- 1Oxford Internet Institute, University of Oxford, Oxford, United Kingdom
- 2Alan Turing Institute, London, United Kingdom
The Everyday Sexism Project documents everyday examples of sexism reported by volunteer contributors from all around the world. It collected 100,000 entries in 13+ languages within the first 3 years of its existence. The content of reports in various languages submitted to Everyday Sexism is a valuable source of crowdsourced information with great potential for feminist and gender studies. In this paper, we take a computational approach to analyze the content of reports. We use topic-modeling techniques to extract emerging topics and concepts from the reports, and to map the semantic relations between those topics. The resulting picture closely resembles and adds to that arrived at through qualitative analysis, showing that this form of topic modeling could be useful for sifting through datasets that had not previously been subject to any analysis. More precisely, we come up with a map of topics for two different resolutions of our topic model and discuss the connection between the identified topics. In the low-resolution picture, for instance, we found Public space/Street, Online, Work related/Office, Transport, School, Media harassment, and Domestic abuse. Among these, the strongest connection is between Public space/Street harassment and Domestic abuse and sexism in personal relationships. The strength of the relationships between topics illustrates the fluid and ubiquitous nature of sexism, with no single experience being unrelated to another.
Introduction
“Women across the country - and all over the world, in fact - are discovering new ways to leverage the internet to make fundamental progress in the unfinished revolution of feminism” - #FemFutureReport (Femfuture, 2017).
Laura Bates, founder of the Everyday Sexism Project, has signaled that “it seems to be increasingly difficult to talk about sexism, equality, and women's rights” (Bates, 2015). With many theorists suggesting that we have entered a so-called “post-feminist” era in which gender equality has been achieved (McRobbie, 2009), to complain about sexism not only risks being labeled as “uptight,” “prudish,” or a “militant feminist,” but also exposes those who speak out to sustained, and at times vicious, personal attacks (Bates, 2015). Despite these risks, thousands of women are speaking out about their experiences of sexism, and are using digital technologies to do so (Martin and Valenti, 2012), leading to the development of a so-called “fourth wave” of feminism, incorporating a range of feminist practices that are enabled by Web 2.0 digital technologies (Munro, 2013).
The “Everyday Sexism Project,” founded by Bates in 2012, is just one of the digital platforms employed in the fight back against sexism. Since its inception, the site has received over 100,000 submissions in more than 13 different languages, detailing a wide variety of experiences. Submissions are uploaded directly to the website, and via the Twitter account @EverydaySexism and hashtag #everydaysexism. Until now, analysis of posts has been largely qualitative in nature, and there has been no systematic analysis of the nature and type of topics discussed, or whether distinct “types” of sexism emerge from the data. In this paper, we expand the methods used to investigate Everyday Sexism submission data by undertaking a large-scale computational study, with the aim of enriching existing qualitative work in this area (Swim et al., 2001; Becker and Swim, 2011). To the best of our knowledge this is the first time a dataset at this scale is being analyzed to come up with a data-driven typology of sexism. It is important to note, however, that the data under study suffer from intrinsic biases of self-reported experiences that might not represent a complete picture of sexism.
Our analysis of the data is based on Natural Language Processing, using topic-modeling techniques to extract the most distinctly occurring topics and concepts from the submissions. We explored data-driven approaches to community-contributed content as a framework for future studies. Our research seeks to draw on the rich history of gender studies in the social sciences, coupling it with emerging computational methods for topic modeling, to better understand the content of reports to the Everyday Sexism Project and the lived experiences of those who post them.
The analysis of “prejudice, stereotyping, or discrimination, typically against women, on the basis of sex” (OED Online, 2018) has formed a central tenet of both academic inquiry and a radical politics of female emancipation for several decades1. Studies of sexism have considered it to be both attitudinal and behavioral, encompassing both the endorsement of oppressive beliefs based on traditional gender-role ideology, and what we might term more “formal” discrimination against women on the basis of their sex, for example in the workplace or in education (Harper, 2008, p. 21). Peter Glick and Susan T. Fiske build on this definition of sexism in their seminal 1996 study The Ambivalent Sexism Inventory: Differentiating Hostile and Benevolent Sexism, where they present a multidimensional theory of sexism that encompasses two components: “hostile” and “benevolent” sexism. As the authors highlight, traditional definitions of sexism have conceptualized it primarily as a reflection of hostility toward women, but this view neglects a significant further aspect of sexism: the “subjectively positive feelings toward women” that often go hand in hand with sexist apathy (Glick and Fiske, 1996, p. 493).
More recent studies, particularly in the field of psychology, have shifted the focus away from who experiences sexism and how it can be defined, toward an examination of the psychological, personal, and social implications that sexist incidents have for women. As such, research by Buchanan and West (2010), Harper (2008), Moradi and Subich (2002), and Swim et al. (2001) has highlighted the damaging intellectual and mental health outcomes for women who are subject to continual experiences of sexism. Moradi and Subich, for example, argue that sexism combines with other life stressors to create significant psychological distress in women, resulting in low self-esteem and the need to “seek therapy, most commonly for depression and anxiety” (Moradi and Subich, 2002, p. 173). Other research indicates that a relationship exists between experiences of sexism over a woman's lifetime and the extent of conflict she perceives in her romantic heterosexual relationships (Harper, 2008); that continual experiences of sexism in an academic environment results in women believing that they are inferior to men (Ossana et al., 1992); and that disordered eating among college women is related to experiences of sexual objectification (Sabik and Tylka, 2006).
