- 1Ecorobotix SA, Yverdon-les-Bains, Switzerland
- 2Amt für Ernährung, Landwirtschaft und Forsten Deggendorf-Straubing (AELF) Deggendorf-Straubing, Deggendorf, Germany
Weeding is at the heart of agriculture. Today, using herbicides is unavoidable in conventional agriculture. However, increasing ecological awareness, the need to reduce carbon dioxide emissions, and the pursuit of sustainable food have driven industries and academics to explore new approaches for growing and protecting crops. In this context, smart spot sprayers emerge as a highly innovative alternative to broadcast treatments, which overuse pesticides, and mechanical weeding, which is limited by restrictive application conditions. Using artificial intelligence (AI) for plant recognition and ultra-precise spraying technology, herbicides are applied directly to weeds with minimal overspray, reducing their use by 90% compared to conventional methods. Spot sprayers' narrowly targeted spray limits chemicals' contact with the crop, resulting in low phytotoxicity, preserving natural crop development while maximizing yield potential. In addition, site-specific application increases the selectivity of chemical products artificially, enabling more concentrated mixtures to be used. Also, AI enables the selective application of non-selective molecules, supporting their use in established cultures. These characteristics open new opportunities for effective weed control where the traditional solutions fall short. This publication demonstrates the potential of the ultra-precise smart spot sprayer ARA in weed management in onion and sugar beet crops. Although comparing the overall performance of ARA with other smart spot sprayers on the market cannot be done in this article, this technology's potential in rendering weed management on farms more effective, sustainable, and saving labor without compromising yield is nevertheless indicated.
1 Introduction
Agriculture is deeply intertwined with human history, playing a fundamental role in societies' organization both past and present (Kareiva et al., 2007; Carey, 2023). Within the scale of agricultural history, the Green Revolution launched the mechanization of agriculture and introduced inorganic chemicals for crop protection, as well as using mineral fertilizer, crop rotations, and other agronomical approaches. It pinpoints the industrialization and intensification of agriculture, transitioning from self-sufficiency to globalization. Today, agriculture is a key economic sector, contributing 4.32% to the global gross domestic product in 2021 (FAOSTAT, 2023). In recent decades, agrochemical, breeding, and seed companies have pushed crop production to its limits, often treating humans, organisms, and ecosystems as mere tools for food production. This has sparked ecological awareness and a push for producing healthier food more sustainably. Weeding is a major challenge in maintaining food security and high health standards as weeds are estimated to cause 34% of crop losses (Oerke, 2006; Colbach and Cordeau, 2018). In particular, early-season weeds are harmful and impact crop development at different stages (Horvath et al., 2023). Weeds directly influence plant architecture (Horvath et al., 2023); compete with the crop for water, nutrients, and light (Teyker et al., 1991; McGiffen et al., 1992; Rajcan and Swanton, 2001); or inhibit growth by allelopathic effects (Kadioglu et al., 2005). They may parasitize crops (Parker, 2009) or host pests (fungi and/or insects) that compromise crop production (Mantle et al., 1977). During harvesting, weeds can clog machinery, and their seeds can contaminate crop seeds, complicating separation (Begemann et al., 2021). More dramatically, some weeds (plants or seeds) are toxic (Orlando et al., 2019). Finally, the weed seed stock represents a high risk for the next season.
In this agricultural context, myriad weeding solutions, encouraged by national and European initiatives, have flourished (Korres et al., 2019). Some focus on agronomic approaches, while others explore traditional agricultural practices. Additionally, new (bio)-technologies, including genetics, data analysis for oriented decision-making, and predictive agriculture, have developed. Today, agriculture is mutating by integrating artificial intelligence (AI) and machine learning to automatically detect weeds in crops (Murad et al., 2023). The Internet of Things and robotics are also used for smarter, more precise farming (Mesías-Ruiz et al., 2023). The new ambition is to combine performance, wellbeing, and environmental sustainability while combating climatic change and reducing agriculture's carbon footprint without compromising food security or quality. Among all the many conceptual prototypes (Bloomer et al., 2024), some have become mature products and are now commercially available, offering pioneer weeding strategies to farmers. Although weeding tools share a common goal, inventors have adopted different strategies for data acquisition (drones or ground-based), plant detection technology [Red Green Blue (RGB) camera or multispectral sensor; Niemeyer et al., 2024], plant classification (Mesías-Ruiz et al., 2023), plant position annotation [Real Time Kinematic (RTK) or relative position], and management (direct or indirect). While companies like Ecorobotix or Blue River/John Deere favor chemical solutions (Ecorobotix, 2023; Blue River, 2024; See and Spray, 2024), others have chosen mechanical weeding approaches that use hoes (Garford, 2024), knives (Farmdroid, 2024), electricity (Andela, 2024), or, in the most revolutionary designs, even lasers (CarbonRobotics, 2024; WeedBot, 2024). Market differentiation occurs in several areas: weed identification, weeding precision, actuation technology, working speed, crop types, and application stages for both crops and weeds.
This study presents the advantages of an ultra-precise smart spot sprayer that controls weeds while minimizing chemicals' impact on crops and their ecosystems. Two examples illustrate this purpose: weeding sugar beet and onion crops using the ultra-precise smart spot sprayer ARA from Ecorobotix (2023). The pesticide volumes applied in practical cases are discussed as well as the weeding efficacy, selectivity, and yield production. These parameters are evaluated in four different modalities: an untreated control (NT), ARA spot spraying with a selective program (ARA selective), ARA spot spraying with the non-selective herbicide pelargonic acid (ARA non-selective), and the broadcast conventional reference (farmer reference). This summarizes the data collected during one season. With all care taken to provide reproducible and reliable data, it should be noted the results arise from a global combination of local weather, soil composition, the influence of previous crops, pest and weed pressures, and the applied protocols. Therefore, the findings may not be fully replicable in other contexts, and this should be considered when drawing conclusions.
2 Results
While the idea of avoiding chemicals in weeding strategies is appealing, facts show that organic agriculture cannot sustain the global food request in the near future, mostly because inorganic nitrogen fertilizers or hybrid cultivars are not being used, which results in lower yields compared to conventional agriculture (Morais et al., 2021). Economically, organic agriculture is not viable on a larger scale because it requires extensive manual labor and is highly susceptible to environmental changes, such as pest infestations. While organic agriculture might provide enough food under optimal conditions and with a well-managed food system to reduce food waste, it represents a high risk for food security in the case of plague. Furthermore, mechanical weeding approaches, such as the innovative Garford hoeing technique or advanced knife weeding (Farmdroid, 2024; Garford, 2024), are highly restrictive. The crop needs to be well rooted, the tools cannot get too close to the plants, the soil should not be too wet or too dry, and the plants must be perfectly aligned in rows with strict spacing (Hussain et al., 2018). Mechanical weeding is also more energy-intensive than spraying (Hussain et al., 2018). Using herbicides in a sustainable agriculture approach addresses these restrictions by allowing early-stage interventions, weeding as close as possible to the crop independent of the pedologic conditions, and using sowing or transplanting methods. Although chemicals remain unavoidable, their use must be mindful and more restricted. A plant-by-plant application of products on a restrained surface, so-called spot spraying, is a powerful lever to lower pesticide consumption.
2.1 Ultra-precision spot sprayer design
Ecorobotix SA designed and produces the ultra-precise smart spot sprayer ARA (Tanner et al., 2021; Tanner, 2022; Ecorobotix, 2023). ARA distinguishes itself in the market by combining multiple advantages. It can apply the product with great precision. The ARA technology can be transferred to any crop once a training data set is available. ARA is both ambivalent (targeting weeds or crop plants) and polyvalent (offering various weeding strategies across a range of crops, nine so far). ARA performs consistently in both transplanted and sown crops, being flexible and independent of the crop's growth stage, with the restrictions of a 2-cm minimum diameter and a 50-cm maximum height of the crop. ARA comprises three main parts (Figures 1A, B): a front tank for the mixture, an onboard tablet for machine control (Figure 1C), and the 6.04-m-wide sprayer itself. The smart sprayer includes (1) 12 Light Emitting Diode (LED) spotlights for uniform field lighting, 6 high-resolution RGB cameras, and 6 in-depth cameras, together constituting the information acquisition part; (2) 6 processors, constituting the AI core for real-time analysis of the live images and direct decision-making; and (3) a scalable boom with 156 nozzles spaced 4 cm apart, each controlled by solenoid valves for the high-precision actuation spray.
