- Bermuda Institute of Ocean Sciences, St. Georges, Bermuda
The diversity and specific composition of zooplankton communities have a direct effect on the ecosystem services (e.g., carbon export) and structure of ocean food webs. In areas where diversity is high, a detailed characterization of the whole community can represent a hurdle that prevents a complete understanding of those processes and interactions. One such region is the Sargasso Sea, where zooplankton diversity is among the highest in the world oceans. As a consequence, at the Bermuda Atlantic Time-series Study location, most research has focused on zooplankton biomass or on particular groups. To provide new insight into the total community composition at the BATS site, in this study the zooplankton community is investigated by means of metabarcoding of the 18S V9 hypervariable region. Day and night samples from a full year (2015) show the signature of diel vertical migration, driven by those groups with a higher representation in the metabarcoding reads. Seasonal ordination was detected after square-root transformation and, similarly to temperate regions, four seasons could be differentiated based on community composition (post-spring bloom, summer stratification, fall mixing event, and winter mixing), with no correspondence with the zooplankton biomass patterns. This community ordination showed correlation with the measured vertical flux, highlighting the need of understanding community regimes, even in theoretically stable regions such as the oligotrophic ocean, to advance in our understanding of zooplankton-mediated processes
Introduction
The zooplankton community is a key component of the ocean ecosystem. Considering both their direct grazing on primary producers and their grazing of other consumers, zooplankton channel 15 to > 50% of the ocean production toward upper trophic levels (Steinberg and Landry, 2017), key for sustaining ecosystems and fisheries. To properly understand the processes affecting or driven by the zooplankton community, a truly knowledge of their taxonomic composition (i.e., biodiversity) is needed (Chiba et al., 2018). For example, species-level identification has allowed the detection of changes in distribution (Beaugrand et al., 2002; Harris et al., 2015; Yoshiki et al., 2015) and phenology (Mackas et al., 2012) associated to climate change. Locally, seasonal succession in the zooplankton community has an effect on its functional profile, including, the zooplankton trophic role, both as predators and as prey (Pomerleau et al., 2015) or their influence in the carbon cycle (Mayzaud and Pakhomov, 2014).
In the Sargasso Sea, zooplankton diversity reaches one of its highest in the world oceans (Rombouts et al., 2010). Studies of the open ocean zooplankton in the Sargasso Sea started with Moore (1949) and Grice and Hart (1962), however the most exhaustive research in the zooplankton community composition was done by Georgiana Deevey (Deevey, 1968, 1971; Deevey and Brooks, 1971, 1977) at Hydrostation “S,” in the vicinity of Bermuda. The complexity of the zooplankton inhabiting these waters was however a limitation as Deevey and Brooks (1977) indicated that “Because of taxonomic problems and the amount of time that would have been involved, no attempt has been made to identify many small species of such genera as, for example, Paracalanus, Clausocalanus, Spinocalanus, Microcalanus, Scolecithricella, Scaphocalanus, Oithona, and Oncaea. Also, some larger species were not identified”. Still the authors identified more than 300 species of copepods, and it is likely that those uncounted genera (or groups) would have easily accounted for another 100 species at least. A few other studies were carried out in the Sargasso Sea or their vicinity later on, but in general focused on a single or a handful of groups (Angel, 1979; Wiebe et al., 1992). Most of the studies at community level have since used main taxa groups (Eden et al., 2009; Ivory et al., 2018), focused on a single group (Stone and Steinberg, 2014) or processes and biogeochemical cycling (Steinberg et al., 2000; Madin et al., 2001; Schnetzer and Steinberg, 2002; Stone and Steinberg, 2016; Maas et al., 2018) more than in the community composition.
In the last two decades, molecular markers have been developed to be used for species identification, an approach commonly known as barcoding (Hebert et al., 2003). Applied to all environments, including the marine zooplankton, single-individual based analyses identified (and barcoded) 175 holoplankton species in the Sargasso Sea in a single cruise (Bucklin et al., 2010), leaving, however, 100s of species without identification due to time constrains. Nowadays, with the aid of high-throughput sequencing, community-level barcoding can be done in a single analysis, an approach known as metabarcoding (Amaral-Zettler et al., 2009; Fonseca et al., 2010; Taberlet et al., 2012). Applied to the zooplankton, this technique has demonstrated its ability to uncover the existing diversity in very complex samples (Lindeque et al., 2013), determine spatial biographical regions based in community structure (Hirai and Tsuda, 2015; Hirai et al., 2015; Bucklin et al., 2019), capture the vertical structure in the open ocean (Sommer et al., 2017), or show the potential for monitoring of estuarine conditions and seasonality (Abad et al., 2016, 2017). This method is still subject to technical limitations, and there is much to be learn about it, especially the meaning of read counts compared to “classical” studies such as individual counts or biomass (Bucklin et al., 2016; Lamb et al., 2019).
Understanding the community dynamics associated to seasonal cycles is essential to precisely estimate basic questions such as biogeochemical cycling or trophic webs (Burd et al., 2010). Although the Sargasso Sea is often described a low-seasonality location, particle flux shows a clear seasonal signal (Conte et al., 2001) and, compared to truly oligotrophic locations such as ALOHA, the hydrography at the Bermuda Atlantic Time-series Study (BATS) is subject to strong seasonality, with a winter mixing period below the photic layer, summer stratification and fall mixing, influencing nutrients inventory, primary production, particle flux, etc. similarly to temperate locations (Church et al., 2013). Subtropical gyres are characterized by a relatively stable community throughout the year, meanwhile temperate regions show strong seasonality in the community composition. This difference has a strong impact in the effects of the zooplankton in the biogeochemical cycles, from how efficient is to channel the primary production to upper trophic levels, to cycles of secondary production or export of carbon to the mesopelagic (Carroll et al., 1998; Wexels Riser et al., 2002; Mackas et al., 2012; Hannides et al., 2020). Understanding the dynamics of the zooplankton community at BATS, in the boundary of the North Atlantic Subtropical Gyre, is therefore essential to properly address the biogeochemical cycles at this site. For the zooplankton community, studies have previously focused on describing the monthly evolution of single species or taxonomic groups (Deevey, 1971; Deevey and Brooks, 1971; Ivory et al., 2018); a study linking hydrography to shifts in community composition is, however, still missing. From the existing data, the best suited would be those from Deevey studies but, unfortunately, the counts were not available in the original publications, and all efforts done to find the original data from her institution, or at the Bermuda Institute of Ocean Sciences, have been unsuccessful. This study analyses the zooplankton community at the BATS location (32.166667° N, 64.5° W), in the Sargasso Sea, during the year 2015, by means of metabarcoding, using the hypervariable V9 region of the 18S gene (Amaral-Zettler et al., 2009; de Vargas et al., 2015), a region commonly used for zooplankton metabarcoding (Albaina et al., 2016; Abad et al., 2017; Bucklin et al., 2019). The analyses aim to understand the seasonal response of the zooplankton community to hydrographic conditions, the ability of the metabarcoding approach to detect processes such as seasonal and daily (day/night) changes in the community composition of the zooplankton, and to determine if there is correlation between routine biological oceanographic measurements taken within the BATS program and the changes in the zooplankton community.
Materials and Methods
Metadata
Oceanographic data were accessed from the BATS ftp repository1. Sampling, quality control and post-processing methodologies are available on the BATS website2. Temperature, salinity and CTD fluorescence profiles from surface to 1000 m were graphed over time using Ocean Data View Ver. 4.8 (Schlitzer, 2017). Mixed layer depth (MLD) was defined as the depth at which density (sigma-theta; σθ) reached 0.125 kg m–3 difference compared to surface (Levitus, 1982) calculated from CTD profiles. Monthly integrated primary production (as per BATS methodology; Knap et al., 1997) and total Chl-a per square meter were calculated from Dec. 2014 to March 2016. Day and night size-fractionated zooplankton biomass (as per BATS protocol and data) was also analyzed. Both total biomass as well as fractionated biomass were graphed. Sedimentary traps flux data (measuring total biomass, C, N, and P from sedimentary traps during BATS cruises; a trap array, with traps at 150, 200, and 300 m is allowed to drift for 72 h; Steinberg et al., 2001) was also retrieved.
Zooplankton Sampling and Preservation
Zooplankton samples were taken during 2015 and early 2016 onboard the RV Atlantic Explorer during BATS cruises from February 2015 to March 2016 (Table 1). Sampling methods and gear have been previously described in detail in Madin et al. (2001) and Steinberg et al. (2012). In short, zooplankton was sampled using a 1-m2 rectangular, 202 μm mesh net. Oblique tows were done during the day (between 13:59 and 15:40 GMT) and night (between 01:24 and 6:19 GMT) on each cruise, with the exception of the 20314 cruise in which the day tow was canceled due to rough weather. Net tows were done at a ship speed around 1 kN, and paying out 250 m of wire at 15 m per minute both ways. Depth was recorded using a STAR ODDI DST centi-TD depth and temperature logger (effective depths ranging 113–247 m; Table 1). Once on deck, water was drained, and samples were immediately fixed in 95% undenatured alcohol. Samples were then stored at −20°C until returning to BIOS (1 to 3 days). Once at BIOS, the ethanol was replaced and samples stored at 4°C until analysis. If the ethanol was turbid after 24 h, ethanol was replaced again. Samples were stored in 500 mL of ethanol minimum. If the sample occupied more than 1/3 of the jar, it was then transferred to a 1 L jar, and filled to the top with ethanol.
Sample Processing and DNA Extraction
Prior to extraction, each zooplankton sample was split in two subsamples using a Motoda splitter (Motoda, 1959). Half of the sample was set for extraction, meanwhile the other half was kept as archival material at BIOS. Macroplankton (>2 cm; mostly the larger fish larvae) were removed from the sample and stored in a separate jar, not being used for this study.
The Extraction method was based on the SDS-chloroform extraction in Corell and Rodríguez-Ezpeleta (2014) but using the E.Z.N.A.® Mollusc DNA Kit (Omega Bio-Tek, Norcross, GA, United States) to recover the DNA instead of the ethanol precipitation. Three final pseudoreplicates (since they originated from the same zooplankton sample) were obtained for each sample.
Samples were filtered through a 53 μm mesh placed on a home-made sieve that allows for easy removal of the mesh without disturbing the sample, and thoroughly washed with milliQ water to remove as much ethanol as possible. The mesh was them removed and placed in a 50 mL falcon tube, with part of the mesh hanging outside the tube. The falcon tube was then tightly closed, securing the mesh to the edge. The tube was then centrifuged at 3,500 g for 10 min to remove as much water and remainder ethanol as possible. After centrifugation, the pellet was weighted, transferred to a fresh 50 mL falcon tube (carefully checking that no plankton was left on the mesh) and 15 mL of SDS buffer (Tris-HCl 10 mM, EDTA 100 mM pH 8.0, NaCl 200 mM, SDS 1%) was added if total mass was < 10 mg, and 25 mL if above. Samples were then vortexed to break the pellet away and ensure rapid mixing of the plankton with the buffer to prevent DNA degradation. The sample was then ground using a Fisher Scientific Homogenizer FSH125, equipped with a 10 mm × 160 mm steel generator. Samples were ground at 1/3 of the maximum speed (approximately 10,000 rpm; this model of homogenizer does not give precise measurement of the speed) for 5 min. This low speed was chosen since, during initial testing, it was noticed that using higher speeds (i.e., near maximum speeds), even for shorter times, often resulted in excessive DNA fragmentation and rapid heating of the sample, and fragments > 2 kb were difficult to obtain during amplification. Large gastropods, whose shells softened after contacting the buffer, did not get broken often, and were cut using dissecting scissors. After the grinding step, the generator was rinsed using 10 mL of SDS buffer to minimize the loss of sample in the generator. Between samples, the generator was completely disassembled, all parts washed with soap under running water, soaked in bleach 10% for 5 min and rinsed in MilliQ water before assembling and running the next sample. For samples < 10 mg of biomass, extraction was done using the 25 mL of buffer used (15 mL initially + 10 mL for cleaning the generator). For samples with > 10 mg, extra 15 mL of buffer were added, to make up to 40 mL of SDS buffer. Using less buffer caused DNA to degrade during extraction during preliminary tests. No heating of the sample was detected during the grinding process at low speeds.
