Session Alliance Inventory (ISA) found a minor but insignificant increase in scores (60). Despite positive connections made, some participants found chatbots patronising and preferred to connect to a real person. Others reported feelings of loneliness, disconnection and a lack of warmth while engaging with chatbots (17, 69). One study noted that chatbot interactions felt repetitive, contributing to feelings of disconnection (55). In one hybrid coaching intervention, participants valued human support alongside the AI coaching and expressed a desire for continued human support alongside the AI interventions, particularly for motivation, confidence, and technical support (63).
4 Discussion
This review explored how digital health coaching is integrated into DHIs and its impact on lifestyle outcomes and engagement. We identified three primary coaching models: human, AI, and hybrid (a combination of both human and AI coaches). Our findings suggest that both human- and AI-delivered coaching are generally perceived as acceptable and satisfactory components of DHIs, with trends indicating positive effects on health and wellbeing. Engagement and retention were generally high across all coaching models, with higher engagement linked to improvements in lifestyle outcomes. However, engagement and satisfaction were typically higher with human-delivered coaching. While working alliance was strong across all coaching models, participants reported a stronger sense of connection with human-delivered coaching, including within hybrid interventions.
Despite advancements in digital health coaching, studies were predominantly exploratory, early-intervention studies, focusing on feasibility and acceptability of coach-facilitated DHIs. While individual studies reported significant findings, the lack of consistency in study designs and outcome measures prevented clear inferences about broader trends in lifestyle and engagement. Likewise, there was an imbalanced representation of human (n = 18), AI (n = 13), and hybrid (n = 4) coaching interventions. This disparity, along with inconsistencies in outcome measures, intervention designs and coaching characteristics, limited direct comparisons and the generalisability of our findings; therefore, caution is warranted when interpreting the results in this review.
4.1 The role of coaching: delivery, standards, and trends
This review examined the integration of health coaching with DHIs, focusing on delivery methods, coaching roles, and standards for protocols and coach engagement (Table 5). Findings revealed a lack of consistency in reporting delivery methods, which contributed to ambiguity in the coaching protocols used. Only six studies provided comprehensive descriptions of all the extracted coaching characteristics that represent delivery (17, 42, 52, 53, 58, 69). The lack of transparency in coaching delivery methods made it difficult to determine the optimal frequency and intensity of coach-participant engagement for best outcomes. The total number of coaching sessions varied widely, ranging from 2 to 24, with a mean of 8.85 sessions per intervention (Table 5). Additionally, coaching sessions were most commonly conducted weekly (17, 50, 53, 61) and biweekly (44, 52), but no clear pattern emerged linking coaching frequency to delivery mode. Among long-term studies (ranging from 3 to 12 months), coaching was typically staggered, starting with weekly coaching sessions before transitioning to bi-weekly and monthly as the intervention progressed (42, 54, 57). Very few studies reported the qualifications of the coaches, making it difficult to determine who was delivering the coaching. Nonetheless, some trends emerged from the studies that did provide details on coaching delivery methods. Most studies indicated that both AI and human coaches played a central role in DHIs, though eight studies employed coaches in a supportive capacity (27, 37, 39, 40, 44, 46, 54, 58). In these cases, the role of the coach typically focused on supporting participants' technology use and enhancing usability through education around app features (27, 37, 46), reviewing and monitoring participant data (46), providing feedback to participants (39, 40, 44), adding accountability and promoting the implementation of skills gathered from the main intervention (i.e., educational modules and training) (27, 44, 54, 58).
In several studies, the role of the coach extended beyond the traditional role of facilitating health behaviour change and providing lifestyle support (27, 37, 46). Coaches were often tasked with providing technological and lifestyle support. The burden of supporting participants in navigating DHI technology has been recognised (27, 37). However, only one study provided a separate technology support channel for participants (46). In this instance, technology support was carried out by coaches via telephone calls but conducted outside of the coaching sessions. Moreover, communication predominantly occurred synchronously, with human coaches or conversational agents speaking in real time with participants. However, some studies supplemented this mode of interaction with asynchronous communication between coaching sessions (i.e., check-ins and ad hoc questions and feedback) (27, 59, 63).
4.1.2 Coaching standards
Professional and accredited coaches should play a central role in the development of digital health coaching interventions, whether by directly providing coaching or by informing the creation of coaching chatbots. This ensures that the high standards of practice paved by professional bodies like the International Coaching Federation (ICF), the European Mentoring and Coaching Council (EMCC) and the Association for Coaching (AC) are upheld in DHI research. In this review, we identified two key components for maintaining standards: (1) the qualifications and training of the coaches involved and (2) the evidence-based theories and models that inform coaching practices in DHIs. Only ten studies reported the qualifications or training of the human coaches involved in the interventions (39–42, 45, 53, 54, 58, 62, 64). These coaches were typically dietitians (39, 41), nutritional coaches (54), psychologists (40, 64), nurse practitioners (45, 58), and trained health coaches (53). Other studies mentioned that coaches received training from qualified professionals to deliver the intervention (42, 62). None of the hybrid coaching studies reported the qualifications or accreditation of the coaches working alongside AI.
