- 1Applied Artificial Intelligence Institute (I2A), TELUQ University, Montreal, QC, Canada
- 2Open Innovation Laboratory in Health Technologies, CHUM Research Center, Montreal, QC, Canada
- 3Department of Computer Science and Mathematics, University of Quebec at Chicoutimi, Chicoutimi, QC, Canada
Background: Wearable devices offer innovative solutions for chronic pain (CP) management by enabling real-time monitoring and personalized pain control. Although they are increasingly used to monitor pain-related parameters, their potential for predicting CP progression remains underutilized. Current studies focus mainly on correlations between data and pain levels, but rarely use this information for accurate prediction.
Objective: This study aims to review recent advancements in wearable technology for CP management, emphasizing the integration of multimodal data, sensor quality, compliance with data security standards, and the effectiveness of predictive models in identifying CP episodes.
Methods: A systematic search across six major databases identified studies evaluating wearable devices designed to collect pain-related parameters and predict CP. Data extraction focused on device types, sensor quality, compliance with health standards, and the predictive algorithms employed.
Results: Wearable devices show promise in correlating physiological markers with CP, but few studies integrate predictive models. Random Forest and multilevel models have demonstrated consistent performance, while advanced models like Convolutional Neural Network-Long Short-Term Memory have faced challenges with data quality and computational demands. Despite compliance with regulations like General Data Protection Regulation and ISO standards, data security and privacy concerns persist. Additionally, the integration of multimodal data, including physiological, psychological, and demographic factors, remains underexplored, presenting an opportunity to improve prediction accuracy.
Conclusions: Future research should prioritize developing robust predictive models, standardizing data protocols, and addressing security and privacy concerns to maximize wearable devices’ potential in CP management. Enhancing real-time capabilities and fostering interdisciplinary collaborations will improve clinical applicability, enabling personalized and preventive pain management.
1 Introduction
Chronic Pain (CP) is a major public health issue affecting millions of people worldwide, causing significant physical, emotional, and financial challenges (1, 2). Approximately 10% of the global population experiences CP (3, 4), with some studies reporting prevalence rates between 20% and 77% in certain countries (5–9). CP is often associated with various health problems, including musculoskeletal disorders, neuropathies, and chronic diseases, which frequently reduce quality of life and impair functionality. According to the World Health Organization, the most common form of CP is low back pain, which has impacted 619 million people in 2020. This number will increase to 843 million by 2050, primarily due to population growth and aging (10). Traditional methods for reducing CP, such as medications, interventional procedures, physical and psychological therapies, and lifestyle approaches have shown limited long-term effectiveness (2, 11). These approaches lack real-time monitoring, may cause side effects, and are not always tailored to individual pain patterns. This reinforces the need for more effective and customized solutions.
Wearable technologies are emerging as innovative, non-intrusive solutions to pain management. They enable real-time data collection, continuous monitoring, enhanced patient engagement, and reduced dependence on pharmaceuticals (12, 13). Such technologies have been shown to improve communication between patients and healthcare providers (14). However, despite the increasing number of research on digital tools for managing CP, existing studies have primarily focused on detecting pain severity (15–17) and improving adherence to prescribed treatments (18–20). Previous article reviews provided an overview of digital technologies for pain management. Although they reported various physiological signals such as heart rate, muscle activity, and sleep patterns (16, 21, 22) for pain monitoring, and explored the usability and feasibility of health tools (23–25), they do not adequately address the predictive capabilities of these technologies. Many of them emphasize the correlation between pain and physiological data without addressing the quality of the data collected by the different types of sensors and the predictive potential of the wearable device, in order to anticipate CP episodes. Furthermore, most studies are limited to controlled settings, bypassing the importance of real-world data and longitudinal analysis for proactive CP management.
This review focuses on predictive models for CP using wearable devices by highlighting how these technologies can not only monitor CP but also anticipate its intensity through multimodal data. Indeed, pain is a complex phenomenon, encompassing sensory perception as well as behavioral, physiological and psychological responses (26). Therefore, its management requires a multidisciplinary approach, involving expertise in medicine, psychology, physiotherapy and social sciences for a comprehensive understanding and treatment. Another key aspect of this study's contribution is the priority given to data security, privacy protection, and compliance with health data standards in the use of these devices. While much research has explored the usability and technical aspects of wearable devices (21), there is an insufficient consideration given to the security and privacy of sensitive health information. With the rise of cyberattacks and the increasing need to safeguard personal data, data security and privacy in healthcare systems have become crucial (27, 28). This presents a major challenge, as both device and software security must be ensured. Addressing these factors is essential for developing effective tools for CP management. Sensor capabilities, data security, and standards compliance are closely interconnected in the context of predicting CP using wearable devices. Synergy between these aspects is essential to ensure the accuracy of the collected data, its protection against unauthorized access, and its use in compliance with regulatory requirements.
This scoping review presents a novel framework for managing CP by examining device technologies, addressing legal considerations such as data privacy, regulatory compliance, and ethical concerns, and highlighting predictive modeling based on multimodal data from wearable sensors. To the best of our knowledge, no review published to date has emphasized the importance of combining various types of data (physiological, behavioral, environmental factors, etc.) to improve the accuracy and reliability of CP prediction models while deepening the data security and privacy requirements. In doing so, we aim to provide more robust methods for secure, compliant, and comprehensive pain management tools, ultimately benefiting designers, users and healthcare providers.
2 Methods
To systematically analyze studies focusing on CP and wearable devices, this review was conducted in accordance with the Preferred Reporting Items for Systematic Reviews and Meta-Analysis-Scoping Review (PRISMA-ScR) guidelines (29). We followed the structure defined by Arksey and O'Malley (30), comprising five stages: (1) defining the research question; (2) identifying relevant studies; (3) establishing eligibility criteria for study selection; (4) charting the data; (5) summarizing and reporting the results. Adhering to these stages facilitated a comprehensive mapping of the literature and a synthesis of findings related to CP prediction.
2.1 Review questions
This review aims to address the following questions regarding technologies designed to collect data related to CP: (a) Which wearable devices, through their algorithms and monitoring capabilities, can help detect or prevent chronic pain? (b) How do these devices comply with regulatory standards to ensure data security and protect user privacy? (c) What key features contribute to their effectiveness for chronic pain prediction?
