- 1Department of Psychiatry, National Clinical Research Center for Mental Disorders, The Second Xiangya Hospital of Central South University, Changsha, Hunan, China
- 2Department of Psychiatry, First Hospital of Shanxi Medical University, Taiyuan, China
- 3CAS Key Laboratory of Mental Health, Institute of Psychology, Chinese Academy of Sciences, Beijing, China
- 4Department of Psychology, University of Chinese Academy of Sciences, Beijing, China
Aims: Thyroid dysfunction and metabolic disturbances are common in major depressive disorder (MDD) patients. We aimed to assess the relationship between thyroid dysfunction, metabolic disturbances, and clinical symptoms in Chinese first-episode, drug-naïve (FEDN) MDD patients using undirected and Bayesian network methods.
Methods: 1718 FEDN MDD patients were recruited. Serum levels of free triiodothyronine (FT3), free thyroxine (FT4), thyroid stimulating hormone (TSH), anti-thyroglobulin (TgAb), thyroid peroxidases antibody (TPOAb), total cholesterol (TC), total triglycerides (TG), high-density lipoprotein (HDL-C), low-density lipoprotein (LDL-C), and glucose were assessed. Blood pressure and body mass index were measured. Hamilton Rating Scale for Depression (HAMD), Hamilton Rating Scale for Anxiety, and positive subscale of Positive And Negative Syndrome Scales were used to detect clinical symptoms. An undirected network with EBICglasso default and a directed acyclic graph (DAG) using the Bayesian network approach was conducted.
Results: The prevalence rates of clinical symptoms, thyroid dysfunction, and metabolic dysfunction were as follows: anxiety (n=894, 52%), psychotic symptoms (171, 10%), subclinical hypothyroidism (SCH, n=1041, 61%), abnormal TgAb (n=297, 17%), abnormal TPOAb (n=438, 25%), hyperthyroidism (n=5, 0.3%), hypothyroidism (n=3, 0.2%), hyperglycemia (n=241, 14%), hypertriglyceridemia (n=668, 39%), low HDL-C (n=429, 25%), hypercholesterolemia (421, 25%), abnormal TC (357, 21%), abnormal LDL-C (185, 11%). overweight or obesity (n=1026, 60%), and hypertension (n=92, 5.4%). Both networks demonstrated serum TSH and TC levels and the severity of depression played an important role in the pathophysiology of MDD.
Conclusions: MDD patients may have thyroid and metabolic dysfunction in the early stage. Targeting hypercholesterolemia, depressive symptoms, and SCH in MDD patients may hold promise in reducing clinical symptoms, metabolic disturbances, and thyroid dysfunction.
1 Introduction
Major depressive disorder (MDD) is the most common and severe psychiatric disorder, which leads to a significant impact on quality of life and functioning (1, 2). It is the leading cause of disability-adjusted life years in adults and affects approximately 300 million people worldwide (3). Emerging studies have demonstrated biological changes in MDD patients, such as impaired glucose and lipid metabolism, thyroid dysfunction, and obesity (4–6). Previous studies have found that metabolic disorders and/or thyroid dysfunction increase the risk of MDD (4, 7, 8). More importantly, a growing number of studies have shown that metabolic disorders and thyroid dysfunction are positively associated with poor response to antidepressants (9), a higher risk of readmission (10), and more severe comorbidities such as suicide (11) and anxiety (12). Taken together, these findings highlight the important role of metabolic disorders and thyroid dysfunction in the pathophysiology of MDD.
Despite the great interest in the abnormalities of metabolism and thyroid function in MDD patients, there are some important limitations of the current evidence. First, most prior studies have had relatively limited sample sizes and have ignored potential confounding factors such as antidepressants and disease courses (13, 14). Second, although studies in the general population have demonstrated substantial associations between metabolic disorders and thyroid dysfunction (15–19), they are most often studied alone in patients with MDD. Clarifying whether and how metabolic disorders, thyroid disorders, and clinical symptoms reinforce and interact with each other in patients with MDD will deepen our understanding of the pathophysiology of MDD.
Network analysis is a promising tool for understanding the associations between variables (20, 21). In the network model, each variable is represented as a “node”. The edges represent the unique association between two variables after adjusting for the rest of the variables within the network. Through network analysis, we can estimate the interaction between thyroid dysfunction, metabolic disturbance, and clinical symptoms in MDD patients in more detail. It allows us to identify the most influential variables in the network, which may serve as the target for clinical interventions (20).
To date, network approaches have been used to identify associations between pro-inflammatory proteins, lipid markers, genetic factors, and depressive symptoms in patients with MDD (22, 23). However, no previous studies have assessed networks of thyroid dysfunction, metabolic disorders, and clinical symptoms in patients with MDD. To fill this gap, we conducted the present study. We recruited a large sample of first-episode drug-naïve (FEDN) patients with MDD, which minimized the impact of medical therapy, disease duration, and comorbidities. We conducted two networks. The first network was undirected and was used to explore the most relevant connections and core variables in the thyroid-metabolism-clinical network. For the second network, we performed a Bayesian network analysis to obtain directions of potential causal relationships between thyroid dysfunction, metabolic disorders, and clinical symptoms.
2 Materials and methods
2.1 Study setting and procedure
FEDN MDD outpatients were recruited during 2015-2017 at the Department of Psychiatry, First Hospital, Shanxi Medical University. Participants should meet the following criteria: (1) diagnosis of MDD by two independently trained clinical psychiatrists according to the Structured Clinical Interview for DSM-IV (SCID); (2) 17-item Hamilton Rating Scale for Depression (HAMD) total score ≥ 24; (3) age between 18-60 years old and Han Chinese; (4) depressive symptoms were first-episode without any prior medications, including antidepressant, antipsychotic, or thyroid treatment medication; and (4) MDD of disease duration was no more than 24 months.
Exclusion criteria included: (1) the presence of any other major DSM-IV Axis I disorder based on SCID; (2) the presence of serious physical illness, such as organic brain disease or severe infection; (3) any substance abuse or dependence other than tobacco; (3) pregnant or lactating women; and (4) refusal to give informed consent.
The work described has been carried out in accordance with The Code of Ethics of the World Medical Association (Declaration of Helsinki). The study was approved by the Institutional Review Board (IRB) of the First Hospital of Shanxi Medical University (No. 2016-Y27). All participants gave their written informed consent.
2.2 Measurements
2.2.1 Basic information
The demographic information including gender, age, married status, education level, age at first episode onset, and duration of MDD courses were collected through self-administrated questionnaires.
