- 1College of Basic Medical Science, Zhejiang Chinese Medical University, Hangzhou, China
- 2The First Affiliated Hospital of Zhejiang Chinese Medical University, Hangzhou, China
- 3Key Laboratory of Chinese Medicine Rheumatology of Zhejiang Province, Research Institute of Chinese Medical Clinical Foundation and Immunology, College of Basic Medical Science, Zhejiang Chinese Medical University, Hangzhou, China
Background: Several studies have demonstrated an increased risk of periodontitis (PD) among patients diagnosed with systemic lupus erythematosus (SLE). However, the underlying common mechanism between them remains incompletely understood. Accordingly, the aim of this study is to examine diagnostic biomarkers and potential therapeutic targets for SLE and PD by leveraging publicly accessible microarray datasets and transcriptome analysis.
Method: Datasets pertaining to SLE and PD were retrieved from the Gene Expression Omnibus (GEO) database, and subsequently analyzed for differentially expressed genes (DEGs). Key gene modules were identified through weighted gene co-expression network analysis (WGCNA), and shared genes were obtained by overlapping key genes between DEGs and WGCNA. These shared genes were subsequently subjected to Gene Ontology (GO) and Kyoto Encyclopedia of Genes and Genomes (KEGG) pathway enrichment analyses, leading to the establishment of a Protein-Protein Interaction (PPI) network. Random forest (RF) and Least Absolute Shrinkage and Selection Operator (Lasso) regression were employed to identify key hub genes. Receiver operating characteristic (ROC) curves were generated using a new validation dataset to evaluate the performance of candidate genes. Finally, levels of immune cell infiltration in SLE and PD were assessed using CIBERSORTx.
Results: A total of 50 core genes were identified between the genes screened by WGCNA and DEGs. Functional enrichment analysis revealed that these genes are primarily associated with the PI3K-Akt and B-cell receptor signaling pathways. Additionally, using machine learning algorithms and ROC curve analysis, a total of 8 key genes (PLEKHA1, CEACAM1, TNFAIP6, TCN2, GLDC, GNG7, LY96, VCAN) were identified Finally, immune infiltration analysis highlighted the significant roles of neutrophils, monocytes, plasma cells, and gammadelta T cells (γδ T cells) in the pathogenesis of both SLE and PD.
Conclusion: This study identifies 8 hub genes that could potentially serve as diagnostic markers for both SLE and PD, highlighting the importance of VCAN and LY96 in diagnosis. Moreover, the involvement of the PI3K-Akt signaling pathway in both diseases suggests its significant role. These identified key genes and signaling pathways lay the groundwork for deeper comprehension of the interplay between SLE and PD.
1 Introduction
Systemic lupus erythematosus (SLE) is a complex inflammatory autoimmune disease characterized by the interplay of genetic, immunological, and environmental factors (Akhil et al., 2023). The multi-system involvement of SLE results in a broad spectrum of clinical manifestations, often affecting the skin, joints, kidneys, cardiovascular, and respiratory systems (Fava and Petri, 2019; Ameer et al., 2022). In recent years, there has been growing attention to oral health complications in SLE patients, including temporomandibular joint dysfunction, tooth loss, and oral mucosal lesions (Aceves-Avila et al., 2013; Benli et al., 2021). Notably, the incidence of dental caries is significantly higher in SLE patients than in the general population, closely linked to reduced salivary secretion and alterations in the oral microbiome (Loyola Rodriguez et al., 2016). These oral health issues not only diminish the quality of life but also may exacerbate the systemic burden of the disease (Corrêa et al., 2018).
Periodontitis (PD) is a widespread chronic inflammatory disease, with a global prevalence estimated between 20% and 50% (Nazir, 2017). The pathophysiology of PD is primarily driven by bacterial infection, leading to persistent inflammation and the destruction of periodontal tissues (Sedghi et al., 2021). The progression of PD includes gingival inflammation, formation of periodontal pockets, bone resorption, and eventual tooth loss (Papapanou et al., 2018; Tonetti et al., 2018). PD is closely associated with several systemic diseases, including cardiovascular diseases, diabetes, rheumatoid arthritis, and SLE (Rutter-Locher et al., 2017; Baima et al., 2022; Kobayashi and Bartold, 2023). Among these, the relationship between SLE and PD has received particular attention. Epidemiological studies indicate that SLE patients are at a significantly increased risk of developing PD (Hussain et al., 2022). Clinical studies further reveal a bidirectional influence between SLE and PD. One study demonstrated that periodontal treatment in SLE patients significantly reduced disease activity, suggesting that effective management of periodontitis may positively impact the systemic condition of SLE (Fabbri et al., 2014).
Genetic studies reveal that SLE and PD share specific pathogenic genes and signaling pathways. For instance, polymorphisms in the Fcγ receptor gene play a crucial role in both diseases (Kobayashi et al., 2003; Kobayashi et al., 2007). Certain Fcγ receptor alleles in SLE patients are associated with more severe periodontal destruction, highlighting the importance of genetic susceptibility in the shared pathogenesis of these two diseases (Kobayashi et al., 2003). Additionally, Toll-like receptor (TLR) signaling pathways are abnormally activated in both SLE and PD, further suggesting an immunopathological link between them (Marques et al., 2016; Wallet et al., 2018). From a microbiological standpoint, immune dysfunction in SLE patients leads to an imbalance in the oral microbiome, facilitating the overgrowth of periodontal pathogens (Bagavant et al., 2018; Pessoa et al., 2019). Specifically, Porphyromonas gingivalis is believed to play a central role in the pathogenesis of both PD and SLE. Studies have shown that the abundance of this pathogen is significantly higher in the oral cavities of SLE patients and is correlated with the severity of periodontal tissue destruction (Graves et al., 2019). Furthermore, elevated levels of pro-inflammatory cytokines (such as TNF-α and IL-6) in SLE patients exacerbate periodontal inflammation, resulting in more severe damage to periodontal tissues (Martínez-García and Hernández-Lemus, 2021). The existing evidence strongly supports a close biological connection between SLE and PD. Further exploration of the shared mechanisms underlying these two diseases could not only enhance the understanding of their pathogenesis but also provide novel therapeutic approaches for their combined management.
In this study, we comprehensively analyzed gene expression datasets for both SLE and PD obtained from the GEO database. The identification of shared genes between SLE and PD was achieved through the detection of DEGs and WGCNA of the dataset. Subsequently, we conducted GO and KEGG analyses on the identified genes, followed by the construction of PPI networks. To substantiate our findings, we employed machine learning algorithms, specifically RF and LASSO, to ascertain common core genes possessing the utmost diagnostic significance in both afflictions. Finally, we conducted immune cell infiltration analysis to examine the differences in immune cell composition between SLE and PD patients, and assessed whether these differences correlate with the expression levels of shared core genes. Through this comprehensive bioinformatics analysis, we aim to offer new insights and perspectives for the clinical management of SLE concurrent with PD by enhancing our understanding of the biological connections between SLE and PD.
2 Materials and methods
2.1 Data download
The research process is illustrated in Figure 1. Data sets GSE72326, GSE16134, GSE81622, and GSE10334, relevant to SLE and PD, were obtained from the GEO database (https://www.ncbi.nlm.nih.gov/geo/) (Barrett et al., 2013). The GSE72326 dataset comprised 157 samples from individuals with SLE and 20 healthy controls. GSE16134 encompassed 241 samples from individuals with PD alongside 69 healthy controls. Additionally, we designated GSE81622 and GSE10334 as validation datasets, with GSE81622 comprising 30 SLE samples and 25 healthy controls, while GSE10334 included 183 samples from individuals with PD and 64 healthy controls.
To ensure consistency across the different platforms of these datasets, we performed several preprocessing steps. Initially, all gene identifiers were converted to gene symbols. Subsequently, we assessed the normality of the data distribution. For datasets exhibiting skewed distributions, a log2 transformation was applied to normalize the data. These steps were critical in minimizing platform-specific biases and ensuring the comparability of the datasets.
2.2 Differential gene expression analysis
We employed the R software package “Limma” to conduct an analysis of distinctions between the datasets GSE72326 and GSE16134. Differential gene screening was performed using thresholds of P. Value < 0.05 and |log2FC| > 0.5 (Lv et al., 2022). Volcano plots were generated using R software to visualize these DEGs. Additionally, the shared DEGs between SLE and PD were visualized using a Venn diagram.