2.2 Identifying relevant studies
The search strategy was developed using keywords and terms existing on pain management technologies. It included three main steps: step 1) identifying terms related to chronic pain (e.g., persistent pain); step 2) defining terms related to wearable devices (e.g., wearable technology); and step 3) combining parameters from steps 1 and 2 to retrieve references covering both concepts. Studies were extracted across six databases (PubMed, Web of Science, Scopus, Engineering Village, IEEE Xplore, and Google Scholar) from the inception of the database to November 4, 2024, with no limit on publication year applied. The first 155 results from Google Scholar and all identified studies from the other databases were imported into Covidence systematic review software (31) for duplicate removal. Furthermore, an additional manual search of reference lists within relevant systematic reviews was conducted. The search strategy was tailored to each database, giving priority to articles in English or French. The detailed search strategy is presented in Supplementary Material.
2.3 Eligibility criteria
Eligible studies for addressing our third review question must include participants experiencing chronic pain, defined by the International Association for the Study of Pain (IASP), as “pain that persists or recurs for longer than three months” (32). Only studies utilizing wearable devices for CP prediction were included. Studies involving invasive technologies, non-autonomous systems, robotic systems or exoskeletons, and studies only exploring relationships between biometrics data (e.g., heart rate variability, skin conductance) and CP without developing predictive models were excluded. To extend the literature scope and guide future research, all experimental study designs were considered. However, protocols, reviews, books, abstracts, editorials, commentaries, dissertations, and poster presentations were excluded. In cases where the same author has multiple publications on this review topic, the publication with the most comprehensive and updated data was prioritized while ensuring relevance to the scoping review objectives. Studies were also excluded if they focused on activity recognition or virtual reality systems, or had other purposes.
2.4 Data charting
The relevant information was extracted and synthesized: (1) authors, publication year and country; (2) sample size and participants characteristics; (3) intervention settings (e.g., hospital, home, or laboratory); (4) type of the wearable device used; (5) prediction models and their accuracy; (6) outcomes, focusing on technical effectiveness, usability, data security, and conformity to relevant norms and standards.
2.5 Summary and report of the results
The summary and report included an overview of current wearable devices described in the literature, the representation of the most used prediction models, sensing elements and biometric variables across the extracted studies. Additionally, a table is included to illustrate how these devices align with different health standards. An in-depth analysis of the included studies was conducted to identify limitations in the predictive models, thereby providing valuable information to guide future research.
3 Results
The literature search identified 613 references, which were screened for relevance. After removing duplicates, 334 studies were retained for further analysis. Of these, 72 studies were eligible for full-text review. Sixty-two (62) studies were excluded from the analysis for several reasons: they either did not use recorded parameters to predict pain, did not involve patients with CP, or focused exclusively on interventions aimed at reducing CP (e.g., physical therapy, medication trials) without addressing predictive methodologies, such as the use of predictive algorithms. As a result, 10 studies met the inclusion criteria for this scoping review. The study selection process is detailed in the flowchart presented in Figure 1.
3.1 Sensing elements and predictive models for chronic pain
Different tools have been used over the past three years in scientific papers for CP prediction/detection (Figure 2). Wearable sensors, including accelerometers and optical sensors, enable real-time monitoring of physiological parameters such as heart rate and step count. These advancements not only aid in the prediction of CP but also enhance patient engagement and preventive health management. Figure 3 illustrates the various algorithms, sensing elements, and predicted variables used in CP prediction. Methods such as Random Forest algorithms, accelerometer sensors, and key variables like movement intensity, heart rate, heart rate variability, and electrodermal activity (EDA) have shown particular effectiveness. These approaches provide valuable information into CP intensity and its modulation, which could enable more precise and personalized pain management strategies.
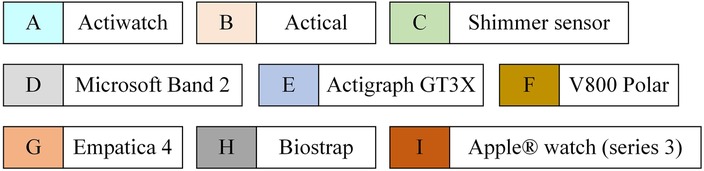
Figure 2. Overview of wearable chronic pain management devices. For more information on these devices, please refer to the following ref. [21, 38, 40, 42, 43, 45, 64].
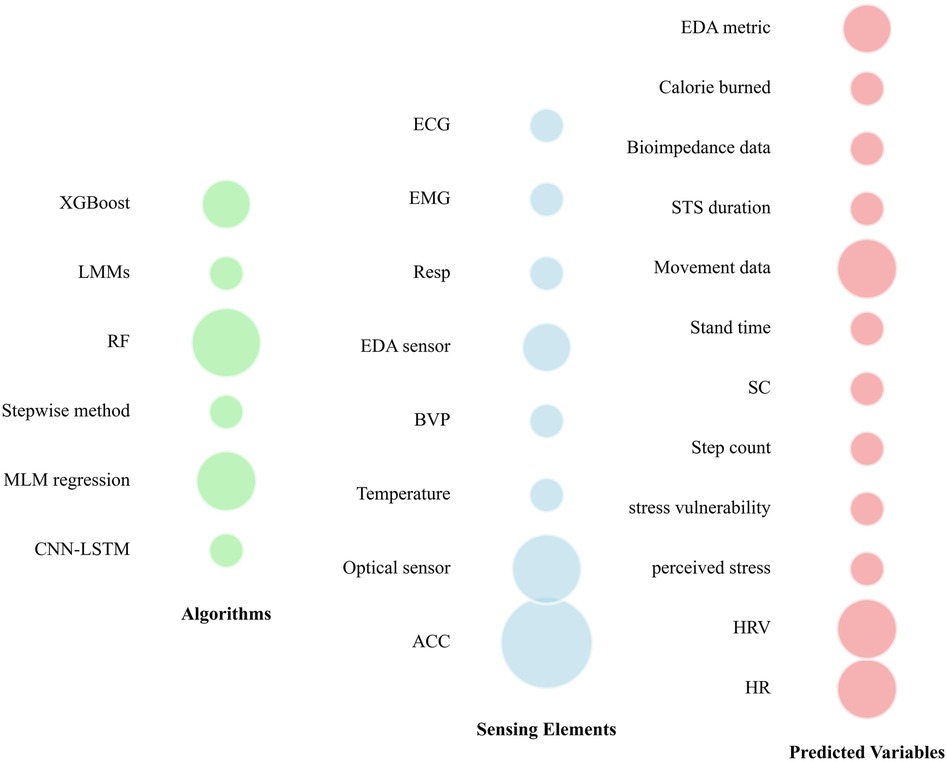
Figure 3. Predictive models, sensors, and variables used across literature for predicting chronic pain. CNN-LSTM, convolutional neural network-long short-term memory; MLM, multilevel model; RF, random forest; LMMs, linear mixed-effects models; ACC, accelerometer; BVP, blood volume pulse; EDA, electrodermal activity; Resp, respiration sensor; EMG, electromyography; ECG, electrocardiogram; HR, heart rate; HRV, heart rate variability; SC, skin conductance; STS: sit-to-stand.