Given its increasing ubiquity in everyday life, it is hardly surprising that the relationship between technology and sexism has also sparked interest from contemporary researchers in the field. Indeed, several studies have explored the intersection between gender and power online, with Susan Herring's work on gender differences in computer-mediated communication being of particular note (cf. Herring, 2008). Feminist academics have argued that the way that women fight back against sexism in the digital era is fundamentally shaped by the properties, affordances, and dynamics of the “web 2.0” environments in which much current feminist activism takes place, with social media sites uniquely facilitating “communication, information sharing, collaboration, community building and networking” in ways that neither the static websites of Web 1.0 nor the face-to-face interactions of earlier feminist waves have been able to Carstensen (2009) and Keller (2012).
Theorists in the field of psychology have focused on the impact that using digital technology, and particularly Web 2.0 technologies, to talk about sexism can have on women's well-being. Mindi D. Foster's 2015 study, for example, found that when women tweeted about sexism, and in particular when they used tweets to (a) name the problem, (b) criticize it, or (c) to suggest change, they viewed their actions as effective and had enhanced life satisfaction, and therefore felt empowered (Foster, 2015, p. 21). These findings are particularly relevant to this study, given the range of channels offered by the Everyday Sexism project to those seeking to “call out” sexism that they've experienced or witnessed both online and off.
Despite the diversity of research on sexism and its impact, there remain some notable gaps in understanding. In particular, as this study hopes to highlight, little previous research on sexism has considered the different and overlapping ways in which sexism is experienced by women, or the sites in which these experiences occur, beyond an identification of the workplace and the education system as contexts in which sexism often manifests (as per Klein, 1992; Barnett, 2005; Watkins et al., 2006). Furthermore, research focusing on sexism has thus far been largely qualitative in nature. Although a small number of studies have employed quantitative methods (cf. Becker and Wright, 2011; Brandt, 2011), none have used computational approaches to analyse the wealth of available online data on sexism. Here we seek to fill such a gap. By providing much needed analysis of a large-scale crowd sourced data set on sexism, it is our hope that knowledge gained from this study will advance both the sociological understanding of women's lived experiences of sexism, and methodological understandings of the suitability of computational topic modeling for conducting this kind of research. In other research topic modeling has been extensively used (Puschmann and Scheffler, 2016) to study the history of computational linguistics (Hall et al., 2008), U.S. news media in the wake of terror attacks (Bonilla and Grimmer, 2013), online health discourse (Ghosh and Guha, 2013; Paul and Dredze, 2014), historical shifts in news writing (Yang et al., 2011), political discourse (Koltsova and Koltcov, 2013), and online electoral campaigns (McElwee and Yasseri, 2017). In this project we are interested in discussing in particular, what the emerging topics can tell us about the ways in which sexism is manifested in everyday life.
Data and Methods
We collected the content of posts on the Everyday Sexism website in February 2015, with the permission of the website owner, through a simple web crawler. The project adhered at all times to the Oxford Central University Research Ethics Committee's (CUREC) Best Practice Guidance 06_Version 4.0 on Internet-Based Research (IBR). None of the project entries is quoted in the article, and the approach deliberately looked for patterns and connections rather than isolating individual accounts or contributors.
In processing the data, after cleaning the posts that were not in English, we ended up with 78,783 posts containing 3,221,784 words. We then removed all punctuation and English language stop-words (such as “and,” “it,” “in” etc.) from the data, this is a standard practice in the literature (Wallach et al., 2009). The data were then split into individual words, which were stemmed using an nltk English language snowball stemmer (Perkins, 2010).
Topic modeling is a technique that seeks to automatically discover the topics contained within a group of documents. “Documents” in this context could refer to text items as lengthy as individual books, or as short as sentences within a paragraph. For instance, if we assumed that each sentence of a corpus of text is a “document” we would have:
• Document 1: I like to eat kippers for breakfast.
• Document 2: I love all animals, but kittens are the cutest.
• Document 3: My kitten eats kippers too.
We therefore assume that each sentence contains a mixture of different topics and that a “topic” is a collection of words that are more likely to appear together in a document.
The algorithm is initiated by setting the number of topics that it needs to extract. It is hard to guess this number without having insight into the topics, but one can think of this as a resolution tuning parameter. The smaller the number of topics is set, the more general the bag of words in each topic would be, and the looser the connections between them.
The algorithm loops through all of the words in each document, assigning every word to one of the topics in a temporary and semi-random manner. This initial assignment is arbitrary and it is easy to show that different initializations lead to the same results in long run. Once each word has been assigned a temporary topic, the algorithm then re-iterates through each word in each document to update the topic assignment using two criteria: (1) How prevalent is the word in question across topics? and (2) How prevalent are the topics in the document?
To quantify these two, the algorithm calculates the likelihood of the words appearing in each document assuming the assignment of words to topics (word-topic matrix) and topics to documents (topic-document matrix). Words can appear in different topics and more than one topic can appear in a document. But the iterative algorithm seeks to maximize the self-consistency of the assignment by maximizing the likelihood of the observed word-document statistics.