Likewise, coaching theories played a crucial role in underpinning DHIs across all coaching models. Cognitive behavioural therapy, behaviour change theories, and positive psychology were the most commonly used (Table 5). All AI coaching interventions presented theories that underpinned the development of their conversational agents. Although six studies using human coaches failed to report theories used, two of which were hybrid coaching interventions (43, 47, 53, 54, 59, 63). This suggests that coaching theories were not only vital for active coaching but also influenced the design of DHIs and AI chatbots. The use of validated, evidence-based theories in the development of chatbots demonstrates that studies are moving towards validated, evidence-based approaches for chatbot creation. However, it also underscores the need for standardised quality and ethical guidelines for AI coaching.
The Designing AI Coach (DAIC) framework developed by Terblanche (70) provides a structured approach for assessing AI standards in coaching. The framework emphasises adapting human-efficacy elements and theoretical models for specific and narrow coaching tasks (i.e., goal setting), in line with ethical codes of conduct from accrediting coaching bodies (i.e., ICF, EMCC, AC). In this review, we found that all AI coaching studies, either intentionally or unintentionally, followed the first two principles of the DAIC framework: adapting human-efficacy and incorporating theoretical models in chatbot development (Table 5). However, the third principle, ethical conduct (covering privacy, autonomy, liability, and bias), was only addressed in three studies (49, 55, 65). The fourth principle, using AI chatbots for narrow, specialised tasks, was met in all but two studies. Notably, Maher et al. (56) used AI for both nutritional and physical activity interventions. Likewise, Dhinagaran et al. (51) and Ollier et al. (60) extended their chatbot's role to cover a wide range of lifestyle areas. Dhinagaran et al. (51) included diet, exercise, sleep, and stress support, while Ollier et al. (60) focused on psychological well-being, healthy eating, physical activity, and sleep. The DAIC framework is a valuable framework for guiding the development of AI coaching chatbots. Further research and standardisation are essential to ensure that AI coaching aligns with ethical and professional standards. This is critical for ensuring high-quality and safe AI interventions in digital health.
4.2 Tends in engagement, satisfaction, and working alliance
Engagement and retention rates have traditionally been considered low for DHIs (71), suggesting that this is a common issue in digital health research. However, a recent review by Boucher and Raiker (72) argued that engagement rates are not inherently low but rather vary widely across studies. They also highlighted a high degree of variability in how engagement is defined and measured (72). Our findings align with those of Boucher and Raiker (72), revealing that engagement was inconsistently reported, causing limitations for comparisons across studies. Retention and completion rates were the most consistently reported metrics. These metrics inferred higher retention and completion rates for human coaching interventions compared to AI and hybrid interventions. While AI retention rates were similar to those for human coaching, hybrid interventions showed a comparable difference. Engagement metrics offered valuable insight into adherence and retention but did not clarify the nature of meaningful interactions that may influence adherence. Boucher & Raiker (72) recommended moving away from the one-size-fits-all approach to assessing engagement and instead encourage researchers to focus on patterns of engagement.
Engagement as behaviour (i.e., quantitative engagement measures like app logins, text messages sent, and coaching sessions attended) provides valuable insight into the dynamics of DHIs and engagement trends (73). However, this construct was also inconsistently reported across studies, making it challenging to draw definitive conclusions (Table 6). Engagement as behaviour suggested that engagement with both human and AI coaches yielded positive outcomes. Among studies that reported engagement as behaviour, trends indicated early engagement and increased engagement with both human and AI coaches led to better outcomes, increased retention, adherence and goal attainment (37, 38, 40, 44, 49, 56, 58, 61, 63, 65, 66). Satisfaction rates offered further insight into participants' experiences with different coaching modalities. Overall, satisfaction was highest in interventions using human-facilitated coaching interventions (27, 40, 53, 62). AI coaching showed more variable satisfaction rates (17, 38, 51, 66, 69). While hybrid approaches demonstrated higher rates of satisfaction over AI-only, they still lagged behind human-only interventions (44, 59, 63).
Participants' experiences were further uncovered through the exploration of working alliances developed between coaches and participants. Prior research has consistently linked strong working alliances with positive coaching outcomes (74). A positive working alliance was formed between participants and AI and human coaches during DHI studies (17, 55, 60, 61, 63, 64, 67, 69). However, AI chatbots reported limitations to the alliances formed, with some participants reporting a sense of disconnection, repetitiveness and lack of warmth with chatbots (17, 55, 69). This trend in working alliance, favouring human-facilitated coaching interventions, was reflected by one hybrid coaching intervention (63). This study reported that participants valued human support along with AI to increase motivation and confidence and support technology navigation (63). Overall, while engagement, satisfaction, and working alliance remained consistent across both human and AI coaching interventions, human-facilitated coaching interventions foster stronger connections and higher satisfaction. Although hybrid and AI models could promote engagement and offer positive participant experiences, they could not replicate the interpersonal elements of human-delivered interventions that enhanced the overall individual experience. While engagement remains a key indicator of adherence, Boucher and Raiker (72) suggested that the true measure of success in DHIs should focus on the ability to foster meaningful lifestyle changes rather than being based on the frequency or duration of engagement.