3.2 Multimodal for chronic pain prediction
The studies summarized in this scoping review have achieved varying levels of accuracy, using a number of technologies (Figure 2) and models (Table 1). More than half of the studies focused on patients with an average age above 40 years (Table 1). Notably, one study was conducted in a naturalistic setting, collecting data from 688 patients with CP. The remaining were conducted in clinical, home and/or laboratory settings. A variety of subjective pain scales, including self-report measures such as the Visual Analog Scale (VAS), the McGill Pain Questionnaire, and the Brief Pain Inventory, are employed by these studies to validate pain intensity. In addition, our scoping review specifically examined how physiological data were combined to other parameters for predicting pain intensity. While some studies demonstrate a combination of specific physiological markers correlated with self-reports pain, no study has revealed how factors such as demographic and clinical characteristics, environmental factors, and technology adoption can provide more comprehensive information on chronic pain prediction (Figure 4). Table 2 summarizes the use of multimodal inputs and highlights their potential complementary contributions to CP prediction, emphasizing the missed opportunity for more integrated modeling approaches in the current literature.
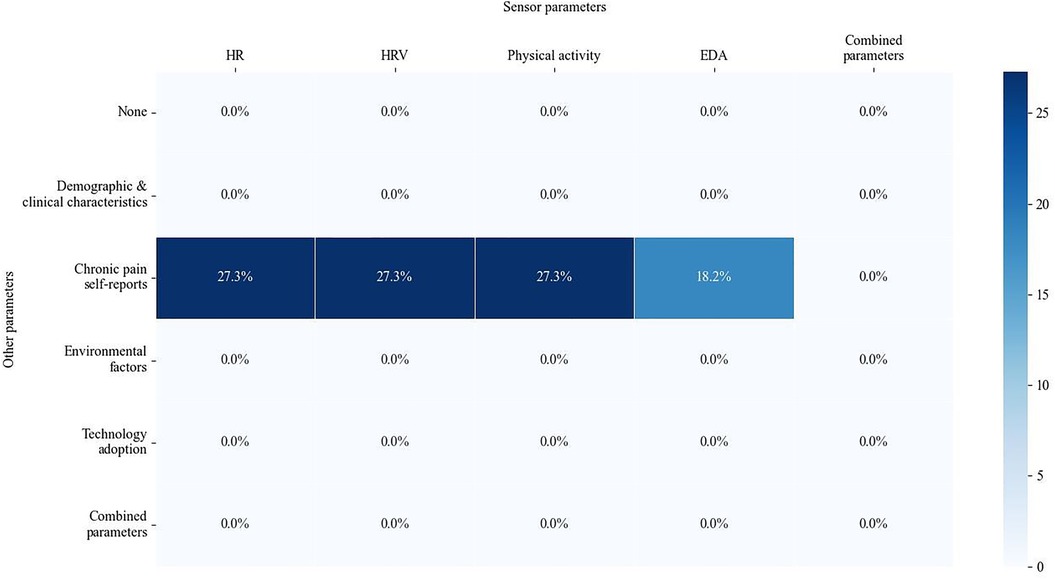
Figure 4. Percentage of studies by parameter combination for chronic assessment purpose. HR, heart rate; HRV, heart rate variability; EDA, electrodermal activity.
3.3 Data security, privacy and standards compliance in wearable devices for CP
While all these devices (Figure 2) are wearable smart devices, they collect, store, process, treat, and deliver data. These data are considered personal data and must be protected and handled securely to ensure the privacy of healthcare information. For these reasons, many industry standards and regulations, presented in Table 3, provide guidelines and techniques to ensure data security in connected devices, including healthcare devices. Indeed, industrial standards like ISO 27001 require conformity with techniques such as data encryption, user authentication, data masking, access control, secure development lifecycle, backup and restore management, protection against malware, equipment maintenance, and compliance with data protection regulations. Table 4 outlines the challenges, methods as well as some recommendations that should be considered when using those techniques. These recommendations are based on several different sources, showing the different challenges and methods of the techniques used. Personal data protection regulations such as the GDPR (General Data Protection Regulation) require that individuals whose data is being collected must be informed about the data being collected, and they must be able to access, rectify, or delete it. The regulation also defines restrictions and special requirements for data transferred both locally and internationally. GDPR mandates that data collection be minimized to what is strictly necessary for the intended purposes. The results reported in Table 5 show the conformity of each device with one or more of the mentioned industrial standards and regulations.
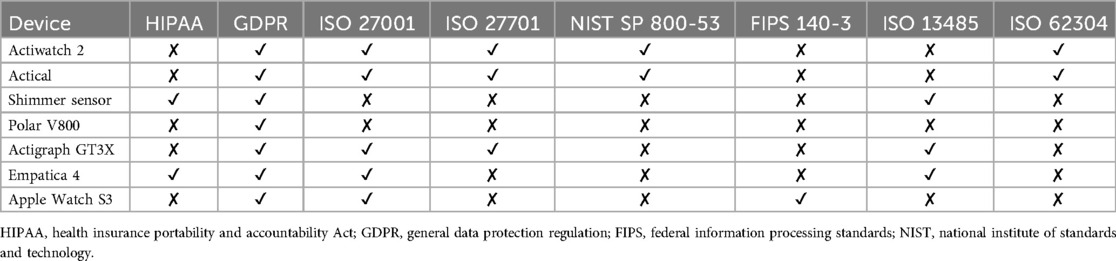
Table 5. Health standards applied to the wearable devices displayed in Figure 2.
