- 1Division of Immunotherapy, Institute of Human Virology, University of Maryland School of Medicine, Baltimore, MD, United States
- 2OncoImmune, Inc., Rockville, MD, United States
CD24 is a glycosyl-phosphatidyl-inositol linked glycoprotein expressed in a broad range of cell types including cancer cells. Although it is overexpressed in nearly 70% of human cancers, copy number variation of the CD24 locus has not been reported for any cancer. Here, we analyzed the genomics, transcriptomics, and clinical data of 1082 breast cancer (BRCA) samples and other cancer samples from the clinically annotated genomic database, The Cancer Genome Atlas (TCGA). The GISTIC2 method was applied to stratify the CD24 copy number, and Cox regression was performed to compare hazard ratio (HR) of CD24 overexpression, amplification and other traditional prognosis features for overall survival (OS). Our data demonstrated that CD24 amplification strongly correlated with its mRNA overexpression as well as TP53 mutant, cancer proliferation and metastasis features. In particular, CD24 amplification was enriched in basal-like subtype samples and associated with poor clinical outcome. Surprisingly, based on the univariate Cox regression analysis, CD24 overexpression (HR = 1.62, P = 0.010) and copy number amplification (HR = 1.79, P = 0.022) was more relevant to OS than TP53 mutant, mutation counts, diagnosis age, and BRCA subtypes. And based on multivariate survival analysis, CD24 amplification remained the most significant and independent predictor for worse OS (HR = 1.88, P = 0.015).
Introduction
CD24 is located on chromosome 6q21 and encodes a glycosylphosphatidylinositol-linked cell surface glycoprotein (Hough et al., 1994). It is expressed on hematopoietic cells (Li et al., 2004; Israel et al., 2005), neural cells (Nielsen and Cohen, 1996), epithelial cells (Sleeman et al., 2006), muscle cells (Higuchi et al., 1999), stem cells (Lawson et al., 2006; Shackleton et al., 2006), and many other cell types including cancer cells (Kristiansen et al., 2002; Fillmore and Kuperwasser, 2007; Sagiv et al., 2008). In addition to the immunological functions (Li et al., 2004, 2006; Liu and Zheng, 2007), recent studies have implicated CD24 function in tumorigenesis and progression of multiple cancer types, including carcinomas in lung, prostate, ovarian, breast, and brain (Kristiansen et al., 2002, 2003; Fillmore and Kuperwasser, 2007; Sagiv et al., 2008). Cell surface CD24 has been shown to contribute to tumor metastasis and Src oncogene activation. More recently, we and others have reported that CD24 is translocated to nuclei, where it affects the stability of tumor suppressor gene P14ARF and TP53 (Wang et al., 2015). In particular, we have found that CD24 silencing could prevent functional inactivation of p53 by both somatic mutation and viral oncogenes, and that TP53 mutated at a higher rate among glioma and prostate cancer samples with higher CD24 mRNA levels (Wang et al., 2015). As a result, several tumor cell lines have been shown to be oncogenic addicted to CD24 overexpression as their growth and metastasis are diminished upon inactivation of CD24 expression. However, despite the well-documented function of CD24 overexpression in tumorigenesis, the driving force of CD24 overexpression in cancer has not been systematically investigated.
Tumorigenesis is driven by a combination of inherited and acquired genetic alterations. Many studies, including reports from TCGA project, have made use of multiplatform genomic analysis to identify known and new genetic drivers of tumor phenotypes (Hodis et al., 2012; Chen et al., 2016). Copy number variation refers to either extra or missing copies of a gene. Gene copy number amplification is a major genetic mechanism to increase the expression of oncogenes. For example, amplification of ERBB2, the gene encoding human epidermal growth factor receptor two, has been reported in approximately 20% of BRCAs and used for therapeutic decision (Bartlett et al., 2001). Likewise, the MYC oncogene amplification has been established in numerous cancer types and has emerged as a defining feature for the classification of medulloblastoma (Ramaswamy et al., 2016). Therefore, it is of considerable interest to determine whether CD24 is amplified in human cancers, and if so whether such amplification corresponds to CD24 overexpression and clinical outcome. Here, we investigated the copy number status and expression level of CD24 in BRCA, ovarian cancer, lung cancer, and prostate cancer. We reported CD24 amplification in carcinoma of breast, ovarian, lung but not in the prostate, and the copy number amplification was strongly correlated with CD24 mRNA overexpression, which in turn correlated with signature genes of tumor growth and metastasis. Most importantly, CD24 gene amplification seemed to be the most impactful genetic alteration for the prognosis of BRCA.
Materials and Methods
Datasets
We collected the largest publicly available cancer genomics database namely TCGA with genomic, transcriptomic, and clinical data (Figure 1). We accessed the TCGA data portal1 and downloaded mRNA expression quantification profiles (HTSeq–FPKM) and masked copy number segment profiles for BRCA (N = 1082), prostate cancer (N = 496), lung squamous cell carcinoma (N = 500), and ovarian cancer (N = 365). Clinical data files and annotated mutation files of cancer samples were downloaded from cBioPortal for Cancer Genomics2.
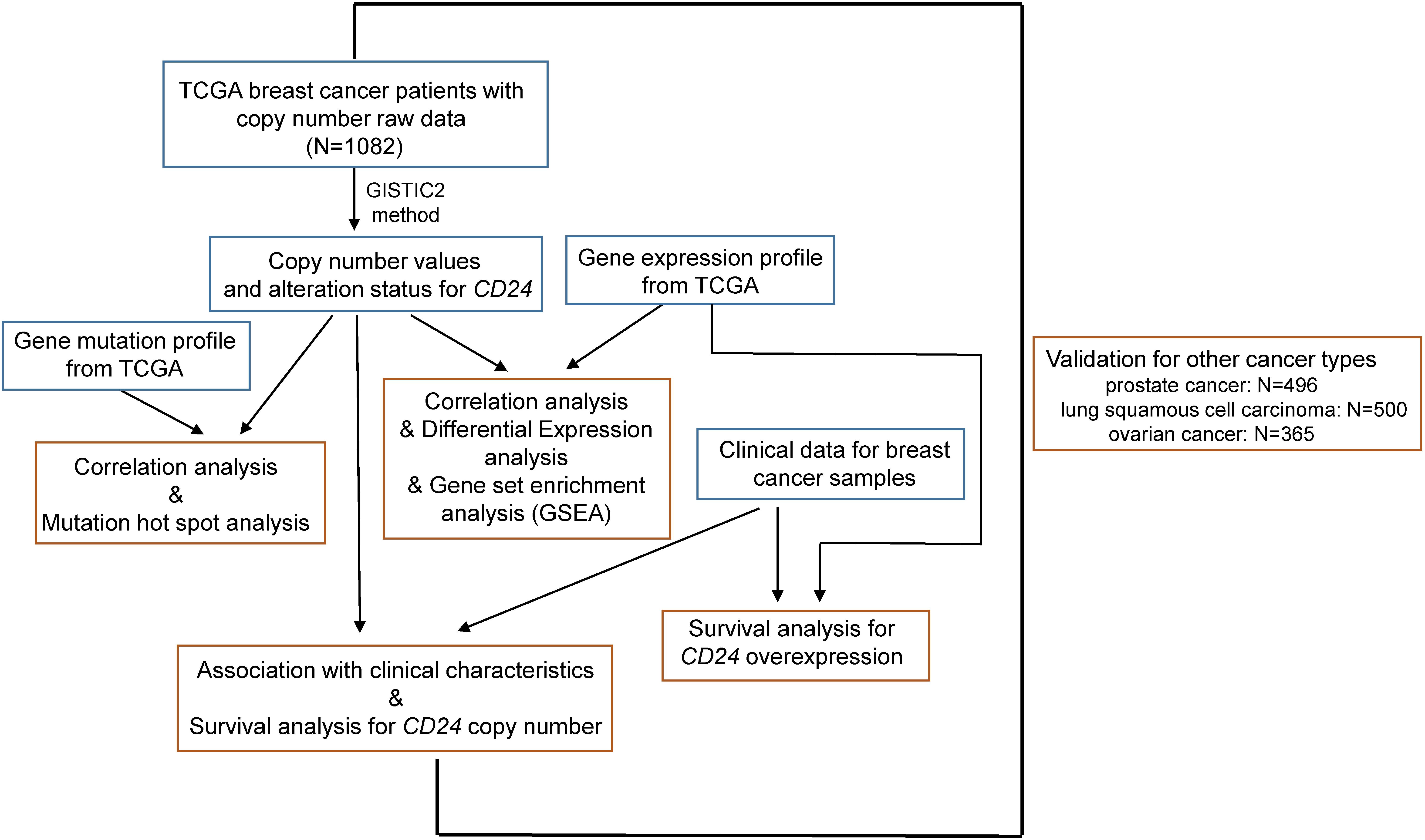
Figure 1. Illustration of study workflow. The flowchart of data collection and method implementation in this work.