4.3 Effectiveness of coaching for lifestyle change
The review findings suggest that human and AI-facilitated coaching could produce positive effects on lifestyle to varying degrees. While AI-powered coaching demonstrated more consistent positive trends on physical activity levels (48, 51, 56, 66), human-facilitated coaching yielded more consistent positive outcomes for psychological wellbeing and stress management (27, 40, 45, 47, 62, 68). Likewise, both human and AI coaching reported overall improvements in healthy eating (39, 41, 42, 48, 50, 51, 56, 58, 61, 62). Human coaching reported improvements in sleep, whereas AI did not (27, 51, 53, 59). Finally, substance use was only assessed with human coaches, precluding direct comparisons (57, 58). However, the varying effects of lifestyle outcomes between human and AI delivery systems were minimal, indicating that both AI and human coaching are acceptable and feasible interventions for supporting lifestyle changes. This finding aligns with existing literature suggesting that AI can be equally effective in providing support, particularly when deployed for narrow, specific tasks (32). Such findings suggest that AI could be a promising avenue for delivering scalable and effective lifestyle interventions (75, 76). However, studies using hybrid coaching approaches failed to produce consistent, robust findings compared to AI- and human-only interventions. Although some hybrid studies showed promising effects in physical activity, psychological wellbeing, and sleep, one study indicated that these benefits did not persist after human support ended (63). This suggests that further refinement of the integration between human and AI elements is needed to optimise long-term behaviour change.
4.4 Exploring the hybrid AI-human coaching model: benefits, limitations, and future potential
The lack of significant engagement and lifestyle outcomes in hybrid studies was unexpected. AI pioneers have established the value and complementary nature of combining AI and humans (77), with a growing body of literature on AI-human symbiosis indicating the shifting division of work between humans and machines (77–79). This comes from longstanding research asserting that computers plus humans do better than either alone (77, 79, 80). AI and humans hold different values and capabilities. While AI has superior computational and analytical skills, humans can better deal with uncertainty and have greater aptitude for intuition, creativity, and holistic work (77, 79). By harnessing human-AI symbioses, it becomes possible to pull the best traits of AI and humans and compensate for the limitations of both (77, 80).
In digital health coaching, hybrid models have been proposed as a promising way to combine the scalability of AI with the relational depth of human coaching (31, 81). This hybrid approach draws on the strengths of AI and human coaches to create high-quality, meaningful, accessible, and scalable coaching conversations (31, 81). Despite this theoretical promise, hybrid coaching studies included in this review did not reflect this. Several factors may explain this, including methodical constraints, the limited role of human coaches, and inconsistencies in AI implementation. Firstly, only four studies in this review examined hybrid coaching, making it difficult to assess its full impact. Secondly, human coaching was commonly offered as a supportive or optional component of hybrid DHIs (37, 82). Three studies highlighted this as a limitation, acknowledging greater outcomes when coaching was the central component of DHIs (37). Participants also expressed a preference for periodic human interaction alongside AI-driven support, reinforcing the importance of sustained human involvement (44, 63). Furthermore, these studies indicated that consistent human support was important for maintaining improvements in lifestyle and sustaining engagement (37, 44, 63). This aligns with existing literature, which suggests that AI should extend rather than replace human coaching capabilities (83).
The AI components used in hybrid coaching varied across studies. Two studies employed basic automation for delivering tailored messages and advice (37, 44), while another used a decision-support system to provide personalised recommendations (59). The fourth study integrated a digital coach similar to a chatbot but with limited interactive capabilities (63). The study that most closely aligned with AI coaching models showed significant improvements in physical activity; however, these benefits diminished once human support was removed (Table 3). This reflects broader findings that AI is effective for structured tasks like goal-setting but lacks the relational depth and working alliance needed for long-term behavioural change (83). This suggests that hybrid coaching is still an emerging area with limited empirical evaluation. While these studies confirmed the feasibility, more research is needed to refine hybrid coaching models and optimise their effectiveness. Additionally, trends suggest that hybrid models should prioritise human connection as the foundation of coaching while leveraging AI to enhance efficiency and scalability. Future hybrid interventions should, therefore, prioritise AI as an enhancement to human coaching rather than a substitute.
4.5 Strengths and limitations
This systematic review provided a comprehensive exploration of coach-facilitated digital DHIs, a novel and growing field. By synthesising existing evidence, this review highlighted the feasibility, acceptability, and impact of human, AI and hybrid coaching modalities on engagement and lifestyle outcomes. Adherence to PRISMA guidelines and the use of the MMAT ensured a rigorous and transparent methodological approach. There are well-established, foundational reviews that have significantly advanced digital health research in the context of health promotion and disease prevention (6, 84, 85). This review builds on this existing foundation to synthesise the existing body of knowledge on the integration of different health coaching modalities within DHIs. The insights gained from this review can inform the development and optimisation of future coach-led DHIs.
However, several limitations of this review should be noted. First, while full-text screening, data-extraction and quality appraisal was done in duplicate, the title and abstract screening was conducted by one reviewer only, increasing the risk of reviewer bias. Additionally, due to the use of broad search terms with multiple definitions, it is difficult to confirm that all relevant articles have been included. Furthermore, Significant heterogeneity in reporting coaching protocol, engagement metrics, and lifestyle outcomes across studies affects the ability to compare findings directly. This heterogeneity hindered the synthesis of results, making it difficult to draw consistent conclusions. Many of the included studies primarily focused on assessing the acceptability and feasibility of coach-facilitated DHIs rather than investigating the impact of specific components of the DHIs (e.g., active coaching, educational modules, tracking tools) and their interactions in influencing lifestyle outcomes. As a result, this review cannot definitively establish the full impact of coaching within DHIs, and its findings should be interpreted with caution. The review's generalisability is also constrained by the heterogeneity of participant demographics. Finally, the inclusion of only peer-reviewed studies may have introduced publication bias, potentially skewing the findings. Despite these limitations, this review offers valuable insights into the current state and potential future directions of digital health coaching in DHIs, providing a foundation for advancing scalable, high-quality, and evidence-based interventions.
