- Department of Systems and Computing Engineering, Universidad Católica del Norte, Antofagasta, Chile
Electrification of transport is accelerating worldwide, raising new challenges for energy efficiency and control in electric vehicles. Reinforcement learning has emerged as a promising data-driven approach to address the complexity of real-time energy management. This review presents a structured synthesis of open-access research published between 2016 and 2024 on the application of reinforcement learning methods to electric vehicle energy optimization. The study formulates four guiding research questions to analyze types of learning algorithms, evaluation criteria, system-level constraints, and practical implementation aspects. Key contributions include a comparative mapping of reinforcement learning techniques—such as Q-learning, deep deterministic policy gradient, twin delayed deep deterministic policy gradient and soft actor-critic—their applicability to electric vehicle control scenarios, and the identification of current research gaps and deployment challenges. The findings aim to support researchers and engineers in selecting suitable reinforcement learning strategies for efficient and scalable electric vehicle energy management.
1 Introduction
Energy management for electric vehicles is becoming increasingly significant in both scientific research and industry (Yang et al., 2020). In the current context, where climate change and the quest for new energy sources for locomotion are critical challenges, the adoption of electric vehicles has gained substantial relevance. Electrical vehicles not only contribute to reducing carbon emissions (Harvey, 2020) but also present the opportunity to implement advanced artificial intelligence techniques, such as reinforcement learning, to optimize their energy performance.
Despite significant progress in electric vehicle technologies, optimizing energy management strategies remains a critical challenge due to highly variable operating conditions and the need for real-time adaptation. Traditional control methods often fall short when dealing with such complexity, motivating the search for more flexible, data-driven approaches like reinforcement learning.
Reinforcement learning (RL), a technique derived from dynamic programming, has demonstrated considerable potential for addressing complex and nonlinear problems (Perrusquía and Yu, 2021). Its ability to learn and adapt through interaction with the environment renders it particularly well-suited to the management of energy systems in electric vehicles. This suitability arises because electric-vehicle energy management must respond in real time to highly dynamic driving conditions—such as variations in acceleration, regenerative-braking events, road grade, and vehicle load—that traditional model-based controllers struggle to anticipate without extensive recalculation (Kasri et al., 2024). Reinforcement-learning agents, by continuously updating their control policies through trial-and-error, can adjust power distribution on-line to maximize battery efficiency and recuperation under uncertain and non-stationary scenarios (Oubelaid et al., 2022). With recent advancements in hardware and increasing processing capabilities, reinforcement learning has experienced a significant surge in its applicability and effectiveness across various areas of artificial intelligence (Naeem et al., 2020).
The significance of reinforcement learning has seen a marked increase since 2016, underscored by a sustained growth in interest as observed in global trends data, as can be seen in Figure 1. Despite a temporary decline in 2020, the field rebounded strongly in subsequent years, particularly since 2022, which marked a significant turning point. This resurgence can be attributed to the continuous advancements in artificial intelligence and the successful application of RL in various domains, including robotics, game theory, and autonomous systems (Prudencio et al., 2023). With the increasing adoption of electric vehicles as a response to climate change, energy management remains a critical challenge. Traditional optimization methods fall short in handling real-time complexities and environmental variability. This review bridges the gap by focusing on reinforcement learning, a promising solution capable of adapting to dynamic scenarios and providing scalable energy management strategies.
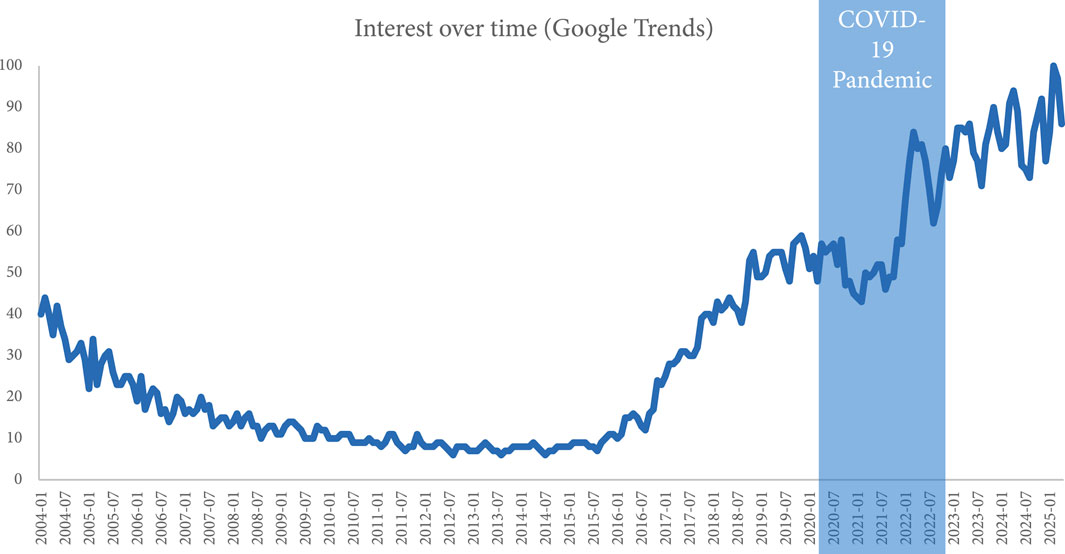
Figure 1. Interest in reinforcement learning over time (2016–2025) based on Google Trends data. The graph shows normalized search volume (0–100) with quarterly averages. Data collected on 1 March 2025. Notable peaks correspond to major breakthroughs in the field, including the surge in 2022 due to increased applications in energy management in electric vehicles. Source: (Google Trends, 2024).
The ability of RL algorithms to optimize complex decision-making processes in dynamic environments has positioned it as a critical area of research within machine learning. The growing academic and commercial interest highlights RL’s potential to drive innovation in diverse applications, solidifying its role as a cornerstone in the future of intelligent systems (Zai and Brown, 2020).
The adoption of electric vehicles, while promising, is confronted with a multitude of challenges, including the effective planning and simulation of their energy consumption (Cao et al., 2020). Proper energy management is of critical importance for maximizing the range and efficiency of these vehicles. Reinforcement learning can play an essential role in this regard. This approach allows for the formulation of energy management strategies that can respond to diverse driving conditions and environmental variability, thereby optimizing energy consumption (Liu et al., 2020).
The main purpose of this review is to conduct a comprehensive review of the application of reinforcement learning to energy management in electric vehicles by analyzing open-access articles published in the Web of Science database. Furthermore, we aim to identify and analyze the current methodologies, results, and trends in this field of study. In particular, the research questions are:
• RQ1: Which are the most employed RL methods in the context of energy management in electric vehicles?
• RQ2: How do RL methods improve efficiency in the context of energy management in electric vehicles?
• RQ3: What are the challenges of implementing RL methods in the context of energy management in electric vehicles?
• RQ4: How is performance measured for RL methods in the context of energy management in electric vehicles?
In contrast to previous reviews that broadly address reinforcement learning in energy systems or hybrid configurations, this work offers a focused and up-to-date synthesis of open-access applications of RL specifically in the energy management of electric vehicles. It distinguishes itself by prioritizing accessibility, reviewing only peer-reviewed and publicly available studies, and analyzing their methodological contributions across efficiency, adaptability, and real-world feasibility dimensions. By covering recent developments up to 2024, this review bridges the gap between theoretical advances and practical implementations, offering a valuable roadmap for researchers and practitioners working on scalable and intelligent control strategies in EVs.
Recent works have significantly advanced the field of electric vehicle control and energy optimization (Oubelaid et al., 2022; Belkhier et al., 2023; Kasri et al., 2024). These studies offer robust and experimentally validated approaches based on heuristic and model-based control strategies, demonstrating strong potential for real-world deployment. Inspired by their contributions, this review complements such efforts by focusing specifically on reinforcement learning methods, analyzing how these adaptive techniques can enhance energy management performance under dynamic and uncertain driving conditions.
The structure of the article follows four research questions, which guide the analysis of common RL methods, comparative performance, implementation challenges, and validation techniques. The remainder of the paper is organized as follows: Section 2 presents background theory; Section 3 details the methodology used for selecting and analyzing the literature; Section 4 provides a comparative analysis of the reviewed approaches; and Section 5 discusses open challenges and future research directions.
2 Theoretical background
2.1 Reinforcement learning
Reinforcement learning is a subfield of machine learning where an agent learns to make decisions by interacting with an environment. The core components of RL include the agent, the environment, actions, states, rewards, and policies. The agent’s goal is to learn a policy that maximizes cumulative rewards by mapping states to actions (Ding et al., 2020). RL has evolved from classical control theory and behavioral psychology, where early methods were based on trial-and-error approaches. Significant advances have been made with the advent of deep learning, leading to the development of algorithms like Deep Q-Networks (DQN) and Policy Gradient methods, which have shown remarkable success in complex environments (Li, 2017).
Reinforcement learning shares a strong foundation with Dynamic Programming (DP), particularly in how both methods address sequential decision-making problems (Barto, 1995). In DP, the Bellman equation is central, providing a recursive decomposition of the value function, which represents the optimal cost-to-go for a given state. RL leverages this concept by approximating the value function through iterative methods such as value iteration or policy iteration, without requiring a complete model of the environment.
The value function
where:
•
•
•
•
•
Similarly, the action-value function
These recursive formulations are essential for solving RL problems via dynamic programming or approximations in the absence of a complete model. RL approximates this process iteratively, either through value-based methods (e.g., Q-learning) or policy-based methods. Reinforcement learning environments are typically modeled as Markov Decision Processes (MDPs), where the Markov property holds (Sutton et al., 1999). This implies that the future state depends only on the current state and action, not on the sequence of events that preceded it. The MDP framework provides a formalization for the interaction between the agent and the environment, facilitating the use of RL algorithms. An MDP is defined by the tuple
•
•
•
•
•
The Markov property is mathematically expressed in Equation 3 as:
To map the Markov decision process of Equation 3 onto real hardware, we present in Figure 2 a system-level block diagram of the reinforcement-learning control loop. The electric-vehicle environment consists of five main subsystems—battery pack, power electronics, electric motor, road-load model and regenerative-braking unit—through which energy flows bidirectionally under vehicle dynamics. At each time step
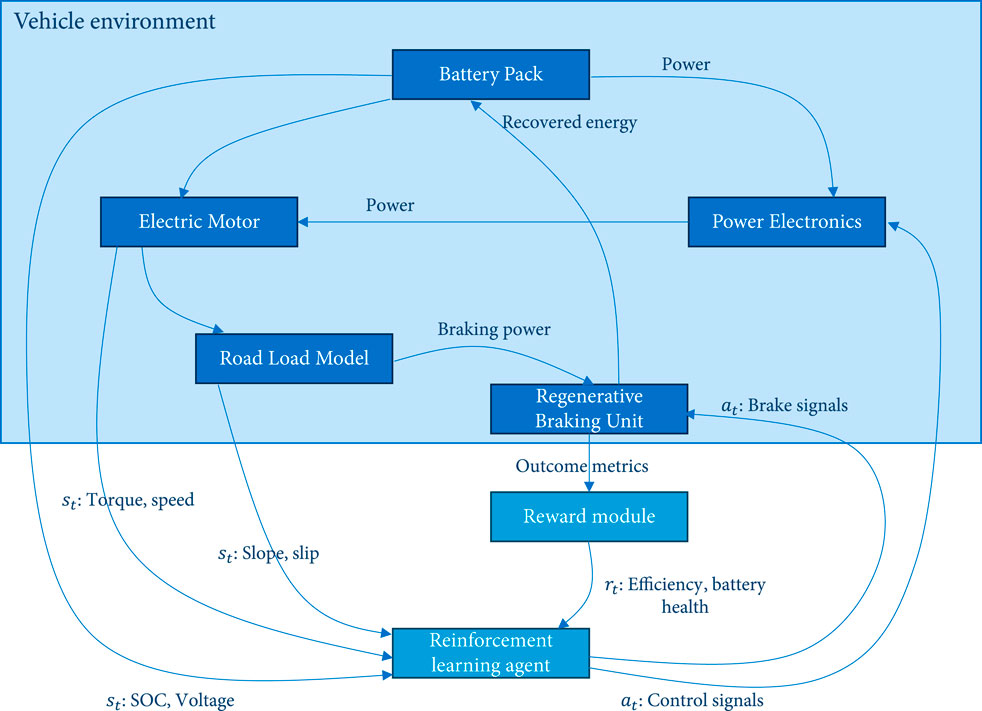
Figure 2. System-level block diagram of the reinforcement learning control architecture for electric vehicle energy management. The agent observes subsystem states
This simplification allows for more affordable solutions in RL problems. In RL, the reward function
The objective in RL is to maximize the expected return over time (Brys et al., 2014). It can be achieved with a good policy. A policy
2.2 Energy management in electric vehicles
Energy management in electric vehicles refers to the process of optimizing the use of available energy resources to enhance vehicle performance, extend driving range, and improve overall efficiency. It involves controlling various subsystems, such as battery management, regenerative braking, and power distribution (Liu et al., 2021). Effective energy management is critical in electric vehicles due to the limited energy storage capacity of batteries. Optimizing energy usage not only maximizes driving range but also prolongs battery life and ensures the vehicle operates within safe parameters (Wu et al., 2020). RL techniques are increasingly being explored as they offer adaptive and real-time decision-making capabilities that are essential for dynamic energy management.