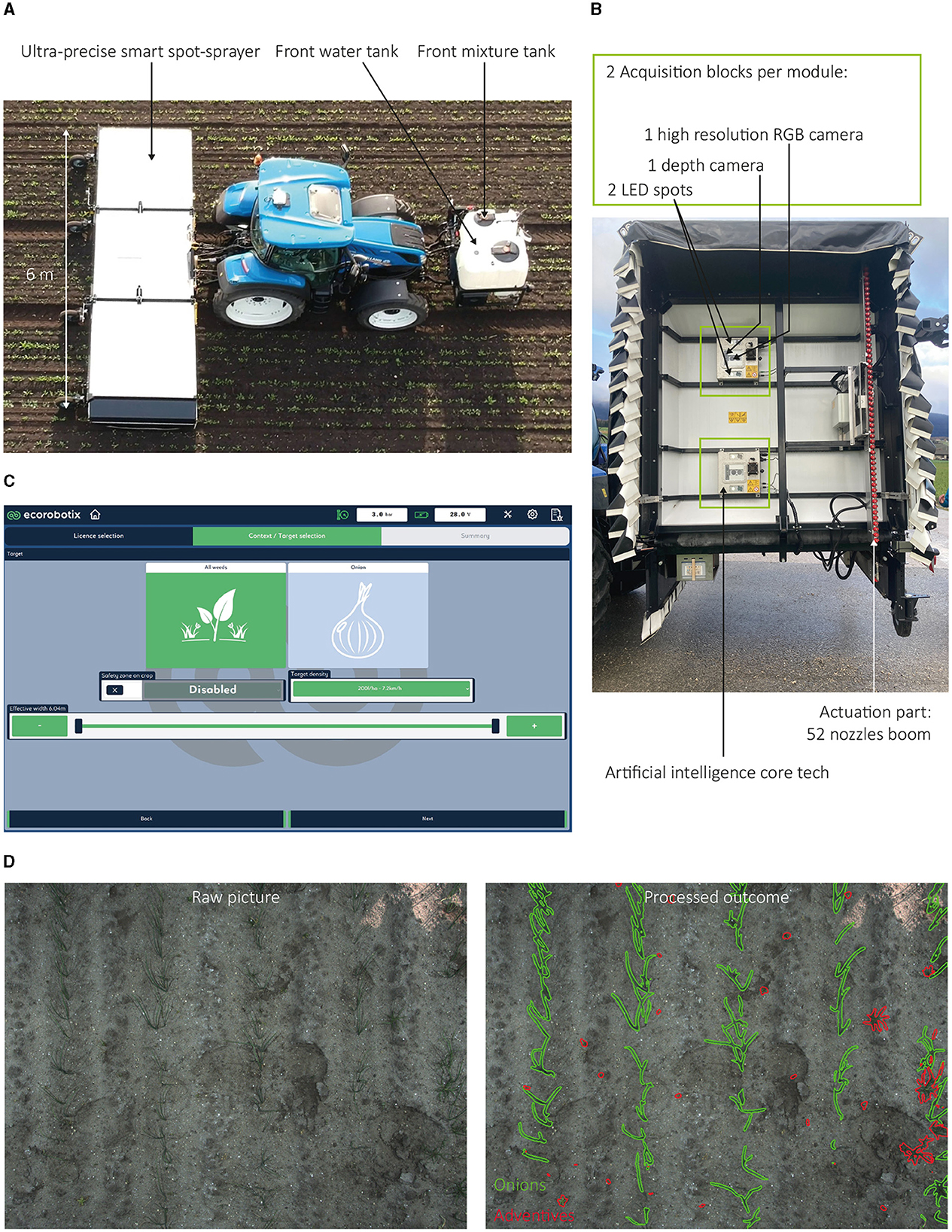
Figure 1. ARA brings high technology and artificial intelligence in the field to spray specific target. (A) Aerial view of a working ARA in the field. ARA is composed of two frontal tanks, an onboarded tablet in the cabin and the sprayer itself in the back composed of three modules of 2 meter large. (B) Bottom view of the left module, composed of 4 LED spotlights, 2 high resolution RGB cameras, 2 depth cameras, 2 computers and 56 nozzles. (C) Screenshot of the user interface controlling the ARA. (D) Example of a picture taken by the ARA in onion crop (left panel) and the output annotation after the model has been ran on (right panel). Red: adventives, green: crop.
The ARA technology relies on supervised machine learning with algorithms trained on a large database of crop pictures acquired in real conditions in the field. To ensure robust decision-making, the onboarded algorithms on ARA exhibit F1 scores for weeds >90%.
During operation, ARA captures live images of the field, which are processed by its AI to classify each pixel as crop, soil, or weed (Figure 1D). The required mixture volume is then calculated and synchronized with the nozzle control, reducing the spray area to a 6 cm × 6 cm minimum on the selected target (crop, soil, or weeds). The product volume can be adjusted within standard sprayer ranges and adapted to the tractor's speed for efficient weeding. ARA's design allows it to operate close to the crop, reducing drift by more than 95% (DGAL/SDSPV/2023-282, 2023; van Steenbergen et al., 2024). This feature is essential as it minimizes phytotoxicity, protects the environment, and promotes biodiversity.
2.2 ARA reduces the use of plant protection products
Conventional broadcast spraying solutions typically spread pesticide diluted in 150–300 L/ha of water (note that water-diluted herbicides will be designated, hereafter, as “herbicide volumes”).
However, ARA's spot-spraying methodology, which applies products only where needed, significantly reduces these inputs. Data from our ARA dashboard, extracted and anonymized, show that among 57 ARA machines performing 1,682 weeding missions on onion fields over 1 ha in 2023, 78.9% of the herbicide volume was saved across 8,266 ha (8.3 kha) compared to traditional broadcast treatments. Remarkably, one third of the treated area saved more than 90% of the herbicide volume (Figure 2A). Similarly, in sugar beet fields, 79.8% of the herbicide volume was saved (33 ARA machines, 480 weeding missions over 2 kha), with 47.6% of the treated area saving more than 90% of the volume (Figure 2A). Those two real-world examples advocate ARA's ability to drastically reduce herbicide inputs in the field, providing significant savings and environmental benefits for end users.
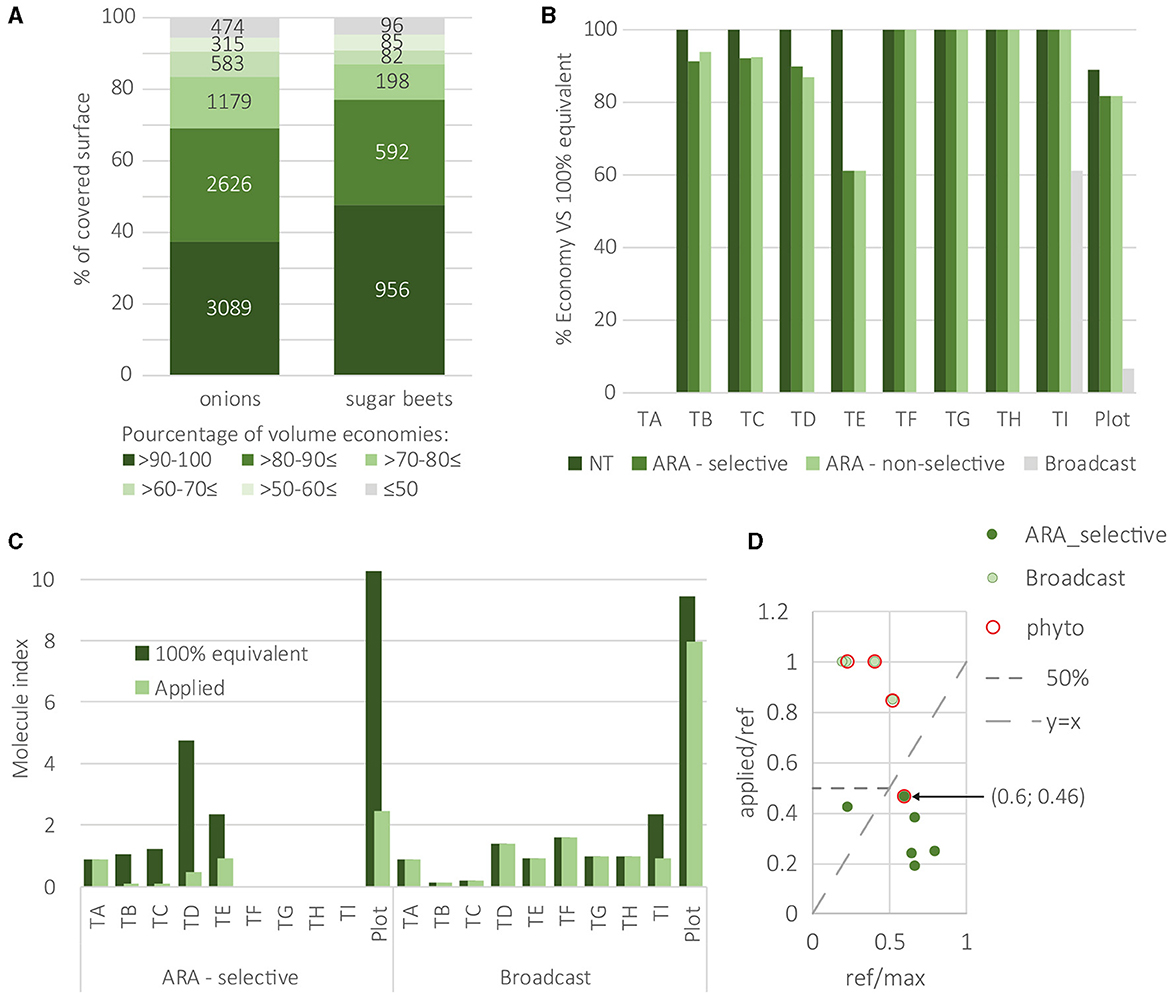
Figure 2. ARA allows mixture volume savings and reduces molecule quantities applied in the field. (A) Percentage of surface allowing a certain volume economy in onions and sugar beets. Numbers indicates the surface areas (ha) per category of savings. Extracted and anonymized data from 2023 season on surfaces greater than 1 ha. (B) Volume saving on the onion trial over the treatments A-I (TA-TI) and the mean saving over the plot for the different modalities in comparison with an 100% broadcast treatment. (C) Quantity of applied molecules during the different treatments and the cumulative applied molecules on the plot. (D) Graphical representation of the quantity of applied molecules compared to the tank mixture composition (ref) and the maximal legal reference (max). Red circles indicate strong phytotoxicity that last all over the crop development. The 50% threshold represents the 50% of molecule economy compared to the mixture composition in the tank. The higher the ref/max ratio is, the more stringent the mixture is.
