- 1Department of Botany, Mohanlal Sukhadia University, Udaipur, India
- 2Laboratory of Phytopathology and Microbial Biotechnology, Department of Botany, Mohanlal Sukhadia University, Udaipur, India
Industrialization, population burst, and changing lifestyles have resulted in the genesis of non-degradable pollutants languishing the environment and human health. Biological approaches using microorganisms are gaining importance as an eco-friendly and cost-effective substitute to mitigate the pollution load. Microorganisms can survive in a divergent environment and produce metabolites that can degrade and transform pollutants making it possible to revive contaminated sites naturally. Modern omics technologies like metagenomics, transcriptomics, proteomics, etc. have been used nowadays design strategies to study ecology and diversity of microorganisms and their application in environmental monitoring and bioremediation. The present article will focus on the omics techniques reportedly used in environmental monitoring to tackle the pollution load.
Introduction
Pollution load is increasing at an alarming rate as a result of industrialization and population outburst. The industrialization has caused in the utilization and production of chemicals for hi-tech innovations which ensued the generation of non-biodegradable pollutants like xenobiotics, hydrocarbons, heavy metals, etc. (Labie, 2007). These toxic pollutants remain persistent in the environment and pose a serious threat to living organisms. Increasing awareness has generated numerous approaches using advanced scientific technology to audit and curtail this arduous global issue. The widely accepted technique for decontaminating a polluted environment in an eco-friendly and sustainable manner is bioremediation (Paul et al., 2005; Raghunandan et al., 2018).
Bioremediation is an enticing process to detoxify environmental contaminants. Microorganisms, such as fungi, yeast, and bacteria have been considered to be outstanding organisms for detoxification of pollutants (Zhong and Zhou, 2002; Luciene et al., 2015; Abou Seeda et al., 2017). It assures a cheap, simple, and eco-friendly cleanup method (Lovley, 2003). Microorganisms are nutritionally flexible and have the flair to adapt to extreme environmental conditions. They also possess numerous intracellular and extracellular enzymes which utilize the complex pollutants and convert them into carbon and energy source (Nojiri and Tsuda, 2005; Thakur et al., 2019). They also undergo a rapid genetic transformation which enables them to acquire new metabolic routes for deterioration of xenobiotics (Poirier et al., 2013; Igiri et al., 2018).
Microbial bioremediation strategies can be either ex-situ or in-situ. Ex-situ techniques consist of transporting pollutants from polluted sites to another site for treatment while in situ techniques treat polluted substances at the site itself. Ex-situ remediation technique owing to its limitations is not considered a choice of cleanup by many researchers. It may or may not be lucrative at particular sites and may be possible that the microorganisms which assisted in clean-up of pollutants under in-vitro conditions fail to remove them effectively under in-vivo conditions (Head et al., 2003; Barupal et al., 2019a,b). The mode of action and growth of microorganisms in polluted sites needs to be more studied for a better understanding (Lovley, 2003). Thus, extensive research needs to be focused on elementary and applied facets of microbial synergism to assess the biodegrading capacity microorganisms present in polluted sites and the changes in their structure and metabolic pathways.
Microbial bioremediation strategies are based on the consortia of diverse organisms that are indigenous to the polluted sites and possess immense metabolic potential. Isolating and purifying such indigenous microorganisms gives some knowledge of the microbial metabolites and the degradation processes. The method to access the microbial world remains immensely perplexing, as the majority of microbes in the environment are non-culturable under in-vitro conditions (Handelsman, 2004). Only a petite percent of microorganisms from diverse environment samples are actively culturable effortlessly and thus not accessible for fundamental research (Dickson et al., 2014; Bursle and Robson, 2016; Awasthi et al., 2020).
The recent advances in molecular tools enabled a cutting edge to assess the unculturable organisms from natural environments (Handelsman, 2004; Gilbert and Dupont, 2011). Molecular approaches like genomics, proteomics, transcriptomics, metabolomics, fluxomics, etc. gave more vision about the microbial communities inhabiting a particular environmental niche (De Sousa et al., 2018; Gupta et al., 2020; Rodríguez et al., 2020). The onsets of next-generation sequencing methods and in-silico analyses have facilitated researchers to address the problems associated with unculturable microorganisms (Maphosa et al., 2010a; Malla et al., 2018). These technologies have abetted the collection exhaustive biological data about microorganisms, their cellular mechanisms, and biomolecules like enzymes associated with bioremediation (Zhang et al., 2010; Misra et al., 2018; Pandey et al., 2019).
Bioremediation holds future assurance for detoxifying the contaminated sites. However, the technique is still riddled with complications that need to be addressed. The most critical step is to comprehend the indigenous microbiome, their synergy with the environment, and its associated factors which help in the expression of their genotype (Chakraborty et al., 2012; Callaghan, 2013). Hence, a multidisciplinary approach is required to unveil the pathways and chemistry that remains hidden for making bioremediation a versatile technique for environmental monitoring. Earlier published research papers have emphasized on individual genomic approaches in-depth for microbe mediated bioremediation. Hence, the present review aims to discuss a brief overview of the prevalent multi-omics techniques to investigate and understand the structural and functional aspects of the microbial community involved in bioremediation. This review article also provides a comparative analysis of commonly employed sequencing platforms and reviews a few recent signs of progress of integrated omics approaches to analyze complex microbial communities in diverse environmental samples. Therefore, the present article will discuss the modern and effective method of “omics” technologies for environmental monitoring and bioremediation.
Genomics and 16s rRNA for Bioremediation
The emergence of genomic technologies has boosted the treatment of contaminated environments in a sustainable manner. Cultivation-independent analyses of microorganisms from contaminated sites have amplified the perception of community dynamics and myriad of microbes actively involved in bioremediation (Kapley et al., 2007; Desai et al., 2010). The identification of the microbial communities using modern genomic tools has enabled the detection of distinctive microorganisms that were not approachable by culture-based techniques. Gene amplification (using PCR) and sequencing techniques have proven exceptionally useful in evaluating the microbial community (Malik et al., 2008; Rani et al., 2008; Gołebiewski and Tretyn, 2020).
The discovery of a highly conserved and variable gene sequence, 16S rRNA in all microorganisms is considered as the highest quality level for describing phylogenetic similarities among organisms in microbial communities (Lovley et al., 1991; Lovley, 2003). 16S rRNA gene sequence analysis can be used for a complete assessment of microbial diversity by selectively amplifying and sequencing the hypervariable regions of the 16S rRNA gene. It is a highly efficient and cost-effective technology easily accessible by various bioinformatics tools and has become a frequently used technique for profiling intricate microbial communities (Han et al., 2020). It can be used to identify novel, unculturable, and phenotypically unidentifiable microbes (Clarridge, 2004).
The phylogenetic organization of the microbes linked with bioremediation processes can be determined by investigating the 16S rRNA sequences obtained from contaminated environments (Lovley, 2003; Rogers and McClure, 2003). 16S rRNA was used to elucidate the composition of microbial communities and the multifariousness of the dioxygenase genes in the soil of a coal tar mixing plant to study the genetics of PAH degradation (Kumar and Khanna, 2010; Viant and Sommer, 2013; Sakshi Haritash, 2020). Kou et al. (2018) reported 16S rRNA gene amplicon sequencing to study the abundance and diversity of the microbial community in soil polluted with heavy metals like lead, zinc, and copper in Shanghai. 16S rRNA gene sequencing along with membrane fatty acid profile was used to identify soil bacterium Pseudomonas species capable of degrading polyurethane from a site containing an abundance of fragile plastic waste (Cárdenas Espinosa et al., 2020).
Microarrays
DNA microarray is widely known as a DNA chip or a biochip is one of the most promising methods in functional genomics. It is an assortment of microscopic DNA spots deposited or synthesized in a two dimensional or three-dimensional arrays on a solid surface like glass, silicon chips, or nylon membrane by covalent or non-covalent interactions. It allows the analysis of multiple genes at once without PCR amplification of the individual genes. DNA microarrays have been reported to be used to assess the physiology and catabolic gene expression profile of microorganisms isolated from environmental samples (Schut et al., 2001; Dennis et al., 2003; Chandran et al., 2020). Rhee et al. (2004) established an extensive 50-mer-based oligonucleotide microarray to identify genes associated with biodegradation and biotransformation in microbial communities. DNA microarrays were used in transcriptional profiling, quantify genes in biological samples, stress gene analysis of microbial genomes, etc. (Cho and Tiedje, 2002; Muffler et al., 2002; Greene and Voordouw, 2003; Chittora et al., 2020). Various microarrays designed to examine microbial communities are discussed below.
PhyloChip Arrays
It is an ease quality chip microarray created by the business head Affymetrix to recognize different bacterial and archaeal organisms from complex microbial communities. It gives a progressively quick, complete, and precise testing method for samples retrieved from the environment without the use of any culture techniques. These chips contain large information of genes and are widely used in hybridization-based identification and study of mutations and polymorphisms like single nucleotide polymorphisms or disease- pertinent mutations analysis. They have been used to study microbial profile in extreme biological systems like sun powered salterns, industrial effluents, olive-mill squanders, coral reefs, etc. (Nikolaki and Tsiamis, 2013). Phylochip arrays have been reportedly used to explore the bacterial diversity and community structure in chromium contaminated industrial wastewater (Katsaveli et al., 2012), Australian sugar cane processing plants (Sharmin et al., 2013).
Functional Gene Arrays (FGAs)
They are the particular kind of DNA microarrays incorporating probes for pivotal genes associated with microbial functional progressions (Cho and Tiedje, 2001; Palmer et al., 2006). FGAs are useful for evaluating the functional composition and network of microbial communities (Zhou et al., 2015; Shi et al., 2019). It is commonly employed for the synchronized inspection of multiple functional genes (Greene and Voordouw, 2003; Palmer et al., 2006). These arrays are widely used to study microbial communities from environmental samples because the majority of microbes in such samples are highly diverse, metabolically flexible, and unculturable (van Nostrand et al., 2011).
GeoChip Arrays
It is the most extensive DNA microarray accessible for contemplating microbial communities related to biogeochemical cycling, ecosystem management, environmental restoration, and remediation, etc. It can be utilized to study microbial association in soil, water, air, human and animal sources. It does not entail any preceding information of the microbial community and can detect less abundant microorganisms preventing annotation bias. The process is comparatively fast, and uses either DNA or RNA and utilizes 56,990 gene sequences from 292 functional genes consuming 27,812 probes. GeoChip uses key enzymes or genes to spot various microbe mediated mechanisms for biogeochemical cycles of nitrogen, sulfur, phosphorus, etc. resistance mechanisms for heavy metals like silver, cadmium, copper, mercury, nickel, lead, zinc, etc. (van Nostrand et al., 2011; Zehra et al., 2020), degradation pathways to identify and track the degradation of xenobiotics frequently found in the environment (He et al., 2010; Xie et al., 2011). It has been reported as an efficient, sensitive, perceptive and quantitative tool for investigating the functional structure of microbial communities (Zhou et al., 2008; Xue et al., 2016) from divergent environments like soils, aquatic systems, extreme environments, contaminated habitats, etc. (He et al., 2018; Shi et al., 2019).
Omics Approaches
Advances in molecular techniques like proteomics, transcriptomics, metagenomics, etc. have opened new strategies and opportunities in environment management (Plewniak et al., 2018; Rodríguez et al., 2020). These methods have accelerated the study of microbial community structure which was earlier dependent on culture technologies (Iwamoto and Nasu, 2001; Gutleben et al., 2018). It possesses the potential to evaluate the genetic diversity of environmentally pertinent microorganisms and analyze novel functional genes related to the catabolism of pollutants (Eyers et al., 2004; Meena et al., 2019). Figure 1 depicts the various types of omics approaches commonly used for microbial community analysis.
Omics technology is the molecular biological approach, which facilitates the analysis of biomolecules like DNA, RNA, proteins, and metabolites from individual organisms and the whole community at the same time (Gutierrez et al., 2018). To study gene regulation in the anthropogenic environment, mRNA expression (transcriptomics), and whole community expression (metatranscriptomics) can be studied (Schneegurt and Kulpa, 1998; Seo et al., 2009; Roume et al., 2015). Anthropogenically induced proteins from the contaminated sites can be tracked by studying the proteins using metaproteomics (Desai et al., 2010). Metagenomics analyzes the microbial communities directly from the ecological environment which in turn reduces the labor of screening and culture of microbes (Riesenfeld et al., 2004; Panagiotou et al., 2007; Ghazanfar et al., 2010). The study of protein through which anthropogenically induced proteins can be tracked from the contaminated sites is called metaproteomics (Desai et al., 2010). The analysis of primary and secondary proteinaceous metabolites synthesized by the organisms during distinct environmental situations is metabolomics (Desai et al., 2010). Multi-omics approaches are commonly reported to study microbial communities because a single omics analysis cannot disclose the functional activity of the microbial community (Shah et al., 2013; Meena et al., 2018).
Metagenomics
A major share of the microorganisms in the environment is non-culturable under laboratory conditions (Rashid and Stingl, 2015). Metagenomics can be used to explore such non-culturable microbes thriving in different environments (Oulas et al., 2015; Bilal et al., 2018). Metagenomic sequences reveal DNA sequences of uncultured microbes thriving in the environment which can be used for new biotechnology applications. The metagenomic information will enable researchers to integrate pure culture study with genomics (Hodkinson and Grice, 2015). It uses the pool of environmental genomes of microorganisms which increases the probability to discover unique genes and diverse pathways with new enzymes containing highly specific catalytic properties (Scholz et al., 2012; Yergeau et al., 2017; Awasthi et al., 2020). Due to the dearth of high-throughput sequencing technologies and software early metagenomic studies were focused on environments like acid mine drainage and the human gut microbiome. With the advancement of powerful software tools and molecular technologies extreme environments with extreme temperature, alkalinity, acidity, low oxygen, deep-sea hydrothermal vents, heavy metal polluted soils, etc. have been studied using metagenomics which provides infinite chances for bioprospecting and exploring of novel biomolecules like proteins, enzymes, etc. (Oulas et al., 2015; Awasthi et al., 2020).
Metagenomic assessment analysis commences with the isolation of nucleic acid (DNA or RNA) from the environmental samples. Dynamic microbial populaces in polluted environments can be evaluated by genome improvement monitored by metagenome investigation (Chen and Murrell, 2010). Stable Isotope Probing (SIP) cataloging can be utilized to specifically enhance the RNA, DNA, or phospholipids of dynamic microbial populaces. Metagenomic reference libraries are prepared by cloning DNA fragments isolated from the environmental samples in an appropriate vector [phage, plasmid, bacterial artificial chromosome (BAC)] and then reconstructed into a host bacterium. The consequential transformants can be screened for phylogenetic markers, conserved genes, expression of specific traits, etc. (Handelsman, 2004). Biological information from metagenomic libraries can be acquired based on two types of analysis: function-driven or sequence-driven analysis. The function-driven analysis is based on the identification of clones that express their functional activity while sequence driven analysis is based on the sequencing of clones with phylogenetic anchors or conserved DNA sequences that is the plausible origin of the DNA fragment (Wu et al., 2010; Felczykowska et al., 2015; Wong, 2018).