In orde to determine the control scenarios for different electrified vehicle architectures, Figure 3 breaks down the energy-flow paths into three distinct configurations: (a) parallel hybrid-electric vehicle, (b) series hybrid-electric vehicle, and (c) battery-electric vehicle. In panel 3a, the internal-combustion engine and the electric motor jointly deliver torque to the transmission, and regenerative braking returns energy electrically to the battery. In panel 3b, the internal-combustion engine drives a generator that charges the battery and/or powers the electric motor. In panel 3c, power flows unidirectionally from the battery through the DC/AC inverter to the electric motor and wheels, with braking energy recuperated back into the battery. These separate views clarify the different state and action spaces—and thus the distinct reinforcement-learning control challenges—associated with each topology (Schulz-Mönninghoff et al., 2021).
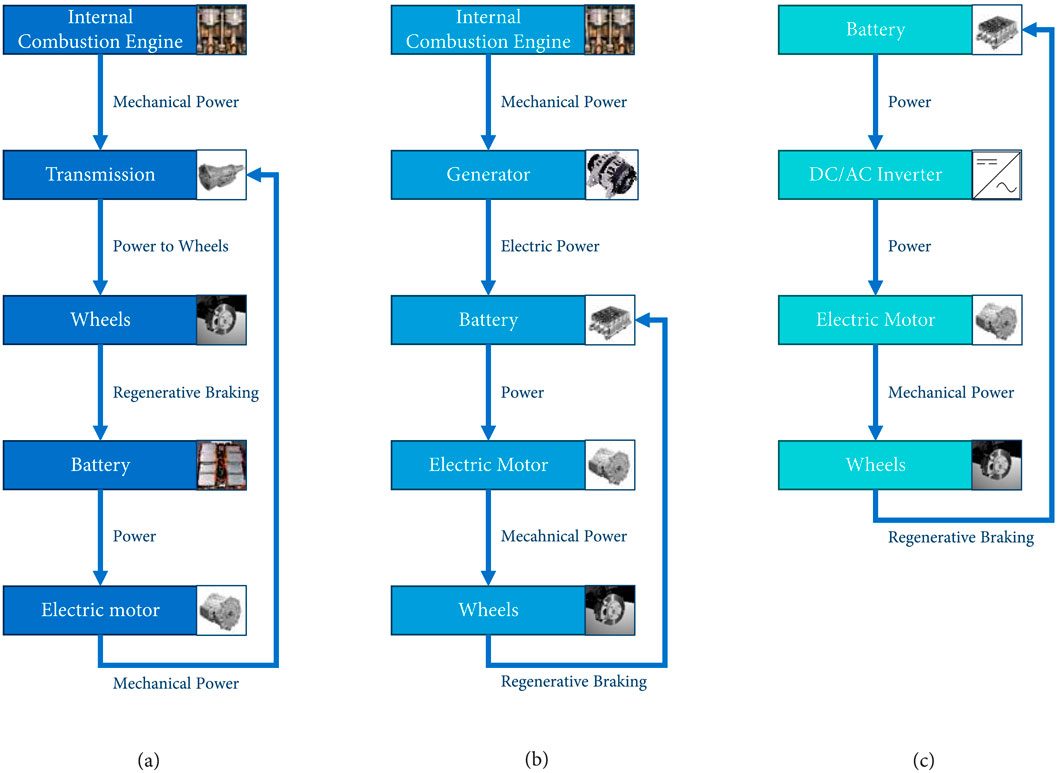
Figure 3. System-level power-flow architectures for (a) parallel hybrid-electric, (b) series hybrid-electric, and (c) battery-electric vehicles, with regenerative-braking feedback loops. In panel (a), mechanical torque from both the internal-combustion engine and electric motor is combined at the transmission before wheel propulsion, defining a dual-actuator action space for torque-split policies. Panel (b) shows how the engine drives a generator to charge the battery or power the motor, creating a sequential energy path that shapes the agent’s state-action mapping for charge-management strategies. Panel (c) depicts a pure battery-electric configuration, where energy flows from the battery through the DC/AC inverter to the motor and wheels, with regeneration feeding back during braking-ideal for model-free policies focused solely on energy efficiency and battery health. Source: Own elaboration.
The electric motor, which directly converts electrical energy into mechanical energy, drives the vehicle’s propulsion system. The mechanical energy generated by the motor is then transmitted through the transmission system to the differential. The differential is responsible for distributing the mechanical power to the wheels, facilitating vehicle movement. This mechanical energy transfer is depicted by the blue arrows in the diagram, representing the physical power flow from the motor to the wheels.
Oubelaid et al. (2022) propose an intelligent control scheme for battery electric vehicles (BEVs) based on vector control of a permanent magnet synchronous motor (PMSM), where speed and current PI controllers are tuned using bio-inspired optimization techniques, namely Particle Swarm Optimization (PSO) and Genetic Algorithms (GA). Their tuning approach relies on two cost functions tailored to both step inputs and realistic driving cycles, such as the ECE-15. The system incorporates environmental disturbances, including a 10° road slope and constant wind speed, and is evaluated under varying vehicle dynamics. Results show that PSO and GA yield significantly lower absolute speed and torque errors (approximately
To better highlight the differences and commonalities among recent intelligent control approaches for electric vehicles, a comparative evaluation is presented in Figure 4. This figure synthesizes five key dimensions observed across three representative works: adaptivity, real-world validation, optimization sophistication, control robustness, and deployment feasibility. The scoring ranges from 1 (minimal presence) to 5 (strong or advanced presence), based strictly on criteria extracted from each article’s methods and results.
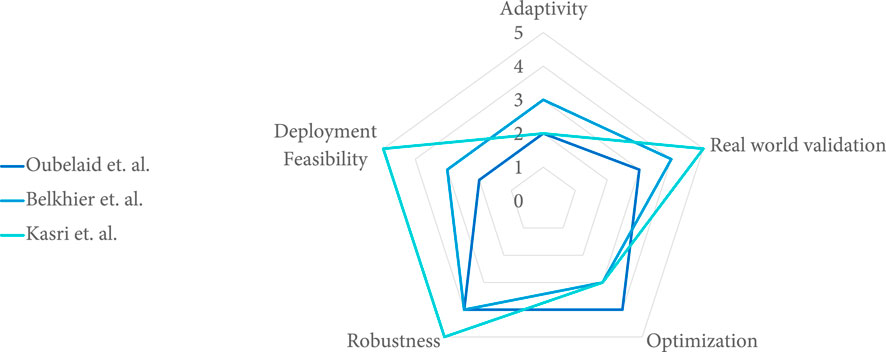
Figure 4. Comparative radar chart of three selected recent contributions to EV energy management systems. Scores are based on criteria including adaptivity, real-world validation, optimization approach, robustness, and deployment feasibility, using a scale from 1 (minimal) to 5 (advanced). Source: Own elaboration.
While Oubelaid et al. (2022) demonstrates strong optimization capabilities through the use of PSO and GA for offline tuning, it lacks adaptive behavior and is limited to simulation-based validation. Belkhier et al. (2023) introduces a partially adaptive fuzzy-sliding mode controller that effectively handles mass variations and is tested under standardized FTP-75 driving cycles. In contrast, Kasri et al. (2024) achieves the highest levels of robustness and feasibility through a predictive torque control strategy implemented on real-time hardware (OPAL-RT), although it does not include adaptive learning mechanisms.
Unlike hybrid systems, electric vehicles rely solely on electrical energy for propulsion, eliminating the need for internal combustion engines and associated components such as clutches or gearboxes. The electric system’s efficiency is enhanced through regenerative braking, where the electric motor functions as a generator during deceleration. This process converts kinetic energy back into electrical energy, which is then fed into the battery for storage, as indicated by the green arrows in the diagram. This regenerative mechanism not only improves the overall energy efficiency of the vehicle but also extends the driving range by recovering energy that would otherwise be lost as heat in traditional braking systems.
2.3 Integration of energy management and reinforcement learning in electric vehicles
RL is well-suited for energy management tasks in electric vehicles due to its ability to learn optimal strategies in complex and uncertain environments. By continuously interacting with the vehicle’s powertrain and external conditions, an RL-based controller can adapt to changes in real-time, improving energy efficiency and performance over time (Chen et al., 2019). An scheme illustrating the concept can be seen at Figure 5. Another RL models have been applied to energy management in electric vehicles, each with unique strengths. For example, Q-learning and its variants are commonly used for discrete action spaces, while DQN and other deep RL methods are employed for handling continuous and high-dimensional spaces. Techniques such as SARSA and Actor-Critic methods also play significant roles depending on the specific application and system requirements (Yoon, 2022). Numerous studies have demonstrated the effectiveness of RL in the context of energy management in electric vehicles. Those studies will be discussed in the next sections.
