- 1BGI Research, Chongqing, China
- 2BGI Research, Shenzhen, China
- 3College of Life Sciences, University of Chinese Academy of Sciences, Beijing, China
- 4Shenzhen Key Laboratory of Transomics Biotechnologies, BGI Research, Shenzhen, China
- 5Department of Neurology, The Eighth Affiliated Hospital, Sun Yat-Sen University, Shenzhen, China
- 6High Altitude Health Science Research Center, Tibet University, Lhasa, Tibet, China
- 7College of Life Sciences and Oceanography, Shenzhen University, Shenzhen, Guangdong, China
- 8School of Life Sciences, Lanzhou University, Lanzhou, Gansu, China
- 9College of Plant Protection, Hunan Agricultural University, Changsha, Hunan, China
- 10School of Biology and Biological Engineering, South China University of Technology, Guangzhou, China
- 11BGI Research, Wuhan, China
- 12State Key Laboratory of Genome and Multi-omics Technologies, BGI Research, Shenzhen, China
The hypoxia-ischemia (H-I) diseases share some common mechanisms which may help to delay the diseases’ processing. However, the shared features are still unclear due to the lack of large scale high-quality multi - omics data that specifically target the same disease, population, and tissues/cells. In this study, we developed a novel risk assessment method to analyze four H–I diseases including eclampsia/preeclampsia (PE), pulmonary arterial hypertension (PAH), high-altitude polycythemia (HAPC), and ischemic stroke (IS). A combined new evaluation score was designed to integrate evaluation information from genomics, transcriptomics, proteomics, and metabolomics in previous researches. Genes were then divided into different groups according to their risk assessment score. The most significant group (direct biomarkers) contained genes with direct evidence of association to H-I disease: PIEZO2 and HPGD (shared), TSIX and SAA1 (PAH - specific), GSTM1, DNTT, and IGKC (HAPC - specific), LEP, SERPINA3, and ARHGEF4 (PE - specific), CD3D, ITK, and RPL18A (IS - specific). The groups ‘Intermediate crucial biomarkers’ contained genes played important roles in H-I disease related biological processes: CXCL8 (shared), HBG2, GRIN2A, and FGFBP1 (PAH - specific), FAM111B (HAPC - specific), C12orf39 and SLAMF1 (PE - specific). The genes lacking disease-association evidence but with similar characteristics with the above two groups were considered as ‘potential minor-effect biomarkers’: are SRRM2 - AS1 (shared), ATP8A1 (PAH - specific), RXFP1 and HJURP (HAPC - specific), HIST1H1T (PE - specific). With the development of biological experiments, these intermediate crucial and potential minor-effect biomarkers may be proved to be direct biomarkers in the future. Therefore, these biomarkers may serve as an entry point for subsequent research and are of great significance.
1 Introduction
Hypoxic-ischemic (H-I) diseases arise from diverse etiologies and can be categorized into four primary types based on their core pathophysiological characteristics including environment exposure, special physiological stage, and cerebrovascular/cardiovascular conditions. Of which, environment-related disorders, such as high-altitude polycythemia (HAPC), are directly linked to specific environmental factors like hypoxic exposure. Similarly, physiological-stage-related conditions exemplified by eclampsia/preeclampsia (PE) are closely associated with physiological changes during particular life stages, such as pregnancy. Cerebrovascular H-I disorder-including several diseases such as ischemic stroke (IS), hypoxic-ischemic encephalopathy (HIE), and cerebral small vessel disease (CSVD), primarily involve impaired cerebral perfusion or local hypoxia. Cardiovascular H-I disorders, such as pulmonary arterial hypertension (PAH) and myocardial infarction (MI), are characterized by systemic or regional circulatory dysfunction that leads to tissue ischemia and hypoxia. These classifications reflect distinct mechanisms through which hypoxia and ischemia manifest across different organ systems and contexts. All these can lead to severe injuries such as cerebral palsy, brain damage, and even death since it may trigger massive cellular malfunction and cell death (Zhang Z. et al., 2018).
Many researches focused on the common mechanisms of these H-I diseases and found subtle causal relationships among them. Perinatal hypoxia, which was one of the key points of eclampsia, was shown to increase susceptibility to high-altitude polycythemia and attendant pulmonary vascular dysfunction (Julian et al., 2015). Besides, since preeclampsia is a pregnancy-specific disorder resulting in hypertension and multiorgan dysfunction, it was shown to be associated with a 2-fold increased risk in stroke as indicated by a meta-analysis based on 22 studies covering >6.4 million women (Zamudio, 2007). The development of PAH was speculated to be related to polycythemia vera in case reports (Nand and Orfei, 1994; Tachibana et al., 2017). It is possible that the heart and pulmonary vasculature are affected by myeloproliferative process more commonly than is realized. Besides, pulmonary hypertension is a known complication of myeloproliferative neoplasms (MPN) with estimated prevalence as high as 50%. Patients with polycythemia vera (PV) report a wide spectrum of symptoms that significantly overlap with those reported by patients with PAH. Yet, it is not known how PAH affects outcomes and survival in patients with PV(Gazda et al., 2024). Stroke is a major non-cardiac morbidity in patients with pulmonary hypertension as indicated by meta-analysis based on 14 studies including 32,523 participants (Shah et al., 2019). Ischemic stroke is considered as a presenting manifestation of polycythemia vera and validated in a meta-analysis (Burattini et al., 2022).
However, the common mechanisms among these diseases were not clear, especially on H-I level. Previous studies have demonstrated that the phosphatidylinositol-3 kinase (PI3K)/protein kinase B (AKT) signaling pathway, which regulates a wide range of cellular functions, is involved in the resistance response to H-I through the activation of proteins associated with survival and inactivation of apoptosis-associated proteins (Zhang Z. et al., 2018). This indicated that further investigation of biological functions in a systematic way may provide further insights of the potential targets for treating diseases accompanied by H-I.
We hypothesize that the observed variations among ischemic-hypoxic diseases may be attributed to differences in vascular perfusion patterns or circulatory mechanisms within the body. To investigate this hypothesis, we selected the four representative diseases mentioned above (PH, HPAC, IS, and PE) (Figure 1A) as model conditions for comparative analysis.
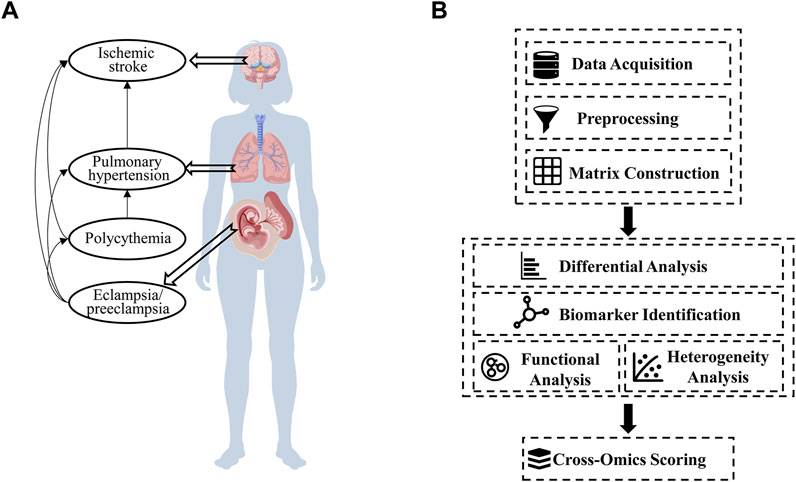
Figure 1. Hypothetical disease-network relationships and the construction of a cross-omics analysis pipeline. (A) A proposed network showing the interconnections among ischemic stroke, pulmonary hypertension, polycythemia, and eclampsia/preeclampsia (Created with BioGDP.com) (Jiang et al., 2025). (B) The steps of the cross-omics analysis pipeline, including data acquisition, preprocessing, differential analysis, biomarker identification, functional analysis, and cross-omics scoring.
Based on this, we constructed a new H-I disease profile by integrating of multiple transcriptomic datasets. Novel scores were designed for the risk assessment by incorporating intermediate and result data from other omics. This study provides an important theoretical basis for subsequent related research.
2 Materials and methods
This work was performed using the following pipeline shown in Figure 1B.
2.1 Public H-I datasets
A total of 15 publicly available hypoxia-ischemia (H-I) datasets were retrieved from the Gene Expression Omnibus (GEO) database (Edgar et al., 2002). These datasets include 397 cases, representing samples from four H-I disease groups, and 305 controls, representing samples from the corresponding control groups. The datasets encompass a wide range of experimental data, offering valuable insights into the molecular profiles of H-I diseases. The detailed characteristics of these datasets, including sample features and disease classifications, can be found in Table 1. These datasets provide a comprehensive resource for further investigation into the molecular mechanisms underlying H-I diseases.
2.2 Data normalization and statistical analyses
All the datasets were normalized using the following steps.
(1) Feature filter: genes with expression values of zero/missing in more than 75% of the samples were filtered to reduce the impact of low expression levels or extensive missing data.
(2) Missing data imputation: all the left missing values were imputed using zeros.
(3) Min-Max Normalization: each feature was normalized as follows:
where
(4) Disease profile construction: the datasets of a same disease were firstly merged by gene names, row-wise normalization was then performed to standardize the data scale. Different H-I disease datasets (without control) were merged using the same way and batch effect correction was applied using R package sva (Leek et al., 2012) (ComBat function). Outliers with expression values outside the 10th to 90th percentile range were excluded to enhance data quality and improve the accuracy of subsequent analyses.
(5) Differentially expressed gene (DEG) analyses: the expression differences between case and control groups for each gene were analyzed using Wilcoxon Rank-Sum Test. P-values were adjusted using false discovery rate (FDR). Fold change (FC) for each gene was calculated as the ratio of the median and mean expression level between case and control groups. In the analysis process, individual datasets used the median for FC calculation to reduce outlier sensitivity, while integrated datasets employed the mean to improve statistical stability across aggregated samples. All genes were categorized as follows: 1) Upregulated DEGs: genes with FC > 1 and FDR ≤0.05; 2) Downregulated DEGs: genes with FC < 1 and FDR ≤0.05; 3) Non-significant: genes with FC = 1 or FDR >0.05, indicating no significant expression difference between the case and control groups.
2.3 Functional analyses
(1) Enrichment analyses: DEGs were subjected to functional enrichment analyses using the clusterProfiler package (Yu et al., 2012) to investigate Gene Ontology (GO) and Kyoto Encyclopedia of Genes and Genomes (KEGG) pathways (Kanehisa and Goto, 2000). A p-value threshold of ≤0.05 was applied to identify significantly enriched GO terms and KEGG pathways, ensuring the statistical significance of the selected functional categories and pathways.