We can illustrate this process and its outcome by going back to the example above. A topic modeling approach might use the process above to discover the following topics across our documents; the numbers in brackets, show the loading of each topic in the document:
• Document 1: I like to eat kippers for breakfast. [100% Topic A]
• Document 2: I love all animals, but kittens are the cutest. [100% Topic B]
• Document 3: My kitten eats kippers too. [67% Topic A, 33% Topic B],
where
• Topic A: eat, kippers, breakfast
• Topic B: animals, kittens
Topic modeling defines each topic as a so-called “bag of words,” but it is the researcher's responsibility to decide upon an appropriate label for each topic based on their understanding of language and context. Going back to our example, the algorithm might classify the underlined words under Topic A, which we could then label as “food” based on our understanding of what the words mean. Similarly, the italicized words might be classified under a separate topic, Topic B, which we could label “animals.” In this simple example the word “eat” has appeared in a sentence dominated by Topic A, but also in a sentence with some association to Topic B. It can therefore be seen as a connector of the two topics.
We used a similar approach to first extract the main topics reflected in the reports made to the Everyday Sexism Project website. Optimize the log-likelihood of the results. However, this approach suggests that the number of topics 7–20 give practically the same goodness for the model. Therefore, we analyse and discuss the results for both cases of number of topics set to 7 and 20.
To annotate the topics (bags of words) we looked at the top 50 words for each topic, then assigned them to well-known types of everyday sexism. To further disambiguate in cases of topics with mixed bags of words, we referred to the original accounts that were assigned to the topic to check these annotations were accurate.
We then extracted the relation between the sexism-related topics and concepts based on the overlap between the bags of words of each topic. For this we used a simple implementation of the LDA algorithm for topic modeling (Pritchard et al., 2000; Blei et al., 2003).
Results
Tables 1, 2 list the topics that are detected by topic modeling algorithm for two different numbers of topics n = 7 and n = 20. Each row shows the top 50 words that are most prevalent in each topic. The 3rd column shows the qualitative annotations. By increasing the number of topics, we will have less granularity however, the annotation task becomes more difficult as topics become more diverse. However, combining the two pictures we shed light on the most apparent images of sexism as reported on the Everyday Sexism website.
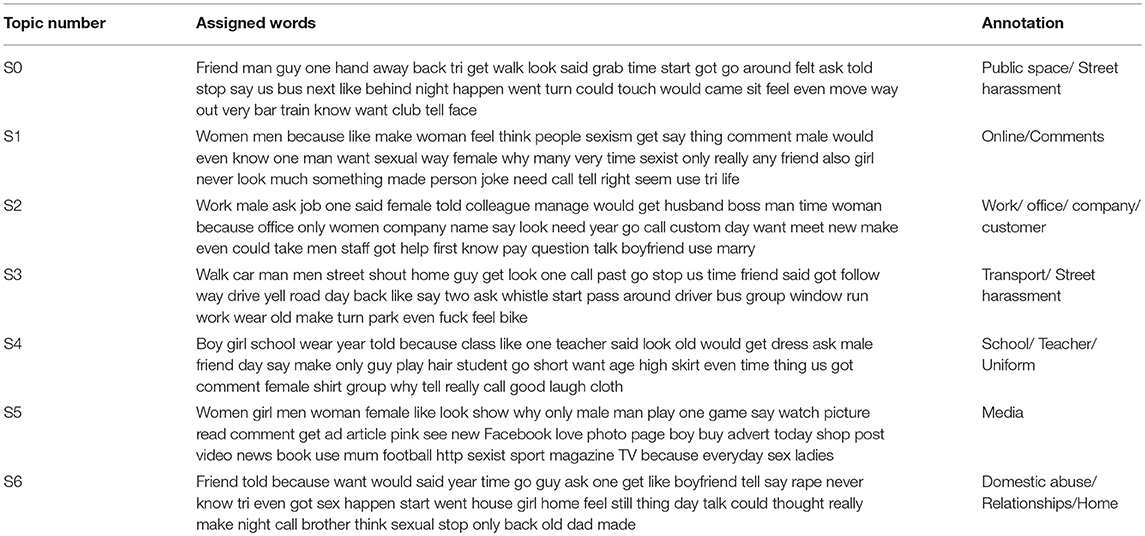
Table 1. Topics computationally extracted from the Everyday Sexism website content and annotated qualitatively for n = 7.