Sequence-based screening is increasingly used owing to the availability of several software for data analysis and the ease to assess metagenomic sequencing data. This approach is predominantly influenced by the precision of genome annotation, the integrity of the available data, algorithms, and facts in databases to ascertain the function of novel genes (Ferrer et al., 2009). It may not be useful if the sequence analogy does not complement to a functional association or the original gene has less analogy to some genes whose products have been investigated biochemically or a specific gene is capable to accomplish diverse tasks in the cell (Hallin et al., 2008). In such cases, function-driven screening is preferred to discover genes with novel functions or to explore the sequence variation of protein families (Singh et al., 2009; Meena et al., 2016). The flowchart in Figure 2 represents the general procedures adopted for metagenomic research.
Metagenomic analysis has opened new frontiers to analyze microbial communities, their genetic diversity, and metabolic pathways. It has provided opportunities to discover microbial consortia and genes involved in the bioremediation of xenobiotic compounds. Phenol degrading pathways of uncultivated bacteria in activated sludge was studied using metagenomics (Sueoka et al., 2009). The metagenomic approach was used to characterize genes and metabolic pathways associated with the degradation of phenol and other aromatic compounds in sludge from a petroleum refinery wastewater treatment system (Silva et al., 2013). Vigneron et al. (2017) accomplished a comparative metagenomic study to understand the community structure and diverse functions of marine seep ecosystems in the Gulf of Mexico and reported that microorganisms in deep cold seeps have the potential to consume a range of non-methane hydrocarbons. Metagenome sequencing was employed to outline the functional potential, taxonomic community composition, and to predict the breakdown of chemical compounds of soils with organophosphorus pesticide exposure (Jeffries et al., 2018). Metagenomic data analysis of microbial consortium emerging on diesel revealed the bacterial genera, genes, and enzymes involved in diesel degradation (Garrido-Sanz et al., 2019).
Gaytán et al. (2020) combined physical and chemical analysis with metagenomics to explicate probable metabolic pathways associated with polyurethane-degrading to alleviate plastics and xenobiotics pollution. The persistent impact of petroleum pollutants on the taxonomic and metabolic structure of microbial mats were studied using metagenome and enriched mRNA metatranscriptome sequencing (Aubé et al., 2020).
Metagenome Screening Methods
It can be screened using an activity-based (function-driven) or genome-driven (sequence-driven) approach (Desai et al., 2010). A small insert library can be used to discover a single gene or small operons enciphering new metabolic function while large insert libraries are more preferred for a large number of gene groups or DNA fragments (Daniel, 2005; Meena et al., 2015). Commonly employed activity-based screening strategies to acquire metagenome derived biocatalysts are agar plate screening, microfluidics-based screening, FACs based screening, microtiter plate screening, etc. It is based on the generation of a zone of clearance/inhibition, production of secondary metabolites (pigments, antibiotics, etc.), chromophore or fluorophore by the clones (Popovic et al., 2017; Ngara and Zhang, 2018).
In genome driven approach reporter gene-based in vivo screening and selection strategies are employed. The ease in accessing metagenomics sequencing data and the availability of multiple softwares makes this approach in demand for exploring metagenomic libraries (Lin et al., 2006; Dai et al., 2018). Genome driven approach is dependent on reporter gene present in the clones which may be colorimetric (LacZ), fluorescent (GFP), bioluminescent (LuxCDABE), conditional survival (CAT), acidification (AraBAD), ice nucleation (InaZ), motility (CheZ), cell display (LamB), etc. The commonly employed reporter based strategies are transcriptional regulator based (synthetic/natural), ribozyme based, post-translational modified reporter-based and fusion-based strategy. Among the reporter based strategies transcriptional regulator based approach is the most employed selection strategy (Dietrich et al., 2010; van Rossum et al., 2013).
The effectiveness of sequence-based approaches relies on precise genome annotation and available data (Ferrer et al., 2009). It uses algorithms and databases to conclude the functions of novel genes. Thus, these approaches may lag if the sequence analogy does not complement to a functional relationship, or if the novel gene has a feeble resemblance to any genes whose products have been analyzed biochemically, or a specific gene is competent to perform multiple functions in the cell (Ferrer et al., 2009). Function-driven screening is the chosen method for discovering novel genes or for probing the sequence diversity of protein families (Singh et al., 2009; Zhuang et al., 2010; Bier et al., 2018).
Genome Sequencing Technologies
Complete genome sequencing progressed through three technical (mechanical) transformations: first-generation sequencing (complete genome shotgun sequencing), next-generation sequencing (high throughput sequencing), and the third-generation sequencing (single molecule long-read sequencing) (Loman and Pallen, 2015).
First generation sequencing
Frederick Sanger and Allen Maxam—Walter Gilbert sequencing techniques were categorized as the first generation DNA sequencing methods (Liu et al., 2012). Sanger sequencing uses denatured DNA template, radioactively labeled primer, DNA polymerase, and chemically modified nucleotides called di-deoxynucleotides to generate DNA fragments with various lengths. Size of the DNA fragment is determined by the incorporated dNTPs. The DNA fragments are detached based on their lengths on gel electrophoresis and can be viewed by an X-ray or UV-light imaging system (El-Metwally et al., 2014; Meena et al., 2017a,b). This technique is expensive, time-consuming and do not permit the sequencing of complex genomes hence, it is widely utilized for single or low-throughput DNA sequencing nowadays (Sanger and Coulson, 1975; Kchouk et al., 2017). Maxam-Gilbert sequencing is recognized as the chemical degradation method because it uses chemicals to cleave nucleotides. Chemical treatment generates breaks in nucleotide bases which lead to the generation of a series of marked fragments that can be detached based on their length/size by gel electrophoresis. It is considered hazardous owing to the usage of noxious and radioactive chemicals (Maxam and Gilbert, 1977). These techniques were the most routinely used sequencing techniques by researchers till the rise of new sequencing technologies which opened new frontiers for genome analysis.
Next generation sequencing
The second-generation sequencing technology speeded up the process of sequencing by generating sequences with very high throughput at a low cost. It produces a massively parallel analysis from multiple samples where small sections of DNA are ligated with adaptors for random reads during DNA amplification which provides enormous data within a short duration (Zhang et al., 2011). Next-generation sequencing technology involves library preparation, sequencing, base calling, alignment to the established genome, and assorted annotation. Library preparation begins with the fragmentation of DNA into multiple fragments by sonication, enzymatic digestion, or transposase followed by ligation with adaptor sequences. The prepared library is then amplified using clonal amplification and PCR methods to generate DNA replicas. DNA replicas are then sequenced using different approaches (Samorodnitsky et al., 2015). The major platforms used for microbiome studies in next-generation sequencing are pyrosequencing (Roche/454 sequencing), Illumina, SOLiD, Ion Torrent, PacBio RS, etc. (Hodkinson and Grice, 2015). Each sequencing platforms has its peculiar distinct advantages and disadvantages and selection of sequencing platform depend on the required read length, sequence accuracy, depth, and cost (Table 1).
(i) Pyrosequencing technique
The pyrosequencing technique is sequencing by synthesis approach based on the detection of pyrophosphate release after the addition of a nucleotide to the newly synthesized DNA strand (Mardis, 2008; Kchouk et al., 2017). Figure 3 shows the diagrammatic presentation of the pyrosequencing approach. DNA molecule is sheared and ligated with oligonucleotide adapters, affixed to a 28-μm bead, and amplified using PCR in an oil-water emulsion and pyrosequenced (Ronaghi et al., 1996). The beads containing amplicon are then trapped in a picoliter sized well and pyrosequenced. The inclusion of non-labeled nucleotide results in enzyme-mediated liberation of inorganic pyrophosphate which can be detected computationally. Thus, repetitive pyrogenic cycles produce a DNA sequence with a read length of up to 1,000 base pairs and can produce ~1 million reads per run (El-Metwally et al., 2014). The first bacterium to be sequenced through the pyrosequencing technique was a soil bacteria Myxococcus xanthus (Vos and Velicer, 2006). Later, it has been employed to investigate microbial communities from divergent environment sources like freshwater, underground mine water, marine water, corals, fish, terrestrial animals, and mosquitoes (Dinsdale et al., 2008).
(ii) Sequencing by ligation on beads
It consists of multiple sequencing rounds. Figure 4 shows the ligase-mediated sequencing method of the Sequence by Oligonucleotide Ligation and Detection (SOLiD) sequencer (Life Technologies) and its step by step process. It begins with the attachment of adapters to DNA fragments fixed on 1 μm paramagnetic beads and amplified by PCR in an oil-water emulsion. PCR amplicons in beads were secured on a compact planar substrate and hybridized with a universal PCR primer paired with an adaptor. During the event of sequencing, the positional identity of the nucleotide is revealed by ligating universal primer to a fluorescently labeled DNA octamer. The cycle is continued till every base is sequenced two times which enhances the accuracy of this platform (Shendure et al., 2005; Mardis, 2008; Meena et al., 2017c) (Figure 4).
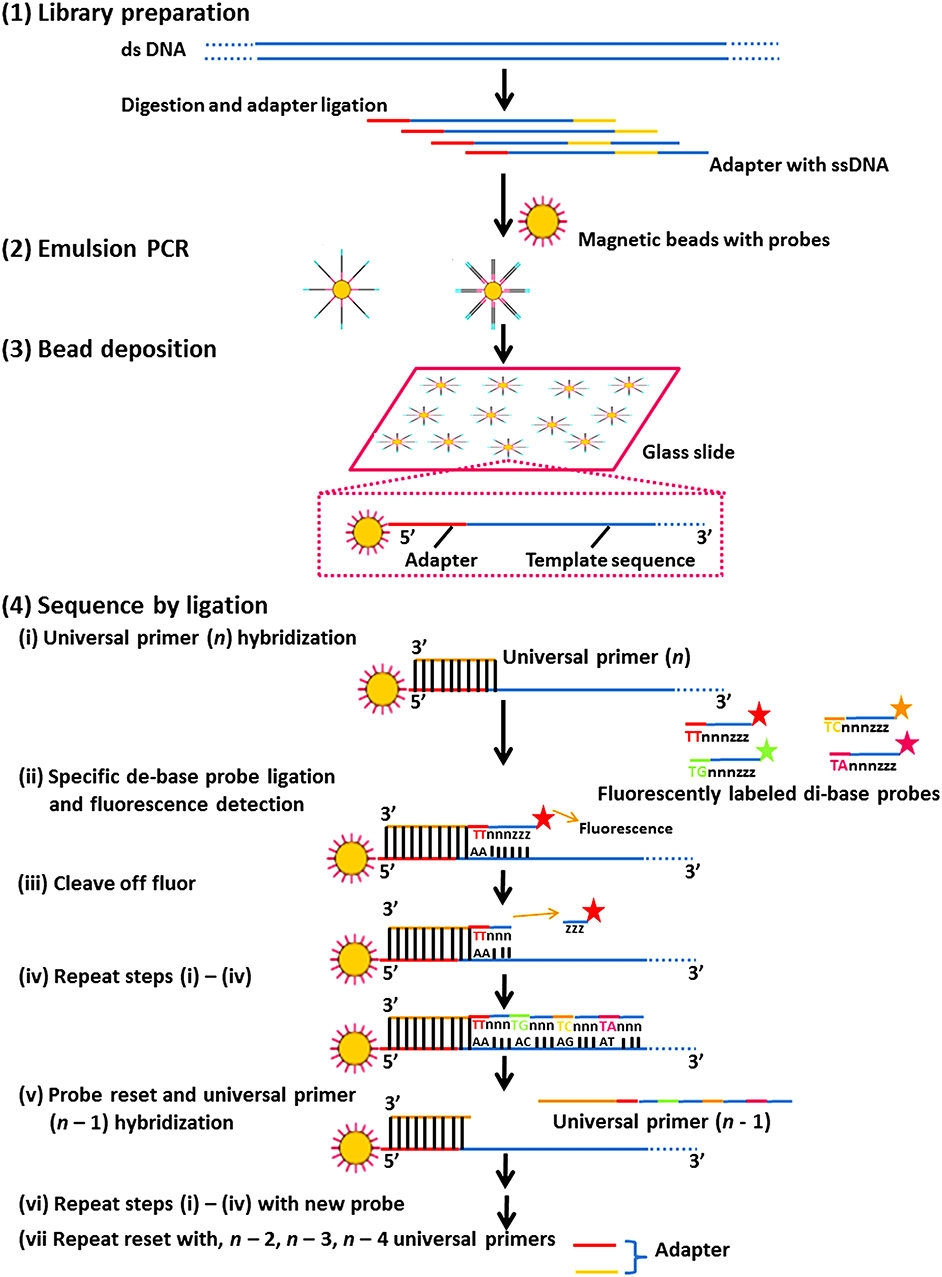
Figure 4. The ligase-mediated sequencing approach of the Sequence by Oligonucleotide Ligation and Detection (SOLiD) sequencer (Life Technologies). (1) Library preparation: two distinctive adapters are ligated to sheared genomic DNA. (2) Emulsion PCR: emulsion PCR is managed with magnetic beads to generate “bead clones,” in which each comprises a solitary nucleic acid species. (3) Bead deposition: the beads are then associated to the outside surface of a glass slide. (4) Sequencing by ligation: ligase-mediated sequencing initiates by annealing a universal primer to the mutual adapter sequences on each amplified fragment (i), and subsequently DNA ligase is given accompanied by precise fluorescently labeled 8-mers, in which the two bases at the 3′ end of the probe are encoded by the appended fluorescent cluster. Every ligation step is monitored by fluorescence recognition (ii), after which a repossession step eliminates the bases from the ligated 8-mer (counting the fluorescent cluster) (iii), and correspondingly readies the extended probe for additional round of ligation (iv–vii). Subsequently every fluorescent cluster on a ligated 8-mer distinguishes a two-base amalgam, the consequential sequence peruses can be screened for base-calling errors vs. either correct polymorphisms or single base removals, by adjusting the individual states to a realized excellent reference sequence.
(iii) Illumina/Solexa sequencing
The DNA library preparation starts with random fragmentation of DNA into sequences followed by ligation of adapters to both ends of each sequence (Adessi et al., 2000; Fedurco et al., 2006; Turcatti et al., 2008). Linkers are used to attach forward and reverse primers with complementarity to the adaptor on a glass surface. Bridge PCR intensifies the DNA fragment resulting in a “cluster” with multiple copies of a single DNA template. Each cluster comprises around one million replicas of the initial sequence. Nucleotide in the sequences is determined using sequencing by synthesis approach using reversible terminators where four amended nucleotides, sequencing primers, and DNA polymerases are incorporated so that the primers are hybridized to the sequences (Meena and Samal, 2019).