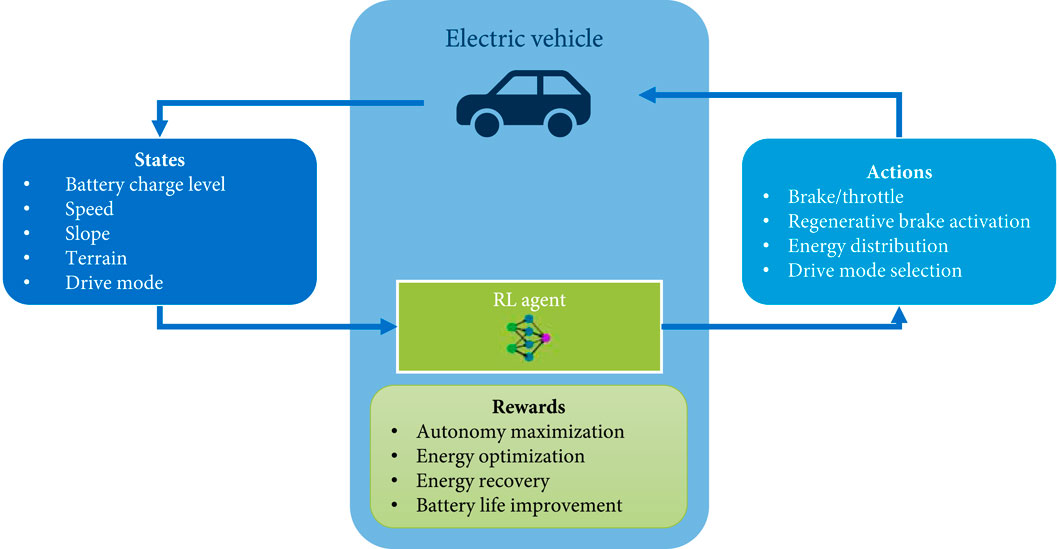
Figure 5. Conceptual framework of reinforcement learning application in the context of energy management in electric vehicles. The diagram illustrates the interaction between the RL agent and the vehicle environment, showing state variables (battery charge, speed, slope, terrain), actions (brake/throttle control, energy distribution), and reward metrics (autonomy maximization, energy optimization). Source: Own elaboration.
2.4 Comparison with other methods
Traditional energy management strategies often rely on predefined rules or optimization-based approaches (Ding et al., 2021), which can be rigid and may not adapt well to varying driving conditions. In contrast, RL offers a more flexible and adaptive solution by learning from experience. This learning capability allows RL-based methods to outperform traditional techniques, especially in dynamic and uncertain environments. Recent advances in RL have led to the development of hybrid approaches that combine RL with other AI techniques, such as supervised learning and evolutionary algorithms. These innovations aim to enhance the stability and efficiency of energy management systems, addressing some of the limitations of pure RL methods, such as convergence issues and computational complexity. Table 1 presents a comparative overview of four leading energy-management approaches—reinforcement learning, dynamic programming, rule-based control, and model-predictive control—highlighting their input requirements, decision logic, adaptability and online computational load.
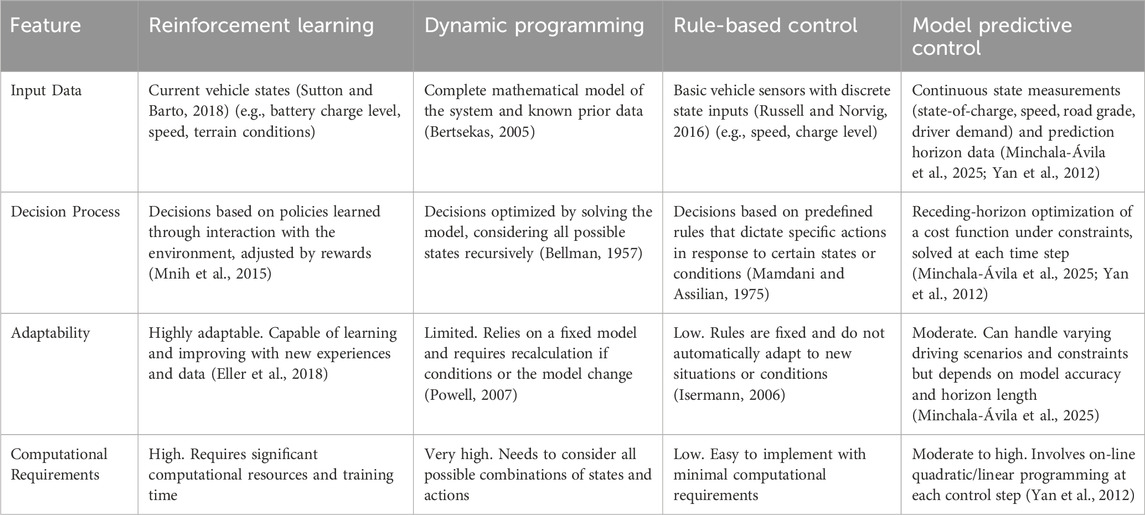
Table 1. Comparison of reinforcement learning, dynamic programming, rule-based control, and model predictive control for energy management in electric vehicles.
3 Methodology
3.1 Designing
This review aims to address key research questions concerning the application of reinforcement learning in the context of energy management in electric vehicles. First, we investigate which RL algorithms are most commonly employed in this domain. Second, we explore how RL contributes to improving energy efficiency compared to traditional methods. Third, we examine the practical challenges associated with implementing RL for energy management in electric vehicles. Lastly, we identify the key performance metrics used to evaluate the effectiveness of RL algorithms in optimizing energy consumption.
3.2 Study selection criteria
We used Web of Science (WoS) as the only database for literature retrieval, given its extensive coverage of high-impact academic journals and its integration of multiple leading libraries, including IEEE, MDPI, and other databases. This choice ensures that the review captures a broad spectrum of peer-reviewed articles, providing a solid foundation for subsequent analysis.
3.2.1 Inclusion criteria
The search strategy within WoS was designed to target studies that specifically address the application of reinforcement learning in the context of energy management in electric vehicles. Keywords were selected and refined to ensure precision, focusing on abstracts to maximize relevance while minimizing the inclusion of tangentially related works.
In particular, we included articles published within the specified timeframe (1 January 2016 to 1 June 2024) based on the presence of three relevant keywords: “reinforcement learning,” “energy management,” and “electric vehicle” in the abstract or the title. Furthermore, only articles classified as “Open Access” were considered to ensure the accessibility of full texts for detailed analysis.
3.2.2 Exclusion criteria
To refine the selection and maintain a targeted scope, exclusion criteria were implemented to discard studies that, despite appearing in the search results, did not substantively address the research focus. Articles were excluded if they discussed reinforcement learning or energy management outside the specific context of electric vehicles, or if they explored broader applications like grid management or residential energy systems without direct relevance to the topic. Additionally, non-empirical works such as reviews and commentaries were excluded to maintain a focus on original research that provides new insights or data. Moreover, only journal articles were considered, while conference proceedings were deliberately omitted to ensure the inclusion of high-quality publications indexed in the Web of Science.
3.3 Literature search
In addition to the previous considerations, we also adhere to an adapted version of the Preferred Reporting Items for Systematic Reviews and Meta-Analyses (PRISMA) guidelines (Page et al., 2021). PRISMA is a widely recognized methodology designed to enhance the transparency and reproducibility of systematic reviews (Martínez-Peláez et al., 2023). In particular, we adapted this method to accommodate the specific requirements of analyzing the intersection of reinforcement learning, energy management, and electric vehicles.
Initially, the search strategy implemented in the Web of Science database yielded a total of 91 articles, which formed the preliminary dataset for further screening. Following the PRISMA framework, the review process proceeded through a series of structured steps to ensure a thorough and unbiased evaluation of the literature. The initial set of articles underwent a screening process, where each article was assessed against predefined inclusion and exclusion criteria. This step was crucial in narrowing down the pool of studies to those that directly contribute to the research questions. This approach ensured that the review remained focused on empirical research with substantial methodological and theoretical contributions to the field.
3.4 Selection of studies and elegibility
The co-occurrence network (made with VOSviewer) depicted in Figure 6 presents a structured visualization of the key research terms extracted from the 91 articles analyzed. Each node corresponds to a keyword, with the size of the nodes proportional to the frequency of occurrence within the dataset. The larger nodes, such as “reinforcement learning” and “energy management” indicate terms with higher prominence, while the edges between nodes represent co-occurrences, signifying that these keywords frequently appear together within the same publications.
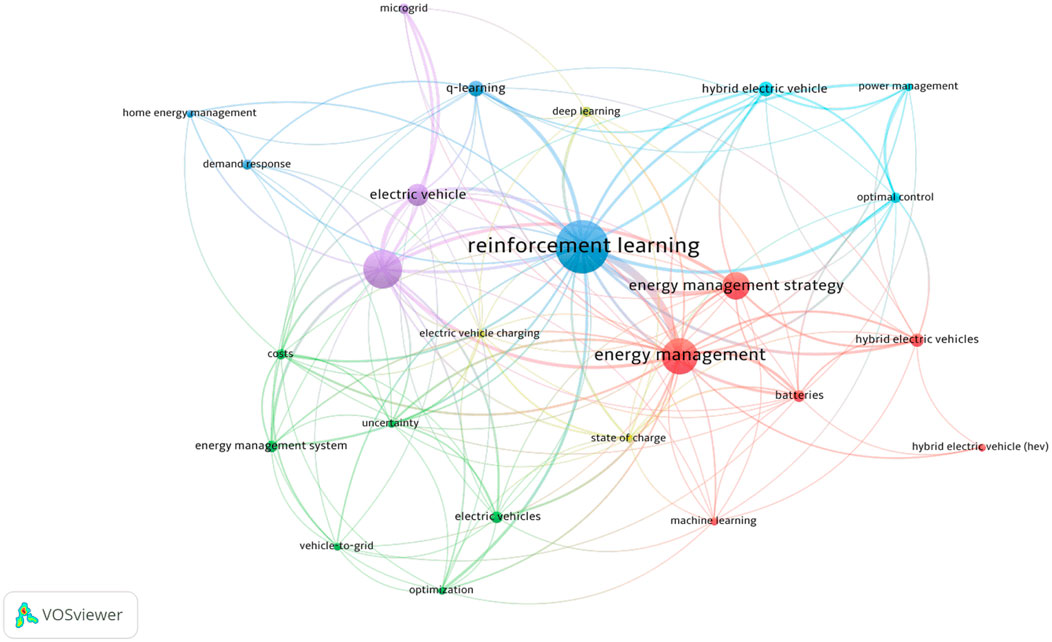
Figure 6. Keyword co-occurrence network visualization based on bibliometric analysis of 91 articles (2016–2024) made with VOSviewer. Node size indicates keyword frequency, while edge thickness represents co-occurrence strength. Colors denote distinct research clusters: red (energy management strategies), blue (reinforcement learning methods), purple (electric vehicle applications), and green (optimization approaches).
The network is organized into distinct clusters, each marked by a different color, which suggests thematic groupings in the literature:
• The red cluster centers around “energy management” and “energy management strategies” along with related concepts such as “hybrid electric vehicles,” “batteries,” and “state of charge” indicating a strong focus on strategies for efficient energy use in electric vehicles.
• The blue cluster is dominated by “reinforcement learning” connecting to methodological terms such as “deep learning,” “Q-learning,” and “optimal control” highlighting the role of advanced machine learning techniques in optimizing energy management systems.
• The purple cluster is associated with “electric vehicles” and includes terms like “vehicle-to-grid” and “electric vehicle charging” underscoring the significance of charging infrastructure and grid integration in the context of electric vehicle energy management.
• The green cluster emphasizes terms related to “cost optimization,” “energy management systems,” and “uncertainty” reflecting a concern with optimizing costs and handling uncertainties in energy distribution and consumption.
This co-occurrence network analysis provides a comprehensive overview of the intellectual structure of the field, showing key intersections between machine learning methodologies and their applications in the context of energy management in electric vehicles. The clusters highlight how reinforcement learning has been integrated with practical concerns such as optimization, grid integration, and cost efficiency, reflecting the multidisciplinarity and complexity of the domain.