Copy Number Analysis
GISTIC2 (Mermel et al., 2011) method was applied to the transformed copy number segment data, with a noise threshold used to determine copy gain or loss. We performed GISTIC version 2.0.22 by using the Homo sapiens (hg38) RefSeq gene annotations3. The copy number values were obtained by examining the distribution of log2 ratios to identify peaks associated with copy number states. The default GISTIC threshold for identifying gains and losses (0.1 and −0.1, respectively) were used. Other GISTIC parameters were the following: genegistic = 1, maxseg = 2,000, js = 2, cap = 1.5, broad = 1, brlen = 0.7, conf = 0.98, armpeel = 1, rx = 0 and gcm = extreme. The GISTIC algorithm takes into account both high and low thresholds for copy number determination across all the input samples to assign significance to copy number variation. The copy number status of low-level gene amplification, high-level gene amplification, low-level gene deletion, and high-level gene deletion was inferred using the “thresholded” calls.
Gene-Set Enrichment Analysis
Gene-set enrichment analysis was performed with the GSEA program (v. 3.0) (Subramanian et al., 2005). The Broad Molecular Signatures Database (MSigDB v6.0) set H (hallmark gene sets) was used, which summarize and represent specific well-defined biological states or processes. The GSEA program was run with 1,000 permutations for statistical significance estimation, and the default signal-to-noise metric between the two phenotypes was used to rank all genes.
Survival Analysis
Univariate and multivariate survival analysis was performed by using the Cox proportional hazard regression model with OS time (5-year) to assess the prognostic value of gene expression, copy number variation, and clinical characteristics (Figure 1). The prognostic value of discrete variables was estimated by Kaplan–Meier survival curves, and the log-rank test was employed to estimate the significance among different survival curves.
Biostatistical Analysis
Data were analyzed by using an unpaired Mann-Whitney test to compare between two groups and one-way analysis of variance (ANOVA) for multiple comparisons. Fisher’s exact test was used for enrichment analysis. The Spearman correlation coefficient was performed to estimate the strength and significance of the association between two continuous variables, such as putative copy number values and mRNA expression (Figure 1). For the differential expression analysis, the Mann-Whitney test with multiple testing adjustment (False Discovery Rate, FDR) determined the significant difference. In the graphs, y-axis error bars represent median with 95% CI as indicated. Statistical calculations were performed using GraphPad Prism software (GraphPad Software, San Diego, CA) or R Software4.
Results
CD24 Overexpression Predicts Adverse Prognosis in BRCA Patients
We first determined the mRNA expression of CD24 in BRCA samples from TCGA, which revealed the transcripts of CD24 were dramatically enhanced in paired BRCA samples (N = 112) compared with adjacent breast normal tissues (P° < °0.0001) (Figure 2A). The upregulation was also observed when unpaired tumors were compared with normal samples (P° < °0.0001) (Supplementary Figure S1A). Since CD24 is abundantly expressed in hematopoietic cells, we evaluated whether CD24 transcripts were affected by the extent of leukocyte infiltration using the common leukocyte antigen CD45 as a reference. We observed no difference in CD45 transcripts between CD24high and CD24low BRCA samples (Supplementary Figure S1B). Therefore, the difference in CD24 transcript levels among BRCA samples was not due to a difference in leukocyte infiltration. Then, we investigated the clinical implication of CD24 overexpression in BRCA patients. Kaplan–Meier survival analysis revealed that higher CD24 mRNA expression significantly associated with worse OS in patients with BRCA (N = 1079, P = 0.0102; Figure 2B). And our in silico analysis of the other two independent BRCA cohorts (Chanrion et al., 2008; Clarke et al., 2013) confirmed this correlation (Supplementary Figure S1C). Besides, higher CD24 expression also predicted poor metastasis-free survival for patients in BRCA metastasis patient cohorts (Desmedt et al., 2007; Minn et al., 2007), as evidenced by Kaplan–Meier curves shown in Supplementary Figure S1D. In summary, CD24 is significantly upregulated in BRCA, and its overexpression is an adverse prognostic factor for BRCA patients.
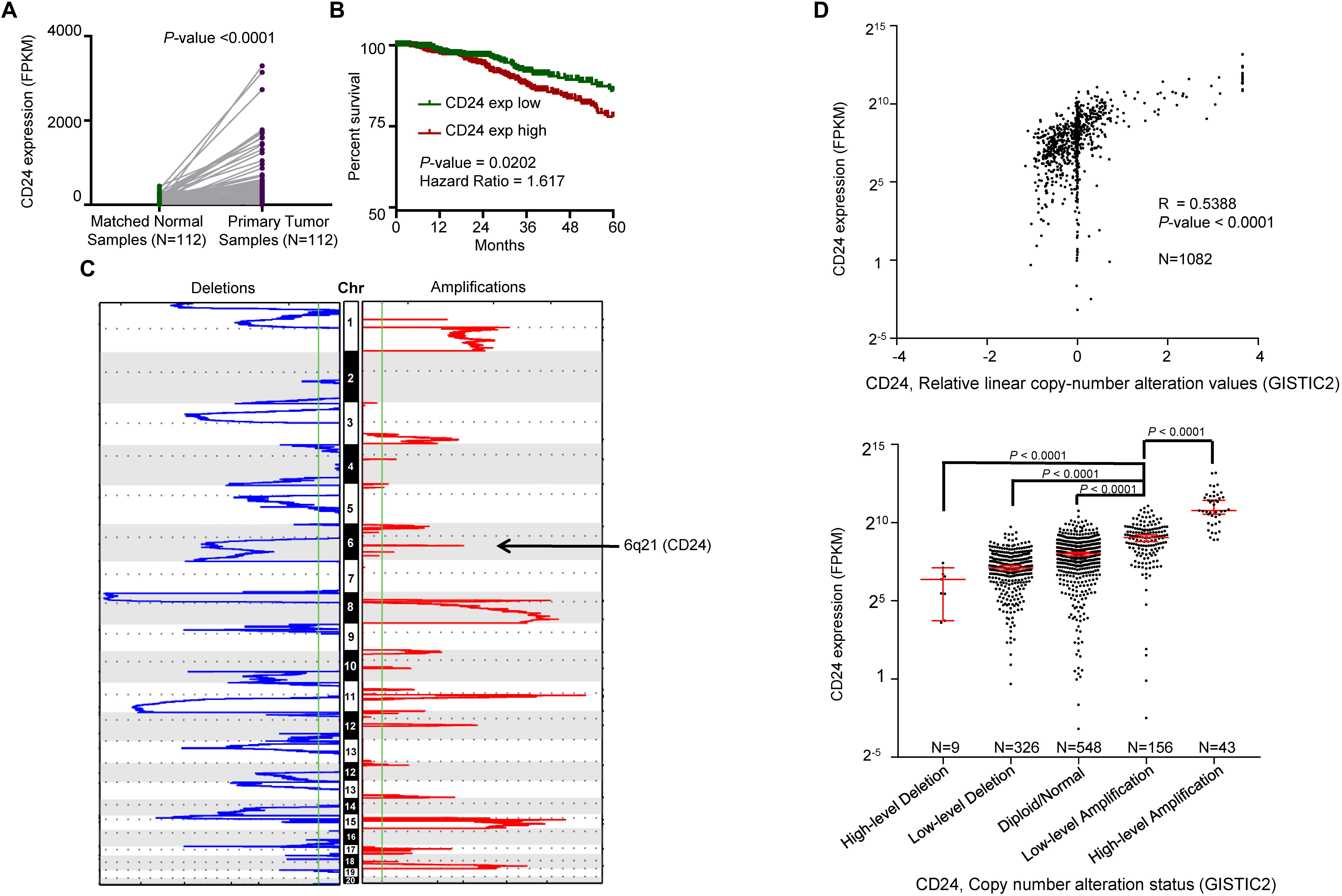
Figure 2. Correlation between CD24 copy number alterations and mRNA expression. (A) Change of CD24 mRNA expression between tumor samples and matched normal samples from TCGA BRCA studies (N = 112). (B) Kaplan–Meier survival curve comparing the high (N = 533) and low (N = 532) expression value of CD24 (determined by the median value) for the TCGA BRCA patient cohort. (C) GISTIC heat map showing genomic copy-number profiles from TCGA BRCA studies. Gain (red) and loss (blue) of each peak are shown. Regions of copy-number gain (6q21) were highlighted. (D) Scatter plot (top) and dot plot (bottom) showing the strong positive correlation between CD24 copy number values defined by GISTIC2 approach and mRNA expression values quantified by FPKM (N = 1082). GISTIC2 method stratified the CD24 copy number values into five categories: high-level copy number deletion (N = 9), low-level copy number deletion (N = 326), diploid normal copy (N = 548), low-level copy number amplification (N = 156), and high-level copy number amplification (N = 43). Statistical significance was determined by the Paired t-test in panel (A), log-rank test in panel (B) and the One-way ANOVA in panel (D) (bottom). The Spearman coefficient of correlation (R) and significance in panel (D) (top) was determined by linear regression.