(2) GO term clustering: simplifyGOFromMultipleLists function from the simplifyEnrichment package (Gu and Hübschmann, 2023) was used to find clusters among significantly enriched GO BP terms (p-adj cutoff of 0.05) using org. Hs.eg.db as the annotation database. Clusters with p-adj less than 0.05 were kept.
2.4 Cross-omics evaluation risk scores
Six risk scores on different omics levels and one final score were designed to evaluate the roles each gene performed in each disease as follows.
2.4.1 ClinVar score ( )
This score is used to assess the potential significance of the association between a gene and a disease, based on the number of variants associated with each gene in the ClinVar database (Landrum et al., 2014). The score is calculated by applying a logarithmic transformation to the variant count (Score) followed by min-max normalization. The formula is as follows:
Where Score represents the number of variants in the gene. The logarithmic transformation,
2.4.2 pLI score ( )
pLI Score (
Where pLI is the raw value, representing the gene’s importance to biological function, and
2.4.3 Transcriptome score ( )
This score is used to assess the importance of a gene at the transcriptome level, calculated as:
In the differential expression analysis for each disease, a
2.4.4 Proteome Score ( )
Proteome Score is used to assess the importance of a gene’s corresponding protein in the protein-protein interaction network (PPIN) from STRING (Szklarczyk et al., 2023). The score is based on the degree of each protein in the interaction network, where the degree represents the number of interactions a protein has with other proteins. A higher degree indicates stronger connectivity within the network, suggesting that the protein may play a more crucial role in biological processes. The specific scoring formula is as follows:
2.4.5 Metabolome Score ( )
Metabolome Score (
Where the most frequent Pathway refers to the pathway that appears most frequently across all genes in the Pathway distribution, and the total number of matching genes refers to the total number of genes that can match any given Pathway. The score naturally falls within the range of [0, 1], with higher scores indicating that the gene may play a key role in the metabolic network, while low scores or a score of 0 suggest a weaker association with major pathways.
2.4.6 Tissue specificity score ( )
We employed the method proposed by Sevahn K. Vorperian et al. for cell lineage tracing of the samples, thereby obtaining the corresponding cell contribution scores (Vorperian et al., 2022). The score
The number of tissues where the gene exhibits significant specificity (
To normalize the metrics and ensure consistency across genes, the total score (
Additionally,
The combined tissue specificity score (
2.4.7 Final score ( )
The integrated final score (
In this formula,
The integrated score is then computed as the weighted sum of standardized scores from all omics layers:
2.4.8 Clustering of H-I profile
To explore the relationships between the four diseases based on
2.4.9 Biomarker type classification
All the biomarkers identified were classified into the following 3 types according to the literature search results: 1) direct biomarker: genes played a crucial role in at least one stage of the occurrence, development, and prognosis of a H-I disease; 2) intermediate crucial biomarkers: genes played a key role in a certain crucial biological process related to the occurrence, development, and prognosis of a H-I disease; 3) potential minor - effect biomarkers: genes that currently have no relevant research to confirm their association with the studied disease but possess similar characteristics to the above two types of biomarkers.
3 Results
3.1 Pan-ischemic hypoxic disease profiles construction
In this study, we analyzed multi-omics datasets from four H-I diseases. For pulmonary arterial hypertension (PAH), GSE254617 (Hong et al., 2024) and GSE168905 (Li et al., 2021) were included. The raw average number of detectable features was 20,962 ± 3,387, which decreased to 18,723 ± 220 after normalization. 3,544 ± 2,513 DEGs were identified across these datasets. For high-altitude polycythemia (HAPC), GSE29977 and GSE145802 (Tan and Meier-Abt, 2021) were analyzed. A significant difference was observed between these datasets (Figure 2), with the standard deviation of the raw feature counts in GSE145802 being 7,857, which is 2.3 times higher than that of GSE29977. This indicated substantial heterogeneity between datasets. For ischemic stroke (IS), GSE162955, GSE16561 (Barr et al., 2010), and GSE202709 (Kanki et al., 2023) were analyzed. The average number of detectable features before preprocessing was 21,305 ± 4,526, which was reduced to 18,565 ± 1,462 after preprocessing. The average number of DEGs identified across these datasets was 469 ± 384, reflecting a moderate degree of internal variability. For preeclampsia (PE), the data were derived from an integration of datasets from prior studies (Benton et al., 2018). Rigorous preprocessing and analysis of these datasets significantly improved data quality and consistency. Subsequent differential expression analysis of the preeclampsia dataset (comprising 14,651 genomic features) revealed 6,124 statistically significant differentially expressed genes (DEGs). Despite notable differences in the number of detectable features and DEGs across datasets for each disease, the comparison of internal standard deviations provided useful insights into the variability within and among datasets. This variability highlights the inherent heterogeneity and complexity of multi-omics data associated with different H-I diseases.
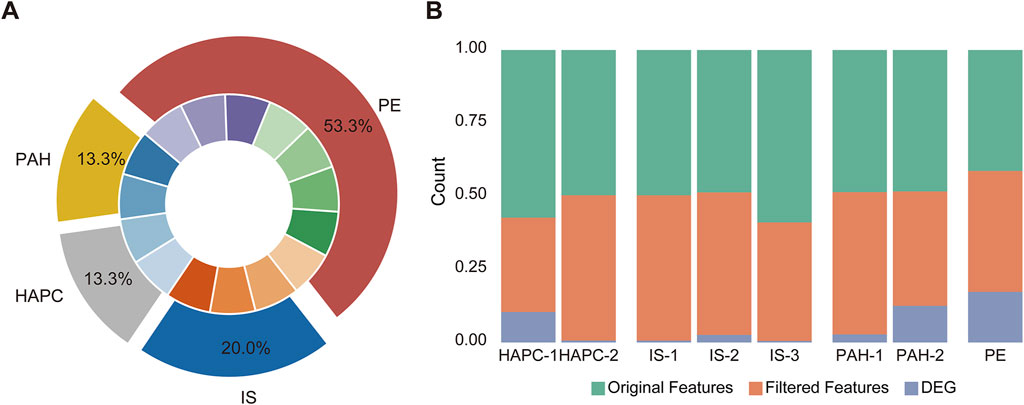
Figure 2. Data distribution and feature processing in the disease datasets. (A) The chart illustrates the distribution of diseases across corresponding datasets. The datasets for high-altitude polycythemia (HAPC) are labeled as HAPC-1 (GSE145802) and HAPC-2 (GSE29977), for ischemic stroke (IS) as IS-1 (GSE162955), IS-2 (GSE16561), and IS-3 (GSE202709), for pulmonary arterial hypertension (PAH) as PAH-1 (GSE168905) and PAH-2 (GSE254617), and for eclampsia/preeclampsia (PE) as a combined dataset from GSE75010 along with seven other datasets. (B) Feature processing analysis across the datasets. The stacked bar charts depict the proportions of original features, filtered features, and differentially expressed genes (DEGs) for each dataset, including HAPC (HAPC-1 and HAPC-2), IS (IS-1, IS-2, IS-3), PAH (PAH-1 and PAH-2), and PE. These datasets underwent feature filtering and DEG identification, with the processed data shown for each condition.
3.2 Shared mechanisms among H-I disease profile
We conducted a comprehensive gene scoring analysis across seven layers for H-I disease profile. This resulted in the construction of the HI-R-DP scoring matrix, consisting of 28 columns, where each column represents scores from a specific layer, and each row corresponds to a gene’s performance across these layers. Subsequently, we calculated the total
As shown in Figure 3A, the degree of gene sharing across different diseases varies greatly. The number of pan-disease genes is higher than other types indicating the shared mechanism among H-I diseases. For genes that received scores in all four diseases, the distribution of GF scores was shown in Figure 3B. The Kruskal-Wallis test revealed significant differences in
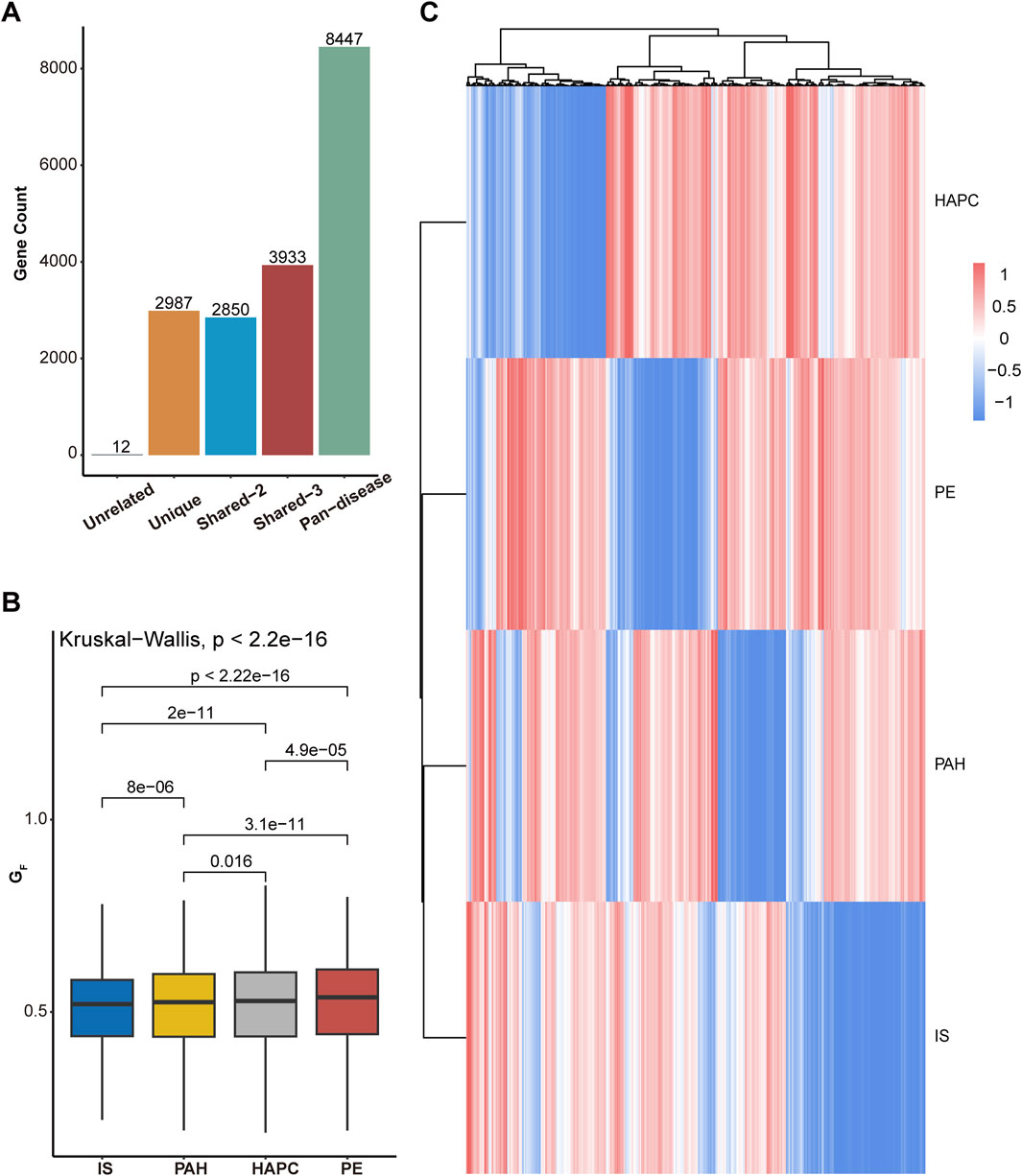
Figure 3. Cross-omics gene scoring and analysis across diseases. (A)Distribution of genes into five groups based on cross-omics scoring: “Unrelated” (genes not present in any disease), “Unique” (genes unique to a single disease), “Shared-2” (genes shared between two diseases), “Shared-3” (genes shared between three diseases), and “Pan-disease” (genes present in all diseases). The bar chart shows the number of genes in each group. (B) Differences in the total cross-omics scores across the four diseases: ischemic stroke (IS), pulmonary arterial hypertension (PAH), high-altitude polycythemia (HAPC), and eclampsia/preeclampsia (PE). The box plot illustrates the distribution of
To further explore the common characteristics of the disease spectrum, we selected genes present in at least three diseases and performed clustering analysis (Figure 3C; Supplementary Figure S1). The analysis revealed that IS and PAH share the most similar gene scoring patterns, suggesting a strong molecular regulatory connection between them. PE displayed a relatively independent gene profile, retaining pregnancy-specific vascular regulation and placental adaptation features, despite some association with IS and PAH. In contrast, HAPC exhibited a gene pattern distinct from the other diseases, likely influenced by mechanisms related to erythropoiesis, oxygen transport, and adaptation to high-altitude hypoxia. This clustering analysis highlights both the similarities and differences between diseases, helping to identify gene modules that may play common roles across multiple diseases. These findings provide new insights for future research on disease mechanisms and potential cross-disease therapeutic strategies.