It employs modified dNTPs containing a terminator with a fluorescent label at the 3′-OH end that blocks polymerization and allows only a single base addition to each growing DNA strand. Sequencing proceeds in cycles and single bases are added to all templates identically in each cycle, followed by the chemical cleavage of the fluorescent terminator which recognizes the incorporated nucleotide (Bahamonde et al., 2016; Arazoe et al., 2018). Lasers are passed onto the DNA clusters to trigger the fluorescent label incorporated in the nucleotide base which are further detected and recorded by a camera. Multiple computer softwares are employed to interpret these signals into various nucleotide sequences. The process proceeds with the exclusion of the fluorescently-labeled terminator group with the incorporation of a new fluorescently-labeled terminator base until billions of clusters have been sequenced (Bentley et al., 2008; Reuter et al., 2015). Illumina sequencing is extremely precise because the DNA sequence is explored base-by-base.
(iv) Ion torrent sequencing
It is analogous to pyrosequencing technology. Instead of using fluorescent-labeled nucleotides this approach relies on the release of a proton when a dNTP is added to DNA polymer. Adaptors are added to the fragmented DNA or RNA, and one molecule is placed onto beads and amplified by emulsion PCR. Each bead is laid into a single well of a slide containing single species of dNTPs, polymerase, and buffer. Nucleotide incorporation into DNA strand by polymerase releases hydrogen ion as a byproduct that decreases the pH. The decrease in pH is sensed by a pH sensor at the base of the microwell and transformed into a voltage which is proportional to the number of nucleotides incorporated. It is proficient to produce reads lengths of 200, 400, and 600 bp.
Third generation sequencing
Third generations sequencing offers lower sequencing charge and contented sample preparation without PCR amplification. The two most widely used sequencing platforms in third generations sequencing are Pacific Biosciences, Oxford Nanopore technology, and Heliscope technology. Pacific Biosciences developed the first genomic sequencer using single-molecule real-time technology (SMRT) approach (Eid et al., 2009; Meena et al., 2017d). This approach makes use of fluorescent labeling like other sequencing technologies. It detects nucleotide signals in real-time. It employs a framework made of several SMRT cells which comprises microfabricated nanostructures called zero-mode waveguides (ZMWs) (Rhoads and Au, 2015). The bases of each ZMW contain DNA polymerase which binds the target DNA and starts replication. Fluorescently labeled nucleotides with different colors are then added to the reaction wells. With the enzymatic incorporation of each base, a flash of light is generated that recognizes the base and analyzed repetitively to produce the DNA sequence (Rhoads and Au, 2015). It can produce a read length of about 1,500 bp.
Oxford nanopore technology uses electrophoresis to pass the DNA/RNA molecule through a nanopore. It makes use of electrolytic solutions and the utilization of a perpetual electric field. In this technology, double-stranded DNA is sheared and blunt-ended DNA molecules are formed with a termination repair stage. Two adaptors (Y adapter and a hairpin adaptor) conjugated with special motor protein are then added to the DNA which helps in unzipping the double-stranded DNA at the Y adapter and moves the DNA as a single strand through the nanopore. As the nucleic acid moves through the nanopore motor protein action generates a difference in ionic current due to the mobile nucleotides filling the pore. The deviation in ionic current is documented on a graphic model and then elucidated for sequence identification (Jain et al., 2016; Lu et al., 2016).
HeliScope is another technology platform utilizing an extremely perceptive fluorescence detection system for single DNA molecule sequencing. DNA strands are fragmented using restriction enzymes detected by the addition of poly-A tail. The DNA fragments are hybridized to the flow cell plate with billions of oligo(dT) chains bound to its surface to produce an array of a primer annealed single DNA templates. Labeling is accomplished in “quads” comprising of 4 cycles each for each of the four nucleotide bases. Fluorescently labeled bases are added one at a time resulting in a template-dependent extension. A laser light illuminates the label, taking a read of the strands that have taken up a specifically labeled base which is further detected and recorded by a camera. Various computer programs interpret these signals into a nucleotide sequence. The label is then cleaved, and the next cycle proceeds with a new base (Braslavsky et al., 2003; Meena et al., 2017e).
Shotgun sequencing
Shotgun metagenomic sequencing is a powerful technique in microbial ecology because it provides a vigorous and reliable evaluation of microbial diversity (Hillmann et al., 2018). It does not depend on PCR amplification and used to examine the functional potential and microbial composition of the community. It is the only way to study the microbial community with no markers like viruses (Quince et al., 2017; Vermote et al., 2018). It allows strain-level remodeling in the taxonomic analysis and pathway predictions for the functional annotation of the microbiome under study (Han et al., 2020).
It is an emerging molecular method to bridge the gap amid community structure and functional competence. It also helps in understanding the strategies adopted by microorganisms to thrive in adverse conditions (Sharpton, 2014; Peabody et al., 2015; Ranjan et al., 2016). The functional activity of innate communities can be studied using the metagenomic shotgun sequencing approach. Figure 5 shows a schematic illustration signifying the standard metagenomic workflow. It has been used to new and existing microbial community, analyze new viruses (Yozwiak et al., 2012; Quince et al., 2017; Vermote et al., 2018), uncultured bacteria (Wrighton et al., 2012), unique proteins (Godzik, 2011), and metabolic pathways (Morgan et al., 2012).
Shotgun metagenomic sequencing of genomic DNA from a sample has equipped by library planning. The library planning workflow is analogous to consistent whole-genome sequencing, comprising of random DNA fragmentation and adapter ligation. Shotgun metagenomic sequencing workflow for taxonomy analysis consists of quality pruning and evaluation of a reference database involving whole genomes or specifically designed marker genes to create a taxonomy profile. Since, it contain all genetic information in a sample, the information can be used for supplementary analyses like metagenomic assembly and binning, metabolic function profiling, antibiotic resistance gene profiling, etc.
Whole-genome shotgun sequencing of environmental pooled DNA from seawater samples of Sargasso Sea near Bermuda elucidated gene content, diversity, and relative abundance (RA) of the organisms within these environmental samples (Venter et al., 2004). Shotgun metagenomic sequencing of the cave microbiome in Thailand was studied to explore the taxonomic composition and metabolic potential of microorganisms in the soil (Wiseschart et al., 2019). Shotgun metagenomic analysis of microbial communities from deep seabed petroleum seeps in the Eastern Gulf of Mexico revealed the presence of diverse communities of chemoheterotrophs and chemolithotrophs (Dong et al., 2019). Whole-genome shotgun sequencing was engaged to identify the taxonomic diversity and gene repertoire of bacteria isolated from tannery effluents and petrol polluted soil samples for degradation of persistent organic pollutants like naphthalene, toluene, petrol, xylene, etc. (Muccee and Ejaz, 2020).
Transcriptomics
The subdivision of genes transcribed in an organism is known as transcriptome. It is a potent network amid the proteome, genome, and cellular phenotype. It is also called gene expression profiling because it provides the understanding of up or down-regulation of genes under various environments in microbial communities. mRNAs analysis provides a direct vision of cell and tissue specific gene manifestation like (i) the existence, non-existence, and assessment of transcript, (ii) assessment of alternative splicing to foresee protein isoforms, and (iii) quantitative evaluation of genotype impact on gene expression via expression assessable trait loci analysis or allele-specific expression.
Microarray and sequencing techniques are employed for transcriptome analysis (Maroli et al., 2018). Microarrays help to assess the expression of genes while RNA-sequencing uses next-generation sequencing to determine the amount of RNA in a sample. The RNA-microarray strategy uses pre-designed probes which make this approach cheaper, potent, and an enhanced technique for studying protein expression. RNA-sequencing is extensive as it facilitates different types of RNA at a much-advanced coverage and broad discovery studies (Shendure, 2008; Nagalakshmi et al., 2010). The generation of raw transcriptome data involves purification of fine RNA of interest followed by transformation of RNA to complementary DNA (cDNA), fragmenting cDNA to build a library using sequence by synthesis (RNA-sequencing), running the microarray or sequence through superior software platform and carrying out ad hoc QC.
DNA microarray is a powerful technique in transcriptomics that supports in reviewing and evaluating mRNA expression of every single gene existing in an organism. The whole-genome DNA microarray technology has made it possible to define and investigate expressions of genes subjected to modulation with a fluctuating environment (Gao et al., 2004). It has been employed to evaluate variance in metabolic and catabolic gene expressions, to analyze the microbial community physiology from diverse environments, identify new bacterial species, etc. (Dennis et al., 2003; Greene and Voordouw, 2003).
Expression quantitative trait loci (eQTL) mapping is used as a persuasive strategy to explore and consolidate gene expression (RNA data) with genetic variants (DNA data). eQTL evaluation helps in identifying genetic variants that dominate mRNA expression levels. They furnish the relation between genetic variants and gene expression and can thus be used to exploit the fundamental molecular networks linked with a peculiar trait (Yao et al., 2015). The regulation of gene expression can also be studied by the collective analysis of mRNA and microRNA levels. MicroRNAs (mRNAs) are short, non-coding RNA molecules that control transcription of mRNA. The precise binding of mRNAs to a target mRNA (by sequence homology) either impedes mRNA binding to the ribosome or targets it for degradation. Hence, it is therefore obvious that not only miRNA levels, but also their regulation by mRNAs are vital for an extensive gene expression dynamics (Pritchard et al., 2012). mRNA profiling along with miRNA expression can be used to explore variations in the transcriptome profile, particularly to identify the miRNA transcripts that are subjected to regulation, emphasizing the probable molecular pathways supporting a particular trait or condition. This method has been, successfully used to explore gene expression in specific subtypes of gastrointestinal tumors (Pantaleo et al., 2016), estimate modification of gene expression in wound-healing impaired fibroblasts from diabetic patients (Liang et al., 2016).
Comparative transcriptomics revealed highly-upregulated degradation pathways and putative transporters for phenol to improve phenol tolerance and utilization by lipid-accumulating Rhodococcus opacus PD630 (Yoneda et al., 2016). Transcriptome analysis of activated sludge microbiomes decoded the role of the nitrifying organisms in heavy oil degradation (Sato et al., 2019). Transcriptome analyses of crude oil degrading Pseudomonas aeruginosa strains revealed the significance of differentially expressed genes implicated in crude oil degradation (Das et al., 2020).
Proteomics
A proteome is the set of proteins formed inside a cell, tissue, organ, or organism. The branch of science that explores and study of proteomes is called proteomics. The proteomic analysis helps in decoding molecular mechanisms, metabolic pathways, post-translational modifications, etc. inside the cell. It has enabled the tracking and analysis of universal expression of proteins in microorganisms residing in contaminated areas due to human-induced activities (Kim et al., 2004). Meta proteomics or community proteomics is the analysis of complete protein profile from microbial communities inhabiting in a specific environment (Hart et al., 2018). It is also defined as a functional genomics approach because it helps in exploring the protein expression pattern of one organism and helps in generating a protein map of all expressed proteins by one organism thriving in a selected environment. Metaproteomic analyses are dependent on the effectiveness of proteins extraction, the techniques adopted for proteins fractionation from an intricate mixture, and the explicit identification of fractionated peptides/proteins, database search, and data interpretation (Verberkmoes et al., 2009). Apart from this potent quantification methods are essential to relate expression patterns of proteins under different conditions. It relies on techniques like two-dimensional electrophoresis and mass spectroscopy to analyze the role of various microbial catabolic enzymes in biodegradation pathways (Wilmes and Bond, 2006; Meena and Swapnil, 2019).
For analyzing the proteome of the microbial communities from random environments following steps have been developed. Figure 6 shows the highlights of the general workflow of proteomic analysis for microbial community studies. Environmental samples being complex requires unambiguous strategies for sample collection or protein extraction. Two strategies have been developed for protein separation and identification. One strategy is based on one-dimensional or two-dimensional polyacrylamide gel electrophoresis (2-D PAGE). Proteolytic enzymes like trypsin is used to digest the excised targeted protein bands to peptides which are later analyzed by mass spectrometry or tandem MS analysis, database probing, and bioinformatic analysis (Wang et al., 2014). The second strategy is based on liquid chromatography coupled with MS/MS (LC-MS/MS) where proteases are used to digest the entire proteome into a complex peptide mixture and are purified by cation exchange chromatography or microcapillary reverse-phase. This method increases the proteome coverage, allows high-throughput recognition of proteins within a short duration (Verberkmoes et al., 2009), and allows detection of insoluble membrane proteins (Wu and Yates, 2003). The liquid chromatography centered methodology approach has become the cascade of microbial community proteomic studies. The disadvantage of this strategy is reproducibility, dynamic range, and database availability.
For comparative and quantitative proteomics data with high reproducibility and precision is useful. It aims to determine the divergence in protein expression in various environmental gradients (e.g., nutrient and salinity gradients) as well as biological conditions like control vs. treatment, healthy vs. disease, specific genotype vs. wild type. Various labeling techniques have been developed for proteomics like stable isotope labeling using amino acids, peptides (Geiger et al., 2011; Lau et al., 2014), tandem mass tags (Ward et al., 2014), isotope dilution (Bettmer, 2010), isotope-coded affinity tags (Hägglund et al., 2008), isobaric tags for relative and absolute quantification (Martínez-Esteso et al., 2014). A label-free quantitative proteomic approach is possible with the progress in computational software which allows extensive profiling of proteins with flexibility. The label-free method is deployed on the abundance of proteins by comparing the peak intensity or the spectral count of the same peptide/protein. The mass-spectrometry based label-free approach is cost-effective, more prevalent, and has become the key research methodology in metaproteomics.
The metabolism of an organism is dependent on environmental factors. Changing external stimulus triggers changes in protein expression and assessing such changes can be effective in bioremediation approaches (Mattarozzi et al., 2017). Several studies report the characterization and quantification of proteins expressed by microorganisms present in diverse ecosystems like soil, marine, and freshwater environments, sediment, soil, activated sludge, acid mine drainage biofilms, human or animal microbiome and plant-associated microorganisms (Siggins et al., 2012; Wang et al., 2016). Metaproteome data from such ecosystems helps in understanding microbial community structure, dynamics, and functioning. Metaproteomic analysis together with community genomics has been reported to be a dynamic tool to discover microbial diversity and to differentiate closely related organisms in a biofilm colonizing acid mine drainage (Simmons et al., 2008; Denef et al., 2009, 2010). Metaproteomics was reportedly used to analyze an anaerobic microbial community degrading toluene (Jehmlich et al., 2010). Siggins et al. (2012) investigated the impact of temperature and exposure to trichloroethylene (TCE) on proteins expression by the microbial community in laboratory-scale anaerobic conditions.
Metaproteomics has also been reportedly used to distinguish metabolic activities of microbes significant to the bioremediation of contaminated environments. The expression of catabolic enzymes, such as catechol 2,3-dioxygenases, 1,2-cis-dihydrodiol dehydrogenase, and 2-hydroxymuconic semialdehyde was reported in Sphingomonadales and uncultured bacteria assisting the bioremediation of compost (Bastida et al., 2016). A culture-dependent community proteomic study reported that soil microbial community becomes convoluted in hydrocarbon-contaminated soil compared to untreated soil (Bastida et al., 2014).