After the grinding protocol, proteinase K (Sigma-Aldrich; St. Louis, MO, United States) was added to the buffer (0.2 mg mL–1 final concentration), as well as 5 mL of sterilized stainless steel Shot, 3/32′′ Ellipse (Rio Grande, CA, United States). The tubes were then placed in an oven at 60°C for 4 h, vortexing at maximum speed for 30 s every 30 min. After the 4 h, the tubes were centrifuged at 3,500 g for 15 min. Three replicates of 400 μL of the supernatant were transferred to 1.5 mL Eppendorf tubes. Three additional cryovials (2 mL of supernatant each) were stored at −80°C, and the rest of the supernatant was transferred to a clean 15 mL Falcon tube and stored at −20°C. For the three Eppendorf tubes, if the supernatant still contained small particles that would not precipitate at the aforementioned centrifugation forces, the Eppendorf tubes were then centrifuged at 10,000 g for 5 min or until complete precipitation of the particles, and then supernatant transferred to a new tube. After this step, DNA was extracted following the E.Z.N.A.® Mollusc DNA Kit (Omega Bio-Tek, Norcross, GA, United States), from the addition of chloroform:Isoamyl alcohol 24:1 step on. A modification to the standard protocol included a 14 min centrifugation step to separate the aqueous and the organic phases at this point. From that, only 300 mL of the aqueous phase were carried to the next step, to ensure avoiding the milky interface and the organic phase. All the other steps followed the manufacturer conditions. Final elution was done using 2 × 200 μL of Ambion® nuclease-free water (not DEPC-Treated; Life Technologies, Foster City, CA, United States). DNA was then stored at −20°C until amplification after quantification (NanoDropTM One). DNA output ranged between 16 and 100 μg of DNA per pseudoreplicate.
18S V9 Amplification
One PCR reaction was done for each pseudoreplicate (total 3 PCR per sample). Amplification of the V9 region was done using the primers 1389F and 1510R (Amaral-Zettler et al., 2009) integrated in an oligonucleotide consisting of an Illumina adapter, an 8-nt index sequence and a 10-nt pad sequence identical for all oligonucleotides (Supplementary Table S1 in Supplementary File S1). Amplifications were done using the AccuPower® ProFi Taq PCR PreMix (20 μL reaction; BIONEER; South Korea). PCR steps were performed such as to minimize amplification bias and formation of chimeras, including 300–500 ng of DNA template (to minimize the number of PCR cycles needed and the associated bias), fewer PCR cycles (12 cycles) and lower temperatures (Aird et al., 2011; Ahn et al., 2012; Gonzalez et al., 2012). The premix includes the DNA polymerase, reaction buffer, dNTPs, tracking dye, and a patented stabilizer. To the premix, 1 μL of each primer (10 μM) were added; the amplification protocol consisted in an initial denaturation at 94°C for 3 min, followed by 12 cycles of 94°C for 30 s, annealing at 56°C for 30 s and extension at 72°C for 30 s; a final extension step was done at 72°C for 15 min, and then kept at 4°C. After amplification, PCR success was tested using 3 μL of the PCR reaction in a 1.5% agarose gel in TBE buffer. For each sample, the remainder of the PCR product from the pseudoreplicates were pooled and sent for sequencing at the Microbial Analysis, Resources, and Services (MARS) unit of the University of Connecticut Biotechnology Bioservices Center. Sequencing was performed in an Illumina MiSeq using the MiSeq Reagent Kit v2 (500-cycles; 2 × 250) V2 chemistry. Sequence files are available at the NCBI site under the bioproject https://www.ncbi.nlm.nih.gov/bioproject/PRJNA540654. To test for replicability within the procedure, two samples were submitted using different indexes in separate batches.
Bioinformatics Pipeline
Demultiplexed samples were analyzed in MOTHUR ver. 1.39.5. (Schloss et al., 2009). The full script, annotated, is available at https://github.com/blancobercial/BATS_zooplankton. Contigs were made allowing for trimming outside the overlapping region (therefore only reads giving full length in both directions will be retained in a later cleaning step), and bases that are compared to a gap in the other fragment were eliminated if the quality score was below 30. After pairing, all reads containing any ambiguity or shorter than 115 bp were removed. Unique sequences were aligned against the V9 region of the SILVA 128 release database (Quast et al., 2013). Sequences were trimmed to the length of the V9 region, and only those showing completeness (starting in the first base and ending in the last base of the alignment) were kept, avoiding artificial OTUs due to unfinished amplifications. After gap removal, chimeras were removed using UCHIME (Edgar et al., 2011) as implemented in MOTHUR, and unique sequences selected (since after trimming and processing a few sequences may become identical). Distances between reads were calculated, considering gaps as a single event per gap no matter the length. The effect of base by base threshold clustering was studied running an initial clustering step using the nearest neighbor algorithm, with a 0.10 cutoff and 1000 precision. After graphical identification of the clustering level corresponding to 2 bp (= 0.016), final clustering was done using the OptiClust algorithm (Westcott and Schloss, 2017) with distance set to 0.016. This distance would only remove PCR and sequencing errors, since it would only cluster the true reads with the derived reads with 1 bp-error (2 bp distance between two reads with one error each). This approach is similar in concept to the TARA oceans 1 bp swarming procedure (de Vargas et al., 2015). OTUs were taxonomically assigned to a database developed from the complete SILVA 128 release database for SSU (SILVA_128_SSURef_tax_silva; not the NR99 available from www.MOTHUR.org)3. Bash scripts used to transform the SILVA files to MOTHUR format are available in the repository containing all scripts used in this manuscript4. Using the NR99 would have worsened the taxonomic assignment, since the 18S is a highly conserved gene, and it was observed that the same V9 region sequence was often shared between components belonging to the same Superfamily. Based on this classification, only metazoan data were selected for all downstream analyses. For identification of taxa (OTUs) flagged by ecological analyses (see below), each of the OTU were BLASTed against the GenBank and results individually analyzed, to take into account the limits of taxonomic assignment of the V9 region and the existing references.
Data Analyses
Diversity Analyses
To analyze the effect of read numbers (i.e., read depth) per sample on OTU richness (i.e., diversity), unfiltered and non-standardized read counts were analyzed in iNEXT online (Hsieh et al., 2016; Chao et al., 2018). Interpolated (rarefaction) curves and the extrapolation curves (to the maximum number of reads per sample) were calculated for each sample for species (OTU) richness (Chao et al., 2014). Confidence intervals were obtained after 100 bootstrap repetitions. Same analyses (rarefaction and extrapolation) were done after removal of singletons and doubletons for the whole dataset (not per sample).
Standardized Analyses
For community analyses, all samples were standardized to 36,471 reads (the minimum per sample), and all OTU abundances values < 1 after transformation assumed to be zero (to make it equal to the sample with less reads); and then any OTU with global abundances < 2 removed (global singletons). Two parallel analyses were run using PRIMER ver. 7 (Clarke and Gorley, 2015). The first considered only the relative abundances of the individuals at each sample as obtained after the “standardize by the total” procedure in PRIMER (that is, in result, a normalization to percentage of the total; ranging between 0 and 100); meanwhile the second analyses included a subsequent square root transformation (Hellinger standardization; Legendre and Gallagher, 2001), to reduce the weight of the highly abundant OTUs. Similarity matrices were built using Bray–Curtis similarity index, and graphically represented using non-metric MultiDimensional Scaling (nMDS). Non-hierarchical, k-R Clustering analyses were run for grouping levels from 2 to 12, selecting R Cluster mode with 1000 restarts. The results were compared to ANOSIM pairwise tests at those levels of clustering, with 10,000 permutations to test for significance. To compare to a hierarchical clustering approach, a group average clustering (paired with a Simprof analysis for significance of the clusters/nodes with 10,000 permutations) was also carried out. Grouping was analyzed in terms of a significant increase of the R statistic from the k-R approach (equivalent to the ANOSIM R) as well as for ecological significance.
SIMPER analyses were used to identify the OTUs contributing to both the similarity within, and dissimilarity between, the groups chosen for each analyses. Initial analyses included all samples and OTUs, meanwhile a more evenly sampled set, including only core BATS cruises (sampled then approximately once per month) from 2015 were analyzed, to avoid heterogeneity due to different time lags between consecutive sampling events (Table 1). Environmental variables (MLD, PP and Chl-a per cruise) were compared against the nMDS ordination using the BIO-ENV analyses in PRIMER. MLD has shown to drive changes in the biogeochemistry and the community composition in other groups (Carlson et al., 1994; Steinberg et al., 2001; Carlson et al., 2009), and provides a better measure than a metric such as average temperature, that could not reflect the temperature the organisms are exposed to (since it averages above and below the thermocline). Chla is considered a proxy for phytoplankton biomass, and PP has showed to play a role in resource availability for the zooplankton, and both, although correlated, often show different maxima during the year. It was chosen to keep this short list of variables, instead of adding others such as nutrient levels (N, P, etc.) that would be already influenced by MLD and affecting PP. This would also reduce the risk of finding spurious correlations between the variables and the nMDS ordination.
The relationship between sedimentary traps flux (measuring total biomass, C, N, and P from sedimentary traps during BATS cruises; a trap array, with traps at 150, 200, and 300 m is allowed to drift for 72 h; Steinberg et al., 2001) to the community composition was interrogated using a mantel test (Spearman rank; significance tested after 10,000 permutations) between the similarity matrices derived from the community composition (as described above) and from the BATS flux components measured per month using the sedimentary traps.
For direct comparison with image analyses existing for BATS, clustering and nMDS analyses were carried out grouping the OTUs by main taxa groups to levels commonly used in automated image scanning in systems such as ZooSCAN/Ecotaxa (Gorsky et al., 2010; Picheral et al., 2017), and following the classification used from images carried out from BATS data by Ivory et al. (2018).
Results
Hydrography
The observed seasonality of the water column has been previously described for the BATS station in several publications (e.g., Steinberg et al., 2001; and references therein; Lomas et al., 2013). Briefly, temperatures showed deep convective mixing early in the year (winter and early spring), moving to a strong summer stratification in surface for July, August and September, with deepening of the mixed layer later in the year (November and December) leading to the deep mixing during winter (Figure 1). During October 2015, Hurricane Joaquin passed near Bermuda (missing data in the graphs) and likely produce surface mixing. No data was registered from the BATS program due to the associated rough weather conditions, and profiles were obtained from Mid-Atlantic Glider Initiative & Collaboration (MAGIC5) program (R. Curry, unpublished), revealing abrupt mixing of the column after the passage of Joaquin (Figure 1). Data gaps early in both years correspond to scheduled shipyard repairs for the RV Atlantic Explorer. CTD fluorescence showed higher concentrations during spring bloom in late February (i.e., winter), with some intermittent smaller peaks during the year between 70 and 100 m depth. When considering all casts made during each BATS cruise, the fluorescence peaks roughly follows the measured Chl-a (Figure 2) or Phaeopigments (Turner fluorometer; see BATS methods). MLD showed a patter similar to those already described for BATS (e.g., Church et al., 2013), with a deeper layer in winter, abruptly shallowing in spring and summer and gradually deepening during the fall despite the hurricane (Figure 2). Primary production showed maximum peaks in late winter/early spring and August, with a minimum during the fall (Figure 2) also in agreement with previously published observations for BATS (e.g., Steinberg et al., 2001).