5 Conclusion
The studies included in this review contribute to our understanding of how digital health coaching can be effectively integrated into DHIs, highlighting the trends, opportunities, and challenges related to its impact on lifestyle outcomes and engagement. Despite inconsistencies in reporting coaching delivery methods, engagement metrics, satisfaction, and lifestyle outcomes, this review emphasises the potential positive impact of coach-facilitated DHIs on participant engagement and lifestyle outcomes. We confirm the acceptability and feasibility of integrating AI and human coaching into DHIs and identify a gap in the research on hybrid coaching approaches. Digital health coaching in DHIs is complex and multifaceted, making it difficult to isolate components of the intervention to understand their effect (i.e., coaching, wearables, environment). Further research is necessary to better understand these complexities to advance the development of quality, evidence-based, coach-led DHIs that promote participant engagement and positive lifestyle changes. Additionally, it is crucial for future research to explore how the benefits of both AI and human coaching, as reported in this review, can be leveraged through hybrid approaches. Such strategies could help overcome the barriers of meaningful engagement in AI coaching and the scalability and accessibility of human coaching to create a scalable coach-led DHI without decreasing the quality of health coaching.
Data availability statement
The original contributions presented in the study are included in the article/Supplementary Material, further inquiries can be directed to the corresponding author.
Author contributions
CL: Conceptualization, Data curation, Formal analysis, Funding acquisition, Investigation, Methodology, Project administration, Resources, Software, Validation, Visualization, Writing – original draft, Writing – review & editing. JL: Formal analysis, Data curation, Validation, Investigation, Writing – review & Editing. RO: Data curation, Formal analysis, Investigation, Methodology, Software, Supervision, Validation, Writing – review & editing. PD: Funding acquisition, Investigation, Project administration, Supervision, Validation, Visualization, Writing – review & editing.
Funding
The author(s) declare that financial support was received for the research and/or publication of this article. This work was supported by the Irish Research Council (grant number: EBPPG/2022/122).
Conflict of interest
The authors declare that the research was conducted in the absence of any commercial or financial relationships that could be construed as a potential conflict of interest.
The author(s) declared that they were an editorial board member of Frontiers, at the time of submission. This had no impact on the peer review process and the final decision.
Generative AI statement
The author(s) declare that no Generative AI was used in the creation of this manuscript.
Publisher's note
All claims expressed in this article are solely those of the authors and do not necessarily represent those of their affiliated organizations, or those of the publisher, the editors and the reviewers. Any product that may be evaluated in this article, or claim that may be made by its manufacturer, is not guaranteed or endorsed by the publisher.
Supplementary material
The Supplementary Material for this article can be found online at: https://www.frontiersin.org/articles/10.3389/fdgth.2025.1536416/full#supplementary-material
References
1. Nations U. Global Sustainable Development Report 2015. New York: United Nations Department of Economic and Social Affairs (2015).
2. Organization WH. Global Strategy on Digital Health 2020–2025. Geneva: World Health Organisation (WHO) (2021).
3. Zanaboni P, Ngangue P, Mbemba GIC, Schopf TR, Bergmo TS, Gagnon M-P. Methods to evaluate the effects of internet-based digital health interventions for citizens: systematic review of reviews. J Med Internet Res. (2018) 20(6):e10202. doi: 10.2196/10202
4. Murray E, Hekler EB, Andersson G, Collins LM, Doherty A, Hollis C, et al. Evaluating digital health interventions: key questions and approaches. Am J Prev Med. (2016) 51(5):843–51. doi: 10.1016/j.amepre.2016.06.008
5. Mair JL, Salamanca-Sanabria A, Augsburger M, Frese BF, Abend S, Jakob R, et al. Effective behavior change techniques in digital health interventions for the prevention or management of noncommunicable diseases: an umbrella review. Ann Behav Med. (2023) 57(10):817–35. doi: 10.1093/abm/kaad041
6. Castro R, Ribeiro-Alves M, Oliveira C, Romero CP, Perazzo H, Simjanoski M, et al. What are we measuring when we evaluate digital interventions for improving lifestyle? A scoping meta-review. Front Public Health. (2022) 9:735624. doi: 10.3389/fpubh.2021.735624
7. Groot J, MacLellan A, Butler M, Todor E, Zulfiqar M, Thackrah T, et al. The effectiveness of fully automated digital interventions in promoting mental well-being in the general population: systematic review and meta-analysis. JMIR Ment Health. (2023) 10(1):e44658. doi: 10.2196/44658