2.2.2 Clinical assessments
Two trained psychiatrists independently administrated the SCID to each participant. They assessed depression, anxiety, and psychotic symptoms using the Chinese version of the HAMD, the Hamilton Anxiety Rating Scale (HAMA), and the Positive and Negative Symptom Scale (PANSS) positive subscale, respectively (24, 25). Following the previous study (26), patients were divided into the without or possible anxiety group (HAMA scores 0-13), mild to moderate anxiety group (HAMA scores 14-20), significant anxiety group (HAMA scores 21-28), and severe anxiety group (HAMA scores >28) based on the HAMA scores. A cutoff point of 15 was used to determine the presence of psychotic symptoms (27). The inter-observer correlation coefficients of the scores on the three scales were >0.8.
2.2.3 Thyroid function and metabolic parameters
Before participants received any medication, their fasting blood samples were collected between 6 am and 8 am. Serum levels of the following biochemical parameters were assessed: free triiodothyronine (FT3), free thyroxine (FT4), thyroid stimulating hormone (TSH), anti-thyroglobulin (TgAb), thyroid peroxidases antibody (TPOAb), total cholesterol (TC), total triglycerides (TG), high-density lipoprotein (HDL-C), low-density lipoprotein (LDL-C), and fasting glucose. Thyroid hormones (TSH, TPOAb, TgAb, FT3, and FT4) were measured on a Roche C6000 Electrochemiluminescence Immunoassay Analyzer (Roche Diagnostics, Indianapolis, IN, USA), while metabolic parameters (HDL-C, LDL-C, TC, TG, and glucose) were assessed on a Cobas E610 (Roche, Basel, Switzerland) in the laboratory of Shanxi Medical University. The blood pressure, height, and weight were taken by trained nurses. Body mass index (BMI) was calculated with the equation: BMI= Weight (kg)/Height (m)2. The definition of metabolic disturbances and thyroid dysfunction were as follows: (1) overweight or obesity: BMI≥24; (2) hyperglycemia: glucose≥6.1mmol/L; (3) hypertension: SBP≥140 mmHg and/or DBP≥90mmHg; (4) hypertriglyceridemia: TG≥2.3 mmol/L; (5) low HDL: HDL-C ≤ 1.0 mmol/L; (6) hypercholesterolemia: TC≥6.2 mmol/L or LDL-C≥4.1 mmol/L; (7)abnormal TgAb: TgAb≥115 IU/L; (8) abnormal TPOAb: TPOAb ≥34 IU/L; (9) subclinical hypothyroidism (SCH): TSH >4.2 mIU/L with a normal fT4 concentration (10–23 pmol/L); (10) hyperthyroidism: TSH<0.27 mIU/L and FT4 over 23 pmol/L, and (11) hypothyroidism: TSH >4.2 mIU/L with a low FT4 concentration (<10 pmol/L).
2.3 Statistical analysis
According to the Shapiro-Wilk test, the continuous variables in this study were not normally distributed. Therefore, we denoted continuous variables as the median and interquartile range (IRQ; 25-75%) and categorical variables as frequencies and percentages. All statistical analyses were performed on R(ver.4.20). We conducted the partial correlation test to evaluate the association between clinical symptoms (HAMA, HAMD, PANSS), thyroid hormones (TPOAb, TgAb, TSH, FT3, FT4), and metabolic parameters (HDL-C, LDL-C, TC, TG, glucose, BMI, SBP, and DBP). Basic information including age, age at first episode onset, illness duration, gender, education, and married status were controlled as covariates.
2.4 Undirected network analysis
Thyroid hormones, metabolic parameters, and clinical symptoms were included in the network analyses. Following previous studies, we converted the data set to normal using a non-normal transformation through the R package “huge” (28). The network was estimated using the EBICglasso model, which is the most widely used model in psychopathology networks (29, 30). The R packages “qgraph” and “bootnet” were applied for the visualization of the network. In the network, each “node” represented one item of thyroid hormones, metabolic parameters, and clinical information. After adjusting the other nodes in the network, the unique connection between two nodes is visualized as an “edge” between the nodes. The thickness and color of the edges indicate the strength and direction of the relationship. The thicker the edge, the stronger the association. Red and blue edges represent negative and positive associations, respectively.
We calculate the centrality index “strength” to identify the central nodes in the network. The strength is the sum of the absolute edge weights that directly connect a node to the other nodes in the network. Nodes with the highest strength are considered central nodes, and they have the strongest influence on other variables in the network. Central nodes are important in the creation of networks (31–33) and can be used as potential targets for clinical interventions. We also assessed the “predictability” of each node by Rpackage “MGM”. Similar to the adjusted R2 in regression models, the predictability of a node indicates the extent to which the variance of a node can be predicted by other nodes in the network (34). Nodes with high predictability might be easily changed by changing their related nodes.
The case-dropping procedure was applied to evaluate the stability of our network. The correlation stability coefficient (CS-C) of node strength was calculated, with CS-C above 0.5 implying high stability. To test the accuracy of edges estimated within the network, we conducted the nonparametric bootstrapping with 1000 bootstrap samples.
We used a case-dropping procedure to assess the stability of our network. The correlation stability coefficient (CS-C) of the node strength was calculated, and a CS-C above 0.5 indicated high stability. To test the accuracy of the estimated edges within the network, we performed nonparametric bootstrapping with 1000 bootstrap samples.
We further compared our networks with the Rpackage “Network Comparison Tool”. Three subgroups were analyzed including gender (female versus male), illness duration (≤5 months versus >5 months), and age (18-45 years old versus 46-60 years old). The overall strength (absolute sum value of all edge weights) and network structure (distribution of edge weights) were evaluated between subgroups of networks.
2.5 Bayesian network analysis
Finally, we performed directed acyclic graphs (DAG) to assess the putative direction of causal relationships between thyroid dysfunction, metabolic disorders, and clinical symptoms. DAG is an emerging method for network analysis. It allows us to detect and represent the most likely direction of causality between variables based on the conditional dependence between each pair of variables in the presence of other variables in the network (35, 36). More importantly, studies have shown that variables at the top of the DAG may have a higher predictive priority and more salience and should receive higher priority in treatment (37, 38). Following previous studies (36, 39), we performed DAG by the R package “bnlearn”. A hill-climbing algorithm was chosen to estimate the network. According to the protocol of Scutari M & Nagarajan R (40), the optimal cut point method was used to retain edges with high sensitivity and specificity.