4 Discussion
Advancements in artificial intelligence and predictive analytics offer promising opportunities for predicting chronic pain (CP) intensity and tailoring interventions. In the following subsections, we discuss various aspects involved in designing wearable devices for managing CP.
4.1 Sensing elements
Sensing elements play a crucial role in acquiring biometric and environmental data. Our findings reveal that certain sensors, such as the accelerometer and optical sensors, are widely used (Figure 3), underlining their importance in pain management. These sensors seem to be preferred for their availability, ease of integration into wearable devices and ability to provide reliable data in real time. For example, accelerometer are essential for applications such as step counting and posture analysis (33–36), while optical sensors provide precise heart rate measurements using photoplethysmographic methods (37–40). In contrast, other sensors such as temperature, ECG, and EMG, although valuable in specific contexts, are less commonly used (Figure 3). This may be due to constraints such as higher cost, larger size, or probably the complexity of interpreting the data they generate. Finally, the use of specialized sensors, such as EDA sensors, reflects a growing interest in psychophysiological measures such as perceived stress (38, 41). The use of such type of sensor (EDA) indicates a trend towards more focused sensors for CP assessment. Overall, our findings underline the evolution of demand for advanced instrumentation, with an emphasis on the preference for versatile and easily integrable sensors. This present scoping review also suggests a reconsideration of design strategies to incorporate specialized sensors where they can enhance predictive models.
4.2 Sensor data quality
When assessing the quality of sensor data for CP monitoring, only devices such as Empatica 4, Polar V800, and Shimmer Sensor (Figure 2) appear to provide features that are suited to tracking physiological and behavioral parameters associated with pain (42, 43). Indeed, high-quality sensors ensure accurate computation of critical parameters such as heart rate variability, skin conductance, movement patterns, and sleep quality, which are critical indicators in chronic pain studies (38, 41). Moreover, Shimmer sensor and Empatica 4, which comply with standards such as ISO 13485 and HIPAA (Health Insurance Portability and Accountability), demonstrate the reliability and accuracy required for medical-grade applications (Table 5). Specifically, Empatica 4 stands out in particular in the field of continuous monitoring, thanks to its ability to detect substantial changes in stress and autonomic responses, facilitating the assessment of CP (38, 41). The accurate and consistent data provided by these sensors offer valuable information into pain triggers, individual response patterns, and treatment effectiveness, making them indispensable tools for researchers and clinicians in CP management.
4.3 Predictive models for chronic pain
Random Forest (RF) demonstrated strong performance across multiple studies, achieving notable accuracy levels such as 0.768 ± 0.012 (39); 91.67% (41) and 84.52% (44) (Figure 3, Table 1). This consistency highlights its efficiency in handling large datasets with non-linear relationships. In addition, its area under the curve (AUC) of 0.79 ± 0.18 in one study (45) demonstrates its robustness in classifying pain vs. no pain, particularly in scenarios requiring a balance between sensitivity and specificity. Multilevel Model (MLM) regression also shows significant predictive power, with a t-test coefficient of 0.012 and p-value of 0.005, indicating its statistical reliability in capturing relevant data patterns for CP prediction (40). Similarly, the stepwise method, although less used across studies (Figure 3), achieved an adjusted R² of 0.154, p = 0.001 (Table 1), indicating moderate predictive ability in the dataset used (38). In contrast, deep learning methods such as Convolutional Neural Network-Long Short-Term Memory (CNN-LSTM) produced weaker results, with AUC values of 0.60 for validation and 0.57 for testing (46), which may reflect challenges in optimizing these models. However, their ability to extract features and model time series suggests that, with improved data quality or tuning, they could provide more significant advantages (47). XGBoost delivered an accuracy of 74.63%, demonstrating its reliability in scenarios with well-preprocessed datasets (48). This performance makes it a viable alternative for real-time CP prediction. Our review highlights the trade-offs inherent in the choice of predictive algorithms. Indeed, for predicting CP, we can note that traditional machine learning models such as RF and MLM regression are more dominant (Figure 3), likely due to their reliability and ease of implementation. However, advanced techniques such as CNN-LSTM and XGBoost are also becoming more popular, especially in scenarios requiring computational efficiency (49). Our results underline the importance of aligning algorithm selection with the specific characteristics of the dataset and the prediction of CP.
4.4 Multimodal data integration
This study also emphasizes the crucial importance of integrating multimodal data to improve the prediction of CP. While physiological data such as HR, HRV, physical activity and EDA present objective information (41, 45), they may fail to capture the subjective and contextual dimensions of pain. Similarly, demographic and clinical characteristics present essential information, but lack specificity when used alone. The absence of studies (Figure 4) exploiting fully combined parameters shows a missed opportunity to model the complex and multifaceted nature of CP. Combining diverse data sources could improve predictive accuracy by capturing the interplay between biological, psychological and environmental factors, ultimately leading to more personalized and effective CP management strategies.
To facilitate this integration, a structured approach is necessary. Multimodal data can be processed using advanced modeling techniques such as feature-level fusion (e.g., concatenating features before model input) (50), decision-level fusion (e.g., ensembling predictions from unimodal models) (51), or representation learning approaches like deep learning, which can automatically capture cross-modal interactions (52). For example, synchronizing physiological signals with self-report pain ratings (Table 1) could provide a more comprehensive view of CP experience. Table 2 summarizes the various data types and their potential contributions to CP prediction, highlighting how each modality could add unique value. Such integration will not only enhance model interpretability and generalizability, but also enable the way for real-time, adaptive systems capable of supporting dynamic, personalized CP management.
4.5 Data security, privacy and compliance with health standards
Manufacturers like Philips, Shimmer, Polar, ActiGraph, Empatica and Apple, reported their compliance with several industry standards and regulations related to data security and privacy. However, companies like Microsoft and Biosignalsplux, have not reported any compliance with any of the mentioned standards and regulations (Table 3). Table 5 summarizes the compliance status of different devices with the industry standards and regulations.