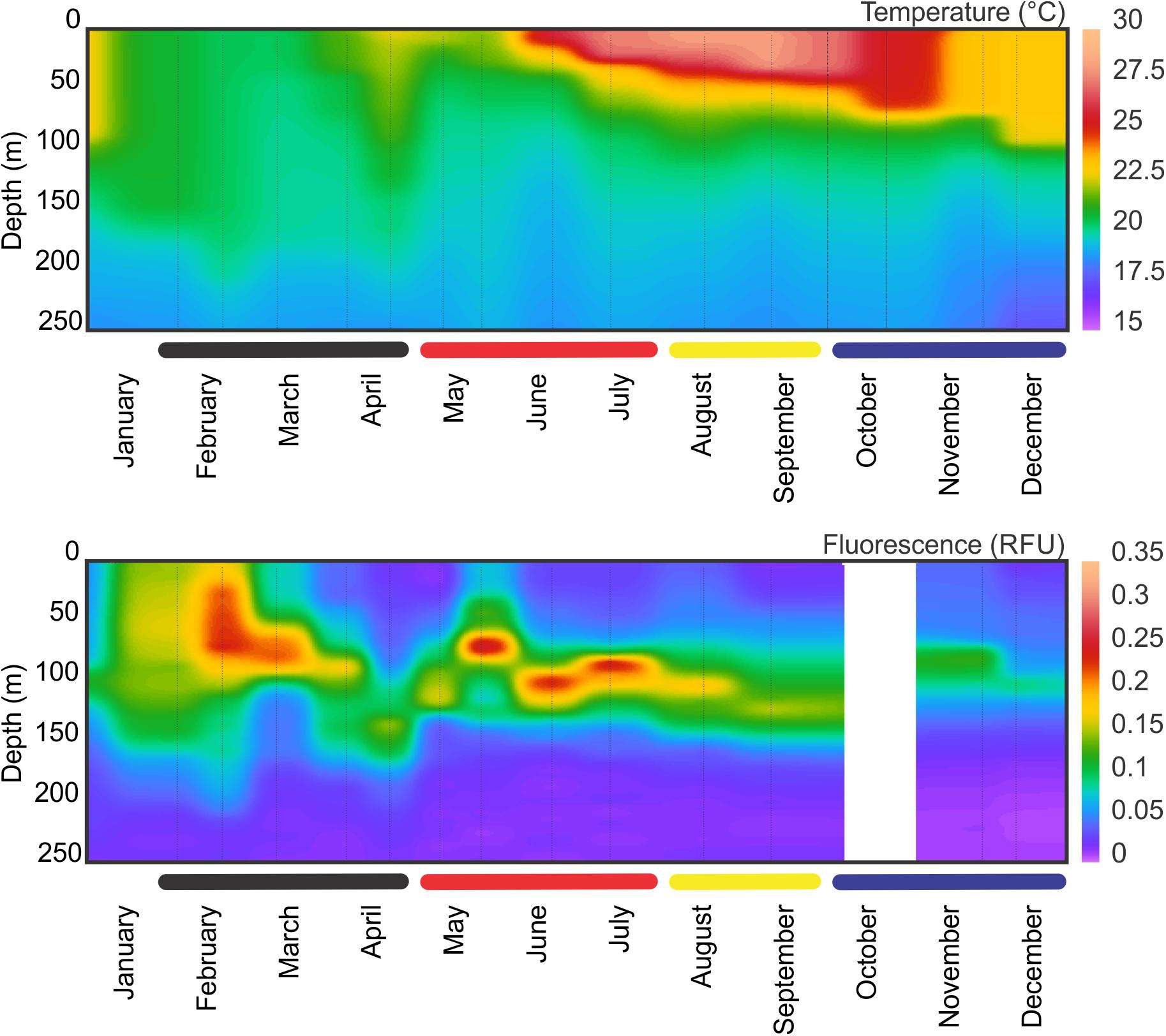
Figure 1. CTD temperature and fluorescence profiles at BATS station during 2015. Thin vertical lines indicate sampling events. Data is missing for October due to the Hurricane Joaquin. For temperature, two extra profiles were obtained from glider data (MAGIC program, BIOS) to give a better coverage between the last CTD sampling before the hurricane and the one after.
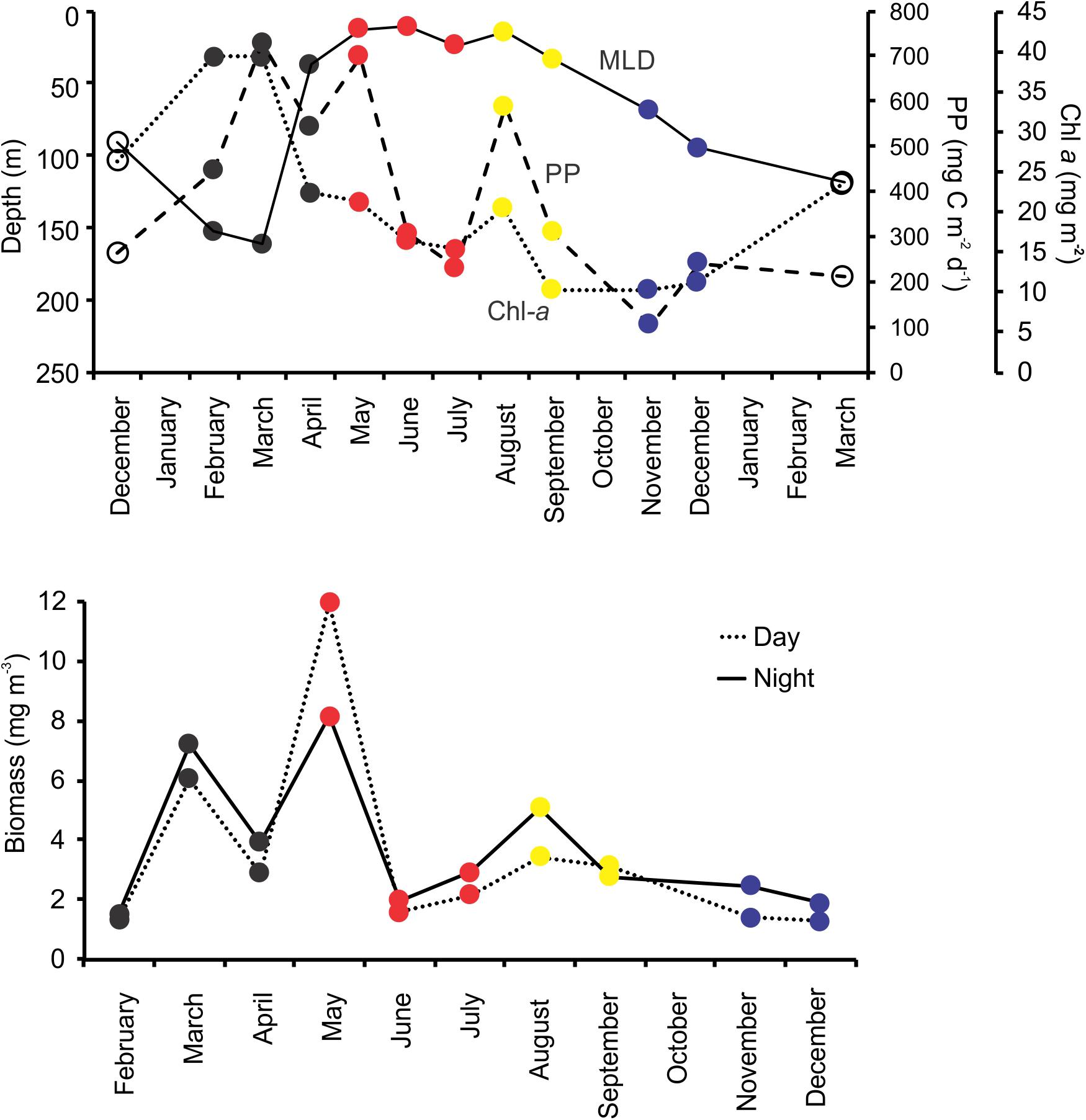
Figure 2. Upper panel shows the depth of the mixed layer depth (MLD), total Chl-a per square meter, and total primary production (PP) per square meter during BATS core cruises. Lower panel depicts the day and night dry biomass of zooplankton (mg per cubic meter) during the same cruises. Marker colors correspond to the four zooplankton community regimes as established based on the community composition (see section “Results”).
Zooplankton biomass showed maximum values in March, May and August, with night values being higher for monthly pairs with the exception the May and September (Figure 2 and Supplementary Table S2 in Supplementary File S1). In general, each fraction followed the same common trend, with similar maximum and minimum relative values.
Laboratory and Bioinformatics
All samples succeeded in producing DNA, and amplification success was 100%. A total of 7,199,536 reads were produced, of which 5,144,471 passed quality control and chimera search, with 141,553 unique sequences. After clustering at 2 bp difference, 20,114 metazoan OTUs remained, accounting for 3,781,379 reads. The remaining reads belonged to other Eukaryotes OTUs (SAR, unclassified, etc.) and were not included in downstream analyses. Raw data is provided in an excel file including OTU abundances per sample, OTU taxonomic assignment and OTU representative sequence (Supplementary File S2).
Replicability
The test for replicability (raw counts; without any prefiltering) showed very strong agreement between both pairs of samples submitted from different amplification and sequencing batches using different illumina index (for both pairs Pearson ρ > 0.99; p < 0.001). The general trends in the most abundant groups were kept. Abundances for rare taxa (abundances below 0.5%), showed in some cases discrepancies, a behavior commonly observed in metabarcoding (Leray and Knowlton, 2017), despite the technical procedures carried out to minimize this effect (large amount of template, low number of cycles and pooling of three independent PCR reactions).
Effects of Sequencing Depth and Prefiltering on Diversity Estimates
Diversity analyses without prefiltering or standardization (3,781,379 reads in 20,114 OTUs) showed insufficient sampling (with the exception of a few samples such as 20313D), and the extrapolated curves indicated 1239 to 3890 OTUs per sample (Figure 3). The measured number of OTUs showed a higher dependence of the number of reads available to any other factor such as Day/Night or seasonality (Pearson correlation r = 0.96, p < 0.001). The extrapolated to 350,000 reads showed a worse correlation to the number of reads (r = 0.51, p < 0.01) and the interpolated (rarefied) to 36,471 reads showed no correlation to the number of reads per sample (r = 0.04, p > 0.85). Furthermore, the OTU numbers obtained from the rarified dataset were the less variable of the three comparisons (Figure 4 and Supplementary Figure S1 from Supplementary File S2).
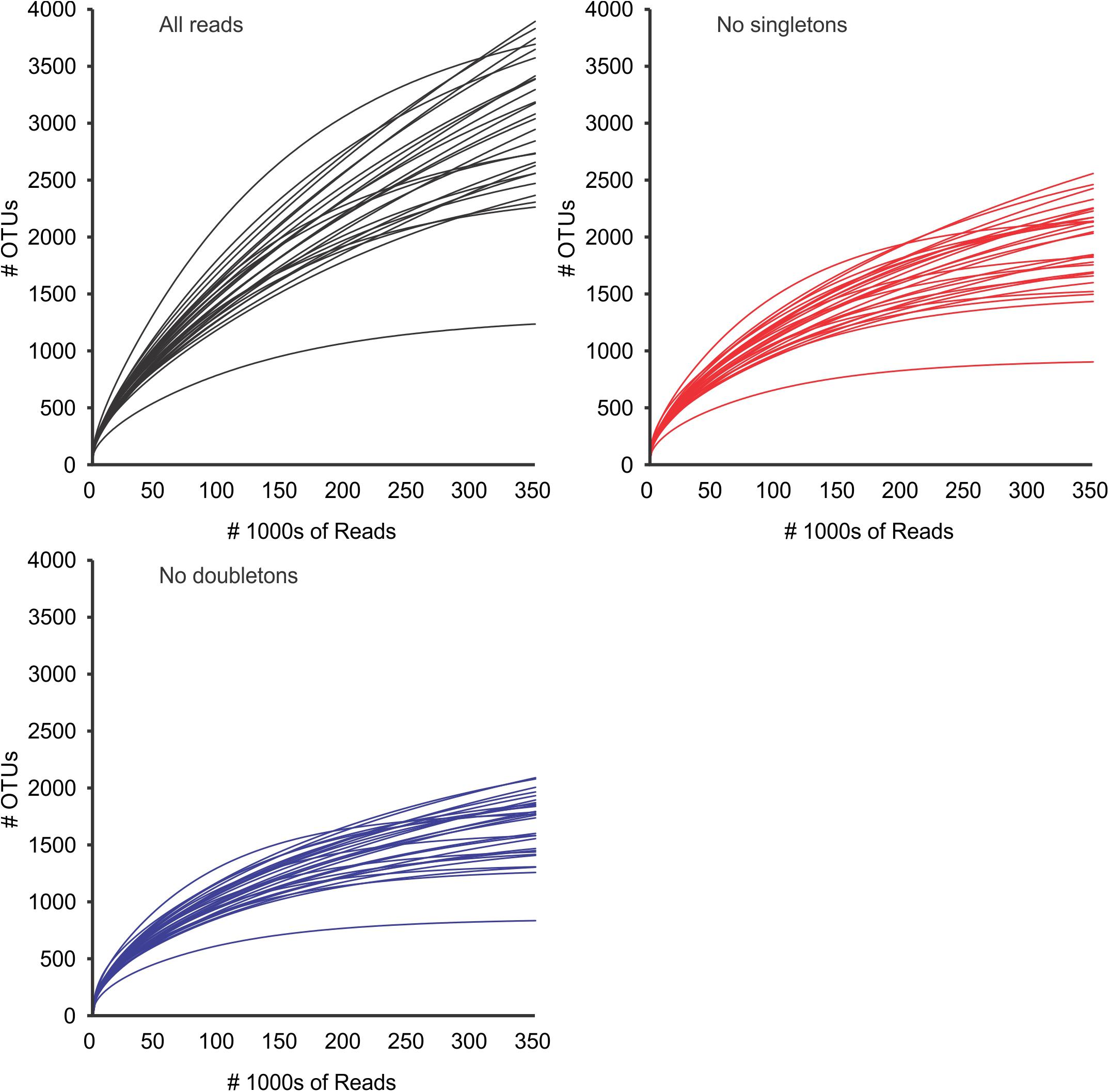
Figure 3. OTU accumulation curves for all samples, using all reads (black), and after removal of global singletons (red) and doubletons (blue). Curves represent values extrapolated to 350,000 reads per sample (see methods). After removal of doubletons, most samples appeared to have reached a quasi-asymptotic profile, that would indicate that all OTUs had been detected. Standard deviations (after 100 repetitions) were too small to be seen in the graph. SD (not represented) were always ≤ 5% of the value.