3 Result
3.1 Sample characteristics
A total of 1718 patients (female: 1130, male: 588) with FEDN MDD were recruited (Table 1). The median age at the first episode onset was 34 (23, 41) years. 43% (n=740), 32% (n=549), and 25% (n=429) of the participants were between 18-30, 31-45, and 46-60 years old, respectively. 171 (10%) suffered from psychotic symptoms. According to HAMA scores, the participants could be divided into without or possible anxiety (n=1, 0.1%), mild to moderate anxiety (n=853, 47.9%), significant anxiety (n=849, 49.4%), and severe anxiety (n=45, 2.6%). The prevalence of thyroid dysfunction and metabolic disorders was as follows: SCH (n=1041, 61%), TgAb abnormalities (n=297, 17%), TPOAb abnormalities (n=438, 25%), hyperthyroidism (n=5, 0.3%), hypothyroidism (n=3, 0. 2%), hyperglycemia (n=241, 14%), hypertriglyceridemia (n=668, 39%)), low HDL-C (n=429, 25%), hypercholesterolemia (421, 25%), abnormal TC (357, 21%), abnormal LDL-C (185, 11%), overweight or obese (n=1026, 60%) and hypertensive (n=92, 5.4%).
3.2 Correlation between thyroid dysfunction, metabolic disturbances, and clinical symptoms
We found that clinical symptoms, metabolic disturbances, and thyroid dysfunction were strongly correlated after adjusting for basic information (Table S1). Specifically, the partial correlation test demonstrated substantial inter-relationships between three clinical symptoms: HAMA-HAMD (r=0.615), HAMD-PANSS (r=0.539), and HAMA-PANSS (r=0.610) (all p<0.001). Notably, TSH levels were strongly and positively associated with HAMD (r=0.466), HAMA (r=0.344), PANSS (r=0.367), glucose (r=0.444), TC (r=0.545), SBP (r=0.551), and DBP (r=0.356) (all p<0.001). We also found a solid positive relationship between TC and HAMD (r=0.553, p<0.001).
3.3 Undirected network of thyroid dysfunction, metabolic disturbance, and clinical symptoms in FEDN MDD patients
Figure 1 illustrates the network of thyroid dysfunction, metabolic disorders, and clinical symptoms in patients with FEND MDD. The network consists of 16 nodes with a density of 0.44 (53/120). Visually, the node TSH is located in the center of the network. It shows a strong relationship with metabolic disorders (i.e. SBP, Glucose, and TC). It was also positively correlated with HAMA, HAMD, and PANSS. We also observed a strong relationship between HAMA, HAMD, and PANSS. The ten strongest edges in the network were SBP-DBP, followed by TPOAb-TgAb, TC-LDL-C, HAMD-PANSS, HAMA-PANSS, TSH-Glucose, HAMD-HAMA, HAMD-TC, TSH-TC, and TSH-TC. According to the results of the nonparametric bootstrap procedure, these edges were statistically stronger than the other edges within the network (Figure S1). The value of the edges is listed in Table S2.
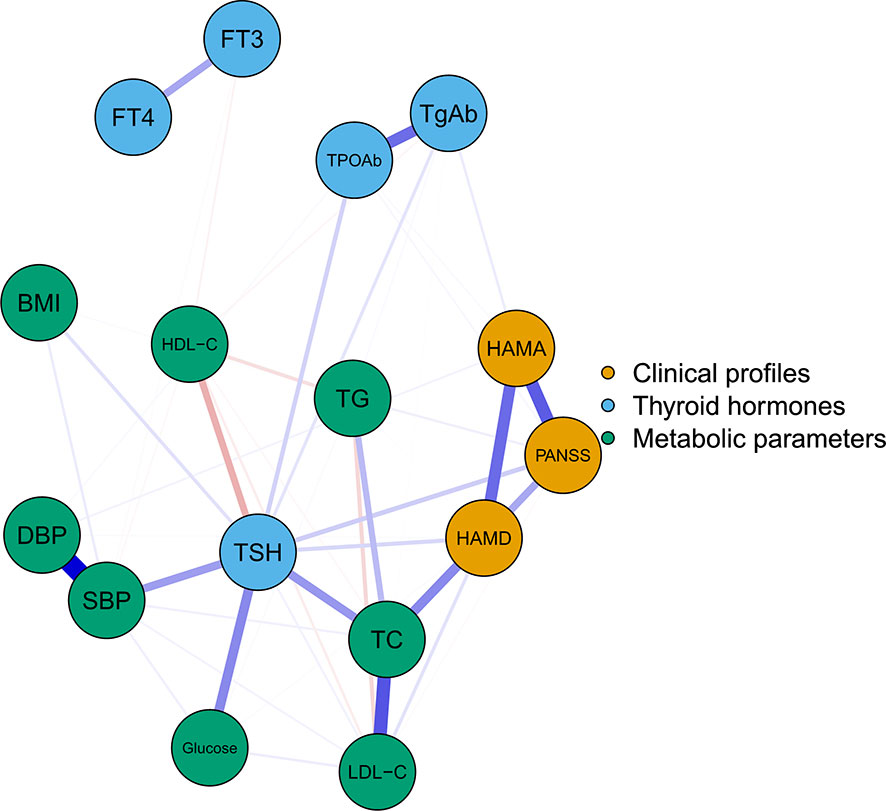
Figure 1 The undirected network of thyroid hormones, metabolic parameters, and clinical symptoms The blue, green, and orange nodes represent thyroid hormones, metabolic parameters, and clinical profiles, respectively. Blue edges indicate positive association while red edges indicate negative association. Thicker edge implies a stronger association. HAMD, Hamilton Depression Rating Scale; HAMA, Hamilton Anxiety Rating Scale; PANSS, the Positive and Negative Syndrome Scale; TSH, thyroid-stimulating hormone; FT3, free triiodothyronine; FT4, free thyroxine; TgAb, antithyroglobulin; TPOAb, thyroid peroxidases antibody; TC, total cholesterol; HDL-C, high-density lipoprotein; LDL-C, low-density lipoprotein; TG, total triglycerides; BMI, body mass index.
The centrality plot (Figure 2) shows that TSH, TC, and HAMD scores have the highest strength, implying that they are the central nodes in the network. They have a statistically higher strength than the other nodes (Figure S2). The predictability of the nodes within the network is shown in Table S3. Notably, the predictability of HAMD was 0.604, HAMA was 0.456, and PANSS was 0.516, indicating that half of the severity of depression, anxiety, and psychotic symptoms could be explained by the other nodes in the network.