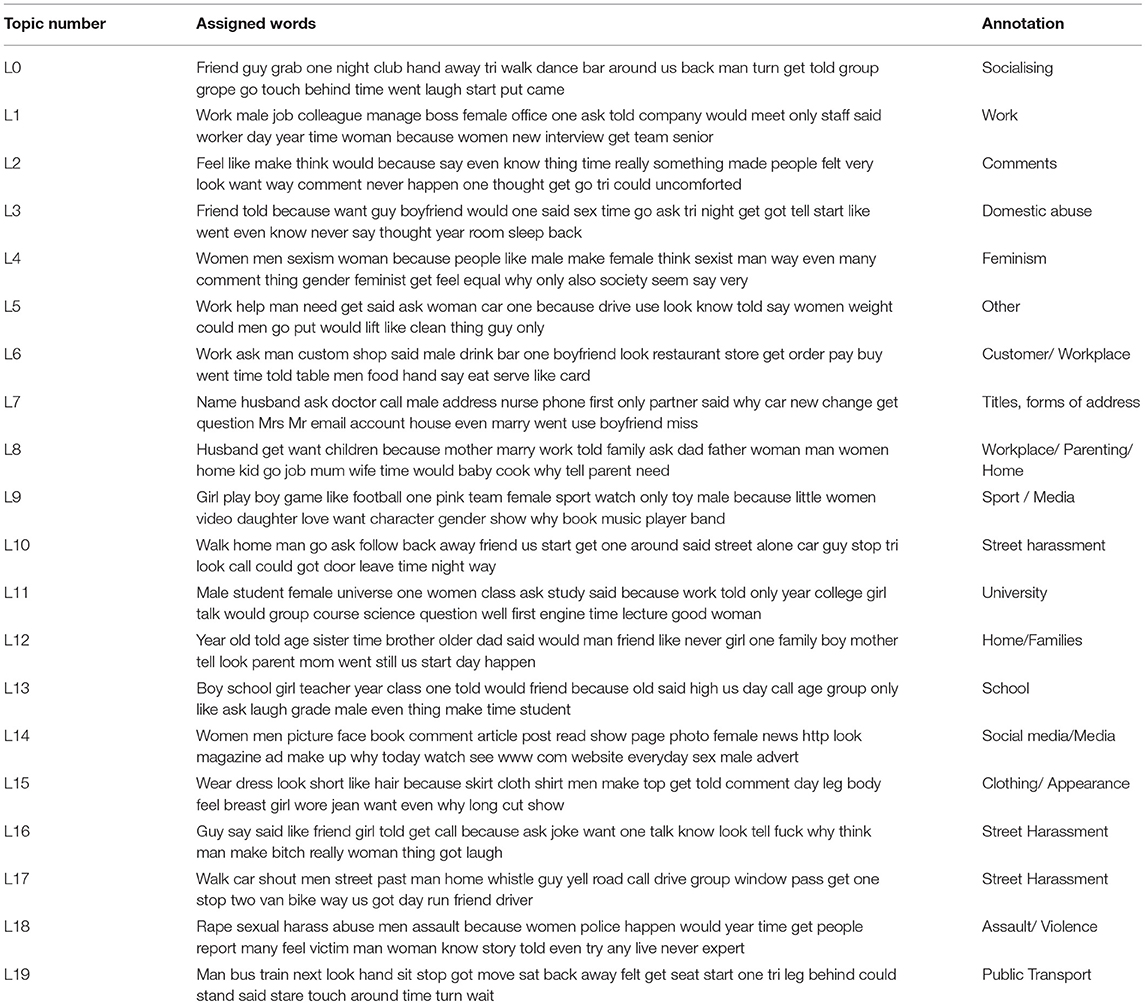
Table 2. Topics computationally extracted from the Everyday Sexism website content and annotated qualitatively for n = 20.
Figure 1 shows the number of posts that are primarily assigned to each topic for n = 7 and 20 respectively. One should note that, because of the way in which topic modeling was implemented in this work, topics would emerge with comparable sizes in terms of the number of documents assigned to them. Hence these histograms might be biased and considering the fact that the original dataset has its own natural biases of self-reported sample, the frequency analysis cannot be used to draw any conclusions.
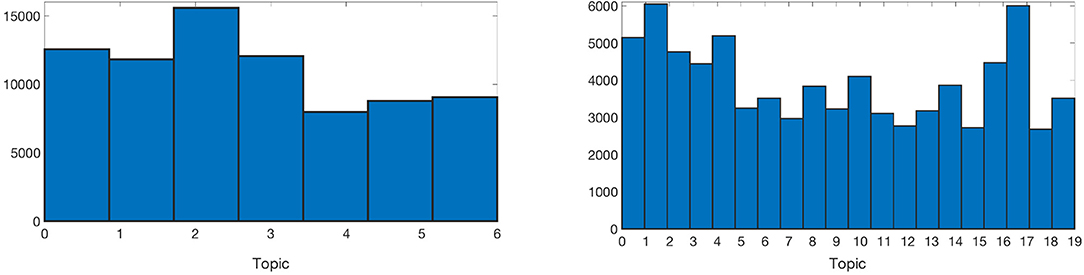
Figure 1. The number of posts that are primarily assigned to each topic for n =7 (Left) and 20 (Right).
In the next step, we consider the similarity between topics. This can be done in two ways: (1) by comparing how words are assigned to each pairs of topics and (2) by comparing how documents are assigned to each topic. The first approach is more suitable when we have smaller number of topics and hence larger overlap between the words assigned to each topic whereas the second approach can be used when there are more topics and each document is assigned to multiple topics at the same time.
We quantified these similarities by calculating the cosine similarity between the vectors of word weights and topic weights in the word-topic and topic-document matrixes. Then we used the cosine similarity as the weight of the connection between topics as depicted in Figure 2 for n = 7 and 20. In these diagrams, each node (circle) represents a topic and the edges (lines) represent the strength of the similarity between each pairs of topics.
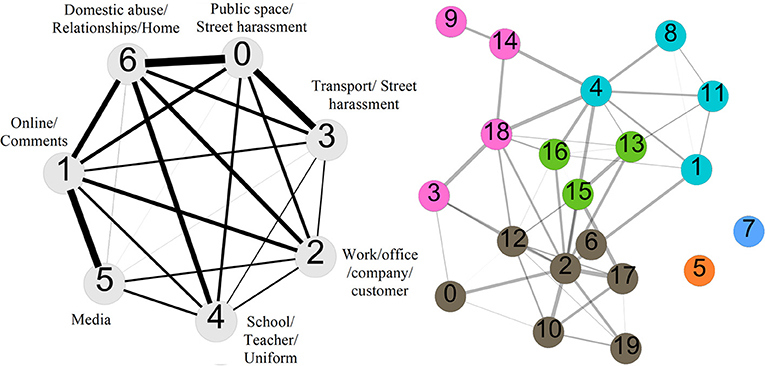
Figure 2. Network visualizations of the topics for n =7 (Left) and 20 (Right). The weight of the connections between pairs of the topics is based on the similarity of how the words are assigned to them in the left panel and how topics are assigned in the right panel. We removed connections with cosine similarities smaller than 0.2 for clarity. The color-code of the right panel is based on the communities that are detected using the Gephi implementation of the Leuven algorithm of community detection in networks.