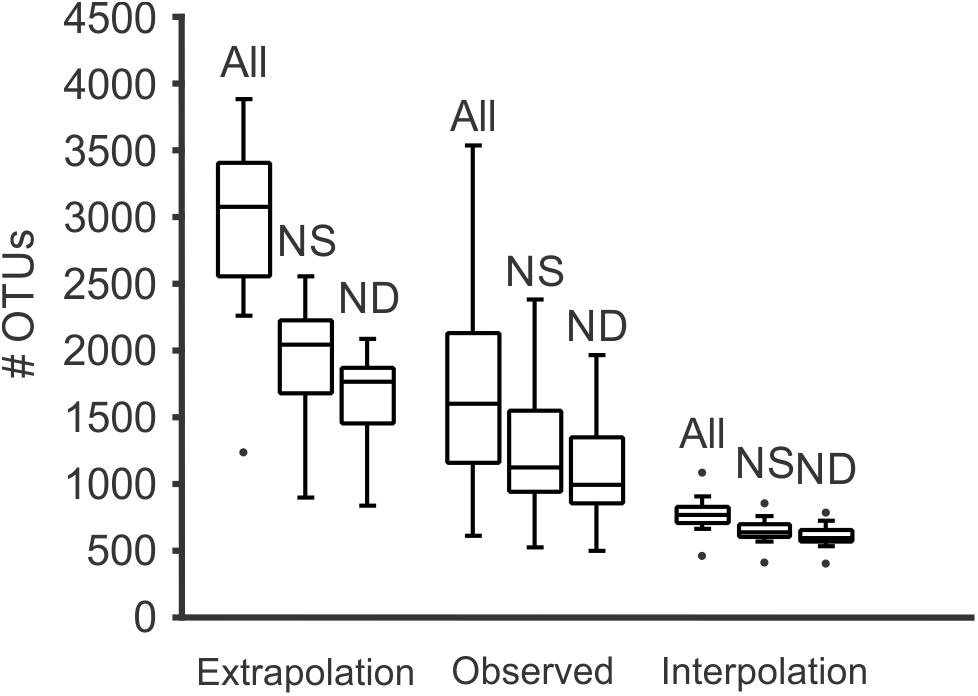
Figure 4. Box and whiskers plot representing the number of OTUs detected for all samples, for both the number of reads obtained for each sample (observed), extrapolated to 350,000 reads (near the maximum of reads obtained for a single sample, and interpolated to the minimum number of reads obtained for a sample (equivalent to a rarefaction). At each of those levels, it is shown the effect of removals of global singletons (NS) and singletons and doubletons (ND). Meanwhile the effect of singletons and doubletons is clear in those samples with more reads, if standardized to the minimum number of reads per sample, the effect is minimal and all samples contained a similar number of OTUs.
After removal of singletons (3,768,816 reads, 7,551 OTUs) many species accumulation curves still did not show attenuation, although many more curves did show attenuation compared to the original data (Figure 3). Further removal of doubletons (3,763,740 reads, 5,013 OTUs) did not significantly improve much (i.e., increased saturation) the species accumulation curves nor mitigate the species detection dependency on the number of reads per sample. In all cases, the variability of OTUs detected per sample was much lower in the interpolation to the minimum number of reads compared to the observed or extrapolated. Based on the interpolated data, more taxonomic units were detected in the night samples compared to the corresponding day samples (paired t-test, p < 0.05), however there was no seasonal pattern in the number of units detected (Supplementary Figure S1 in Supplementary File S2).
Standardized Analyses
All samples were reduced to 36,471 reads per sample and only core BATS cruises were considered. Taxonomically, calanoid copepods overwhelmingly dominated the community in all samples, representing 45–65% of the community (Figure 5). No other of the taxa groups considered reached values over 13% in any case. At this major taxa group level, no clear seasonal pattern was appreciated, with proportions remaining more or less constant.
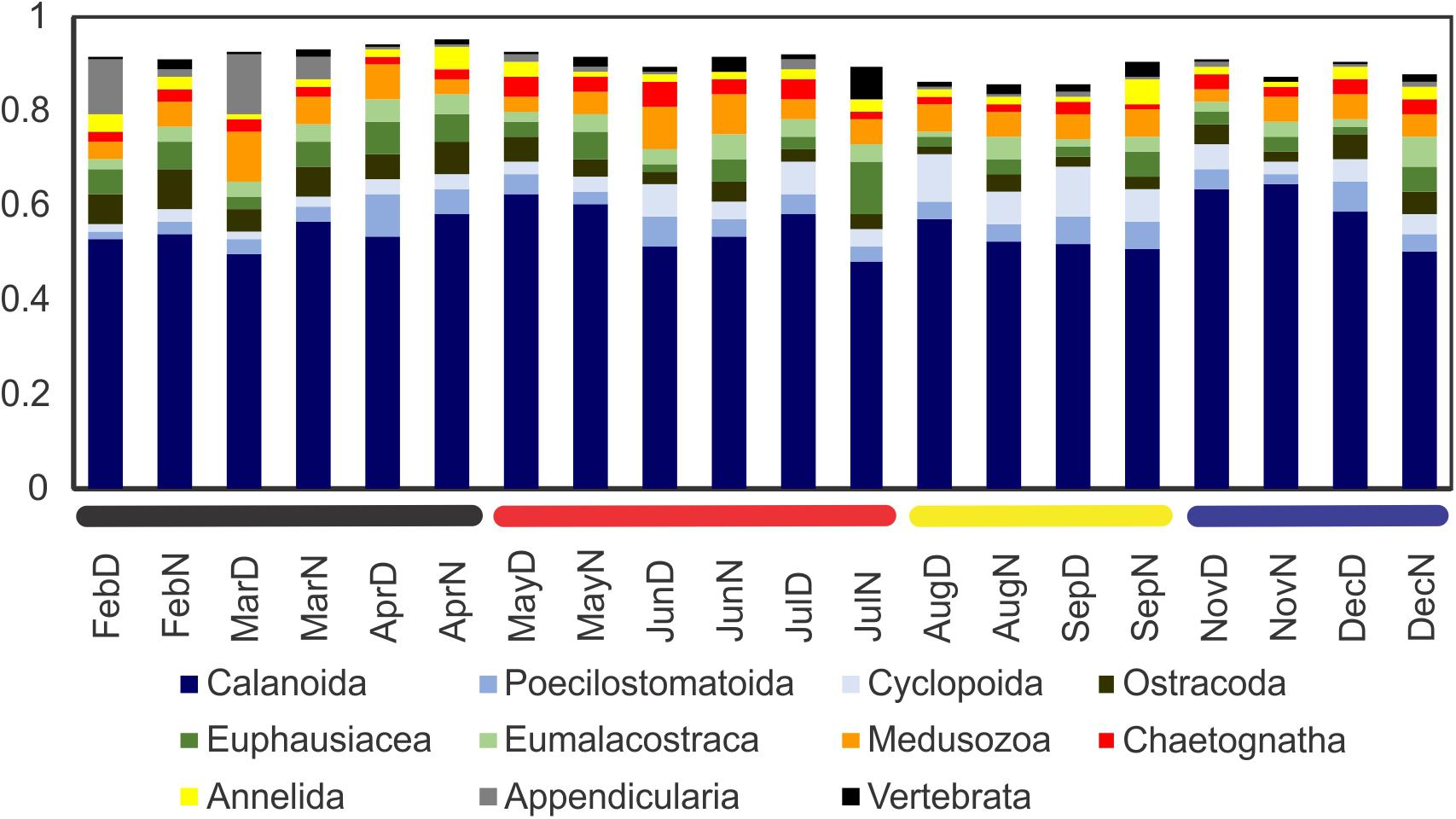
Figure 5. Proportion of the most abundant large taxa groups (those achieving 5% of the reads per sample at least once during 2015). The community was dominated by calanoid copepods, with secondary taxa more variable throughout the year. Horizontal bars in the base of the graph correspond to the zooplankton community regimes as established based on the community composition (see results).
After building the Bray–Curtis similarity matrix, a nMDS analyses on the non-transformed data showed little seasonality pattern, however day/night samples were separated to a certain degree, showing statistically significant grouping by this factor despite the partial mixing (ANOSIM R = 0.289, p < 0.005; Figure 6). The taxa that contributed most (up to 25%) to the differences between day and night samples, were typically OTUs with a higher number of reads, in particular OTUs 1, 2, 3, 5, 11, and 7 (Table 2). They contributed more than 4% each to the dissimilarity, accounting for up to 35% of the total dissimilarity. GenBank BLAST analyses indicated that their identity would correspond to (1) the Family Calanidae (Copepoda, Calanoida; several genera and species of the family shared the same V9 region), (2) Pleuromamma spp. (Gaussia princeps shares this sequence, it is however unlikely due to its meso-bathy-abyssopelagic habitat; Metridia does not share this sequence), (3) the Euchaetidae or Aetideidae; (5) unknown members of the Superfamily Clausocalanoidea, (11) the Superfamily Eucalanoidea (sensu Blanco-Bercial et al., 2011; again, the V9 region is common to several genera within this superfamily, across families) and (7) the Family Euphausiidae (Order Euphausiacea; several genera of the shared this sequence) respectively (Table 2). Contribution to the dissimilarity progressively decreased for the other OTUs, and only 20 contribute more than 1% (Supplementary Table S3 in Supplementary File S1).
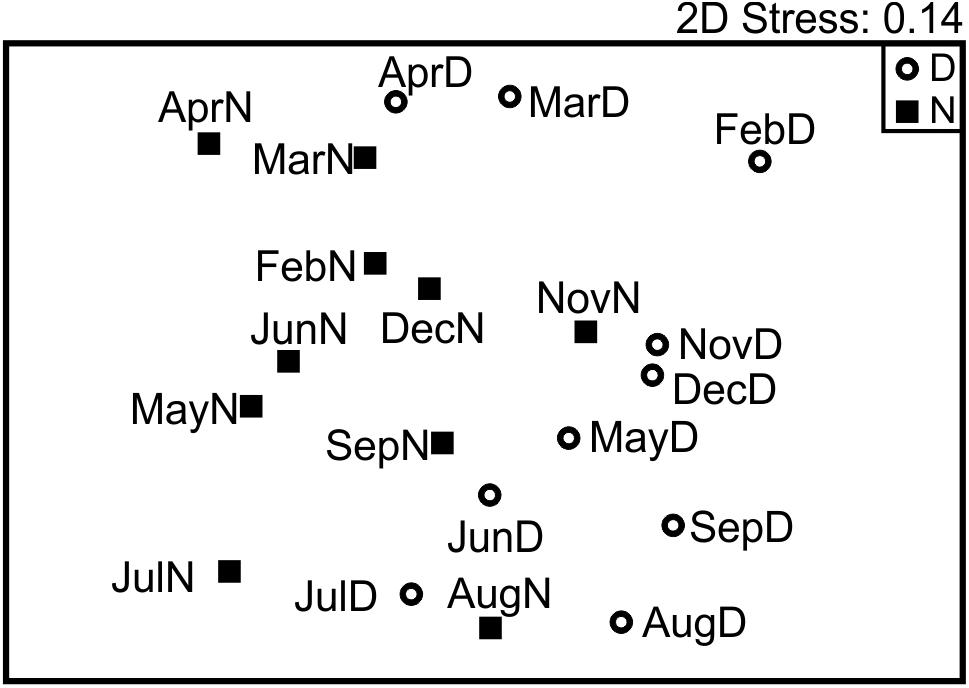
Figure 6. Non-metric multidimensional scaling representing the similarity between all samples base in community composition (relative abundances). Day versus night grouping was statistically supported; however, no seasonal pattern was detected.
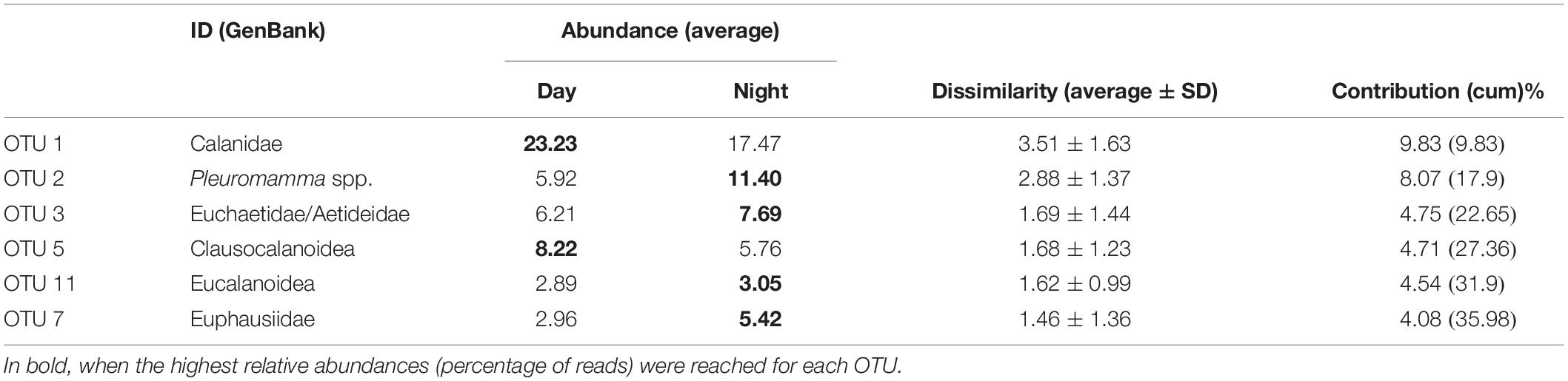
Table 2. OTUs contributing the most to the difference between day and night samples (average dissimilarity = 35.71).