8. Indra B, Palmasutra V, Setyawan FA. Effectiveness of digital interventions in reducing occupational stress: a systematic review. Port J Public Health. (2024) 42(3):252–65. doi: 10.1159/000540748
9. Zheng S, Edney SM, Goh CH, Tai BC, Mair JL, Castro O, et al. Effectiveness of holistic mobile health interventions on diet, and physical, and mental health outcomes: a systematic review and meta-analysis. EClinicalMedicine. (2023) 66:102309. doi: 10.1016/j.eclinm.2023.102309
10. Duan Y, Shang B, Liang W, Du G, Yang M, Rhodes RE. Effects of eHealth-based multiple health behavior change interventions on physical activity, healthy diet, and weight in people with noncommunicable diseases: systematic review and meta-analysis. J Med Internet Res. (2021) 23(2):e23786. doi: 10.2196/23786
11. Widmer RJ, Collins NM, Collins CS, West CP, Lerman LO, Lerman A, editors. Digital health interventions for the prevention of cardiovascular disease: a systematic review and meta-analysis. Mayo Clinic Proceedings; Elsevier; (2015). doi: 10.1016/j.mayocp.2014.12.026
12. Howarth A, Quesada J, Silva J, Judycki S, Mills PR. The impact of digital health interventions on health-related outcomes in the workplace: a systematic review. Digit Health. (2018) 4:1–18. doi: 10.1177/2055207618770861
13. Balwan WK, Kour S. Lifestyle diseases: the link between modern lifestyle and threat to public health. Saudi J Med Pharm Sci. (2021) 7(4):179–84. doi: 10.36348/sjmps.2021.v07i04.003
14. Willems SH, Rao J, Bhambere S, Patel D, Biggins Y, Guite JW. Digital solutions to alleviate the burden on health systems during a public health care crisis: COVID-19 as an opportunity. JMIR Mhealth Uhealth. (2021) 9(6):e25021. doi: 10.2196/25021
15. O’connor S, Hanlon P, O’donnell CA, Garcia S, Glanville J, Mair FS. Understanding factors affecting patient and public engagement and recruitment to digital health interventions: a systematic review of qualitative studies. BMC Med Inform Decis Mak. (2016) 16:1–15. doi: 10.1186/s12911-016-0359-3
16. Fadhil A, Wang Y, Reiterer H. Assistive conversational agent for health coaching: a validation study. Methods Inf Med. (2019) 58(1):9–23. doi: 10.1055/s-0039-1688757
17. Aymerich-Franch L, Ferrer I. Investigating the use of speech-based conversational agents for life coaching. Int J Hum Comput Stud. (2022) 159:102745. doi: 10.1016/j.ijhcs.2021.102745
18. Meyerowitz-Katz G, Ravi S, Arnolda L, Feng X, Maberly G, Astell-Burt T. Rates of attrition and dropout in app-based interventions for chronic disease: systematic review and meta-analysis. J Med Internet Res. (2020) 22(9):e20283. doi: 10.2196/20283
19. Kernebeck S, Busse TS, Ehlers JP, Vollmar HC. Adherence to digital health interventions: definitions, methods, and open questions. Bundesgesundheitsblatt-Gesundheitsforschung-Gesundheitsschutz. (2021) 64(10):1278–84. doi: 10.1007/s00103-021-03415-9
20. Torous J, Michalak EE, O’Brien HL. Digital health and engagement—looking behind the measures and methods. JAMA Netw Open. (2020) 3(7):e2010918. doi: 10.1001/jamanetworkopen.2020.10918
21. Silvestri F, Vital P, Saboga-Nunes L, Bittlingmayer U. Health coaching: the node of health literacy & health promotion. Eur J Public Health. (2024) 34(Supplement_3):ckae144.833. doi: 10.1093/eurpub/ckae144.833
22. Miller BR, Klein AA, Leheste JR. Health coaching: America’s overlooked personalized health solution. Med Res Arch. (2024) 12(8):1–10. doi: 10.18103/mra.v12i8.5662
23. Olsen JM, editor. Health Coaching: A Concept Analysis. Nursing Forum. Wiley Online Library (2014). doi: 10.1111/nuf.12042
24. Thom DH. Keeping pace with the expanding role of health coaching. J Gen Intern Med. (2019) 34:5–6. doi: 10.1007/s11606-018-4730-1
25. McGlynn A, O’Callaghan C, McDougall B, Osborne J, Harris-Roxas B. Translating health coaching training into clinical practice. Int J Environ Res Public Health. (2022) 19(23):16075. doi: 10.3390/ijerph192316075
26. Castro Sweet CM, Chiguluri V, Gumpina R, Abbott P, Madero EN, Payne M, et al. Outcomes of a digital health program with human coaching for diabetes risk reduction in a medicare population. J Aging Health. (2018) 30(5):692–710. doi: 10.1177/0898264316688791
27. Chow PI, Drago F, Kennedy EM, Cohn WF. A novel mobile phone app intervention with phone coaching to reduce symptoms of depression in survivors of women’s cancer: pre-post pilot study. JMIR Cancer. (2020) 6(1):e15750. doi: 10.2196/15750
28. Serio C, Gabarda A, Uyar-Morency F, Silfee V, Ludwig J, Szigethy E, et al. Strengthening the impact of digital cognitive behavioral interventions through a dual intervention: proficient motivational interviewing–based health coaching plus in-application techniques. JMIR Form Res. (2022) 6(5):e34552. doi: 10.2196/34552
29. Passmore J, Liu Q, Tewald S. Future trends in coaching: results from a global coach survey, 2021. Coach Psychol. (2021) 17(2):41–51. doi: 10.2196/34552