Copy Number Amplification Correlates to CD24 Up-Regulation in BRCA
We interrogated the chromosomal segment value of TCGA BRCA dataset for significant copy number alterations. As expected, our analysis confirmed many known copy number amplifications, including those observed in 3q26.1 (PIK3CA) (Wu et al., 2005), 8q24.21 (MYC) (Rodriguez-Pinilla et al., 2007), and 11q13 (CNCD1, EMS1) (Ormandy et al., 2003). We also observed the chromosomal region of 6q21 (encompassing CD24) harbored a major amplification (Figure 2C). And a significant correlation between CD24 copy number values and mRNA expression was found among all BRCA samples (Figure 2D, R = 0.5388, P-value <0.0001). Moreover, we stratified BRCA patients into five groups (High-level Deletion, N = 9; Low-level Deletion, N = 326; Diploid, N = 548; Low-level Amplification, N = 156; High-level Amplification, N = 43) based on CD24 copy number values by using GISTIC2 framework. As shown in Figure 2D, approximately one-fifth of all BRCA samples harbored CD24 amplification, which was on par with ERBB2 amplification. Consistently, BRCA samples harboring CD24 amplification exhibited highest mRNA expression than those that exhibit diploid CD24, while CD24 deletion samples had the lowest mRNA expression of CD24 (Figure 2D). Therefore, the gain of copy number is probably a major mechanism that contributes to the up-regulation of CD24 in BRCA.
CD24 Amplification Positively Correlated With Cell Proliferation and MYC Signaling Pathway
To gain insights into the molecular mechanisms underlying pro-tumorigenic action of CD24 amplification in BRCA cells, we first analyzed differential gene expression patterns between CD24 amplified samples (N = 199) and CD24 non-amplified samples (N = 883) in TCGA BRCA dataset (Figure 3A). In total, 79 genes were identified as significantly up-regulated genes, which include six genes (ATG5, C6orf203, QRSL1, PREP, RTN4IP1, and AMD1) located very close to CD24 genomic region at chromosomal loci. More interesting, we found four cell cycle genes (CENPW, CDC20, FOXM1, and PRP11) and four cytokeratin genes (KRT16, KRT6B, KRT17, and KRT81) of these up-regulated expressed genes, which indicating that multiple genes involved in cancer cell proliferation and invasion pathway may be activated concordantly. Besides, several B cell receptor genes were enriched among CD24hi BRCA, the significance of such enrichment remains to be elucidated.
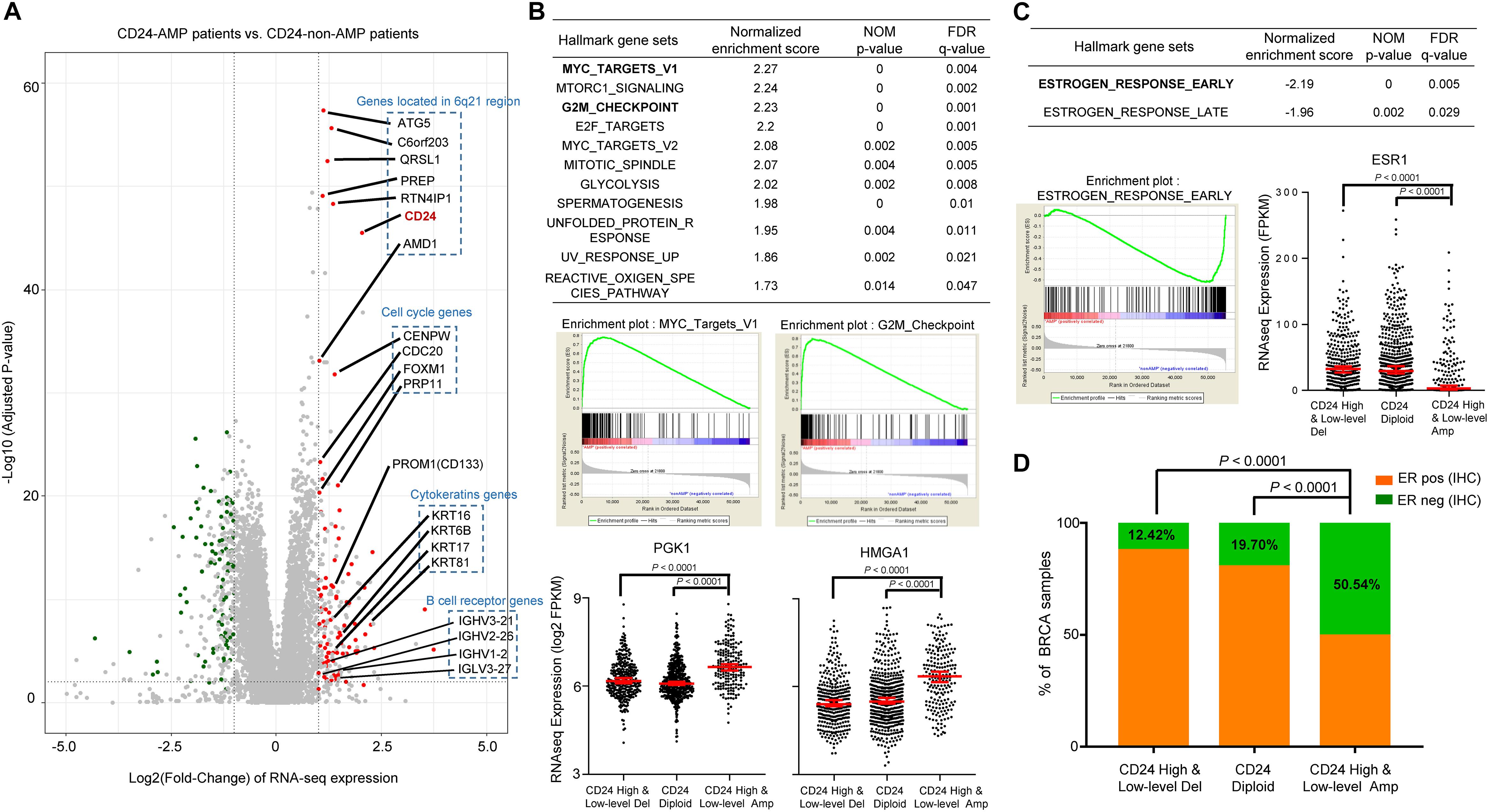
Figure 3. CD24 amplification correlated with cell cycle and estrogen response activity. (A) Volcano plot of mRNA expressions change between BRCA samples harboring CD24-amplification and non-CD24-amplification. The x-axis specifies the fold-changes (FC) and the y-axis specifies the negative logarithm to the base 10 of the adjusted p-values. Gray vertical and horizontal dashed lines reflect the filtering criteria. Red and green dots represent genes expressed at significantly higher (n = °79) or lower (n = °68) levels, respectively. The genes located in the 6q21 chromosomal region and enriched in functional patterns are highlighted. (B) GSEA comparing gene-expression signatures of TCGA breast tumors with the CD24-amplification and non-CD24-amplification. GSEA positive result table (top) showing significant enrichment (FDR < 0.05) of the hallmark gene sets from MSigDB. The enrichment plot on the middle left (right, respectively) shows the distribution of MYC targets (G2M checkpoint, respectively) genes that are positively correlated with the CD24-amplification phenotype. The distribution plot on the bottom shows the marker genes (PGK1 and HMGA1) expression difference among BRCA patients with different CD24 copy number status. (C) GSEA negative association table (top) showing significant enrichment (FDR < 0.05) of the hallmark gene sets from MSigDB. The enrichment plot on the bottom left shows the distribution of estrogen response early genes that are negatively correlated with the CD24-amplification phenotype. The distribution plot on the bottom right shows the marker genes (ESR1) expression difference among BRCA patients with different CD24 copy number status. (D) Distribution of ER-negative (based on IHC) samples among different CD24 copy number groups shows the patients with CD24-amplification are enriched in ER-negative status. Normalized enrichment score (NES) and FDR q-value of GSEA are shown in panels (B,C). Statistical significance was determined by the One-way ANOVA in panels (B,C), and the exact Fisher’s test in panel (D).