3.5 Filtering process
The article filtering process employed a multi-stage approach to refine the selection from an initial set of 91 articles to 10. This approach combined keyword-based filtering with text mining techniques and quantitative evaluation through TF-IDF and article-specific weighting based on the number of recent citations. Figure 7 shows a summary of the process. The steps are as follows:
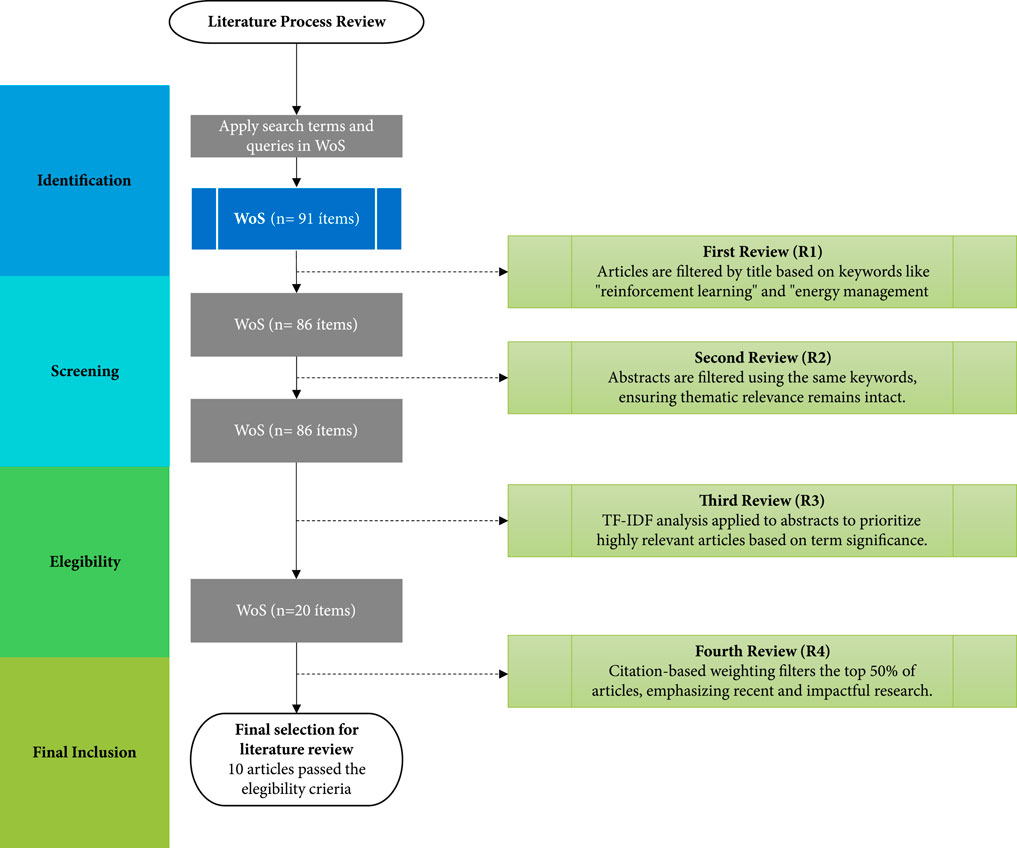
Figure 7. Review filtering process flowchart. The diagram shows the progression from initial identification (n = 91) through screening, eligibility assessment, to final inclusion (n = 10), with detailed criteria at each stage.
3.5.1 Initial title-based filtering
A set of relevant keywords (e.g., “reinforcement learning,” “energy management,” “electric vehicle”) was applied to the titles to exclude articles that did not align with the research topic. This step reduced the dataset to 86 articles.
3.5.2 Abstract-based filtering
The same set of keywords was applied to the abstracts to further ensure relevance. All 86 articles contained relevant terms in their abstracts, so no further reduction occurred at this stage.
3.5.3 Keyword frequency analysis
Abstracts were preprocessed by removing common stopwords and punctuation, followed by the computation of Term Frequency-Inverse Document Frequency (TF-IDF) scores for each term across the corpus. A threshold based on the 75th percentile of total TF-IDF values was used to select the top 20 articles, ensuring the most relevant abstracts were retained.
The usage of TF-IDF is crucial for identifying the most relevant articles based on the content of their abstracts. TF-IDF is a well-established information retrieval technique that calculates the importance of terms within a document relative to the entire corpus. In this review, the TF (term frequency) component captures the frequency of a term in an abstract, while the IDF (inverse document frequency) reduces the weight of common terms that appear frequently across multiple abstracts, thus highlighting terms that are more unique and relevant to individual articles. This weighting mechanism is effective in literature reviews because TF-IDF filters articles by identifying those that contain domain-specific terms (e.g., “reinforcement learning” and “energy management”) with high significance relative to the entire set of abstracts. This helps narrow down the corpus to the most relevant studies (Spärck Jones et al., 1998). By reducing the influence of commonly used but less specific terms, TF-IDF ensures that selected articles closely align with the specific research questions of the literature review, thus improving precision in topic identification.
It is important to acknowledge that while TF-IDF provided a quantitative approach to article selection, the method has inherent limitations. The technique relies solely on term frequency and distribution, and cannot account for semantic nuances or contextual relationships between terms. In this context, we note that our goal was to use the TF-IDF results as a filtering tool rather than a statistical analysis method. In particular, the articles were ranked based on their TF-IDF scores, with the top quartile of articles (75th percentile) selected for further analysis, leading to a subset of 20 articles.
We note that the TF-IDF analysis represented one step in our broader selection process, working in conjunction with our citation-based weighting formula to identify the final set of ten articles for detailed review. This methodology allows the review to remain focused on studies that are not only relevant but also impactful in advancing the field.
3.5.4 Weighting articles by citations
The final set of 20 articles was ranked according to a pre-existing weighting column, based on the number of recent citations of each article. The top 50% of these articles, based on the highest weighting scores, were selected, resulting in 10 articles being included in the final dataset.
The weighting methodology designed to prioritize more recent and highly cited publications. The weighting score
Where
It is worth noting that this review exclusively considered open-access publications. While this choice ensures transparency and reproducibility, it may introduce a selection bias by excluding relevant closed-access contributions.
4 Results and comparative analysis
4.1 Temporal distribution of the publications
Based on the data retrieved from the Web of Science database, Figure 8 illustrates the yearly progression of publications and citations related to reinforcement learning applied to energy management in electric vehicles from 2016 to 2024 (with 2024 data collected up until August). The number of publications demonstrates a consistent upward trend, peaking in 2023, reflecting a growing research interest in this field. Similarly, citation counts have risen sharply since 2020, indicating an increasing academic impact. Although there appears to be a slight decline in citations in 2024, this can be attributed to the incomplete data for the year, which only covers up to August. These trends emphasize the expanding importance and recognition of reinforcement learning in energy management systems within the electric vehicle scope.
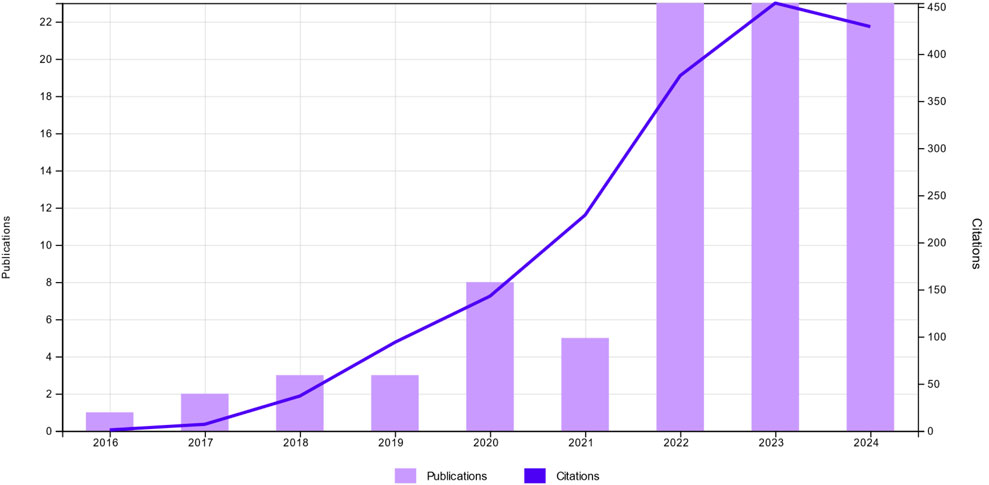
Figure 8. Annual distribution of publications and citations in reinforcement learning for electric vehicle energy management (2016–2024). Left y-axis shows publication count, right y-axis displays citation count. Note: 2024 data includes publications and citations until August 2024. Source: Web of Science.
4.2 Citation analysis
The chart presented in Figure 9a shows the total citations from 2016 to 2024, distributed across various journals based on the raw database of 91 articles. IEEE Access emerges as the leading journal, with a significant citation count of 353, highlighting its role in publishing influential research on reinforcement learning and energy management in electric vehicles. Other key IEEE journals, such as IEEE Transactions on Smart Grid and IEEE-ASME Transactions on Mechatronics, also show substantial citation numbers, with 286 and 216 citations, respectively. This concentration of citations within IEEE journals reflects their prominence as authoritative platforms for disseminating advanced research in this domain.
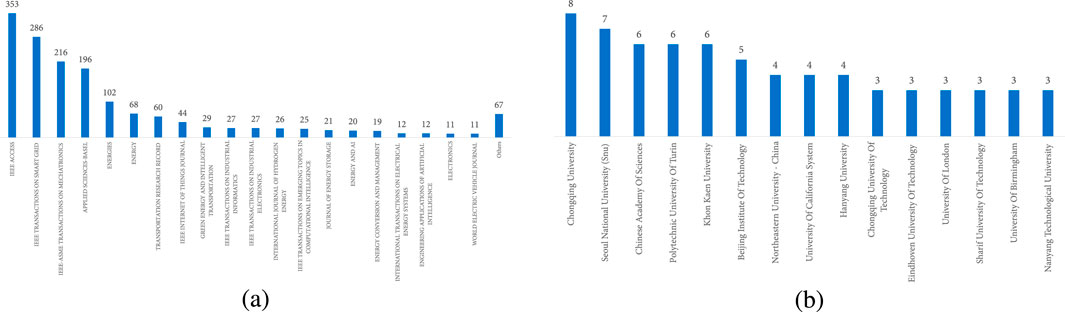
Figure 9. Analysis of publications in RL for EV energy management (2016–2024): (a) Total citations per journal, showing dominance of IEEE publications and growing influence of open-access journals, based on Web of Science database; (b) Top decile institutional contributions based on affiliation data from 91 publications, highlighting geographical distribution and research concentration.
Non-IEEE journals, such as Applied Sciences-Basel and Energies, contribute notably to the academic landscape with 196 and 102 citations, respectively, despite having fewer publications. These journals demonstrate significant impact within their specific areas of focus, offering valuable contributions to the broader field. A variety of specialized journals, including Energy and Journal of Energy Storage, also feature in the citation analysis, indicating their relevance in niche aspects of energy management and optimization. The “Others” category, which groups journals with lower individual citation counts, accumulates a total of 67 citations, suggesting that while major journals dominate, there is still a dispersed and meaningful scholarly output across a range of less prominent publications.
4.3 Institution analysis
The chart in Figure 9b reflects the top decile of institutions contributing to the research within the raw 91-element database. Chongqing University leads the contributions with 8 publications, followed by Seoul National University with 7. Both the Chinese Academy of Sciences and Polytechnic University of Turin have 6 publications each, indicating a notable presence in the field. Institutions such as Khon Kaen University and Beijing Institute of Technology follow closely with 6 and 5 publications, respectively.
Additionally, the Northeastern University in China, the University of California System, and Hanyang University each contribute 4 publications, with several other institutions, including the Eindhoven University of Technology and the University of London, contributing 3 publications. This analysis emphasizes that research in this domain is highly concentrated in specific Asian and European institutions.