It can be utilized to explain complex community interplay associated with in situ bioremediation of contaminated soil sites (Bastida et al., 2010). Morris et al. (2010) conducted a comparative metaproteomic study to investigate the membrane proteins expressed by the microbial communities from surface waters in the South Atlantic. A combination of metaproteomics with geochemical and biological data was used to examine the behavior of biofilm-forming microbial communities along environmental gradients. Jehmlich et al. (2010) reported the metaproteome of an anaerobic community, initiating from a batch sulfate reducing enrichment culture, exposed to toluene. A metaproteome analysis in bioremediation thus encompasses the identification of differentially expressed proteins and their respective genes, assessing changes in the abundance of biomarkers, protein structure, and function characterization.
The metabolic adaptation of Arthrobacter phenanthrenivorans Sphe3 supplemented with phenanthrene, phthalate, glucose as sole carbon and energy source was investigated using comparative quantitative proteomics. A gel-free quantitative proteomic analysis using nano LC-MS/MS was used to explore the phenanthrene catabolic pathway as well as the fluctuations in proteins when A. phenanthrenivorans Sphe3 cells were grown on various substrates (Vandera et al., 2015). A proteomic study using nano LC-MS/MS has contributed awareness into the metabolic and regulatory networks of Psuedomonas aeruginosa san ai exposed to plastic additive 2,6-di-tert-butylphenol (Medić et al., 2019). LC–MS/MS shotgun proteomics was reportedly used to determine variations in the proteome of hydrocarbon-degrading psychrophile Oleispira antarctica RB-8 when grown on n-alkanes in cold temperatures (Gregson et al., 2020).
Metabolomics
A metabolome is the total metabolites in an organism and the study of the metabolite profile of a cell within a given condition is called metabolomics (Beale et al., 2017). A cell produces a range of primary and secondary metabolites when subjected to external stress which has enabled us to understand and analyze the effect of environmental conditions on the metabolome of organisms (Malla et al., 2018). Metabolome based approaches have facilitated to establish models that can predict microbial activities under bioremediation strategies. It permits us to comprehend the potent activities of the microbial communities and their functional inputs to the environments in which they flourish. Various spectroscopic techniques can be used to characterize metabolites produced by organisms (Bargiela et al., 2015).
Metabolomics analyzes the metabolites produced by the cell in response to changing environmental conditions which in turn provide information about the regulatory events in a cell (Krumsiek et al., 2015). These metabolites can be used as bioindicators to screen the biological effects of the contaminants waste for a better perception of the environment. Advances in analytical instruments, statistical tools and bioinformatics tools made access to assess, extract, and interpret various metabolites and elucidate their pathway (Hill et al., 2015). The major strategies to analyze the metabolome involve metabolite profiling, targeted analysis, and metabolic fingerprinting (Wang et al., 2010). Metabolite profiling is a semi-quantitative process that enables the detection of both known and unknown metabolites while target analysis is a quantitative approach that uses various detection techniques to detect metabolites involved in a specific pathway. Metabolic fingerprinting is a high throughput procedure that generates fingerprints of specific metabolites in a sample.
A metabolomics analysis workflow starts with sample acquisition and preparation followed by separation and detection of analytes. Detection and quantification of metabolites is normally accomplished through an amalgamation of chromatography techniques (liquid chromatography and gas chromatography) and detection systems like mass spectrometry and nuclear magnetic resonance (Aldridge and Rhee, 2014). The most extensively deployed analytical instruments in metabolomics research are nuclear magnetic resonance spectrometers and mass spectrometry (Healy et al., 1995; Pan and Raftery, 2007). Among the mass spectrometry (MS) technology liquid chromatography MS is widely used due to lesser ion suppression, higher resolution, and non-requirement of chemical derivatization steps. Mass spectrometry produces spectra comprising of a pattern of peaks that can be used to analyze and quantify metabolites. These patterns are saved in spectral databases, allowing automated analysis, and generation of metabolomic profiles. Two methods are commonly used to analyze metabolic data. The first method uses statistics and clustering algorithms while the second method makes use of networks to envisage the spatial and temporal properties of the data. Several software tools like MetaboAnalyst, XCMS, MetATT, Matlab, etc. are used for metabolomics data analysis. Apart from data analysis several network visualization software tools like MetScape Plugin, MetaMapp, MAVEN, Pathomx, etc. have been developed to visualize graphical representations of metabolic pathways as diagrams or heat maps (Hill et al., 2015).
Mallick et al. (2019) used a computational framework called Model-based Genomically Informed High-dimensional Predictor of Microbial Community Metabolic Profiles (MelonnPan) to predict community metabolomes from a microbial community. This approach predicted and correlated the possible metabolic profiles across many samples at low cost compared to metabolomics, thus offering new opportunities for economical study designs. It also enabled the identification of functionally pertinent gene families with species or strain-specific metabolic associations, expediting biologically significant mechanisms at finer taxonomic resolution. This analysis in the future may thus provide metabolic understandings and hypothesis generation in numerous samples for which only metagenomic data are currently available.
Metabolomics along with bioinformatics tools and databases have enabled a better understanding of microbial community, their catabolic pathways, and genes responsible for encoding catabolic enzymes. It is thus a powerful strategy for discovering novel metabolic pathways and characterizing metabolic networks (Villas-Bôas et al., 2007; Kim et al., 2016; McMahon et al., 2018). Metagenomic, geochemical, and metabolomic analyses were used to study the role of microbial communities in the degradation of detrital biomass and petroleum hydrocarbons (Keum et al., 2008; Dong et al., 2019). Targeted and untargeted metabolomics using LC/MS/MS system was used to investigate reprogrammed-metabolism underlying biofilm formation for deciphering the metabolism for biofilm formation (Lu et al., 2019). Proteogenomic and metabolomic approaches were used to identify the pathways and enzymes used by marine bacteria Mycobacterium sp. DBP42 and Halomonas sp. ATBC28 to degrade plasticizers like dibutyl phthalate (DBP), bis(2-ethyl hexyl) phthalate (DEHP), and acetyl tributyl citrate (ATBC) (Wright et al., 2020).
Metabolite pathway databases and repositories can be used to supervise, investigate, and disseminate information about metabolites and their pathways. They provide a databank on metabolic information as well as helps in the unification of complex data into metabolic pathways. These databases and repositories also help in modeling metabolic pathways that can be investigated and prompted using mathematical modeling techniques. A variety of pathway databases and repositories, such as Reactome, KEGG (Kyoto Encyclopedia of Genes and Genomes), PANTHER pathway, etc. are available which provide information about specific metabolite pathways, reactions and enzymes, names, chemical structures, molecular weight, the occurrence of metabolites in pathways, etc. (Hill et al., 2015). Metabolomics is thus promptly expanding omic technology that can provide integrated insight into various metabolites and metabolic pathways. The collective uses of modern instrumental analytical methodologies, bioinformatics softwares have unraveled the detection of metabolites that cannot be accomplished by single-analysis techniques. Advancement in analytical platforms will boost the incorporation of metabolomics into systems biology.
Fluxomics
Fluxomics is a quantitative approach that studies the rates of metabolic reactions, changes in metabolic rates inside a biological individual. Fluxome is the complete set of metabolic fluxes in a cell which provides facts on several cellular processes, thus it is a distinctive phenotypic characteristic of cells. Flux analysis offers vital information about the phenotype since it assesses the metabolome in its functional communications with the genome and environment (Dettmer et al., 2007). Fluxomic approaches analyze metabolic interfaces prominent to the progression and logical design of cellular functions. Metabolic reaction rates evaluate the in vivo enzymatic activities that cannot be obtained from the transcriptomic, proteomic, or metabolomic data alone. A successful fluxomic analysis is dependent on the precision of quantitative metabolomics data, isotopic labeling measurements, and the reorganization of metabolic networks describing stoichiometry and regulation of metabolic reactions.
Two techniques commonly used for flux quantification are flux balance analysis or stoichiometric metabolic flux analysis and tracer-based metabolomics which uses a firm isotope of carbon 13C to trace the carbon distributing through diverse pathways followed by analytical techniques like nuclear magnetic resonance (NMR) or mass spectroscopy (MS) analysis for identification and characterization of the labeled compounds. Flux balance analysis uses the stoichiometry of metabolic reactions in concurrence with biological, chemical, and thermodynamic parameters to produce a controlled model of metabolic flux. In 13C isotope labeling, cells are fed with 13C labeled substrates which can be metabolized (e.g., Glucose) resulting in metabolites containing 13C atoms. Depending on the metabolic pathway 13C atoms are amalgamated into the newly designed metabolites in diverse positions and numbers. Therefore, for every single metabolite, there may be various isotopomers (numerous molecules of the same metabolite with different labeling states). Metabolomic analytical platforms are used to regulate the concentration and isotopomeric dissemination of these metabolites (Lee and Go, 2005).
The comparative and analytical approaches have been used as valid methods to estimate flux ratios. Tracer-based metabolomics data can be used to analyze metabolic flux distribution by using the comparative analytical approach and the integrative approach. Comparative analysis of tracer-based metabolomics data is also known as MIDA (mass isotopomer distribution analysis) which does not require bioinformatics tools and requires minimal statistics knowledge. It is a functional tool for the characterization of cell metabolic flux distribution by comparing the labeling patterns obtained with appropriate labeling distributions depending on the metabolic pathway followed by the tracer (Hellerstein and Neese, 1999; Kumari et al., 2018; Barupal et al., 2020). It provides predictable estimates of relative metabolic flux distributions. The perceptive nature of this approach has contributed to its extensive use in the interpretation of tracer-based experiments. This approach suffers the limitation that it does not allow the full characterization of the fluxome.
The integrative approach uses the integration of tracer-based metabolomic data into software packages to estimate the flux distribution by fitting the experimental data into the framework of more complete metabolic models (Wiechert, 2001). The 13C-based metabolic flux investigation has been used mainly to quantify the intracellular fluxes of an extensive range of microorganisms (Sauer, 2006). Software packages like 13C-FLUX have been developed which calculate sets of fluxes (Wiechert et al., 2001). The fluxome profile evaluation of marine bacteria Shewanella using biochemical, GC-MS analyzes, statistical and genetic algorithms revealed the presence of co-metabolic routes for the biodegradation of lethal metals, halogenated organic compounds, and radionuclides. The bacteria exhibited a responsive metabolic flux when revealed toward different carbon sources (Tang et al., 2007). Consolidated molecular diagnostic and kinetic modeling enhanced cognizance about organohalide-respiring bacteria (ORB) and their metabolite dynamics in an in-situ dechlorinating bioreactor (Maphosa et al., 2010b). Metabolic flux investigation revealed valuable evidence of a phenol overproducing strain resulting from Psuedomonas putida S12 during metabolic engineering (Wierckx et al., 2008, 2009). Metabolic flux analysis was used to investigate the constitutive metabolic network for the co-utilization of sugar and aromatic carbons in Pseudomonas putida (Kukurugya et al., 2019). Flux distributions using 13C-MFA (Metabolic flux analysis) was used to identify the effect of phenol on the carbon metabolism in wild type E. coli cultured under varying phenol concentrations (Kitamura et al., 2019).
Flux analysis can provide strategies for optimizing a biological system. Fluxomics is expected to progress and provide significant novel results for the development of biological systems and systems biology. Technical difficulties in the development of flux analysis and intricate metabolic regulatory mechanisms have inadequate the opportunity of fluxomics. It is thus essential to combine high throughput “omics” and molecular biology techniques to insistence and clarify challenges in the fluxomics for its future development.
Conclusion
The immense threat posed to the environment due to anthropogenic activities has led to think about novel strategies for decontamination and clean up. It is challenging to realize and discover the interplay between the microbial communities in polluted environments. Modern era omic-approaches like genomics, proteomics, transcriptomics, metabolomics, and fluxomics have eliminated the boundaries to study the mechanisms involved in various bioremediation pathways. It has enabled to incorporate new strategies for efficient bioremediation process. Omics-approaches have the potency to anticipate microbial metabolism in polluted environments. These high-throughput analyses would aid in tracking novel organisms for bioremediation, provide excellent and novel insights into their key biodegradative pathways at the molecular level. These multi-omics approaches will enable us to make new hypotheses, theories, and archetypes for bioremediation of polluted surroundings.
Author Contributions
MM and HC conceived the idea of the review, provided the general concept and inputs for each specific section, drafted part of the manuscript, and wrote the review after collecting literature. MM edited, compiled, and finalized the draft. HC, MM, and KS read and approved it for publication. All authors contributed to the article and approved the submitted version.
Conflict of Interest
The authors declare that the research was conducted in the absence of any commercial or financial relationships that could be construed as a potential conflict of interest.
Acknowledgments
MM was thankful to Mohanlal Sukhadia University, Udaipur for providing the necessary facilities during the course of study. This study was supported by Startup Research Grant (UGC Faculty Research Promotion Scheme; FRPS) and sustained by Mohanlal Sukhadia University, Udaipur, Rajasthan, India.
References
Abou Seeda, M. A., Yassen, A. A., and Abou El-Nour, E. Z. A. A. (2017). Microorganism as a tool of bioremediation technology for cleaning waste and industrial water. Biosci. Res. 14, 633–636.