In the case of 20 topics, we can also try to cluster topics into groups based on simple clustering algorithms in network science that group nodes of a network based on the strengths of their connections, i.e., a subset of nodes that are more densely connected between themselves compared to other nodes, would be put in the same cluster. The right panel of Figure 2 shows such grouping color-coded based on the Leuvain algorithm (Blondel et al., 2008) as implemented in Gephi (Bastian et al., 2009) with the resolution 1.0 in the settings.
Qualitative Coding
In order to shed further light on the topics and automated annotation of the posts, we also performed qualitative coding based on human judgment on a sample of posts. First, we coded a sample of 150 randomly selected posts into the 7 categories that are presented in Table 1, by two independent coders. The intercoder agreement has been calculated using a set of measures and are reported in Table 3.

Table 3. Intercoder agreement scores for a randomly selected sample of posts coded by two human coders.
This result shows a considerable agreement between independent coders that indicates the robustness of the extracted coding scheme using topic modeling. In the next step we coded a sample of 400 posts and compared the results with the categories assigned to each post by the topic model algorithm. Here we see less agreement between the computational model and the human coding. Table 4 shows the intercoder agreement scores.

Table 4. Intercoder agreement scores for a randomly selected sample of posts coded by a human coder and the topic model.
In the process of coding, we observed that some posts contain multiple stories and experiences and that potentially is a problem for the computational coding, particularly when we force the algorithm to select only one category for each post. Moreover, the context, and layered nature of the posts that are interpretable by human readers can be out of reach to the computational model. For example, the computational model might categorize a post into the category of Transport Harassment due to prevalence of words such as “bus,” “driver,” “way,” etc., whereas the human coder notices that the post is sent by a student and hence the account refers to the school bus and therefore the category of School is more appropriate. Another observation here is that the topic model works less accurately for shorter posts and where there are complicated references to concepts and use of abbreviations and specific jargons.
In order to understand the mismatch between the topic model assignments and the coding by humans, we considered the posts that are assigned to topics by the algorithm and human coder differently. This way, we built a network, which shows the overlap between topics measured by this “mismatch.” This network is shown in Figure 3.
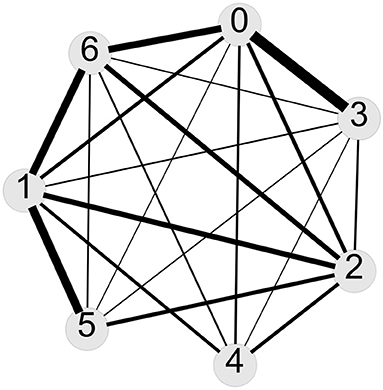
Figure 3. A representation of the mismatch between topic model assignments and human coding. The edges between topics are weighted proportional to the number of posts that are co-assigned to the corresponding topics by the human coder and the algorithm.
The similarity between the networks shown in Figure 3 and the left panel of Figure 2 clarifies the relationship between human coding and computational coding. Where, the loadings of a post to topics are less localized on one topic and the topic model detects more than one significant topic in the post (represented in the left panel of Figure 2), there is a higher chance of a mismatch between the human coding and topic model assignment (Figure 3). This often happens when we have a post that accounts for multiple experiences or reports on multifaceted stories.
Discussion and Conclusions
Analysis of the Everyday Sexism data has hitherto largely been qualitative in nature, with themes and sites associated with experiences of sexism drawn out in Bates' book, Everyday Sexism (Bates, 2015) and journalism. In her book, Bates identifies common sites of sexism drawn from the Everyday Sexism submissions, which include: Young Women Learning, Women in Public Spaces, Women in the Media, Women in the Workplace, and Motherhood (which we might also read as Women in the Home)2. More recently the Everyday Sexism website introduced a new option for tagging experiences using the following groupings: Workplace, Public Space, Home, Public Transport, School, University, Media. In the topics that emerge from our analysis of the Everyday Sexism accounts, these same areas are essentially replicated, referring in the first analysis of seven topics (Table 1) to Young Women Learning (Topic S4), Women in Public Spaces (Topics S0 and S3), Women in the Media (Topics S1 and S5), Women in the Workplace (Topic S2) and Women in the Home (Topic S6). This finding bears out the qualitative categorizations of the data set by Bates, and offers an important understanding of how topic modeling could be useful in processing and beginning to understand similar data sets that have not yet been analyzed.
One area that does not appear as a discrete category in Bates' book, or in the tags on the Everyday Sexism website, is something that we have categorized in n = 7 as “online” sexism, or “comments.” It appears in our analysis as a separate topic, S1, with the word “online” also appearing in four other topics: school, work, media and home. Although the Everyday Sexism accounts are submitted through the website, or via Twitter, the purpose of the site is to log everyday instances of sexism, both on and offline, and the majority of topics relate to offline experiences of sexism. One of the main findings from this study is that experiences of sexism, even loosely grouped in the ways that we have described, are located everywhere. They are connected and they are pervasive. The appearance of “online” as a separate topic, together with its appearance in four of the seven n = 7 topics, suggests the prevalence of sexism as mediated through digital tools, with the “online” sphere constituting a quasi-public, quasi-private “space” in which sexism can be enacted, and as a nexus through which sexist abuse enacted in other spheres can be continued and reinforced.