2.2.1 Volume savings
To correlate the volume savings with weed pressure, we recorded the percentage of the total surface covered by the weeds in the non-treated control (shown on the secondary y-axis; Supplementary Figure S1). In the treated modalities, the weed pressure is expressed as a percentage of herbicide efficacy. Here, 100% indicates complete weed eradication relative to the non-treated control (EPPO Standards: General Standards, 2023) and is displayed on the primary y-axis (Supplementary Figure S1). A 0% indicates no obvious differences compared to the non-treated control, signifying herbicide treatment inefficacy. Additionally, we documented the volume of mixture applied across the trials.
After an initial broadcast pre-emergence treatment (TA), ARA spot-spraying treatments resulted in approximately 90% product savings for treatment B (TB), treatment C (TC), and treatment D (TD) in onions both in the ARA selective and non-selective modalities compared to a broadcast treatment (Figure 2B). Interestingly, weed coverage was estimated at 0.7% in the non-treated control at the start of the trial (TB), and 17.75 L/ha of diluted herbicides were applied in the ARA selective modality. Treatment E (TE) mainly targeted Amaranthus retroflexus (AMARE), which germinates later in the season (Supplementary Figure S1A). In this catch-up TE treatment, the AMARE coverage was estimated to 3% in the non-treated control (Supplementary Figure S1A), and 77.6 L/ha was applied over the field. The difference in mixture consumption between TB and TE shows that the volume consumption is directly proportional to the weed coverage. Volume savings are proportional to weed coverage but do not equal it because the minimum spray print resolution is 6 cm × 6 cm, larger than small emerging weeds. Similar savings were observed in sugar beets where 58% of volume was saved, although including an initial pre-emergence broadcast treatment (in both the ARA selective and non-selective modalities), with an average savings of 87% per treatment when using ARA (see TB and TC in Supplementary Figure S2A).
Thus, ARA's potential for volume economy must be considered in the weed pressure framework. Strong weed pressure may result in only mild volume savings, but ARA spot spraying will still use lower or equivalent volumes compared to broadcast treatments. Interestingly, after a first broadcast pre-emergence treatment, the total savings on the onion trial plot represented 67% compared to the broadcast treatment (both ARA selective and non-selective; Figure 2B) and 58% in the sugar beet trial plot (Supplementary Figure S2A), going beyond the European goal of reducing pesticides by 50% by 2030 (Farm to Fork Strategy, 2023). Also, a single spot-spraying treatment with ARA in a broadcast program saved 6.8% of the total volume (Figure 2B), showing ARA's utility in ultra-localized catch-up treatments to fight late-emerging weeds where broadcast treatments would be overkill on patchy weeds.
2.2.2 Molecule savings
While ARA achieves an impressive reduction in herbicide savings, evaluating its impact on molecule savings is important. Notably, these savings can reach up to 90% when the quantities applied using ARA are compared to those in a 100% application scenario (Supplementary Figure S3). To quantify these molecule savings, the applied molecule dosage, the volume of liquid sprayed at an equivalent flow of 200 L/ha, and the maximum legal dosage (here, Swiss legislation is taken as reference; Office fédéral de la sécurité alimentaire et des affaires vétérinaires OSAV – Index des produits phytosanitaires, 2023) were considered. The savings are calculated as follows:
For the plot:
For the treatment:
For a single molecule:
Note that the index of the mixture contained in the tank corresponds to
In the onion trial, the overall molecule savings reached 69% in the ARA selective modality compared to the broadly applied equivalent treatment (Applied IndexPlot vs. 100% equivalent IndexPlot; Figure 2C). Although the ARA selective program includes nearly as many molecules as the farmer treatment program, in just five ARA applications vs. nine for the farmer (Figure 2C; ARA selective 100% equivalent IndexPlot of 10.27 vs. 9.43 in the farmer modality), the final Applied IndexPlot in ARA selective modality is 3.2 times lower than the farmer reference (2.47 vs. 7.98), showing that ARA can apply drastic mixtures locally while significantly reducing the overall inputs (Supplementary Figure S3A).
Interestingly, TEARA − selective/TIFarmer was a concentrated and complex treatment compared to the rest of the farmer weeding program (Treatment index: 2.37 representing 29% of the farmer Applied IndexPlot−7.98; Figure 2C, Table 1). Yet, its application by ARA allowed a molecule savings of 15% in the farmer modality, indicating that stringent mixtures applied by ARA have agronomic benefits while lowering the Applied IndexPlot in the farmer modality. Higher herbicide concentrations increase weeding efficacy and reduce the risk of resistance emerging from repetitive low-concentration applications. Figure 2D illustrates how ARA applies stringent programs while saving molecules. The Applied Index Plot and 100% equivalent IndexPlot are normalized to the Maximal IndexPlot, representing the maximal legal dosage. This graph compiles data collected from different trials led on other crops not described in this study. Strikingly, three clusters pop up: Soft programs applied in broadcast, stringent programs applied with ARA, and soft programs applied with ARA. No stringent programs are shown because they would compromise the crop due to high phytotoxicity.
The clusters reveal that even with highly concentrated mixtures (high 100% equivalent IndexPlot/Maximal IndexPlot ratio), ARA applies only a small fraction of molecules on the field (low Applied IndexPlot/Maximal IndexPlot ratio) compared to broadcast treatments (low 100% equivalent IndexPlot/Maximal IndexPlot ratio but high Applied IndexPlot/Maximal IndexPlot ratio). Remarkably, the red dot in ARA selective modality (x:0.6; y:0.46) corresponds to a trial on spinach. The corresponding algorithm was judged as not performing well enough because it induced strong phytotoxicity in the crop.
This suggests the mixture was not suited for ARA as is. The spinach algorithm needs further development for accuracy in recognizing spinach vs. weeds and improving spray precision to reduce inadvertent crop application. Nevertheless, Figure 2D suggests that ARA can apply more stringent programs (with the restriction of an efficient-enough algorithm) while decreasing the field molecule inputs.
In the sugar beets trial, molecule savings were 58% in both the ARA selective and non-selective modalities (Supplementary Figures S2B, S3B). This indicates that ARA spot spraying not only reduces volumes but also cuts molecule inputs by more than 50% compared to the 100% equivalent of planned programs. However, ensuring that reduced volumes and molecules are effective for proper weeding and that the stringent mixtures applied with ARA do not compromise crop health is essential.
2.3 ARA effectively tackles weeds
Both mechanical and traditional chemical broadcast weeding process the entire field uniformly, applying maximum efficacy whether weeds are present or not. In contrast, spot-spraying weeding methods, like ARA, target only specific areas where weeds are present. Weeding efficiency can be compromised if the spot sprayer algorithm is not accurate or the applied mixture is not suitable for the specific weed species. To evaluate the performance of ARA's algorithm, plant coverage and treatment efficacy were evaluated in the tested modalities over time and compared to the non-treated control modality and the tested conditions. Briefly, the ARA selective modality corresponds to a concentrated stringent chemical mixture, ARA's non-selective modality explores the efficacy of pelargonic acid at 16 L/ha, and the broadcast farmer reference modality corresponds to conventional mixtures and dosages. Information on the programs applied in different modalities is available in Tables 1–3.