Adessi, C., Matton, G., Ayala, G., Turcatti, G., Mermod, J. J., Mayer, P., et al. (2000). Solid phase DNA amplification: characterisation of primer attachment and amplification mechanisms. Nucleic Acids Res. 28:E87. doi: 10.1093/nar/28.20.e87
Aldridge, B. B., and Rhee, K. Y. (2014). Microbial metabolomics: innovation, application, insight. Curr. Opin. Microbiol. 19, 90–96. doi: 10.1016/j.mib.2014.06.009
Arazoe, T., Kondo, A., and Nishida, K. (2018). Targeted nucleotide editing technologies for microbial metabolic engineering. Biotechnol. J. 13:e1700596. doi: 10.1002/biot.201700596
Aubé, J., Senin, P., Bonin, P., Pringault, O., Jeziorski, C., Bouchez, O., et al. (2020). Meta-omics provides insights into the impact of hydrocarbon contamination on microbial mat functioning. Microb. Ecol. 80, 286–295. doi: 10.1007/s00248-020-01493-x
Awasthi, M. K., Ravindran, B., Sarsaiya, S., Chen, H., Wainaina, S., Singh, E., et al. (2020). Metagenomics for taxonomy profiling: tools and approaches. Bioengineered 11, 356–374. doi: 10.1080/21655979.2020.1736238
Bahamonde, P. A., Feswick, A., Isaacs, M. A., Munkittrick, K. R., and Martyniuk, C. J. (2016). Defining the role of omics in assessing ecosystem health: perspectives from the Canadian environmental monitoring program. Environ. Toxicol. Chem. 35, 20–35. doi: 10.1002/etc.3218
Bargiela, R., Herbst, F. A., Martínez-Martínez, M., Seifert, J., Rojo, D., Cappello, S., et al. (2015). Metaproteomics and metabolomics analyses of chronically petroleum-polluted sites reveal the importance of general anaerobic processes uncoupled with degradation. Proteomics 15, 3508–3520. doi: 10.1002/pmic.201400614
Barupal, T., Chittora, D., and Meena, M. (2019a). “Solid waste: characterization, assessment, monitoring, and remediation,” in Advances in Waste-to-Energy Technologies, eds R. P. Singh, V. Prasad, and B. Vaish (Boca Raton; London; New York, NY: Taylor & Francis; CRC Press), 1–19. doi: 10.1201/9780429423376-1
Barupal, T., Meena, M., and Sharma, K. (2019b). Inhibitory effects of leaf extract of Lawsonia inermis on Curvularia lunata and characterization of novel inhibitory compounds by GC–MS analysis. Biotechnol. Rep. 23:e00335. doi: 10.1016/j.btre.2019.e00335
Barupal, T., Meena, M., and Sharma, K. (2020). A study on preventive effects of Lawsonia inermis L. bioformulations against leaf spot disease of maize. Biocatal. Agric. Biotechnol. 23:101473. doi: 10.1016/j.bcab.2019.101473
Bastida, F., Hernandez, T., and Garcia, C. (2014). Metaproteomics of soils from semiarid environment: functional and phylogenetic information obtained with different protein extraction methods. J. Proteomics 101, 31–42. doi: 10.1016/j.jprot.2014.02.006
Bastida, F., Jehmlich, N., Lima, K., Morris, B. E. L., Richnow, H. H., Hernández, T., et al. (2016). The ecological and physiological responses of the microbial community from a semiarid soil to hydrocarbon contamination and its bioremediation using compost amendment. J. Proteomics 135, 162–169. doi: 10.1016/j.jprot.2015.07.023
Bastida, F., Nicolás, C., Moreno, J., Hernández, T., and Garcia, C. (2010). Tracing changes in the microbial community of a hydrocarbon-polluted soil by culture-dependent proteomics. Pedosphere 20, 479–485. doi: 10.1016/S1002-0160(10)60037-9
Beale, D. J., Karpe, A. V., Ahmed, W., Cook, S., Morrison, P. D., Staley, C., et al. (2017). A community multi-omics approach towards the assessment of surface water quality in an urban river system. Int. J. Environ. Res. Public Health 14:E303. doi: 10.3390/ijerph14030303
Bentley, D. R., Balasubramanian, S., Swerdlow, H. P., Smith, G. P., Milton, J., Brown, C. B., et al. (2008). Accurate whole human genome sequencing using reversible terminator chemistry. Nature 456, 53–59. doi: 10.1038/nature07517
Bettmer, J. (2010). Application of isotope dilution ICP-MS techniques to quantitative proteomics. Anal. Bioanal. Chem. 397, 3495–3502. doi: 10.1007/s00216-010-3861-y
Bier, E., Harrison, M. M., O'Connor-Giles, K. M., and Wildonger, J. (2018). Advances in engineering the fly genome with the CRISPR-Cas system. Genetics 208, 1–18. doi: 10.1534/genetics.117.1113
Bilal, T., Malik, B., and Hakeem, K. R. (2018). Metagenomic analysis of uncultured microorganisms and their enzymatic attributes. J. Microbiol. Methods 155, 65–69. doi: 10.1016/j.mimet.2018.11.014
Braslavsky, I., Hebert, B., Kartalov, E., and Quake, S. R. (2003). Sequence information can be obtained from single DNA molecules. Proc. Natl. Acad. Sci. U.S.A. 100, 3960–3964. doi: 10.1073/pnas.0230489100
Bursle, E., and Robson, J. (2016). Non-culture methods for detecting infection. Aust. Prescr. 39, 171–175. doi: 10.18773/austprescr.2016.059
Callaghan, A. V. (2013). Metabolomic investigations of anaerobic hydrocarbon-impacted environments. Curr. Opin. Biotechnol. 24, 506–515. doi: 10.1016/j.copbio.2012.08.012
Cárdenas Espinosa, M. J., Colina Blanco, A., Schmidgall, T., Atanasoff-Kardjalieff, A. K., Kappelmeyer, U., Tischler, D., et al. (2020). Toward biorecycling: isolation of a soil bacterium that grows on a polyurethane oligomer and monomer. Front. Microbiol. 11:404. doi: 10.3389/fmicb.2020.00404
Chakraborty, R., Wu, C. H., and Hazen, T. C. (2012). Systems biology approach to bioremediation. Curr. Opin. Biotechnol. 23, 483–490. doi: 10.1016/j.copbio.2012.01.015
Chandran, H., Meena, M., Barupal, T., and Sharma, K. (2020). Plant tissue culture as a perpetual source for production of industrially important bioactive compounds. Biotechnol. Rep. 26:e00450. doi: 10.1016/j.btre.2020.e00450
Chen, Y., and Murrell, J. C. (2010). When metagenomics meets stable-isotope probing: progress and perspectives. Trends Microbiol. 18, 157–163. doi: 10.1016/j.tim.2010.02.002
Chittora, D., Meena, M., Barupal, T., Swapnil, P., and Sharma, K. (2020). Cyanobacteria as a source of biofertilizers for sustainable agriculture. Biochem. Biophys. Rep. 22:100737. doi: 10.1016/j.bbrep.2020.100737
Cho, J. C., and Tiedje, J. M. (2001). Bacterial species determination from DNA-DNA hybridization by using genome fragments and DNA microarrays. Appl. Environ. Microbiol. 67, 3677–3682. doi: 10.1128/AEM.67.8.3677-3682.2001
Cho, J. C., and Tiedje, J. M. (2002). Quantitative detection of microbial genes by using DNA microarrays. Appl. Environ. Microbiol. 68, 1425–1430. doi: 10.1128/AEM.68.3.1425-1430.2002
Clarridge, J. E. (2004). Impact of 16S rRNA gene sequence analysis for identification of bacteria on clinical microbiology and infectious diseases. Clin. Microbiol. Rev. 17, 840–862. doi: 10.1128/CMR.17.4.840-862.2004
Dai, Z., Zhang, S., Yang, Q., Zhang, W., Qian, X., Dong, W., et al. (2018). Genetic tool development and systemic regulation in biosynthetic technology. Biotechnol. Biofuels 11:152. doi: 10.1186/s13068-018-1153-5
Daniel, R. (2005). The metagenomics of soil. Nat. Rev. Microbiol. 3, 470–478. doi: 10.1038/nrmicro1160
Das, D., Mawlong, G. T., Sarki, Y. N., Singh, A. K., Chikkaputtaiah, C., and Boruah, H. P. D. (2020). Transcriptome analysis of crude oil degrading Pseudomonas aeruginosa strains for identification of potential genes involved in crude oil degradation. Gene 755:144909. doi: 10.1016/j.gene.2020.144909
De Sousa, C. S., Hassan, S. S., Pinto, A. C., Silva, W. M., De Almeida, S. S., Soares, S. D. C., et al. (2018). “Microbial omics: applications in biotechnology,” in Omics Technologies and Bio-Engineering, eds D. Barh and V. Azevedo (Cambridge, MA: Academic Press), 3–20. doi: 10.1016/B978-0-12-815870-8.00001-2
Denef, V. J., Kalnejais, L. H., Mueller, R. S., Wilmes, P., Baker, B. J., Thomas, B. C., et al. (2010). Proteogenomic basis for ecological divergence of closely related bacteria in natural acidophilic microbial communities. Proc. Natl. Acad. Sci. U.S.A. 107, 2383–2390. doi: 10.1073/pnas.0907041107
Denef, V. J., VerBerkmoes, N. C., Shah, M. B., Abraham, P. E., Lefsrud, M., Hettich, R. L., et al. (2009). Proteomics-inferred genome typing (PIGT) demonstrates inter-population recombination as a strategy for environmental adaptation. Environ. Microbiol. 11, 313–325. doi: 10.1111/j.1462-2920.2008.01769.x
Dennis, P., Edwards, E. A., Liss, S. N., and Fulthorpe, R. (2003). Monitoring gene expression in mixed microbial communities by using DNA microarrays. Appl. Environ. Microbiol. 69, 769–778. doi: 10.1128/AEM.69.2.769-778.2003
Desai, C., Pathak, H., and Madamwar, D. (2010). Advances in molecular and ‘-omics’ technologies to gauge microbial communities and bioremediation at xenobiotic/anthropogen contaminated sites. Bioresour. Technol. 101, 1558–1569. doi: 10.1016/j.biortech.2009.10.080
Dettmer, K., Aronov, P. A., and Hammock, B. D. (2007). Mass spectrometry based metabolomics. Mass Spectrom. Rev. 26, 51–58. doi: 10.1002/mas.20108
Dickson, R. P., Erb-Downward, J. R., Prescott, H. C., Martinez, F. J., Curtis, J. L., Lama, V. N., et al. (2014). Analysis of culture-dependent versus culture-independent techniques for identification of bacteria in clinically obtained bronchoalveolar lavage fluid. J. Clin. Microbiol. 52, 3605–3613. doi: 10.1128/JCM.01028-14
Dietrich, J. A., McKee, A. E., and Keasling, J. D. (2010). High-throughput metabolic engineering: advances in small-molecule screening and selection. Annu. Rev. Biochem. 79, 563–590. doi: 10.1146/annurev-biochem-062608-095938
Dinsdale, E. A., Edwards, R. A., Hall, D., Angly, F., Breitbart, M., Brulc, J. M., et al. (2008). Functional metagenomic profiling of nine biomes. Nature 452, 629–632. doi: 10.1038/nature06810
Dong, X., Greening, C., Rattray, J. E., Chakraborty, A., Chuvochina, M., Mayumi, D., et al. (2019). Metabolic potential of uncultured bacteria and archaea associated with petroleum seepage in deep-sea sediments. Nat. Commun. 10:1816. doi: 10.1038/s41467-019-09747-0
Eid, J., Fehr, A., Gray, J., Luong, K., Lyle, J., Otto, G., et al. (2009). Real-time DNA sequencing from single polymerase molecules. Science 323, 133–138. doi: 10.1126/science.1162986
El-Metwally, S., Ouda, O. M., and Helmy, M. (2014). Next Generation Sequencing Technologies and Challenges in Sequence Assembly. New York, NY: Springer Verlag.
Eyers, L., George, I., Schuler, L., Stenuit, B., Agathos, S. N., and El Fantroussi, S. (2004). Environmental genomics: exploring the unmined richness of microbes to degrade xenobiotics. Appl. Microbiol. Biotechnol. 66, 123–130. doi: 10.1007/s00253-004-1703-6
Fedurco, M., Romieu, A., Williams, S., Lawrence, I., and Turcatti, G. (2006). BTA, a novel reagent for DNA attachment on glass and efficient generation of solid-phase amplified DNA colonies. Nucleic Acids Res. 34:e22. doi: 10.1093/nar/gnj023
Felczykowska, A., Krajewska, A., Zielińska, S., Łoś, J. M., Bloch, S. K., and Nejman-Faleńczyk, B. (2015). The most widespread problems in the function-based microbial metagenomics. Acta Biochim. Pol. 62, 161–166. doi: 10.18388/abp.2014_917
Ferrer, M., Beloqui, A., Vieites, J. M., Guazzaroni, M. E., Berger, I., and Aharoni, A. (2009). Interplay of metagenomics and in vitro compartmentalization. Microb. Biotechnol. 2, 31–39. doi: 10.1111/j.1751-7915.2008.00057.x
Gao, H., Wang, Y., Liu, X., Yan, T., Wu, L., Alm, E., et al. (2004). Global transcriptome analysis of the heat shock response of Shewanella oneidensis. J. Bacteriol. 186, 7796–7803. doi: 10.1128/JB.186.22.7796-7803.2004
Garrido-Sanz, D., Redondo-Nieto, M., Guirado, M., Pindado Jiménez, O., Millán, R., Martin, M., et al. (2019). Metagenomic insights into the bacterial functions of a diesel-degrading consortium for the rhizoremediation of diesel-polluted soil. Genes 10:456. doi: 10.3390/genes10060456
Gaytán, I., Sánchez-Reyes, A., Burelo, M., Vargas-Suárez, M., Liachko, I., and Press, M. (2020). Degradation of recalcitrant polyurethane and xenobiotic additives by a selected landfill microbial community and its biodegradative potential revealed by proximity ligation-based metagenomic analysis. Front. Microbiol. 10:2986. doi: 10.3389/fmicb.2019.02986
Geiger, T., Wisniewski, J. R., Cox, J., Zanivan, S., Kruger, M., Ishihama, Y., et al. (2011). Use of stable isotope labeling by amino acids in cell culture as a spike-in standard in quantitative proteomics. Nat. Protoc. 6, 147–157. doi: 10.1038/nprot.2010.192
Ghazanfar, S., Azim, A., Ghazanfar, M. A., Anjum, M. I., and Begum, I. (2010). Metagenomics and its application in soil microbial community studies: biotechnological prospects. J. Anim. Plant Sci. 6, 611–622.
Gilbert, J. A., and Dupont, C. L. (2011). Microbial metagenomics: beyond the genome. Annu. Rev. Mar. Sci. 3, 347–371. doi: 10.1146/annurev-marine-120709-142811
Godzik, A. (2011). Metagenomics and the protein universe. Curr. Opin. Struct. Biol. 21, 398–403. doi: 10.1016/j.sbi.2011.03.010
Gołebiewski, M., and Tretyn, A. (2020). Generating amplicon reads for microbial community assessment with next generation sequencing. J. Appl. Microbiol. 128, 330–354. doi: 10.1111/jam.14380
Greene, E. A., and Voordouw, G. (2003). Analysis of environmental microbial communities by reverse sample genome probing. J. Microbiol. Methods 53, 211–219. doi: 10.1016/S0167-7012(03)00024-1
Gregson, B. H., Metodieva, G., Metodiev, M. V., Golyshin, P. N., and McKew, B. A. (2020). Protein expression in the obligate hydrocarbon-degrading psychrophile Oleispira antarctica RB-8 during alkane degradation and cold tolerance. Environ. Microbiol. 22, 1870–1883. doi: 10.1111/1462-2920.14956
Gupta, K., Biswas, R., and Sarkar, A. (2020). “Advancement of omics: prospects for bioremediation of contaminated soils,” in Microbial Bioremediation & Biodegradation, ed M. Shah (Singapore: Springer), 113–42.
