- 1Skidaway Institute of Oceanography, University of Georgia, Savannah, GA, United States
- 2Division of Marine Science, School of Ocean Science and Engineering, The University of Southern Mississippi, Stennis Space Center, MS, United States
- 3Dauphin Island Sea Lab, University of South Alabama, Dauphin Island, AL, United States
- 4Institute of Environment, Florida International University, Miami, FL, United States
- 5Department of Ocean & Mechanical Engineering, Florida Atlantic University, Boca Raton, FL, United States
- 6Harbor Branch Oceanographic Institute, Florida Atlantic University, Fort Pierce, FL, United States
- 7U.S. Naval Research Laboratory, Stennis Space Center, MS, United States
- 8Bureau of Ocean Energy Management, New Orleans, LA, United States
- 9School of Marine Science & Policy, University of Delaware, Lewes, DE, United States
Observing multiple size classes of organisms, along with oceanographic properties and water mass origins, can improve our understanding of the drivers of aggregations, yet acquiring these measurements remains a fundamental challenge in biological oceanography. By deploying multiple biological sampling systems, from conventional bottle and net sampling to in situ imaging and acoustics, we describe the spatial patterns of different size classes of marine organisms (several microns to ∼10 cm) in relation to local and regional (m to km) physical oceanographic conditions on the Delaware continental shelf. The imaging and acoustic systems deployed included (in ascending order of target organism size) an imaging flow cytometer (CytoSense), a digital holographic imaging system (HOLOCAM), an In Situ Ichthyoplankton Imaging System (ISIIS, 2 cameras with different pixel resolutions), and multi-frequency acoustics (SIMRAD, 18 and 38 kHz). Spatial patterns generated by the different systems showed size-dependent aggregations and differing connections to horizontal and vertical salinity and temperature gradients that would not have been detected with traditional station-based sampling (∼9-km resolution). A direct comparison of the two ISIIS cameras showed composition and spatial patchiness changes that depended on the organism size, morphology, and camera pixel resolution. Large zooplankton near the surface, primarily composed of appendicularians and gelatinous organisms, tended to be more abundant offshore near the shelf break. This region was also associated with high phytoplankton biomass and higher overall organism abundances in the ISIIS, acoustics, and targeted net sampling. In contrast, the inshore region was dominated by hard-bodied zooplankton and had relatively low acoustic backscatter. The nets showed a community dominated by copepods, but they also showed high relative abundances of soft-bodied organisms in the offshore region where these organisms were quantified by the ISIIS. The HOLOCAM detected dense patches of ciliates that were too small to be captured in the nets or ISIIS imagery. This near-simultaneous deployment of different systems enables the description of the spatial patterns of different organism size classes, their spatial relation to potential prey and predators, and their association with specific oceanographic conditions. These datasets can also be used to evaluate the efficacy of sampling techniques, ultimately aiding in the design of efficient, hypothesis-driven sampling programs that incorporate these complementary technologies.
Introduction
Accurate measurements of size and abundance of organisms and particles are fundamental to process-oriented research in biological oceanography (Blanchard et al., 2017). Size correlates with many ecological properties of plankton and nekton and is a “taxa-transcending trait” that plays a fundamental role in ecosystem structure in both marine and terrestrial realms (Andersen et al., 2016; Kiørboe et al., 2018; Woodson et al., 2018). For example, size dictates which prey are available for consumption and influences metabolic rates. In general, marine prey are 0.1–1.0% of the mass of a predator (Jennings et al., 2001; Kiørboe, 2008); however, there are many exceptions where predators (or something to a similar effect, like parasites) are smaller (e.g., Wakabayashi et al., 2012; Peacock et al., 2014; Feunteun et al., 2018) or orders of magnitude larger (Sutherland et al., 2010; Henschke et al., 2016; Conley et al., 2018; Dadon-Pilosof et al., 2019) than their prey. Despite the widely accepted importance of size in affecting marine ecosystem functioning, measurement of size distributions from micro-organisms to nektonic animals is challenging due to limitations or taxonomic biases for various sampling gears (Cowen et al., 2013; Skjoldal et al., 2013; Wiebe et al., 2017). In addition, the data generated from coarse sampling gears, such as net tows, are not easily associated with the spatial scales of oceanographic variability from m to km that may structure the abundances of different organism sizes classes.