2.3 WGCNA analysis
We conducted WGCNA on the datasets GSE72326 and GSE16134. The function “pickSoftThreshold” was utilized to screen gene modules (Xia et al., 2023). The top 25% of genes ranked by absolute median deviation were chosen for WGCNA. After eliminating missing values and outliers, we constructed the adjacency matrix using the soft thresholding method with a scale-free R^2 > 0.8 criterion. Subsequently, the adjacency matrix was transformed into a topological overlap matrix. Genes were clustered based on their topological overlap matrix using the average linkage hierarchical clustering method. We defined key modules requiring a minimum of 30 genes and a cut height of 0.25. Finally, Pearson correlation analysis was conducted to assess the relationship between these modules and disease. Core modules with the highest Pearson coefficients were then selected for further analysis.
2.4 Enrichment analysis and PPI network construction
After integrating the WGCNA results with DEGs, we conducted GO term annotation and KEGG pathway analysis using Metascape (http://metascape.org/gp/index.html#/main/step1). The GO enrichment analysis was categorized into three main domains: BP, CC, and MF. Additionally, KEGG pathway analysis was performed to identify significant biological pathways associated with DEGs. The visualization of GO and KEGG enrichment results was generated using the Bioinformatics Online Platform (http://www.bioinformatics.com.cn/).
We integrated WGCNA results with DEGs and uploaded them to the STRING database for PPI network construction. The required interaction score was set to >0.4. Subsequently, we visualized the results with Cytoscape software and identified the central gene using the CytoHubba plugin within Cytoscape (http://www.cytoscape.org).
2.5 Machine learning-based candidate gene selection
We applied the LASSO algorithm, a regression method for variable selection and predictive performance enhancement, along with the RF algorithm to identify hub genes. The analyses were conducted using the “glmnet” and “randomForest” packages in R (Díaz-Uriarte and Alvarez de Andrés, 2006; Garcia-Magariños et al., 2010). In the LASSO model, the regularization parameter (lambda) was optimized by selecting the value that minimized the mean cross-validation error, while the RF model was constructed with 1,000 decision trees. Subsequently, we performed ROC curve analysis using the “rms” package to evaluate the overlapping genes identified by both LASSO and RF. Genes with an AUC exceeding 0.6 were considered potential candidate biomarkers.
2.6 Construction and validation of line plots for candidate biomarker
In order to further validate the potential of the selected genes for diagnosis, we assessed the ROC curves of these overlapping genes using the GSE81622 and GSE10334 datasets. Genes with an Area Under the Curve (AUC) value exceeding 0.6 were considered to have significant diagnostic utility.
2.7 Immune cell infiltration analysis
We employed the CIBERSORT algorithm to evaluate the distribution of immune cells between disease and normal samples (Newman et al., 2015). Specifically, this study investigated the distribution of 22 immune cell types in samples from GSE72326 and GSE16134, with significance determined by p-values < 0.05. Additionally, Pearson correlation analysis was conducted between differentially coexpressed genes and immunoinfiltrating cells. Data visualization utilized the vioplot and pheatmap R packages.
3 Results
3.1 Analysis of differential gene expression
DEGs in datasets SLE GSE72326 and PD GSE16134 underwent analysis via the limma R package. Visualization via volcano plots depicted the DEGs. In the SLE dataset GSE72326, 444 DEGs were identified, comprising 302 genes showing upregulation and 142 genes exhibiting downregulation (Figure 2A). Dataset PD GSE16134 yielded 1,149 DEGs, comprising 688 genes showing upregulation and 461 genes displaying downregulation (Figure 2B). Moreover, 46 DEGs overlapped between GSE72326 and GSE16134 (Figure 2C).
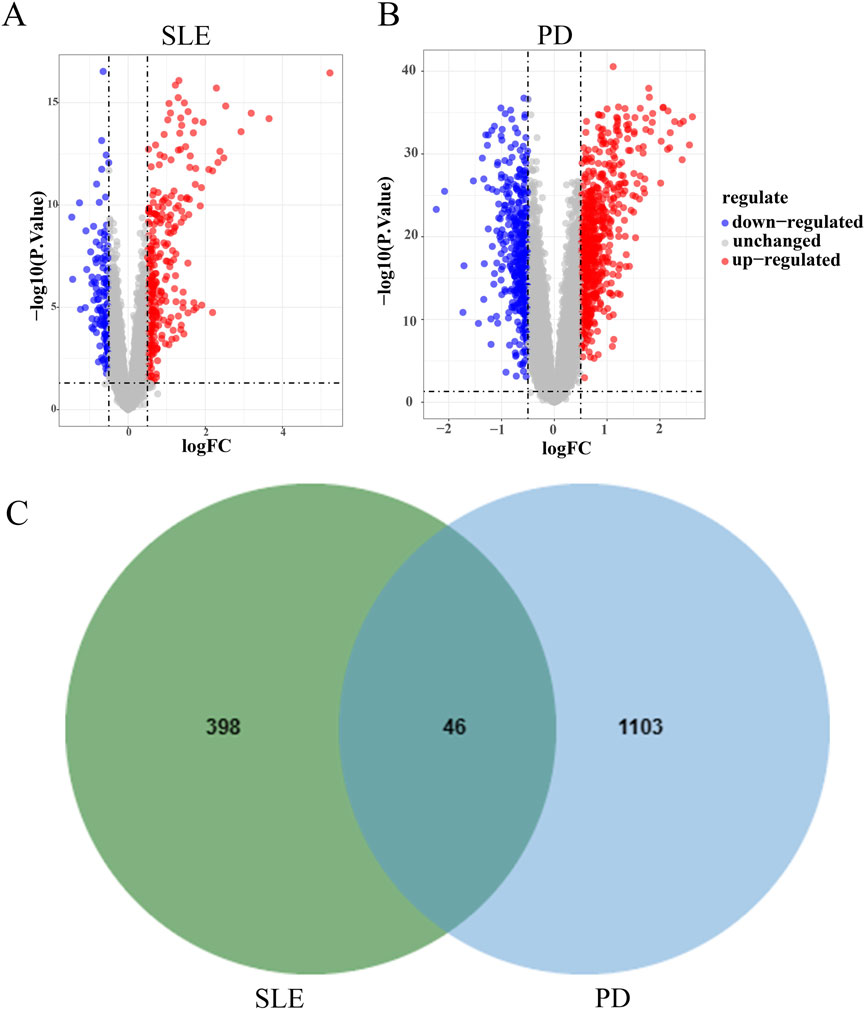
Figure 2. Analysis of differential gene expression. (A, B) Volcano plots illustrated the presence of DEGs in datasets GSE72326 and GSE16134. (C) Overlapping DEGs of GSE72326 and GSE16134.
3.2 WGCNA analysis
WGCNA analysis was conducted on the datasets GSE72326 and GSE16134, respectively. Optimal soft-thresholding values of 14 and 18 were determined for GSE72326 (Figure 3A) and GSE16134 (Figure 3B), respectively. GSE72326 revealed 10 modules, whereas GSE16134 identified 7 modules (Figures 3C, D). Subsequently, each module was assessed for its association with the disease. In the GSE72326 dataset, the pink module exhibited the strongest association with SLE (r = 0.36, p = 1e-06), comprising 38 genes (Figure 3E). In the analysis of PD using the GSE16134 dataset, the blue module demonstrated the most significant positive association (r = 0.61, p = 2e-32), containing 380 genes (Figure 3F). Nine genes overlapped between the positively correlated modules of SLE and PD (Figure 3G). Additionally, these genes are closely associated with the pathogenesis of both diseases, prompting further in-depth research.