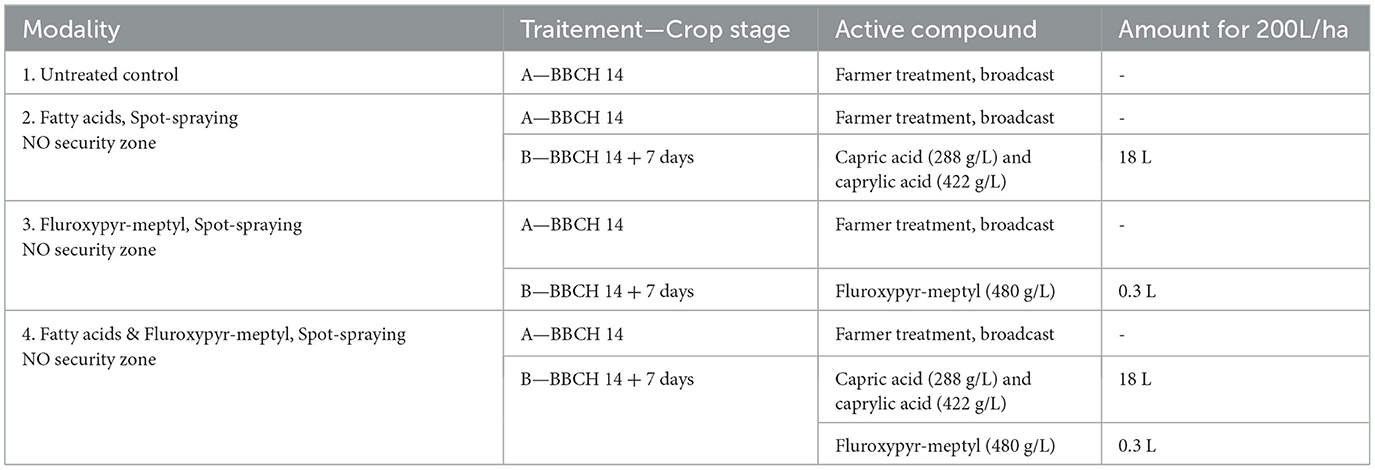
Table 3. Swiss program against volunteer potatoes herbicide treatments in onion crop in the different modalities.
Despite the low quantity of chemicals applied in ARA modalities in the onion trial (Figures 2B, C), the ARA selective modality displays a global efficacy of 92%, ARA non-selective 87% (compared to 99% for the broadcast treatment; Figure 3A, Supplementary Figure S1A). These results are satisfying (Figures 3A, B, Supplementary Figure S1A). Although ARA modalities (both selective and non-selective) showed slightly lower efficacy than the broadcast treatment, no significant differences in weeding efficacy were found.
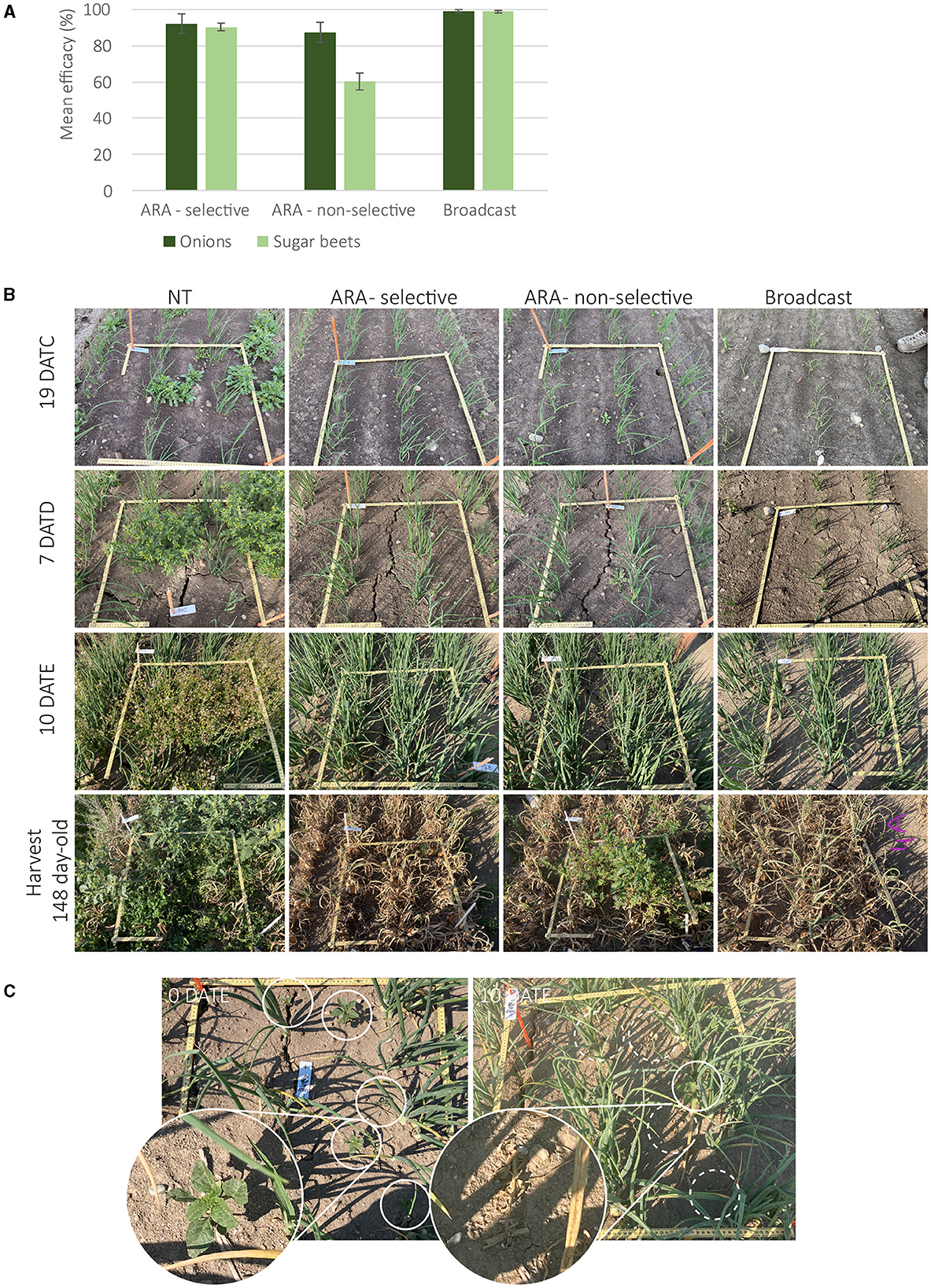
Figure 3. Small amounts of mixtures applied by ARA effectively control weeds. (A) Mean efficacy of weed control in onion and sugar beet trials for the different modalities. (B) Time-lapse of the evolution of the field in the different modalities for the onion trial. Pictures were taken 19 day-after-treatment C (19 DATC), 7 DATD, 10 DATE and at the harvest. The square represents 0.36 m2. (C) Catch-up treatments on AMARE are efficient in onion crops. Continuous circles indicate vigorous AMARE, dash circles dead ones 10 DATE. The square represents 0.36 m2.
Notably, ARA required fewer interventions to achieve comparable weeding efficacy than the broadcast program (5 vs. 9 treatments; Table 1) and used lower volumes and fewer molecules amounts than the broadcast treatment (Figures 2B, C, 3B, Supplementary Figure S3A). This suggests that ARA's onion algorithm performs well, and the mixtures applied were strong enough to control the weeds present in this trial.
Examining individual weeds, the ARA non-selective modality shows some difficulty in controlling Polygonum persicaria (POLPE, 86%). In comparison, the ARA selective modality controlled 95.75% of the POLPE and 100% for the broadcast modality (Supplementary Figure S1A). This confirms a previous study showing that pelargonic acid has difficulty controlling POLPE (Loddo et al., 2023). The control of Senecio vulgaris (SENVU) reached only 66% in the ARA non-selective modality (compared to 97.5% in the ARA selective and 100% in broadcast modalities; Supplementary Figure S1A). This can be explained by the high density of SENVU (40% in the NT) and indicates that TD should have been applied earlier if conditions had been favorable and that an extra treatment may have been necessary to compensate for the pelargonic acid's low efficacy on older weed stages. Overall, these results are very satisfying, demonstrating that ultra-precise smart spot spraying can be an effective alternative to broadcast treatment in controlling weeds in onion crops with both selective and non-selective programs. As expected, the weed density was very high in the sugar beet trial in this very fertile organic soil (e.g., Chenopodium album, CHEAL, covered almost 50% of the surface in the non-treated control; Supplementary Figures S1B, S2C). Nevertheless, the data show a comparable and respectable efficacy between the ARA's selective and stringent programs and their broadcast-equivalent treatments, with general mean efficacies of 90.3% and 98.8%, respectively (Figure 3A). For now, it is challenging to identify the rationale behind the efficacy gap between ARA selective modality and full spray, as it is difficult to monitor each weed. No difference can be attributed to failed detection and a weak herbicide effect. After three applications of pelargonic acid on sugar beets, the general mean efficacy only reached 60% (Figure 3A). Detailed data showed that three splits of pelargonic acid were sufficient to control Stellaria media (STEME, 98%) but lacked efficacy for other species evaluated in this trial (Supplementary Figure S1B). Despite the high weed pressure, the ARA's non-selective modality managed to lower CHEAL density by 50%. Yet, in conditions of very fertile organic soil and high weed pressure, pelargonic acid does not seem to be an effective standalone approach but should be considered as a complement to a broadcast treatment or a selective spot-spraying program. Nevertheless, pelargonic acid applied using ARA can be considered a weeding solution for sugar beets when the weed pressure is lower. These results show that despite the low mixture volume and molecules applied, ARA controls weeds in both onions and sugar beet crops well, with an efficacy comparable to a broadcast program.