We further performed gene set enrichment analysis (GSEA) using the MSigDB hallmark gene sets (Subramanian et al., 2005), which revealed that a large number of gene sets were positively enriched in samples harboring CD24 amplification compared with CD24 non-amplification samples (Figure 3B). Among the 11 significantly enriched gene sets, the groups of “MYC targets” (including V1 and V2), whose expression is connected to c-Myc-dependent phenotypes such as cellular proliferation, transformation, or apoptosis, showed particularly strong enrichment, which was confirmed by dramatically up-regulated expression value of PGK1 (Tang et al., 2009), a MYC target marker gene, in CD24 amplification samples (P < 0.0001; Figure 3B). Notably, genes involved in the “G2/M checkpoint” (genes involved in progression through the cell division cycle) and “E2F targets” (genes encoding cell cycle related targets of E2F transcription factors) were also highly positively enriched in patients harboring CD24 amplification. As an example, HMGA1, an E2F target gene that marks the G2M checkpoint (Schuldenfrei et al., 2011), was significant up-regulated CD24 amplification samples (P < 0.0001; Figure 3B). In summary, these data demonstrate a positive correlation between CD24 amplification and cell proliferation, a key step in oncogenesis.
CD24 Amplification Negatively Correlates With Estrogen Response Activity in BRCA
Gene set enrichment analysis showed that only two hallmark gene sets (“estrogen response early” and “estrogen response late”) were negatively correlated with CD24 amplification (Figure 3C). Then we examined the mRNA expression of human ER (ESR1) among BRCA patients with different CD24 copy number status. As shown in Figure 3C, ESR1 decreased dramatically in BRCA patients harboring CD24 amplification (P < 0.0001). We further accessed the ER status based on IHC data of TCGA BRCA patients and compared the ER-negative rates among different CD24 copy number groups. We observed a significantly higher rate of ER-negative patients in CD24 amplification groups compared with CD24 diploid and deletion groups (Figure 3D, P < 0.0001).
CD24 Amplified Samples Exhibit a Selective Increase of TP53 Mutations
To determine whether CD24 amplification tumors were enriched for the mutations of driver genes, we evaluated its association with the mutation profile of three most commonly altered genes (PIK3CA, TP53, and GATA3) in BRCA. We used the OncoPrint function of cBioPortal for Cancer Genomics tools5 to explore the mutation rate of these three genes in TCGA BRCA dataset (Figure 4A). Remarkably, the mutation rate of TP53 in CD24 amplified samples is 2.6-fold higher than the non-amplified samples, reaching nearly 65% of the total samples (Figure 4B). In contrast, the CD24 amplified samples have reduced mutation rate of PIK3CA and GATA3 genes. Nevertheless, there was no hotspot mutation of TP53 that was specifically enriched in any groups (Figure 4C). These data are consistent with our previous report showing a critical role for CD24 in inactivation of mutant p53 proteins (Wang et al., 2015).
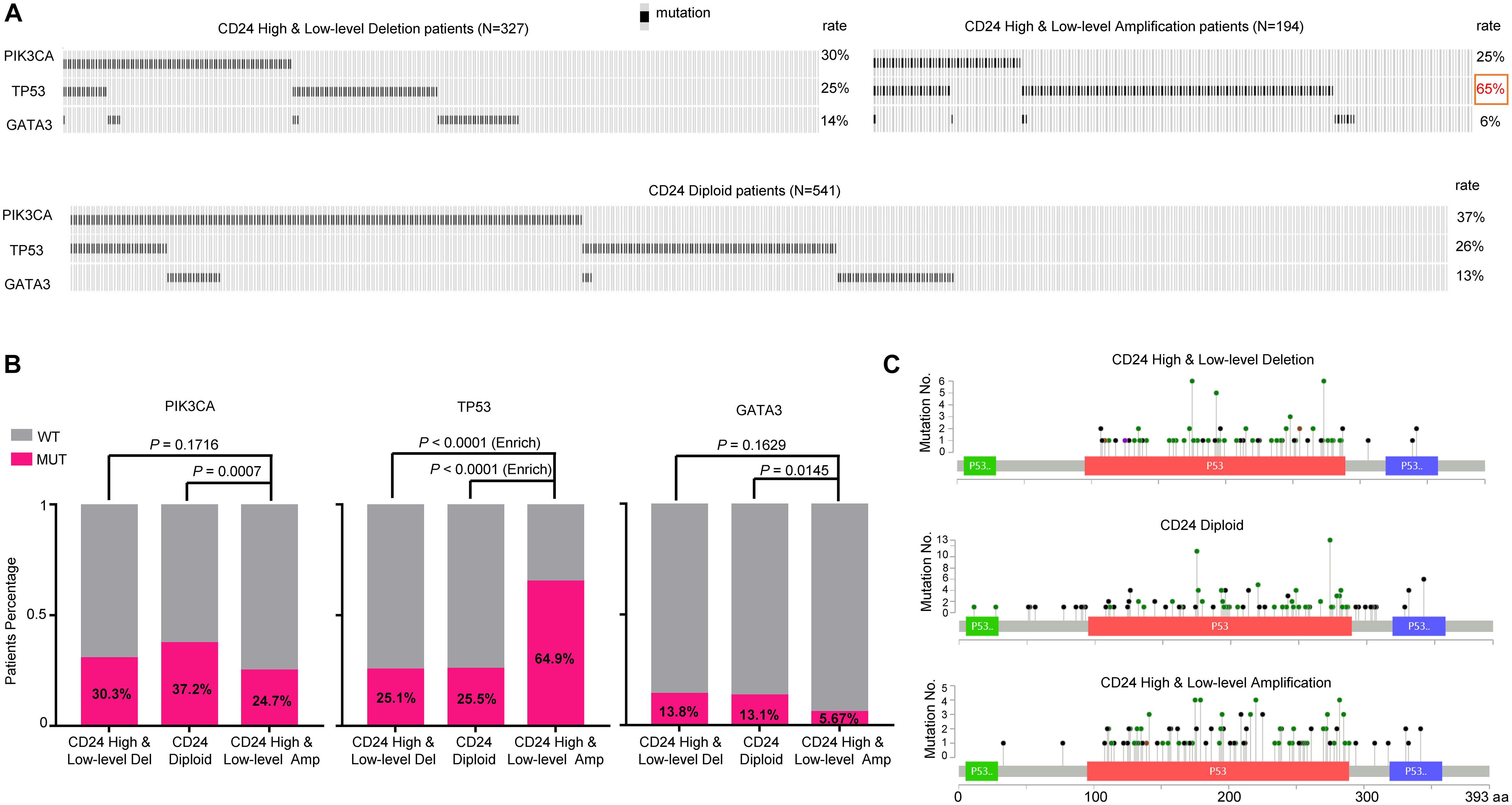
Figure 4. TP53 mutation is selectively enriched in CD24 amplification samples. (A) cBioPortal OncoPrint plot showing the distribution of PIK3CA, TP53 and GATA3 mutation rate in the TCGA BRCA dataset. (B) Bar graphs showing the percentage of TCGA BRCA samples with mutations in PIK3CA, TP53, and GATA3 by different CD24 copy number groups. (C) cBioPortal Lollipop plot showing the loci distribution of mutations in TP53 across TCGA BRCA samples.
Prognostic Significance of CD24 Amplification in BRCA
Breast cancer is a heterogeneous disease with diverse pathological features and survival outcomes (Sorlie et al., 2003), and the basal-like or triple-negative BRCAs (TNBCs, lacking expression of the ER, PR and HER2), characterized by a poor prognosis and no specific targeted therapies group (Badve et al., 2011). We first investigated the distribution of basal-like patients among different CD24 copy number groups. As shown in Figure 5A, Basal-like patients were significantly enriched in CD24 amplification groups compared with CD24 diploid and deletion groups (both P < 0.0001), which was confirmed by dramatically up-regulated expression of six basal-like genes (FOXC1 (Ray et al., 2010), VGLL1 (Castilla et al., 2014), TTK (Rakha et al., 2008), EGFR (Cheang et al., 2008), KRT6B (Lehmann et al., 2011), KRT81 (Lehmann et al., 2011); Figure 5B).