When we increase the number of topics to 20 (Table 2), this allows us to break down these experiences into separate but connected sites of sexism. For example, young women are clearly experiencing sexism in their learning environments, as evident in Topic S4 of our initial analysis but in the larger sample, we see sexism being experienced in both the school and University (Topics L11 and L13), areas connected by being associated with learning and with formative experiences of gender relations and expectations. The patterns of sexism experienced in the classroom at school may well pave the way for similar behavior in the lecture hall or university classroom, with the majority of words in both topics overlapping. We also see issues around gender and sport surfacing (Topic L9), with “girl,” “boy,” “football,” “sport,” and “pink” suggesting gendered notions of what constitutes appropriate forms of exercise and recreation, and reflecting the early age at which these gender stereotypes are operational (Eccles et al., 1990). Subtle differences in the ways in which these educational, professional and leisure spaces operate can be exposed by this more finely tuned analysis.
In our analysis of the larger number of topics (n = 20), work becomes a more complex setting for types of sexism, with topics L1, L5, and L8 all referring to the workplace as a site of sexism, either through “manager” “boss” or “colleague,” or through the division of domestic labor in the home, where we see “job” and “work” being juxtaposed with “mother” and “father,” “children” and “kid,” and “husband” and “wife.” In this way, we see the layering of experiences of sexism in the public sphere of work, education and business on top of sexism experienced at home, with inequalities in the workforce perhaps compounded by inequalities in the division of household and parenting tasks. The home is a hugely influential space in which children begin to witness and absorb expectations around gendered roles and behavior. Bates often refers to this as a type of “institutional sexism,” and argues that these early experiences can shape and dictate a woman's interests, activities and behavior (Bates, 2015). Topic L12 draws together a picture of sexism in the family, and points to the power of the home and familial relationships in encoding attitudes to sexism. Family relationships (“brother,” “dad,” “sister,” “mother”) are clustered with words like “would,” “start,” and “happen,” and comparative qualitative examination of the reports reinforces the ways in which formative experiences of sexism, both positive and negative, can have a tremendous impact on the way in which future encounters are experienced and articulated. A perhaps subtler experience of sexism, still within the home, is expressed in topic L7, where titles and forms of address are prevalent, reflecting the ways in which these can become “vehicles by which people establish or contest their positions within communities of practice” (Mills, 2003).
Analysis of the larger number of topics draws out numerous topics associated with what we may cluster together as street harassment, or Women in Public Spaces. Separating these topics out allows us to arrive at a more complex view of the reports that generate these clusters. Topics 10 and 17 suggest the frequency of accounts of women being verbally harassed, followed and threatened in the street while simply going about their daily lives. Topic 16 reveals the co-location of “laugh” and “joke,” with “said,” “told,” “call,” and “talk,” suggesting that this topic is dominated by accounts of street harassment which women are expected to laugh off. A qualitative examination of a random sample of reports in this topic confirmed this reading, painting a picture where sexist remarks and cat calling are often dressed up as a “joke” when challenged. The presence of “bitch” and “fuck” in this topic suggests that such interactions can often turn sour. This is a theme drawn out by Bates' qualitative reading of the accounts, and evokes the close relationship between what Glick and Fiske refer to as “benevolent sexism” and “hostile sexism,” and the way in which the former (seeking positive reinforcement) quickly becomes the latter, further reinforcing the connectivity between these different accounts (Glick and Fiske, 1996; Bates, 2015). Topic L19, which clusters “bus,” “train,” “stop,” and “seat” suggests that using public transport offers no defense against experiences of everyday sexism, with “hand,” “felt,” “leg,” “behind,” “stare,” and “touch” indicative of experiences commonly identified by victims of sexual assault. Topic L18, in which we find “rape,” “sexual harass,” “abuse,” “assault,” “police,” and “victim” creates a stark picture of the culmination of these threatening behaviors.
It is also possible to extract themes in the data through relationships between topics exposed through our analysis, shown in Figure 2. In the smaller group of topics (n = 7), the relationship strength is shown through the thickness of the connective lines. Topics S0 (Public space/Street harassment) and S3 (Transport/Street harassment) have a strong and obvious connection, as do topics S1 (Online/Comments) and S5 (Media). Other connections are superficially less clear. Topics S0 (Public space/Street harassment) and S6 (Home/Relationships), for example, are very strongly connected. While this may seem baffling at first glance, it ties in with Bates' observations of how sexism is reinforced in the home when victims of sexual harassment are subject to judgment and blame when reporting incidents to those close to them (Bates, 2015, pp. 34–41).
For the larger group of topics (n = 20), relationships are depicted through the different colored groupings (Figure 2). The groups are identified based on the strength of the connections between topics assessed through the overlap of documents co-assigned to them. This picture shows how various sub-topics are interconnected and the experience of sexism is not isolated in one shape or form. However, the two topics, L5 and L7, appear unconnected to the other topics, with no ties either strong or weak. In fact, these topics appear to be quite general, remaining both distanced from and yet relevant to other topics. In Topic L5, it is difficult to categorize this group of words into a discrete topic, as signifying words such as “work,” “drive,” “clean,” “weight,” “car” are difficult to cluster. In Topic L7, the use or misuse of appropriate titles and forms of address are experienced as everyday sexism, which may emerge across a range of backdrops. The presence of topics L5 and L7, alongside topic S1 (online/comments) in our n = 7 sample, serves to remind us that sexism can be both focused, on particular sites, roles and activities, and all encompassing, bypassing neat categorization.