Catch-up treatments and fight against volunteer potatoes
On top of reducing treatment volume with good weeding efficacy, ARA allows for effective catch-up treatments, as exemplified in the onion trial with TE fighting late-germinating AMARE. Targeted ARA sprays eradicated AMARE at BBCH17, with an efficacy >90% after a single herbicide path of a stringent mixture of molecules (Figure 3C, Supplementary Figure S1A, Table 1). This result is impressive, as a broadcast treatment could not have been considered due to the risk to the crop or would have been less effective with a milder mixture.
Similarly, volunteer potatoes in onions can be problematic in some cultural contexts and rotation cycles. Currently, effective herbicide solutions to combat volunteer potatoes in onions are lacking, with no legal molecule available that provides satisfying efficacy. To address this, an ARA spot-spraying treatment was added to the farmer's routine, composed of 18 L/ha of capric acid (288 g/L), caprylic acid (422 g/L) fatty acids, and 0.3 L/ha of fluroxypyr-methyl (480 g/L; Table 3). This program enabled ARA to eradicate 79% of the volunteer potatoes 18 days after treatment (Supplementary Figure S4), with the remaining 21% significantly affected. Notably, no phytotoxicity was observed on the crop. The treatment's success is attributed to using concentrated solutions, the synergistic effect between the three molecules (as a single application of fatty acids or fluroxypyr-methyl did not achieve the 80% efficacy when combined; Supplementary Figure S4B), and a reasonably low density of volunteer potatoes, which allowed efficient weeding without compromising the crop. It is important to note that a higher density of volunteer potatoes might induce collateral phytotoxicity damages on the crop because more product would need to be applied.
In conclusion, ARA controls weeds well and offers new agronomic solutions to emerging problems in which conventional agriculture has gaps.
2.4 ARA improves selectivity
Although the ARA ultra-precise smart spot sprayer is an efficient weeding solution, ensuring crop health remains a priority. Crop health was monitored throughout the trial, focusing on indicators such as leaf necrosis, plant stunting, thinning, and chlorosis, as defined by European and Mediterranean Plant Protection Organization (EPPO) guidelines (EPPO Standards: General Standards, 2023).
In the onion trials, early phytotoxicity was observed in the broadcast modality, with stunting effects (evaluated at 15% 21 days after TC [21 DATC] and 3% at harvest) and some thinning (17.5%; Figures 3B, 4A, E), indicating a risky program for the farmer (Table 1).
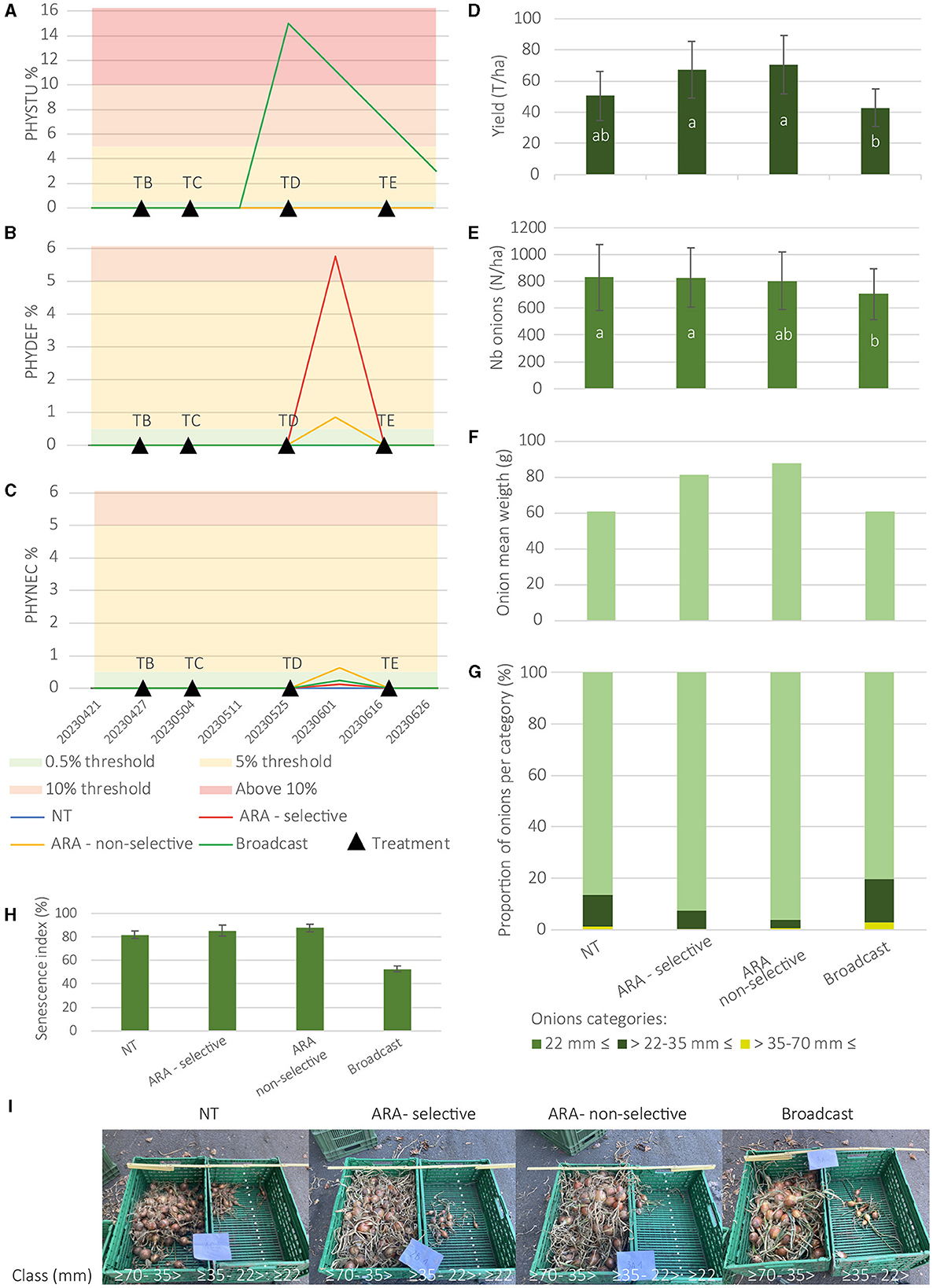
Figure 4. ARA maximizes crop potential by lowering phytotoxicity in onion crops. (A) Percentage of stunting phytotoxicity evaluation over time in the different modalities. (B) Percentage of deformation phytotoxicity evaluation over time in the different modalities, (C) Percentage of necrosis phytotoxicity evaluation over time in the different modalities. (A–C) Horizontal axis indicates the time, vertical axis indicates the percentage of phytotoxicity. (D) Onion yield in ton/hectare in the different modalities. Letters indicate statistical differences. ANOVA: p < 0.01 followed by a Tukey test. (E) Onion yield expressed in number bulbs/hectare in the different modalities. Letters indicate statistical differences. ANOVA: p < 0.05 followed by Tukey test. (F) Extrapolated onion mean weight in grams for the different modalities. (G) Proportion of onions in the different size categories for the different modalities. The proportions represent the number of onions per category. (H) Senescence index evaluated at the harvest. 100% indicates a complete senescence stage. (I) Representative pictures of the onions content for the different modalities and harvested on 1.5 m2.
Despite the high concentration of molecules in the ARA selective modality, no stunting phytotoxicity was observed, suggesting that ARA sprays did not affect the crop at early stages. However, ARA selective TD caused mild leaf deformation (5.8%) 7 days after TD (7 DATD), but the onions quickly recovered, with no deformation observed 22 days after TD (Figure 4B). Additionally, pelargonic acid caused minimal necrosis on onion leaves, evaluated at 0.6% 7 days after TD (Figure 4C). This suggests that the erect shape and waxy properties of onion leaves protect the crop from damage caused by sprays targeting nearby weeds.