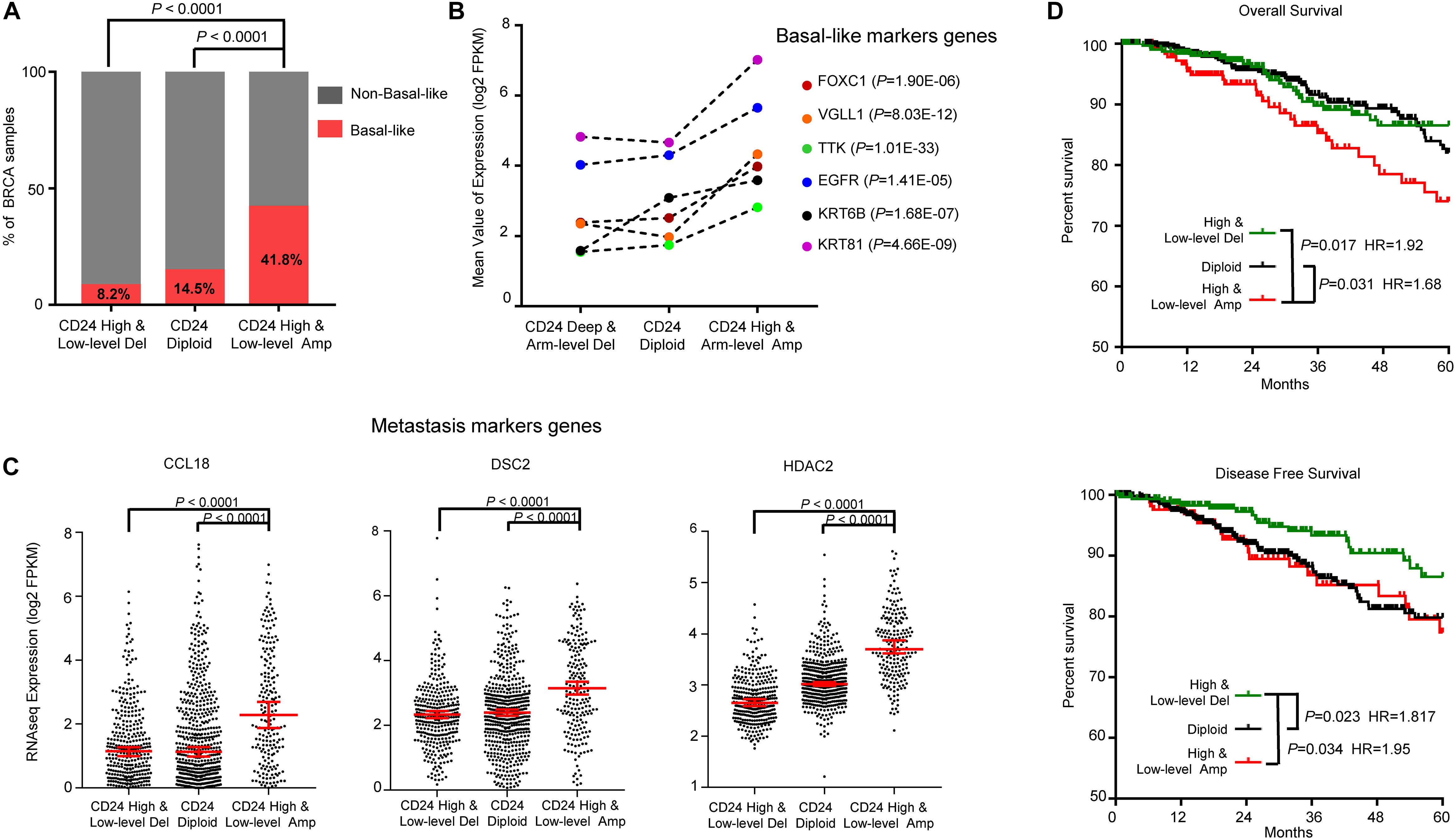
Figure 5. CD24 amplification associated with basal-like subtype and poorer survival of BRCA patients. (A) Distribution of Basal-like samples among different CD24 copy number status subtypes of BRCA from TCGA patient cohort. (B) Mean value of Basal-like marker genes in BRCA. (C) The distribution plot of breast metastasis marker genes expression among BRCA patients with different CD24 copy number status. (D) Kaplan–Meier survival curves comparing the OS and disease-free survival time for different CD24 copy number subtypes in BRCA. Statistical significance was determined by the exact Fisher’s test in panel (A), Wilcoxon test in panel (B), One-way ANOVA in panel (C), and log-rank test in panel (D).
We further examined the distribution of three BRCA metastasis marker genes (CCL18 (Chen et al., 2011), DSC2 (Landemaine et al., 2008) and HDAC2 (Roy et al., 2014); Figure 5C) expression values. Consistent with the correlation between CD24 amplification and BRCA metastasis (Supplementary Figure S1D), the marker genes of BRCA metastasis are significantly up-regulated in CD24 amplification groups.
To determine if the copy number amplification of CD24 has prognostic value on the clinical outcome of BRCA patients, we employed a Kaplan–Meier survival analysis for TCGA BRCA patient cohort. As shown in Figure 5D, BRCA patients with the CD24 amplification had significantly poorer survival rates compared with the CD24 diploid (P = 0.031) and CD24 deletion (P = 0.017) patients. In addition, CD24 deletion increased the probability of disease-free survival (Figure 5D).
To further assess the prognostic potential of CD24 amplification in BRCA, we performed the univariate and multivariate Cox regression analysis, including diagnosis age, tumor subtype, mutation count, TP53 mutant status and CD24 expression value. The multivariate result adjusted for standard clinical and pathological parameters confirmed that the impact of CD24 amplification on OS was independent of BRCA subtype and TP53 mutant status (Table 1). Altogether, the results show that CD24 amplification is a biomarker to predict both clinical Basal-like stratification and adverse outcome for BRCA patients.
CD24 Amplification in Prostate, Lung and Ovarian Cancers
To determine the general significance of CD24 amplification and cancer prognosis, we also analyzed the correlation between CD24 gene copy number and mRNA expression among prostate, lung and ovarian cancer, all known to overexpress CD24 (Fang et al., 2010). As shown in Figure 6A, CD24 amplification was observed at a high rate among ovarian cancer (20.3%) and lung cancer (19.6%), while prostate cancer rarely (0.4%) showed CD24 amplification. Nevertheless, a positive correlation was observed between gene copy number and CD24 mRNA levels. Besides, a strong association was observed between CD24 amplification and OS of lung cancer patients (Figure 6B). Surprisingly, despite a high rate of CD24 amplification in ovarian cancer, such amplification has no prognostic value for OS rate (Figure 6B).
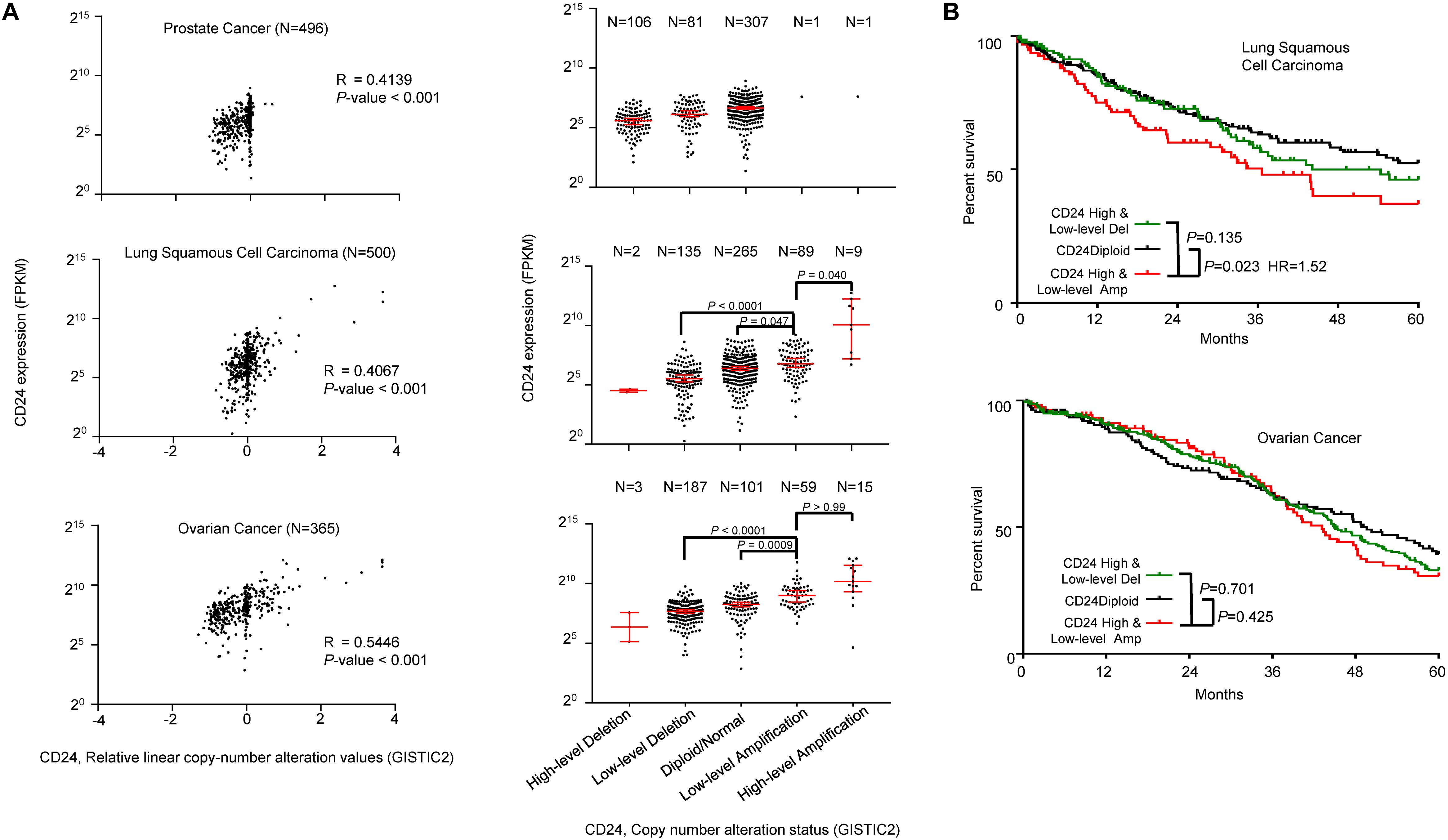
Figure 6. CD24 amplification in different cancer types. (A) Scatter plot (left) and dot plot (right) showing the positive correlation between CD24 copy number values defined by GISTIC2 approach and mRNA expression values quantified by FPKM in prostate cancer (top), lung squamous cell carcinoma (middle) and ovarian cancer (bottom). (B) Kaplan–Meier survival curve comparing the OS time for different CD24 copy number subtypes in lung squamous cell carcinoma (top) and ovarian cancer (bottom). The Spearman coefficient of correlation (R) and significance in panel (A) (left) was determined by linear regression. Statistical significance was determined by the One-way ANOVA in panel (A) (right) and log-rank test in panel (B).