After standardization by the total and square root transformation, K-R Clustering showed that the maximum improvement in R occurred between 3 and 4 clusters (Figure 7A). The defined groups coincided with the four defined clusters by hierarchical group average method (Figure 7B). The nMDS analyses reflected a seasonal cycle based in those four clusters (Figure 7C), corresponding to seasonal hydrographic regimes: winter deep mixing and bloom (February to April; spring bloom happened in February, Figure 1), spring (May to July; coincident with the largest zooplankton biomass), summer stratification (August and September) and fall mixing event (November and December; post-hurricane Joaquin). Environmental variables (MLD, PP and Chla) showed high correlation with the nMDS ordination and grouping (Pearson ρ = 0.458; p < 0.005). ANOSIM analyses showed high significance for the four groups (p < 0.001) meanwhile it resulted in non-significant grouping of Day/Night (p > 0.25). A mantel test comparing the community composition with measured vertical particle flux measured at BATS (RELATE procedure) showed a statistical correlation between both similarity matrices (ρ = 0.348; p < 0.001). A direct correlation between zooplankton biomass (measured during the day, during the night, or the average of both) did not result in significant correlations when compared with the exported total mass. The raw data for the vertical flux can be found in the Supplementary File S1 in Supplementary Table S4.
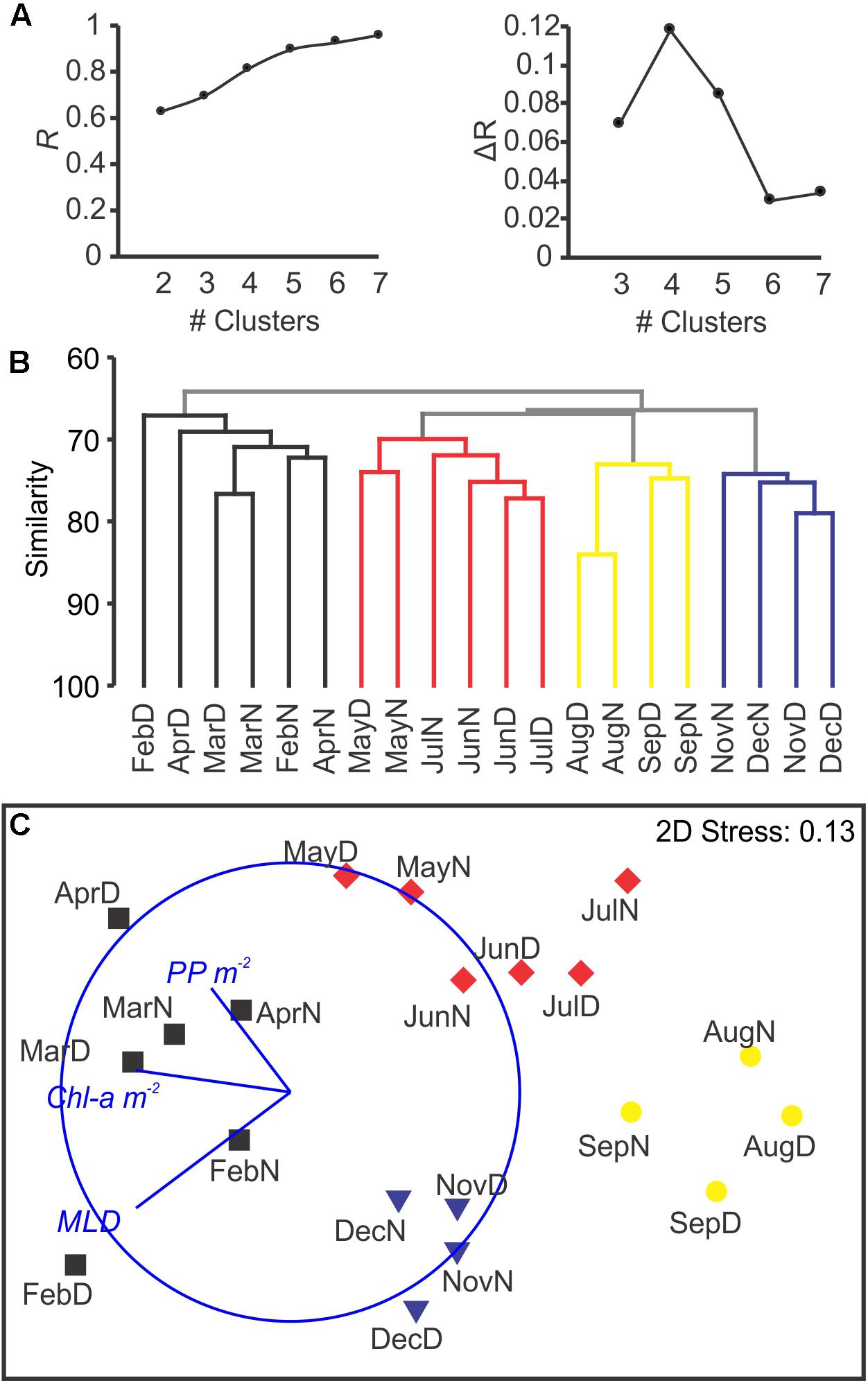
Figure 7. Clustering and nMDS after Hellinger transformation, based on Bray–Curtis similarity. The k-R non-hierarchical clustering shows the maximum increment in the R statistic at K = 4, with R > 0.8 (A). The addition of subsequent clusters had a smaller effect on the R, and did not result in ecologically meaningful clusters. In (B), group average clustering showed four clusters (in colors) matching those detected by the non-hierarchical clustering. In (C), the nMDS organized the samples reflecting the seasonality based in the community composition. Colors match the clusters detected by the previous approaches (A,B). The overlay represents Pearson correlations between the three environmental parameters and the spatial ordination of the samples.
SIMPER analyses between the four groups (Table 3) indicate that the transitions between seasons were due to many OTUs, each contributing at the most 1 to 2% to the dissimilarity between seasons (detailed results in Supplementary Table S5 in Supplementary File S1). Taxonomic assignment by BLAST gave a wide range of IDs, from several phyla, covering both holoplankton to meroplankton. The deep mixing and spring bloom to late spring transition was marked by the increase of Eucalanoidea (2 OTUs) and Calanidae copepods, with a decrease of winter taxa such as appendicularians, euphausiidae and Pleuromamma sp. Toward the summer, Poecilostomatoid copepods, and Acartia longiremis increased their relative abundances, meanwhile during the fall there is an increase of an Eumalacostraca and an OTU belonging to the Euchaetidae/Aetideidae clade. The transition to the winter is again characterized by increases in an appendicularian, Pleuromamma sp. and Euphausiidae, and a decrease in the groups dominant in summer and fall. Similarity within groups were caused mostly by the most abundant OTUs (especially 1, 2, 3, 5, 6, 7, 9, and 11). Some other taxa were, however, typical of only one or two of the groups, such as Poecilostomatoid (OTU 18) for the summer and the unknown Eumalacostraca (OTU 15) in the fall. In case of Eucalanoidea, it was possible to appreciate a gradual decrease in their relative contribution to the groups, with a maximum in spring, decreasing toward the summer and fall.
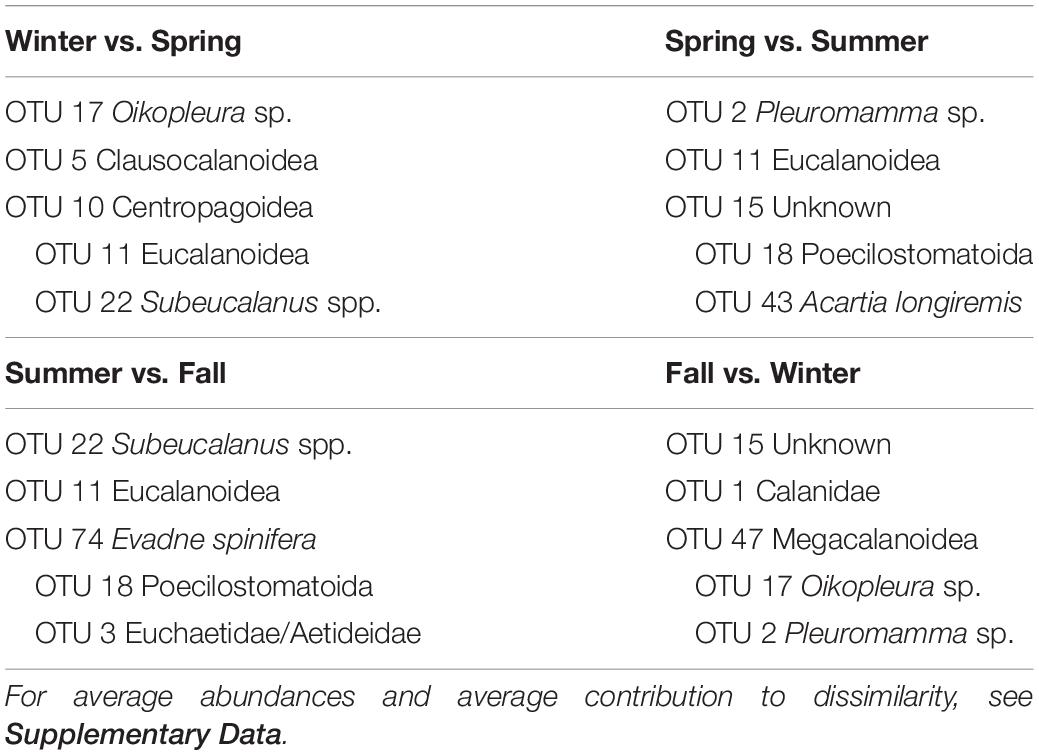
Table 3. OTUs contributing the most to the dissimilarity between consecutive pairs of seasonal zooplankton regimens.
Grouping the OTUs by main taxa groups following the classification used from images carried out from BATS data (Ivory et al., 2018) resulted in a lack of ability to detect seasonality. Samples analyzed this way were clustered into three groups, one containing summer (June to September) samples and the other two the rest of them. Day/night pairs were, however, mixed within groups, and even same-month day/night samples fell in separate groups (Supplementary Figure S2 in Supplementary File S2).
Discussion
This manuscript shows how seasonality influences the mesozooplankton assemblage at the BATS site, indicating the presence of four distinct community regimes. Although some previous works have described certain (or all) components of the community in detail (Deevey, 1971; Deevey and Brooks, 1971, 1977; Stone and Steinberg, 2014) or organized by main taxonomic groups (Ivory et al., 2018), none of the previous described any shifts in the seasonal patterns at community level. These seasonal regimes were characterized by changes in the relative abundances of both key and secondary players of the community, with only a smaller influence based on the fluctuations of the dominant players of the community. The four-community pattern described here is similar to those described for temperate oceans (responding to hydrographic conditions), and it is more clear than when only biomass (e.g., Madin et al., 2001; this study) is considered. In particular, at least during the year 2015 (analyzed here) the maxima and minima biomass values are mixed within a statistical cluster; both in total biomass and for each size fraction, considering just day or night, or as a monthly measurement. While some of the community shifts are predictable based on a priori understanding of the ecosystem (linked, for example, to the end of the mixing of the water column to the stratified conditions), the more gradual transitions (spring/early summer to summer, and fall to winter) would not be obvious without this detailed biodiversity data. Furthermore, for the very rapid winter to spring transition (represented by the formation of shallow stratification), there was a month of delay in the zooplankton response, showing an apparent disconnect between hydrography and zooplankton community composition. This lag would be due to the known delay in the response of the zooplankton, attributed to the longer generation times compared to phytoplankton (Ceballos and Álvarez-Marqués, 2006).