For Actiwatch2 and Actical, Philips, the manufacturer, mentioned several implemented measures to protect personal data and ensure all data security collected by those devices (53). For shimmer sensors and Polar V800, the manufacturer does not have access to the data. All collected, transferred and stocked data are handled by the end user. When a device is returned for maintenance, the manufacturer ensures that all data is immediately deleted. For these reasons, the applicable techniques and methods (Table 4) are not relevant. Thus, there is no information about the maintenance of the software and the hardware (54). The Microsoft band 2 lacks detailed documentation since it is no longer available on the market. We have not found any reliable information about the techniques and methods used. ActiGraph GTX3 and Empatica4, both of the manufacturers, ensure that they take reasonable and appropriate measures to protect personal data, but there is not enough information for the used methods (55, 56). Regarding the Apple Watch Series 3, Apple decided that this model will no longer receive updates to watchOs 9, and support for watchOs 8 will also be discontinued (57). So, this device becomes vulnerable.
Two main issues arise across these devices. First, there is a huge amount of data to be stored and transferred which increases the complexity of the data security process. Second, devices like Biosignalsplux, which do not provide data storage in cloud or in-premise servers, place the recorded data in the hands of the end user. Despite compliance with industrial standards and regulations by manufacturers, data privacy and protection continue to be at risk, as end users, including researchers, must also follow the good practices described in Table 4.
4.6 Challenges and perspectives for improvement
Beyond the promise of technology integration in CP management, significant challenges remain in realizing the full potential of these innovations. Data privacy concerns, interoperability issues between different systems and disparities in access to technology are key obstacles to the widespread adoption of technological CP management solutions. The need to ensure that patient data is protected and that technologies work smoothly across different platforms is essential to building trust and efficiency (58, 59). Similarly, in the context of predictive algorithms and sensing elements, there are notable challenges. One of the main issues is the variability in model performance across studies, as shown by the large fluctuations in the accuracy of models, whose results range from 37.72% to 91.67% (Table 1). Furthermore, the CNN-LSTM model showed low AUC values (0.60 for validation and 0.57 for testing), highlighting the need for greater consistency in predictive capabilities. These inconsistencies underline the need to improve the quality and standardization of the datasets used for training and validation to ensure more reliable and reproducible results. Furthermore, the computational complexity of advanced models such as CNN-LSTM could limit their applicability in real-time systems, particularly in resource-constrained environments. Additionally, integrating various sensing elements such as EDA, ECG, accelerometers, and others presents challenges related to sensor fusion and data synchronization (60). These issues can affect prediction accuracy, making it difficult to achieve consistent and meaningful results across diverse populations and conditions.
To overcome these challenges, future research should focus on a variety of strategies. Standardizing data collection and preprocessing will help ensure data quality and comparability, facilitating the training of more robust and generalizable models. Optimizing feature selection techniques, tailored specifically to the characteristics of the collected data, is essential to improve the performance of predictive algorithms (41). Adapting models to the specific application will help improve their relevance and effectiveness in the real world (52). Refining the real-time capabilities of these systems is also crucial, as the ability to provide immediate feedback based on continuous data streams could greatly improve patient outcomes. Moreover, promoting interdisciplinary collaboration between data scientists, clinicians, engineers, and healthcare providers will be crucial to develop more practical and reliable predictive systems that can be integrated into clinical practice.
Longitudinal studies are needed to investigate the temporal relationships between physiological changes and CP episodes. Such research would help to understand how individual physiological profiles change over time and how these changes correlate with CP outcomes (61). Personalized algorithms that predict CP outcomes based on these profiles could enable great potential to improve pain management by tailoring interventions to the unique needs of each patient. In addition, emerging technologies, such as wearable sensors and advanced machine learning algorithms (21), provide exciting opportunities for real-time monitoring. These innovations could enable continuous monitoring of pain-related physiological parameters, allowing for more dynamic and responsive pain management strategies that adapt to the needs of the individual in real time. However, integrating these technologies into clinical practice will require overcoming existing barriers and ensuring that the systems are both clinically effective and accessible to diverse populations. Moreover, compliance with industry standards and regulations (Table 3) will be essential to ensure data security and privacy.
Conclusion
Wearable devices offer significant potential for chronic pain (CP) management through real-time monitoring and personalized treatments. However, their ability to predict the evolution of CP remains limited, as most studies focus on the correlation between physiological markers and CP rather than predicting CP episodes. In this scoping review, we focused on predictive models for CP as well as the possibility of integrating multimodal data, combining physiological, psychological, and demographic factors. While models such as Random Forest are promising, more complex algorithms face challenges related to data quality and computational limitations. Data security and privacy also remain major concerns. Although many proposed devices for CP adhere to regulations such as GDPR and ISO, there are still gaps in user data protection. Future research should focus on developing robust predictive models, addressing security issues, and standardizing data protocols. These efforts will enhance the efficacy and clinical applicability of wearable devices, facilitating more efficient and personalized pain management solutions.
Data availability statement
The original contributions presented in the study are included in the article/Supplementary Material, further inquiries can be directed to the corresponding author.
Author contributions
JA: Conceptualization, Data curation, Formal analysis, Investigation, Methodology, Software, Visualization, Writing – original draft, Writing – review & editing. AB: Data curation, Investigation, Methodology, Visualization, Writing – original draft, Writing – review & editing. MB: Conceptualization, Data curation, Investigation, Methodology, Visualization, Writing – original draft, Writing – review & editing. YO: Formal analysis, Resources, Writing – review & editing. KL: Formal analysis, Resources, Writing – review & editing. DA: Data curation, Resources, Validation, Visualization, Writing – review & editing. IS: Data curation, Funding acquisition, Project administration, Resources, Validation, Visualization, Writing – review & editing. LE: Data curation, Funding acquisition, Project administration, Resources, Validation, Writing – review & editing. NM: Conceptualization, Data curation, Funding acquisition, Investigation, Project administration, Resources, Supervision, Validation, Visualization, Writing – review & editing.
Funding
The author(s) declare that financial support was received for the research and/or publication of this article. This research was supported by the TELUQ University and the Canada Research Chair on Biomedical Data Mining (950-231214).
Conflict of interest
The authors declare that the research was conducted in the absence of any commercial or financial relationships that could be construed as a potential conflict of interest.
Generative AI statement
The author(s) declare that no Generative AI was used in the creation of this manuscript.
Publisher's note
All claims expressed in this article are solely those of the authors and do not necessarily represent those of their affiliated organizations, or those of the publisher, the editors and the reviewers. Any product that may be evaluated in this article, or claim that may be made by its manufacturer, is not guaranteed or endorsed by the publisher.