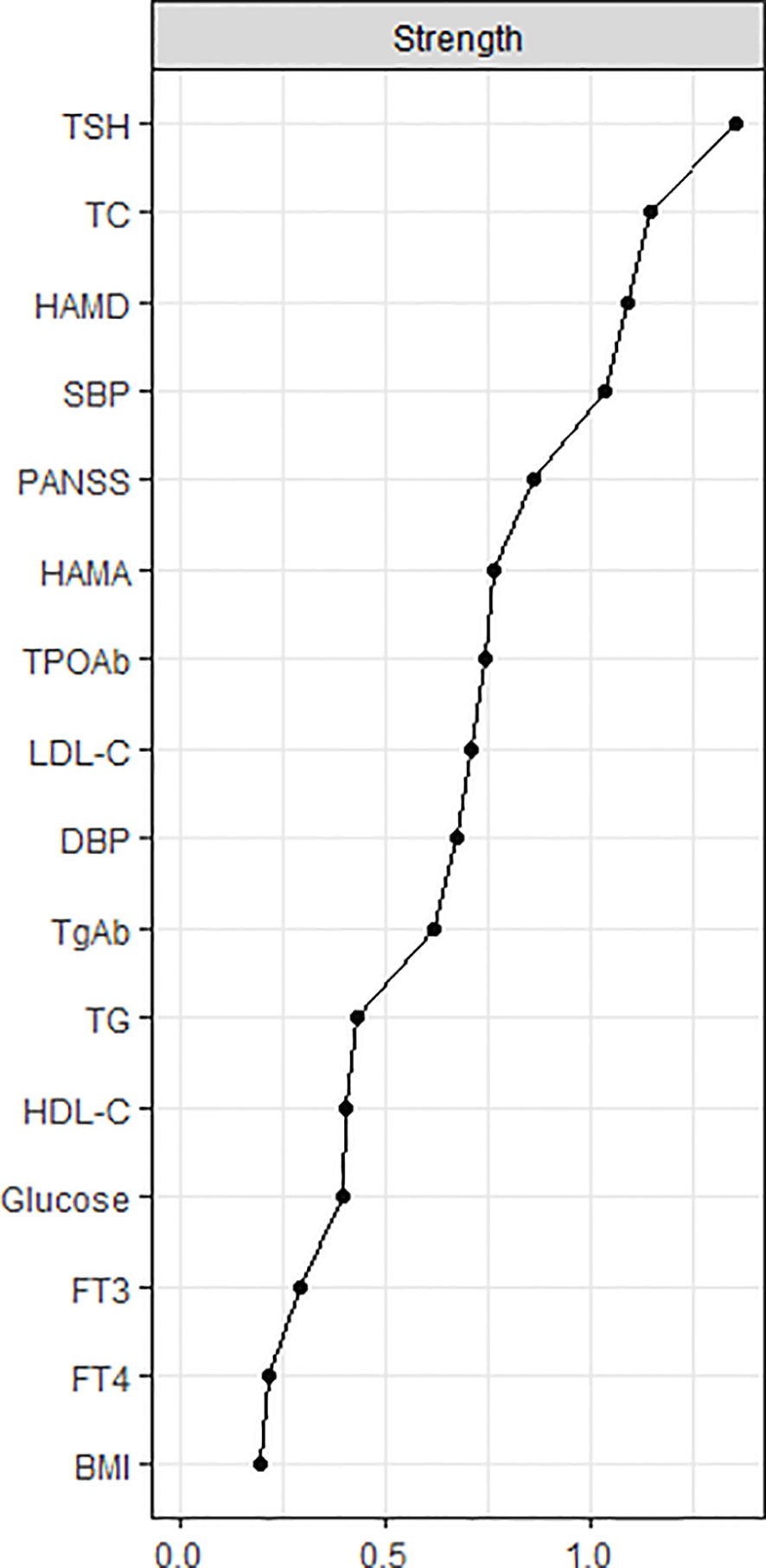
Figure 2 The central nodes of the network. The X-rays represented the strength of each node. Nodes with higher strength have stronger impact in other nodes within the network. HAMD, Hamilton Depression Rating Scale; HAMA, Hamilton Anxiety Rating Scale; PANSS, the Positive and Negative Syndrome Scale; TSH, thyroid-stimulating hormone; FT3, free triiodothyronine; FT4, free thyroxine; TgAb, antithyroglobulin; TPOAb, thyroid peroxidases antibody; TC, total cholesterol; HDL-C, high-density lipoprotein; LDL-C, low-density lipoprotein; TG, total triglycerides; BMI, body mass index.
The network had good stability and accuracy (Figures S3, S4). The CS-C was 0.75, indicating that the network was highly correlated with the original network even after discarding 75% of the original data (r=0.7).
3.4 Network comparison test
We compared the networks of thyroid dysfunction, metabolic disorders, and clinical symptoms according to gender, age, and illness duration (Figure S5). We did not observe any differences in the overall strength and network structure of the networks between the three subgroups.
3.5 Bayesian network analysis
Figure 3 illustrates the DAG of thyroid disorders, metabolic disorders, and clinical symptoms in patients with FEDN MDD. Consistent with the undirected network analysis, HAMD, TSH, and TC were located at the top of the DAG, indicating that they may trigger and maintain metabolic disorders, thyroid dysfunction, and clinical symptoms in patients with FEDN MDD. Similarly, we found that TSH was closely associated with metabolic disorders. It was upstream of glucose disturbance, hypertension, obesity, and HDL-C. The DAG also suggests that TSH may contribute to more severe psychotic symptoms.
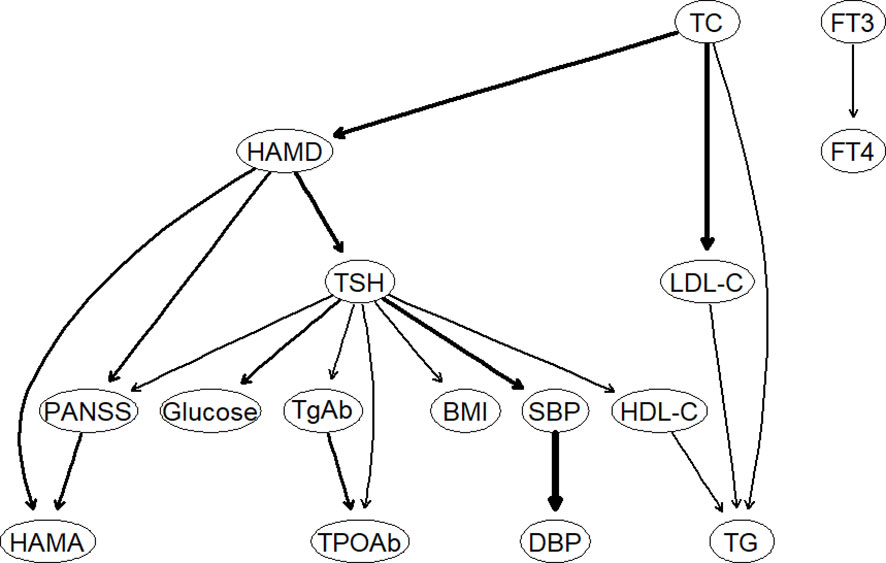
Figure 3 The Bayesian network of thyroid hormones, metabolic parameters, and clinical symptoms. HAMD, Hamilton Depression Rating Scale; HAMA, Hamilton Anxiety Rating Scale; PANSS, the Positive and Negative Syndrome Scale; TSH, thyroid-stimulating hormone; FT3, free triiodothyronine; FT4, free thyroxine; TgAb, antithyroglobulin; TPOAb, thyroid peroxidases antibody; TC, total cholesterol; HDL-C, high-density lipoprotein; LDL-C, low-density lipoprotein; TG, total triglycerides; BMI, body mass index.