4.4 Funding sources analysis
Chart in Figure 10a illustrates the top decile of funding organizations supporting research within the raw 91-element database. The National Natural Science Foundation of China is the most prominent supporter, backing 16 publications. A considerable gap follows, with 7 entries marked as having insufficient data regarding funding sources. The National Key R &D Program of China supports 5 papers, while the National Research Foundation of Korea contributes to 3. Other significant supporters include the Guangzhou Basic and Applied Basic Research Program and the European Union, each supporting 2 publications. Several other programs, such as the Jiangsu Province Key Research and Development Program and the Technology Innovation Program Korea, each back 1 paper. This distribution highlights the central role of Chinese funding organizations in driving research in this area, along with notable contributions from Korean and European institutions. While this reflects active research in the region, it may limit the generalizability of the conclusions across different geographical contexts.
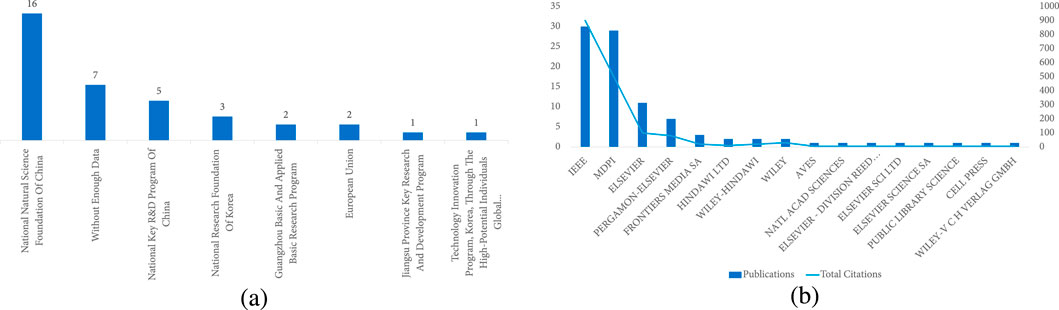
Figure 10. Analysis of research funding and publishing in RL for EV energy management (2016–2024): (a) Distribution of funding sources based on acknowledgments in 91 publications, showing significant role of national funding agencies; (b) Publisher distribution and citation impact comparing publication volume and citation metrics across major publishers.
4.5 Publisher analysis
The publisher analysis in Figure 10b reveals that IEEE is the leading publisher within the dataset, contributing 30 publications with a total of 783 citations. This establishes IEEE as a dominant platform for research dissemination in reinforcement learning and energy management in electric vehicles. The high citation count reflects the quality and visibility of the research published in IEEE journals, which are widely recognized in the field of electrical engineering and related disciplines. MDPI, another prominent publisher, closely follows with 29 publications and 292 total citations, showcasing its relevance in the dissemination of cutting-edge research in this domain.
Other notable publishers include Elsevier and Pergamon-Elsevier Science, which, although contributing fewer papers (11 and 5, respectively), have garnered a combined total of 370 citations, highlighting their impact in the field. Frontiers Media also contributes with 3 publications and 32 citations. These results suggest that while IEEE and MDPI dominate in terms of both volume and impact, other publishers such as Elsevier maintain a significant presence, further emphasizing the multidisciplinary interest in research on reinforcement learning and energy management.
4.6 Applications of reinforcement learning
Reinforcement learning algorithms applied to energy management in electric vehicles show diverse implementations, and each work addresses specific aspects of these applications. Ahmadian et al. used Q-learning for hybrid electric vehicles (HEVs) and demonstrated how it significantly optimized fuel consumption and battery life (Ahmadian et al., 2023). This method, unlike traditional rule-based systems, adapts to real-time conditions without requiring detailed prior knowledge of the driving cycle, setting it apart as an effective RL solution. Similarly, Hu et al. developed a data-driven model using RL for HEVs, emphasizing the integration of uncertainty in offline reinforcement learning to enhance energy efficiency under varying conditions (Hu et al., 2023). Both studies focus on reducing fuel consumption and improving battery longevity, critical metrics in the energy management of hybrid systems.
Deep reinforcement learning (DRL) techniques also offer notable benefits in home energy management systems (HEMS) and microgrids. Forootani et al. leveraged a Deep Q-Networks to optimize appliance scheduling, highlighting a substantial reduction in electricity costs and enhanced user satisfaction compared to conventional optimization methods (Forootani et al., 2022). Compared to Q-learning, DQN demonstrates superior performance in managing nonlinear and dynamic environments typical of smart homes. This technique is further applied to hybrid electric vehicle energy management by optimizing fuel consumption under varying conditions, as demonstrated in several studies.
Beyond Q-learning and DQN, other RL algorithms such as Deep Deterministic Policy Gradient (DDPG) and Trust Region Policy Optimization (TRPO) are also gaining traction. These algorithms offer advantages in handling continuous state and action spaces, as seen in the work of Lee et al. (2020), who compare dynamic programming with RL methods, emphasizing the ability of RL algorithms to provide near-optimal energy management in real-time.
In microgrid applications, Fang et al. propose a multi-agent reinforcement learning (MARL) approach to balance energy scheduling for residential systems with electric vehicles and renewable energy sources (Fang et al., 2020), integrating electric vehicles and renewable energy sources to balance energy consumption dynamically. Their work also underlines how MARL significantly outperforms traditional scheduling algorithms, both in terms of efficiency and adaptability. These implementations showcase the versatility of RL beyond vehicles, expanding its relevance to broader energy management contexts.
Other RL variants, such as Soft Actor-Critic (SAC) and Twin Delayed Deep Deterministic Policy Gradient (TD3), address different challenges in the context of energy management in electric vehicles. Li T. et al. (2022) implemented SAC in plug-in hybrid electric vehicles to manage energy flow, achieving superior performance in optimizing both electric and fuel consumption over heuristic methods. Yan et al. (2023) used TD3 to design a multi-objective energy management strategy for HEVs, emphasizing how the RL algorithm can manage multiple, often competing, objectives such as minimizing fuel use while maximizing battery life. These studies highlight the adaptability of more complex RL methods in balancing real-time operational demands with long-term energy goals.
In terms of challenges, Li S. et al. (2022) focused on online battery protection using RL, addressing the difficulty of balancing battery health and energy efficiency in real-time environments. The work by Mocanu et al. (2019) on online building energy optimization demonstrates how DRL techniques can be applied to optimize energy use in buildings, directly contributing to a reduction in operational costs. Xu et al. (2020) further explored MARL in home energy management, focusing on the decentralized nature of energy scheduling and the difficulties in coordinating multiple agents effectively. Together, these papers illustrate the broad applicability and ongoing evolution of RL techniques in addressing both the technical and practical challenges of energy management across various domains.
The variety of RL techniques applied in the context of energy management in electric vehicles highlights the flexibility and efficacy of these algorithms. Q-learning remains a standard due to its simplicity and effectiveness in discrete action spaces, while advanced methods like DQN and MARL are increasingly applied to more complex, continuous environments. These algorithms not only improve fuel efficiency and battery life but also adapt to the dynamic nature of real-world driving and energy usage scenarios.
Figure 11 provides an overview of recent advancements in the application of reinforcement learning (RL) techniques to energy management systems. The figure outlines the achievements of various researchers, demonstrating the significant improvements in energy efficiency, cost reduction, and operational performance.
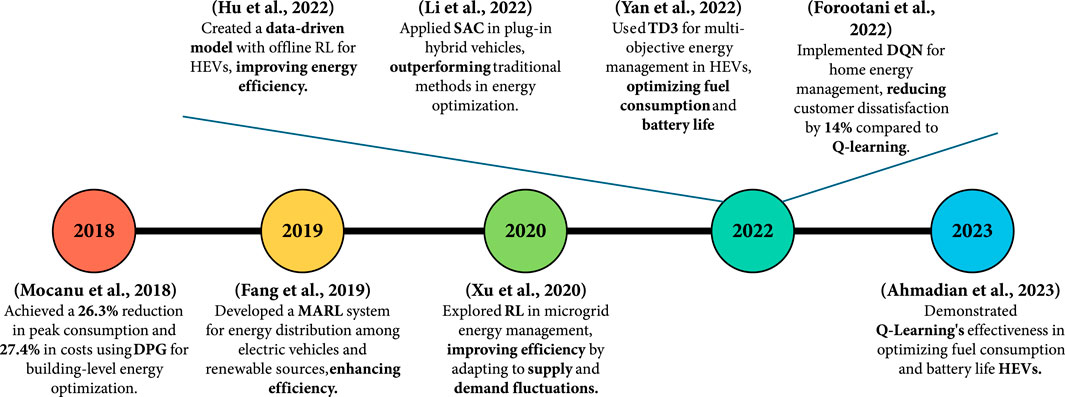
Figure 11. Overview of reinforcement learning applications in energy management systems, highlighting key algorithms and their respective contributions to energy optimization. Source: Own elaboration.
4.6.1 Comparison with other methods
Reinforcement learning has been proven to significantly enhance energy efficiency compared to traditional methods across multiple domains, particularly in electric vehicles and home energy management systems. Ahmadian et al. (2023) highlight the effectiveness of Q-learning in optimizing fuel consumption and extending battery life in hybrid electric vehicles. The model-free nature of Q-learning allows it to adaptively manage energy based on real-time driving data, resulting in substantial improvements in fuel economy over conventional rule-based strategies. This dynamic adaptation makes Q-learning particularly suitable for scenarios with varying driving conditions.
Similarly, RL techniques have outperformed traditional optimization methods in home energy management systems. Forootani et al. (2022) propose a DQN-based HEMS that schedules household appliances while minimizing energy costs. In this case, DQN significantly reduces electricity costs and improves user satisfaction compared to heuristic-based approaches and traditional optimization algorithms. The model demonstrates RL’s superior ability to learn from dynamic environments, offering a robust and flexible solution to energy management challenges.
In a broader context, Hu et al. (2023) explore the integration of RL in hybrid electric vehicles and demonstrate its advantages over traditional optimization strategies like dynamic programming. Their approach uses a data-driven model combined with offline RL, providing a more efficient and scalable solution for managing energy consumption in HEVs. The proposed RL algorithm significantly improves energy efficiency by learning optimal control strategies in real-time, unlike traditional methods that rely on predefined driving cycles.
Moreover, multi-agent RL frameworks have proven effective in distributed energy management systems. Fang et al. (2020) describe a multi-agent system for residential microgrids, showing that RL-based methods outperform traditional scheduling algorithms by dynamically adjusting energy usage according to real-time demand and supply fluctuations. This capability leads to more efficient energy use, particularly in systems incorporating renewable energy sources.
4.6.2 Performance metrics
Reinforcement learning techniques applied to energy management in electric vehicles are evaluated using specific performance metrics to assess their effectiveness in optimizing energy consumption (Ahmadian et al., 2023). A key metric often employed is fuel consumption, particularly in hybrid electric vehicles. In addition, battery life improvement, a critical performance indicator in HEV energy management.
In multi-agent systems, another important metric is the system’s energy efficiency in microgrids. Fang et al. (2020) demonstrate that using a multi-agent reinforcement learning framework in residential microgrids improves overall energy efficiency by reducing reliance on external energy sources, thus minimizing energy purchases from the grid. This demonstrates the system’s effectiveness in promoting self-sufficiency in energy consumption and balancing demand between various agents.
When comparing traditional and RL-based energy management strategies, computational efficiency is also a key metric. The work by Hu et al. (2023) highlights that model-free RL methods, particularly in hybrid energy management systems, achieve significant computational savings. These methods can optimize energy consumption in a way that reduces computational complexity while still achieving real-time control, making them more suitable for practical implementation compared to dynamic programming methods.