Gutierrez, D. B., Gant-Branum, R. L., Romer, C. E., Farrow, M. A., Allen, J. L., Dahal, N., et al. (2018). An integrated, high-throughput strategy for “multi-omic” systems level analysis. J. Proteome Res. 17, 3396–3408. doi: 10.1021/acs.jproteome.8b00302
Gutleben, J., Chaib De Mares, M., van Elsas, J. D., Smidt, H., Overmann, J., and Sipkema, D. (2018). The multi-omics promise in context: From sequence to microbial isolate. Crit. Rev. Microbiol. 44, 212–229. doi: 10.1080/1040841X.2017.1332003
Hägglund, P., Bunkenborg, J., Maeda, K., and Svensson, B. (2008). Identification of thioredoxin disulfide targets using a quantitative proteomics approach based on isotope-coded affinity tags. J. Proteome Res. 7, 5270–5276. doi: 10.1021/pr800633y
Hallin, P. F., Binnewies, T. T., and Ussery, D. W. (2008). The genome BLAST atlas-a Gene Wiz extension for visualization of whole-genome homology. Mol. Biosyst. 4, 363–371. doi: 10.1039/b717118h
Han, D., Gao, P., Li, R., Tan, P., Xie, J., Zhang, R., et al. (2020). Multicenter assessment of microbial community profiling using 16S rRNA gene sequencing and shotgun metagenomic sequencing. J. Adv. Res. doi: 10.1016/j.jare.2020.07.010
Handelsman, J. (2004). Metagenomics: application of genomics to uncultured microorganisms. Microbiol. Mol. Bio. Rev. 68, 669–685. doi: 10.1128/MMBR.68.4.669-685.2004
Hart, E. H., Creevey, C. J., Hitch, T., and Kingston-Smith, A. H. (2018). Meta-proteomics of rumen microbiota indicates niche compartmentalisation and functional dominance in a limited number of metabolic pathways between abundant bacteria. Sci. Rep. 8:10504. doi: 10.1038/s41598-018-28827-7
He, Z., Deng, Y., van Nostrand, J. D., Xu, M., Hemme, L. H., Tu, Q., et al. (2010). GeoChip 3.0 as a high-throughput tool for analyzing microbial community composition, structure and functional activity. ISME J. 4, 1167–1179. doi: 10.1038/ismej.2010.46
He, Z., Zhang, P., Wu, L., Rocha, A. M., Tu, Q., Shi, Z., et al. (2018). Microbial functional gene diversity predicts groundwater contamination and ecosystem functioning. mBio. 9:e02435-17. doi: 10.1128/mBio.02435-17
Head, I. M., Singleton, I., and Milner, M. G. (2003). Bioremediation: A Critical Review. Wymondham: Horizon Scientific Press.
Healy, F. G., Ray, R. M., Aldrich, H. C., Wilkie, A. C., Ingram, L. O., and Shanmugam, K. T. (1995). Direct isolation of functional genes encoding cellulases from the microbial consortia in a thermophilic, anaerobic digester maintained on lignocelluloses. Appl. Microbiol. Biotechnol. 43, 667–674. doi: 10.1007/BF00164771
Hellerstein, M. K., and Neese, R. A. (1999). Mass isotopomer distribution analysis at eight years: theoretical, analytic, and experimental considerations. Am. J. Physiol. 276, E1146–E1170. doi: 10.1152/ajpendo.1999.276.6.E1146
Hill, C. B., Czauderna, T., Klapperstück, M., Roessner, U., and Schreiber, F. (2015). Metabolomics, standards, and metabolic modeling for synthetic biology in plants. Front. Bioeng. Biotechnol. 3:167. doi: 10.3389/fbioe.2015.00167
Hillmann, B., Al-Ghalith, G. A., Shields-Cutler, R. R., Zhu, Q., Gohl, D. M., Beckman, K. B., et al. (2018). Evaluating the information content of shallow shotgun metagenomics. mSystems 3:e00069-18. doi: 10.1128/mSystems.00069-18
Hodkinson, B. P., and Grice, E. A. (2015). Next-generation sequencing: a review of technologies and tools for wound microbiome research. Adv. Wound Care 4, 50–58. doi: 10.1089/wound.2014.0542
Igiri, B. E., Okoduwa, S. I. R., Idoko, G. O., Akabuogu, E. P., Adeyi, A. O., and Ejiogu, I. K. (2018). Toxicity and bioremediation of heavy metals contaminated ecosystem from tannery wastewater: a review. J. Toxicol. 2018:2568038. doi: 10.1155/2018/2568038
Iwamoto, T., and Nasu, M. (2001). Current bioremediation practice and perspective. J. Biosci. Bioeng. 92, 1–8. doi: 10.1016/S1389-1723(01)80190-0
Jain, M., Hugh, E. O., Paten, B., and Akeson, M. (2016). The oxford nanopore MinION: Delivery of nanopore sequencing to the genomics community. Genome Biol. 17:239. doi: 10.1186/s13059-016-1103-0
Jeffries, T. C., Rayu, S., Nielsen, U. N., Lai, K., Ijaz, A., Nazaries, L., et al. (2018). Metagenomic functional potential predicts degradation rates of a model organophosphorus xenobiotic in pesticide contaminated soils. Front. Microbiol. 9:147. doi: 10.3389/fmicb.2018.00147
Jehmlich, N., Kleinsteuber, S., Vogt, C., Benndorf, D., Harms, H., Schmidt, F., et al. (2010). Phylogenetic and proteomic analysis of an anaerobic toluene-degrading community. J. Appl. Microbiol. 109, 1937–1945. doi: 10.1111/j.1365-2672.2010.04823.x
Kapley, A., Prasad, S., and Purohit, H. J. (2007). Changes in microbial diversity in fed-batch reactor operation with wastewater containing nitroaromatic residues. Bioresour. Technol. 98, 2479–2484. doi: 10.1016/j.biortech.2006.09.012
Katsaveli, K., Vayenas, D., Tsiamis, G., and Bourtzis, K. (2012). Bacterial diversity in Cr(VI) and Cr(III)-contaminated industrial wastewaters. Extremophiles 16, 285–296. doi: 10.1007/s00792-012-0429-0
Kchouk, M., Gibrat, J. F., and Elloumi, M. (2017). Generations of sequencing technologies: from first to next generation. Biol. Med. 9:395. doi: 10.4172/0974-8369.1000395
Keum, Y. S., Seo, J. S., Li, Q. X., and Kim, J. H. (2008). Comparative metabolomic analysis of Sinorhizobium sp. C4 during the degradation of phenanthrene. Appl. Microbiol. Biotechnol. 80, 863–872. doi: 10.1007/s00253-008-1581-4
Kim, H., Kwon, K. K., Seong, W., and Lee, S. G. (2016). Multi-enzyme screening using a high-throughput genetic enzyme screening system. J. Vis. Exp. 114:54059. doi: 10.3791/54059
Kim, H. J., Ishidou, E., Kitagawa, E., Momose, Y., and Iwahashi, H. (2004). A yeast DNA microarray for the evaluation of toxicity in environmental water containing burned ash. Environ. Monit. Assess. 92, 253–272. doi: 10.1023/B:EMAS.0000014504.03500.41
Kitamura, S., Toya, Y., and Shimizu, H. (2019). 13C-Metabolic flux analysis reveals effect of phenol on central carbon metabolism in Escherichia coli. Front. Microbiol. 10:1010. doi: 10.3389/fmicb.2019.01010
Kou, S., Vincent, G., Gonzalez, E., Pitre, F. E., Labrecque, M., and Brereton, N. J. B. (2018). The response of a 16S ribosomal RNA gene fragment amplified community to lead, zinc, and copper pollution in a Shanghai field trial. Front. Microbiol. 9:366. doi: 10.3389/fmicb.2018.00366
Krumsiek, J., Mittelstrass, K., Do, K. T., Stückler, F., Ried, J., Adamski, J., et al. (2015). Gender-specific pathway differences in the human serum metabolome. Metabolomics 11, 1815–1833. doi: 10.1007/s11306-015-0829-0
Kukurugya, M. A., Mendonca, C. M., Solhtalab, M., RWilkes, R. A., Thannhauser, T. W., and Aristilde, L. (2019). Multi-omics analysis unravels a segregated metabolic flux network that tunes co-utilization of sugar and aromatic carbons in Pseudomonas putida. J. Biol. Chem. 294, 8464–8479. doi: 10.1074/jbc.RA119.007885
Kumar, M., and Khanna, S. (2010). Diversity of 16S rRNA and dioxygenase genes detected in coal-tar-contaminated site undergoing active bioremediation. J. Appl. Microbiol. 108, 1252–1262. doi: 10.1111/j.1365-2672.2009.04523.x
Kumari, P., Meena, M., and Upadhyay, R. S. (2018). Characterization of plant growth promoting rhizobacteria (PGPR) isolated from the rhizosphere of Vigna radiata (mung bean). Biocatal. Agric. Biotechnol. 16, 155–162. doi: 10.1016/j.bcab.2018.07.029
Labie, D. (2007). Developmental neurotoxicity of industrial chemicals. Med. Sci. 23, 868–872. doi: 10.1051/medsci/20072310868
Lau, H. T., Suh, H. W., Golkowski, M., and Ong, S. E. (2014). Comparing SILAC- and stable isotope dimethyl-labeling approaches for quantitative proteomics. J. Proteome Res. 13, 4164–4174. doi: 10.1021/pr500630a
Lee, W. N., and Go, V. L. (2005). Nutrient-gene interaction: tracer-based metabolomics. J. Nutr. 135, 3027S−3032S. doi: 10.1093/jn/135.12.3027S
Liang, L., Stone, R. C., Stojadinovic, O., Ramirez, H., Pastar, I., Maione, A. G., et al. (2016). Integrative analysis of miRNA and mRNA paired expression profiling of primary fibroblast derived from diabetic foot ulcers reveals multiple impaired cellular functions. Wound Repair Regen. 24, 943–953. doi: 10.1111/wrr.12470
Lin, C. Y., Viant, M. R., and Tjeerdema, R. S. (2006). Metabolomics: methodologies and applications in the environmental sciences. J. Pestic. Sci. 31, 245–251. doi: 10.1584/jpestics.31.245
Liu, L., Li, Y. H., Li, S., Hu, N., He, Y., Pong, R., et al. (2012). Comparison of next-generation sequencing systems. J. Biomed. Biotechnol. 2012:251364. doi: 10.1155/2012/251364
Loman, N. J., and Pallen, M. J. (2015). Twenty years of bacterial genome sequencing. Nat. Rev. Microbiol. 13, 1–9. doi: 10.1038/nrmicro3565
Lovley, D. R. (2003). Cleaning up with genomics: applying molecular biology to bioremediation. Nat. Rev. Microbiol. 1, 35–44. doi: 10.1038/nrmicro731
Lovley, D. R., Phillips, E. J. P., Gorby, Y. A., and Landa, E. R. (1991). Microbial reduction of uranium. Nature 350, 413–416. doi: 10.1038/350413a0
Lu, H., Giordano, F., and Ning, Z. (2016). Oxford nanopore MinION sequencing and genome assembly. Genom. Proteom. Bioinfo. 14, 265–279. doi: 10.1016/j.gpb.2016.05.004
Lu, H., Que, Y., Wu, X., Guan, T., and Guo, H. (2019). Metabolomics deciphered metabolic reprogramming required for biofilm formation. Sci. Rep. 9:13160. doi: 10.1038/s41598-019-49603-1
Luciene, M. C., Helen, C. R., Luciana, M. C., Priscila, A. R., de Sousa Danielle, F. O. M., and Nívia, M. M. C. (2015). “Bioremediation of polluted waters using microorganisms,” in Advances in Bioremediation of Wastewater and Polluted Soil, ed N. Shiomi (London: IntechOpen), 1–22. doi: 10.5772/60770
Malik, S., Beer, M., Megharaj, M., and Naidu, R. (2008). The use of molecular techniques to characterize the microbial communities in contaminated soil and water. Environ. Int. 34, 265–276. doi: 10.1016/j.envint.2007.09.001
Malla, M. A., Dubey, A., Yadav, S., Kumar, A., Hashem, A., and Abd Allah, E. F. (2018). Understanding and designing the strategies for the microbe-mediated remediation of environmental contaminants using omics approaches. Front. Microbiol. 9:1132. doi: 10.3389/fmicb.2018.01132
Mallick, H., Franzosa, E. A., Mclver, L. J., Banerjee, S., Sirota-Madi, A., Kostic, A. D., et al. (2019). Predictive metabolomic profiling of microbial communities using amplicon or metagenomic sequences. Nat. Commun. 10:3136. doi: 10.1038/s41467-019-10927-1
Maphosa, F., de Vos, W. M., and Smidt, H. (2010a). Exploiting the ecogenomics toolbox for environmental diagnostics of organohalide-respiring bacteria. Trends Biotechnol. 28, 308–316. doi: 10.1016/j.tibtech.2010.03.005
Maphosa, F., Smidt, H., de Vos, W. M., and Roling, W. H. F. M. (2010b). Microbial community and metabolite dynamics of an anoxic dechlorinating bioreactor. Stud. Environ. Sci. 44, 4884–4890. doi: 10.1021/es903721s
Mardis, E. R. (2008). Next-generation DNA sequencing methods. Annu. Rev. Genomics Hum. Genet. 9, 387–402. doi: 10.1146/annurev.genom.9.081307.164359
Maroli, A. S., Gaines, T. A., Foley, M. E., Duke, S. O., Doğramacı, M., Anderson, J. V., et al. (2018). Omics in weed science: A perspective from genomics, transcriptomics, and metabolomics approaches. Weed Sci. 66, 681–695. doi: 10.1017/wsc.2018.33
Martínez-Esteso, M. J., Casado-Vela, J., Sellés-Marchart, S., Pedreño, M. A., and Bru-Martínez, R. (2014). Differential plant proteome analysis by isobaric tags for relative and absolute quantitation (iTRAQ). Methods Mol. Biol. 1072, 155–169. doi: 10.1007/978-1-62703-631-3_12
Mattarozzi, M., Manfredi, M., Montanini, B., Gosetti, F., Sanangelantoni, A. M., Marengo, E., et al. (2017). A metaproteomic approach dissecting major bacterial functions in the rhizosphere of plants living in serpentine soil. Anal. Bioanal. Chem. 409, 2327–2339. doi: 10.1007/s00216-016-0175-8
Maxam, A. M., and Gilbert, W. A. (1977). A new method for sequencing DNA. Proc. Natl. Acad. Sci. U.S.A. 74, 560–564. doi: 10.1073/pnas.74.2.560
McMahon, M. A., Prakash, T. P., Cleveland, D. W., Bennett, C. F., and Rahdar, M. (2018). Chemically modified Cpf1-CRISPR RNAs mediate efficient genome editing in mammalian cells. Mol. Ther. 26, 1228–1240. doi: 10.1016/j.ymthe.2018.02.031
Medić, A., Stojanović, K., Izrael-Živković, L., Beškoski, V., Lončarević, B., Kazazić, S., et al. (2019). A comprehensive study of conditions of the biodegradation of a plastic additive 2,6-di-tert-butylphenol and proteomic changes in the degrader Pseudomonas aeruginosa san ai. RSC Adv. 9, 23696–23710. doi: 10.1039/C9RA04298A
Meena, M., Aamir, M., Vikas, K., Swapnil, P., and Upadhyay, R. S. (2018). Evaluation of morpho-physiological growth parameters of tomato in response to Cd induced toxicity and characterization of metal sensitive NRAMP3 transporter protein. Environ. Exp. Bot. 148, 144–167. doi: 10.1016/j.envexpbot.2018.01.007
Meena, M., Divyanshu, K., Kumar, S., Swapnil, P., Zehra, A., Shukla, V., et al. (2019). Regulation of L-proline biosynthesis, signal transduction, transport, accumulation and its vital role in plants during variable environmental conditions. Heliyon 5:e02951. doi: 10.1016/j.heliyon.2019.e02952
Meena, M., Dubey, M. K., Swapnil, P., Zehra, A., Singh, S., Kumari, P., et al. (2017a). “The rhizosphere microbial community and methods of its analysis,” in Advances in PGPR Research, eds H. B. Singh, B. K. Sarma, and C. Keswani (Boston, MA: CAB International), 275–295.