What can topic modeling of the Everyday Sexism data set tell us about experiences of sexism? The topic modeling approach delivers word bags containing highly distilled elements of commonly experienced sexist encounters, creating stark pictures of interrelated sites, languages and relationships in which sexism is enacted. This analysis suggests that sexism is fluid; it's not limited to a certain space, class, culture, or time. It takes different forms and shapes but these are connected. Sexism penetrates all aspects of our lives, it can be subtle and small, and it can be violent and traumatizing, but it is rarely an isolated experience.
What does this method add to a qualitative analysis, and how can this sort of study be useful? In summary, topic modeling provides an effective means of analyzing a large data set to produce high level as well as subtler and more finely drawn themes and commonalities. Using a data set like the Everyday Sexism reports, which have already been subject to extensive qualitative analysis, allows us to test this method against qualitative findings, producing consistent results. One concern at the beginning of this project was that this method may seem reductive, producing the most common and therefore, it could be argued, most affecting or important experiences or sites of sexism. The topic modeling approach in fact offers a largely inclusive set of findings, highlighting distinct topics but visualizing connections between these topics, providing the opportunity to tease out connected but subtly different topics, which can then be contextualized by qualitative readings of the reports.
The results presented here are based on preliminary analysis, but in the future a more sophisticated approach both in the sense of methods of topic modeling and using larger and more representative datasets could potentially improve the results significantly. This could allow researchers to use computational methods to extract concepts and patterns that could then inform policy agendas.
Author Contributions
KE and TY designed the research. SM and TY performed the computational analysis. KE performed the qualitative analysis. SM, KE, and TY wrote the manuscript.
Funding
This publication arises from research funded by the John Fell Oxford University Press (OUP) Research Fund, grant number: 143/087. TY was partially supported by The Alan Turing Institute under the EPSRC grant EP/N510129/1.
Conflict of Interest Statement
The authors declare that the research was conducted in the absence of any commercial or financial relationships that could be construed as a potential conflict of interest.
Acknowledgments
We would like to thank Laura Bates for useful comments and suggestions throughout the project.
Footnotes
1. ^Cf. de Beauvoir (1949), Friedan (1963), Firestone (1971), Hartsock (1983), and Hooks (2000).
2. ^Bates is careful to include outlying, or less common yet equally relevant and important experiences of sexism. We have taken care to avoid a quantitative approach that might count and rank experiences of sexism from most common (and therefore important) to least.
References
Bastian, M., Heymann, S., and Jacomy, M. (2009). Gephi: an open source software for exploring and manipulating networks. IcwsmICWSM, 8, 361–362. doi: 10.13140/2.1.1341.1520
Bates, L. (2015). Everyday Sexism [online]. Available online at: http://everydaysexism.com (Accessed May 1, 2016).
Becker, J. C., and Swim, J. K. (2011). Seeing the Unseen: Attention to Daily Encounters with Sexism as Way to Reduce Sexist Beliefs. Psychol. Wom.Q. 35, 227–242. doi: 10.1177/0361684310397509
Becker, J. C., and Wright, S. C. (2011). Yet another dark side of chivalry: Benevolent sexism undermines and hostile sexism motivates collective action for social change. J. Person. Soc. Psychol. 101, 62–77. doi: 10.1037/a0022615
Blei, D. M., Ng, A. Y., and Jordan, M. I. (2003). Latent dirichlet allocation. J. Mach. Learn. Res. 3, 993–1022.
Blondel, V. D., Guillaume, J. L., Lambiotte, R., and Lefebvre, E. (2008). Fast unfolding of communities in large networks. J. Statist. Mech. 2008, P10008. doi: 10.1088/1742-5468/2008/10/P10008
Bonilla, T., and Grimmer, J. (2013). Elevated threat levels and decreased expectations: how democracy handles terrorist threats. Poetics 41, 650–669. doi: 10.1016/j.poetic.2013.06.003
Brandt, M. (2011). Sexism and gender inequality across 57 societies. Psychiol. Sci. 22, 1413–1418. doi: 10.1177/0956797611420445
Buchanan, N., and West, C. M. (2010). “Sexual harassment in the lives of women of color,” in Handbook of Diversity in Feminist Psychology, eds N. F. Russo and H. Landrine (New York, NY: Springer Publishing Company), 449–476.
Carstensen, T. (2009). Gender Trouble in Web 2.0.: Gender Relations in Social Network Sites, Wikis and Weblogs Gender Trouble in Web 2.0. Gender Relations in Social Network Sites, Wikis and Weblogs. Int. J. Gend. Sci. Technol. 1, 105–127.
Eccles, J. S., Jacobs, J. E., and Harold, R. D. (1990). Gender role stereotypes, expectancy effects, and parents' socialization of gender differences. J. Soc. Iss. 46, 183–201.
Femfuture (2017). Available online at: http://www.femfuture.com/why-now/ (Accessed Nov 10, 2017).
Foster, M. D. (2015). Tweeting about sexism: the well-being benefits of a social media collective action. Br. J. Soc. Psychol. 54, 629–647. doi: 10.1111/bjso.12101
Ghosh, D., and Guha, R. (2013). What are we “tweeting” about obesity? Mapping tweets with topic modeling and Geographic Information System. Cartogr. Geogr. Inform. Sci. 40, 90–102. doi: 10.1080/15230406.2013.776210
Glick, P., and Fiske, S. T. (1996). The Ambivalent Sexism Inventory: differentiating hostile and benevolent sexism. J. Person. Soc. Psychol. 70, 491–512.