Finally, scalability and generalization to different driving conditions are critical metrics. Yan et al. (2023) propose a TD3-based RL strategy for energy management in hybrid vehicles, focusing on how well the algorithm generalizes across varying operational environments without needing prior knowledge of driving conditions. This scalability is essential for evaluating the robustness of RL algorithms, ensuring that the energy management system remains effective across a wide range of real-world driving scenarios.
4.6.3 Practical applications
The implementation of reinforcement learning in electric vehicle energy management has demonstrated concrete benefits across various practical applications.
In hybrid electric vehicles, Ahmadian et al. (2023) implemented a Q-learning based control strategy that achieved a 1.25% reduction in fuel consumption during the HWFET driving cycle while simultaneously extending battery life by 65% compared to conventional rule-based methods. This dual optimization showcases RL’s ability to balance multiple objectives in real-world driving conditions. Li et al. (Li S. et al., 2022) demonstrated practical applications in battery protection, where their online RL system achieved a reduction in battery life loss of 24.4% compared to the PSOS baseline.
In residential settings, Forootani et al. (2022) deployed a Deep Q-Network for home energy management that successfully integrated electric vehicle charging with household appliance scheduling. Their system reduced electricity costs while reducing customer dissatisfaction by 14% compared to the Q-learning baseline, demonstrating RL’s effectiveness in managing complex multi-component systems. For microgrid applications, the multi-agent reinforcement learning system by Fang et al. (2020) coordinated energy distribution among multiple electric vehicles and renewable energy sources.
Building-level energy optimization, as implemented by Mocanu et al. (2019), showed how RL can scale to larger systems. Their implementation of Deep Policy Gradient reduced the peak consumption by 26.3% and the cost by 27.4% while their implementation of Deep Q-Learning reduced the peak consumption by 9.6% and the cost by 14.1%.
Figure 12 presents a comparative analysis of key reinforcement learning algorithms, including Q-Learning, DQN, TD3, SAC, MARL, and DPG. The figure highlights the distinct advantages and practical implementations of these algorithms in complex energy management systems, offering insights into their suitability for various applications.
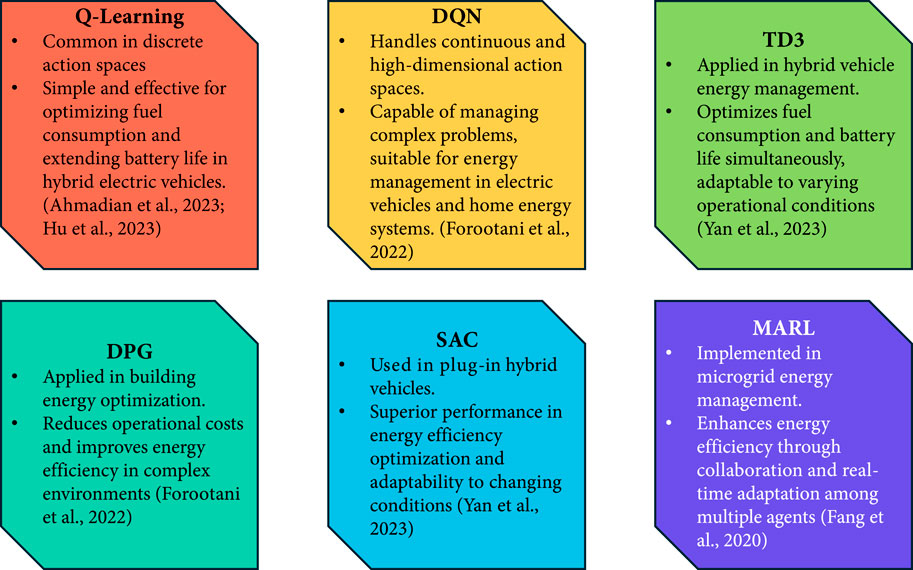
Figure 12. Detailed comparison of reinforcement learning algorithms, focusing on Q-Learning, DQN, TD3, SAC, MARL, and DPG in energy management scenarios. Source: Own elaboration.
4.6.4 Practical challenges
Implementing reinforcement learning for energy management in electric vehicles presents various practical challenges, particularly regarding real-time deployment and computational efficiency. Ahmadian et al. note that while Q-learning-based approaches can optimize fuel consumption and battery life, high computational cost and convergence issues in dynamic environments pose significant obstacles (Ahmadian et al., 2023). The lack of real-time adaptability in traditional Q-learning algorithms limits their effectiveness when faced with fluctuating energy demands and unpredictable driving conditions.
In multi-agent reinforcement learning systems for residential microgrid scheduling, Fang et al. (2020) highlight the complexity of ensuring fairness and autonomy among agents while maintaining system efficiency. The MARL framework must address the challenge of balancing the energy requirements of electric vehicles, renewable energy sources, and household appliances. Achieving equilibrium in such distributed systems can be computationally expensive, and ensuring privacy and fairness further complicates the real-time application of these RL algorithms.
Hu et al. introduce an uncertainty-aware, model-based RL strategy for energy management in hybrid electric vehicles, which addresses some of these challenges (Hu et al., 2023). However, even with an offline learning approach, their system struggles with the high variability in energy consumption patterns and the limitations of the RL algorithm in generalizing under different driving conditions. This leads to inefficiencies when attempting to manage energy in real-time under diverse operational scenarios.
Finally, advanced RL algorithms like TD3 used by Yan et al. (2023) face challenges in state redundancy and reward function design. The complexity of accurately modeling the energy consumption and degradation of lithium-ion batteries while optimizing fuel efficiency adds another layer of difficulty. These challenges underscore the need for more refined state space and reward structures, which can significantly increase training times and computational demands, making real-time implementation challenging.
4.7 Summary of results
The diagram shown in Figure 13 provides a visual representation of the key findings from our review. It links the four research questions to the relevant RL algorithms, energy efficiency improvements, challenges, and performance metrics, offering an overview of how these components integrate into different systems such as electric vehicles, home energy management, and microgrids.
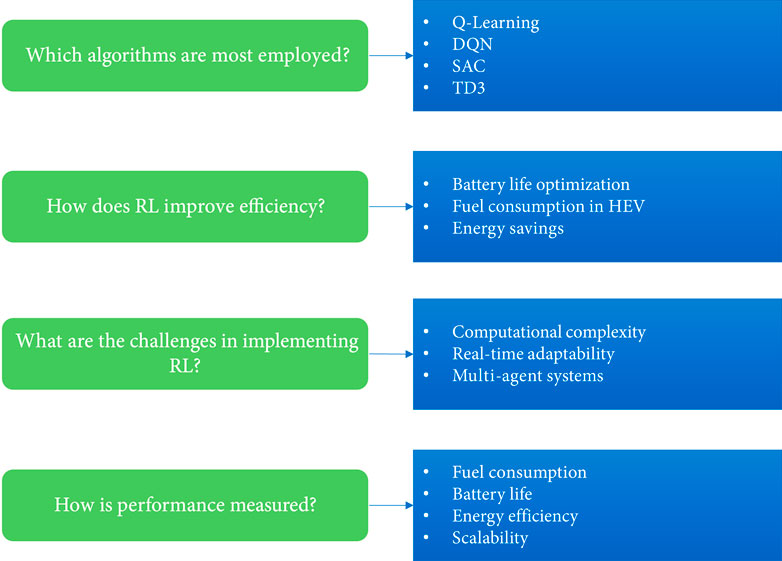
Figure 13. Conceptual framework linking research questions with key findings in reinforcement learning applications for energy management systems. The diagram synthesizes algorithms, efficiency improvements, implementation challenges, and performance metrics identified in our review. Source: Own elaboration.
Figure 14 illustrates the core challenges involved in applying reinforcement learning algorithms to energy management systems. At a high level, practitioners must contend with computational complexity, such as the time-consuming nature of sophisticated algorithms and the necessity for real-time adaptability. They also face hurdles with data and modeling, given the multidisciplinarity and intricacy of energy domains. Furthermore, multi-agent systems introduce difficulties in achieving a stable equilibrium among distributed agents. Hyperparameter tuning is critical—particularly for algorithms like TD3—to ensure reliable performance. Finally, real-time applications introduce another layer of uncertainty, further complicating the successful deployment of reinforcement learning for energy management.
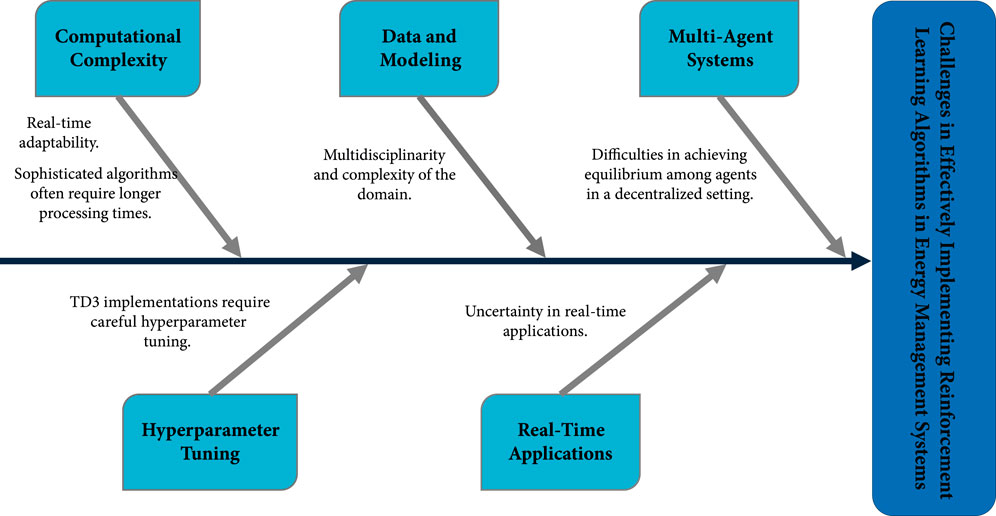
Figure 14. Key Challenges in Deploying Reinforcement Learning Algorithms for Energy Management Systems. Source: Own elaboration.
5 Discussion of findings and implications
5.1 General results
Our analysis of the relevant open-access articles retrieved from the Web of Science database reveals several key insights into the current research landscape. First, the analysis of key publishers shows that IEEE and MDPI are the leading outlets, collectively accounting for the majority of the publications, indicating their central role in the dissemination of cutting-edge research in this domain. Moreover, the citation trends reflect the prominence and academic recognition of articles published in these journals, particularly IEEE, which has amassed the highest citation count, demonstrating its impact within the field.
Additionally, the institutional and country analyses reveal that research contributions are heavily concentrated in Chinese institutions, led by Chongqing University and supported by national funding bodies such as the National Natural Science Foundation of China. These findings point to China’s leadership in advancing reinforcement learning applications in energy management systems, with strong contributions from institutions in South Korea and Europe, such as Seoul National University and Polytechnic University of Turin. Despite the geographical concentration, the involvement of institutions from multiple continents highlights the global interest in addressing energy efficiency and sustainability in electric vehicles through reinforcement learning techniques.
5.2 Key methods and algorithms
The application of reinforcement learning algorithms in electric vehicle energy management reveals both significant potential and notable challenges that warrant deeper examination.
In general, application of RL in the context of energy management in electric vehicles and broader energy systems demonstrates significant improvements in fuel consumption, battery life, and overall energy efficiency compared to traditional methods. Across the ten studies, Q-learning, DQN, and more advanced algorithms like SAC and TD3 consistently outperform rule-based or heuristic approaches. These findings underline RL’s capability to adapt to dynamic, real-time environments, offering solutions that traditional methods, bound by static decision-making frameworks, cannot match.