The functional clustering analysis (Figure 4) revealed that genes in the Pan-disease group are widely involved in biological processes such as development and differentiation, substance transport, and cell cycle regulation. This suggests that these genes play crucial roles in maintaining tissue homeostasis, regulating cell proliferation, and adapting to metabolic changes. They may contribute to disease development by modulating vascular development, oxygen transport systems, and cellular adaptation to hypoxic environments. Notably, in ischemia- and hypoxia-related diseases, these genes are likely involved in vascular remodeling, erythropoiesis regulation, and hypoxia-induced signaling pathways, forming a core cross-disease regulatory network.
In contrast, genes in the Unique group are primarily enriched in development- and differentiation-related pathways, indicating their critical roles in tissue-specific angiogenesis, placental function regulation, and local hypoxia adaptation. Many of these genes are involved in stem cell differentiation, embryonic development, and tissue remodeling of specific organs, suggesting their unique functions in pathological conditions such as placental dysfunction, ischemic brain injury, or pulmonary vascular remodeling (see Supplementary Tables S1,S2 for details). The expression patterns of this gene set exhibit stronger tissue specificity across diseases, potentially influencing cellular adaptation and disease progression under local ischemic and hypoxic conditions.
Overall, genes in the Pan-disease group may act as core regulatory factors across multiple diseases, influencing various hypoxia-related pathological processes. In contrast, genes in the Unique group are more associated with disease-specific tissue adaptation mechanisms, particularly in vascular development, erythropoiesis, and local metabolic regulation.
To conduct an in-depth analysis of the source characteristics of mRNA, this study performed a traceability analysis on the data of HAPC and PAH since the two H-I diseases were shown to be in different clusters (Figure 5C). The top 5 tissues ranked by the cumulative number of all samples of each disease were selected as domain tissue/cell for analysis (results shown in Figures 5A,B). It was found that the mRNA of PAH mainly originated from club cell/type I pneumocyte, endothelial cell, adventitial cell, and type II pneumocyte, which was consistent with clinical knowledge. For HAPC samples, the cells were mostly derived from myeloid progenitor, hematopoietic stem cell, etc., also in line with clinical understanding. Among them, the common cell was basophil, and its correlation with the two diseases had been confirmed by previous studies (Ni et al., 2022; Yuen et al., 2025). Besides, the immune response following hypoxia - ischemia events in various diseases indicated its downstream events in H-I diseases (Eltzschig and Eckle, 2011; Albertsson et al., 2014).
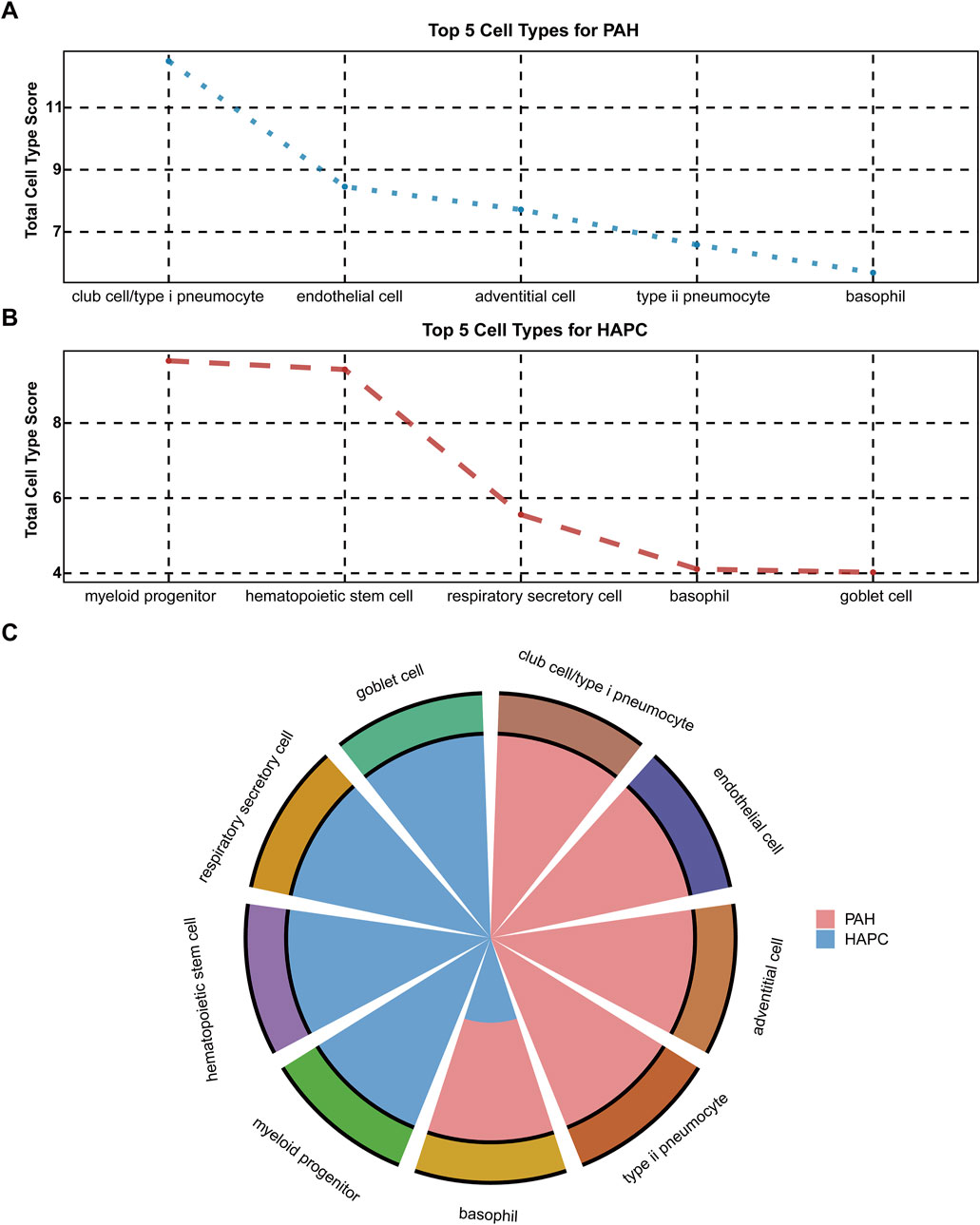
Figure 5. Cell type distribution for PAH and HAPC (A) Top 5 cell types for PAH based on total cell type scores (B) Top 5 cell types for HAPC based on total cell type scores (C) Comparison of cell type distribution for PAH (shown in red) and HAPC (shown in blue).
923 common genes of HAPC and PAH were identified as raw trans-biomarkers. After removing genes overlapping with those specific to IS, PE, and unique disease-specific genes, a final set of 495 trans-biomarkers (herein denoted as Bio-trans) was obtained. These genes showed differential expression in both HAPC and PAH but not in other diseases, suggesting their involvement in shared hypoxia-related molecular mechanisms. The top genes obtained were listed in Table 2 (Figure 6A). DSC2 was proven to be directly associated with myocardial function under the regulation of Cycloastragenol (Ren et al., 2020). Hypoxia - ischemia was considered as secondary injury in spinal cord injury (SCI) (Tator and Fehlings, 1991). The increased expression of Piezo2 was associated with poorer urodynamic parameters in SCI mice (Gotoh et al., 2022). Piezo2 played a key role in PAH, and its deficiency was shown to be associated with PAH by impairing NO synthesis and inducing EndMT (Tian et al., 2022; Wei et al., 2025). There were no relevant literature on polycythemia, so the results of this study suggested a possible relationship. The results of our study also showed upregulation, which was consistent. Downregulation of HPGD could improve the proliferation activity, reduce apoptosis, and enhance adhesion and angiogenesis in endothelial cells (ECs), thus promoting the occurrence and development of hypoxic pulmonary hypertension (He et al., 2023). The results of our study also showed downregulation, which was consistent. There was no previous research on its relationship with polycythemia, so our results suggested a possible relationship. CXCL8 was a promising biomarker of inflammation - sensitized hypoxia, as validated in an inflammation - sensitized hypoxia - ischemia model (Lingam et al., 2021). Currently, there were no studies on PRUNE2 and SRRM2-AS1 in the above - mentioned diseases, so these two genes could be potential new markers. The key themes across these genes highlight their roles in vascular remodeling, inflammation, and metabolic dysregulation. Of which, HPGD and PIEZO2 are central to vascular dysfunction, with HPGD downregulation driving angiogenesis and pulmonary hypertension via enhanced endothelial proliferation and reduced apoptosis, while PIEZO2 deficiency impairs NO synthesis and promotes EndMT, exacerbating PAH and SCI-related complications. CXCL8 emerges as a critical biomarker linking inflammation-sensitized hypoxia to tissue injury, as validated in ischemic models. Meanwhile, SRRM2-AS1 and PRUNE2 represent understudied candidates with potential relevance to vascular or metabolic disorders, warranting further investigation. HPGD’s angiogenic effects and PIEZO2’s disruption of NO signaling indirectly implicate these genes in redox imbalance. Collectively, these genes collectively underscore the interplay between vascular integrity, inflammation, and metabolic adaptation in diseases like PAH, SCI, and ischemia, with emerging roles for non-coding RNAs and novel targets in therapeutic strategies.