In situ imaging systems represent one method of assessing size distributions with high spatial resolution, while providing taxonomic identifications to family or genus level (in most cases). These systems, when compared to net-based or station-based sampling, have been demonstrated to mitigate biases related to organism fragility (Greer et al., 2014, 2018; Luo et al., 2014; Biard et al., 2016), patchiness or fine-scale changes in abundance (Davis and McGillicuddy, 2006; Greer et al., 2016), and size or swimming speed of the organisms of interest (Cowen et al., 2013; Parra et al., 2019). Some imaging systems have shown consistency in comparison to acoustically-derived abundances, particularly for more durable taxa, such as shrimps, chaetognaths, and copepods (Trevorrow et al., 2005; Whitmore et al., 2019). These comparisons are more uncertain when they include gelatinous organisms (Båmstedt et al., 2003).
Describing the degree of patchiness accurately for different biological constituents is key for assessing various biological rates (Letcher and Rice, 1997; Davis and McGillicuddy, 2006; Priyadarshi et al., 2019) and trophic interactions (Benoit-Bird and McManus, 2012; Greer et al., 2016; Schmid et al., 2020) that can also affect marine ecosystem structure, production, and biodiversity (Woodson and Litvin, 2015; Woodson et al., 2018; Priyadarshi et al., 2019). Often the high-resolution systems describing this patchiness, because of their technical complexity and various stages of instrument development, are used in isolation or with more conventional oceanographic sampling methods (CTD, plankton nets, Niskin bottle sampling, etc.). However, there has been a recent push to describe and integrate the observations from these different platforms into a cohesive framework that will enhance our understanding of plankton dynamics (Lombard et al., 2019).
Imaging systems use a variety of lighting techniques, such as strobes [e.g., Video Plankton Recorder (Davis et al., 2005) and the Underwater Vision Profiler (UVP, Picheral et al., 2010)] or back-lit shadowgraphs, such as the Shadowed Image Particle Profiling and Evaluation Recorder (SIPPER, Samson et al., 2001), the In Situ Ichthyoplankton Imaging System (ISIIS, Cowen and Guigand, 2008), and ZooGlider (Ohman et al., 2019). The shadowgraph imagers, and the ISIIS in particular, quantify the larger size range of planktonic organisms (Wiebe et al., 2017; Lombard et al., 2019). Two or more optical systems can be directly compared, such as the Pelagic In Situ Observing System (PELAGIOS) and the UVP (Hoving et al., 2019) or the Optical Plankton Counter to the SIPPER (Remsen et al., 2004), to reveal the size classes or taxa captured by each system. The sample volume (relative to organism abundance), camera resolution, tow speed, or method of deployment, however, are usually given minimal consideration when determining which sampling system is optimal to answer particular biological questions.
As automated sampling platforms are increasingly developed and deployed for describing biological patterns, it is apparent that each system has its own tradeoffs for the kinds of organisms or patterns it can detect (Lombard et al., 2019). Deploying these different systems within similar water masses can be used to assess how their detected patterns compare to one another, generating improved understanding of plankton and nekton distributions in relation to physical and biogeochemical properties. While direct comparisons have been performed for a variety of net systems (Wiebe and Benfield, 2003; Broughton and Lough, 2006; Skjoldal et al., 2013), near simultaneous deployment of multiple imaging systems is less common, and these are also rarely deployed in conjunction with acoustics (Sevadjian et al., 2014; Whitmore et al., 2019). Sometimes a direct comparison can reveal tradeoffs among the systems that would otherwise be obscured if used in isolation (Skjoldal et al., 2013; Wiebe et al., 2017), while simultaneously providing a general understanding of the connection of different biological size classes to the oceanographic environment.
A unique combination of instrumentation was deployed on the continental shelf east of Delaware Bay (United States, western Atlantic) to address two main objectives: (1) To describe and evaluate imaging and acoustical methods in relation to each other and to traditional net-based sampling and (2) to measure biological patterns across a range of spatial scales in relation to variability in hydrographic characteristics and concentrations of nutrients and phytoplankton. For the first objective, we hypothesized that the different characteristics of the image, acoustic, and net based sampling methods would influence the detection of size classes and taxonomic composition. For the second objective, we hypothesized that dense aggregations observed by the multiple sampling methods across multiple size classes would occur at density gradients, but the community compositions would differ based on water mass nutrient concentrations and the biomass and size composition of the phytoplankton community. This approach using multiple imaging and acoustic systems allowed us to quantify the individuals that comprised specific size classes.