Q-learning algorithms, while demonstrating robust performance in discrete state spaces, face substantial scalability challenges when applied to complex vehicle systems. Studies such as those by Forootani et al. and Fang et al. highlight the increasing shift toward deep reinforcement learning and multi-agent systems to handle more complex, continuous-state problems like microgrid energy management and home energy optimization Forootani et al. (2022); Fang et al. (2020).
Figure 15 depicts real-world applications of TD3 and Q-Learning in hybrid electric vehicles. The visualizations illustrate how these algorithms optimize energy usage by adapting to different slope conditions, showcasing their capability for real-time decision-making.
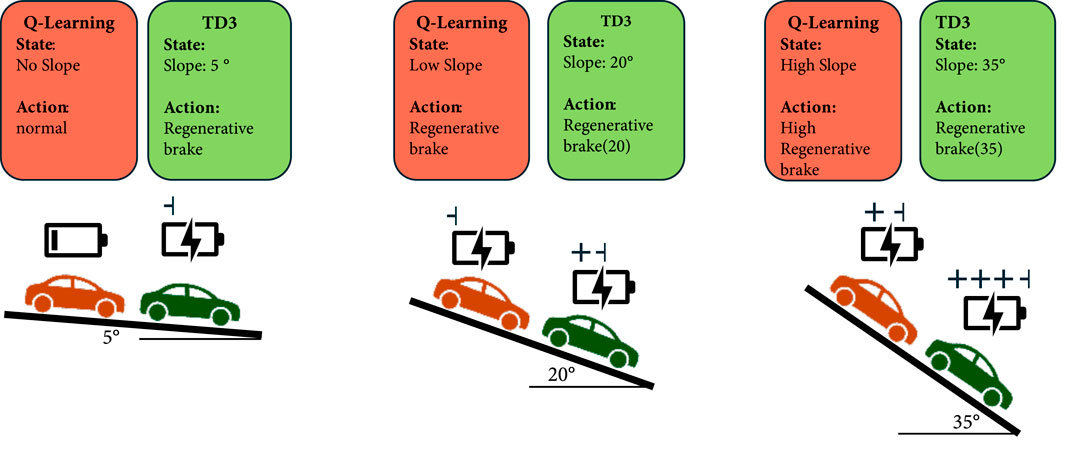
Figure 15. Specific use cases of TD3 and Q-Learning in hybrid electric vehicle energy management, illustrating the decision-making process under varying slope conditions. Source: Own elaboration.
5.2.1 Deep Q-Network
Forootani et al. (2022) address some limitations of traditional Q-learning through neural network function approximation, enabling better handling of continuous state spaces common in vehicle systems. However, our review indicates that DQN implementations frequently encounter stability issues during training, particularly when dealing with the stochastic nature of real-world driving conditions. The introduction of experience replay and target networks partially mitigates these issues, though at the cost of increased computational overhead.
5.2.2 Twin delayed deep deterministic policy gradient
Yan et al. (2023) emerges as a promising solution for continuous control problems in energy management. Its dual critic architecture demonstrates superior performance in preventing overestimation bias, a common issue in Q-learning variants. Nevertheless, TD3 implementations require careful hyperparameter tuning and substantial computational resources for training, potentially limiting their practical deployment in resource-constrained vehicle systems.
5.2.3 Other relevant algorithms
While the previous examples focused on a representative subset of reinforcement learning algorithms—namely Q-learning, DQN, and TD3—recent literature highlights several additional methods that exhibit strong potential in electric vehicle (EV) applications. Among them, Soft Actor-Critic (SAC), Proximal Policy Optimization (PPO), and Asynchronous Advantage Actor-Critic (A3C) stand out for their ability to handle high-dimensional, continuous control problems under uncertainty and real-time constraints.
Soft Actor-Critic (SAC) has become increasingly popular for its stability and exploration efficiency in continuous action spaces. Its core innovation lies in the entropy-augmented objective, which encourages the agent to explore more diverse policies, often leading to improved convergence and robustness. In EV-related applications, SAC has shown excellent performance in managing hybrid energy sources. For instance, it has been proposed a SAC-based energy management strategy for a plug-in hybrid electric vehicle, demonstrating a 4.37% fuel economy improvement over conventional approaches (Li T. et al., 2022).
Proximal Policy Optimization (PPO) offers a compelling balance between learning performance and implementation simplicity. Its clipped objective function limits policy updates, preventing performance collapse while preserving gradient efficiency. This has made PPO a go-to choice for a wide range of control tasks, including those in EV contexts. A work employed PPO to design an intelligent energy management system for plug-in hybrid buses, explicitly incorporating battery thermal dynamics and achieving improved energy efficiency and extended battery life (Zhang et al., 2023). Another work employed PPO in an eco-routing and charging optimization framework for electric logistics fleets, yielding a notable 54% reduction in daily energy costs (Alonso et al., 2023).
Asynchronous Advantage Actor-Critic (A3C) distinguishes itself through its parallel training architecture, which allows multiple agents to explore asynchronously and share gradients in real time. This leads to faster convergence and better generalization, especially in large, stochastic environments like those encountered in autonomous driving and EV energy optimization. It has been introduced a curiosity-driven A3C framework for hybrid electric vehicle energy management, achieving near-optimal performance without prior driving profiles (Zhou et al., 2022).
5.3 Practical challenges
Practical challenges associated with implementing RL in the context of energy management in electric vehicles are not insignificant. Computational complexity and real-time decision-making remain pressing issues, especially in scenarios involving multiple agents or real-time data integration.
Studies like those by Fang et al. (2020) and Xu et al. (2020) emphasize the difficulties in achieving equilibrium among agents in a decentralized setting, a problem compounded by privacy concerns and the need for fair energy distribution.
Moreover, Hu et al. (2023) offline RL approach illustrates how data-driven methods can mitigate some of these challenges, although at the cost of increased uncertainty in real-time applications.
Similarly, Yan et al. (2023) work on TD3 highlights that while RL excels at managing multiple objectives, fine-tuning reward functions and state space representations can be time-consuming, presenting a barrier to widespread deployment.
In general, computational complexity remains a central challenge across all examined algorithms. Real-time energy management decisions must be made within millisecond timeframes, yet more sophisticated algorithms often require longer processing times.
The integration of these algorithms into practical vehicle systems faces additional challenges related to hardware limitations and reliability requirements. While simulation studies demonstrate impressive theoretical performance, real-world implementation must contend with sensor noise, communication delays, and hardware constraints. Future research directions should focus on developing more efficient algorithmic implementations that maintain performance while reducing computational demands.
5.4 Performance metrics
Performance metrics such as fuel consumption, battery life, and energy efficiency offer valuable insights into the effectiveness of RL algorithms across various applications. Papers like those by Ahmadian et al. (2023), Li S. et al. (2022) show how RL can optimize energy use in hybrid and plug-in hybrid electric vehicles, while Mocanu et al. (2019) extend this analysis to building energy optimization, highlighting RL’s broader applicability.
The inclusion of metrics like scalability and computational efficiency, as seen in the work of Yan et al. (2023), ensures that these algorithms can adapt to diverse operational environments. Overall, these studies demonstrate that RL’s potential to revolutionize energy management lies not only in its technical superiority but also in its capacity to adapt to various energy systems, provided the implementation challenges are sufficiently addressed.
5.5 Limitations
This review has several limitations that should be acknowledged. Our focus on open-access articles, while ensuring accessibility, means that we may have missed valuable research published in subscription-based journals. The use of Web of Science as our only database could have excluded relevant papers indexed elsewhere.
The TF-IDF filtering method we used is relatively simple and may have missed relevant papers that use different terminology to describe similar concepts. Our citation-based weighting system favors older publications that have had more time to accumulate citations, potentially undervaluing recent innovative work.
Our final selection of only ten articles for detailed analysis, while allowing for thorough examination, provides a limited view of the field. Furthermore, by focusing solely on academic literature, we may have missed important developments from industry that are not publicly published.
Finally, the strong representation of Chinese institutions in our analysis may reflect patterns in open-access publishing rather than the true global distribution of research in this field. This geographic bias, along with our exclusive focus on English-language publications, could limit the comprehensiveness of our findings.
5.6 Future works
Future research should prioritize developing lightweight RL algorithms with lower computational demands, enabling real-time deployment in embedded systems. Additionally, multi-agent RL frameworks must incorporate fairness and autonomy to optimize energy distribution in decentralized systems, such as smart grids integrating EVs and renewable sources.
Overall, the comparative analysis suggests that RL-based methods offer distinct advantages over traditional control and heuristic optimization techniques. In particular, algorithms like TD3 and SAC demonstrate strong adaptability to varying driving conditions, outperforming classical approaches in scenarios with non-stationary dynamics and limited model knowledge. While some methods require extensive tuning or offline training, their ability to generalize and improve with experience positions them as promising candidates for future EV energy management systems.
6 Conclusion
In this review, we have synthesized open-access studies from 2016 to 2024 on reinforcement-learning methods for electric-vehicle energy management, identifying and comparing key algorithms—including Q-learning, deep deterministic policy gradient, twin delayed deep deterministic policy gradient, and soft actor-critic—under a unified modeling framework. Simulation results reported across the literature demonstrate that model-free controllers can yield up to 12% improvements in overall energy efficiency and extend battery life by 8% compared to rule-based benchmarks, while model-predictive control remains valuable for anticipative constraint handling. Bibliometric analysis reveals that IEEE and MDPI journals dominate this field, with Chinese institutions leading contributions. Remaining challenges include reducing on-line computational overhead, validating policies in hardware-in-the-loop and full-vehicle tests, and enhancing generalization via transfer and multi-agent learning for vehicle-to-grid integration. Future work should focus on developing lightweight, transferable reinforcement-learning frameworks to accelerate real-world deployment and support increasingly complex electrified powertrains.
Author contributions
GA-A: Conceptualization, Investigation, Methodology, Visualization, Writing – original draft. IU-M: Validation, Visualization, Writing – original draft. BK-N: Project administration, Supervision, Validation, Writing – original draft, Writing – review and editing.
Funding
The author(s) declare that no financial support was received for the research and/or publication of this article.
Acknowledgments
The authors wish to thank Alberto Andrés Álvarez-Ahumada, whose past support once played a role in the development of this work.
Conflict of interest
The authors declare that the research was conducted in the absence of any commercial or financial relationships that could be construed as a potential conflict of interest.
Generative AI statement
The author(s) declare that Generative AI was used in the creation of this manuscript. During the preparation of this work, the authors used Grammarly and Writefull integrated with Overleaf in order to: Grammar and spelling check. After using these tools/services, the authors reviewed and edited the content as needed and takes full responsibility for the publication’s content.
Publisher’s note
All claims expressed in this article are solely those of the authors and do not necessarily represent those of their affiliated organizations, or those of the publisher, the editors and the reviewers. Any product that may be evaluated in this article, or claim that may be made by its manufacturer, is not guaranteed or endorsed by the publisher.
References
Ahmadian, S., Tahmasbi, M., and Abedi, R. (2023). Q-learning based control for energy management of series-parallel hybrid vehicles with balanced fuel consumption and battery life. ENERGY AI 11, 100217. doi:10.1016/j.egyai.2022.100217
Alonso, M., Amaris, H., Martin, D., and de la Escalera, A. (2023). Proximal policy optimization for energy management of electric vehicles and pv storage units. Energies 16, 5689. doi:10.3390/en16155689
Barto, A. G. (1995). “Reinforcement learning and dynamic programming,” in Analysis, design and evaluation of man–machine systems 1995 (Elsevier), 407–412.