In the sugar beet trial, no phytotoxicity was observed in the ARA selective modality; however, the same mixture applied in the broadcast modality caused significant stunting, delaying development by 66% compared to the non-treated modality and causing 15% chlorosis at 7 days after TC (DATC; Supplementary Figures S2C, D). Sugar beet plants exhibit planophilic leaves that expose them directly to ARA spot sprays when weeds are close. Unlike onions, sugar beets do not have waxy leaves. Despite this, only 1.6% necrosis was observed in sugar beets 7 DATC, which is negligible 3 weeks post-treatment (data not shown).
Altogether, this suggests that ARA's AI effectively protects crops from herbicide-induced phytotoxicity. ARA enhances the selectivity of herbicides. AI also provides a certain selectivity to non-selective products allowing their use in established crops at high concentrations, as demonstrated with pelargonic acid (680 g/L) at 16 L/ha. This improved selectivity maximizes the action of herbicides against weeds without compromising crop health. ARA thus alleviates restrictions on the available molecules and increases the application concentration thresholds in targeted crops, allowing higher concentrations than in conventional broadcast treatments to be used while reducing the overall number of molecules applied in the field. Consequently, ARA opens new agronomic horizons.
2.5 ARA maximizes crop potential
Crop health directly impacts plant biomass production and final yields. As shown earlier, broadcast treatments induced phytotoxicity in both the sugar beet and onion trials from which the crop never fully recovered. To highlight ARA's effects on the crops' plant biomass, raw yield, the number of plants, or individual plant size was quantified. The stunting phytotoxicity observed in the broadcast modality in sugar beets (66%; Supplementary Figures S2C, D) reduced biomass production by half in 10-week-old plants compared to the NT (Supplementary Figure S2E). In the onion trial, the development delay seen early in the broadcast modality (Figures 3B, 4A) persisted until harvest, as shown by delayed senescence (50% of dried leaves) compared to the ARA modalities (Figures 3B, 4H, I). Global onion yields showed significant differences between the ARA modalities and broadcast treatments. ARA selective and non-selective modalities yielded 67 T/ha and 70 T/ha, respectively, while the broadcast treatment yielded 43 T/ha (Figure 4D), suggesting that the broadcast treatment program lowered the yield compared to ARA modalities. Compared to the non-treated modality, ARA increased yields by approximately 20% despite using a stringent program (Figure 4D, Table 1).
ARA's spot-spraying application preserves crop integrity, as shown by the number of bulbs (Figures 4E, I), which were comparable between ARA modalities and the non-treated modality, unlike the broadcast modality, which lost 15% of onions compared to the non-treated modality, directly affecting global yield. Additionally, ARA modalities produced heavier onions compared to non-treated and broadcast modalities (Figure 4F). The size distribution of onions in the ARA modalities was more restricted to the larger group (35–70 mm), representing 93% in ARA selective and 96% in ARA non-selective, compared to 87% in the non-treated and only 80% in the broadcast modality (Figures 4G, I). This restricted distribution in ARA modalities can be explained by the random distribution of weeds in the field, resulting in “random” ARA spray applications that prevent the accumulation of phytotoxicity seen in broadcast treatments and mitigate the negative impact of weeds on plant development. This further supports using the ultra-precise smart spot sprayer in vegetable crops.
Overall, ARA eliminates phytotoxicity, clears plant competition with surrounding weeds, encourages natural crop development, and maximizes crop potential for optimal yield.
3 Conclusion and perspectives
To conclude, the ARA algorithms tested in this study demonstrate high performance in controlling weeds in onion and sugar beet crops, achieving weeding efficacies comparable to broadcast treatments. The algorithms effectively decipher crops from weeds, applying the product only where needed, resulting in a weeding efficacy rate higher than 90% (Figure 3A), with negligible phytotoxicity in the managed crops (Figures 4A–C, E, Supplementary Figures S2D, S6). Despite these positive results, definitively asserting a yield increase with ARA compared to traditional broadcast weeding approaches would be premature. Nonetheless, academic partners and independent sources confirm our observations regarding volume savings, weeding efficacy, and reduced phytotoxicity (see Supplementary Table S1, Supplementary Figure S6).
More important, ARA's AI improves the selectivity of selective products and provides selectivity to non-selective or poorly selective herbicides. This artificial selectivity opens new avenues for weeding solutions, particularly where traditional herbicides fail due to advanced weed development, weed resistance, or regulatory withdrawal of certain molecules.
Moreover, the agrochemical industry mostly develops phytosanitary products for broad acre crops with bigger markets and wider economic impacts. Weeding strategies for vegetable crops must accommodate those chemicals. They are often not selective enough in vegetable crops to support broadcast treatments at high concentrations. Using efficient herbicide dosages can cause phytotoxicity on vegetable crops when applied with a broadcast sprayer (as shown in our trials). Therefore, common practices revert to lower molecule concentrations to preserve the crop. Unfortunately, such concentrations are not sufficient to kill the weeds in one shot, and farmers have to multiply the frequency of interventions, contributing to weed resistance (Powles and Yu, 2010), and increases carbon dioxide (CO2) emissions.
Ultra-precise smart spot spraying offers a viable solution by (1) allowing the use of higher herbicide concentrations for effective weeding, without crop contact; (2) minimizing the number of interventions, thereby lowering CO2 emissions; (3) reducing overall herbicide volumes, focusing application only where necessary, thus preserving the environment; and (4) lowering phytotoxicity, delivering healthier food, and maximizing yield potential. However, caution is needed when using high concentrations, especially in high weed density contexts, to avoid exceeding regulatory limits and increasing phytotoxicity risks.
The withdrawal of molecules underscores the need for legal flexibility in using spot sprayers to bridge gaps while developing more sustainable solutions. From ecological, ecotoxicological, agronomic, and economic perspectives, establishing guidelines for spot sprayers use is crucial. Considering the benefits, ultra-precise smart spot spraying for other crops should be explored and compared with other innovative weeding solutions, such as laser or hoeing tools, to evaluate efficacy, crop protection, and production gains. Complementary studies will investigate ARA's potential for applying insecticides, fungicides, or foliar fertilizers, aiming to maximize yield while reducing environmental impact. Evaluating the positive environmental impact, including CO2 cost, across different modalities will be essential.
This study highlights that ARA drastically reduces herbicide use to control weed populations. The current index for evaluating bio-control chemicals needs reassessment to consider factors like water solubility, toxicology, and environmental fate. Testing a range of natural and non-selective compounds could enhance the benefits of ultra-precise spot sprayers; reduce reliance on synthetic chemicals, CO2 footprint, and ecological impact; and address weed-resistance issues. The potential for enhancing the spray accuracy and dose control is still broad. To foster acceptance of ultra-precise smart spot spraying, further improvements in spray accuracy and dose control are needed, particularly for non-selective herbicides. Integrating this technology into larger, cost-effective machines will make it accessible for lower value crops.
The ARA sprayer and its AI are constantly being improved, with algorithms upgrades and new functions and crops being implemented. As technology costs decrease, the potential of ultra-precise spot spraying and AI in agriculture is vast. An ultra-precise smart spot-spraying approach has the potential to revolutionize agronomy by bringing a profusion of solutions to the field and meeting evolving agronomic constraints. Ultra-precise spot sprayers optimize resource use and pave the way for future farming to be a more sustainable through enhanced adaptability, reduced phytotoxicity, and maximized yield potential.
4 Material and methods
4.1 Experimental design and cultivation
The onion trial was led in Switzerland (46.951844, 7.151822), within a farmer's field, on a mineral soil following wheat and lettuce crops. The onions were sown in 1.5-m-wide beds, each containing four rows of onions. The onions were sown with a pneumatic vegetable sowing machine Agricola Italiana SNT-2-290 at the density of 90,000 seeds per hectare, variety: crockett (Bejo, https://www.bejo.com/onion/crockett). Two true-repetition 6 × 30-m plots were designed for the modalities (ARA selective, ARA non-selective, farmer). In each true repetition, two pseudo-replicates were designated. This leads to four pseudo-replicates per modality. The non-treated control represented a 2 × 12-m band separating the ARA replicates (Supplementary Figure S5C). The onions were sown on March 23, 2023, and the crop was monitored up to harvesting on August 18, 2023 (Supplementary Figure S5A).
The volunteer potato trial was led on the same field as the onion trial but on a section where potatoes preceded the onion crop.
The second onion trial presented was led in Germany (48.741404, 12.884032) on silty clay soil, following a winter cover crop composed of white mustard (Sinapis alba) and large-seed false flax (Camelina sativa) sown after wheat crop. The data of each modality represent one single replicate evaluation of a 6 × 50-m plot. The treatment program is available in Supplementary Table S1.