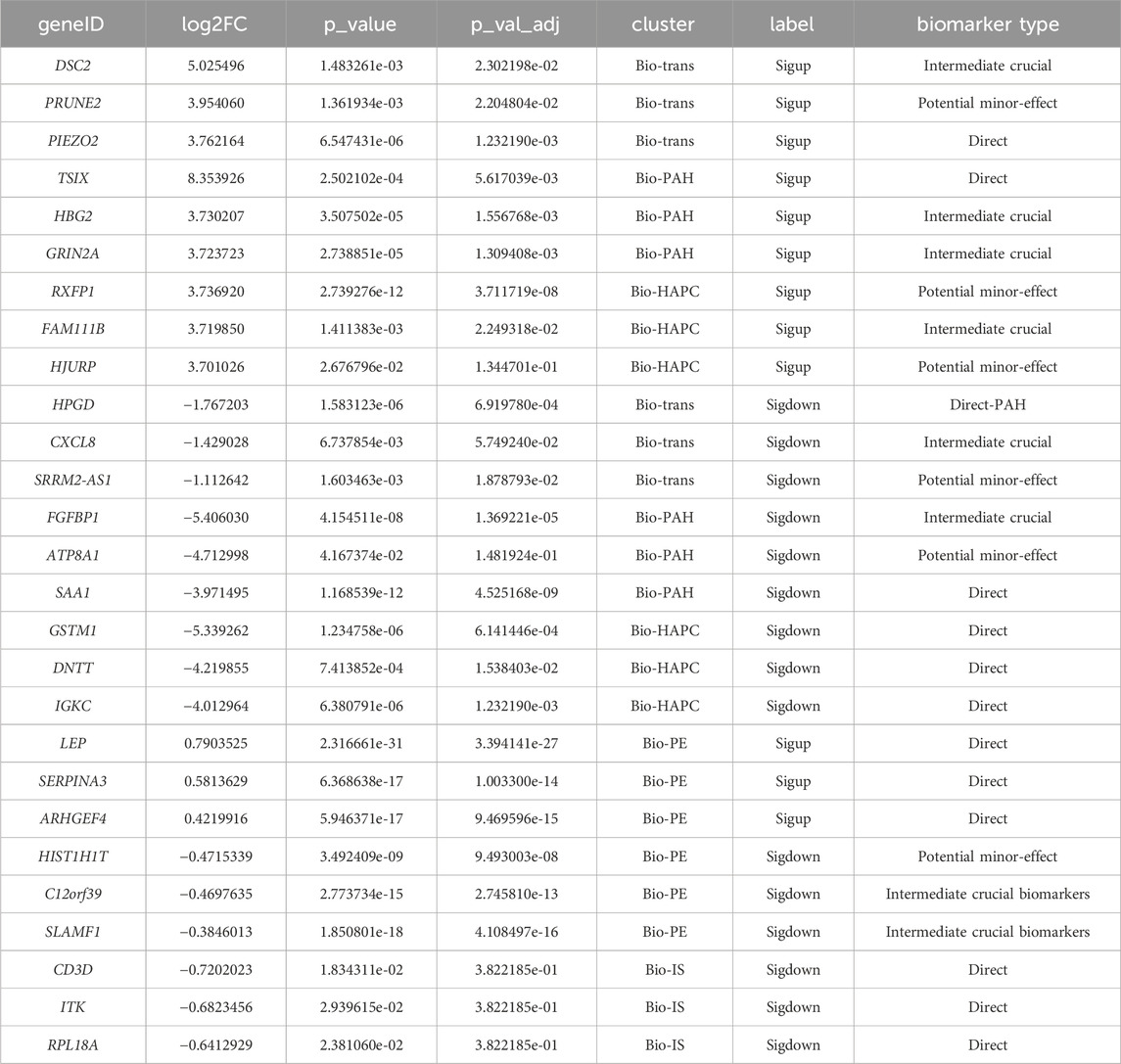
Table 2. Top 3 upregulated and downregulated differentially expressed genes in Bio-trans, Bio-HAPC, and Bio-PAH gene sets.
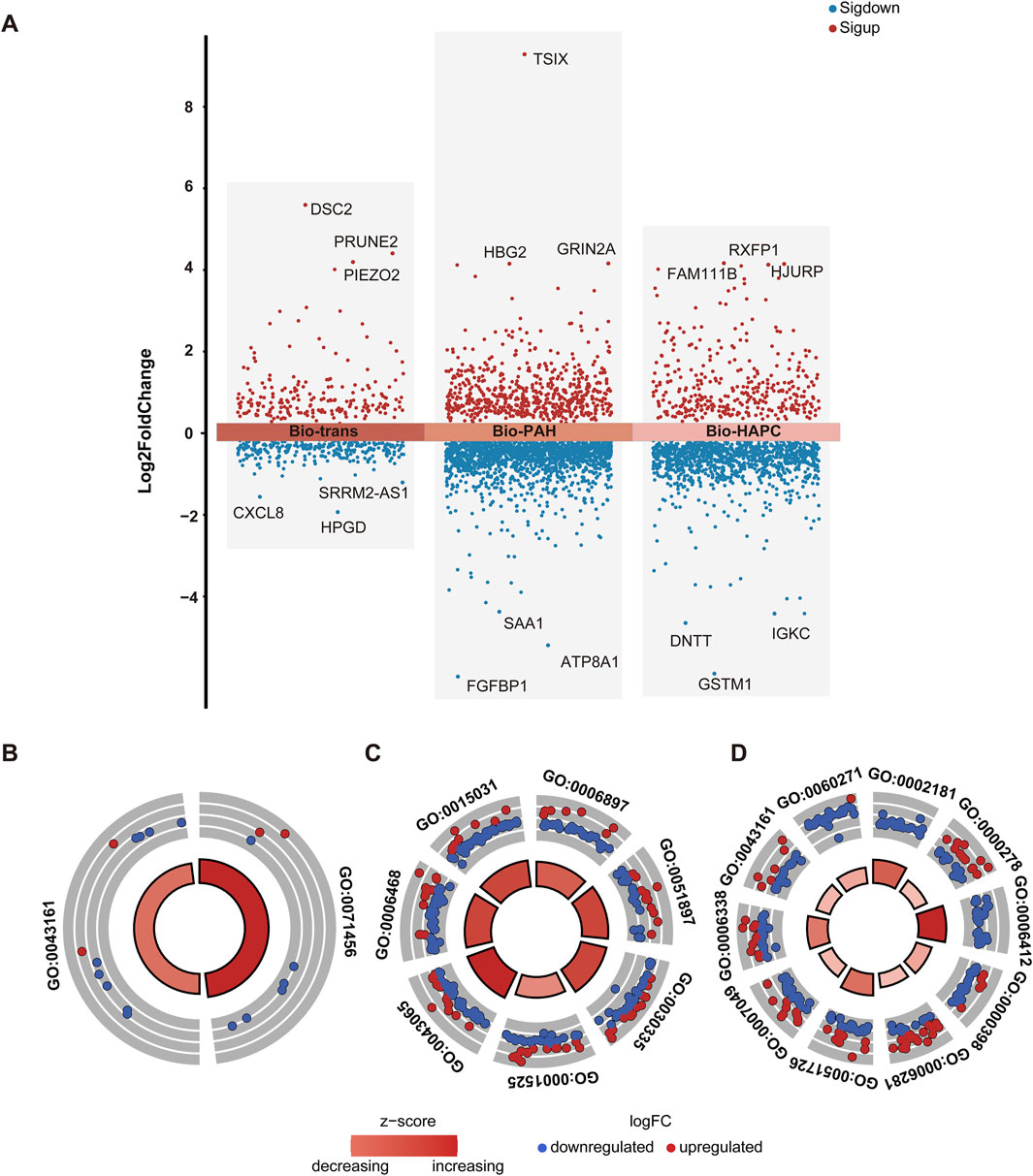
Figure 6. Differential gene distribution and enrichment analysis of Bio-trans, Bio-PAH, and Bio-HAPC gene sets (A) Volcano plot of differential gene expression for Bio-trans, Bio-PAH, and Bio-HAPC, with significant genes (p < 0.05) highlighted in red (upregulated) and blue (downregulated) (B) GO BP enrichment analysis for the Bio-trans gene set, shown in a circular plot (C) GO BP enrichment analysis for the Bio-PAH gene set (D) GO BP enrichment analysis for the Bio- HAPC gene set.
The expression profiles of Bio-trans genes were analyzed using the GTEx (Genotype-Tissue Expression) (GTEx Consortium, 2013) data to determine their cellular and tissue origins Analysis revealed distinct expression patterns across multiple genes. Of which, the HPGD gene was predominantly expressed in vascular endothelial cells of the lung (detected in cells: 9.20%) and alveolar macrophages (detected in cells: 17.95%). Similarly, CXCL8 exhibited primary expression in club epithelial cells of the lung (detected in cells: 3.18%) and alveolar macrophages (detected in cells: 2.05%). SRRM2-AS1 demonstrated tissue-specific expression in lymphatic endothelial cells of mammary tissue (detected in cells: 3.36%) and basal epithelial cells of the lung (detected in cells: 3.52%). Notably, DSC2 showed broad tissue distribution, with high expression in suprabasal epithelial cells of the esophageal mucosa (detected in cells: 40.52%), alveolar type I epithelial cells of the lung (detected in cells: 1.44%), and both cytoplasmic (detected in cells: 8.86%) and non-cytoplasmic (detected in cells: 7.98%) cardiac myocytes in the left ventricle. PRUNE2 was enriched in ciliated epithelial cells of the lung (detected in cells: 20.31%) and adipocytes of the left ventricular heart tissue (detected in cells: 23.32%). Strikingly, PIEZO2 displayed dominant expression in lung fibroblasts (detected in cells: 47.56%), with additional activity in lung lymphatic (detected in cells: 39.82%) and vascular endothelial cells (detected in cells: 7.13%).