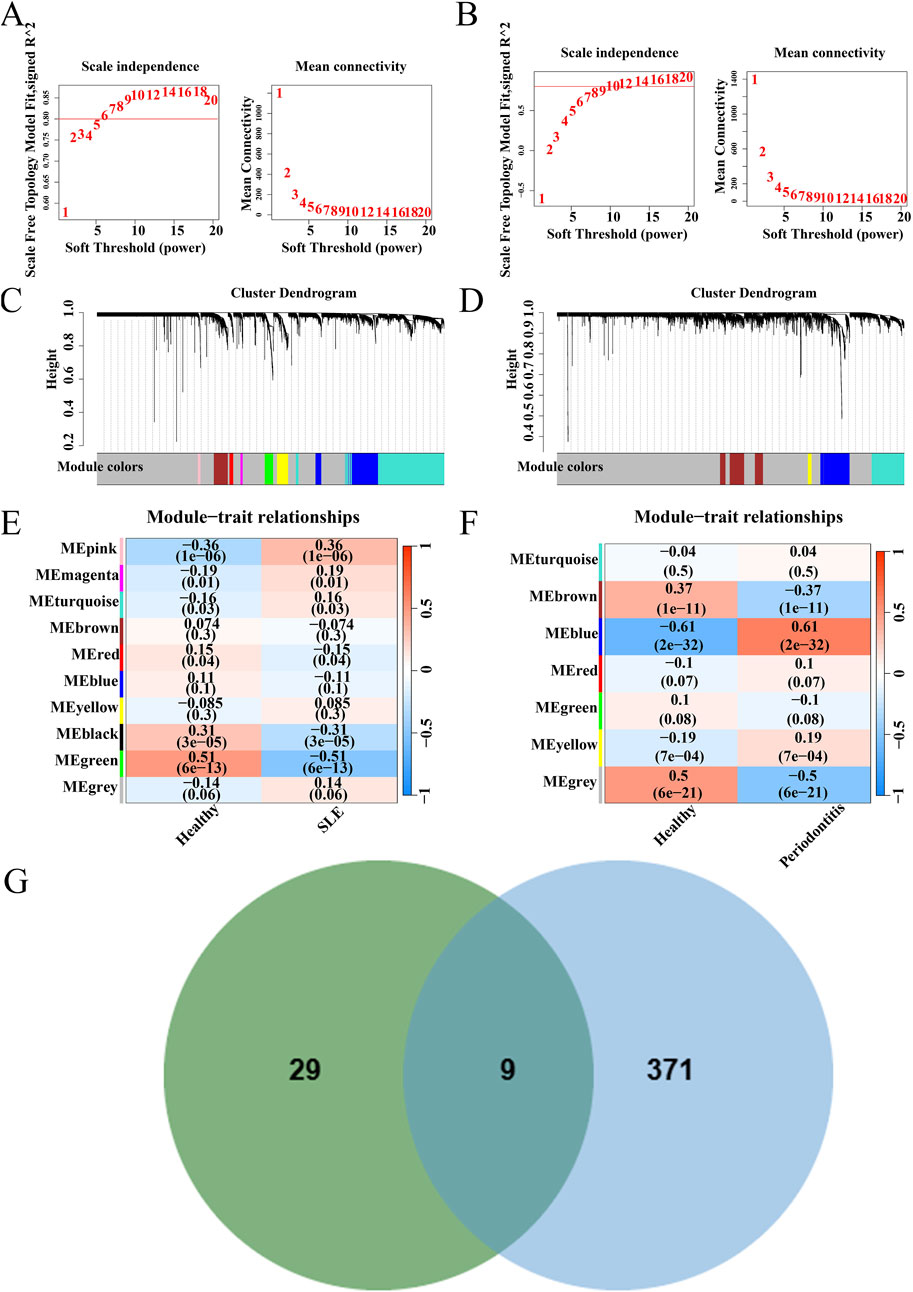
Figure 3. WGCNA of GSE72326 and GSE16134 datasets. (A) Network topology analysis results for dataset GSE72326. (B) Network topology analysis results for dataset GSE16134. (C) Gene modules and clustering results for dataset GSE72326. (D) Gene modules and clustering results for dataset GSE16134. (E, F) Heatmap depicting the correlation between module genes and diseases. (G) Genes overlapping in positively correlated modules.
3.3 Functional analysis of shared genes
Nine genes overlapped between the modules of SLE and PD, with 46 genes shared among the DEGs. DEGs and module genes were combined as candidate genes for further analysis. We obtained a total of 50 candidate genes from DEGs and module genomes, which underwent further analysis.
GO analysis demonstrated that BP were significantly associated with the upregulation of immune response, regulation of immune receptor signaling pathways, and immune signaling pathway regulation. MF were significantly associated with immune receptor activity, co-receptor activity, and cytokine receptor activity. CC was closely associated with the external side of the plasma membrane, membrane, lysosomal vacuole, and lysosome (Figure 4A).
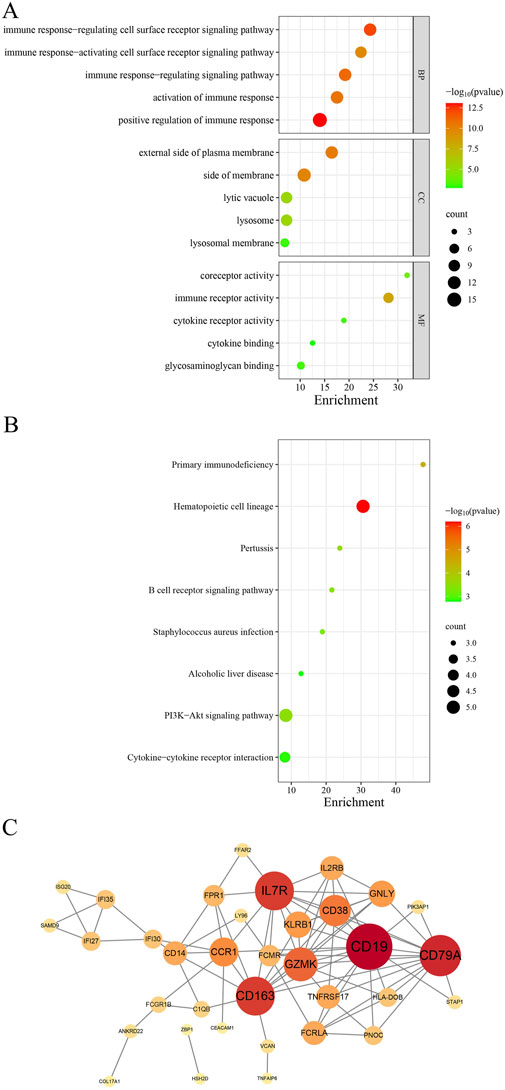
Figure 4. A comprehensive analysis of the shared genes was conducted. (A) GO enrichment analysis. (B) KEGG signaling pathway. (C) PPI network.
The KEGG pathway analysis revealed that these shared genes were predominantly enriched in primary immunodeficiency, the PI3K-Akt signaling pathway, and the B cell receptor signaling pathway (Figure 4B).
We imported these 50 genes into the STRING database to elucidate their interactions. The analysis yielded 85 nodes and 114 edges, with a combined score exceeding 0.4 (Figure 4C). The top five ranked genes were B lymphocyte antigen CD19 (CD19), B lymphocyte antigen CD79A (CD79A), Cluster of Differentiation 163 (CD163), Interleukin 7 receptor (IL7R), and Granzyme K (GZMK).
3.4 Identification of potential shared hub genes through machine learning
To further identify core genes with the highest diagnostic value, we conducted RF and LASSO regression analyses. From the 50 core genes, we selected the top 30 most significant genes for presentation (Figure 5A), with PLEKHA1, MFHAS1, and TMEM140 showing the highest MeanDecreaseGini values. Moreover, LASSO logistic regression identified 18 genes among the 50 core genes (Figures 5B, C). Upon intersecting the genes common to both the RF and LASSO analyses, we identified 11 hub genes shared between them (Figure 5D), which we deemed to hold significant diagnostic value. Subsequently, we used the new datasets GSE81622 and GSE10334 to evaluate the diagnostic performance of these hub genes using ROC curves (Table 1). We excluded three genes with AUC values below 0.6: FCGR1B (0.598), GZMK (0.5777), and IFI27 (0.4837). Among the remaining genes, PLEKHA1 (AUC = 0.882) and CEACAM1 (AUC = 0.788) had the highest AUC values, demonstrating a good ability to diagnose the risk of SLE with PD (Figures 5E, F).