The presence of these four community regimens would also represent changes in the functional profile of the community. The clearest example was the shift from the deep-mixing and spring bloom communities to the late spring community. This was typified by a shift from an early spring bloom community, dominated by of omnivorous/carnivorous (Pleuromamma sp., euphausiids), and appendicularians, small filter feeders able to feed in even very small phytoplankton and respond rapidly to increased resources due to their short life cycle (Acuña et al., 1995; Fernández and Acuña, 2003; Blanco-Bercial et al., 2006; Lobón et al., 2013), to a Calanidae and Eucalanoidea dominated one, specialized in feeding in larger phytoplankton, during the late bloom period.
Omnivorous species (e.g., Pleuromamma or euphausiids), able to respond faster to the spring bloom, decreased their relative abundances in late spring, with an increased relevance of Calanidae (likely Nannocalanus minor s.l., Mesocalanus tenuicornis s.l. and Neocalanus spp.; a family tightly linked to phytoplankton blooms worldwide) and Eucalanoidea, likely with a slower response to the increased phytoplankton biomass. This switch came, however, well after the yearly maximum of Chl-a (February and March). This suggests a delay in the response of the phytoplankton specialists and an advantage in this region for taxa with a wider prey spectrum, which might be able to maintain larger populations under the low resource conditions of fall and winter. The fact that community relative composition alone (without any biomass or total abundance implicit) was also a better predictor for vertical flux than zooplankton biomass also suggests a significant role of the community composition on the oceanic biogeochemistry. All these results would place the zooplankton community dynamics at BATS closer to those typical from temperate oceans than to subtropical oligotrophic gyres, including the seasonality signal correlated with flux. The seasonality in the flux (and in the flux attenuation) was already known from this region (Helmke et al., 2010; Church et al., 2013); the described zooplankton cycle might contribute to explain these cycles and the difference between BATS and other locations with a weaker seasonality such as HOT or ESTOC. Further investigations, using both field and modeling approaches, should help connecting these observations.
Although a deep analysis of functional biodiversity profiles was not done (see below about limitations of this study), it is known that functional analyses might be able to perceive more subtleties when characterizing community dynamics (Pomerleau et al., 2015), which could subsequently affect relevant processes such as carbon flux (Steinberg et al., 2008). If the identified community regimes have a relevant impact on such processes, as the correlation between flux and seasonal groupings suggests, identification of the functional profiles and analysis of their role in biogeochemical cycling, is something that should be addressed in future research.
These observed seasonal changes do not, however, affect the general profile of the community based on main taxa grouping or for the most dominant taxa, which stay dominant throughout the year (with small changes in the relative abundances). Nor do they correlate with the diversity of the community (at least the indices calculate here). Most OTUs with a relatively significant presence were detected in all samples; cases in which an OTU would be a significant part of the community (more than 1%) for only part of the year were non-existent. These results would support the hypothesis that the community has been deeply sampled (deeper than could be attained with classical microscope counts), without missing OTU due to undersampling, and that changes in relative abundances would be based in balancing changes involving many taxa, with very little final influence on the average diversity of the community.
The inability to detect the same groups, or even well-defined groups, when biodiversity was assessed by main taxa groups (similar to detection levels achieved by other methods such as scanning and machine-aided identification), highlights the power of the metabarcoding approach despite the known existing limitations (see below). This should not be interpreted as any method being better or worse, but as a need to integrate several approaches to gain a full understanding of the processes in the zooplankton community. Total biomass, particle size profiles and real abundances, etc., will be enriched by the power of metabarcoding to detect changes in the community ecology.
Limitations and Capabilities of 18S V9 Metabarcoding
When performing any study, the limitations of the approach should be always carefully considered, and metabarcoding is not an exception (Creer et al., 2010; Pilgrim et al., 2011; Bucklin et al., 2016). The most commonly questioned or criticized facet of the metabarcoding is how quantitative metabarcoding is (if at all). Reviewed recently by Lamb et al. (2019), there is in general a correlation between number of reads and biomass, although with large uncertainties. In the present study, the top 10 OTUs would correspond to taxa or clades that are known to be among the most dominant at the BATS site (Deevey, 1971; Deevey and Brooks, 1977; Steinberg et al., 2000), however no morphological counts were done for the same samples. It is also important to remember that metabarcoding only provides an equivalent to relative abundances/biomass. In our case, if weighing the relative abundances by the biomass for each month and day/night, it was observed that the total maximum peaks for several of those taxa would correspond with the sample of maximum biomass (May; 10314 cruise), and not the maximum of relative abundance (figure not shown). Finding the best way to transform these metabarcoding counts into real abundances/biomass should be one of the priorities for the whole scientific community.
In the pre-processing steps, the most critical step appeared to be the rarefaction to the minimum number of reads. This process has the associated problem of loss of information (Colwell et al., 2012; Chao et al., 2014), however it increased the differences between samples, biasing diversity toward those with more original reads. If this is an effect of artificial inflation during sequencing is something to be studied in the future. The removal of singletons had a stronger effect within the extrapolated data, while the additional removal of doubletons was minimal, especially in the interpolated dataset. All these results suggest that number of taxa were not be inflated due to methodological errors (that would have been marked by a more dramatic decrease in OTUs when removing singletons and doubletons). The approach in this manuscript is very conservative, and our clustering (theoretically) just removed PCR errors, and was equivalent to a 100% similarity matrix after de-noising (some OTUs are still 1 bp different, since they were the centroids of their respective error-derived cluster of reads). It did not, however, result in an excessively high final number of OTUs for the region, considering existing literature. One of the advantage of the V9 region is its short length (∼130 bp), therefore a complete overlapping between F and R reads was obtained. Discarding those pairs or reads that did not completely agree with a Q > 30 might have helped removing PCR and sequencing errors, and likely dropped chimeras. Both are known to be factors that might be causing the apparent inflation in the number of taxonomic units in metabarcoding studies.
Another limitation in this particular study is the taxonomic resolution of the V9 region. Despite the fact that it has been widely used for community characterization in the zooplankton (Amaral-Zettler et al., 2009; de Vargas et al., 2015; Abad et al., 2017), its ability to resolve species is somewhat limited in zooplankton (Wu et al., 2015). For the V9 alone, even identification at the genus level would have only an 80% success for Copepoda (Wu et al., 2015). A similar problem was also detected for euphausiids in the present manuscript (where several species and genera shared the same sequence), and for pteropods (Stewart et al., 2018), suggesting it would likely be for all taxonomic groups, if studied in detail. For Copepoda, it can also be observed that the resolution is not equal across the phylogenetic tree. Within the order Calanoida, the oldest planktonic families, Arietelloidea (= Augaptiloidea Blanco-Bercial et al., 2011) and Diaptomoidea showed good resolution to genus and even to the species level (e.g., the several Pleuromamma OTUs; or the Centropages sp. detected). If considering at the most modern superfamilies, whose planktonic radiation happened more recently (Bradford-Grieve, 2002), the same V9 is shared by whole families (Calanidae) or even across several families within the most modern superfamily, the Clausocalanoidea. This is a widespread issue (Albaina et al., 2016; Abad et al., 2017), and this asymmetry of the resolution would influence estimates of diversity, with a closer accuracy in areas where the Arietelloidea or Diaptomoidea are dominant groups (e.g., the mesopelagic or coastal environments, respectively) compared to niches where the other superfamilies are more common (e.g., the epipelagic open ocean). In the BATS region, Pleuromamma spp. and Lucicutia spp. (Metridinidae and Lucicutiidae; Arietelloidea) are significant contributors to the zooplankton biomass (Deevey and Brooks, 1977; Schnetzer and Steinberg, 2002), however the other superfamilies also contribute significantly to the community (pers. obs.; Deevey and Brooks, 1977). In terms of total number of species, 241 copepod OTUs passed the different thresholds in this study. Compared to morphological analyses, 326 species were found by Deevey and Brooks (1977) from the surface to 2000 m depth, 128 of those in the epipelagic, although they acknowledge many more (especially small ones) were not identified and were left at the genus level. A similar number of morphological species has been obtained (159 species) during summer 2016 in the upper 200 m at BATS (Blanco-Bercial and Maas, unpublished), where likely a higher species-level resolution was attained compared to Deevey and Brooks (1977). The higher numbers obtained in this metabarcoding analysis could be attributed to the fact that a whole year was sampled. Furthermore, in the metabarcoding approach, all of the sample is analyzed, while in the morphological counts a subsampling of some kind is needed in order to make the counting feasible. It would be also possible that, in fact, metabarcoding would be detecting more of the rare community, which in the Sargasso Sea it makes a significant fraction of the zooplankton. Considering the difficulty in attaining species-level ID between congeneric groups in this oceanic region, the expected number of species could easily double that number (around 500 species). That would still not be out of scale, considering the high diversity of this area and the understudied non-calanoid copepods. For the subtropical NW Atlantic, at least 713 named copepod species have been indicated (Razouls et al., 2005–2019). Although many would not be distributed in the Sargasso Sea, most of them could potentially be present in the samples analyzed here. More accordance was found in pteropods (but a very complete reference was available from Burridge et al., 2017) or in Ostracods, when metabarcoding identified 110 OTUs for ∼120 species described for the subtropical North Atlantic (Angel et al., 2008). Considering these three groups, this dataset does not seem to have inflated the existing diversity, and might be relatively well-representing the community composition.
Understanding the limits (and the capabilities) of metabarcoding is essential to make use of its full potential, while avoiding over interpretation of the results. The final goal of the metabarcoding should not to be viewed as the complete and perfect description of the community. Lacking complete databases, and working with a marker that is neither able to discriminate among nor efficiently amplify all species, among others, are limitations that, at least at present, seem unavoidable. These limitations, and many others, have been discussed in several reviews (Creer et al., 2010; Pilgrim et al., 2011; Bucklin et al., 2016). In the present study, however, metabarcoding effectively revealed community regimes, with correlation to environment and biogeochemical processes, providing a step toward a better understanding of ecological succession and processes in the Sargasso Sea zooplankton, and allowing for the development of new working hypothesis regarding the zooplankton community at the BATS site. Many potential studies are furthermore possible based on this dataset; e.g., biological interactions (Lima-Mendez et al., 2015; Stewart et al., 2018), in depth studies in relation to biogeochemical cycles (Guidi et al., 2016), among others. The equivalent analyses of the zooplankton community, done by classical methods (morphology), would have needed a much larger effort (time-wise) and several experts while the faster imaging (zooSCAN or similar) analyses do not provide the same kind of information. It is time to incorporate metabarcoding as a standard procedure in time-series, environmental monitoring, and regular research, not as a substitute of, but as a complement to, the present methods used in biological oceanographic research.
Data Availability Statement
The datasets generated for this study can be found in the NCBI BioProject 540654, accessible at https://www.ncbi.nlm.nih.gov/bioproject/PRJNA540654. All scripts used in this study can be found in the github repository https://github.com/blancobercial/BATS_zooplankton. Data from the Bermuda Atlantic Time-Series Study analyzed in this study can be found at BATS data repository http://bats.bios.edu/bats-data/.
Author Contributions
The author confirms being the sole contributor of this work and has approved it for publication.
Conflict of Interest
The author declares that the research was conducted in the absence of any commercial or financial relationships that could be construed as a potential conflict of interest.
Funding
This research was partially funded by the Simon’s Foundation International as part of the BIOSSCOPE project (http://scope.bios.edu/), as well as startup funding from the Bermuda Institute of Ocean Sciences to LB-B.
Acknowledgments
Special thanks are given to Samiah Alam for her help in the initial development of the extraction protocol; to the Bermuda Atlantic Time-series Study program (BATS; NSF OCE-1756105) and BATS personnel for their logistical support at sea; to Ruth Curry (MAGIC lab http://magic.bios.edu/) for providing hydrographic data corresponding to the passage of Hurricane Joaquin; to the Captains and crew of the RV Atlantic Explorer; and to Amy Maas for English proofreading of the manuscript. This research made use of the FCMMF laboratory equipment at BIOS (NSF DBI-1522206). This is contribution #282, Bermuda Biodiversity Project (BBP), Bermuda Aquarium, Museum and Zoo, Department of Environment & Natural Resources.