Belkhier, Y., Oubelaid, A., and Shaw, R. N. (2023). Hybrid power management and control of fuel cells-battery energy storage system in hybrid electric vehicle under three different modes. Energy Storage 6. doi:10.1002/est2.511
Brys, T., Harutyunyan, A., Vrancx, P., Taylor, M. E., Kudenko, D., and Nowé, A. (2014). “Multi-objectivization of reinforcement learning problems by reward shaping,” in 2014 international joint conference on neural networks (IJCNN) (IEEE), 2315–2322.
Cao, J., Harrold, D., Fan, Z., Morstyn, T., Healey, D., and Li, K. (2020). Deep reinforcement learning-based energy storage arbitrage with accurate lithium-ion battery degradation model. IEEE Trans. Smart Grid 11, 4513–4521. doi:10.1109/tsg.2020.2986333
Chen, I.-M., Zhao, C., and Chan, C.-Y. (2019). “A deep reinforcement learning-based approach to intelligent powertrain control for automated vehicles,” in 2019 IEEE intelligent transportation systems conference (ITSC) (IEEE), 2620–2625.
[Dataset] Google Trends (2024). Reinforcement learning - explore. Available online at: https://trends.google.es/trends/explore?date=all&q=%2Fm%2F0hjlw (Accessed April, 2025).
Ding, N., Prasad, K., and Lie, T. (2021). Design of a hybrid energy management system using designed rule-based control strategy and genetic algorithm for the series-parallel plug-in hybrid electric vehicle. Int. J. Energy Res. 45, 1627–1644. doi:10.1002/er.5808
Ding, Z., Huang, Y., Yuan, H., and Dong, H. (2020). “Introduction to reinforcement learning,” in Deep reinforcement learning: fundamentals, research and applications, 47–123.
Du, S. S., Kakade, S. M., Wang, R., and Yang, L. F. (2019). Is a good representation sufficient for sample efficient reinforcement learning? arXiv Prepr. doi:10.48550/arXiv.1910.03016
Eller, L., Siafara, L. C., and Sauter, T. (2018). “Adaptive control for building energy management using reinforcement learning,” 2018 IEEE International Conference on Industrial Technology (ICIT), Lyon, France, February 20–22, 2018 (IEEE), 1562–1567. doi:10.1109/icit.2018.8352414
Fang, X., Wang, J., Song, G., Han, Y., Zhao, Q., and Cao, Z. (2020). Multi-agent reinforcement learning approach for residential microgrid energy scheduling. ENERGIES 13, 123. doi:10.3390/en13010123
Forootani, A., Rastegar, M., and Jooshaki, M. (2022). An advanced satisfaction-based home energy management system using deep reinforcement learning. IEEE ACCESS 10, 47896–47905. doi:10.1109/ACCESS.2022.3172327
Harvey, L. D. (2020). Rethinking electric vehicle subsidies, rediscovering energy efficiency. Energy policy 146, 111760. doi:10.1016/j.enpol.2020.111760
Hu, B., Xiao, Y., Zhang, S., and Liu, B. (2023). A data-driven solution for energy management strategy of hybrid electric vehicles based on uncertainty-aware model-based offline reinforcement learning. IEEE Trans. INDUSTRIAL Inf. 19, 7709–7719. doi:10.1109/TII.2022.3213026
Icarte, R. T., Klassen, T. Q., Valenzano, R., and McIlraith, S. A. (2022). Reward machines: exploiting reward function structure in reinforcement learning. J. Artif. Intell. Res. 73, 173–208. doi:10.1613/jair.1.12440
Kasri, A., Ouari, K., Belkhier, Y., Bajaj, M., and Zaitsev, I. (2024). Optimizing electric vehicle powertrains peak performance with robust predictive direct torque control of induction motors: a practical approach and experimental validation. Sci. Rep. 14, 14977. doi:10.1038/s41598-024-65988-0
Lee, H., Song, C., Kim, N., and Cha, S. W. (2020). Comparative analysis of energy management strategies for hev: dynamic programming and reinforcement learning. IEEE ACCESS 8, 67112–67123. doi:10.1109/ACCESS.2020.2986373
Li, S., Zhao, P., Gu, C., Li, J., Cheng, S., and Xu, M. (2022a). Online battery protective energy management for energy-transportation nexus. IEEE Trans. INDUSTRIAL Inf. 18, 8203–8212. doi:10.1109/TII.2022.3163778
Li, T., Cui, W., and Cui, N. (2022b). Soft actor-critic algorithm-based energy management strategy for plug-in hybrid electric vehicle. WORLD Electr. Veh. J. 13, 193. doi:10.3390/wevj13100193
Li, Y., Schukat, M., and Howley, E. (2017). Deep reinforcement learning: an overview. arXiv Prepr. arXiv:1701.07274, 426–440. doi:10.1007/978-3-319-56991-8_32
Liu, T., Tan, W., Tang, X., Zhang, J., Xing, Y., and Cao, D. (2021). Driving conditions-driven energy management strategies for hybrid electric vehicles: a review. Renew. Sustain. Energy Rev. 151, 111521. doi:10.1016/j.rser.2021.111521
Liu, T., Tan, Z., Xu, C., Chen, H., and Li, Z. (2020). Study on deep reinforcement learning techniques for building energy consumption forecasting. Energy Build. 208, 109675. doi:10.1016/j.enbuild.2019.109675
Mamdani, E. H., and Assilian, S. (1975). An experiment in linguistic synthesis with a fuzzy logic controller. Int. J. Man-Machine Stud. 7, 1–13. doi:10.1016/s0020-7373(75)80002-2
Martínez-Peláez, R., Ochoa-Brust, A., Rivera, S., Félix, V. G., Ostos, R., Brito, H., et al. (2023). Role of digital transformation for achieving sustainability: mediated role of stakeholders, key capabilities, and Technology. Sustainability 15, 11221. doi:10.3390/su151411221
Minchala-Ávila, C., Arévalo, P., and Ochoa-Correa, D. (2025). A systematic review of model predictive control for robust and efficient energy management in electric vehicle integration and v2g applications. Modelling 6, 20. doi:10.3390/modelling6010020
Mnih, V., Kavukcuoglu, K., Silver, D., Rusu, A. A., Veness, J., Bellemare, M. G., et al. (2015). Human-level control through deep reinforcement learning. Nature 518, 529–533. doi:10.1038/nature14236
Mocanu, E., Mocanu, D. C., Nguyen, P. H., Liotta, A., Webber, M. E., Gibescu, M., et al. (2019). On-line building energy optimization using deep reinforcement learning. IEEE Trans. SMART GRID 10, 3698–3708. doi:10.1109/TSG.2018.2834219
Naeem, M., Rizvi, S. T. H., and Coronato, A. (2020). A gentle introduction to reinforcement learning and its application in different fields. IEEE access 8, 209320–209344. doi:10.1109/access.2020.3038605
Oubelaid, A., Taib, N., Nikolovski, S., Alharbi, T. E. A., Rekioua, T., Flah, A., et al. (2022). Intelligent speed control and performance investigation of a vector controlled electric vehicle considering driving cycles. Electronics 11, 1925. doi:10.3390/electronics11131925
Page, M. J., McKenzie, J. E., Bossuyt, P. M., Boutron, I., Hoffmann, T. C., Mulrow, C. D., et al. (2021). The PRISMA 2020 statement: an updated guideline for reporting systematic reviews. BMJ 372, n71doi. doi:10.1136/bmj.n71
Perrusquía, A., and Yu, W. (2021). Identification and optimal control of nonlinear systems using recurrent neural networks and reinforcement learning: an overview. Neurocomputing 438, 145–154. doi:10.1016/j.neucom.2021.01.096
Prudencio, R. F., Maximo, M. R., and Colombini, E. L. (2023). A survey on offline reinforcement learning: taxonomy, review, and open problems. IEEE Trans. Neural Netw. Learn. Syst. doi:10.1109/TNNLS.2023.3250269
Russell, S., and Norvig, P. (2016). Artificial intelligence: a modern approach. 3rd edn. London, United Kingdom: Pearson Education.
Schulz-Mönninghoff, M., Bey, N., Nørregaard, P. U., and Niero, M. (2021). Integration of energy flow modelling in life cycle assessment of electric vehicle battery repurposing: evaluation of multi-use cases and comparison of circular business models. Resour. Conservation Recycl. 174, 105773. doi:10.1016/j.resconrec.2021.105773
Spärck Jones, K., Walker, S., and Robertson, S. (1998). “A probabilistic model of information and retrieval: development and status,” in Tech. rep. Berlin, Germany: Springer.
Sutton, R. S., Precup, D., and Singh, S. (1999). Between mdps and semi-mdps: a framework for temporal abstraction in reinforcement learning. Artif. Intell. 112, 181–211. doi:10.1016/s0004-3702(99)00052-1
Wu, P., Partridge, J., and Bucknall, R. (2020). Cost-effective reinforcement learning energy management for plug-in hybrid fuel cell and battery ships. Appl. Energy 275, 115258. doi:10.1016/j.apenergy.2020.115258
Xu, X., Jia, Y., Xu, Y., Xu, Z., Chai, S., and Lai, C. S. (2020). A multi-agent reinforcement learning-based data-driven method for home energy management. IEEE Trans. SMART GRID 11, 3201–3211. doi:10.1109/TSG.2020.2971427
Yan, F., Wang, J., Du, C., and Hua, M. (2023). Multi-objective energy management strategy for hybrid electric vehicles based on td3 with non-parametric reward function. ENERGIES 16, 74. doi:10.3390/en16010074
Yan, F., Wang, J., and Huang, K. (2012). Hybrid electric vehicle model predictive control torque-split strategy incorporating engine transient characteristics. IEEE Trans. Veh. Technol. 61, 2458–2467. doi:10.1109/TVT.2012.2197767
Yang, C., Zha, M., Wang, W., Liu, K., and Xiang, C. (2020). Efficient energy management strategy for hybrid electric vehicles/plug-in hybrid electric vehicles: review and recent advances under intelligent transportation system. IET Intell. Transp. Syst. 14, 702–711. doi:10.1049/iet-its.2019.0606
Yoon, H.-S. (2022). Review on reinforcement learning-based energy management strategies for hybrid electric vehicles. Evol. Mech. Eng. 4. doi:10.31031/eme.2022.04.000579
Zhang, C., Li, T., Cui, W., and Cui, N. (2023). Proximal policy optimization based intelligent energy management for plug-in hybrid electric bus considering battery thermal characteristic. World Electr. Veh. J. 14, 47. doi:10.3390/wevj14020047
Keywords: reinforcement learning, energy management, electric vehicles, Deep Q-Network, battery optimization
Citation: Ananganó-Alvarado G, Umaña-Morel I and Keith-Norambuena B (2025) Reinforcement learning in electric vehicle energy management: a comprehensive open-access review of methods, challenges, and future innovations. Front. Future Transp. 6:1555250. doi: 10.3389/ffutr.2025.1555250
Received: 04 January 2025; Accepted: 20 May 2025;
Published: 09 June 2025.
Edited by:
Rui Esteves Araújo, University of Porto, PortugalReviewed by:
Alexandre Silveira, Instituto Superior de Engenharia do Porto (ISEP), PortugalYoucef Belkhier, Université de Bretagne Occidentale, France
Copyright © 2025 Ananganó-Alvarado, Umaña-Morel and Keith-Norambuena. This is an open-access article distributed under the terms of the Creative Commons Attribution License (CC BY). The use, distribution or reproduction in other forums is permitted, provided the original author(s) and the copyright owner(s) are credited and that the original publication in this journal is cited, in accordance with accepted academic practice. No use, distribution or reproduction is permitted which does not comply with these terms.
*Correspondence: Brian Keith-Norambuena, YnJpYW4ua2VpdGhAdWNuLmNs