GO biological process (BP) enrichment analysis of these trans - biomarkers identified two significantly enriched pathways: cellular response to hypoxia (GO:0071456) and proteasome-mediated ubiquitin-dependent protein catabolic process (GO:0043161) (Figure 6B). Hypoxia is a well - known trigger for PAH, with mitochondrial dysfunction and oxidative stress playing critical roles in its pathogenesis (Ahmed et al., 2024). Similarly, in polycythemia, hypoxia resulting from pulmonary vascular abnormalities may lead to disease progression (Lertzman et al., 1964). Alterations in the ubiquitin-proteasome system (UPS) due to hypoxia have been implicated in PAH (Wade et al., 2018), and dysfunction of the UPS has also been linked to abnormal red blood cell production in polycythemia (Meyer et al., 2007). Collectively, these findings suggest that hypoxia - related molecular mechanisms underlie both PAH and polycythemia.
3.3 Disease-specific biomarkers and function
3.3.1 PAH - Specific biomarkers
For PAH-specific biomarkers (Bio-PAH), 2,242 genes were obtained after excluding genes overlapping with those of HAPC, IS, and PE. The top genes obtained were listed in Table 2 (Figure 6A). These genes were divided into the following types Knockdown of TSIX could improve functional recovery and attenuate the inflammation response and cell apoptosis via the miR - 30a/SOCS3 axis (Pan et al., 2024). It could also aggravate spinal cord injury (SCI) by regulating the PI3K/AKT pathway via the miR-532-3p/DDOST axis (Dong et al., 2023). Besides, upregulation of TSIX could partially explain the sexual dimorphism of female pulmonary artery endothelial cells (ECs). This is consistent with our results, as this gene was also upregulated in our study (Carman et al., 2024). Regarding HBG2, although it is related to hypoxia, no direct research on its association with PAH has been found. Hypoxia can induce the transcription of γ - globin genes, including HBG2, by stabilizing HIF1α, enabling the HIF1α - HIF1β heterodimers to bind to the DNA elements of the BGLT3 gene downstream of HBG2 (Feng R. et al., 2022). GRIN2A is related to myocardial infarction, yet no direct research on its connection with PAH has been reported. GRIN2A was considered a candidate biomarker of acute myocardial infarction (Wu et al., 2022), which is closely related to PAH (Møller et al., 2005). Pathway analysis shows an association between FGFBP1 and hypertension (Tomaszewski et al., 2011). However, in this previous study, FGFBP1 was upregulated, while in our study, it was downregulated. This difference might be attributed to the distinction between hypertension and pulmonary hypertension. SAA1 was shown to be related to the pathogenesis of idiopathic pulmonary arterial hypertension, regardless of sex differences (Xu et al., 2021). Currently, no research on the relationship between ATP8A1 and pulmonary hypertension has been found. Given its minor effect, we speculate that this gene may function jointly with other genes.
GO BP enrichment analysis identified seven significantly enriched pathways (Figure 6C). These pathways include endocytosis (GO:0006897), positive regulation of the PI3K/Akt signaling pathway (GO:0051897), angiogenesis (GO:0001525), positive regulation of cell migration (GO:0030335), positive regulation of the apoptotic process (GO:0043065), protein transport (GO:0015031), and protein phosphorylation (GO:0006468). Disrupted endocytosis contributes to PAH progression by affecting surface protein internalization and trafficking (Chichger et al., 2019). The PI3K/Akt signaling pathway has been implicated in hypoxic PAH, with studies showing that improvements in this pathway can mitigate endothelial and mitochondrial dysfunction (Shi et al., 2023). Vascular remodeling, driven by angiogenesis, cell migration, and apoptosis, is a hallmark of PAH and involves processes such as cell hypertrophy, proliferation, and migration (Tajsic and Morrell, 2011). Additionally, protein phosphorylation plays an essential role in cellular signaling, with alterations in phosphorylation pathways contributing to PAH pathogenesis (Zhang J. et al., 2018; Li et al., 2024).
3.3.2 HAPC-specific biomarkers
For HAPC-specific biomarkers (Bio-HAPC), 1,425 genes were obtained after excluding genes overlapping with those of PAH, IS, and PE. The top genes obtained were listed in Table 2. A significant reduction in RXFP1 expression was observed in the ischemic myocardium (Gao et al., 2019). Mutations in the FAM111B gene may predict the severity of pulmonary fibrosis and a poor prognosis (Arowolo et al., 2022). Pulmonary fibrosis may be one of the causes of polycythemia (Ghosh et al., 2021). In the Jordanian population, the GSTM1 null genotype alone and in combination with the CYP1A1 m1 genotype may be predisposing risk factors for polycythemia vera (Naffa et al., 2012). DNTT was found to be downregulated in polycythemia vera and considered as a diagnostic marker (Baumeister et al., 2021). IGKC was downregulated in polycythemia vera (Gangaraju et al., 2020). Currently, there is no research on the relationship between HJURP and polycythemia.
GO BP enrichment analysis revealed 10 significantly enriched pathways, all related to the cell cycle (Figure 6D). These findings reflect the hyperproliferative characteristics of polycythemia, highlighting the role of cell cycle regulation in the development and progression of the disease (Lertzman et al., 1964). Dysregulation of the cell cycle may drive excessive red blood cell production, contributing to the pathological mechanisms underlying HAPC.
3.3.3 PE-specific biomarkers
For PE-specific biomarkers (Bio-PE), 3496 genes were obtained after excluding genes overlapping with those of PAH, HAPC, and IS. The top genes obtained were listed in Table 2 (Figure 6A). LEP and ARHGEF4, involved in metabolic and hypoxia/angiogenesis pathways, are shown to be upregulated (in accordance with our result) in placentas from severe preeclampsia (sPE) patients across ancestries, suggesting its contribution to the pathophysiology of preeclampsia (Aisagbonhi et al., 2023). The SERPINA3 gene is identified as a key diagnostic biomarker for preeclampsia, showing differential expression in affected placentas and associations with immune cell infiltration (Yang et al., 2022).
The HIST1H1T gene plays a critical role in sperm maturation by facilitating histone-to-protamine replacement in spermatocytes, and its disruption in double-knockout mouse models synergizes with other genes (e.g., Mcsp) to impair sperm function (e.g., morphology, motility, fertilization) and severely compromise fertility, indirectly impacting embryonic development by disrupting successful fertilization (Nayernia et al., 2003). The C12orf39 gene exhibits differential expression in placental tissue from mothers with antenatal depression and those using antidepressants during pregnancy, suggesting its potential role in placental dysfunction associated with maternal mental health or pharmacological exposure (Olivier et al., 2014). The SLAMF1 gene is downregulated in placental microvascular endothelial cells from severe intrauterine growth restriction (IUGR) cases compared to controls, and its expression differences are confirmed in placental tissue microarray analyses, suggesting its role in vascular dysfunction in pregnancy complications like PE (Dunk et al., 2012).
3.3.4 IS-specific biomarkers
For IS-specific biomarkers (Bio-IS), 405 genes were obtained after excluding genes overlapping with those of PAH, HAPC, and PE. The top genes obtained were listed in Table 2. CD3D, ITK were considered to be IS biomarkers by former researches (Feng S. et al., 2022; Wei et al., 2023; Wang et al., 2024). The RPL18 gene is a key mediator in stroke pathophysiology, as its dysregulation is linked to cerebral ischemia, and its restoration via traditional medicines (e.g., BYHW, NXT, YYTN) improves outcomes by modulating the gut microbiota-brain axis and suppressing neuroinflammation (microglia/astrocyte hyperactivation) (Yin et al., 2022).
4 Discussion
In this study, we devised a novel risk assessment method to infer the shared features among four H-I diseases in the presence of heterogenous omics data. Traditional bioinformatics analyses were performed to construct a H-I disease profile based on transcriptomic data since mRNAs responded promptly to abnormal physiological states in the human body and were easy to detect. Considering the lack of large scale high-quality multi - omics data that specifically target the same disease, population, and tissues, we exploited several public databases/datasets to design various risk assessment scores. A final new evaluation score was designed to integrate evaluation information from genomics, proteomics, and metabolomics in previous researches. The similarity and differences among these H-I diseases were then analyzed on both feature molecules and functional levels.
We divided all the genes in pan disease profile into groups. The ‘pan-disease genes’ were shown to play a central role in the occurrence and development of multiple diseases. Specifically, they may influence the pathological processes of these diseases by regulating some common biological processes, such as angiogenesis, hypoxic response, and cell proliferation. Further analysis may reveal the common regulatory roles of these genes in different diseases and provide clues for cross-disease biomarkers. Compared with this, unique group genes are crucial for disease - specific tissue adaptation, especially in vascular and metabolic aspects, which advances our understanding of disease genetic mechanisms.
The four diseases investigated in this study present distinct clinical manifestations. However, at the molecular level, all of them are associated with ischemia or hypoxia. Besides, these features may also be explained from the body’s circulatory system. IS is a typical systemic circulation disease. It occurs when there is an obstruction in the blood vessels of the brain, which are part of the systemic circulation network. The lack of blood supply to the brain tissue due to blockages in arteries like the carotid artery or its branches leads to ischemic injury. The pathophysiological mechanisms involve factors such as thrombosis formation, embolism, and atherosclerotic plaque rupture within the systemic arterial system, with little direct connection to the pulmonary circulation in its primary etiology. PE is a condition unique to pregnancy, mainly affects the systemic circulation. It is characterized by systemic small - vessel vasospasm, endothelial cell injury, and subsequent organ dysfunction. The placenta, which is part of the maternal - fetal circulatory system (a specialized part of the systemic circulation during pregnancy), plays a crucial role. The abnormal placentation and reduced placental perfusion can trigger a cascade of systemic responses, leading to hypertension, proteinuria, and potential involvement of multiple organs such as the kidneys, liver, and heart. In severe cases, PE can also impact the pulmonary circulation, causing pulmonary edema, indicating its complex relationship with both circulatory systems. HAPC is closely related to the pulmonary circulation. In individuals with HAPC, the body’s adaptation to the hypoxic environment at high altitudes leads to an increase in red blood cell production. This process is mainly regulated within the context of the pulmonary circulation as the lungs are the primary organs sensing the low - oxygen condition. The subsequent elevation in hematocrit aims to enhance oxygen - carrying capacity, but it also brings about changes in the pulmonary vascular bed, such as increased blood viscosity, which may affect pulmonary hemodynamics. PAH has a complex relationship with both the systemic and pulmonary circulations. Initially, it is considered a pulmonary circulation disorder, where abnormal remodeling of the pulmonary arteries occurs, leading to increased pulmonary vascular resistance. This results in elevated pulmonary arterial pressure and impaired right - heart function. However, systemic factors cannot be ignored, which is similar to that of IS. For example, systemic inflammatory cytokines can be released into the bloodstream and reach the pulmonary vasculature, promoting endothelial dysfunction and smooth muscle cell proliferation in the pulmonary arteries. This was also validated in this study (See Figure 3C for details).