Materials and Methods
General Field Sampling Plan
The sampling scheme combined station-based and transect sampling for the different systems (Figure 1), and each system was deployed to maximize spatial coverage (within each system’s limitations). A grid was laid out with a pattern of lettered sampling lines approximately perpendicular to the coast of Delaware and southern New Jersey centered at the mouth of Delaware Bay. Station names are used throughout the text in reference to this grid. Numbered transects ran parallel to the coast starting at the coast. The intersections of these lines defined the sampling grid, with 9.26 km (5 nautical miles) between stations. The research cruise on the RV Hugh R. Sharp encompassed a time period between April 26 and May 09, 2018.
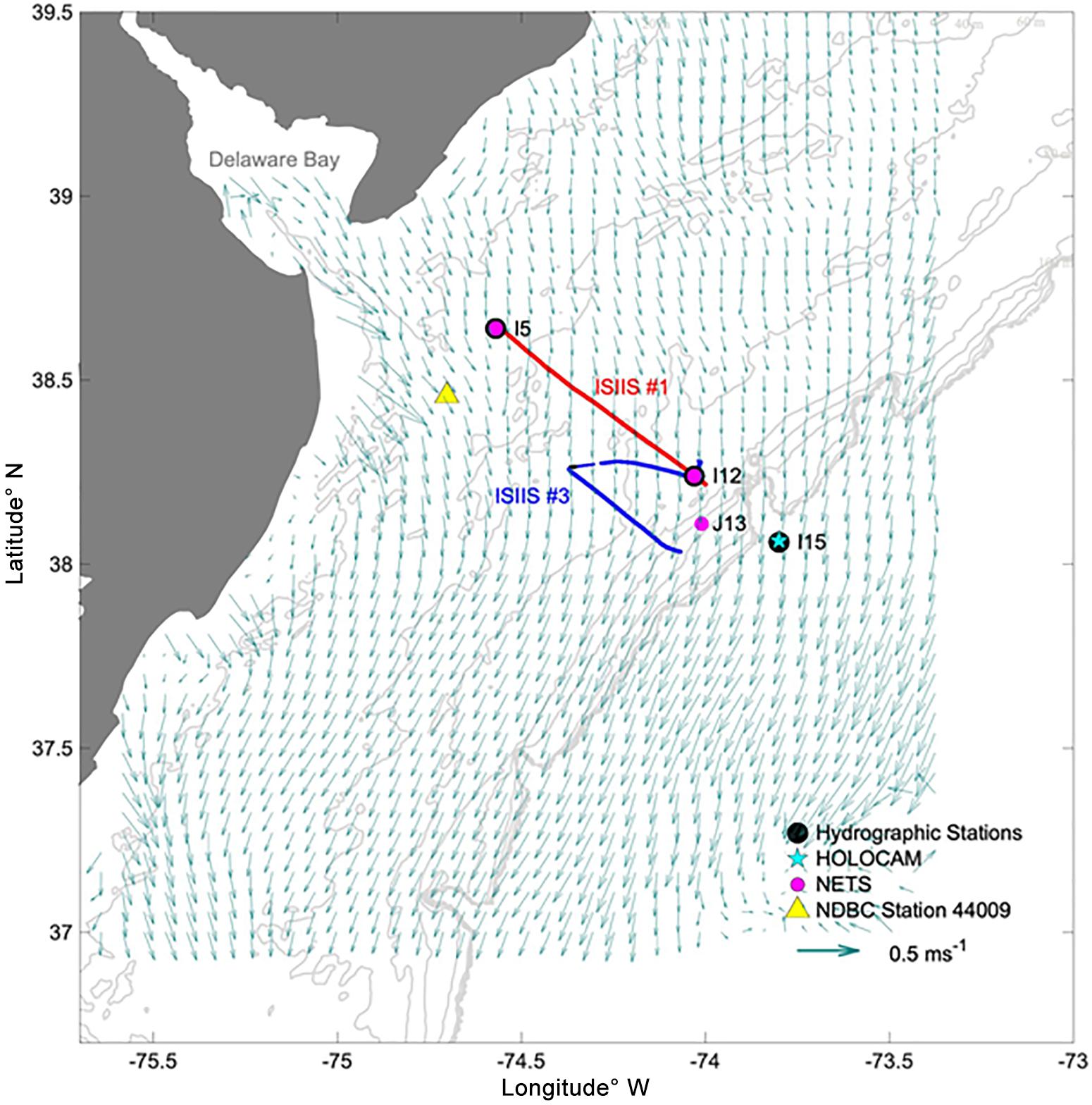
Figure 1. Map of the sampling transects on April 28 and May 01, 2018. The points mark the locations of the hydrographic stations, net tows, HOLOCAM deployment, and wind velocity observations. Acoustic sampling occurred during ISIIS tow #1 but was not conducted during the U-shaped tow. Background arrows indicate the 25 h averaged currents derived from HF radar on April 29–30, 2018.