Meena, M., Gupta, S. K., Swapnil, P., Zehra, A., Dubey, M. K., and Upadhyay, R. S. (2017b). Alternaria toxins: potential virulence factors and genes related to pathogenesis. Front. Microbiol. 8:1451. doi: 10.3389/fmicb.2017.01451
Meena, M., Prasad, V., and Upadhyay, R. S. (2017c). Evaluation of biochemical changes in leaves of tomato infected with Alternaria alternata and its metabolites. Vegetos 30:2. doi: 10.5958/2229-4473.2017.00020.9
Meena, M., Prasad, V., Zehra, A., Gupta, V. K., and Upadhyay, R. S. (2015). Mannitol metabolism during pathogenic fungal–host interactions under stressed conditions. Front. Microbiol. 6, 1019–1026. doi: 10.3389/fmicb.2015.01019
Meena, M., and Samal, S. (2019). Alternaria host-specific (HSTs) toxins: an overview of chemical characterization, target sites, regulation and their toxic effects. Toxicol. Rep. 6, 745–758. doi: 10.1016/j.toxrep.2019.06.021
Meena, M., and Swapnil, P. (2019). Regulation of WRKY genes in plant defense with beneficial fungus Trichoderma: current perspectives and future prospects. Arch. Phytopathol. Plant Protect. 52, 1–17. doi: 10.1080/03235408.2019.1606490
Meena, M., Swapnil, P., and Upadhyay, R. S. (2017d). Isolation, characterization and toxicological potential of tenuazonic acid, alternariol and alternariol monomethyl ether produced by Alternaria species phytopathogenic on plants. Sci. Rep. 7:8777. doi: 10.1038/s41598-017-09138-9
Meena, M., Swapnil, P., Zehra, A., Aamir, M., Dubey, M. K., and Upadhyay, R. S. (2017e). “Beneficial microbes for disease suppression and plant growth promotion,” in Plant-Microbe Interactions in Agro-Ecological Perspectives, eds D. Singh, H. Singh, and R. Prabha (Singapore: Springer), 395–432. doi: 10.1007/978-981-10-6593-4_16
Meena, M., Zehra, A., Dubey, M. K., Aamir, M., Gupta, V. K., and Upadhyay, R. S. (2016). Comparative evaluation of biochemical changes in tomato (Lycopersicon esculentum Mill.) infected by Alternaria alternata and its toxic metabolites (TeA, AOH, and AME). Front. Plant Sci. 7:1408. doi: 10.3389/fpls.2016.01408
Misra, B. B., Langefeld, C. D., Olivier, M., and Cox, L. A. (2018). Integrated omics: tools, advances, and future approaches. J. Mol. Endocrinol. 62, R21–R45. doi: 10.1530/JME-18-0055
Morgan, X. C., Tickle, T. L., Sokol, H., Gevers, D., Devaney, K. L., Ward, D. V., et al. (2012). Dysfunction of the intestinal microbiome in inflammatory bowel disease and treatment. Genome Biol. 13:R79. doi: 10.1186/gb-2012-13-9-r79
Morris, R. M., Nunn, B. L., Frazar, C., Goodlett, D. R., Ting, Y. S., and Rocap, G. (2010). Comparative metaproteomics reveals ocean-scale shifts in microbial nutrient utilization and energy transduction. ISME J. 4, 673–685. doi: 10.1038/ismej.2010.4
Muccee, F., and Ejaz, S. (2020). Whole genome shotgun sequencing of POPs degrading bacterial community dwelling tannery effluents and petrol contaminated soil. Microbiol. Res. 238:126504. doi: 10.1016/j.micres.2020.126504
Muffler, A., Bettermann, S., Haushalter, M., Hörlein, A., Neveling, U., Schramm, M., et al. (2002). Genomewide transcription profiling of Corynebacterium glutamicum after heat shock and during growth on acetate and glucose. J. Biotechnol. 98, 255–268. doi: 10.1016/S0168-1656(02)00136-0
Nagalakshmi, U., Waern, K., and Snyder, M. (2010). RNA-Seq: a method for comprehensive transcriptome analysis. Curr. Protoc. Mol. Biol. 4, 1–13. doi: 10.1002/0471142727.mb0411s89
Ngara, T. R., and Zhang, H. (2018). Recent advances in function-based metagenomic screening. Genom. Proteom. Bioinf. 16, 405–415. doi: 10.1016/j.gpb.2018.01.002
Nikolaki, S., and Tsiamis, G. (2013). Microbial diversity in the era of omic technologies. Biomed. Res. Int. 2013, 958719. doi: 10.1155/2013/958719
Nojiri, H., and Tsuda, M. (2005). Functional evolution of bacteria in degradation of environmental pollutants. Tanpakushitsu Kakusan Koso 50, 1505–1509.
Oulas, A., Pavloudi, C., Polymenakou, P., Pavlopoulos, G. A., Papanikolaou, N., Kotoulas, G., et al. (2015). Metagenomics: tools and insights for analyzing next-generation sequencing data derived from biodiversity studies. Bioinform. Biol. Insights 9, 75–88. doi: 10.4137/BBI.S12462
Palmer, C., Bik, E. M., Eisen, M. B., Eckburg, P. B., Sana, T. R., Wolber, P. K., et al. (2006). Rapid quantitative profiling of complex microbial populations. Nucleic Acids Res. 34:e5. doi: 10.1093/nar/gnj007
Pan, Z., and Raftery, D. (2007). Comparing and combining NMR spectroscopy and mass spectrometry in metabolomics. Anal. Bioanal. Chem. 387, 525–527. doi: 10.1007/s00216-006-0687-8
Panagiotou, G., Kouskoumvekaki, I., Jónsdóttir, S. O., and Olsson, L. (2007). Monitoring novel metabolic pathways using metabolomics and machine learning: induction of the phosphoketolase pathway in Aspergillus nidulans cultivations. Metabolomics 3, 503–516. doi: 10.1007/s11306-007-0061-7
Pandey, A., Tripathi, P. H., Tripathi, A. H., Pandey, S. C., and Gangola, S. (2019). “Omics technology to study bioremediation and respective enzymes,” in Smart Bioremediation Technologies: Microbial Enzymes, ed P. Bhatt (New Delhi: Academic Press), 23–43. doi: 10.1016/B978-0-12-818307-6.00002-0
Pantaleo, M. A., Ravegnini, G., Astolfi, A., Simeon, V., Nannini, M., Saponara, M., et al. (2016). Integrating miRNA and gene expression profiling analysis revealed regulatory networks in gastrointestinal stromal tumors. Epigenomics 8, 1347–1366. doi: 10.2217/epi-2016-0030
Paul, D., Pandey, G., Pandey, J., and Jain, R. K. (2005). Accessing microbial diversity for bioremediation and environmental restoration. Trends Biotechnol. 23, 135–142. doi: 10.1016/j.tibtech.2005.01.001
Peabody, M. A., van Rossum, T., Lo, R., and Brinkman, F. S. L. (2015). Evaluation of shotgun metagenomics sequence classification methods using in silico and in vitro simulated communities. BMC Bioinformatics 16:363. doi: 10.1186/s12859-015-0788-5
Plewniak, F., Crognale, S., Rossetti, S., and Bertin, P. N. (2018). A genomic outlook on bioremediation: the case of arsenic removal. Front. Microbiol. 9:820. doi: 10.3389/fmicb.2018.00820
Poirier, I., Hammann, P., Kuhn, L., and Bertrand, M. (2013). Strategies developed by the marine bacterium Pseudomonas fluorescens BA3SM1 to resist metals: a proteome analysis. Aquat. Toxicol. 128, 215–232. doi: 10.1016/j.aquatox.2012.12.006
Popovic, A., Hai, T., Tchigvintsev, A., Hajighasemi, M., Nocek, B., Khusnutdinova, A. N., et al. (2017). Activity screening of environmental metagenomic libraries reveals novel carboxylesterase families. Sci. Rep. 7:44103. doi: 10.1038/srep44103
Pritchard, C. C., Cheng, H. H., and Tewari, M. (2012). MicroRNA profiling: approaches and considerations. Nat. Rev. Genet. 13, 358–369. doi: 10.1038/nrg3198
Quince, C., Walker, A. W., Simpson, J. T., Loman, N. J., and Segata, N. (2017). Shotgun metagenomics, from sampling to analysis. Nat. Biotechnol. 35, 833–844. doi: 10.1038/nbt.3935
Raghunandan, K., Kumar, A., Kumar, S., Permaul, K., and Singh, S. (2018). Production of gellan gum, an exopolysaccharide, from biodiesel-derived waste glycerol by Sphingomonas spp. 3 Biotech. 8:71. doi: 10.1007/s13205-018-1096-3
Rani, A., Porwal, S., Sharma, R., Kapley, A., Purohit, H. J., and Kalia, V. C. (2008). Assessment of microbial diversity in effluent treatment plants by culture dependent and culture independent approaches. Bioresour. Technol. 99, 7098–7107. doi: 10.1016/j.biortech.2008.01.003
Ranjan, R., Rani, A., Metwally, A., McGee, H. S., and Perkins, D. L. (2016). Analysis of the microbiome: advantages of whole genome shotgun versus 16S amplicon sequencing. Biochem. Biophys. Res. Commun. 469, 967–977. doi: 10.1016/j.bbrc.2015.12.083
Rashid, M., and Stingl, U. (2015). Contemporary molecular tools in microbial ecology and their application to advancing biotechnology. Biotechnol. Adv. 33, 1755–1773. doi: 10.1016/j.biotechadv.2015.09.005
Reuter, J. A., Spacek, D. V., and Snyder, M. P. (2015). High-throughput sequencing technologies. Mol. Cell 58, 586–597. doi: 10.1016/j.molcel.2015.05.004
Rhee, S. K., Liu, X., Wu, L., Chong, S. C., Wan, X., and Zhou, J. (2004). Detection of genes involved in biodegradation and biotransformation in microbial communities by using 50-mer oligonucleotide microarrays. Appl. Environ. Microbiol. 70, 4303–4317. doi: 10.1128/AEM.70.7.4303-4317.2004
Rhoads, A., and Au, K. F. (2015). PacBio sequencing and its applications. Genom. Proteom. Bioinform. 13, 178–289. doi: 10.1016/j.gpb.2015.08.002
Riesenfeld, C. S., Schloss, P. D., and Handelsman, J. (2004). Metagenomics: genomic analysis of microbial communities. Ann. Rev. Genet. 38, 525–552. doi: 10.1146/annurev.genet.38.072902.091216
Rodríguez, A., Castrejón-Godínez, M. L., Salazar-Bustamante, E., Gama-Martínez, Y., Sánchez-Salinas, E., Mussali-Galante, P., et al. (2020). Omics approaches to pesticide biodegradation. Curr. Microbiol. 77, 545–563. doi: 10.1007/s00284-020-01916-5
Rogers, S. L., and McClure, N. (2003). “The role of microbiological studies in bioremediation process optimization,” in Bioremediation: A Critical Review, eds I. M. Head, I. Singleton, and M. Milner (Norfolk: Horizon Scientific Press), 27–59.