In our results, we found PAH and HAPC were more similar to the other two diseases which may be cause partly due to their involvement in pulmonary circulation especially in hypoxic response and vascular regulation. The significant differences between PAH and PE, IS validated that the differences of these diseases may be caused by the interactions of human body systems based on circulation systems. As a pregnancy-related disease, PE may be influenced by unique vascular regulatory mechanisms during pregnancy. IS, on the other hand, involves ischemic injury to the nervous system, fundamentally different from the molecular mechanisms of PAH and HAPC. Therefore, further investigation of the differences in gene regulation among these three diseases will contribute to uncovering their underlying biological disparities. To illustrate the molecular features in details, we traced the mRNA sources in HAPC and PAH and basophil was found to be the common cell type among the top - 5 - ranked cells, which reflected the downstream role of immune response in H - I diseases.
Of the 18 top biomarkers in each group, the number of direct, intermediate crucial, and potential minor - effect biomarkers were 7, 6, and 5, respectively. These indicated our novel method can effectively identify genes related to H - I diseases that have been confirmed in previous experiments, thus validating the effectiveness of our risk assessment score. In summary, the “Bio - HAPC” category has the largest number of direct biomarkers, the “Bio - PAH” category has the largest number of intermediate crucial biomarkers, and the “Bio - trans” category has the largest number of potential minor - effect biomarkers. This indicates that the current types of omics data (in this study, GWAS results related to HAPC could not be obtained from public databases) can basically meet the research needs for HAPC. Regarding PAH, perhaps due to the complexity of its pathogenesis, most of the top - ranked genes are involved in biological processes closely related to the occurrence of PAH, which also validates the effectiveness of this method to some extent. Interestingly, there are currently no other systematic evaluation studies on H - I diseases. Therefore, the potential minor - effect biomarkers identified in this study can serve as an entry point for subsequent research and are of great significance. However, with the development of biological experiments, these intermediate crucial and potential minor-effect biomarkers may be proved to be direct biomarkers in the future.
The interplay among the four H-I diseases underscores a complex network of shared mechanisms and bidirectional risks. HAPC, characterized by hypoxia-induced erythrocytosis, substantially increases blood viscosity and causes vascular endothelial dysfunction, thereby elevating the risk of developing PAH. Moreover, HAPC contributes to cerebrovascular events through thrombosis and microvascular ischemia. PAH, in turn, exacerbates cerebral hypoperfusion, creating an indirect link to IS via shared hypoxic and pro-thrombotic pathways. PE induces systemic endothelial dysfunction. This not only predisposes patients to cerebrovascular complications but also heightens the risk of cardiovascular events. Significantly, PAH is a well - recognized complication of severe PE, highlighting a direct pathophysiological overlap between the two conditions. Conversely, chronic hypoxic states, such as those associated with HAPC, may exacerbate PE - like symptoms in pregnant individuals at high altitudes due to shared mechanisms of placental ischemia and systemic inflammation. These cross - disease relationships, where one condition can both contribute to and be a consequence of others, underscore the necessity for integrated research frameworks and holistic management strategies. Such approaches are essential for effectively addressing the overlapping pathophysiological cascades in H-I disorders.
The bidirectional risks between H-I diseases necessitate proactive clinical strategies. When a patient presents with an H-I condition such as PE/eclampsia, clinicians should initiate aggressive acute - phase management. This includes strict blood pressure control, administration of anticonvulsants (e.g., magnesium sulfate), and timely delivery. Concurrently, comprehensive diagnostic evaluations should be performed. Brain imaging (MRI/CT) is crucial for detecting silent cerebral ischemia or hemorrhage. Echocardiography and right heart catheterization are essential for screening PAH, while blood tests (e.g., D - dimer, blood viscosity) help assess the risk of thrombosis and HAPC. Long - term management requires lifelong cardiac follow - up for PE survivors. Serial echocardiograms can facilitate early detection of PAH. For PE/HAPC patients, stroke risk evaluations using carotid ultrasound and cognitive screening are essential. In individuals with chronic hypoxia or PAH, vigilance against polycythemia - driven thromboembolism is necessary. Multidisciplinary, integrated care is crucial to mitigate comorbidities and improve patient outcomes.
The primary limitations of this study stem from the restricted scope of data sources and sample diversity. First, the datasets relied upon in this analysis were predominantly based on transcriptomic profiles, while proteomic, metabolomic, or epigenetic data were either derived from intermediate analytical outputs or secondary data from public repositories. This limited integration of multi-omics layers constrained our ability to systematically dissect multiscale molecular mechanisms underlying hypoxia-ischemia (H-I) disorders. For instance, the lack of direct experimental data linking gene expression changes to protein post-translational modifications or metabolic pathway perturbations hindered a comprehensive understanding of disease-driven molecular networks. Second, the sample size and ethnic representativeness were insufficient to ensure generalizability. Most data originated from single-center studies or populations of specific ethnic backgrounds (e.g., European ancestry), lacking coverage of diverse geographic, genetic, and environmental exposure groups. This limitation may have led to the omission of critical gene-phenotype associations (e.g., functional racial differences in genes like HIST1H1T, RPL18A, or PIEZO2) and limited our capacity to explore race-specific risk profiles or therapeutic response heterogeneity. Additionally, the absence of longitudinal dynamic data restricted insights into temporal disease progression. H-I pathologies involve dynamic transitions from acute ischemic insults to chronic vascular remodeling, but static datasets cannot capture the temporal evolution of molecular markers (e.g., the timing of hemodynamic changes relative to gene expression shifts).
Despite these constraints, the methodological framework developed in this study is inherently scalable. Future research leveraging large-scale, integrated multi-omics data and ethnically diverse, prospective cohorts could significantly expand our findings by: (1) integrating transcriptomic, proteomic, and epigenetic data to unravel hierarchical regulatory mechanisms; (2) comparing cross-ethnic datasets to identify universal disease-driving modules and race-specific modifiers; and (3) analyzing time-series data to map the spatiotemporal dynamics of key pathways (e.g., endothelial dysfunction-inflammation-vascular remodeling axes).
Data availability statement
Publicly available datasets were analyzed in this study. This data can be found here: The datasets analyzed in this study are publicly available in the Gene Expression Omnibus (GEO) repository. The corresponding accession numbers and direct links are: PAH: GSE254617 (https://www.ncbi.nlm.nih.gov/geo/query/acc.cgi?acc=GSE254617), GSE168905 (https://www.ncbi.nlm.nih.gov/geo/query/acc.cgi?acc=GSE168905) HAPC: GSE29977 (https://www.ncbi.nlm.nih.gov/geo/query/acc.cgi?acc=GSE29977), GSE145802 (https://www.ncbi.nlm.nih.gov/geo/query/acc.cgi?acc=GSE145802) IS: GSE162955 (https://www.ncbi.nlm.nih.gov/geo/query/acc.cgi?acc=GSE162955), GSE16561 (https://www.ncbi.nlm.nih.gov/geo/query/acc.cgi?acc=GSE16561), GSE202709 (https://www.ncbi.nlm.nih.gov/geo/query/acc.cgi?acc=GSE202709) PE: GSE75010 (https://www.ncbi.nlm.nih.gov/geo/query/acc.cgi?acc=GSE75010).
Ethics statement
The studies involving humans were approved by The Institutional Review Board of BGl (BGI-IRB 22190-T5). The studies were conducted in accordance with the local legislation and institutional requirements. Written informed consent for participation was not required from the participants or the participants’ legal guardians/next of kin in accordance with the national legislation and institutional requirements.
Author contributions
YZ: Writing – original draft, Writing – review and editing, Data curation, Formal Analysis, Visualization, Methodology, Software. JL: Writing – original draft, Writing – review and editing, Conceptualization. ZB: Writing – review and editing, Validation, Funding acquisition. QY: Formal Analysis, Writing – review and editing. HC: Formal Analysis, Writing – review and editing. SC: Formal Analysis, Writing – review and editing. SL: Formal Analysis, Writing – review and editing. CL: Formal Analysis, Writing – review and editing. MF: Project administration, Supervision, Writing – review and editing. HL: Project administration, Writing – review and editing. XJ: Funding acquisition, Project administration, Supervision, Writing – review and editing. YW: Conceptualization, Funding acquisition, Project administration, Supervision, Writing – original draft, Writing – review and editing, Methodology.
Funding
The author(s) declare that financial support was received for the research and/or publication of this article. This study is supported by grants from National Key R&D Program of China [2022YFC2502402]. Shenzhen Medical Research Fund [B2404004], Science and Technology Program of Xizang Autonomous Region [XZ202303ZY0008G] and National Natural Science Foundation of China [82241023].
Conflict of interest
The authors declare that the research was conducted in the absence of any commercial or financial relationships that could be construed as a potential conflict of interest.
Generative AI statement
The author(s) declare that no Generative AI was used in the creation of this manuscript.
Publisher’s note
All claims expressed in this article are solely those of the authors and do not necessarily represent those of their affiliated organizations, or those of the publisher, the editors and the reviewers. Any product that may be evaluated in this article, or claim that may be made by its manufacturer, is not guaranteed or endorsed by the publisher.