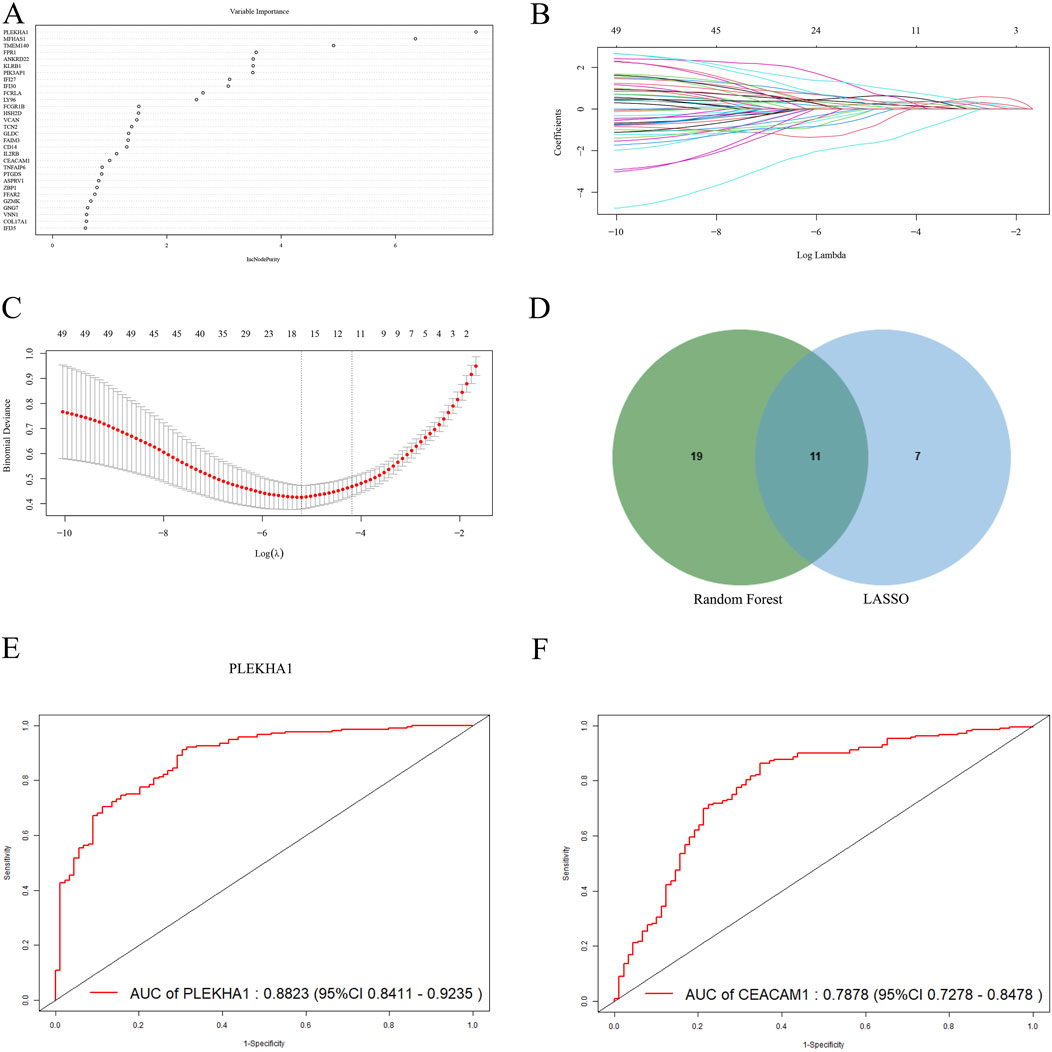
Figure 5. Screening and validating diagnostic biomarkers for SLE and PD. (A) The thirty most significant genes identified using the RF algorithm. (B, C) Cross-validation plot and coefficient contraction plot of LASSO regression analysis. (D) The intersecting genes selected by the RF and LASSO algorithms. (E, F) The ROC curves of PLEKHA1 and CEACAM1.
3.5 Immune infiltration analysis
Next, we used the CIBERSORTx method to analyze immune cell infiltration in the SLE dataset GSE72326 and the PD dataset GSE16134, assessing differences between the disease and healthy groups. The violin plot showed a marked rise in monocytes and neutrophils among SLE patients relative to controls, while CD4 memory-activated T cells and resting NK cells significantly decreased (Figure 6A). PD patients exhibited significant increases in plasma cells, γδ T cells, M0 macrophages, and resting NK cells, while helper T follicular cells and CD8+ T cells markedly decreased (Figure 6B). In addition, we examined the relationship between eight shared core genes and immune cell composition. Heatmap results demonstrated positive correlations between neutrophils, monocytes, and activated dendritic cells with CEACAM1, LY96, and TNFAIP6 in SLE, while showing negative correlations with GNG7 and PLEKHA1 (Figure 6C). In patients with PD, plasma cells and γδ T cells were positively correlated with GLDC, GNG7, LY96, TCN2, TNFAIP6, and VCAN, and negatively correlated with PLEKHA1 (Figure 6D).
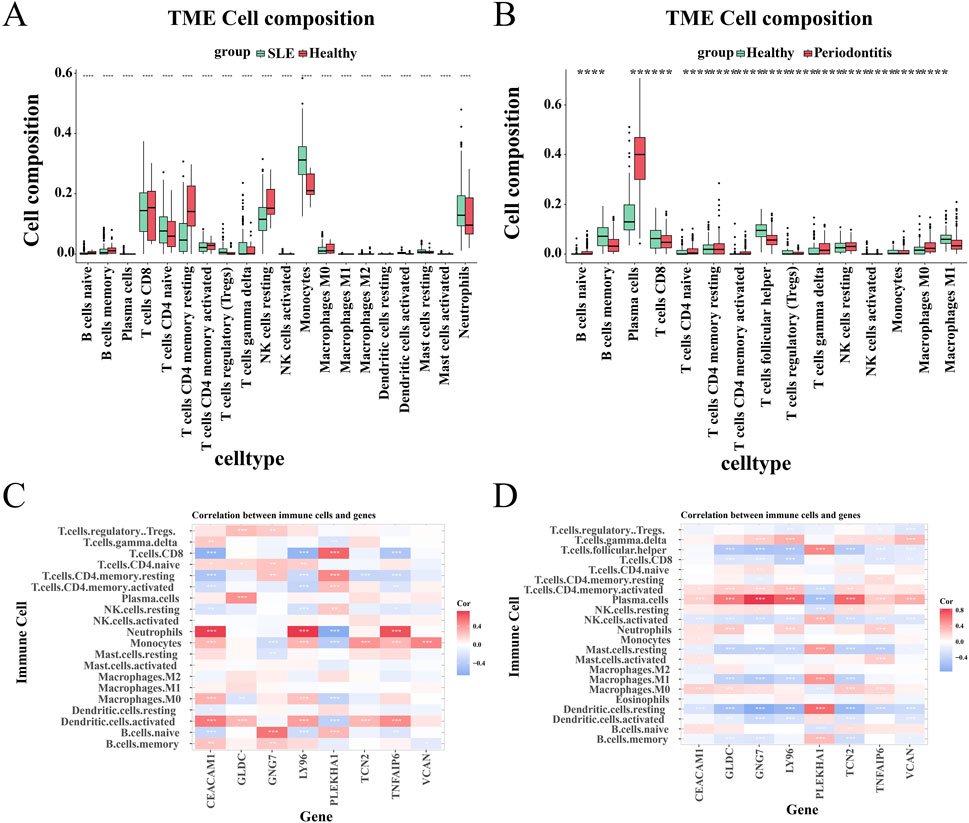
Figure 6. Analysis of immunoinfiltration. (A, B) The proportion of immune cells between patients with the disease and control groups. (C, D) The relationship between shared central genes and immune cells.
4 Discussion
SLE is a multifaceted autoimmune disorder, and its pathophysiological mechanisms remain incompletely understood (Hoi et al., 2024). PD is an infectious disease marked by an imbalance of microbial flora in periodontal tissues, resulting in chronic inflammation and gradual deterioration of periodontal supporting tissues. Recent studies indicate that individuals with SLE face an elevated risk of developing PD, with a risk approximately 1.76–1.78 times higher than the general population (Rutter-Locher et al., 2017; Bolstad et al., 2022). Furthermore, various biological hypotheses propose comparable pathophysiological mechanisms between these conditions, encompassing shared genetic, microbiological, immunological, and environmental risk factors (Sojod et al., 2021). Nevertheless, research investigating the genetic link between SLE and PD remains limited. Thus, the discovery of biomarkers and underlying molecular mechanisms pertaining to both SLE and PD via bioinformatics analysis holds significant clinical relevance.
This study utilized comprehensive bioinformatics analysis employing machine learning algorithms. Initially, we analyzed DEGs from the GSE72326 and GSE16134 datasets. Subsequently, DEGs were integrated with genes in the WGCNA module, leading to the identification of 50 candidate genes shared across the datasets. These 50 common candidate genes underwent enrichment analysis, revealing significant associations with the PI3K-Akt signaling pathway and B-cell receptor signaling pathway. Afterwards, we identified five core genes (CD19, CD79A, CD163, GZMK, IL7R) through PPI network analysis. To enhance the screening of diagnostic genes, machine learning algorithms were utilized to analyze overlapping genes. Ultimately, we identified 11 shared hub genes and validated them using ROC curves.