4 Discussion
To our knowledge, this is the first study to describe the relationship between thyroid dysfunction, metabolic disorders, and clinical symptoms in a large sample of FEDN MDD patients by a network approach. Our study highlights the common metabolic burden and thyroid dysfunction in patients with MDD. Both the undirected network and DAG showed a dominant role of TC, TSH, and depression severity in patients with MDD. Therefore, targeting abnormal TSH, TC, and depressive symptoms may hold great promise for reducing MDD-related thyroid dysfunction, metabolic disturbances, and clinical symptoms.
Our study suggested high TSH levels (i.e., SCH) played a vital role in triggering metabolic disturbances and clinical symptoms in FEDN MDD patients. In our study, the prevalence of SCH in our sample was 61%, much higher than that in the Chinese general population (range: 3.4%-12.93%) (42–44). More importantly, we found that TSH levels were highly correlated with the severity of depressive symptoms in FEDN MDD patients. The shared biological mechanisms between MDD and SCH, such as disturbances in the hypothalamic–pituitary–adrenal axis and changes in the hormone levels including somatostatin and serotonin might contribute to their tight associations (45). In recent years, studies also indicated that the Wnt/β-catenin pathway might play a role in the association between SCH and depression. Interestingly, the DAG indicated that the TSH levels might be the outcome rather than a cause of depressive symptoms in MDD. However, there were very few, if any, longitudinal studies that evaluated whether depressive symptoms could predict increased TSH levels. Further studies are needed to validate our findings.
In addition to depressive symptoms, we demonstrated a strong positive correlation between TSH levels and psychotic symptoms in patients with FEDN MDD. To date, only a few studies have evaluated their relationship and have yielded inconsistent results. For example, Liu et al. found that serum TSH levels were independently associated with psychotic symptoms in 1279 patients with MDD (11). Another large study of 1410 patients with MDD showed that hypothyroidism was positively associated with psychotic symptoms (41). However, Contreras et al. found no difference in TSH blunting in MDD patients with and without psychotic symptoms (46). A possible explanation might be the complicated medication in the treatment of MDD and the varied sample size, which might impact the association between psychotic symptoms and TSH levels. Furthermore, most of the current evidence on the association between TSH and psychosis came from the observational cross-sectional study. The underlying mechanism is still largely unexplored. Hence, further studies were still needed to confirm our findings.
Consistent with previous studies in the general population (18, 47), high TSH levels exhibited substantial associations with impaired metabolism, including hypertension, hyperglycemia, obesity, and hypercholesterolemia. To date, only a few studies have investigated their relationship in the context of MDD (48, 49), and these studies reported similar results to ours. For example, Kim et al. found that SCH increased the risk of metabolic syndrome (MetS) approximately 7-fold in Korean adults with depression (48). Zhao et al. found that SCH was independently associated with BMI, TC, and LDL-C in MDD patients (49). A few possible explanations might account for the high co-occurrence of SCH and metabolic disturbances. First, studies have suggested that high TSH levels could directly impact lipid metabolism by binding the TSH receptor (50). High TSH levels could promote cholesterol synthesis and inhibit cholesterol clearance, which is independent of FT3 and FT4 (50). Second, recent studies suggested that hepatic endoplasmic reticulum stress induced by SCH played an important role in dyslipidemia and impaired glucose metabolism (51, 52). Interestingly, a strong association between SCH and metabolic disorders has been reported in patients with other psychiatric disorders. For example, a recent study showed higher serum TSH levels in schizophrenia patients with comorbid MetS (53). Taken together, the co-occurrence of SCH and metabolic disorders may be common in psychiatric patients, which calls for regular screening of this population.
Further, we found that high TC levels were another important factor in the network of thyroid dysfunction, clinical symptoms, and metabolic disorders in patients with FEDN MDD. Notably, it was highly correlated with the severity of depression in this study, which is consistent with some previous studies (54–56). There are several explanations for their strong association. First, MDD patients with more severe depressive symptoms may have a more irregular lifestyle, such as late nights and an unhealthy diet, which leads to increased TC levels. Second, inflammation may act as a bridge between hypercholesterolemia and MDD. Hypercholesterolemia has been found to trigger the activation of the NLRP3 inflammasome, which leads to a chronic state of inflammation (57). A growing number of studies suggest a bidirectional relationship between MDD and inflammation (58, 59). However, we did not collect the variables associated with inflammation. Therefore, further studies are needed to test our hypothesis.
The present study has several important clinical implications. First, our study highlights the heavy metabolic burden and thyroid dysfunction in patients with FEDN MDD, which calls for regular screening of this population. Second, we found that greater depressive symptoms, higher TSH levels, and higher TC levels may play an important role in metabolic disturbances, thyroid dysfunction, and clinical symptoms, which should be given higher priority in treatment. Several clinical trials have demonstrated the potential effectiveness of anti-SCH and anti-hypercholesterolemia in the treatment of MDD (60–62), which supported our findings. For example, several studies have demonstrated the efficacy and safety of statins (one of the most widely used drugs for the treatment of hypercholesterolemia) in MDD (60, 61). A recent review suggests that thyroid hormone therapy may be a promising strategy for refractory MDD (62). Thyroid hormone therapy was also found to improve lipid metabolism in SCH patients (63). However, it should be noted that the results of thyroid hormone therapy in the treatment of MDD are inconsistent (64, 65), which warranted further studies.
The study has the following limitations. First, it should be acknowledged that the study was observational and cross-sectional, which inhibited us from drawing a causal relationship between clinical symptoms, metabolic disturbances, and thyroid dysfunction among patients with MDD. Second, despite the large sample size, this study was conducted in a single hospital. Whether our findings can be generalized to other populations remains unclear. Third, we did not control for several important potential confounders, such as diet, smoking, drinking, and pro-inflammatory factors. Therefore, further longitudinal studies which provide a more comprehensive assessment of lifestyle factors are needed to validate our findings.
In summary, this study described the association between thyroid dysfunction, metabolic disorders, and clinical symptoms in a large sample of FEDN MDD patients by both undirected and Bayesian network approaches. Both networks suggest that TSH, TC, and depression severity are of high importance in patients with MDD and should be treated with high priority.
Data availability statement
The raw data supporting the conclusions of this article will be made available by the authors, without undue reservation.
Ethics statement
The studies involving human participants were reviewed and approved by Institutional Review Board (IRB) of the First Hospital of Shanxi Medical University. The patients/participants provided their written informed consent to participate in this study.