Supplementary material
The Supplementary Material for this article can be found online at: https://www.frontiersin.org/articles/10.3389/fdgth.2025.1581285/full#supplementary-material
References
1. Anderson R, Bury M. Living with Chronic Illness: The Experience of Patients and Their Families. London: Taylor & Francis (2024).
2. Cohen SP, Vase L, Hooten WM. Chronic pain: an update on burden, best practices, and new advances. Lancet. (2021) 397(10289):2082–97. doi: 10.1016/S0140-6736(21)00393-7
3. Goldberg DS, McGee SJ. Pain as a global public health priority. BMC Public Health. (2011) 11(1):770. doi: 10.1186/1471-2458-11-770
4. Andrews P, Steultjens M, Riskowski J. Chronic widespread pain prevalence in the general population: a systematic review. Eur J Pain. (2018) 22(1):5–18. doi: 10.1002/ejp.1090
5. Aguiar DP, Souza C, Barbosa WJM, Santos-Júnior FFU, Oliveira A. Prevalence of chronic pain in Brazil: systematic review. BrJP. (2021) 4:257–67. doi: 10.5935/2595-0118.20210041
6. Yong RJ, Mullins PM, Bhattacharyya N. Prevalence of chronic pain among adults in the United States. Pain. (2022) 163(2):e328–e32. doi: 10.1097/j.pain.0000000000002291
7. Brown D, Schenk S, Genent D, Zernikow B, Wager J. A scoping review of chronic pain in emerging adults. Pain Rep. (2021) 6(1):e920. doi: 10.1097/PR9.0000000000000920
8. Rikard SM. Chronic pain among adults—United States, 2019–2021. Morb Mortal Wkly Rep. (2023) 72(15):379–85. doi: 10.15585/mmwr.mm7215a1
9. Li X, Zhu W, Li J, Huang C, Yang F. Prevalence and characteristics of chronic pain in the Chinese community-dwelling elderly: a cross-sectional study. BMC Geriatr. (2021) 21:1–10. doi: 10.1186/s12877-021-02432-2
10. World Health Organization. Low Back Pain. Geneva: World Health Organization (2023). Available at: https://www.who.int/news-room/fact-sheets/detail/low-back-pain
11. Gupta R. Non-pharmaceutical management of chronic pain. GSC Adv Res Rev. (2023) 16(2):158–65. doi: 10.30574/gscarr.2023.16.2.0112
12. Perez J, Niburski K, Stoopler M, Ingelmo P. Telehealth and chronic pain management from rapid adaptation to long-term implementation in pain medicine: a narrative review. Pain Rep. (2021) 6(1):e912. doi: 10.1097/PR9.0000000000000912
13. Cavanagh R, Bhargava A, Gleason A, Micheel L, Ciulla R, Hoyt T. Use of health technologies for low back pain in the military health system. J Technol Behav Sci. (2019) 4(4):360–71. doi: 10.1007/s41347-019-00107-9
14. Li YH, Bai L, Mao YX, Ren HL, Qiao Y, Tong X, et al. Rethinking pain communication of patients with Alzheimer’s disease through E-textile interaction design. Front Physiol. (2023) 14:1248893. doi: 10.3389/fphys.2023.1248893
15. Kong Y, Posada-Quintero HF, Chon KH. Pain detection using a smartphone in real time. 2020 42nd Annual International Conference of the IEEE Engineering in Medicine & Biology Society (EMBC); 20-24 July 2020 (2020).
16. Chen J, Abbod M, Shieh J-S. Pain and stress detection using wearable sensors and devices—a review. Sensors (Switzerland). (2021) 21(4):1–18. doi: 10.3390/s21041030
17. Gouverneur P, Li F, Adamczyk WM, Szikszay TM, Luedtke K, Grzegorzek M. Comparison of feature extraction methods for physiological signals for heat-based pain recognition. Sensors. (2021) 21(14):4838. doi: 10.3390/s21144838
18. Kampusch S, Edegger K, Mayr P, Le VH, Kaniusas E, Zeiner K, et al. Integrated platform for the management of chronic low back pain. Stud Health Technol Inform. (2022) 293:260–1. doi: 10.3233/SHTI220378
19. Toor T, Palyo S, Schopmeyer K, Simmons AN, Strigo IA. Exploring the effects of fitbit incentive on treatment outcomes in veterans undergoing intensive pain rehabilitation program. J Patient Rep Outcomes. (2024) 8(1):42. doi: 10.1186/s41687-024-00721-z
20. Saleem A, Langstone B, Ouskine A, Rajabiyazdi F. Design and Development of PainBit: a Portable Device for Supporting Patients with Chronic Pain to Log their Pain. (2024).
21. Avila FR, McLeod CJ, Huayllani MT, Boczar D, Giardi D, Bruce CJ, et al. Wearable electronic devices for chronic pain intensity assessment: a systematic review. Pain Pract. (2021) 21(8):955–65. doi: 10.1111/papr.13047
22. Edgley K, Horne AW, Saunders PTK, Tsanas A. Symptom tracking in endometriosis using digital technologies: knowns, unknowns, and future prospects. Cell Rep Med. (2023) 4(9):101192. doi: 10.1016/j.xcrm.2023.101192
23. Shi JL, Sit RW. Impact of 25 years of Mobile health tools for pain management in patients with chronic musculoskeletal pain: systematic review. J Med Internet Res. (2024) 26:e59358. doi: 10.2196/59358
24. Simon J, Hooijman IS, Van Gorp M, Schepers SA, Michiels EMC, Tissing WJE, et al. Digital health tools for pain monitoring in pediatric oncology: a scoping review and qualitative assessment of barriers and facilitators of implementation. Support Care Cancer. (2023) 31(3):175. doi: 10.1007/s00520-023-07629-2
25. Vitali D, Olugbade T, Eccleston C, Keogh E, Bianchi-Berthouze N, de C Williams AC. Sensing behavior change in chronic pain: a scoping review of sensor technology for use in daily life. Pain. (2024) 165(6):1348–60. doi: 10.1097/j.pain.0000000000003134
26. Ong K, Seymour R. Pain measurement in humans. Surgeon. (2004) 2(1):15–27. doi: 10.1016/S1479-666X(04)80133-1
27. Hathaliya JJ, Tanwar S. An exhaustive survey on security and privacy issues in healthcare 4.0. Comput Commun. (2020) 153:311–35. doi: 10.1016/j.comcom.2020.02.018
28. Keshta I, Odeh A. Security and privacy of electronic health records: concerns and challenges. Egyptian Informatics Journal. (2021) 22(2):177–83. doi: 10.1016/j.eij.2020.07.003
29. Tricco AC, Lillie E, Zarin W, O'Brien KK, Colquhoun H, Levac D, et al. PRISMA extension for scoping reviews (PRISMA-ScR): checklist and explanation. Ann Intern Med. (2018) 169(7):467–73. doi: 10.7326/M18-0850
30. Daudt HM, Van Mossel C, Scott SJ. Enhancing the scoping study methodology: a large, inter-professional team’s experience with Arksey and O’Malley’s framework. BMC Med Res Methodol. (2013) 13:1–9. doi: 10.1186/1471-2288-13-48
31. Veritas Health Innovation. Covidence Systematic Review Software. Melbourne, Australia: Veritas Health Innovation (2014). Available at: https://www.covidence.org (Accessed November 04, 2024).