Our findings indicate a significant involvement of the PI3K-Akt signaling pathway in both the onset and progression of SLE and PD. It is crucial in regulating cell proliferation, differentiation, B cell and T cell receptor signaling, as well as macrophage polarization (Nestle et al., 2005; Dong et al., 2019; Linton et al., 2019; Chen et al., 2020). Similarly, the pathogenesis of SLE involves abnormal B cell activation, T cell dysregulation, and polarization of M1 macrophages (Rawlings et al., 2017; Ma et al., 2019; Tenbrock and Rauen, 2022). B-cell activating receptors such as BCR, CD40, and TLRs are all linked to the PI3K signaling pathway (Okkenhaug and Vanhaesebroeck, 2003; Donahue and Fruman, 2004; Hodson and Turner, 2009). In macrophages, the PI3K signaling pathway regulates the polarization state by modulating metabolic and inflammatory responses. Activation of this pathway suppresses M1-type macrophage polarization and enhances M2-type macrophage polarization (Long et al., 2021; Acosta-Martinez and Cabail, 2022). In clinical practice, focusing on the PI3K-Akt signaling pathway has also shown favorable therapeutic effects in patients with SLE (Shen et al., 2022). For instance, azithromycin can activate M2 macrophages via this pathway, thus reducing inflammation and improving the condition of SLE (Wang et al., 2018). The PI3K-Akt pathway plays a pivotal role in immune imbalance, inflammation regulation, and the maintenance of periodontal tissue homeostasis in PD. Studies have demonstrated that FBLN3 is upregulated in PD tissues, promoting M1 macrophage polarization via the EGFR/PI3K/AKT signaling pathway. This activation enhances the pro-inflammatory response and exacerbates periodontal tissue damage (Mu et al., 2024). Furthermore, PI3K-Akt modulates macrophage metabolism through HIF-1α-mediated glycolysis, sustaining M1 macrophage activation while suppressing M2 macrophage transition within the PD immune microenvironment (Zeng et al., 2024). Beyond immune cell polarization, PI3K-Akt plays a central role in the trained immunity of gingival fibroblasts (GFs). Porphyromonas gingivalis-derived lipopolysaccharide (LPS) has been shown to induce a trained immune response in GFs via PI3K/AKT pathway activation, leading to increased IL-6 and TNF-α secretion and sustaining inflammation through epigenetic modifications (Liu et al., 2024). Moreover, PI3K-Akt regulates osteoclast function, thereby influencing alveolar bone resorption and regeneration. Studies have identified the PI3K/AKT/GSK-3β signaling pathway as a key regulator of LPS-induced osteoclast activation and alveolar bone loss. Inhibiting this pathway has been found to reduce bone resorption, mitigate inflammation, and improve inflammatory bone loss (Lv et al., 2025). Additionally, PI3K-Akt may alleviate oxidative stress by enhancing cellular antioxidant capacity, thus contributing to the preservation of periodontal tissue homeostasis (Fu et al., 2023). In conclusion, the dysregulated activation of PI3K-Akt in PD not only influences immune cell polarization but also perpetuates inflammation and promotes alveolar bone loss by modulating trained immunity and bone metabolism. Given its critical role in both PD and immune dysregulation in SLE, PI3K-Akt represents a promising therapeutic target. Our findings further corroborate its pathological significance in PD and provide supporting evidence for its potential in targeted therapy.
In our research findings, hub genes are equally crucial for the clinical significance of both SLE and PD. We have identified eight key hub genes as diagnostic biomarkers (PLEKHA1, CEACAM1, TNFAIP6, TCN2, GLDC, GNG7, LY96, VCAN). Based on the current research status, we have focused on VCAN and LY96. While direct interactions between VCAN, LY96, and SLE remain undisclosed in current studies, their potential associations with SLE warrant attention. The VCAN gene encodes the Versican protein, a binding protein crucial for tissue morphogenesis and extracellular matrix formation, which is integral to tissue inflammation in response to infection and tissue damage (Wight et al., 2014). Versican exerts pro-inflammatory effects by modulating the adhesion of myeloid and lymphoid cells, particularly T lymphocytes and monocytes, which are regulated by multifunctional proteoglycans (Gill et al., 2010; Potter-Perigo et al., 2010; Evanko et al., 2012). Moreover, Versican interacts with inflammatory cells through two pathways: indirect binding to hyaluronic acid, and direct interaction with receptors like CD44, P-selectin glycoprotein ligand-1 (PSGL-1), and toll-like receptors (TLR), found on both immune and non-immune cell surfaces. These engagements activate signaling cascades that stimulate the synthesis and secretion of inflammatory cytokines, such as TNF-α, IL-6, and NF-κB (Wight et al., 2020). Notably, CD44 stands out as a significant therapeutic target in SLE, as it governs disease progression by modulating B cell activation and proliferation (Yi et al., 2023). Reduced expression of PSGL-1 in neutrophils of active SLE patients may lead to excessive release of Neutrophil Extracellular Traps (NETs). This excessive NETs release may exacerbate the disease by triggering an immune response through their DNA content, worsening inflammation and autoimmune reactions, thereby driving disease progression (Muñoz-Callejas et al., 2023). Additionally, the significance of VCAN in PD warrants attention. Expression levels of TLR4 are markedly elevated in PD patients, positively correlating with disease severity (Promsudthi et al., 2014). LY96, also known as myeloid differentiation factor-2 (MD-2), plays a crucial role in the innate immune response by serving as a ligand receptor tightly bound to (TLR4) (Wu et al., 2022b). TLR4 is involved in the pathogenesis of SLE by regulating the inflammatory response and promoting autoantibody production. Inhibiting TLR4 can alleviate inflammation and ameliorate kidney damage (Ma et al., 2018). Furthermore, TLR4 is implicated in immune response regulation during SLE pathogenesis through diverse pathways, encompassing immune cell activation, autophagy modulation, and interaction with type I IFN signaling pathway (Wu et al., 2022a). Thus, LY96 serves as the primary mediator in linking TLR4 with the association between SLE and PD. In summary, our findings suggest that VCAN and LY96 could represent novel therapeutic targets for both SLE and PD, thereby providing a robust groundwork for elucidating their underlying mechanisms in subsequent studies. Another important finding is that GNG7 demonstrates distinct correlations in SLE and PD, potentially influenced by variations in the immune microenvironment, signaling pathway regulation, and data sources. SLE is typically characterized by widespread immune dysregulation, whereas PD manifests as localized chronic inflammation. These contrasting immune landscapes may result in differences in the regulatory mechanisms of GNG7-mediated signaling pathways in the two diseases. Nevertheless, since CIBERSORTx immune infiltration analysis is applicable to various tissue environments, its findings remain biologically relevant to immune regulation, underscoring the need for further investigation into the underlying mechanisms. Future studies should employ single-cell transcriptomic analysis to elucidate the cell-type-specific roles of GNG7 and validate its regulatory mechanisms in immune cell function through in vitro experiments.