The multi-hour imaging and acoustical instrument deployments were bracketed by vertical water column profile sampling at fixed stations both prior and subsequent to the tows. At each station, we deployed a CTD rosette consisting of temperature, conductivity, pressure, oxygen, and fluorescence measurements (SBE 9plus, SBE 11plus V 5.2- SeaSoft processing software, SBE 43, WET Labs ECO-AFL/FL) and collected discrete samples in Niskin bottles in the surface layer and at targeted depths associated with gradients of density, dissolved oxygen, and fluorescence. At a subset of stations, we deployed an optical profiling package containing a holographic imaging system (HOLOCAM). The CytoSense was used to image water from the discrete samples from the CTD rosette collected near-surface, at the fluorescence maximum, below this maximum, and occasionally at other depths (e.g., oxygen minima or maxima).
The full suite of station-based samples and profiles was taken before and after towing an In Situ Ichthyoplankton Imaging System (Cowen and Guigand, 2008, ISIIS tow #1) at Station I5 on April 27, 2018 and, after the tow was completed, at Station I12 on April 28 (Figure 1A). ISIIS tow #3 was “U-shaped” – the first part of the tow, called transect 1, was initiated at Station I12 and moved westward to K9. A full suite of hydrographic measurements was taken at both I12 and I15 prior to transect 1 to characterize the offshore waters. At station K9, the ship turned with the ISIIS still in the water and began to transit toward station K13, thus initiating transect 2. Depth-stratified net samples (top and bottom halves of the water column) were collected at J13 after ISIIS tow #3 was completed. For details about the ISIIS and other imaging systems used in this study see Table 1 and Appendix I.

Table 1. Method of deployment, optical resolution, and sampling rate for the different camera systems. The ISIIS small and large camera are the only two systems that are deployed in almost exactly the same water mass.
To describe the larger-scale physical conditions influencing the sampled water masses, regional-scale surface current and wind velocities were obtained from various publicly available sources. Surface currents, downloaded from the Mid-Atlantic Regional Association Coastal Ocean Observing System1, are derived from an observation network of SeaSonde-type Coastal Ocean Dynamics Applications Radars (CODAR) instruments deployed in the Delaware Bay region. SeaSonde-type HF radar instruments exploit information in the radiowave backscatter from the ocean surface to infer movement of the near surface water. Wind velocity data for the NDBC station 44009 (located to the south of the Delaware Bay entrance, Figure 1) were obtained the NOAA station website2.
HOLOCAM Data Processing and Analysis
Imagery and oceanographic data were obtained from the HOLOCAM between 12:47 and 15:44 (EDT) on May 1, 2018 at station I15, which was located near the offshore end of the ISIIS tows (Figure 1A). The HOLOCAM package was profiled vertically from the surface to depths of 28–32 m across different profiles. Data were recorded continuously during the downcast profiles, which lasted 5–8.5 min, corresponding to 4,500–7,650 recorded holograms per profile. A total of five profiles were recorded at this station (referred to as profiles a-e hereafter), but profile d is not presented because of data quality concerns. Based on the package descent rate, only every other recorded hologram was processed for analysis to ensure individual particles were not duplicated (in case a particle is present in two successive holograms). Particle counts from each hologram over a 20 cm depth range were averaged to produce one particle concentration value per bin.
The equivalent spherical diameter (ESD) was used to represent the particle sizes and calculated as , where AF is the area of the particle including empty spaces within the particle perimeter. In cases where the image segmentation results in the loss of a few pixels within the particle bounds, the missing pixels are included to represent or obtain the “filled area.” The same formula for ESD was used for all image data from other instruments where applicable. A subsurface peak was identified in the HOLOCAM profiles, and images from this area were manually examined to identify particles based on size and morphology. For each profile, a subset of ten holograms at three different depths within the peak were selected. In these subsets, particles within the broad range of 60–100 μm were selected and enumerated. All particles within that size range were then visually identified to generate the percentage of ciliates.