Supplementary Material
The Supplementary Material for this article can be found online at: https://www.frontiersin.org/articles/10.3389/fmars.2020.00173/full#supplementary-material
Footnotes
- ^ batsftp.bios.edu/
- ^ http://bats.bios.edu
- ^ http://blog.mothur.org/2018/01/10/SILVA-v132-reference-files/
- ^ https://github.com/blancobercial/BATS_zooplankton
- ^ http://magic.bios.edu/
References
Abad, D., Albaina, A., Aguirre, M., and Estonba, A. (2017). 18S V9 metabarcoding correctly depicts plankton estuarine community drivers. Mar. Ecol. Prog. Ser. 584, 31–43. doi: 10.3354/meps12373
Abad, D., Albaina, A., Aguirre, M., Laza-Martínez, A., Uriarte, I., Iriarte, A., et al. (2016). Is metabarcoding suitable for estuarine plankton monitoring? A comparative study with microscopy. Mar. Biol. 163:149. doi: 10.1007/s00227-016-2920-0
Acuña, J. L., Bedo, A. W., Harris, R. P., and Anadón, R. (1995). The Seasonal Succession of Appendicularians (Tunicata: Appendicularia) off Plymouth. J. Mar. Biol. Assoc. U.K. 75, 755–758. doi: 10.1017/S0025315400039187
Ahn, J.-H., Kim, B.-Y., Song, J., and Weon, H.-Y. (2012). Effects of PCR cycle number and DNA polymerase type on the 16S rRNA gene pyrosequencing analysis of bacterial communities. J. Microbiol. 50, 1071–1074. doi: 10.1007/s12275-012-2642-z
Aird, D., Ross, M., Chen, W.-S., Danielsson, M., Fennell, T., Russ, C., et al. (2011). Analyzing and minimizing PCR amplification bias in Illumina sequencing libraries. Genome Biol. 12:R18. doi: 10.1186/gb-2011-12-2-r18
Albaina, A., Aguirre, M., Abad, D., Santos, M., and Estonba, A. (2016). 18S rRNA V9 metabarcoding for diet characterization: a critical evaluation with two sympatric zooplanktivorous fish species. Ecol. Evol. 6, 1809–1824. doi: 10.1002/ece3.1986
Amaral-Zettler, L. A., McCliment, E. A., Ducklow, H. W., and Huse, S. M. (2009). A method for studying protistan diversity using massively parallel sequencing of V9 hypervariable regions of small-subunit ribosomal RNA genes. PLoS One 4:e6372. doi: 10.1371/journal.pone.0006372
Angel, M. V. (1979). Studies on Atlantic halocyprid ostracods: their vertical distributions and community structure in the central gyre region along latitude 30°N from off Africa to Bermuda. Prog. Oceanogr. 8, 3–124. doi: 10.1016/0079-6611(79)90009-0
Angel, M. V., Blachowiak-Samolyk, K., and Chavtur, V. (2008). Atlas of Atlantic Planktonic Ostracods. Available online at: http://www.nhm.ac.uk/research-curation/scientific-resources/biodiversity/global-biodiversity/atlantic-ostracods/ (accessed December 1, 2018).
Beaugrand, G., Reid, P. C., Ibañez, F., Alister, J. L., and Edwards, M. (2002). Reorganization of North Atlantic copepod biodiversity and climate. Science 296, 1692–1694. doi: 10.1126/science.1071329
Blanco-Bercial, L., Alvarez-Marques, F., and Cabal, J. A. (2006). Changes in the mesozooplankton community associated with the hydrography off the northwestern Iberian Peninsula. ICES J. Mar. Sci. 63, 799–810. doi: 10.1016/j.icesjms.2006.03.007
Blanco-Bercial, L., Bradford-Grieve, J., and Bucklin, A. (2011). Molecular phylogeny of the Calanoida (Crustacea: Copepoda). Mol. Phylogenet. Evol. 59, 103–113. doi: 10.1016/j.ympev.2011.01.008
Bradford-Grieve, J. M. (2002). Colonization of the pelagic realm by calanoid copepods. Hydrobiologia 485, 223–244.
Bucklin, A., Lindeque, P. K., Rodriguez-Ezpeleta, N., Albaina, A., and Lehtiniemi, M. (2016). Metabarcoding of marine zooplankton: prospects, progress and pitfalls. J. Plankton Res. 38, 393–400. doi: 10.1093/plankt/fbw023
Bucklin, A., Ortman, B. D., Jennings, R. M., Nigro, L. M., Sweetman, C. J., Copley, N. J., et al. (2010). A “Rosetta Stone” for metazoan zooplankton: DNA barcode analysis of species diversity of the Sargasso Sea (Northwest Atlantic Ocean). Deep Sea Res. Part II Top. Stud. Oceanogr. 57, 2234–2247. doi: 10.1016/j.dsr2.2010.09.025
Bucklin, A., Yeh, H. D., Questel, J. M., Richardson, D. E., Reese, B., Copley, N. J., et al. (2019). Time-series metabarcoding analysis of zooplankton diversity of the NW Atlantic continental shelf. ICES J. Mar. Sci. 76, 1162–1176. doi: 10.1093/icesjms/fsz021
Burd, A. B., Hansell, D. A., Steinberg, D. K., Anderson, T. R., Arístegui, J., Baltar, F., et al. (2010). Assessing the apparent imbalance between geochemical and biochemical indicators of meso- and bathypelagic biological activity: What the @$# is wrong with present calculations of carbon budgets? Deep Sea Res. Part II Top. Stud. Oceanogr. 57, 1557–1571. doi: 10.1016/j.dsr2.2010.02.022
Burridge, A. K., Hörnlein, C., Janssen, A. W., Hughes, M., Bush, S. L., Marlétaz, F., et al. (2017). Time-calibrated molecular phylogeny of pteropods. PLoS One 12:e0177325. doi: 10.1371/journal.pone.0177325
Carlson, C. A., Ducklow, H. W., and Michaels, A. F. (1994). Annual flux of dissolved organic carbon from the euphotic zone in the northwestern Sargasso Sea. Nature 371, 405–408. doi: 10.1038/371405a0
Carlson, C. A., Morris, R., Parsons, R., Treusch, A. H., Giovannoni, S. J., and Vergin, K. (2009). Seasonal dynamics of SAR11 populations in the euphotic and mesopelagic zones of the northwestern Sargasso Sea. ISME J. 3, 283–295. doi: 10.1038/ismej.2008.117
Carroll, M. L., Miquel, J.-C., and Fowler, S. W. (1998). Seasonal patterns and depth-specific trends of zooplankton fecal pellet fluxes in the Northwestern Mediterranean Sea. Deep Sea Res. Part I Oceanogr. Res. Pap. 45, 1303–1318. doi: 10.1016/S0967-0637(98)00013-2
Ceballos, S., and Álvarez-Marqués, F. (2006). Seasonal dynamics of reproductive parameters of the calanoid copepods Calanus helgolandicus and Calanoides carinatus in the Cantabrian Sea (SW Bay of Biscay). Prog. Oceanograp. 70, 1–26. doi: 10.1016/j.pocean.2006.03.002
Chao, A., Gotelli, N. J., Hsieh, T. C., Sander, E. L., Ma, K. H., Colwell, R. K., et al. (2014). Rarefaction and extrapolation with Hill numbers: a framework for sampling and estimation in species diversity studies. Ecol. Monogr. 84, 45–67. doi: 10.1890/13-0133.1
Chao, A., Ma, K. H., and Hsieh, T. C. (2018). iNEXT (iNterpolation and EXTrapolation). Available online at: https://chao.shinyapps.io/iNEXTOnline/ (accessed June 1, 2018).
Chiba, S., Batten, S., Martin, C. S., Ivory, S., Miloslavich, P., and Weatherdon, L. V. (2018). Zooplankton monitoring to contribute towards addressing global biodiversity conservation challenges. J. Plankton Res. 40, 509–518. doi: 10.1093/plankt/fby030
Church, M. J., Lomas, M. W., and Muller-Karger, F. (2013). Sea change: charting the course for biogeochemical ocean time-series research in a new millennium. Deep Sea Res. Part II Top. Stud. Oceanogr. 93, 2–15. doi: 10.1016/j.dsr2.2013.01.035
Colwell, R. K., Chao, A., Gotelli, N. J., Lin, S.-Y., Mao, C. X., Chazdon, R. L., et al. (2012). Models and estimators linking individual-based and sample-based rarefaction, extrapolation and comparison of assemblages. J. Plant Ecol. 5, 3–21. doi: 10.1093/jpe/rtr044
Conte, M. H., Ralph, N., and Ross, E. H. (2001). Seasonal and interannual variability in deep ocean particle fluxes at the Oceanic Flux Program (OFP)/Bermuda Atlantic Time Series (BATS) site in the western Sargasso Sea near Bermuda. Deep Sea Res. Part II Top. Stud. Oceanogr. 48, 1471–1505. doi: 10.1016/S0967-0645(00)00150-8
Corell, J., and Rodríguez-Ezpeleta, N. (2014). Tuning of protocols and marker selection to evaluate the diversity of zooplankton using metabarcoding. Rev. Invest. Mar. AZTI Tecnalia 21, 19–39.
Creer, S., Fonseca, V. G., Porazinska, D. L., Giblin-Davis, R. M., Sung, W., Power, D. M., et al. (2010). Ultrasequencing of the meiofaunal biosphere: practice, pitfalls and promises. Mol. Ecol. 19, 4–20. doi: 10.1111/j.1365-294X.2009.04473.x
de Vargas, C., Audic, S., Henry, N., Decelle, J., Mahé, F., Logares, R., et al. (2015). Eukaryotic plankton diversity in the sunlit ocean. Science 348:1261605. doi: 10.1126/science.1261605
Deevey, G. B. (1968). Pelagic ostracods of the Sargasso Sea off Bermuda: description of species and seasonal and vertical distribution. Peaboy Mus. Nat. His. Yale Univ. Bull. 26, 1–125.
Deevey, G. B. (1971). The annual cycle in quantity and composition of the zooplankton of the Sargasso Sea off Bermuda. I. The upper 500 m1. Limnol. Oceanogr. 16, 219–240. doi: 10.4319/lo.1971.16.2.0219
Deevey, G. B., and Brooks, A. L. (1971). The annual cycle in quantity and composition of the zooplankton of the Sargasso Sea off Bermuda. II. The surface to 2,000 m1. Limnol. Oceanogr. 16, 927–943. doi: 10.4319/lo.1971.16.6.0927
Deevey, G. B., and Brooks, A. L. (1977). Copepods of the Sargasso Sea off Bermuda: Species Composition, and Vertical and Seasonal Distribution between the Surface and 2000 M. Bull. Mar. Sci. 27, 256–291.
Eden, B. R., Steinberg, D. K., Goldthwait, S. A., and McGillicuddy, D. J. (2009). Zooplankton community structure in a cyclonic and mode-water eddy in the Sargasso Sea. Deep Sea Res Part I Oceanogr. Res. Pap. 56, 1757–1776. doi: 10.1016/j.dsr.2009.05.005
Edgar, R. C., Haas, B. J., Clemente, J. C., Quince, C., and Knight, R. (2011). UCHIME improves sensitivity and speed of chimera detection. Bioinformatics 27, 2194–2200. doi: 10.1093/bioinformatics/btr381
Fernández, D., and Acuña, J. L. (2003). Enhancement of marine phytoplankton blooms by appendicularian grazers. Limnol. Oceanogr. 48, 587–593. doi: 10.4319/lo.2003.48.2.0587
Fonseca, V. G., Carvalho, G. R., Sung, W., Johnson, H. F., Power, D. M., Neill, S. P., et al. (2010). Second-generation environmental sequencing unmasks marine metazoan biodiversity. Nat. Commun. 1:98. doi: 10.1038/ncomms1095
Gonzalez, J. M., Portillo, M. C., Belda-Ferre, P., and Mira, A. (2012). Amplification by PCR artificially reduces the proportion of the rare biosphere in microbial communities. PLoS One 7:e29973. doi: 10.1371/journal.pone.0029973
Gorsky, G., Ohman, M. D., Picheral, M., Gasparini, S., Stemmann, L., Romagnan, J.-B., et al. (2010). Digital zooplankton image analysis using the ZooScan integrated system. J. Plankton Res. 32, 285–303. doi: 10.1093/plankt/fbp124
Grice, G. D., and Hart, A. D. (1962). The abundance, seasonal occurrence and distribution of the Epizooplankton between New York and Bermuda. Ecol. Monogr. 32, 287–309. doi: 10.2307/1942377
Guidi, L., Chaffron, S., Bittner, L., Eveillard, D., Larhlimi, A., Roux, S., et al. (2016). Plankton networks driving carbon export in the oligotrophic ocean. Nature 532, 465–470. doi: 10.1038/nature16942
Hannides, C. C. S., Popp, B. N., Close, H. G., Benitez-Nelson, C. R., Ka’apu-Lyons, C. A., Gloeckler, K., et al. (2020). Seasonal dynamics of midwater zooplankton and relation to particle cycling in the North Pacific Subtropical Gyre. Prog. Oceanogr. 182:102266. doi: 10.1016/j.pocean.2020.102266
Harris, V., Olhede, S. C., and Edwards, M. (2015). Multidecadal spatial reorganisation of plankton communities in the North East Atlantic. J. Mar. Syst. 142, 16–24. doi: 10.1016/j.jmarsys.2014.09.002
Hebert, P. D., Cywinska, A., Ball, S. L., and deWaard, J. R. (2003). Biological identifications through DNA barcodes. Proc. R. Soc. B Biol. Sci. 270, 313–321. doi: 10.1098/rspb.2002.2218
Helmke, P., Neuer, S., Lomas, M. W., Conte, M., and Freudenthal, T. (2010). Cross-basin differences in particulate organic carbon export and flux attenuation in the subtropical North Atlantic gyre. Deep Sea Res. Part I Oceanogr. Res. Pap. 57, 213–227. doi: 10.1016/j.dsr.2009.11.001
Hirai, J., and Tsuda, A. (2015). Metagenetic community analysis of epipelagic planktonic copepods in the tropical and subtropical Pacific. Mar. Ecol. Prog. Ser. 534, 65–78. doi: 10.3354/meps11404
Hirai, J., Kuriyama, M., Ichikawa, T., Hidaka, K., and Tsuda, A. (2015). A metagenetic approach for revealing community structure of marine planktonic copepods. Mol. Ecol. Resour. 15, 68–80. doi: 10.1111/1755-0998.12294
Hsieh, T. C., Ma, K. H., and Chao, A. (2016). iNEXT: an R package for rarefaction and extrapolation of species diversity (Hill numbers). Methods Ecol. Evol. 7, 1451–1456. doi: 10.1111/2041-210x.12613
Ivory, J. A., Steinberg, D. K., and Latour, R. J. (2018). Diel, seasonal, and interannual patterns in mesozooplankton abundance in the Sargasso Sea. ICES J. Mar. Sci. 76, 217–231. doi: 10.1093/icesjms/fsy117
Knap, A. H., Michaels, A. F., Steinberg, D., Bahr, F., Bates, N., Bell, S., et al. (1997). BATS Methods Manual. Woods Hole, MA: U.S. JGOFS Planning Office.