Ronaghi, M., Karamohamed, S., Pettersson, B., Uhlén, M., and Nyrén, P. (1996). Real-time DNA sequencing using detection of pyrophosphate release. Anal. Biochem. 242, 84–89. doi: 10.1006/abio.1996.0432
Roume, H., Heintz-Buschart, A., Muller, E. E. L., May, P., Satagopam, V. P., Laczny, C. C., et al. (2015). Comparative integrated omics: identification of key functionalities in microbial community-wide metabolic networks. NPJ Biofilms Microb. 1:15007. doi: 10.1038/npjbiofilms.2015.7
Sakshi and Haritash A. K. (2020). A comprehensive review of metabolic and genomic aspects of PAH-degradation. Arch. Microbiol. 202, 2033–2058. doi: 10.1007/s00203-020-01929-5
Samorodnitsky, E., Jewell, B. M., Hagopian, R., Miya, J., Wing, M. R., Lyon, E., et al. (2015). Evaluation of hybridization capture versus amplicon-based methods for whole-exome sequencing. Hum. Mutat. 36, 903–914. doi: 10.1002/humu.22825
Sanger, F., and Coulson, A. R. (1975). A rapid method for determining sequences in DNA by primed synthesis with DNA polymerase. J. Mol. Biol. 94, 441–448. doi: 10.1016/0022-2836(75)90213-2
Sato, Y., Hori, T., Koike, H., Navarro, R. R., Ogata, A., and Habe, H. (2019). Transcriptome analysis of activated sludge microbiomes reveals an unexpected role of minority nitrifiers in carbon metabolism. Commun. Biol. 2:179. doi: 10.1038/s42003-019-0418-2
Sauer, U. (2006). Metabolic networks in motion: 13C-based flux analysis. Mol. Syst. Biol. 2:62. doi: 10.1038/msb4100109
Schneegurt, M. A., and Kulpa, C. F. (1998). The application of molecular techniques in environmental biotechnology for monitoring microbial systems. Biotechnol. Appl. Biochem. 27, 73–79. doi: 10.1111/j.1470-8744.1998.tb01377.x
Scholz, M. B., Lo, C. C., and Chain, P. S. (2012). Next generation sequencing and bioinformatic bottlenecks: the current state of metagenomic data analysis. Curr. Opin. Biotechnol. 23, 9–15. doi: 10.1016/j.copbio.2011.11.013
Schut, G. J., Zhou, J., and Adams, M. W. (2001). DNA microarray analysis of the hyperthermophilic archaeon Pyrococcus furiosus: evidence for a new type of sulfur-reducing enzyme complex. J. Bacteriol. 183, 7027–7036. doi: 10.1128/JB.183.24.7027-7036.2001
Seo, J. S., Keum, Y. S., and Li, Q. X. (2009). Bacterial degradation of aromatic compounds. Int. J. Environ. Res. Public Health 6, 278–309. doi: 10.3390/ijerph6010278
Shah, V., Zakrzewski, M., Wibberg, D., Eikmeyer, F., Schlüter, A., and Madamwar, D. (2013). Taxonomic profiling and metagenome analysis of a microbial community from a habitat contaminated with industrial discharges. Microb. Ecol. 66, 533–550. doi: 10.1007/s00248-013-0253-9
Sharmin, F., Wakelin, S., Huygens, F., and Hargreaves, M. (2013). Firmicutes dominate the bacterial taxa within sugar-cane processing plants. Sci. Rep. 3:3107. doi: 10.1038/srep03107
Sharpton, T. J. (2014). An introduction to the analysis of shotgun metagenomic data. Front. Plant Sci. 5:209. doi: 10.3389/fpls.2014.00209
Shendure, J. (2008). The beginning of the end for microarrays? Nat. Methods 5, 585–587. doi: 10.1038/nmeth0708-585
Shendure, J., Porreca, G. J., Reppas, N. B., Lin, X., McCutcheon, J. P., and Rosenbaum, A. M. (2005). Accurate multiplex polony sequencing of an evolved bacterial genome. Science 309, 1728–1732. doi: 10.1126/science.1117389
Shi, Z. J., Yin, H., Van Nostrand, J. D., Voordeckers, J. W., Tu, Q., Deng, Y., et al. (2019). Functional gene array-based ultrasensitive and quantitative detection of microbial populations in complex communities. mSystems 4:e00296–e00219. doi: 10.1128/mSystems.00296-19
Siggins, A., Gunnigle, E., and Abram, F. (2012). Exploring mixed microbial community functioning: recent advances in metaproteomics. FEMS Microbiol. Ecol. 80, 265–280. doi: 10.1111/j.1574-6941.2011.01284.x
Silva, C. C., Hayden, H., Sawbridge, T., Mele, P., De Paula, S. O., Silva, L. C. F., et al. (2013). Identification of genes and pathways related to phenol degradation in metagenomic libraries from petroleum refinery wastewater. PLoS ONE 8:e61811. doi: 10.1371/journal.pone.0061811
Simmons, S. L., Dibartolo, G., Denef, V. J., Aliaga Goltsman, D. S., Thelen, M. P., and Banfield, J. F. (2008). Population genomic analysis of strain variation in Leptospirillum group II bacteria involved in acid mine drainage formation. PLoS Biol. 6:e177. doi: 10.1371/journal.pbio.0060177
Singh, J., Behal, A., Singla, N., Joshi, A., Birbian, N., Singh, S., et al. (2009). Metagenomics: Concept, methodology, ecological inference and recent advances. Biotechnol. J. 4, 480–494. doi: 10.1002/biot.200800201
Sueoka, K., Satoh, H., Onuki, M., and Mino, T. (2009). Microorganisms involved in anaerobic phenol degradation in the treatment of synthetic coke-oven wastewater detected by RNA stable-isotope probing. FEMS Microbiol. Lett. 291, 169–174. doi: 10.1111/j.1574-6968.2008.01448.x
Tang, Y. J., Hwang, J. S., Wemmer, D. E., and Keasling, J. D. (2007). Shewanella oneidensis MR-1 fluxome under various oxygen conditions. Appl. Environ. Microbiol. 73, 718–729. doi: 10.1128/AEM.01532-06
Thakur, M., Medintz, I. L., and Walper, S. A. (2019). Enzymatic bioremediation of organophosphate compounds—progress and remaining challenges. Front. Bioeng. Biotechnol. 7, 289. doi: 10.3389/fbioe.2019.00289
Turcatti, G., Romieu, A., Fedurco, M., and Tairi, A. P. (2008). A new class of cleavable fluorescent nucleotides: synthesis and optimization as reversible terminators for DNA sequencing by synthesis. Nucleic Acids Res. 36:e25. doi: 10.1093/nar/gkn021
van Nostrand, J. D., He, Z., and Zhou, J. (2011). “Dynamics of microbes in the natural setting: development of the Geochip,” in Environmental Microbiology: Current Technology and Water Applications, eds K. Sen and N. J. Ashbolt (Norfolk: Caister Academic Press), p. 316.
van Rossum, T., Kengen, S. W., and van der Oost, J. (2013). Reporter-based screening and selection of enzymes. FEBS J. 280, 2979–2996. doi: 10.1111/febs.12281
Vandera, E., Samiotaki, M., Parapouli, M., Panayotou, G., and Koukkou, A. I. (2015). Comparative proteomic analysis of Arthrobacter phenanthrenivorans Sphe3 on phenanthrene, phthalate and glucose. J. Proteom. 113, 73–89. doi: 10.1016/j.jprot.2014.08.018
Venter, J. C., Remington, K., Heidelberg, J. F., Halperna, A. L., Rusch, D., Eisen, J. A., et al. (2004). Environmental genome shotgun sequencing of the Sargasso Sea. Science 304, 66–74. doi: 10.1126/science.1093857
Verberkmoes, N. C., Denef, V. J., Hettich, R. L., and Banfield, J. F. (2009). Systems biology: functional analysis of natural microbial consortia using community proteomics. Nat. Rev. Microbiol. 7, 196–205. doi: 10.1038/nrmicro2080
Vermote, L., Verce, M., de Vuyst, L., and Weckx, S. (2018). Amplicon and shotgun metagenomic sequencing indicates that microbial ecosystems present in cheese brines reflect environmental inoculation during the cheese production process. Int. Dairy J. 87, 44–53. doi: 10.1016/j.idairyj.2018.07.010
Viant, M. R., and Sommer, U. (2013). Mass spectrometry based environmental metabolomics: a primer and review. Metabolomics 9, 144–158. doi: 10.1007/s11306-012-0412-x
Vigneron, A., Alsop, E. B., Cruaud, P., Philibert, G., King, B., Baksmaty, L., et al. (2017). Comparative metagenomics of hydrocarbon and methane seeps of the Gulf of Mexico. Sci. Rep. 7:16015. doi: 10.1038/s41598-017-16375-5
Villas-Bôas, S. G., Nielsen, J., Smedsgaard, J., Hansen, M. A. E., and Roessner-Tunali, U. (2007). Metabolome Analysis: An Introduction. Hoboken, NJ: Wiley-Interscience.
Vos, M., and Velicer, G. J. (2006). Genetic population structure of the soil bacterium Myxococcus xanthus at the centimeter scale. Appl. Environ. Microbiol. 72, 3615–3625. doi: 10.1128/AEM.72.5.3615-3625.2006
Wang, D. Z., Kong, L. F., Li, Y. Y., and Xie, Z. X. (2016). Environmental microbial community proteomics: status, challenges and perspectives. Int. J. Mol. Sci. 17, 1275. doi: 10.3390/ijms17081275
Wang, D. Z., Xie, Z. X., and Zhang, S. F. (2014). Marine metaproteomics: Current status and future directions. J. Proteom. 97, 27–35. doi: 10.1016/j.jprot.2013.08.024
Wang, J. H., Byun, J., and Pennathur, S. (2010). Analytical approaches to metabolomics and applications to systems biology. Semin. Nephrol. 30, 500–511. doi: 10.1016/j.semnephrol.2010.07.007
Ward, M. A., Hyman, B., Scherzer, C., Trisini-Lipsanopoulos, A., Ivinson, A. J., Lößner, C., et al. (2014). Exploration of plasma biomarkers for alzheimer's disease using isotopic tandem mass tags and a combined directed/data-dependent acquisition NLC-MS/MS method. Alzheimers Dement. J. Alzheimers Assoc. 10:P674. doi: 10.1016/j.jalz.2014.05.1215
Wiechert, W. (2001). 13C metabolic flux analysis. Metab. Eng. 3, 195–206. doi: 10.1006/mben.2001.0187
Wiechert, W., Möllney, M., Petersen, S., and de Graaf, A. A. (2001). A universal framework for 13C metabolic flux analysis. Metab. Eng. 3, 265–283. doi: 10.1006/mben.2001.0188
Wierckx, N. J. P., Ballerstedt, H., De Bont, J. A. M., DeWinde, J. H., Ruijssenaars, H. J., and Wery, J. (2008). Transcriptome analysis of a phenol-producing Pseudomonas putida S12 construct: genetic and physiological basis for improved production. J. Bacteriol. 190, 2822–2830. doi: 10.1128/JB.01379-07
Wierckx, N. J. P., Ruijssenaars, H. J., deWinde, J. H., Schmid, A., and Blank, L. M. (2009). Metabolic flux analysis of a phenol producing mutant of Pseudomonas putida S12: verification and complementation of hypotheses derived from transcriptomics. J. Biotechnol. 143, 124–129. doi: 10.1016/j.jbiotec.2009.06.023
Wilmes, P., and Bond, P. L. (2006). Metaproteomics: studying functional gene expression in microbial ecosystems. Trends Microbiol. 14, 92–97. doi: 10.1016/j.tim.2005.12.006
Wiseschart, A., Mhuantong, W., Tangphatsornruang, S., Chantasingh, D., and Pootanakit, K. (2019). Shotgun metagenomic sequencing from Manao-Pee cave, Thailand, reveals insight into the microbial community structure and its metabolic potential. BMC Microbiol. 19:144. doi: 10.1186/s12866-019-1521-8
Wong, D. W. S. (2018). Gene Targeting and Genome Editing. The ABCs of Gene Cloning. Cham: Springer, 187–197. doi: 10.1007/978-3-319-77982-9_20
Wright, R. J., Bosch, R., Gibson, M. I., and Christie-Oleza, J. A. (2020). Plasticizer degradation by marine bacterial isolates: a proteogenomic and metabolomic characterization. Environ. Sci. Technol. 54, 2244–2256. doi: 10.1021/acs.est.9b05228
Wrighton, K. C., Thomas, B. C., Sharon, I., Miller, C. S., Castelle, C. J., and VerBerkmoes, N. C. (2012). Fermentation, hydrogen, and sulfur metabolism in multiple uncultivated bacterial phyla. Science 337, 1661–1665. doi: 10.1126/science.1224041
Wu, C. C., and Yates, J. R. (2003). The application of mass spectrometry to membrane proteomics. Nat. Biotechnol. 21, 262–267. doi: 10.1038/nbt0303-262
Wu, Y. R., Luo, Z. H., Kwok-Kei Chow, R., and Vrijmoed, L. L. P. (2010). Purification and characterization of an extracellular laccase from the anthracene-degrading fungus Fusarium solani MAS2. Bioresour. Technol. 101, 9772–9777. doi: 10.1016/j.biortech.2010.07.091
Xie, J., He, Z., Liu, X., Liu, X., Van Nostrand, J. D., Deng, Y., et al. (2011). GeoChip-based analysis of the functional gene diversity and metabolic potential of microbial communities in acid mine drainage. Appl. Environ. Microbiol. 77, 991–999. doi: 10.1128/AEM.01798-10
Xue, K., Yuan, M. M., Shi, Z. J., Qin, Y., Deng, Y., Cheng, L., et al. (2016). Tundra soil carbon is vulnerable to rapid microbial decomposition under climate warming. Nat. Clim. Chang. 6:595. doi: 10.1038/nclimate2940
Yao, C., Chen, B. H., Joehanes, R., Otlu, B., Zhang, X., Liu, C., et al. (2015). Integromic analysis of genetic variation and gene expression identifies networks for cardiovascular disease phenotypes. Circulation 131, 536–549. doi: 10.1161/CIRCULATIONAHA.114.010696
Yergeau, E., Michel, C., Tremblay, J., Niemi, A., King, T. L., Wyglinski, J., et al. (2017). Metagenomic survey of the taxonomic and functional microbial communities of seawater and sea ice from the Canadian Arctic. Sci. Rep. 7:42242. doi: 10.1038/srep42242
Yoneda, A., Henson, W. R., Goldner, N. K., Park, K. J., Forsberg, K. J., Kim, S. J., et al. (2016). Comparative transcriptomics elucidates adaptive phenol tolerance and utilization in lipid-accumulating Rhodococcus opacus PD630. Nucleic Acids Res. 44, 2240–2254. doi: 10.1093/nar/gkw055
Yozwiak, N. L., Skewes-Cox, P., Stenglein, M. D., Balmaseda, A., Harris, E., and DeRisi, J. L. (2012). Virus identification in unknown tropical febrile illness cases using deep sequencing. PLoS Negl. Trop. Dis. 6:e1485. doi: 10.1371/journal.pntd.0001485
Zehra, A., Meena, M., Swapnil, P., Raytekar, N. A., and Upadhyay, R. S. (2020). “Sustainable approaches to remove heavy metals from water,” in Microbial Biotechnology: Basic Research and Applications, eds J. Singh, A. Vyas, S. Wang, and R. Prasad (Singapore: Springer), 127–146. doi: 10.1007/978-981-15-2817-0_6
Zhang, J., Chiodini, R., Badr, A., and Zhang, G. (2011). The impact of next-generation sequencing on genomics. J. Genet. Genom. 38, 95–109. doi: 10.1016/j.jgg.2011.02.003
Zhang, W., Li, F., and Nie, L. (2010). Integrating multiple 'omics' analysis for microbial biology: application and methodologies. Microbiology 156, 287–301. doi: 10.1099/mic.0.034793-0
Zhong, M., and Zhou, Q. (2002). Molecular-ecological technology of microorganisms and its application to research on environmental pollution. Ying Yong Sheng Tai Xue Bao 13, 247–251.
Zhou, J., He, Z., Yang, Y., Deng, Y., Tringe, S. G., and Alvarez-Cohen, L. (2015). High-throughput metagenomic technologies for complex microbial community analysis: open and closed formats. mBio 6:e02288-14. doi: 10.1128/mBio.02288-14
Zhou, J., Kang, S., Schadt, C. W., and Garten, C. T. (2008). Spatial scaling of functional gene diversity across various microbial taxa. Proc. Natl. Acad. Sci. U.S.A. 105, 7768–7773. doi: 10.1073/pnas.0709016105
Keywords: bioremediation, microorganisms, microarrays, metagenomics, transcriptomics, proteomics, metabolomics, fluxomics
Citation: Chandran H, Meena M and Sharma K (2020) Microbial Biodiversity and Bioremediation Assessment Through Omics Approaches. Front. Environ. Chem. 1:570326. doi: 10.3389/fenvc.2020.570326
Received: 07 June 2020; Accepted: 18 August 2020;
Published: 25 September 2020.
Edited by:
Edward Nxumalo, University of South Africa, South AfricaReviewed by:
Duanping Sun, Guangdong Pharmaceutical University, ChinaMin Lv, Yantai Institute of Coastal Zone Research (CAS), China
Copyright © 2020 Chandran, Meena and Sharma. This is an open-access article distributed under the terms of the Creative Commons Attribution License (CC BY). The use, distribution or reproduction in other forums is permitted, provided the original author(s) and the copyright owner(s) are credited and that the original publication in this journal is cited, in accordance with accepted academic practice. No use, distribution or reproduction is permitted which does not comply with these terms.
*Correspondence: Mukesh Meena, bXVrZXNobWVlbmFtbHN1QGdtYWlsLmNvbQ==; bXVrZXNobWVlbmFiaHVAZ21haWwuY29t