Discussion
Numerous studies have shown CD24 overexpression and its prognostic significance in multiple cancer types (Kristiansen et al., 2003; Fang et al., 2010). However, the cause of CD24 overexpression remained largely enigmatic. Our analysis presented herein established a strong correlation between CD24 overexpression and copy number amplification, thus suggested gene amplification as a potential mechanism for CD24 overexpression. CD24 plays several critical roles in cancer pathogenesis and cell surface CD24 has long been associated with cancer metastasis through its role as selectin ligand (Aigner et al., 1997). Consistently, our data showed CD24 copy number variation was correlated with expression levels of genes known for their association of cancer metastasis, including CCL18, HDAC2, and DSC2. CD24 has oncogenic activity through its regulation of Src/STAT3 pathway (Bretz et al., 2012). We have reported that CD24 over-expression is critically important for the inactivation of mutant p53 protein in cancer cells (Wang et al., 2015). It followed that cancer samples with most TP53 mutations must overexpress CD24. By showing a 2.6-fold enrichment of TP53 mutations among CD24 amplified samples, the data presented here provided further clinical support for the interaction between CD24 and TP53 (Wang et al., 2015; Li et al., 2018). The high rate of CD24 amplification among multiple cancer types satisfies major criteria of CD24 as a bona fide oncogene (Hanahan and Weinberg, 2011). Obviously, while gene amplification provides a genetic fix that facilitates CD24 overexpression in the cancer cell, this is not the only mechanism by which CD24 is induced at high levels among cancer. Only 2/496 prostate cancer patients show CD24 amplification. Therefore, non-amplification mechanisms, such as activation of HIF1α (Thomas et al., 2012), may work either independently or in concert with gene amplification to drive a high level of CD24 expression in cancer.
By comparing the OS of patients with or without CD24 amplification, we showed that CD24 amplification was among the most significant genetic prognostic indicator of OS for BRCA patients. Based onHR, the impact of CD24 amplification (HR = 1.79) was considerably stronger than TP53 mutations (HR = 1.35) and basal-like cancer type (HR = 1.32). Since the association remains robust and significant in multivariate analysis, CD24 amplification can be considered as an independent diagnosis marker for BRCA patient prognosis. Besides, CD24 amplification is enriched in basal-like BRCA patients which has a poorer prognosis, it is of interest to consider whether CD24 amplification is the driving factor for poor prognosis. We consider CD24 gene amplification as the primary factor in prognosis as the impact is undiminished after multivariate analysis and its impact is higher than basal-like features. The significance of CD24 amplification is further enhanced as the frequency of CD24 amplification is relatively high among major cancer types, including breast (18.4%), ovarian (20.3%), and lung cancer (19.6%). Surprisingly, CD24 amplification in ovarian cancer does not associate withOS. One potential interpretation may relate to the fact that only intracellular CD24 appears to affect ovarian cancer survival (Kristiansen et al., 2002). Therefore, factors that control CD24 processing may mask the impact of gene amplification.
To our knowledge, this is the first demonstration of CD24 copy number amplification during carcinogenesis. Despite functional role for CD24 in aspects of cancer development, there is no clinical evidence that CD24 expression is significant enough for cancer (Fang et al., 2010; Kwon et al., 2015). Our analysis revealed, previously undescribed and marked the oncogenic role of CD24 copy number amplification in the BRCA. It is certainly convinced that the oncogenic features associated with CD24 amplification cancers could influence response to BRCA treatment strategies. Lacking independent dataset and experimental validation is a limitation to this work, however, given the remarkably CD24 amplification associated with oncogenic and prognosis signatures, we believe that this phenomenon is generalizable. The future analysis of other datasets with large cancer patients cohort and abundant experimental results will be important to confirm these findings. An increasing amount of evidence demonstrates that non-coding RNAs (ncRNAs), particularly microRNAs (miRNAs) (Lu et al., 2005; Chen et al., 2019) and long non-coding RNAs (lncRNAs) (Huarte, 2015; Chen et al., 2017), are aberrantly expressed in several complex diseases, including various cancers. And many high-quality computational inquiries into the genomic investigation of ncRNA–gene-cancer associations (Chen and Yan, 2013; Chen and Huang, 2017; Chen et al., 2018a, b), revealed the prognosis value and drug target potential for clinical use. There may be many ncRNA-mediated epigenetic changes to CD24 expression to be discovered in the future, as an important complement to overexpression and copy number amplification, provide a more accurate prediction for clinical outcome. Besides, the machine-based-learning approaches are being developed to aid the diagnosis of clinical samples. A variety of these machine learning techniques, including decision trees, Bayesian networks, support vector machines, and convolutional neural networks have been widely applied in cancer research for the development of predictive models, resulting in effective and accurate decision making (Kourou et al., 2015; Montazeri et al., 2016; Esteva et al., 2019; Li et al., 2019). We believe that an approach with integrated molecular feature analysis (like oncogenic copy number amplification analysis) and machine learning prediction models in the future will strengthen the capabilities of cancer diagnosis, prognosis, and even treatment.
Genotyping for CD24 copy number variation could provide a simple selection or stratification factor to identify populations of interest for cancer risk and treatment subtype, and its exploration as a predictive biomarker is warranted. We believe the CD24 amplification could serve as a promising therapeutic target and prognosis marker.
Data Availability
Publicly available datasets were analyzed in this study. This data can be found here: https://portal.gdc.cancer.gov/.
Author Contributions
PeZ performed the analysis and prepared the manuscript. YL and PaZ supervised the studies and revised the manuscript.
Conflict of Interest Statement
YL and PaZ are co-founders of, and have equity interests in OncoImmune, Inc.
The remaining author declares that the research was conducted in the absence of any commercial or financial relationships that could be construed as a potential conflict of interest.
Acknowledgments
Part of the study was performed when the authors were at the Children’s National Medical Center.
Supplementary Material
The Supplementary Material for this article can be found online at: https://www.frontiersin.org/articles/10.3389/fgene.2019.00560/full#supplementary-material
FIGURE S1 | Correlation between CD24 copy number alterations and mRNA expression. (A) Change of CD24 mRNA expression between all tumor samples (N = 1082) and normal samples (N = 112) from TCGA BRCA cancer studies. (B) Change of CD45 mRNA expression between CD24 higher expression groups (CD24 exp high, N = 541) and CD24 lower expression samples (CD24 exp low, N = 541) from TCGA BRCA studies. (C) Kaplan–Meier OS curves comparing the high and low expression value of CD24 for two independent BRCA patient cohorts. (D) Kaplan–Meier metastasis survival curves comparing the high and low expression value of CD24 for two independent BRCA patient cohorts. Statistical significance was determined by One-way ANOVA in panel (A), the Wilcoxon test in panel (B), and the log-rank test in panels (C,D).
Abbreviations
BRCA, breast cancer; ER, estrogen receptor; GSEA, gene-set enrichment analysis; HR, hazard ratio; IHC, immunohistochemistry; OS, overall survival; PR, progesterone receptor; TCGA, the cancer genome atlas; TNBC, triple-negative breast cancers.