The sugar beets trials were led in Switzerland (46.751874, 6.557217), on our experimental platform, in a loamy highly organic soil, following a corn crop. The sugar beets were sown by the grower with a pneumatic single-grain sowing machine at the density of 117,000 seeds per ha (variety: Agueda, KWS; https://www.kws.com/ch/fr/). Four true repetition plots of 6 × 30 m were designed per modality. In each true replicate, two pseudo-replicates were designated. This leads to eight pseudo-replicates per modality. The non-treated control represented three bands of 2 × 36 m, separating the replicates (Supplementary Figure S5D). The sugar beets were sown on March 23, 2023, and the crop was monitored until June 1, 2023 (Supplementary Figure S5B).
4.2 Treatments and mixtures
In this study, the quantities of indicated molecules were diluted to an equivalent of 200 L/ha as ARA works on a basis of 200 L/ha of a full spray equivalent. For every treatment applied in this study, the calculated sprayed volumes, and their relative economies were based on 200 L/ha.
The crop programs (calendars and mixtures) can be consulted in Tables 1–3, Supplementary Table S1, Supplementary Figures S5A, B. The volumes/masses of commercially available formulated herbicides were adjusted to the preparation of batches of a final volume of 30 L of mixture and diluted in water under agitation. This 30-L volume is enough to cover the total surface of each modality based on a 200 L/ha (total surface spray). After the initiation process, the mixtures were spread on the field at the speed of 7.2 km/h and with a working pressure of 3.0 bar (3,000 hPa), as recommended by Ecorobotix, to reach an equivalent flow of 200 L/ha. Depending on the algorithm–herbicide combination, a security zone was either set or not (Tables 1–3). To avoid any residues from one modality contaminating the next, the mixture tank was conscientiously rinsed between two modalities. The broadcast treatment performed in the sugar beets corresponds to a 200 L/ha application and, in the onion trial, to a 300 L/ha application.
As the ARA combines a hardware structure controlled by a software, the ARA sprayer is constantly improved and evolves over time (bug fixes, new functions, new crops, etc.). Supplementary Figures S5A, B recapitulated the treatments applied in our trial and the corresponding version releases of ARA over time.
Only herbicide treatments were applied differently between the modalities. All the other crop management interventions were equally applied to the fields and are believed to have not interfered with the results presented here.
4.3 Agronomic parameters and yield
The herbicide treatments' efficacy and selectivity were evaluated according to the EPPO standards PP1/152(4), PP1/135 (4), and PP1/181 (5) (EPPO Standards: General Standards, 2023). For onions, the specific PP1/075(3) guidelines were used [PP1/075(3) - Weeds in Allium Crops, 2008]. In the sugar beets trial, PP1/052(4) guidelines were followed [PP1/052(4) - Weeds in Sugar and Fodder Beet and Industrial Chicory, 2020]. To estimate the yield of the onions, consecutive onions of two rows of two meters (1.5 m2) were collected. The onions were immediately calibrated according to the reference table provided by the Swiss association of vegetable market (Prescriptions suisses de la qualité pour les légumes, 2023) in three categories ( ≤ 22 mm, >22–35 mm ≤ , >35–70 mm ≤ ). Each category was weighted, and the number of bulbs per category quantified. Yield was extrapolated to standard unit tons/ha or N/ha. Data show the means of four pseudo-replicates. Twenty sugar beets per repetition were rooted out after 10 weeks' growth and immediately weighed. The data show the mean biomass per plant of the four replicates.
4.4 Climatic data
Climatic data were collected by Agrometeo, the Swiss federal climatic service (https://www.agrometeo.ch/). In this study, the provided climatic data correspond to the closest station from the trials: Praz's data were used for the Swiss onion trials, and Pomy's for the sugar beet trials. For the details, see Supplementary Figure S7. For the forecast conditions in the onion trial led in Germany, refer to Supplementary Figure S6E (https://meteostat.net), which shows the Metten station recordings.
4.5 Statistical analysis
Statistical analyses (analysis of variance tests followed by Tukey tests with a confidence level of 95%) were processed on RStudio software (http://www.rstudio.com/) using the Agricolae package (http://cran.r-project.org/web/packages/agricolae/index.html).
Data availability statement
The raw data supporting the conclusions of this article will be made available by the authors, upon reasonable request.
Author contributions
PA: Methodology, Investigation, Formal analysis, Visualization, Writing – review & editing, Writing – original draft. SG: Methodology, Investigation, Writing – review & editing. MG: Methodology, Investigation, Validation, Funding acquisition, Writing – review & editing. ST: Conceptualization, Writing – review & editing.
Funding
The author(s) declare financial support was received for the research, authorship, and/or publication of this article. The Ecorobotix company internally funded its own research on ARA led in Switzerland. The German trial, led in lower Bavaria, was supported by die Bayerisches Staatsministerium für Ernährung, Landwirtschaft und Forsten (the Bavarian State Ministry of Food, Agriculture and Forestry) and carried out by the Bavarian State Institute for Viticulture and Horticulture under the identification A/23/02 (https://www.lwg.bayern.de/gartenbau/gemuesebau/348376/index.php) and owned by MG.
Acknowledgments
First, we would like to thank the farmers who provided the onion field and the tractor to lead our trial in onions and the Centre Pénitentiaire de la Plaine de l'Orbe for providing the sugar beet field and taking care of all the agronomic work regarding soil preparation and sowing tasks. We would like to thank all the Ecorobotix team members, current or former, who have been involved in the development of the ARA. A particular thanks to our first customers who support our solution and the academic and public partners who led weeding field trials with ARA over the past 3 years (Unilet, CTIFL, Südzucker, University of Göttingen, Tereos, la Ferme pilote du groupe Carré, Eureden, HAFL, CRAW, Pfeifer und Langen, la Chambre d'Agriculture du Loiret, Agropole de Molondin). They independently confirmed our observations and helped us improve the ARA technology via their constructive feedback. Thank you to Abbey Flury for the proofreading and strong inputs that improved the overall quality of the manuscript.
Conflict of interest
PA, SG and ST were employed by Ecorobotix SA. Ecorobotix SA had the following involvement in the study: development of the ARA; and financial support to lead the trials performed in Switzerland only.
The remaining authors declare that the research was conducted in the absence of any commercial or financial relationships that could be construed as a potential conflict of interest.
Publisher's note
All claims expressed in this article are solely those of the authors and do not necessarily represent those of their affiliated organizations, or those of the publisher, the editors and the reviewers. Any product that may be evaluated in this article, or claim that may be made by its manufacturer, is not guaranteed or endorsed by the publisher.
Supplementary material
The Supplementary Material for this article can be found online at: https://www.frontiersin.org/articles/10.3389/frevc.2024.1394315/full#supplementary-material
References
Andela (2024). Products of Andela. AndelaTechniek & Innovatie B.V. Available at: https://www.andela-tni.nl/en/ (accessed April 26, 2024).
Begemann, J., Ostovar, S., and Schwake-Anduschus, C. (2021). Facing tropane alkaloid contamination in millet. Qual. Assur. Saf. Crop. 13, 79–86. doi: 10.15586/qas.v13i2.888
Bloomer, D. J., Harrington, K. C., Ghanizadeh, H., and James, T. K. (2024). Robots and shocks: emerging non-herbicide weed control options for vegetable and arable cropping. New Zeal. J. Agricult. Res. 67, 81–103. doi: 10.1080/00288233.2023.2252769
Blue River (2024). Welcome – Welcome | Blue River Technology. Available at: https://bluerivertechnology.com/our-products/ (accessed May 3, 2024).
CarbonRobotics (2024). First & Only Commercial LaserWeeder™. Carbon Robotics. Available at: https://carbonrobotics.com/ (accessed April 26, 2024).
Carey, J. (2023). Unearthing the origins of agriculture. Proc. Nat. Acad. Sci. 120:e2304407120. doi: 10.1073/pnas.2304407120
Colbach, N., and Cordeau, S. (2018). Reduced herbicide use does not increase crop yield loss if it is compensated by alternative preventive and curative measures. Eur. J. Agronomy 94, 67–78. doi: 10.1016/j.eja.2017.12.008
DGAL/SDSPV/2023-282 (2023). Available at: https://info.agriculture.gouv.fr/gedei/site/bo-agri/instruction-2023-282 (accessed November 20, 2023).
Ecorobotix (2023). Ecorobotix: Smart spraying for ultra-localised treatments. Ecorobotix. Available at: https://ecorobotix.com/en/ara/ (accessed November 20, 2023).
EPPO Standards: General Standards (2023). EPPO PP1 – List General Standards. Available at: https://pp1.eppo.int/standards/general (accessed November 20, 2023).