Hall, D., Jurafsky, D., and Manning, C. D. (2008). “Studying the history of ideas using topic models,” in Proceedings of the Conference on Empirical Methods in Natural Language Processing - EMNLP'08, eds M. Lapata & H. T. Ng (Morristown, NJ: Association for Computational Linguistics), 363–371.
Harper, A. J. (2008). The Relationship Between Experiences of Sexism, Ambivalent Sexism, and Relationship Quality in Heterosexual Women. Auburn University.
Hartsock, N. (1983). “The feminist standpoint: developing the ground for a specifically feminist historical materialism,” in Feminism and Methodology: Social Science Issues, ed S. Harding (Bloomington, IN: Indiana University Press), 157–180.
Herring, S. (2008). “Gender and power in on-line communication,” in The Handbook of Language and Gender, eds J. Holmes and M. Meyerhoff (Oxford: Blackwell Publishers), 202–228.
Keller, J. M. (2012). Virtual Feminisms. Inform. Commun. Soc. 15, 429–447. doi: 10.1080/1369118X.2011.642890
Klein, S. (1992). Sex Equity and Sexuality in Education: Breaking the Barriers. Albany, NY: State University of New York Press.
Koltsova, O., and Koltcov, S. (2013). Mapping the public agenda with topic modeling: the case of the Russian LiveJournal. Policy Int. 5, 207–227. doi: 10.1002/1944-2866.POI331
McElwee, L., and Yasseri, T. (2017). Social Media, Money, and Politics: Campaign Finance in the 2016 US Congressional Cycle. arXiv preprint arXiv:1711.10380.
McRobbie, A. (2009). The Aftermath of Feminism: Gender, Culture and Social Change. London: SAGE Publications.
Mills, S. (2003). Caught between sexism, anti-sexism and ‘political correctness’: feminist women's negotiations with naming practices. Discour. Soc. 14, 87–110. doi: 10.1177/0957926503014001931
Moradi, B., and Subich, L. M. (2002). Perceived sexist events and feminist identity development attitudes: links to women's psychological distress. Counsel. Psychol. 30, 44–65. doi: 10.1177/0011000002301003
OED Online (2018). Oxford University Press. Available online at: http://www.oed.com/viewdictionaryentry/Entry/11125 (Accessed December 31, 2018).
Ossana, S., Helms, J., and Leonard, M. (1992). Do “womanist” identity attitudes influence college women's self-esteem and perceptions of environmental bias? J. Counsel. Develop. 70, 402–408. doi: 10.1002/j.1556-6676.1992.tb01624.x
Paul, M. J., and Dredze, M. (2014). Discovering health topics in social media using topic models. PLoS ONE 9:e103408. doi: 10.1371/journal.pone.0103408
Pritchard, J. K., Stephens, M., and Donnelly, P. (2000). Inference of population structure using multilocus genotype data. Genetics 155, 945–959.
Puschmann, C., and Scheffler, T. (2016). Topic Modeling for Media and Communication Research: a Short Primer. HIIG Discussion Paper Series No. 2016-05. Available online at: https://ssrn.com/abstract=2836478
Sabik, N. J., and Tylka, T. L. (2006). Do feminist identity styles moderate the relation between perceived sexist events and disordered eating? Psychol. Wom. Q. 30, 77–84. doi: 10.1111/j.1471-6402.2006.00264.x
Swim, J. K., Hyers, L. L., Cohen, L. L., and Ferguson, M. J. (2001). Everyday sexism: evidence for its incidence, nature, and psychological impact from three daily diary studies. J. Soc. Issues 57, 31–53. doi: 10.1111/0022-4537.00200
Wallach, H. M., Mimno, D. M., and McCallum, A. (2009). “Rethinking LDA: why priors matter,” in Advances in Neural Information Processing Systems, 1973–1981.
Watkins, M. B., Kaplan, S., Brief, A. P., Shull, A., Dietze, J., Mansfield, M.-T., et al. (2006). Does it pay to be sexist? The relationship between modern sexism and career outcomes. J. Vocat. Behav. 69, 524–537. doi: 10.1016/j.jvb.2006.07.004
Keywords: sexism, gender, everyday sexism, topic modeling, content analysis
Citation: Melville S, Eccles K and Yasseri T (2019) Topic Modeling of Everyday Sexism Project Entries. Front. Digit. Humanit. 5:28. doi: 10.3389/fdigh.2018.00028
Received: 24 November 2017; Accepted: 20 December 2018;
Published: 22 January 2019.
Edited by:
Tom Crick, Swansea University, United KingdomReviewed by:
Jonathan Gillard, Cardiff University, United KingdomJudy Robertson, University of Edinburgh, United Kingdom
Copyright © 2019 Melville, Eccles and Yasseri. This is an open-access article distributed under the terms of the Creative Commons Attribution License (CC BY). The use, distribution or reproduction in other forums is permitted, provided the original author(s) and the copyright owner(s) are credited and that the original publication in this journal is cited, in accordance with accepted academic practice. No use, distribution or reproduction is permitted which does not comply with these terms.
*Correspondence: Taha Yasseri, dGFoYS55YXNzZXJpQG9paS5veC5hYy51aw==