30. Geißler H. E-coaching: an overview. In: Greif S, Möller H, Scholl W, Passmore J, Müller F, editors. International Handbook of Evidence-Based Coaching: Theory, Research and Practice. New York: Springer International Publishing (2022):269–80. doi: 10.1007/978-3-030-81938-5
31. Kanatouri S. Digital coaching: a conceptually distinct form of coaching? In: Wegener R, Ackermann S, Amstutz J, Deplazes S, Künzli H, Ryter A, editors. Coaching im Digitalen Wandel. Göttingen, Germany: Vandenhoeck & Ruprecht. (2020). p. 40–50. doi: 10.13109/9783666407420.40
32. Terblanche NH. Artificial intelligence (AI) coaching: redefining people development and organizational performance. J Appl Behav Sci. (2024) 60(4):631–8. doi: 10.1177/00218863241283919
33. Diller SJ, Stenzel L-C, Passmore J. The coach bots are coming: exploring global coaches’ attitudes and responses to the threat of AI coaching. Hum Resour Dev Int. (2024) 27(4):597–621. doi: 10.1080/13678868.2024.2375934
34. Hussain S, Ameri Sianaki O, Ababneh N, editors. A survey on conversational agents/chatbots classification and design techniques. Web, Artificial Intelligence and Network Applications: Proceedings of the Workshops of the 33rd International Conference on Advanced Information Networking and Applications (WAINA-2019). Springer (2019). 33. doi: 10.1007/978-3-030-15035-8_93
35. Page MJ, McKenzie JE, Bossuyt PM, Boutron I, Hoffmann TC, Mulrow CD, et al. The PRISMA 2020 statement: an updated guideline for reporting systematic reviews. BMJ. (2021) 372:1–9. doi: 10.1136/bmj.n71
36. Popay J. Guidance on the Conduct of Narrative Synthesis in Systematic Review: A Product from the ESRC Methods Programme. Lancaster, England: Lancaster University, Institute for Health Research (2006). doi: 10.13140/2.1.1018.4643
37. Damschroder LJ, Buis LR, McCant FA, Kim HM, Evans R, Oddone EZ, et al. Effect of adding telephone-based brief coaching to an mHealth APP (stay strong) for promoting physical activity among veterans: randomized controlled trial. J Med Internet Res. (2020) 22(8):e19216. doi: 10.2196/19216
38. Foran HM, Kubb C, Mueller J, Poff S, Ung M, Li M, et al. An automated conversational agent self-help program: randomized controlled trial. J Med Internet Res. (2024) 26:e53829. doi: 10.2196/53829
39. Han CY, Lim SL, Ong KW, Johal J, Gulyani A. Behavioral lifestyle intervention program using mobile application improves diet quality in adults with prediabetes (D’LITE study): a randomized controlled trial. J Acad Nutr Diet. (2024) 124(3):358–71. doi: 10.1016/j.jand.2023.10.005
40. Heber E, Lehr D, Ebert DD, Berking M, Riper H. Web-based and mobile stress management intervention for employees: a randomized controlled trial. J Med Internet Res. (2016) 18(1):e5112. doi: 10.2196/jmir.5112
41. Partridge SR, McGeechan K, Hebden L, Balestracci K, Wong AT, Denney-Wilson E, et al. Effectiveness of a mHealth lifestyle program with telephone support (TXT2BFiT) to prevent unhealthy weight gain in young adults: randomized controlled trial. JMIR Mhealth Uhealth. (2015) 3(2):e4530. doi: 10.2196/mhealth.4530
42. Spring B, Pellegrini C, McFadden HG, Pfammatter AF, Stump TK, Siddique J, et al. Multicomponent mHealth intervention for large, sustained change in multiple diet and activity risk behaviors: the make better choices 2 randomized controlled trial. J Med Internet Res. (2018) 20(6):e10528. doi: 10.2196/10528
43. Wijsman CA, Westendorp RG, Verhagen EA, Catt M, Slagboom PE, de Craen AJ, et al. Effects of a web-based intervention on physical activity and metabolism in older adults: randomized controlled trial. J Med Internet Res. (2013) 15(11):e2843. doi: 10.2196/jmir.2843
44. Alley S, Jennings C, Plotnikoff RC, Vandelanotte C. Web-based video-coaching to assist an automated computer-tailored physical activity intervention for inactive adults: a randomized controlled trial. J Med Internet Res. (2016) 18(8):e5664. doi: 10.2196/jmir.5664
45. Bakas T, Sampsel D, Israel J, Chamnikar A, Bodnarik B, Clark JG, et al. Using telehealth to optimize healthy independent living for older adults: a feasibility study. Geriatr Nurs (Minneap). (2018) 39(5):566–73. doi: 10.1016/j.gerinurse.2018.04.002
46. Blair CK, Harding E, Wiggins C, Kang H, Schwartz M, Tarnower A, et al. A home-based mobile health intervention to replace sedentary time with light physical activity in older cancer survivors: randomized controlled pilot trial. JMIR Cancer. (2021) 7(2):e18819. doi: 10.2196/18819
47. Chang M-W, Tan A, Wegener DT, Lee RE. A pilot goal-oriented episodic future thinking weight loss intervention for low-income overweight or obese young mothers. Nutrients. (2023) 15(13):3023. doi: 10.3390/nu15133023
48. Chew HSJ, Chew NW, Loong SSE, Lim SL, Tam WSW, Chin YH, et al. Effectiveness of an artificial intelligence-assisted app for improving eating behaviors: mixed methods evaluation. J Med Internet Res. (2024) 26:e46036. doi: 10.2196/46036