Supplementary material
The Supplementary Material for this article can be found online at: https://www.frontiersin.org/articles/10.3389/fgene.2025.1587854/full#supplementary-material
References
Ahmed, A. S. I., Blood, A. B., and Zhang, L. (2024). Hypoxia-induced pulmonary hypertension in adults and newborns: implications for drug development. Drug Discov. Today 29, 104015. doi:10.1016/j.drudis.2024.104015
Aisagbonhi, O., Bui, T., Nasamran, C. A., St Louis, H., Pizzo, D., Meads, M., et al. (2023). High placental expression of FLT1, LEP, PHYHIP and IL3RA - in persons of African ancestry with severe preeclampsia. Placenta 144, 13–22. doi:10.1016/j.placenta.2023.10.008
Albertsson, A.-M., Bi, D., Duan, L., Zhang, X., Leavenworth, J. W., Qiao, L., et al. (2014). The immune response after hypoxia-ischemia in a mouse model of preterm brain injury. J. Neuroinflammation 11, 153. doi:10.1186/s12974-014-0153-z
Arowolo, A., Rhoda, C., and Khumalo, N. (2022). Mutations within the putative protease domain of the human FAM111B gene may predict disease severity and poor prognosis: a review of POIKTMP cases. Exp. Dermatol 31, 648–654. doi:10.1111/exd.14537
Barr, T. L., Conley, Y., Ding, J., Dillman, A., Warach, S., Singleton, A., et al. (2010). Genomic biomarkers and cellular pathways of ischemic stroke by RNA gene expression profiling. Neurology 75, 1009–1014. doi:10.1212/WNL.0b013e3181f2b37f
Baumeister, J., Maié, T., Chatain, N., Gan, L., Weinbergerova, B., de Toledo, M. A. S., et al. (2021). Early and late stage MPN patients show distinct gene expression profiles in CD34(+) cells. Ann. Hematol. 100, 2943–2956. doi:10.1007/s00277-021-04615-8
Benton, S. J., Leavey, K., Grynspan, D., Cox, B. J., and Bainbridge, S. A. (2018). The clinical heterogeneity of preeclampsia is related to both placental gene expression and placental histopathology. Am. J. Obstet. Gynecol. 219, 604.e1–604.e25. doi:10.1016/j.ajog.2018.09.036
Burattini, M., Falsetti, L., Potente, E., Rinaldi, C., Bartolini, M., Buratti, L., et al. (2022). Ischemic stroke as a presenting manifestation of polycythemia vera: a narrative review. Rev. Neurosci. 33, 303–311. doi:10.1515/revneuro-2021-0066
Carman, B. L., Qin, S., Predescu, D. N., Jana, M., Cortese, R., Aldred, M. A., et al. (2024). Dysregulation of the long noncoding RNA X-inactive-specific transcript expression in male patients with pulmonary arterial hypertension. Am. J. Pathol. 194, 1592–1606. doi:10.1016/j.ajpath.2024.04.005
Chichger, H., Rounds, S., and Harrington, E. O. (2019). Endosomes and autophagy: regulators of pulmonary endothelial cell homeostasis in health and disease. Antioxid. Redox Signal 31, 994–1008. doi:10.1089/ars.2019.7817
Dong, J., Wei, Z., and Zhu, Z. (2023). LncRNA TSIX aggravates spinal cord injury by regulating the PI3K/AKT pathway via the miR-532-3p/DDOST axis. J. Biochem. Mol. Toxicol. 37, e23384. doi:10.1002/jbt.23384
Dunk, C. E., Roggensack, A. M., Cox, B., Perkins, J. E., Åsenius, F., Keating, S., et al. (2012). A distinct microvascular endothelial gene expression profile in severe IUGR placentas. Placenta 33, 285–293. doi:10.1016/j.placenta.2011.12.020
Edgar, R., Domrachev, M., and Lash, A. E. (2002). Gene Expression Omnibus: NCBI gene expression and hybridization array data repository. Nucleic Acids Res. 30, 207–210. doi:10.1093/nar/30.1.207
Eltzschig, H. K., and Eckle, T. (2011). Ischemia and reperfusion--from mechanism to translation. Nat. Med. 17, 1391–1401. doi:10.1038/nm.2507
Feng, R., Mayuranathan, T., Huang, P., Doerfler, P. A., Li, Y., Yao, Y., et al. (2022a). Activation of γ-globin expression by hypoxia-inducible factor 1α. Nature 610, 783–790. doi:10.1038/s41586-022-05312-w
Feng, S., Li, R., Zhou, Q., Qu, F., Hu, W., and Liu, X. (2022b). Bioinformatics analysis to identify potential biomarkers and therapeutic targets for ST-segment-elevation myocardial infarction-related ischemic stroke. Front. Neurol. 13, 894289. doi:10.3389/fneur.2022.894289
Frolkis, A., Knox, C., Lim, E., Jewison, T., Law, V., Hau, D. D., et al. (2010). SMPDB: the small molecule pathway database. Nucleic Acids Res. 38, D480–D487. doi:10.1093/nar/gkp1002
Gangaraju, R., Song, J., Kim, S. J., Tashi, T., Reeves, B. N., Sundar, K. M., et al. (2020). Thrombotic, inflammatory, and HIF-regulated genes and thrombosis risk in polycythemia vera and essential thrombocythemia. Blood Adv. 4, 1115–1130. doi:10.1182/bloodadvances.2019001379
Gao, X.-M., Su, Y., Moore, S., Han, L.-P., Kiriazis, H., Lu, Q., et al. (2019). Relaxin mitigates microvascular damage and inflammation following cardiac ischemia-reperfusion. Basic Res. Cardiol. 114, 30. doi:10.1007/s00395-019-0739-9
Gazda, A. J., Pan, D., Erdos, K., Abu-Zeinah, G., Racanelli, A., Horn, E. M., et al. (2024). High pulmonary hypertension risk by echocardiogram shortens survival in polycythemia vera. Blood Adv. 9, 1320–1329. doi:10.1182/bloodadvances.2024013970
Ghosh, M. C., Zhang, D.-L., Ollivierre, W. H., Noguchi, A., Springer, D. A., Linehan, W. M., et al. (2021). Therapeutic inhibition of HIF-2α reverses polycythemia and pulmonary hypertension in murine models of human diseases. Blood 137, 2509–2519. doi:10.1182/blood.2020009138
Gotoh, D., Saito, T., Karnup, S., Morizawa, Y., Hori, S., Nakai, Y., et al. (2022). Therapeutic effects of a soluble guanylate cyclase activator, BAY 60-2770, on lower urinary tract dysfunction in mice with spinal cord injury. Am. J. Physiol. Ren. Physiol. 323, F447–F454. doi:10.1152/ajprenal.00105.2022
GTEx Consortium (2013). The genotype-tissue expression (GTEx) project. Nat. Genet. 45, 580–585. doi:10.1038/ng.2653
Gu, Z., and Hübschmann, D. (2023). simplifyEnrichment: a bioconductor package for clustering and visualizing functional enrichment results. Genomics Proteomics Bioinforma. 21, 190–202. doi:10.1016/j.gpb.2022.04.008
He, M., Tao, K., Xiang, M., and Sun, J. (2023). Hpgd affects the progression of hypoxic pulmonary hypertension by regulating vascular remodeling. BMC Pulm. Med. 23, 116. doi:10.1186/s12890-023-02401-y
Hong, J., Medzikovic, L., Sun, W., Wong, B., Ruffenach, G., Rhodes, C. J., et al. (2024). Integrative multiomics in the lung reveals a protective role of asporin in pulmonary arterial hypertension. Circulation 150, 1268–1287. doi:10.1161/CIRCULATIONAHA.124.069864
Jiang, S., Li, H., Zhang, L., Mu, W., Zhang, Y., Chen, T., et al. (2025). Generic Diagramming Platform (GDP): a comprehensive database of high-quality biomedical graphics. Nucleic Acids Res. 53, D1670–D1676. doi:10.1093/nar/gkae973
Julian, C. G., Gonzales, M., Rodriguez, A., Bellido, D., Salmon, C. S., Ladenburger, A., et al. (2015). Perinatal hypoxia increases susceptibility to high-altitude polycythemia and attendant pulmonary vascular dysfunction. Am. J. Physiology-Heart Circulatory Physiology 309, H565–H573. doi:10.1152/ajpheart.00296.2015
Kanehisa, M., and Goto, S. (2000). KEGG: kyoto encyclopedia of genes and genomes. Nucleic Acids Res. 28, 27–30. doi:10.1093/nar/28.1.27
Kanki, H., Matsumoto, H., Togami, Y., Okuzaki, D., Ogura, H., Sasaki, T., et al. (2023). Importance of microRNAs by mRNA-microRNA integration analysis in acute ischemic stroke patients. J. Stroke Cerebrovasc. Dis. 32, 107277. doi:10.1016/j.jstrokecerebrovasdis.2023.107277
Karczewski, K. J., Francioli, L. C., Tiao, G., Cummings, B. B., Alföldi, J., Wang, Q., et al. (2020). The mutational constraint spectrum quantified from variation in 141,456 humans. Nature 581, 434–443. doi:10.1038/s41586-020-2308-7
Landrum, M. J., Lee, J. M., Riley, G. R., Jang, W., Rubinstein, W. S., Church, D. M., et al. (2014). ClinVar: public archive of relationships among sequence variation and human phenotype. Nucleic Acids Res. 42, D980–D985. doi:10.1093/nar/gkt1113
Leek, J. T., Johnson, W. E., Parker, H. S., Jaffe, A. E., and Storey, J. D. (2012). The sva package for removing batch effects and other unwanted variation in high-throughput experiments. Bioinformatics 28, 882–883. doi:10.1093/bioinformatics/bts034
Lertzman, M., Frome, B., and Cherniack, R. (1964). Hypoxia in polycythemia vera. Ann. Intern. Med. 60, 409–417. doi:10.7326/0003-4819-60-3-409
Li, D., Shao, N.-Y., Moonen, J.-R., Zhao, Z., Shi, M., Otsuki, S., et al. (2021). ALDH1A3 coordinates metabolism with gene regulation in pulmonary arterial hypertension. Circulation 143, 2074–2090. doi:10.1161/CIRCULATIONAHA.120.048845
Li, X.-C., Zhu, X.-Y., Wang, Y.-Y., Tong, S.-L., Chen, Z.-L., Lu, Z.-Y., et al. (2024). Canagliflozin alleviates pulmonary hypertension by activating PPARγ and inhibiting its S225 phosphorylation. Acta Pharmacol. Sin. 45, 1861–1878. doi:10.1038/s41401-024-01286-9
Lingam, I., Avdic-Belltheus, A., Meehan, C., Martinello, K., Ragab, S., Peebles, D., et al. (2021). Serial blood cytokine and chemokine mRNA and microRNA over 48 h are insult specific in a piglet model of inflammation-sensitized hypoxia-ischaemia. Pediatr. Res. 89, 464–475. doi:10.1038/s41390-020-0986-3