32. Kang Y, Trewern L, Jackman J, McCartney D, Soni A. Chronic pain: definitions and diagnosis. Br Med J. (2023) 381:e076036. doi: 10.1136/bmj-2023-076036
33. Berger M, Bertrand AM, Robert T, Chèze L. Measuring objective physical activity in people with chronic low back pain using accelerometers: a scoping review. Front Sports Act Living. (2023) 5:1236143. doi: 10.3389/fspor.2023.1236143
34. Ogawa T, Castelo-Branco L, Hatta K, Usui C. Association between step count measured with a smartphone app (pain-note) and pain level in patients with chronic pain: observational study. JMIR Form Res. (2022) 6(4):e23657. doi: 10.2196/23657
35. Bayartai M-E, Taulaniemi A, Tokola K, Vähä-Ypyä H, Parkkari J, Husu P, et al. Role of the interaction between lumbar kinematics and accelerometer-measured physical activity in bodily pain, physical functioning and work ability among health care workers with low back pain. J Electromyogr Kinesiol. (2023) 69:102744. doi: 10.1016/j.jelekin.2023.102744
36. Norha J, Hautala AJ, Sjöros T, Laine S, Garthwaite T, Knuuti J, et al. Standing time and daily proportion of sedentary time are associated with pain-related disability in a one month accelerometer measurement in adults with overweight or obesity. Scand J Pain. (2022) 22(2):317–24. doi: 10.1515/sjpain-2021-0108
37. Chow H-W, Yang C-C. Accuracy of optical heart rate sensing technology in wearable fitness trackers for young and older adults: validation and comparison study. JMIR Mhealth Uhealth. (2020) 8(4):e14707. doi: 10.2196/14707
38. Gungormus DB, Garcia-Moreno FM, Bermudez-Edo M, Sánchez-Bermejo L, Garrido JL, Rodríguez-Fórtiz MJ, et al. A semi-automatic mHealth system using wearable devices for identifying pain-related parameters in elderly individuals. Int J Med Inform. (2024) 184:105371. doi: 10.1016/j.ijmedinf.2024.105371
39. Heros R, Patterson D, Huygen F, Skaribas I, Schultz D, Wilson D, et al. Objective wearable measures and subjective questionnaires for predicting response to neurostimulation in people with chronic pain. Bioelectron Med. (2023) 9(1):13. doi: 10.1186/s42234-023-00115-4
40. Dudarev V, Barral O, Radaeva M, Davis G, Enns JT. Night time heart rate predicts next-day pain in fibromyalgia and primary back pain. Pain Rep. (2024) 9(2):e1119. doi: 10.1097/PR9.0000000000001119
41. Luebke L, Gouverneur P, Szikszay TM, Adamczyk WM, Luedtke K, Grzegorzek M. Objective measurement of subjective pain perception with autonomic body reactions in healthy subjects and chronic back pain patients: an experimental heat pain study. Sensors. (2023) 23(19):8231. doi: 10.3390/s23198231
42. Shimmer Discovery in Motion. Shimmer3 IMU Unit 2024. Available at: https://shimmersensing.com/product/shimmer3-imu-unit/ (Accessed June 10, 2024).
43. Cilhoroz B, Giles D, Zaleski A, Taylor B, Fernhall B, Pescatello L. Validation of the polar V800 heart rate monitor and comparison of artifact correction methods among adults with hypertension. PLoS One. (2020) 15(10):e0240220. doi: 10.1371/journal.pone.0240220
44. Tomlinson WJ, Abarca F, Chowdhury KR, Stojanovic M, Yu C. Experimental assessment of human-body-like tissue as a communication channel for galvanic coupling. 2015 IEEE 12th International Conference on Wearable and Implantable Body Sensor Networks (BSN) (2015).
45. Sett N, Mac Namee B, Calvo F, Caulfield B, Costello J, Donnelly SC, et al. Are you in pain? Predicting pain and stiffness from wearable sensor activity data. Artificial Intelligence XXXVI: 39th SGAI International Conference on Artificial Intelligence, AI 2019; Cambridge, UK: Springer (2019).
46. Dorris H, Oh J, Jacobson N. Wearable movement data as a potential digital biomarker for chronic pain: an investigation using deep learning. J Phys Act Health. (2024) 8(1):83–92. doi: 10.5334/paah.329
47. Ismail Fawaz H, Forestier G, Weber J, Idoumghar L, Muller P-A. Deep learning for time series classification: a review. Data Min Knowl Discov. (2019) 33(4):917–63. doi: 10.1007/s10618-019-00619-1
48. Jacobson NC, O'Cleirigh C. Objective digital phenotypes of worry severity, pain severity and pain chronicity in persons living with HIV. Br J Psychiatry. (2021) 218(3):165–7. doi: 10.1192/bjp.2019.168
49. Shi Z, Hu Y, Mo G, Wu J. Attention-based CNN-LSTM and XGBoost hybrid model for stock prediction. arXiv [Preprint]. arXiv:2204.02623 (2022). doi: 10.48550/arXiv.2204.02623
50. Xiahou X, Li Z, Xia J, Zhou Z, Li Q. A feature-level fusion-based multimodal analysis of recognition and classification of awkward working postures in construction. J Constr Eng Manag. (2023) 149(12):04023138. doi: 10.1061/JCEMD4.COENG-13795
51. Gumaei A, Ismail WN, Hassan MR, Hassan MM, Mohamed E, Alelaiwi A, et al. A decision-level fusion method for COVID-19 patient health prediction. Big Data Research. (2022) 27:100287. doi: 10.1016/j.bdr.2021.100287
52. Jemal I, Mezghani N, Abou-Abbas L, Mitiche A. An interpretable deep learning classifier for epileptic seizure prediction using EEG data. IEEE Access. (2022) 10:60141–50. doi: 10.1109/ACCESS.2022.3176367
53. Philips. Committed to proactively addressing our customers’ security and privacy concerns. (2023).
54. Shimmer. Regulatory & Quality Certifications (2024) Available at: https://shimmersensing.com/about/certifications/ (Accessed November 15, 2024).