Author contributions
PP: Formal analysis, Writing - original draft. QW: Writing – review & editing. XL: Writing – review & editing. TL: Conceptualization, Writing–review & editing. X-YZ: Conceptualization, Writing–review & editing. All authors contributed to the article and approved the submitted version.
Funding
This study was supported by the Provincial Natural Science Foundation of Hunan (Grant No. 2020JJ4795 to Tieqiao Liu). These sources had no further role in this study design, in the data collection and analysis, in the writing of the report, and in the decision to submit the paper for publication.
Acknowledgments
We would like to acknowledge all the participants.
Conflict of interest
The authors declare that the research was conducted in the absence of any commercial or financial relationships that could be construed as a potential conflict of interest.
Publisher’s note
All claims expressed in this article are solely those of the authors and do not necessarily represent those of their affiliated organizations, or those of the publisher, the editors and the reviewers. Any product that may be evaluated in this article, or claim that may be made by its manufacturer, is not guaranteed or endorsed by the publisher.
Supplementary material
The Supplementary Material for this article can be found online at: https://www.frontiersin.org/articles/10.3389/fendo.2023.1138233/full#supplementary-material
References
2. Otte C, Gold SM, Penninx BW, Pariante CM, Etkin A, Fava M, et al. Major depressive disorder. Nat Rev Dis Primers (2016) 2:16066. doi: 10.1038/nrdp.2016.66
3. GBD 2019 Diseases and Injuries Collaborators. GBD 2019 diseases and injuries collaborators. global burden of 369 diseases and injuries in 204 countries and territories, 1990-2019: a systematic analysis for the global burden of disease study 2019. Lancet (2020) 396:1204–22. doi: 10.1016/S0140-6736(20)30925-9
4. Tang R, Wang J, Yang L, Ding X, Zhong Y, Pan J, et al. Subclinical hypothyroidism and depression: A systematic review and meta-analysis. Front Endocrinol (Lausanne) (2019) 10:340. doi: 10.3389/fendo.2019.00340
5. Vancampfort D, Stubbs B, Mitchell AJ, De Hert M, Wampers M, Ward PB, et al. Risk of metabolic syndrome and its components in people with schizophrenia and related psychotic disorders, bipolar disorder and major depressive disorder: a systematic review and meta-analysis. World Psychiatry (2015) 14:339–47. doi: 10.1002/wps.20252
6. Vancampfort D, Correll CU, Wampers M, Sienaert P, Mitchell AJ, De Herdt A, et al. Metabolic syndrome and metabolic abnormalities in patients with major depressive disorder: a meta-analysis of prevalences and moderating variables. Psychol Med (2014) 44:2017–28. doi: 10.1017/S0033291713002778
7. Han K-M, Kim MS, Kim A, Paik J-W, Lee J, Ham B-J. Chronic medical conditions and metabolic syndrome as risk factors for incidence of major depressive disorder: A longitudinal study based on 4.7 million adults in south Korea. J Affect Disord (2019) 257:486–94. doi: 10.1016/j.jad.2019.07.003
8. Fugger G, Dold M, Bartova L, Kautzky A, Souery D, Mendlewicz J, et al. Comorbid thyroid disease in patients with major depressive disorder - results from the European group for the study of resistant depression (GSRD). Eur Neuropsychopharmacol (2018) 28:752–60. doi: 10.1016/j.euroneuro.2018.03.011
9. Hough CM, Bersani FS, Mellon SH, Morford AE, Lindqvist D, Reus VI, et al. Pre-treatment allostatic load and metabolic dysregulation predict SSRI response in major depressive disorder: a preliminary report. Psychol Med (2021) 51(12):2117–25. doi: 10.1017/S0033291720000896
10. Yang L, Yang X, Yang T, Wu X, Sun P, Zhu Y, et al. The effect of thyroid function on the risk of psychiatric readmission after hospitalization for major depressive disorder. Psychiatry Res (2021) 305:114205. doi: 10.1016/j.psychres.2021.114205
11. Liu W, Wu Z, Sun M, Zhang S, Yuan J, Zhu D, et al. Association between fasting blood glucose and thyroid stimulating hormones and suicidal tendency and disease severity in patients with major depressive disorder. Bosn J Basic Med Sci (2022) 22:635–42. doi: 10.17305/bjbms.2021.6754
12. Bagherniya M, Khayyatzadeh SS, Avan A, Safarian M, Nematy M, Ferns GA, et al. Metabolic syndrome and its components are related to psychological disorders: A population based study. Diabetes Metab Syndr (2017) 11 Suppl 2:S561–6. doi: 10.1016/j.dsx.2017.04.005
13. Caye A, Pilz LK, Maia AL, Hidalgo MP, Furukawa TA, Kieling C. The impact of selective serotonin reuptake inhibitors on the thyroid function among patients with major depressive disorder: A systematic review and meta-analysis. Eur Neuropsychopharmacol (2020) 33:139–45. doi: 10.1016/j.euroneuro.2020.01.011
14. Lee SH, Paz-Filho G, Mastronardi C, Licinio J, Wong M-L. Is increased antidepressant exposure a contributory factor to the obesity pandemic? Transl Psychiatry (2016) 6:e759. doi: 10.1038/tp.2016.25
15. Liu F-H, Hwang J-S, Kuo C-F, Ko Y-S, Chen S-T, Lin J-D. Subclinical hypothyroidism and metabolic risk factors association: A health examination-based study in northern Taiwan. BioMed J (2018) 41:52–8. doi: 10.1016/j.bj.2018.02.002
16. Li R-C, Zhang L, Luo H, Lei Y, Zeng L, Zhu J, et al. Subclinical hypothyroidism and anxiety may contribute to metabolic syndrome in sichuan of China: a hospital-based population study. Sci Rep (2020) 10:2261. doi: 10.1038/s41598-020-58973-w
17. Ding X, Zhao Y, Zhu C-Y, Wu L-P, Wang Y, Peng Z-Y, et al. The association between subclinical hypothyroidism and metabolic syndrome: an update meta-analysis of observational studies. Endocr J (2021) 68:1043–56. doi: 10.1507/endocrj.EJ20-0796
18. He J, Lai Y, Yang J, Yao Y, Li Y, Teng W, et al. The relationship between thyroid function and metabolic syndrome and its components: A cross-sectional study in a Chinese population. Front Endocrinol (Lausanne) (2021) 12:661160. doi: 10.3389/fendo.2021.661160