Meyer, L., Deau, B., Forejtníková, H., Duménil, D., Margottin-Goguet, F., Lacombe, C., et al. (2007). beta-Trcp mediates ubiquitination and degradation of the erythropoietin receptor and controls cell proliferation. Blood 109, 5215–5222. doi:10.1182/blood-2006-10-055350
Møller, J. E., Hillis, G. S., Oh, J. K., and Pellikka, P. A. (2005). Prognostic importance of secondary pulmonary hypertension after acute myocardial infarction. Am. J. Cardiol. 96, 199–203. doi:10.1016/j.amjcard.2005.03.043
Naffa, R. G., Awidi, A. S., Yousef, A.-M. F., and Ismail, S. I. (2012). CYP1AI, glutathione S-transferase gene polymorphisms and risk of Polycythemia vera. Cancer Epidemiol. 36, 68–72. doi:10.1016/j.canep.2011.05.001
Nand, S., and Orfei, E. (1994). Pulmonary hypertension in polycythemia vera. Am. J Hematol 47, 242–244. doi:10.1002/ajh.2830470320
Nayernia, K., Meinhardt, A., Drabent, B., Adham, I. M., Müller, C., Steckel, M., et al. (2003). Synergistic effects of germ cell expressed genes on male fertility in mice. Cytogenet Genome Res. 103, 314–320. doi:10.1159/000076819
Ni, S., Ji, T., Dong, J., Chen, F., Feng, H., Zhao, H., et al. (2022). Immune cells in pulmonary arterial hypertension. Heart Lung Circ. 31, 934–943. doi:10.1016/j.hlc.2022.02.007
Olivier, J. D. A., Åkerud, H., Skalkidou, A., Kaihola, H., and Sundström-Poromaa, I. (2014). The effects of antenatal depression and antidepressant treatment on placental gene expression. Front. Cell Neurosci. 8, 465. doi:10.3389/fncel.2014.00465
Pan, Z., Huang, K., Li, N., Duan, P., Huang, J., Yang, D., et al. (2024). LncRNA TSIX knockdown restores spinal cord injury repair through miR-30a/SOCS3 axis. Biotechnol. Genet. Eng. Rev. 40, 765–787. doi:10.1080/02648725.2023.2190948
Ren, Y.-S., Li, H.-H., Yao, J.-C., Tan, Y.-J., Pan, L.-H., Peng, T., et al. (2020). Application quantitative proteomics approach to identify differentially expressed proteins associated with cardiac protection mediated by cycloastragenol in acute myocardial infarction rats. J. Proteomics 222, 103691. doi:10.1016/j.jprot.2020.103691
Shah, T. G., Sutaria, J. M., and Vyas, M. V. (2019). The association between pulmonary hypertension and stroke: a systematic review and meta-analysis. Int. J. Cardiol. 295, 21–24. doi:10.1016/j.ijcard.2019.07.085
Shi, Y., Liu, J., Zhang, R., Zhang, M., Cui, H., Wang, L., et al. (2023). Targeting endothelial ENO1 (Alpha-Enolase) -PI3K-Akt-mTOR Axis alleviates hypoxic pulmonary hypertension. Hypertension 80, 1035–1047. doi:10.1161/HYPERTENSIONAHA.122.19857
Szklarczyk, D., Kirsch, R., Koutrouli, M., Nastou, K., Mehryary, F., Hachilif, R., et al. (2023). The STRING database in 2023: protein-protein association networks and functional enrichment analyses for any sequenced genome of interest. Nucleic Acids Res. 51, D638–D646. doi:10.1093/nar/gkac1000
Tachibana, T., Nakayama, N., Matsumura, A., Nakajima, Y., Takahashi, H., Miyazaki, T., et al. (2017). Pulmonary hypertension associated with pulmonary veno-occlusive disease in patients with polycythemia vera. Intern. Med. 56, 2487–2492. doi:10.2169/internalmedicine.8629-16
Tajsic, T., and Morrell, N. W. (2011). Smooth muscle cell hypertrophy, proliferation, migration and apoptosis in pulmonary hypertension. Compr. Physiol. 1, 295–317. doi:10.1002/cphy.c100026
Tan, G., and Meier-Abt, F. (2021). Differential expression of hydroxyurea transporters in normal and polycythemia vera hematopoietic stem and progenitor cell subpopulations. Exp. Hematol. 97, 47–56.e5. doi:10.1016/j.exphem.2021.02.011
Tator, C. H., and Fehlings, M. G. (1991). Review of the secondary injury theory of acute spinal cord trauma with emphasis on vascular mechanisms. J. Neurosurg. 75, 15–26. doi:10.3171/jns.1991.75.1.0015
Tian, S., Cai, Z., Sen, P., van Uden, D., van de Kamp, E., Thuillet, R., et al. (2022). Loss of lung microvascular endothelial Piezo2 expression impairs NO synthesis, induces EndMT, and is associated with pulmonary hypertension. Am. J. Physiol. Heart Circ. Physiol. 323, H958–H974. doi:10.1152/ajpheart.00220.2022
Tomaszewski, M., Charchar, F. J., Nelson, C. P., Barnes, T., Denniff, M., Kaiser, M., et al. (2011). Pathway analysis shows association between FGFBP1 and hypertension. J. Am. Soc. Nephrol. 22, 947–955. doi:10.1681/ASN.2010080829
Vorperian, S. K., Moufarrej, M. N., and Quake, S. R. (2022). Cell types of origin of the cell-free transcriptome. Nat. Biotechnol. 40, 855–861. doi:10.1038/s41587-021-01188-9
Wade, B. E., Zhao, J., Ma, J., Hart, C. M., and Sutliff, R. L. (2018). Hypoxia-induced alterations in the lung ubiquitin proteasome system during pulmonary hypertension pathogenesis. Pulm. Circ. 8, 2045894018788267. doi:10.1177/2045894018788267
Wang, S., Tai, Y., Yang, X., Li, P., Wang, H., Tan, Y., et al. (2024). Identification of potential biomarkers and drug of ischemic stroke in patients with COVID-19 through machine learning. Heliyon 10, e39039. doi:10.1016/j.heliyon.2024.e39039
Wei, D., Chen, X., Xu, J., and He, W. (2023). Identification of molecular subtypes of ischaemic stroke based on immune-related genes and weighted co-expression network analysis. IET Syst. Biol. 17, 58–69. doi:10.1049/syb2.12059
Wei, F., Lin, Z., Lu, W., Luo, H., Feng, H., Liu, S., et al. (2025). Deficiency of endothelial Piezo2 impairs pulmonary vascular angiogenesis and predisposes pulmonary hypertension. Hypertension 82, 583–597. doi:10.1161/HYPERTENSIONAHA.124.22948
Wu, J., Li, C., Lei, Z., Cai, H., Hu, Y., Zhu, Y., et al. (2022). Comprehensive analysis of circRNA-miRNA-mRNA regulatory network and novel potential biomarkers in acute myocardial infarction. Front. Cardiovasc Med. 9, 850991. doi:10.3389/fcvm.2022.850991
Xu, J., Yang, Y., Yang, Y., and Xiong, C. (2021). Identification of potential risk genes and the immune landscape of idiopathic pulmonary arterial hypertension via microarray gene expression dataset reanalysis. Genes (Basel) 12, 125. doi:10.3390/genes12010125
Yang, M.-Y., Ji, M.-H., Shen, T., and Lei, L. (2022). Integrated analysis identifies four genes as novel diagnostic biomarkers which correlate with immune infiltration in preeclampsia. J. Immunol. Res. 2022, 2373694. doi:10.1155/2022/2373694
Yin, J., Yang, J., Zhao, B., Zhao, C., Fu, W., He, Y., et al. (2022). Experimental evidence of buyang huanwu decoction and related modern preparations (naoxintong capsule and yangyin tongnao granule) in treating cerebral ischemia: intestinal microorganisms and transcriptomics in rats. Evid. Based Complement. Altern. Med. 2022, 4016935. doi:10.1155/2022/4016935
Yu, G., Wang, L.-G., Han, Y., and He, Q.-Y. (2012). clusterProfiler: an R package for comparing biological themes among gene clusters. OMICS 16, 284–287. doi:10.1089/omi.2011.0118
Yuen, L., Gogakos, T., Boiocchi, L., Hobbs, G., and Hasserjian, R. (2025). Basophilia predicts poorer outcomes in essential thrombocythemia, polycythemia vera, primary myelofibrosis, and myeloproliferative neoplasm, unclassifiable. Am. J. Hematol. 100, 320–322. doi:10.1002/ajh.27530
Zamudio, S. (2007). High-altitude hypoxia and preeclampsia. Front. Biosci. 12, 2967–2977. doi:10.2741/2286
Zhang, J., Dong, J., Martin, M., He, M., Gongol, B., Marin, T. L., et al. (2018a). AMP-Activated protein kinase phosphorylation of angiotensin-converting enzyme 2 in endothelium mitigates pulmonary hypertension. Am. J. Respir. Crit. Care Med. 198, 509–520. doi:10.1164/rccm.201712-2570OC
Keywords: risk assessment, hypoxia-ischemia, shared features, disease profile, omics
Citation: Zhang Y, Liu J, Basang Z, Yang Q, Chen H, Chen S, Li S, Lei C, Fang M, Liu H, Jin X and Wang Y (2025) Shared genetic features inference among hypoxia-ischemia diseases in the presence of heterogenous omics data based on a novel risk assessment method. Front. Genet. 16:1587854. doi: 10.3389/fgene.2025.1587854
Received: 05 March 2025; Accepted: 14 April 2025;
Published: 28 April 2025.
Edited by:
Pu-Feng Du, Tianjin University, ChinaReviewed by:
Abdur Rasool, University of Hawaii at Manoa, United StatesZhenguo Yang, First Affiliated Hospital of Jinan University, China
Copyright © 2025 Zhang, Liu, Basang, Yang, Chen, Chen, Li, Lei, Fang, Liu, Jin and Wang. This is an open-access article distributed under the terms of the Creative Commons Attribution License (CC BY). The use, distribution or reproduction in other forums is permitted, provided the original author(s) and the copyright owner(s) are credited and that the original publication in this journal is cited, in accordance with accepted academic practice. No use, distribution or reproduction is permitted which does not comply with these terms.
*Correspondence: Yingying Wang, d2FuZ3lpbmd5aW5nM0BnZW5vbWljcy5jbg==; Xin Jin, eGluamluQGdlbm9taWNzLmNu
†These authors share first authorship