ISIIS Data Processing and Analysis
The imagery data from the ISIIS underwent a series of processing steps to extract and size particles and plankton in the images. First, the images were transformed using a “flat-fielding” procedure that evened out background gray level and improved particle contrast relative to the background. The images were then segmented (i.e., regions of interest were extracted) using a binary image gray level threshold of 170. The choice of this threshold value was based on experimenting with different individual images from different regions of the tow, and the chosen value was similar to thresholds used in previous work (e.g., Greer et al., 2018). It is important to note, however, that any chosen threshold will result in features within an image that are detected or ignored, and an optimal threshold detects individual particles or organisms without being too sensitive to faint particles in the background. An overly sensitive threshold value or method can generate many large “particles” composed of several different organisms, prohibiting accurate classification. The choice of these thresholds has not been systematically evaluated for different plankton taxa but likely has a profound impact on the objects detected and how imagery data are interpreted (Giering et al., 2020). After applying the threshold, all black particles were extracted above a certain size limit, along with particle characteristics, including the area of the particle (including white space in the middle of particles, which is common for gelatinous organisms). For the small camera, the particle areas extracted ranged from 400 to 2,500 pixels (0.95–2.37 mm ESD). The particles from the large camera were 800 pixels (1.88 mm ESD) to over 13,000 pixels (7.59 mm ESD, upper limit was ∼40 mm ESD). The flat fielding and segmentation procedures were both implemented in ImageJ (v1.52a, Schneider et al., 2012).
Each extracted particle was merged to the corresponding physical data (depth, temperature, salinity, etc.) using the nearest timestamp. The particles were then binned into 20 m horizontal bins, the approximate distance for the large camera to sample 1 m3 of water (assuming a 50 cm depth of field), and the mean oceanographic variables were calculated for each bin to generate a dataset of particle concentrations, oceanographic variables, and their locations along the transects. With the 0.2 m s–1 vertical movement of the vehicle, these 20 m horizontal bins corresponded to vertical bins of approximately 1.6 m (narrower near surface and bottom when the vehicle was turning). The counts from the small camera were multiplied by 15.67 to generate fine-scale concentrations because that camera system samples 0.0638 (1/15.67) of the volume of water compared the large camera when towed over the equivalent horizontal distance (field of view × depth of field × distance). To examine the abundances vs. particle size, the particles were assigned discrete size classes of roughly equal value (larger size ranges for rarer and larger particles) and were standardized by dividing the abundances in each size category by the bin width of the size category (producing units of individuals m–3 mm–1). The bin width was calculated from the difference in ESD between the largest and smallest particles for that size class. The mean and standard deviations of the concentrations were calculated for each size class and plotted using the midpoint ESD for each size class (3rd quartile of 20,908 pixels or 9.63 mm ESD for the largest size class). These calculations and analyses were performed in R (v3.6.1) with extensive use of the packages ‘plyr,’ ‘reshape2,’ and ‘ggplot2’ (Wickham, 2016). The potential density anomaly was calculated using the R package ‘gsw’ (Kelley et al., 2017). The ISIIS sensor data and organism abundances were linearly interpolated using the R package ‘akima’ (Akima and Gebhardt, 2016).
To examine changes in composition among the different size classes detected in the small and large camera systems, 2,000 image segments were randomly extracted for each size class and categorized into one of 15 categories. These categories included appendicularian (animal), appendicularian house (no animal visible), chaetognath, copepod, ctenophore, diatom, echinoderm larva, fish larva, hydromedusa, pteropod veliger, marine snow aggregate, other (identifiable but too rare to influence proportions, data not shown), shrimp, siphonophore, and unknown (cannot be determined from the image). These image segments were classified using customized keyboard shortcuts in ImageJ. By multiplying the concentration of total particles by the composition across a particular size range, abundance estimates for different taxa could be obtained.
A series of steps and additional calculations were made to directly compare the two camera systems on the ISIIS. First, the particles from the small camera that were larger than 1.88 ESD and the particles less than 2.37 mm ESD from the large camera (overlapping size classes) were enumerated and interpolated across the length of ISIIS tow #3 for both cameras (2 transects). To quantify the degree of spatial aggregation, the Lloyd’s patchiness index (Bez, 2000) was applied to evenly distributed size bins (based on pixel area) for particles ≤ 5.73 mm ESD.