19. Nakajima Y, Yamada M, Akuzawa M, Ishii S, Masamura Y, Satoh T, et al. Subclinical hypothyroidism and indices for metabolic syndrome in Japanese women: one-year follow-up study. J Clin Endocrinol Metab (2013) 98:3280–7. doi: 10.1210/jc.2013-1353
20. McInerney AM, Lindekilde N, Nouwen A, Schmitz N, Deschênes SS. Diabetes distress, depressive symptoms, and anxiety symptoms in people with type 2 diabetes: A network analysis approach to understanding comorbidity. Diabetes Care (2022) 45(8):1715–23. doi: 10.2337/dc21-2297
21. Borsboom D, Deserno MK, Rhemtulla M, Epskamp S, Fried EI, McNally RJ, et al. Network analysis of multivariate data in psychological science. Nat Rev Methods Primers (2021) 1:1–18. doi: 10.1038/s43586-021-00055-w
22. Zainal NH, Newman MG. Prospective network analysis of proinflammatory proteins, lipid markers, and depression components in midlife community women. Psychol Med (2022) 4:1–12. doi: 10.1017/S003329172200232X
23. Garcia-Mondragon L, Konac D, Newbury JB, Young KS, Ing A, Fürtjes AE, et al. Role of polygenic and environmental factors in the co-occurrence of depression and psychosis symptoms: a network analysis. Transl Psychiatry (2022) 12:259. doi: 10.1038/s41398-022-02022-9
24. Duan Y, Wei J, Geng W, Jiang J, Zhao X, Li T, et al. Research on cognitive function in anxious depression patients in China. J Affect Disord (2021) 280:121–6. doi: 10.1016/j.jad.2020.11.078
25. Li Z, Xue M, Zhao L, Zhou Y, Wu X, Xie X, et al. Comorbid major depression in first-episode drug-naïve patients with schizophrenia: Analysis of the depression in schizophrenia in China (DISC) study. J Affect Disord (2021) 294:33–8. doi: 10.1016/j.jad.2021.06.075
26. Zhou Y, Wang Q, Ren H, Yang WFZ, Ma Y, Wu Q, et al. Prevalence and related factors of anxiety in first episode and drug naïve Chinese han outpatients with psychotic major depression. J Affect Disord (2022) 316:217–22. doi: 10.1016/j.jad.2022.08.018
27. Peng P, Wang Q, Ren H, Zhou Y, Hao Y, Chen S, et al. Association between thyroid hormones and comorbid psychotic symptoms in patients with first-episode and drug-naïve major depressive disorder. Psychiatry Res (2023) 320:115052. doi: 10.1016/j.psychres.2023.115052
28. Skjerdingstad N, Johnson MS, Johnson SU, Hoffart A, Ebrahimi OV. Feelings of worthlessness links depressive symptoms and parental stress: A network analysis during the COVID-19 pandemic. Eur Psychiatry (2021) 64:e50. doi: 10.1192/j.eurpsy.2021.2223
29. Epskamp S, Borsboom D, Fried EI. Estimating psychological networks and their accuracy: A tutorial paper. Behav Res (2018) 50:195–212. doi: 10.3758/s13428-017-0862-1
30. Hevey D. Network analysis: a brief overview and tutorial. Health Psychol Behav Med (2018) 6:301–28. doi: 10.1080/21642850.2018.1521283
31. Wang Y, Hu Z, Feng Y, Wilson A, Chen R. Changes in network centrality of psychopathology symptoms between the COVID-19 outbreak and after peak. Mol Psychiatry (2020) 25:3140–9. doi: 10.1038/s41380-020-00881-6
32. Fonseca-Pedrero E. Network analysis: A new way of understanding psychopathology? Rev Psiquiatría Y Salud Ment (English Ed) (2017) 10:206–15. doi: 10.1016/j.rpsmen.2017.10.005
33. Malgaroli M, Calderon A, Bonanno GA. Networks of major depressive disorder: A systematic review. Clin Psychol Rev (2021) 85:102000. doi: 10.1016/j.cpr.2021.102000
34. Ren L, Wang Y, Wu L, Wei Z, Cui L-B, Wei X, et al. Network structure of depression and anxiety symptoms in Chinese female nursing students. BMC Psychiatry (2021) 21:279. doi: 10.1186/s12888-021-03276-1
35. McNally RJ. Can network analysis transform psychopathology? Behav Res Ther (2016) 86:95–104. doi: 10.1016/j.brat.2016.06.006
36. Jones PJ, Mair P, Riemann BC, Mugno BL, McNally RJ. A network perspective on comorbid depression in adolescents with obsessive-compulsive disorder. J Anxiety Disord (2018) 53:1–8. doi: 10.1016/j.janxdis.2017.09.008
37. França AB, Gordon AL, Samra R, Rodolpho Duarte ES, Jacinto AF. Symptoms of mood disorders in family carers of older people with dementia who experience caregiver burden: a network approach. Age Ageing (2020) 49:628–33. doi: 10.1093/ageing/afaa008
38. Zhou J, Fan A, Zhou X, Pao C, Xiao L, Feng Y, et al. Interrelationships between childhood maltreatment, depressive symptoms, functional impairment, and quality of life in patients with major depressive disorder: A network analysis approach. Child Abuse Negl (2022) 132:105787. doi: 10.1016/j.chiabu.2022.105787
39. McNally RJ, Mair P, Mugno BL, Riemann BC. Co-Morbid obsessive-compulsive disorder and depression: a Bayesian network approach. Psychol Med (2017) 47:1204–14. doi: 10.1017/S0033291716003287
40. Scutari M, Nagarajan R. Identifying significant edges in graphical models of molecular networks. Artif Intell Med (2013) 57:207–17. doi: 10.1016/j.artmed.2012.12.006
41. Sharif K, Tiosano S, Watad A, Comaneshter D, Cohen AD, Shoenfeld Y, et al. The link between schizophrenia and hypothyroidism: a population-based study. Immunol Res (2018) 66:663–7. doi: 10.1007/s12026-018-9030-7
42. Gu F, Ding G, Lou X, Wang X, Mo Z, Zhu W, et al. Incidence of thyroid diseases in zhejiang province, China, after 15 years of salt iodization. J Trace Elem Med Biol (2016) 36:57–64. doi: 10.1016/j.jtemb.2016.04.003
43. Zhang J, Gu Y, Meng G, Zhang Q, Liu L, Wu H, et al. Association between dietary onion intake and subclinical hypothyroidism in adults: a population-based study from an iodine-replete area. Endocrine (2021) 74:616–24. doi: 10.1007/s12020-021-02790-2
44. Li Y, Teng D, Ba J, Chen B, Du J, He L, et al. Efficacy and safety of long-term universal salt iodization on thyroid disorders: Epidemiological evidence from 31 provinces of mainland China. Thyroid (2020) 30:568–79. doi: 10.1089/thy.2019.0067