Footnotes
- ^ https://portal.gdc.cancer.gov/, Accessed on February 2018
- ^ http://www.cbioportal.org/index.do, Accessed on February 2018
- ^ ftp://ftp.broadinstitute.org/pub/GISTIC2.0/refgenes/
- ^ https://www.r-project.org/
- ^ http://www.cbioportal.org/
References
Aigner, S., Sthoeger, Z. M., Fogel, M., Weber, E., Zarn, J., Ruppert, M., et al. (1997). CD24, a mucin-type glycoprotein, is a ligand for p-selectin on human tumor cells. Blood 89, 3385–3395. doi: 10.1063/1.4773993
Badve, S., Dabbs, D. J., Schnitt, S. J., Baehner, F. L., Decker, T., Eusebi, V., et al. (2011). Basal-like and triple-negative breast cancers: a critical review with an emphasis on the implications for pathologists and oncologists. Mod. Pathol. 24, 157–167. doi: 10.1038/modpathol.2010.200
Bartlett, J. M. S., Going, J. J., Mallon, E. A., Watters, A. D., Reeves, J. R., Stanton, P., et al. (2001). Evaluating HER2 amplification and overexpression in breast cancer. J. Pathol. 195, 422–428. doi: 10.1002/path.971
Bretz, N. P., Salnikov, A. V., Perne, C., Keller, S., Wang, X., Mierke, C. T., et al. (2012). CD24 controls Src/STAT3 activity in human tumors. Cell. Mol. Life Sci. 69, 3863–3879. doi: 10.1007/s00018-012-1055-9
Castilla, M. Á, López-García, M. Á, Atienza, M. R., Rosa-Rosa, J. M., Diáz-Martín, J., Pecero, M. L., et al. (2014). VGLL1 expression is associated with a triple-negative basal-like phenotype in breast cancer. Endocr. Relat. Cancer 21, 587–599. doi: 10.1530/ERC-13-0485
Chanrion, M., Negre, V., Fontaine, H., Salvetat, N., Bibeau, F., Mac Grogan, G., et al. (2008). A gene expression signature that can predict the recurrence of tamoxifen-treated primary breast cancer. Clin. Cancer Res. 14, 1744–1752. doi: 10.1158/1078-0432.CCR-07-1833
Cheang, M. C. U., Voduc, D., Bajdik, C., Leung, S., McKinney, S., Chia, S. K., et al. (2008). Basal-like breast cancer defined by five biomarkers has superior prognostic value than triple-negative phenotype. Clin. Cancer Res. 14, 1368–1376. doi: 10.1158/1078-0432.CCR-07-1658
Chen, J., Yao, Y., Gong, C., Yu, F., Su, S., Chen, J., et al. (2011). CCL18 from tumor-associated macrophages promotes breast cancer metastasis via PITPNM3. Cancer Cell 19, 541–555. doi: 10.1016/j.ccr.2011.02.006
Chen, J. C., Alvarez, M. J., Talos, F., Dhruv, H., Rieckhof, G. E., Iyer, A., et al. (2016). Erratum: identification of causal genetic drivers of human disease through systems-level analysis of regulatory networks. Cell 166:1055. doi: 10.1016/j.cell.2016.07.036
Chen, X., and Huang, L. (2017). LRSSLMDA: laplacian regularized sparse subspace learning for MiRNA-disease association prediction. PLoS Comput. Biol. 13:e1005912. doi: 10.1371/journal.pcbi.1005912
Chen, X., Wang, L., Qu, J., Guan, N.-N., and Li, J.-Q. (2018a). Predicting miRNA–disease association based on inductive matrix completion. Bioinformatics 34, 4256–4265. doi: 10.1093/bioinformatics/bty503
Chen, X., Yin, J., Qu, J., and Huang, L. (2018b). MDHGI: matrix decomposition and heterogeneous graph inference for miRNA-disease association prediction. PLoS Comput. Biol. 14:e1006418. doi: 10.1371/journal.pcbi.1006418
Chen, X., Xie, D., Zhao, Q., and You, Z.-H. (2019). MicroRNAs and complex diseases: from experimental results to computational models. Brief. Bioinform. 20, 515–539. doi: 10.1093/bib/bbx130
Chen, X., Yan, C. C., Zhang, X., and You, Z.-H. (2017). Long non-coding RNAs and complex diseases: from experimental results to computational models. Brief. Bioinform. 18, 558–576. doi: 10.1093/bib/bbw060
Chen, X., and Yan, G.-Y. (2013). Novel human lncRNA–disease association inference based on lncRNA expression profiles. Bioinformatics 29, 2617–2624. doi: 10.1093/bioinformatics/btt426
Clarke, C., Madden, S. F., Doolan, P., Aherne, S. T., Joyce, H., O’Driscoll, L., et al. (2013). Correlating transcriptional networks to breast cancer survival: a large-scale coexpression analysis. Carcinogenesis 34, 2300–2308. doi: 10.1093/carcin/bgt208
Desmedt, C., Piette, F., Loi, S., Wang, Y., Lallemand, F., Haibe-Kains, B., et al. (2007). Strong time dependence of the 76-gene prognostic signature for node-negative breast cancer patients in the TRANSBIG multicenter independent validation series. Clin. Cancer Res. 13, 3207–3214. doi: 10.1158/1078-0432.ccr-06-2765
Esteva, A., Robicquet, A., Ramsundar, B., Kuleshov, V., DePristo, M., Chou, K., et al. (2019). A guide to deep learning in healthcare. Nat. Med. 25, 24–29. doi: 10.1038/s41591-018-0316-z
Fang, X., Zheng, P., Tang, J., and Liu, Y. (2010). CD24: from A to Z. Cell. Mol. Immunol. 7, 100–103. doi: 10.1038/cmi.2009.119
Fillmore, C., and Kuperwasser, C. (2007). Human breast cancer stem cell markers CD44 and CD24: enriching for cells with functional properties in mice or in man? Breast Cancer Res. 3, 9–11. doi: 10.1186/bcr1673
Hanahan, D., and Weinberg, R. A. (2011). Hallmarks of cancer: the next generation. Cell 144, 646–674. doi: 10.1016/j.cell.2011.02.013
Higuchi, I., Kawai, H., Kawajiri, M., Fukunaga, H., Horikiri, T., Niiyama, T., et al. (1999). Statistically significant differences in the number of CD24 positive muscle fibers and satellite cells between sarcoglycanopathy and age-matched becker muscular dystrophy patients. Intern. Med. 38, 412–415. doi: 10.2169/internalmedicine.38.412
Hodis, E., Watson, I. R., Kryukov, G. V., Arold, S. T., Imielinski, M., Theurillat, J. P., et al. (2012). A landscape of driver mutations in melanoma. Cell 150, 251–263. doi: 10.1016/j.cell.2012.06.024
Hough, M. R., Rosten, P. M., Sexton, T. L., Kay, R., and Humphries, R. K. (1994). Mapping of CD24 and homologous sequences to multiple chromosomal loci. Genomics 22, 154–161. doi: 10.1006/geno.1994.1356
Huarte, M. (2015). The emerging role of lncRNAs in cancer. Nat. Med. 21, 1253–1261. doi: 10.1038/nm.3981
Israel, E., Kapelushnik, J., Yermiahu, T., Levi, I., Yaniv, I., Shpilberg, O., et al. (2005). Expression of CD24 on CD19-CD79a+early B-cell progenitors in human bone marrow. Cell. Immunol. 236, 171–178. doi: 10.1016/j.cellimm.2005.08.026
Kourou, K., Exarchos, T. P., Exarchos, K. P., Karamouzis, M. V., and Fotiadis, D. I. (2015). Machine learning applications in cancer prognosis and prediction. Comput. Struct. Biotechnol. J. 13, 8–17. doi: 10.1016/j.csbj.2014.11.005
Kristiansen, G., Denkert, C., Dahl, E., Pilarsky, C., and Hauptmann, S. (2002). CD24 is expressed in ovarian cancer and is a new independent prognostic marker of patient survival. Am. J. Pathol. 161, 1215–1221. doi: 10.1016/S0002-9440(10)64398-2
Kristiansen, G., Winzer, K.-J., Mayordomo, E., Bellach, J., Schlüns, K., Denkert, C., et al. (2003). CD24 expression is a new prognostic marker in breast cancer. Clin. Cancer Res. 9, 4906–4913.