Hydroacoustic System and Data Collection
A pair of split-beam echosounders were used in tandem with the ISIIS tow #1 operating at 18 and 38 kHz (SIMRAD ES18 and ES38-10). Split-beam echosounders have the advantage of remotely sampling large swaths of the full water-column, while underway or stationary, and can be integrated with traditional sampling methods (e.g., net or optical sampling) that are typically volume limited (i.e., taking snap-shots of discrete layers). Split-beam echosounders can detect a wide-range of size classes, from krill swarms to large mega-fauna; and with the correct combination of frequencies, can be used to remotely discriminate taxa observed in the water column (Korneliussen et al., 2008; Koslow, 2009). The beam angle and pulse duration for both instruments was 10° and 1.024 μs, respectively. The 18 kHz transducer was deployed to 2.5 m depth, with a rotating pole along the side of the ship. The 38 kHz transducer was deployed to 3.9 m depth, inside the keel of the ship. Technical drawings of the ship were used to measure the spatial offset between the two transducers, and post-processing techniques were used to synchronize the data in time and space. Transect data were collected on April 27, 2018 from 20:00 to 06:00 (EDT) the following morning. The two echosounders were calibrated at sea according to the standard sphere calibration procedures described by Demer et al. (2015).
Hydroacoustic Data Processing and Analysis
Data were manually scrutinized and processed in Echoview (v10.0, Echoview Software Pty Ltd.). The upper 10 m of the water column was excluded due to nearfield-noise and bubble wash along the transducer faces. Data within 2 m from the bottom were also excluded. Noise artifacts were filtered and excluded following D’Elia et al. (2016). A threshold of −85 dB re 1 m–1 was applied to the filtered data to ensure detection of a mixed assemblage of zooplankton groups (e.g., pteropods, copepods, euphausiids, etc.). The full water column was then echo-integrated in 100 m horizontal by 5 m vertical cells to derive estimates of the Nautical Area Scattering Coefficient (NASC m2 nmi–2), which is considered to be proportional to “acoustic biomass” or energy density (Simmonds and MacLennan, 2005). NASC estimates from both the 18 and 38 kHz transducers were used as an index of scattering in the water column attributed to detritus, plankton, and fish along the transect (Simmonds and MacLennan, 2005).
To account for the separation between the ISIIS (towed) and echosounder (hull-mounted) data, telemetry information from the ISIIS was used to identify a narrow corridor of near-coincident data along the ISIIS sampling path. A 33.0 s time offset was applied to the acoustic data based on the average vessel speed (∼2.5 m s–1) and length of the cable attached to the ISIIS (∼100 m) to obtain comparable datasets. In Echoview, acoustic data collected within >2.5 m from the ISIIS flight path (5 m corridor) were excluded, allowing for a paired comparison between NASC estimates and particle concentrations, as measured by the ISIIS, at discrete depths along the transect (accounting for errors in matching acoustics with the ISIIS positioning). Particle concentration data from the ISIIS was divided into three size classes (5.60–7.59, 7.6–9.4, and >9.41 mm ESD) to determine the degree of correlation between NASC and particle concentration at different sizes. Total particle concentration (>4.6 mm ESD) independent of size class, was also compared to NASC estimates by calculating Spearman’s correlation coefficient (ρ).
Nutrient and Phytoplankton Concentrations
Nutrient samples were collected from Niskin bottles and stored at −20°C until analysis. Nutrient samples were analyzed for nitrate plus nitrite (NO3– + NO2–), nitrite (NO2–), phosphate (PO43–), and silicate [Si(OH)4] using fluorometric (N species) and spectrophotometric (PO43–), and Si(OH)4 methods on an Astoria-Pacific Astoria2 (A2) nutrient auto-analyzer (Method #A179, A027, A205, and A221; Astoria-Pacific International, OR, United States). Nitrate concentrations were subsequently calculated by difference of nitrate plus nitrite and nitrite concentrations.
Phytoplankton pigment samples were collected onto filters under a low light environment. Seawater samples for phytoplankton pigment analysis were vacuum filtered through 25-mm GF/F filters (Whatman, 0.7-μm pore size) until color appeared on the filter and the volume of seawater filtered was recorded. Filters were placed in cryo filter capsules and submerged in liquid nitrogen for storage until analysis. Pigments were analyzed by high performance liquid chromatography (HPLC) following the method of Hooker et al. (2005).