Additionally, alterations in immune cell populations appear to be pivotal in the interplay between SLE and PD. Our findings indicate that various immune cells are upregulated in SLE, including neutrophils and monocytes, while their expression is less pronounced in PD. Activated neutrophils are capable of generating a multitude of cytokines and chemokines, potentially leading to dysregulated functions of B and T cells, thereby exacerbating SLE pathogenesis (Smith and Kaplan, 2015). Aberrant monocyte activation in SLE stimulates dendritic cells and T/B cells through extensive interferon-alpha (IFN-α) and other inflammatory mediator secretion, culminating in autoantibody generation and tissue inflammation (Hirose et al., 2019). Conversely, In PD, substantial increases were observed in plasma cells, γδ T cells, M0 macrophages, and resting NK cells. The involvement of plasma cells is pivotal in the pathogenesis of PD, contributing to antibody production, immune response regulation, and inflammation-induced bone loss (Zouali, 2017). γδ T cells, abundant in epithelial tissues such as gingival tissues, may exert their functions in PD through diverse mechanisms, including immune surveillance, maintenance of immune homeostasis, and modulation of epithelial tissue repair processes (Figueredo et al., 2019). Notably, despite variations in the immune cell profiles upregulated in SLE and PD, there exists a close interconnection among these cells. Specifically, neutrophils support the survival and function of plasma cells by releasing B cell activating factor (BAFF) and directly interacting with them, thereby augmenting the immune response through enhanced plasma cell survival and antibody production (Zhao et al., 2019; Sabat et al., 2023). Additionally, γδ T cells modulate neutrophil activation and polarization by secreting IL-17, and encourage neutrophils to engage in suppressing T cell immune responses (Coffelt et al., 2015). In monocytes and γδ T cells, the v-γ9vδ2 + T cell subset within γδ T cells can activate monocytes by secreting inflammatory molecules like IFN-γ and TNF-α, inducing their adhesion and aggregation, and enhancing monocyte survival. Conversely, monocytes can induce the activation of Vγ9Vδ2+ T cells by accumulating and presenting phosphate antigens, thereby establishing a bidirectional regulatory interaction between these cell types (Chan et al., 2022). Monocytes critically contribute to the development and function of plasma cells by secreting factors vital for plasma cell survival and development, and by facilitating plasma cell proliferation through intercellular interactions (Mohr et al., 2009). The results of immune infiltration underscore the indispensable role of these immune cells in both SLE and PD.
5 Conclusion
In summary, our study clarifies the common molecular mechanisms underlying SLE and PD. We identified eight candidate genes (PLEKHA1, CEACAM1, TNFAIP6, TCN2, GLDC, GNG7, LY96, VCAN) as potential biomarkers for diagnosis. Analysis of immune infiltration demonstrated their intimate association with immune cells. Moreover, the PI3K-AKT signaling pathway likely plays a significant role in the interaction between SLE and PD. These discoveries establish a molecular basis for further investigating the relationship between SLE and PD.
Data availability statement
We confirm that the datasets can be found in the following online repositories. The names of the repositories and accession numbers are as follows: GSE72326: https://www.ncbi.nlm.nih.gov/geo/query/acc.cgi?acc=GSE72326, GSE16134: https://www.ncbi.nlm.nih.gov/geo/query/acc.cgi?acc=GSE16134, GSE81622: https://www.ncbi.nlm.nih.gov/geo/query/acc.cgi?acc=GSE81622, GSE10334: https://www.ncbi.nlm.nih.gov/geo/query/acc.cgi?acc=GSE10334.
Author contributions
KZ: Conceptualization, Data curation, Software, Writing – original draft. XL: Data curation, Methodology, Software, Writing – original draft. QZ: Investigation, Software, Writing – original draft. MZ: Investigation, Project administration, Supervision, Writing – review and editing. JH: Formal Analysis, Investigation, Project administration, Writing – review and editing. TZ: Funding acquisition, Writing – review and editing.
Funding
The author(s) declare that financial support was received for the research and/or publication of this article. This work was supported by the National Natural Science Foundation of China (Grant No. 82104798) Natural Science Foundation of Zhejiang Province (Grant No. ZCLMS25H2704) Zhejiang Chinese Medical University school-level research fund project – Natural Science Youth Exploration Project (Grant No. 2024JKZKTS20).
Conflict of interest
The authors declare that the research was conducted in the absence of any commercial or financial relationships that could be construed as a potential conflict of interest.
Generative AI statement
The author(s) declare that no Generative AI was used in the creation of this manuscript.
Publisher’s note
All claims expressed in this article are solely those of the authors and do not necessarily represent those of their affiliated organizations, or those of the publisher, the editors and the reviewers. Any product that may be evaluated in this article, or claim that may be made by its manufacturer, is not guaranteed or endorsed by the publisher.
References
Aceves-Avila, F. J., Chávez-López, M., Chavira-González, J. R., and Ramos-Remus, C. (2013). Temporomandibular joint dysfunction in various rheumatic diseases. Reumatismo 65, 126–130. doi:10.4081/reumatismo.2013.126
Acosta-Martinez, M., and Cabail, M. Z. (2022). The PI3K/akt pathway in meta-inflammation. Int. J. Mol. Sci. 23, 15330. doi:10.3390/ijms232315330
Akhil, A., Bansal, R., Anupam, K., Tandon, A., and Bhatnagar, A. (2023). Systemic lupus erythematosus: latest insight into etiopathogenesis. Rheumatol. Int. 43, 1381–1393. doi:10.1007/s00296-023-05346-x
Ameer, M. A., Chaudhry, H., Mushtaq, J., Khan, O. S., Babar, M., Hashim, T., et al. (2022). An overview of systemic lupus erythematosus (SLE) pathogenesis, classification, and management. Cureus 14, e30330. doi:10.7759/cureus.30330
Bagavant, H., Dunkleberger, M. L., Wolska, N., Rybakowska, P., Sroka, M., Rasmussen, A., et al. (2018). Periodontal pathogen exposure facilitates disease activity in Systemic Lupus Erythematosus. J. Immunol. 200 (45), 45.7–7. doi:10.4049/jimmunol.200.Supp.45.7
Baima, G., Massano, A., Squillace, E., Caviglia, G. P., Buduneli, N., Ribaldone, D. G., et al. (2022). Shared microbiological and immunological patterns in periodontitis and IBD: a scoping review. Oral Dis. 28, 1029–1041. doi:10.1111/odi.13843
Barrett, T., Wilhite, S. E., Ledoux, P., Evangelista, C., Kim, I. F., Tomashevsky, M., et al. (2013). NCBI GEO: archive for functional genomics data sets—update. Nucleic Acids Res. 41, D991–D995. doi:10.1093/nar/gks1193
Benli, M., Batool, F., Stutz, C., Petit, C., Jung, S., and Huck, O. (2021). Orofacial manifestations and dental management of systemic lupus erythematosus: a review. Oral Dis. 27, 151–167. doi:10.1111/odi.13271
Bolstad, A. I., Sehjpal, P., Lie, S. A., and Fevang, B.-T. S. (2022). Periodontitis in patients with systemic lupus erythematosus: a nationwide study of 1,990 patients. J. Periodontol. 93, 364–372. doi:10.1002/JPER.21-0181
Chan, K. F., Duarte, J. D. G., Ostrouska, S., and Behren, A. (2022). Γδ T cells in the tumor microenvironment-interactions with other immune cells. Front. Immunol. 13, 894315. doi:10.3389/fimmu.2022.894315
Chen, L., Ouyang, J., Wienand, K., Bojarczuk, K., Hao, Y., Chapuy, B., et al. (2020). CXCR4 upregulation is an indicator of sensitivity to B-cell receptor/PI3K blockade and a potential resistance mechanism in B-cell receptor-dependent diffuse large B-cell lymphomas. Haematologica 105, 1361–1368. doi:10.3324/haematol.2019.216218