45. Loh HH, Lim LL, Yee A, Loh HS. Association between subclinical hypothyroidism and depression: an updated systematic review and meta-analysis. BMC Psychiatry (2019) 19:12. doi: 10.1186/s12888-018-2006-2
46. Contreras F, Menchon JM, Urretavizcaya M, Navarro MA, Vallejo J, Parker G. Hormonal differences between psychotic and non-psychotic melancholic depression. J Affect Disord (2007) 100:65–73. doi: 10.1016/j.jad.2006.09.021
47. Chang C-H, Yeh Y-C, Caffrey JL, Shih S-R, Chuang L-M, Tu Y-K. Metabolic syndrome is associated with an increased incidence of subclinical hypothyroidism - a cohort study. Sci Rep (2017) 7:6754. doi: 10.1038/s41598-017-07004-2
48. Kim M-D, Yang H-J, Kang NR, Park JH, Jung Y-E. Association between subclinical hypothyroidism and metabolic syndrome among individuals with depression. J Affect Disord (2020) 264:494–7. doi: 10.1016/j.jad.2019.11.080
49. Zhao S, Chen Z, Wang X, Yao Z, Lu Q. Increased prevalence of subclinical hypothyroidism in female hospitalized patients with depression. Endocrine (2021) 72:479–85. doi: 10.1007/s12020-020-02490-3
50. Liu H, Peng D. Update on dyslipidemia in hypothyroidism: the mechanism of dyslipidemia in hypothyroidism. Endocr Connect (2022) 11:e210002. doi: 10.1530/EC-21-0002
51. Xu C, Zhou L, Wu K, Li Y, Xu J, Jiang D, et al. Abnormal glucose metabolism and insulin resistance are induced via the IRE1α/XBP-1 pathway in subclinical hypothyroidism. Front Endocrinol (Lausanne) (2019) 10:303. doi: 10.3389/fendo.2019.00303
52. Zhou L, Ding S, Li Y, Wang L, Chen W, Bo T, et al. Endoplasmic reticulum stress may play a pivotal role in lipid metabolic disorders in a novel mouse model of subclinical hypothyroidism. Sci Rep (2016) 6:31381. doi: 10.1038/srep31381
53. Kalinowska S, Trześniowska-Drukała B, Safranow K, Pełka-Wysiecka J, Kłoda K, Misiak B, et al. Association between thyroid function and metabolic syndrome in male and female schizophrenia patients. Psychiatry Res (2019) 274:167–75. doi: 10.1016/j.psychres.2019.02.029
54. Wagner CJ, Musenbichler C, Böhm L, Färber K, Fischer A-I, von Nippold F, et al. LDL cholesterol relates to depression, its severity, and the prospective course. Prog Neuropsychopharmacol Biol Psychiatry (2019) 92:405–11. doi: 10.1016/j.pnpbp.2019.01.010
55. Park JH, Jung SJ, Jung Y, Ahn SV, Lee E, Kim HC. Association between the change of total cholesterol during adolescence and depressive symptoms in early adulthood. Eur Child Adolesc Psychiatry (2021) 30:261–9. doi: 10.1007/s00787-020-01511-w
56. Beydoun MA, Beydoun HA, Dore GA, Fanelli-Kuczmarski MT, Evans MK, Zonderman AB. Total serum cholesterol, atherogenic indices and their longitudinal association with depressive symptoms among US adults. Transl Psychiatry (2015) 5:e518. doi: 10.1038/tp.2015.4
57. Haneklaus M, O’Neill LAJ. NLRP3 at the interface of metabolism and inflammation. Immunol Rev (2015) 265:53–62. doi: 10.1111/imr.12285
58. Colasanto M, Madigan S, Korczak DJ. Depression and inflammation among children and adolescents: A meta-analysis. J Affect Disord (2020) 277:940–8. doi: 10.1016/j.jad.2020.09.025
59. Beurel E, Toups M, Nemeroff CB. The bidirectional relationship of depression and inflammation: Double trouble. Neuron (2020) 107:234–56. doi: 10.1016/j.neuron.2020.06.002
60. De Giorgi R, Rizzo Pesci N, Quinton A, De Crescenzo F, Cowen PJ, Harmer CJ. Statins in depression: An evidence-based overview of mechanisms and clinical studies. Front Psychiatry (2021) 12:702617. doi: 10.3389/fpsyt.2021.702617
61. Kim S-W, Kang H-J, Jhon M, Kim J-W, Lee J-Y, Walker AJ, et al. Statins and inflammation: New therapeutic opportunities in psychiatry. Front Psychiatry (2019) 10:103. doi: 10.3389/fpsyt.2019.00103
62. Bauer M, Whybrow PC. Role of thyroid hormone therapy in depressive disorders. J Endocrinol Invest (2021) 44:2341–7. doi: 10.1007/s40618-021-01600-w
63. Zhao C, Wang Y, Xiao L, Li L. Effect of levothyroxine on older patients with subclinical hypothyroidism: A systematic review and meta-analysis. Front Endocrinol (Lausanne) (2022) 13:913749. doi: 10.3389/fendo.2022.913749
64. Zhao T, Chen BM, Zhao XM, Shan ZY. Subclinical hypothyroidism and depression: a meta-analysis. Transl Psychiatry (2018) 8:239. doi: 10.1038/s41398-018-0283-7
65. Wildisen L, Feller M, Del Giovane C, Moutzouri E, Du Puy RS, Mooijaart SP, et al. Effect of levothyroxine therapy on the development of depressive symptoms in older adults with subclinical hypothyroidism: An ancillary study of a randomized clinical trial. JAMA Netw Open (2021) 4:e2036645. doi: 10.1001/jamanetworkopen.2020.36645
Keywords: major depressive disorder, network analysis, metabolic disturbances, thyroid dysfunction, anxiety, psychotic symptoms
Citation: Peng P, Wang Q, Lang X, Liu T and Zhang X-Y (2023) Association between thyroid dysfunction, metabolic disturbances, and clinical symptoms in first-episode, untreated Chinese patients with major depressive disorder: Undirected and Bayesian network analyses. Front. Endocrinol. 14:1138233. doi: 10.3389/fendo.2023.1138233
Received: 05 January 2023; Accepted: 14 February 2023;
Published: 28 February 2023.
Edited by:
Divya Vohora, Jamia Hamdard University, IndiaReviewed by:
Xuebing Liu, Huazhong University of Science and Technology, ChinaNi Fan, Guangzhou Medical University, China
Copyright © 2023 Peng, Wang, Lang, Liu and Zhang. This is an open-access article distributed under the terms of the Creative Commons Attribution License (CC BY). The use, distribution or reproduction in other forums is permitted, provided the original author(s) and the copyright owner(s) are credited and that the original publication in this journal is cited, in accordance with accepted academic practice. No use, distribution or reproduction is permitted which does not comply with these terms.
*Correspondence: Tieqiao Liu, liutieqiao123@csu.edu.cn; Xiang-Yang Zhang, zhangxy@psych.ac.cn