49. Daley K, Hungerbuehler I, Cavanagh K, Claro HG, Swinton PA, Kapps M. Preliminary evaluation of the engagement and effectiveness of a mental health chatbot. Front Digit Health. (2020) 2:576361. doi: 10.3389/fdgth.2020.576361
50. D’Avolio D, Gropper SS, Appelbaum M, Thiengtham S, Holt J, Newman D. The impact of a pilot telehealth coaching intervention to improve caregiver stress and well-being and to increase dietary protein intake of caregivers and their family members with dementia–interrupted by COVID-19. Dementia. (2023) 22(6):1241–58. doi: 10.1177/14713012231177491
51. Dhinagaran DA, Sathish T, Soong A, Theng Y-L, Best J, Car LT. Conversational agent for healthy lifestyle behavior change: web-based feasibility study. JMIR Form Res. (2021) 5(12):e27956. doi: 10.2196/27956
52. Gabrielli S, Rizzi S, Bassi G, Carbone S, Maimone R, Marchesoni M, et al. Engagement and effectiveness of a healthy-coping intervention via chatbot for university students during the COVID-19 pandemic: mixed methods proof-of-concept study. JMIR Mhealth Uhealth. (2021) 9(5):e27965. doi: 10.2196/27965
53. Gudenkauf LM, Li X, Hoogland AI, Oswald LB, Lmanirad I, Permuth JB, et al. Feasibility and acceptability of C-PRIME: a health promotion intervention for family caregivers of patients with colorectal cancer. Support Care Cancer. (2024) 32(3):198. doi: 10.1007/s00520-024-08395-5
54. Horn CE, Seely EW, Levkoff SE, Isley BC, Nicklas JM. Postpartum women’s experiences in a randomized controlled trial of a web-based lifestyle intervention following gestational diabetes: a qualitative study. J Matern Fetal Neonatal Med. (2023) 36(1):2194012. doi: 10.1080/14767058.2023.2194012
55. Ly K, Ly A, Andersson G. A fully automated conversational agent for promoting mental well-being: a pilot RCT using mixed methods. Internet Interv. (2017) 10:39–46. doi: 10.1016/j.invent.2017.10.002
56. Maher CA, Davis CR, Curtis RG, Short CE, Murphy KJ. A physical activity and diet program delivered by artificially intelligent virtual health coach: proof-of-concept study. JMIR Mhealth Uhealth. (2020) 8(7):e17558. doi: 10.2196/17558
57. Marler JD, Fujii CA, Utley DS, Tesfamariam LJ, Galanko JA, Patrick H. Initial assessment of a comprehensive digital smoking cessation program that incorporates a mobile app, breath sensor, and coaching: cohort study. JMIR Mhealth Uhealth. (2019) 7(2):e12609. doi: 10.2196/12609
58. McGuire AM, Porter-Steele J, McDonald N, Burgess SC, Anderson DJ, Seib C. Reducing noncommunicable disease risk in midlife adults using eHealth: the GroWell for health program feasibility study. Collegian. (2022) 29(3):328–36. doi: 10.1016/j.colegn.2021.09.003
59. Moreno-Blanco D, Solana-Sánchez J, Sánchez-González P, Jiménez-Hernando M, Cattaneo G, Roca A, et al. Intelligent coaching assistant for the promotion of healthy habits in a multidomain mHealth-based intervention for brain health. Int J Environ Res Public Health. (2021) 18(20):10774. doi: 10.3390/ijerph182010774
60. Ollier J, Suryapalli P, Fleisch E, Wangenheim FV, Mair JL, Salamanca-Sanabria A, et al. Can digital health researchers make a difference during the pandemic? Results of the single-arm, chatbot-Led Elena+: care for COVID-19 interventional study. Front Public Health. (2023) 11:1185702. doi: 10.3389/fpubh.2023.1185702
61. Price J, Brunet J. Understanding rural-living young adult cancer survivors’ motivation during a telehealth behavior change intervention within a single-arm feasibility trial. Health Informatics J. (2022) 28(1):1–20. doi: 10.1177/14604582221075560
62. Sacher PM, Fulton E, Rogers V, Wilson J, Gramatica M, Dent JE, et al. Impact of a health coach–led, text-based digital behavior change intervention on weight loss and psychological well-being in patients receiving a procedureless intragastric balloon program: prospective single-arm study. JMIR Form Res. (2024) 8:e54723. doi: 10.2196/54723
63. Santini S, Fabbietti P, Galassi F, Merizzi A, Kropf J, Hungerländer N, et al. The impact of digital coaching intervention for improving healthy ageing dimensions among older adults during their transition from work to retirement. Int J Environ Res Public Health. (2023) 20(5):4034. doi: 10.3390/ijerph20054034
64. Smart MH, Nabulsi NA, Gerber BS, Gupta I, Di Eugenio B, Ziebart B, et al. A remote health coaching, text-based walking program in ethnic minority primary care patients with overweight and obesity: feasibility and acceptability pilot study. JMIR Form Res. (2022) 6(1):e31989. doi: 10.2196/31989
65. Terblanche N, Molyn J, De Haan E, Nilsson VO. Coaching at scale: investigating the efficacy of artificial intelligence coaching. Int J Evid Based Coach Mentor. (2022) 20(2):20–26. doi: 10.24384/5cgf-ab69
66. To QG, Green C, Vandelanotte C. Feasibility, usability, and effectiveness of a machine learning–based physical activity chatbot: quasi-experimental study. JMIR Mhealth Uhealth. (2021) 9(11):e28577. doi: 10.2196/28577