Coffelt, S. B., Kersten, K., Doornebal, C. W., Weiden, J., Vrijland, K., Hau, C.-S., et al. (2015). IL-17-producing γδ T cells and neutrophils conspire to promote breast cancer metastasis. Nature 522, 345–348. doi:10.1038/nature14282
Corrêa, J. D., Branco, L. G. A., Calderaro, D. C., Mendonça, S. M. S., Travassos, D. V., Ferreira, G. A., et al. (2018). Impact of systemic lupus erythematosus on oral health-related quality of life. Lupus 27, 283–289. doi:10.1177/0961203317719147
Díaz-Uriarte, R., and Alvarez de Andrés, S. (2006). Gene selection and classification of microarray data using random forest. BMC Bioinforma. 7, 3. doi:10.1186/1471-2105-7-3
Donahue, A. C., and Fruman, D. A. (2004). PI3K signaling controls cell fate at many points in B lymphocyte development and activation. Semin. Cell Dev. Biol. 15, 183–197. doi:10.1016/j.semcdb.2003.12.024
Dong, S., Harrington, B. K., Hu, E. Y., Greene, J. T., Lehman, A. M., Tran, M., et al. (2019). PI3K p110δ inactivation antagonizes chronic lymphocytic leukemia and reverses T cell immune suppression. J. Clin. Invest. 129, 122–136. doi:10.1172/JCI99386
Evanko, S. P., Potter-Perigo, S., Bollyky, P. L., Nepom, G. T., and Wight, T. N. (2012). Hyaluronan and versican in the control of human T-lymphocyte adhesion and migration. Matrix Biol. 31, 90–100. doi:10.1016/j.matbio.2011.10.004
Fabbri, C., Fuller, R., Bonfá, E., Guedes, L. K. N., D’Alleva, P. S. R., and Borba, E. F. (2014). Periodontitis treatment improves systemic lupus erythematosus response to immunosuppressive therapy. Clin. Rheumatol. 33, 505–509. doi:10.1007/s10067-013-2473-2
Fava, A., and Petri, M. (2019). Systemic lupus erythematosus: diagnosis and clinical management. J. Autoimmun. 96, 1–13. doi:10.1016/j.jaut.2018.11.001
Figueredo, C. M., Lira-Junior, R., and Love, R. M. (2019). T and B Cells in periodontal disease: new functions in A complex scenario. Int. J. Mol. Sci. 20, 3949. doi:10.3390/ijms20163949
Fu, H., Sen, L., Zhang, F., Liu, S., Wang, M., Mi, H., et al. (2023). Mesenchymal stem cells-derived extracellular vesicles protect against oxidative stress-induced xenogeneic biological root injury via adaptive regulation of the PI3K/Akt/NRF2 pathway. J. Nanobiotechnology 21, 466. doi:10.1186/s12951-023-02214-5
Garcia-Magariños, M., Antoniadis, A., Cao, R., and Gonzãlez-Manteiga, W. (2010). Lasso logistic regression, GSoft and the cyclic coordinate descent algorithm: application to gene expression data. Stat. Appl. Genet. Mol. Biol. 9, Article30. doi:10.2202/1544-6115.1536
Gill, S., Wight, T. N., and Frevert, C. W. (2010). Proteoglycans: key regulators of pulmonary inflammation and the innate immune response to lung infection. Anat. Rec. Hob. 293, 968–981. doi:10.1002/ar.21094
Graves, D. T., Corrêa, J. D., and Silva, T. A. (2019). The oral microbiota is modified by systemic diseases. J. Dent. Res. 98, 148–156. doi:10.1177/0022034518805739
Hirose, S., Lin, Q., Ohtsuji, M., Nishimura, H., and Verbeek, J. S. (2019). Monocyte subsets involved in the development of systemic lupus erythematosus and rheumatoid arthritis. Int. Immunol. 31, 687–696. doi:10.1093/intimm/dxz036
Hodson, D. J., and Turner, M. (2009). The role of PI3K signalling in the B cell response to antigen. Adv. Exp. Med. Biol. 633, 43–53. doi:10.1007/978-0-387-79311-5_5
Hoi, A., Igel, T., Mok, C. C., and Arnaud, L. (2024). Systemic lupus erythematosus. Lancet 403, 2326–2338. doi:10.1016/S0140-6736(24)00398-2
Hussain, S. B., Leira, Y., Zehra, S. A., Botelho, J., Machado, V., Ciurtin, C., et al. (2022). Periodontitis and systemic lupus erythematosus: a systematic review and meta-analysis. J. Periodontal Res. 57, 1–10. doi:10.1111/jre.12936
Kobayashi, T., and Bartold, P. M. (2023). Periodontitis and periodontopathic bacteria as risk factors for rheumatoid arthritis: a review of the last 10 years. Jpn. Dent. Sci. Rev. 59, 263–272. doi:10.1016/j.jdsr.2023.08.002
Kobayashi, T., Ito, S., Yamamoto, K., Hasegawa, H., Sugita, N., Kuroda, T., et al. (2003). Risk of periodontitis in systemic lupus erythematosus is associated with Fcgamma receptor polymorphisms. J. Periodontol. 74, 378–384. doi:10.1902/jop.2003.74.3.378
Kobayashi, T., Ito, S., Yasuda, K., Kuroda, T., Yamamoto, K., Sugita, N., et al. (2007). The combined genotypes of stimulatory and inhibitory Fc gamma receptors associated with systemic lupus erythematosus and periodontitis in Japanese adults. J. Periodontol. 78, 467–474. doi:10.1902/jop.2007.060194
Linton, M. F., Moslehi, J. J., and Babaev, V. R. (2019). Akt signaling in macrophage polarization, survival, and atherosclerosis. Int. J. Mol. Sci. 20, 2703. doi:10.3390/ijms20112703
Liu, J., Tian, H., Ju, J., Nie, F, Yin, Q., Zhao, J., et al. (2024). Porphyromonas gingivalis-Lipopolysaccharide Induced Gingival Fibroblasts Trained Immunity Sustains Inflammation in Periodontitis. J Periodontal Res. 12, doi:10.1111/jre.13372
Long, H.-Z., Cheng, Y., Zhou, Z.-W., Luo, H.-Y., Wen, D.-D., and Gao, L.-C. (2021). PI3K/AKT signal pathway: a target of natural products in the prevention and treatment of alzheimer’s disease and Parkinson’s disease. Front. Pharmacol. 12, 648636. doi:10.3389/fphar.2021.648636
Loyola Rodriguez, J. P., Galvan Torres, L. J., Martinez Martinez, R. E., Abud Mendoza, C., Medina Solis, C. E., Ramos Coronel, S., et al. (2016). Frequency of dental caries in active and inactive systemic lupus erythematous patients: salivary and bacterial factors. Lupus 25, 1349–1356. doi:10.1177/0961203316640909
Lv, H., Luo, H., Tan, W., Zhong, J., Xiong, J., Liu, Z., et al. (2025). Kurarinone mitigates LPS-induced inflammatory osteolysis by inhibiting osteoclastogenesis through the reduction of ROS levels and suppression of the PI3K/AKT signaling pathway. Inflammation. doi:10.1007/s10753-025-02244-1
Lv, Y., Zhang, T., Cai, J., Huang, C., Zhan, S., and Liu, J. (2022). Bioinformatics and systems biology approach to identify the pathogenetic link of Long COVID and Myalgic Encephalomyelitis/Chronic Fatigue Syndrome. Front. Immunol. 13, 952987. doi:10.3389/fimmu.2022.952987
Ma, C., Xia, Y., Yang, Q., and Zhao, Y. (2019). The contribution of macrophages to systemic lupus erythematosus. Clin. Immunol. 207, 1–9. doi:10.1016/j.clim.2019.06.009
Ma, K., Li, J., Wang, X., Lin, X., Du, W., Yang, X., et al. (2018). TLR4+CXCR4+ plasma cells drive nephritis development in systemic lupus erythematosus. Ann. Rheum. Dis. 77, 1498–1506. doi:10.1136/annrheumdis-2018-213615
Marques, C. P. C., Maor, Y., de Andrade, M. S., Rodrigues, V. P., and Benatti, B. B. (2016). Possible evidence of systemic lupus erythematosus and periodontal disease association mediated by Toll-like receptors 2 and 4. Clin. Exp. Immunol. 183, 187–192. doi:10.1111/cei.12708
Martínez-García, M., and Hernández-Lemus, E. (2021). Periodontal inflammation and systemic diseases: an overview. Front. Physiol. 12, 709438. doi:10.3389/fphys.2021.709438
Mohr, E., Serre, K., Manz, R. A., Cunningham, A. F., Khan, M., Hardie, D. L., et al. (2009). Dendritic cells and monocyte/macrophages that create the IL-6/APRIL-rich lymph node microenvironments where plasmablasts mature. J. Immunol. 182, 2113–2123. doi:10.4049/jimmunol.0802771
Mu, H., Yang, B., Wang, Y., Wang, S., Yu, W., Jia, M., et al. (2024). Inhibition of fibulin-3 ameliorates periodontal inflammation through reducing M1 macrophage polarization via EGFR/PI3K/AKT pathway. J. Periodontol. doi:10.1002/JPER.24-0405
Muñoz-Callejas, A., González-Sánchez, E., Silván, J., San Antonio, E., González-Tajuelo, R., Ramos-Manzano, A., et al. (2023). Low P-selectin glycoprotein ligand-1 expression in neutrophils associates with disease activity and deregulated NET formation in systemic lupus erythematosus. Int. J. Mol. Sci. 24, 6144. doi:10.3390/ijms24076144
Nazir, M. A. (2017). Prevalence of periodontal disease, its association with systemic diseases and prevention. Int. J. Health Sci. (Qassim) 11, 72–80.