Kwon, M. J., Han, J., Seo, J. H., Song, K., Jeong, H. M., Choi, J.-S., et al. (2015). CD24 overexpression is associated with poor prognosis in luminal a and triple-negative breast cancer. PLoS One 10:e0139112. doi: 10.1371/journal.pone.0139112
Landemaine, T., Jackson, A., Bellahcène, A., Rucci, N., Sin, S., Abad, B. M., et al. (2008). A six-gene signature predicting breast cancer lung metastasis. Cancer Res. 68, 6092–6099. doi: 10.1158/0008-5472.CAN-08-0436
Lawson, D. A., Xin, L., Lukacs, R. U., Cheng, D., and Witte, O. N. (2006). Isolation and functional characterization of murine prostate stem cells. Proc. Natl. Acad. Sci. U.S.A. 104, 181–186. doi: 10.1073/pnas.0609684104
Lehmann, B. D., Bauer, J. A., Chen, X., Sanders, M. E., Chakravarthy, A. B., Shyr, Y., et al. (2011). Identification of human triple-negative breast cancer subtypes and preclinical models for selection of targeted therapies. J. Clin. Investig. 121, 2750–2767. doi: 10.1172/JCI45014DS1
Li, D., Hu, M., Liu, Y., Ye, P., Du, P., Li, C. S., et al. (2018). CD24-p53 axis suppresses diethylnitrosamine-induced hepatocellular carcinogenesis by sustaining intrahepatic macrophages. Cell Discov. 4:6. doi: 10.1038/s41421-017-0007-9
Li, O., Chang, X., Zhang, H., Kocak, E., Ding, C., Zheng, P., et al. (2006). Massive and destructive T cell response to homeostatic cue in CD24-deficient lymphopenic hosts. J. Exp. Med. 203, 1713–1720. doi: 10.1084/jem.20052293
Li, O., Zheng, P., and Liu, Y. (2004). CD24 expression on T cells is required for optimal T cell proliferation in lymphopenic host. J. Exp. Med. 200, 1083–1089. doi: 10.1084/jem.20040779
Li, Y., Huang, C., Ding, L., Li, Z., Pan, Y., and Gao, X. (2019). Deep learning in bioinformatics: introduction, application, and perspective in the big data era. arXiv doi: 10.1016/j.ymeth.2019.04.008
Liu, Y., and Zheng, P. (2007). CD24: a genetic checkpoint in T cell homeostasis and autoimmune diseases. Trends Immunol. 28, 315–320. doi: 10.1016/j.it.2007.05.001
Lu, J., Getz, G., Miska, E. A., Alvarez-Saavedra, E., Lamb, J., Peck, D., et al. (2005). MicroRNA expression profiles classify human cancers. Nature 435, 834–838. doi: 10.1038/nature03702
Mermel, C. H., Schumacher, S. E., Hill, B., Meyerson, M. L., Beroukhim, R., and Getz, G. (2011). GISTIC2.0 facilitates sensitive and confident localization of the targets of focal somatic copy-number alteration in human cancers. Genome Biol. 12, 1–14. doi: 10.1186/gb-2011-12-4-r41
Minn, A. J., Gupta, G. P., Padua, D., Bos, P., Nguyen, D. X., Nuyten, D., et al. (2007). Lung metastasis genes couple breast tumor size and metastatic spread. Proc. Natl. Acad. Sci. U.S.A. 104, 6740–6745. doi: 10.1073/pnas.0701138104
Montazeri, M., Montazeri, M., Montazeri, M., and Beigzadeh, A. (2016). Machine learning models in breast cancer survival prediction. Technol. Health Care 24, 31–42. doi: 10.3233/THC-151071
Nielsen, P., and Cohen, J. (1996). mCD24, a glycoprotein transiently inhibitor of neurite outgrowth expressed by neurons, is an inhibitor of neurite outgrowth. Neuroscience 16, 2624–2634. doi: 10.1523/jneurosci.16-08-02624.1996
Ormandy, C. J., Musgrove, E. A., Hui, R., Daly, R. J., and Sutherland, R. L. (2003). Cyclin D1, EMS1 and 11q13 ampli cation in breast cancer. Breast Cancer Res. Treat. 78, 323–335. doi: 10.1023/A:1023033708204
Rakha, E. A., Reis-Filho, J. S., and Ellis, I. O. (2008). Basal-like breast cancer: a critical review. J. Clin. Oncol. 26, 2568–2581. doi: 10.1200/JCO.2007.13.1748
Ramaswamy, V., Remke, M., Bouffet, E., Bailey, S., Clifford, S. C., Doz, F., et al. (2016). Risk stratification of childhood medulloblastoma in the molecular era: the current consensus. Acta Neuropathol. 131, 821–831. doi: 10.1007/s00401-016-1569-6
Ray, P. S., Wang, J., Qu, Y., Sim, M. S., Shamonki, J., Bagaria, S. P., et al. (2010). FOXC1 is a potential prognostic biomarker with functional significance in basal-like breast cancer. Cancer Res. 70, 3870–3876. doi: 10.1158/0008-5472.CAN-09-4120
Rodriguez-Pinilla, S. M., Jones, R. L., Lambros, M. B. K., Arriola, E., Savage, K., James, M., et al. (2007). MYC amplification in breast cancer: a chromogenic in situ hybridisation study. J. Clin. Pathol. 60, 1017–1023. doi: 10.1136/jcp.2006.043869
Roy, S. S., Gonugunta, V. K., Bandyopadhyay, A., Rao, M. K., Goodall, G. J., Sun, L. Z., et al. (2014). Significance of PELP1/HDAC2/miR-200 regulatory network in EMT and metastasis of breast cancer. Oncogene 33, 3707–3716. doi: 10.1038/onc.2013.332
Sagiv, E., Starr, A., Rozovski, U., Khosravi, R., Altevogt, P., and Wang, T. (2008). Targeting CD24 for treatment of colorectal and pancreatic cancer by monoclonal antibodies or small interfering RNA. Cancer Res. 68, 2803–2813.
Schuldenfrei, A., Belton, A., Kowalski, J., Talbot, C. C., Di Cello, F., Poh, W., et al. (2011). HMGA1 drives stem cell, inflammatory pathway, and cell cycle progression genes during lymphoid tumorigenesis. BMC Genomics 12:549. doi: 10.1186/1471-2164-12-549
Shackleton, M., Simpson, K. J., Stingl, J., Smyth, G. K., Wu, L., Lindeman, G. J., et al. (2006). Generation of a functional mammary gland from a single stem cell. Nature 439, 84–88. doi: 10.1038/nature04372
Sleeman, K. E., Kendrick, H., Ashworth, A., Isacke, C. M., and Smalley, M. J. (2006). CD24 staining of mouse mammary gland cells defines luminal epithelial, myoepithelial / basal and non-epithelial cells. Breast Cancer Res. 8, 6–11. doi: 10.1186/bcr1371
Sorlie, T., Tibshirani, R., Parker, J., Hastie, T., Marron, J. S., Nobel, A., et al. (2003). Repeated observation of breast tumor subtypes in independent gene expression data sets. Proc. Natl. Acad. Sci. U.S.A. 100, 8418–8423. doi: 10.1073/pnas.0932692100
Subramanian, A., Tamayo, P., Mootha, V. K., Mukherjee, S., Ebert, B. L., Gillette, M. A., et al. (2005). Gene set enrichment analysis: a knowledge-based approach for interpreting genome-wide expression profiles. Proc. Natl. Acad. Sci. U.S.A. 102, 15545–15550. doi: 10.1073/pnas.0506580102
Tang, S. W., Chang, W. H., Su, Y. C., Chen, Y. C., Lai, Y. H., Wu, P. T., et al. (2009). MYC pathway is activated in clear cell renal cell carcinoma and essential for proliferation of clear cell renal cell carcinoma cells. Cancer Lett. 273, 35–43. doi: 10.1016/j.canlet.2008.07.038
Thomas, S., Harding, M. A., Smith, S. C., Overdevest, J. B., Nitz, M. D., Frierson, H. F., et al. (2012). CD24 is an effector of HIF-1-driven primary tumor growth and metastasis. Cancer Res. 72, 5600–5612. doi: 10.1158/0008-5472.CAN-11-3666
Wang, L., Liu, R., Ye, P., Wong, C., Chen, G.-Y., Zhou, P., et al. (2015). Intracellular CD24 disrupts the ARF–NPM interaction and enables mutational and viral oncogene-mediated p53 inactivation. Nat. Commun. 6:5909. doi: 10.1038/ncomms6909
Keywords: breast cancer, biomarker, copy number variation, CD24, TP53, basal-like
Citation: Zhang P, Zheng P and Liu Y (2019) Amplification of the CD24 Gene Is an Independent Predictor for Poor Prognosis of Breast Cancer. Front. Genet. 10:560. doi: 10.3389/fgene.2019.00560
Received: 22 March 2019; Accepted: 29 May 2019;
Published: 12 June 2019.
Edited by:
Xing Chen, China University of Mining and Technology, ChinaReviewed by:
Sungwon Jung, Gachon University, South KoreaQi Zhao, Shenyang Aerospace University, China
Huiluo Cao, The University of Hong Kong, Hong Kong
Copyright © 2019 Zhang, Zheng and Liu. This is an open-access article distributed under the terms of the Creative Commons Attribution License (CC BY). The use, distribution or reproduction in other forums is permitted, provided the original author(s) and the copyright owner(s) are credited and that the original publication in this journal is cited, in accordance with accepted academic practice. No use, distribution or reproduction is permitted which does not comply with these terms.
*Correspondence: Pan Zheng, pzheng@ihv.umaryland.edu; Yang Liu, yaliu@ihv.umaryland.edu