55. Actigraph. ActiGraph Compliance Center (2024) Available at: https://theactigraph.com/compliance (Accessed November 15, 2024).
56. Empatica. Legal & Compliance Available at: https://www.empatica.com/en-eu/legal/ (Accessed November 15, 2024).
57. Intego. Apple stops selling Watch Series 3 — eight months after its last security update. (2023) Available at: https://www.intego.com/mac-security-blog/apple-stops-selling-watch-series-3-eight-months-after-its-last-security-update/ (Accessed November 15, 2024).
58. Abouelmehdi K, Beni-Hessane A, Khaloufi H. Big healthcare data: preserving security and privacy. J Big Data. (2018) 5(1):1–18. doi: 10.1186/s40537-017-0110-7
59. Haleem A, Javaid M, Singh RP, Suman R. Medical 4.0 technologies for healthcare: features, capabilities, and applications. Internet Things Cyber-Phys Syst. (2022) 2:12–30. doi: 10.1016/j.iotcps.2022.04.001
60. Lahat D, Adali T, Jutten C. Multimodal data fusion: an overview of methods, challenges, and prospects. Proc IEEE. (2015) 103(9):1449–77. doi: 10.1109/JPROC.2015.2460697
61. Jones AM. The fourth dimension: physiological resilience as an independent determinant of endurance exercise performance. J Physiol (Lond). (2024) 602(17):4113–28. doi: 10.1113/JP284205
62. Perraudin CG, Illiano VP, Calvo F, O’Hare E, Donnelly SC, Mullan RH, et al. Observational study of a wearable sensor and smartphone application supporting unsupervised exercises to assess pain and stiffness. Digit Biomark. (2019) 2(3):106–25. doi: 10.1159/000493277
63. Critcher S, Parmelee P, Freeborn TJ. Localized multi-site knee bioimpedance as a predictor for knee osteoarthritis associated pain within older adults during free-living. IEEE Open J Eng Med Biol. (2023) 4:1–10. doi: 10.1109/OJEMB.2023.3256181
64. Stojancic RS, Subramaniam A, Vuong C, Utkarsh K, Golbasi N, Fernandez O, et al. Predicting pain in people with sickle cell disease in the day hospital using the commercial wearable apple watch: feasibility study. JMIR Form Res. (2023) 7:e45355. doi: 10.2196/45355
65. Zhang Q. An overview and analysis of hybrid encryption: the combination of symmetric encryption and asymmetric encryption. 2021 2nd International Conference on Computing and Data Science (CDS) (2021).
66. Das S, Namasudra S. A novel hybrid encryption method to secure healthcare data in IoT-enabled healthcare infrastructure. Comput Electr Eng. (2022) 101:107991. doi: 10.1016/j.compeleceng.2022.107991
67. Nyangaresi VO, Ogundoyin SO. Certificate based authentication scheme for smart homes. 2021 3rd Global Power, Energy and Communication Conference (GPECOM) (2021).
68. Suleski T, Ahmed M, Yang W, Wang E. A review of multi-factor authentication in the internet of healthcare things. Digit Health. (2023) 9:20552076231177144. doi: 10.1177/20552076231177144
69. Jain RB, Puri M. An Approach Towards the Development of Scalable Data Masking for Preserving Privacy of Sensitive Business Data. Artificial Intelligence and Evolutionary Computations in Engineering Systems. Singapore: Springer (2020).
71. Qiu J, Tian Z, Du C, Zuo Q, Su S, Fang B. A survey on access control in the age of internet of things. IEEE Internet Things J. (2020) 7(6):4682–96. doi: 10.1109/JIOT.2020.2969326
72. Ramesh G, Logeshwaran J, Aravindarajan V. A secured database monitoring method to improve data backup and recovery operations in cloud computing. BOHR Int J Comput Sci. (2022) 2(1):1–7. doi: 10.54646/bijcs.019
73. Perception point. Malware Protection: Types, Tools and Best Practices. (2024). Available at: https://perception-point.io/guides/malware/malware-protection-types-tools-best-practices/ (Accessed January 06, 2025).
74. Thales. What is a Software Maintenance Process? 4 Types of Software Maintenance. Paris: Thales Group (2024). Available at: https://cpl.thalesgroup.com/software-monetization/four-types-of-software-maintenance
Keywords: chronic pain, wearable device, privacy, standardization, predictive analytics
Citation: Ayena JC, Bouayed A, Ben Arous M, Ouakrim Y, Loulou K, Ameyed D, Savard I, El Kamel L and Mezghani N (2025) Predicting chronic pain using wearable devices: a scoping review of sensor capabilities, data security, and standards compliance. Front. Digit. Health 7:1581285. doi: 10.3389/fdgth.2025.1581285
Received: 21 February 2025; Accepted: 5 May 2025;
Published: 22 May 2025.
Edited by:
Shameer Khader, Sanofi, FranceReviewed by:
Deana Davalos, Colorado State University, United StatesZarina Shameer, AbbVie, United States
Copyright: © 2025 Ayena, Bouayed, Ben Arous, Ouakrim, Loulou, Ameyed, Savard, El Kamel and Mezghani. This is an open-access article distributed under the terms of the Creative Commons Attribution License (CC BY). The use, distribution or reproduction in other forums is permitted, provided the original author(s) and the copyright owner(s) are credited and that the original publication in this journal is cited, in accordance with accepted academic practice. No use, distribution or reproduction is permitted which does not comply with these terms.
*Correspondence: Johannes C. Ayena, am9oYW5uZXMuYXllbmFAdGVsdXEuY2E=