67. Ulrich S, Lienhard N, Künzli H, Kowatsch T. A chatbot-delivered stress management coaching for students (MISHA app): pilot randomized controlled trial. JMIR Mhealth Uhealth. (2024) 12:e54945. doi: 10.2196/54945
68. Wiegand B, Luedtke K, Friscia D, Nair M, Aleles M, McCloskey R. Efficacy of a comprehensive program for reducing stress in women: a prospective, randomized trial. Curr Med Res Opin. (2010) 26(4):991–1002. doi: 10.1185/03007991003688193
69. Williams R, Hopkins S, Frampton C, Holt-Quick C, Merry SN, Stasiak K. 21-day stress detox: open trial of a universal well-being chatbot for young adults. Soc Sci. (2021) 10(11):416. doi: 10.3390/socsci10110416
70. Terblanche N. A design framework to create artificial intelligence coaches. Int J Evid Based Coach Mentor. (2020) 18(2):152165. doi: 10.24384/b7gs-3h05
71. Eysenbach G. The law of attrition. J Med Internet Res. (2005) 7(1):e402. doi: 10.2196/jmir.7.1.e11
72. Boucher EM, Raiker JS. Engagement and retention in digital mental health interventions: a narrative review. BMC Digit Health. (2024) 2(1):52. doi: 10.1186/s44247-024-00105-9
73. Ryan C, Bergin M, Wells JS. Theoretical perspectives of adherence to web-based interventions: a scoping review. Int J Behav Med. (2018) 25:17–29. doi: 10.1007/s12529-017-9678-8
74. Graßmann C, Schölmerich F, Schermuly CC. The relationship between working alliance and client outcomes in coaching: a meta-analysis. Hum Relat. (2020) 73(1):35–58. doi: 10.1177/0018726718819725
75. Fadhil A, Gabrielli S, editors. Addressing challenges in promoting healthy lifestyles. Proceedings of the 11th EAI International Conference on Pervasive Computing Technologies for Healthcare, PervasiveHealth; (2017). doi: 10.1145/3154862.3154914
76. Aggarwal A, Tam CC, Wu D, Li X, Qiao S. Artificial intelligence–based chatbots for promoting health behavioral changes: systematic review. J Med Internet Res. (2023) 25:e40789. doi: 10.2196/40789
77. Jarrahi MH. Artificial intelligence and the future of work: human-AI symbiosis in organizational decision making. Bus Horiz. (2018) 61(4):577–86. doi: 10.1016/j.bushor.2018.03.007
78. Nagao K, Nagao K. Symbiosis between humans and artificial intelligence. In: Nagao E, editor. Artificial Intelligence Accelerates Human Learning: Discussion Data Analytics. Singapore: Springer (2019). p. 135–51. doi: 10.1007/978-981-13-6175-3_6
79. Grigsby SS, editor. Artificial intelligence for advanced human-machine symbiosis. Augmented Cognition: Intelligent Technologies: 12th International Conference, AC 2018, Held as Part of HCI International 2018; July 15–20, 2018; Las Vegas, NV, USA (2018). Springer. Proceedings, Part I. doi: 10.1007/978-3-319-91470-1_22
80. Licklider JC. Man-computer symbiosis. IRE Trans Hum Factors Electron. (1960) 1:4–11. doi: 10.1109/THFE2.1960.4503259
81. Clutterbuck D. The challenges of coaching and mentoring in a digitally connected world. In: Wegener R, Ackermann S, Amstutz J, Deplazes S, Künzli H, Ryter A, editors. Coaching im Digitalen Wandel. Göttingen, Germany: Vandenhoeck & Ruprecht (2020):19–29. doi: 10.13109/9783666407420.19
82. Godino JG, Merchant G, Norman GJ, Donohue MC, Marshall SJ, Fowler JH, et al. Using social and mobile tools for weight loss in overweight and obese young adults (project SMART): a 2 year, parallel-group, randomised, controlled trial. Lancet Diabetes Endocrinol. (2016) 4(9):747–55. doi: 10.1016/S2213-8587(16)30105-X
83. Graßmann C, Schermuly CC. Coaching with artificial intelligence: concepts and capabilities. Hum Resour Dev Rev. (2021) 20(1):106–26. doi: 10.1177/1534484320982891
84. Chatterjee A, Prinz A, Gerdes M, Martinez S. Digital interventions on healthy lifestyle management: systematic review. J Med Internet Res. (2021) 23(11):e26931. doi: 10.2196/26931
Keywords: digital health interventions, positive health, health promotion, disease prevention, coaching, health coaching
Citation: Loughnane C, Laiti J, O’Donovan R and Dunne PJ (2025) Systematic review exploring human, AI, and hybrid health coaching in digital health interventions: trends, engagement, and lifestyle outcomes. Front. Digit. Health 7:1536416. doi: 10.3389/fdgth.2025.1536416
Received: 28 November 2024; Accepted: 24 March 2025;
Published: 24 April 2025.
Edited by:
Ben Singh, University of South Australia, AustraliaReviewed by:
Oscar Castro, ETH Zürich, SingaporeAnna Dysart, Virginia Tech, United States
Ana Catarina Martins, New University of Lisbon, Portugal
Copyright: © 2025 Loughnane, Laiti, O’Donovan and Dunne. This is an open-access article distributed under the terms of the Creative Commons Attribution License (CC BY). The use, distribution or reproduction in other forums is permitted, provided the original author(s) and the copyright owner(s) are credited and that the original publication in this journal is cited, in accordance with accepted academic practice. No use, distribution or reproduction is permitted which does not comply with these terms.
*Correspondence: Croía Loughnane, Y3JvaWFsb3VnaG5hbmUyMkByY3NpLmNvbQ==