FAOSTAT (2023). FAOSTAT. Available at: https://www.fao.org/faostat/en/#data/SDGB (accessed November 20, 2023).
Farm to Fork Strategy (2023). Farm to Fork Strategy. Food Safety. Available at: https://food.ec.europa.eu/horizontal-topics/farm-fork-strategy_en (accessed November 20, 2023).
Farmdroid (2024). Transform Your Farm with FarmDroid: Unleash Sustainable Growth. FarmDroid. Available at: https://farmdroid.com/ (accessed April 26, 2024).
Garford (2024). Garford Farm Machinery Ltd: Precision Guided Hoes & InRow Weeders. Garford Farm Machinery. Available at: https://garford.com/ (accessed April 26, 2024).
Horvath, D. P., Clay, S. A., Swanton, C. J., Anderson, J. V., and Chao, W. S. (2023). Weed-induced crop yield loss: a new paradigm and new challenges. Trends Plant Sci. 28, 567–582. doi: 10.1016/j.tplants.2022.12.014
Hussain, M., Farooq, S., Merfield, C., and Jabran, K. (2018). “Mechanical weed control,” in Non-Chemical Weed Control (London: Elsevier), 133–155.
Kadioglu, I., Yanar, Y., and Asav, U. (2005). Allelopathic effects of weeds extracts against seed germination of some plants. J. Environ. Biol. 26, 169–73.
Kareiva, P., Watts, S., McDonald, R., and Boucher, T. (2007). Domesticated nature: shaping landscapes and ecosystems for human welfare. Science 316, 1866–1869. doi: 10.1126/science.1140170
Korres, N. E., Burgos, N. R., Travlos, I., Vurro, M., Gitsopoulos, T. K., Varanasi, V. K., et al. (2019). “New directions for integrated weed management: modern technologies, tools and knowledge discovery. Adv. Agron. 155, 243–319. doi: 10.1016/bs.agron.2019.01.006
Loddo, D., Jagarapu, K. K., Strati, E., Trespidi, G., Nikolić, N., Masin, R., et al. (2023). Assessing herbicide efficacy of pelargonic acid on several weed species. Agronomy 13:1511. doi: 10.3390/agronomy13061511
Mantle, P. G., Shaw, S., and Doling, D. A. (1977). Role of weed grasses in the etiology of ergot disease in wheat. Ann. Appl. Biol. 86, 339–351. doi: 10.1111/j.1744-7348.1977.tb01848.x
McGiffen, M. E., Masiunas, J. B., and Huck, M. G. (1992). Tomato and nightshade (Solanum nigrum L. and S. ptycanthum Dun.) effects on soil water content. J. Am. Soc. Horticult. Sci. 117, 730–735. doi: 10.21273/JASHS.117.5.730
Mesías-Ruiz, G. A., Pérez-Ortiz, M., Dorado, J., de Castro, A. I., and Peña, J. M. (2023). Boosting precision crop protection towards agriculture 5.0 via machine learning and emerging technologies: a contextual review. Front. Plant Sci. 14:1143326. doi: 10.3389/fpls.2023.1143326
Morais, T. G., Teixeira, R. F. M., Lauk, C., Theurl, M. C., Winiwarter, W., Mayer, A., et al. (2021). Agroecological measures and circular economy strategies to ensure sufficient nitrogen for sustainable farming. Global Environm. Change 69:102313. doi: 10.1016/j.gloenvcha.2021.102313
Murad, N. Y., Mahmood, T., Forkan, A. R. M., Morshed, A., Jayaraman, P. P., and Siddiqui, M. S. (2023). Weed detection using deep learning: a systematic literature review. Sensors 23:3670. doi: 10.3390/s23073670
Niemeyer, M., Renz, M., Pukrop, M., Hagemann, D., Zurheide, T., Di Marco, D., et al. (2024). Cognitive Weeding: An Approach to Single-Plant Specific Weed Regulation. Switzerland: KI - Künstliche Intelligenz.
Oerke, E.-C. (2006). Crop losses to pests. J. Agric. Sci. 144, 31–43. doi: 10.1017/S0021859605005708
Office fédéral de la sécurité alimentaire et des affaires vétérinaires OSAV – Index des produits phytosanitaires (2023). Available at: https://www.psm.admin.ch/fr/produkte (accessed November 20, 2023).
Orlando, B., Carrera, A., Bibard, V., and Meleard, B. (2019). Datura Stramonium Dans Le Maïs : Enjeux Sanitaires Et Complexite De Gestion. Available at: https://ecophytopic.fr/sites/default/files/2020-12/2020_PHLOEME_Alcaloides_Ergot%26Datura.pdf (accessed August 5, 2024).
Parker, C. (2009). Observations on the current status of Orobanche and Striga problems worldwide. Pest Manag. Sci. 65, 453–459. doi: 10.1002/ps.1713
Powles, S. B., and Yu, Q. (2010). Evolution in action: plants resistant to herbicides. Annu. Rev. Plant Biol. 61, 317–347. doi: 10.1146/annurev-arplant-042809-112119
PP1/052(4) - Weeds in Sugar and Fodder Beet and Industrial Chicory (2020). EPPO PP1 - PP1/052(4) - Weeds in sugar and fodder beet and industrial chicory. Available at: https://pp1.eppo.int/standards/PP1-052-4 (accessed November 20, 2023).
PP1/075(3) - Weeds in Allium Crops (2008). EPPO PP1 - PP1/075(3) - Weeds in Allium crops. Available at: https://pp1.eppo.int/standards/PP1-075-3 (accessed November 20, 2023).
Prescriptions suisses de la qualité pour les légumes (2023). Prescriptions suisses de la qualité pour les légumes. Qualiservice. Available at: https://www.qualiservice.ch/legumes.html (accessed November 20, 2023).
Rajcan, I., and Swanton, C. J. (2001). Understanding maize–weed competition: resource competition, light quality and the whole plant. Field Crops Res. 71, 139–150. doi: 10.1016/S0378-4290(01)00159-9
See and Spray (2024). John Deere. See & Spray™ Ultimate | Precision Ag | John Deere US. Available at: https://www.deere.com/en/sprayers/see-spray-ultimate/ (accessed May 3, 2024).
Tanner, S. (2022). Procédé et système de pulvérisation par points pour une application de haute précision de produits agrochimiques. Available at: https://worldwide.espacenet.com/patent/search/family/075267430/publication/WO2022195380A1?q=pn%3DWO2022195380A1 (accessed November 20, 2023).
Tanner, S., Demaurex, A., and Mondana, G. (2021). Robot vehicle and method using a robot for an automatic treatment of vegetable organisms. Available at: https://worldwide.espacenet.com/patent/search/family/054207259/publication/PL3316673T3?q=pn%3DPL3316673T3 (accessed November 20, 2023).
Teyker, R. H., Hoelzer, H. D., and Liebl, R. A. (1991). Maize and pigweed response to nitrogen supply and form. Plant Soil 135, 287–292. doi: 10.1007/BF00010918
van Steenbergen, G., de Hoog, D., Djouhri-Touri, M., Holterman, H. J., and van de Zande, J. (2024). Spray Drift Evaluation of the Ecorobotix ARA Spot Sprayer: A Technical Performance Comparison Between the Ecorobotix ARA and the Shielded Bed Sprayer. Available online at: https://edepot.wur.nl/642717 (accessed August 5, 2024).
WeedBot (2024). Available at: https://weedbot.eu/ (accessed May 3, 2024).
Keywords: precision agriculture, ultra-precise spot sprayer, herbicides, weeding, yield increase, onions, sugar beets, artificial intelligence
Citation: Anne P, Gasser S, Göttl M and Tanner S (2024) The reduction of chemical inputs by ultra-precise smart spot sprayer technology maximizes crop potential by lowering phytotoxicity. Front. Environ. Econ. 3:1394315. doi: 10.3389/frevc.2024.1394315
Received: 01 March 2024; Accepted: 22 July 2024;
Published: 23 September 2024.
Edited by:
Philipp Aerni, School of Management Fribourg (HES-SO), SwitzerlandReviewed by:
Michael Kock, Dr. Kock Consulting, SwitzerlandSimerjeet Kaur, Punjab Agricultural University, India
Copyright © 2024 Anne, Gasser, Göttl and Tanner. This is an open-access article distributed under the terms of the Creative Commons Attribution License (CC BY). The use, distribution or reproduction in other forums is permitted, provided the original author(s) and the copyright owner(s) are credited and that the original publication in this journal is cited, in accordance with accepted academic practice. No use, distribution or reproduction is permitted which does not comply with these terms.
*Correspondence: Pauline Anne, UGF1bGluZS5hbm5lQGVjb3JvYm90aXguY29t; Steve Tanner, U3RldmUudGFubmVyQGVjb3JvYm90aXguY29t