Chlorophyll a (chl-a) and a set of diagnostic pigments were used to assign taxonomic groups and size classes (Uitz et al., 2006). Specifically, the taxonomic biomarkers were: fucoxanthin (diatoms); peridinin (dinoflagellates); 19-hexanoyloxyfucoxanthin (chromophytes and nanoflagellates); 19-butanoyloxyfucoxanthin (chromophytes and nanoflagellates); alloxanthin (cryptophytes); chlorophyll b (green flagellates); and zeaxanthin (cyanobacteria). Pigment:chlorophyll a ratios compiled by Uitz et al. (2006) were used to normalize the measured pigment concentrations to total phytoplankton biomass (chlorophyll a). The pigment data were further used to calculate the size fractions of microplankton (f_micro), nanoplankton (f_nano), and picoplankton (f_pico) where f_micro was comprised of fucoxanthin and peridin, f_nano was comprised of 19-hexanoyloxyfucoxanthin, 19-butanoyloxyfucoxanthin, and alloxanthin, and f_pico was comprised of chlorophyll b and zeaxanthin. Though these size groupings based on pigment concentrations do not strictly conform to specific size ranges, traditionally microplankton are defined to represent the >20 μm size range in equivalent spherical diameter, nanoplankton represent the 2–20 μm size range, and picoplankton represent the 0.2–2 μm size range.
Plankton Net Sampling
Mesozooplankton were sampled with vertical, depth-stratified ring net casts (0.75 m diameter net, 200 μm mesh) to obtain the zooplankton community composition at the beginning and end of each ISIIS tow. The net was fitted with a General Oceanics 2030R mechanical flowmeter to quantify volume sampled (5.95 m3, mean ± 1.92 standard deviation), and a General Oceanics double trip mechanism to control net opening and closure. At a given station, the closed net was lowered to ∼1 m off bottom, where it was then opened and recovered at 0.5 m s–1 to mid-water column and closed. The net was then recovered, rinsed, and the sample fixed in 4% borax-buffered formaldehyde for later processing. Immediately following the first cast, the closed net was lowered to the mid-depth where the previous sample ended. The net was then opened and recovered to the surface for processing as with the first cast.
Fixed samples were digitally analyzed with a Hydroptic ZooScan optical scanner, with subsequent processing using ZooProcess and PkID software (Gorsky et al., 2010). Each sample was transferred to freshwater and sieved into three size fractions (>1,000, >500, and >200 μm) to minimize loss of larger taxa in the splitting process. Each size fraction was then split by Folsom splitter to obtain ∼1,000 individuals in the scan. Images were processed by normalizing their gray levels, then extracting and measuring individual objects (i.e., sections of image with individual zooplankters), including calculation of object equivalent size diameter. A “random forest” algorithm was used to automatically classify extracted objects into 17 predicted categories (i.e., zooplankton taxa) using a learning set developed for these samples. Each object’s classification was manually validated before back-calculating abundance of each taxon based on count, volume sampled, and split fraction.
Results
Physical Oceanographic Properties
Larger-scale measurements indicated the influence of freshwater discharge in the study area during the time of sampling. The HF radar showed a generally offshore trajectory of surface currents from the mouth of the Bay, followed by a southward turn near the shelf break (Figure 1). Hourly averaged 10-m wind velocities from NDBC station 44009 (located to the south of the Delaware Bay, Figure 1) from April 30 to May 03 showed weak and variable direction winds until May 01, followed by upwelling favorable (northward) winds (Figure 2A). The USGS Delaware River daily discharge record at Trenton, NJ indicated high river discharge ∼1,000 m3 s–1 around April 19, 2018 (Figure 2B, note that monthly climatology of the Delaware River discharge is around 630 m3 s–1 for April and 401 m3 s–1 for May). The discharge record at Trenton, NJ is proportional to outflow at the Bay mouth with about an 8-day time lag (Sanders and Garvine, 2001). Therefore, this elevated outflow of fresher water would reach the Delaware Bay mouth around April 27–30. It is known that during upwelling favorable winds, Delaware Bay outflow water masses are mixed with offshore saltier water, and they are advected offshore and to the north (Whitney and Garvine, 2006).