Lamb, P. D., Hunter, E., Pinnegar, J. K., Creer, S., Davies, R. G., and Taylor, M. I. (2019). How quantitative is metabarcoding: a meta-analytical approach. Mol. Ecol. 28, 420–430. doi: 10.1111/mec.14920
Legendre, P., and Gallagher, E. D. (2001). Ecologically meaningful transformations for ordination of species data. Oecologia 129, 271–280. doi: 10.1007/s004420100716
Leray, M., and Knowlton, N. (2017). Random sampling causes the low reproducibility of rare eukaryotic OTUs in Illumina COI metabarcoding. PeerJ 5:e3006. doi: 10.7717/peerj.3006
Levitus, S. (1982). Climatological Atlas of the World Ocean, NOAA/ERL GFDL Professional Paper 13. (Rockville, MD: U.S. Gov. Printing Office), 173.
Lima-Mendez, G., Faust, K., Henry, N., Decelle, J., Colin, S., Carcillo, F., et al. (2015). Determinants of community structure in the global plankton interactome. Science 348:1262073. doi: 10.1126/science.1262073
Lindeque, P. K., Parry, H. E., Harmer, R. A., Somerfield, P. J., and Atkinson, A. (2013). Next generation sequencing reveals the hidden diversity of zooplankton assemblages. PLoS One 8:e81327. doi: 10.1371/journal.pone.0081327
Lobón, C. M., Bouquet, J.-M., Reeve, M., Novac, A., Acuña, J. L., Thompson, E. M., et al. (2013). Response of the pelagic tunicate appendicularian, Oikopleura dioica to controlled simulations of a strong bloom condition: a bottom-up perspective. Limnol. Oceanogr. 58, 215–226. doi: 10.1111/mec.15321
Lomas, M. W., Bates, N. R., Johnson, R. J., Knap, A. H., Steinberg, D. K., and Carlson, C. A. (2013). Two decades and counting: 24-years of sustained open ocean biogeochemical measurements in the Sargasso Sea. Deep Sea Res. Part II Top. Stud. Oceanogr. 93, 16–32. doi: 10.1016/j.dsr2.2013.01.008
Maas, A. E., Blanco-Bercial, L., Lo, A., Tarrant, A. M., and Timmins-Schiffman, E. (2018). Variations in copepod proteome and respiration rate in association with diel vertical migration and circadian cycle. Biol. Bull. 235, 30–42. doi: 10.1086/699219
Mackas, D. L., Greve, W., Edwards, M., Chiba, S., Tadokoro, K., Eloire, D., et al. (2012). Changing zooplankton seasonality in a changing ocean: Comparing time series of zooplankton phenology. Prog. Oceanogr. 97–100, 31–62. doi: 10.1016/j.pocean.2011.11.005
Madin, L. P., Horgan, E. F., and Steinberg, D. K. (2001). Zooplankton at the Bermuda Atlantic Time-series Study (BATS) station: diel, seasonal and interannual variation in biomass, 1994-1998. Deep Sea Res. II 48, 2063–2082. doi: 10.1016/s0967-0645(00)00171-5
Mayzaud, P., and Pakhomov, E. A. (2014). The role of zooplankton communities in carbon recycling in the Ocean: the case of the Southern Ocean. J. Plankton Res. 36, 1543–1556. doi: 10.1093/plankt/fbu076
Moore, H. B. (1949). The zooplankton of the upper waters of the Bermuda area of the North Atlantic. Bull. Bingham Oceanogr. Collect. 12, 1–97.
Picheral, M., Colin, S., and Irisson, J.-O. (2017). EcoTaxa, a Tool for the Taxonomic Classification of Images. Available online at: http://ecotaxa.obs-vlfr.fr
Pilgrim, E. M., Jackson, S. A., Swenson, S., Turcsanyi, I., Friedman, E., Weigt, L., et al. (2011). Incorporation of DNA barcoding into a large-scale biomonitoring program: opportunities and pitfalls. J. North Am. Benthol. Soc. 30, 217–231. doi: 10.1899/10-012.1
Pomerleau, C., Sastri, A. R., and Beisner, B. E. (2015). Evaluation of functional trait diversity for marine zooplankton communities in the Northeast subarctic Pacific Ocean. J. Plankton Res. 37, 712–726. doi: 10.1093/plankt/fbv045
Quast, C., Pruesse, E., Yilmaz, P., Gerken, J., Schweer, T., Yarza, P., et al. (2013). The SILVA ribosomal RNA gene database project: improved data processing and web-based tools. Nucleic Acids Res. 41, D590–D596. doi: 10.1093/nar/gks1219
Razouls, C., de Bovée, F., Kouwenberg, J., and Desreumaux, N. (2005–2019). Diversity and Geographic Distribution of Marine Planktonic Copepods. Available online at: http://copepodes.obs-banyuls.fr/en (accessed April 02, 2019).
Rombouts, I., Beaugrand, G., Ibañez, F., Gasparini, S., Chiba, S., and Legendre, L. (2010). A multivariate approach to large-scale variation in marine planktonic copepod diversity and its environmental correlates. Limnol. Oceanogr. 55, 2219–2229. doi: 10.4319/lo.2010.55.5.2219
Schlitzer, R. (2017). Ocean Data View. Available online at: https://odv.awi.de
Schloss, P. D., Westcott, S. L., Ryabin, T., Hall, J. R., Hartmann, M., Hollister, E. B., et al. (2009). Introducing mothur: open-source, platform-independent, community-supported software for describing and comparing microbial communities. Appl. Environ. Microbiol. 75, 7537–7541. doi: 10.1128/aem.01541-09
Schnetzer, A., and Steinberg, D. K. (2002). Active transport of particulate organic carbon and nitrogen by vertically migrating zooplankton in the Sargasso Sea. Mar. Ecol. Prog. Ser. 234, 71–84. doi: 10.3354/meps234071
Sommer, S. A., Van Woudenberg, L., Lenz, P. H., Cepeda, G., and Goetze, E. (2017). Vertical gradients in species richness and community composition across the twilight zone in the North Pacific Subtropical Gyre. Mol. Ecol. 26, 6136–6156. doi: 10.1111/mec.14286
Steinberg, D. K., and Landry, M. R. (2017). Zooplankton and the Ocean Carbon Cycle. Annu. Rev. Mar. Sci. 9, 413–444. doi: 10.1146/annurev-marine-010814-015924
Steinberg, D. K., Carlson, C. A., Bates, N. R., Goldthwait, S. A., Madin, L. P., and Michaels, A. F. (2000). Zooplankton vertical migration and the active transport of dissolved organic and inorganic carbon in the Sargasso Sea. Deep Sea Res. Part I Oceanogr. Res. Pap. 47, 137–158. doi: 10.1016/S0967-0637(99)00052-7
Steinberg, D. K., Carlson, C. A., Bates, N. R., Johnson, R. J., Michaels, A. F., and Knap, A. H. (2001). Overview of the US JGOFS Bermuda Atlantic Time-series Study (BATS): a decade-scale look at ocean biology and biogeochemistry. Deep Sea Res. Part II Top. Stud. Oceanogr. 48, 1405–1447. doi: 10.1016/S0967-0645(00)00148-X
Steinberg, D. K., Cope, J. S., Wilson, S. E., and Kobari, T. (2008). A comparison of mesopelagic mesozooplankton community structure in the subtropical and subarctic North Pacific Ocean. Deep Sea Res. Part II Top. Stud. Oceanogr. 55, 1615–1635. doi: 10.1016/j.dsr2.2008.04.025
Steinberg, D. K., Lomas, M. W., and Cope, J. S. (2012). Long-term increase in mesozooplankton biomass in the Sargasso Sea: Linkage to climate and implications for food web dynamics and biogeochemical cycling. Glob. Biogeochem. Cycles 26, GB1004. doi: 10.1029/2010gb004026
Stewart, L. M., Blanco-Bercial, L., and Maas, A. E. (2018). “Beyond biodiversity: metabarcoding as a tool of ecological exploration,” in Proceedings of the 2018 Ocean Sciences Meeting, Portland, OR.
Stone, J. P., and Steinberg, D. K. (2014). Long-term time-series study of salp population dynamics in the Sargasso Sea. Mar. Ecol. Prog. Ser. 510, 111–127. doi: 10.3354/meps10985
Stone, J. P., and Steinberg, D. K. (2016). Salp contributions to vertical carbon flux in the Sargasso Sea. Deep Sea Res. Part I Oceanogr. Res. Pap. 113, 90–100. doi: 10.1016/j.dsr.2016.04.007
Taberlet, P., Coissac, E., Pompanon, F., Brochmann, C., and Willerslev, E. (2012). Towards next-generation biodiversity assessment using DNA metabarcoding. Mol. Ecol. 21, 2045–2050. doi: 10.1111/j.1365-294X.2012.05470.x
Westcott, S. L., and Schloss, P. D. (2017). OptiClust, an improved method for assigning amplicon-based sequence data to operational taxonomic units. mSphere 2:e00073-17. doi: 10.1128/mSphereDirect.00073-17
Wexels Riser, C., Wassmann, P., Olli, K., Pasternak, A., and Arashkevich, E. (2002). Seasonal variation in production, retention and export of zooplankton faecal pellets in the marginal ice zone and central Barents Sea. J. Mar. Syst. 38, 175–188. doi: 10.1016/S0924-7963(02)00176-8
Wiebe, P. H., Copley, N. J., and Boyd, S. H. (1992). Coarse-scale horizontal patchiness and vertical migration of zooplankton in Gulf Stream warm-core ring 82-H. Deep Sea Res. Part A Oceanogr. Res. Pap. 39, S247–S278. doi: 10.1016/S0198-0149(11)80015-4
Wu, S., Xiong, J., and Yu, Y. (2015). Taxonomic resolutions based on 18S rRNA Genes: a case study of subclass Copepoda. PLoS One 10:e0131498. doi: 10.1371/journal.pone.0131498
Keywords: zooplankton, metabarcoding, Sargasso Sea, Bermuda Atlantic Time-series Study, seasonality
Citation: Blanco-Bercial L (2020) Metabarcoding Analyses and Seasonality of the Zooplankton Community at BATS. Front. Mar. Sci. 7:173. doi: 10.3389/fmars.2020.00173
Received: 21 November 2019; Accepted: 05 March 2020;
Published: 24 March 2020.
Edited by:
Ciro Rico, The University of the South Pacific, FijiReviewed by:
Ulisses Miranda Azeiteiro, University of Aveiro, PortugalPablo Henrique dos Santos Picapedra, Universidade Estadual do Oeste do Paraná, Brazil
Akash Sastri, Department of Fisheries and Oceans (Canada), Canada
Copyright © 2020 Blanco-Bercial. This is an open-access article distributed under the terms of the Creative Commons Attribution License (CC BY). The use, distribution or reproduction in other forums is permitted, provided the original author(s) and the copyright owner(s) are credited and that the original publication in this journal is cited, in accordance with accepted academic practice. No use, distribution or reproduction is permitted which does not comply with these terms.
*Correspondence: Leocadio Blanco-Bercial, bGVvY2FkaW9AYmlvcy5lZHU=