Nestle, F. O., Conrad, C., Tun-Kyi, A., Homey, B., Gombert, M., Boyman, O., et al. (2005). Plasmacytoid predendritic cells initiate psoriasis through interferon-alpha production. J. Exp. Med. 202, 135–143. doi:10.1084/jem.20050500
Newman, A. M., Liu, C. L., Green, M. R., Gentles, A. J., Feng, W., Xu, Y., et al. (2015). Robust enumeration of cell subsets from tissue expression profiles. Nat. Methods 12, 453–457. doi:10.1038/nmeth.3337
Okkenhaug, K., and Vanhaesebroeck, B. (2003). PI3K in lymphocyte development, differentiation and activation. Nat. Rev. Immunol. 3, 317–330. doi:10.1038/nri1056
Papapanou, P. N., Sanz, M., Buduneli, N., Dietrich, T., Feres, M., Fine, D. H., et al. (2018). Periodontitis: consensus report of workgroup 2 of the 2017 world workshop on the classification of periodontal and peri-implant diseases and conditions. J. Clin. Periodontol. 45 (Suppl. 20), S162–S170. doi:10.1111/jcpe.12946
Pessoa, L., Aleti, G., Choudhury, S., Nguyen, D., Yaskell, T., Zhang, Y., et al. (2019). Host-microbial interactions in systemic lupus erythematosus and periodontitis. Front. Immunol. 10, 2602. doi:10.3389/fimmu.2019.02602
Potter-Perigo, S., Johnson, P. Y., Evanko, S. P., Chan, C. K., Braun, K. R., Wilkinson, T. S., et al. (2010). Polyinosine-polycytidylic acid stimulates versican accumulation in the extracellular matrix promoting monocyte adhesion. Am. J. Respir. Cell Mol. Biol. 43, 109–120. doi:10.1165/rcmb.2009-0081OC
Promsudthi, A., Poomsawat, S., and Limsricharoen, W. (2014). The role of Toll-like receptor 2 and 4 in gingival tissues of chronic periodontitis subjects with type 2 diabetes. J. Periodontal Res. 49, 346–354. doi:10.1111/jre.12112
Rawlings, D. J., Metzler, G., Wray-Dutra, M., and Jackson, S. W. (2017). Altered B cell signalling in autoimmunity. Nat. Rev. Immunol. 17, 421–436. doi:10.1038/nri.2017.24
Rutter-Locher, Z., Smith, T. O., Giles, I., and Sofat, N. (2017). Association between systemic lupus erythematosus and periodontitis: a systematic review and meta-analysis. Front. Immunol. 8, 1295. doi:10.3389/fimmu.2017.01295
Sabat, R., Šimaitė, D., Gudjonsson, J. E., Brembach, T.-C., Witte, K., Krause, T., et al. (2023). Neutrophilic granulocyte-derived B-cell activating factor supports B cells in skin lesions in hidradenitis suppurativa. J. Allergy Clin. Immunol. 151, 1015–1026. doi:10.1016/j.jaci.2022.10.034
Sedghi, L. M., Bacino, M., and Kapila, Y. L. (2021). Periodontal disease: the good, the bad, and the unknown. Front. Cell Infect. Microbiol. 11, 766944. doi:10.3389/fcimb.2021.766944
Shen, M., Yu, H., Jin, Y., Mo, J., Sui, J., Qian, X., et al. (2022). Metformin facilitates osteoblastic differentiation and M2 macrophage polarization by PI3K/AKT/mTOR pathway in human umbilical cord mesenchymal stem cells. Stem Cells Int. 2022, 9498876. doi:10.1155/2022/9498876
Smith, C. K., and Kaplan, M. J. (2015). The role of neutrophils in the pathogenesis of systemic lupus erythematosus. Curr. Opin. Rheumatol. 27, 448–453. doi:10.1097/BOR.0000000000000197
Sojod, B., Pidorodeski Nagano, C., Garcia Lopez, G. M., Zalcberg, A., Dridi, S. M., and Anagnostou, F. (2021). Systemic lupus erythematosus and periodontal disease: a complex clinical and biological interplay. J. Clin. Med. 10, 1957. doi:10.3390/jcm10091957
Tenbrock, K., and Rauen, T. (2022). T cell dysregulation in SLE. Clin. Immunol. 239, 109031. doi:10.1016/j.clim.2022.109031
Tonetti, M. S., Greenwell, H., and Kornman, K. S. (2018). Staging and grading of periodontitis: framework and proposal of a new classification and case definition. J. Periodontol. 89 (Suppl. 1), S159–S172. doi:10.1002/JPER.18-0006
Wallet, S. M., Puri, V., and Gibson, F. C. (2018). Linkage of infection to adverse systemic complications: periodontal disease, toll-like receptors, and other pattern recognition systems. Vaccines (Basel) 6, 21. doi:10.3390/vaccines6020021
Wang, J., Xie, L., Wang, S., Lin, J., Liang, J., and Xu, J. (2018). Azithromycin promotes alternatively activated macrophage phenotype in systematic lupus erythematosus via PI3K/Akt signaling pathway. Cell Death Dis. 9, 1080. doi:10.1038/s41419-018-1097-5
Wight, T. N., Kang, I., Evanko, S. P., Harten, I. A., Chang, M. Y., Pearce, O. M. T., et al. (2020). Versican-A critical extracellular matrix regulator of immunity and inflammation. Front. Immunol. 11, 512. doi:10.3389/fimmu.2020.00512
Wight, T. N., Kang, I., and Merrilees, M. J. (2014). Versican and the control of inflammation. Matrix Biol. 35, 152–161. doi:10.1016/j.matbio.2014.01.015
Wu, J., Singh, K., Lin, A., Meadows, A. M., Wu, K., Shing, V., et al. (2022a). Boosting NAD+ blunts TLR4-induced type I IFN in control and systemic lupus erythematosus monocytes. J. Clin. Invest. 132, e139828. doi:10.1172/JCI139828
Wu, S., Lin, C., Zhang, T., Zhang, B., Jin, Y., Wang, H., et al. (2022b). Pentamidine alleviates inflammation and lipopolysaccharide-induced sepsis by inhibiting TLR4 activation via targeting MD2. Front. Pharmacol. 13, 835081. doi:10.3389/fphar.2022.835081
Xia, J., Hou, Y., Cai, A., Xu, Y., Yang, W., Huang, M., et al. (2023). An integrated co-expression network analysis reveals novel genetic biomarkers for immune cell infiltration in chronic kidney disease. Front. Immunol. 14, 1129524. doi:10.3389/fimmu.2023.1129524
Yi, P., Cao, P., Yang, M., Xiong, F., Jiang, J., Mei, Y., et al. (2023). Overexpressed CD44 is associated with B-cell activation via the HA-CD44-AIM2 pathway in lupus B cells. Clin. Immunol. 255, 109710. doi:10.1016/j.clim.2023.109710
Zeng, X., Li, T., Yang, K., Jiang, Y., Chen, S., Yang, S., et al. (2024). Natural compound phloretin restores periodontal immune homeostasis via HIF-1α-regulated PI3K/Akt and glycolysis in macrophages. Int. Immunopharmacol. 141, 112933. doi:10.1016/j.intimp.2024.112933
Zhao, Z., Luo, Z., Yang, Q., Chang, H., Liu, P., Li, Z., et al. (2019). Neutrophil-derived MRP14 supports plasma cell commitment and protects myeloma cells from apoptosis. J. Immunol. Res. 2019, 9561350. doi:10.1155/2019/9561350
Keywords: systemic lupus erythematosus, periodontitis, PI3K-Akt signaling pathway, VCAN, LY96
Citation: Zhao K, Li X, Zhu Q, Zhu M, Huang J and Zhao T (2025) The potential crosstalk genes and molecular mechanisms between systemic lupus erythematosus and periodontitis. Front. Genet. 16:1527713. doi: 10.3389/fgene.2025.1527713
Received: 14 November 2024; Accepted: 31 March 2025;
Published: 16 April 2025.
Edited by:
Ahmed Rebai, Centre of Biotechnology of Sfax, TunisiaReviewed by:
Archana Prabahar, Cleveland State University, United StatesWei Zhao, Virginia Commonwealth University, United States
Copyright © 2025 Zhao, Li, Zhu, Zhu, Huang and Zhao. This is an open-access article distributed under the terms of the Creative Commons Attribution License (CC BY). The use, distribution or reproduction in other forums is permitted, provided the original author(s) and the copyright owner(s) are credited and that the original publication in this journal is cited, in accordance with accepted academic practice. No use, distribution or reproduction is permitted which does not comply with these terms.
*Correspondence: Ting Zhao, emhhb3RpbmdAemNtdS5lZHUuY24=
†These authors have contributed equally to this work