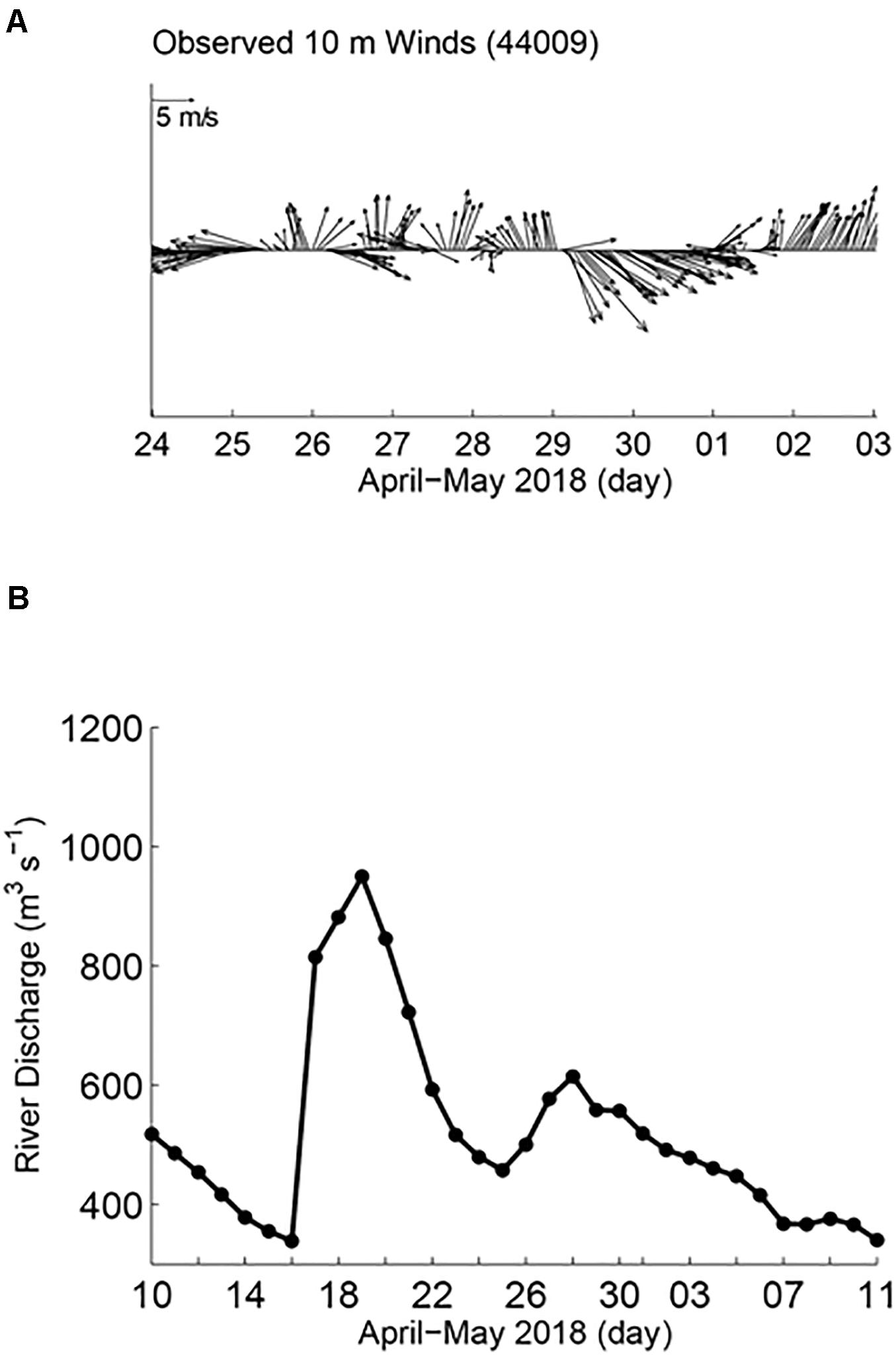
Figure 2. Broader scale physical properties influencing the study area including, (A) time-series of wind direction and speed and (B) river discharge volume in the Delaware River.
Finer-scale physical oceanographic data collected by the ISIIS also suggested influence from freshwater sources on the inner shelf. During ISIIS tow #1 on April 27–28, inner shelf surface waters were substantially warmer and lower in salinity (Figures 3A,B). Salinity became relatively uniform vertically around the middle of the tow, adjacent to deeper saltier waters offshore. The combination of temperature and salinity resulted in isopycnals sloping upward from the shelf toward deeper waters offshore. A tongue of high oxygen waters (∼8.7 mg L–1) had a similar trajectory to the isopycnals at ∼40 km along the transect (Figure 3C). ISIIS tow #3 commenced on the evening of May 1 (2 transects, U-shaped tow). The salinity range for both transects combined was similar to ISIIS tow #1, but the low salinities were confined to a narrow vertical range on the inshore side. In deeper waters further offshore, salinity reached a peak of ∼33.7 (Figure 4A). Warmer waters were generally confined to the surface 5–10 m for both transects (Figure 4B). A peak in dissolved oxygen generally resided between the surface and 20 m throughout most of the tow as well (Figure 4C), and similar to ISIIS tow #1, dissolved oxygen tended to follow the trajectory of the isopycnals.
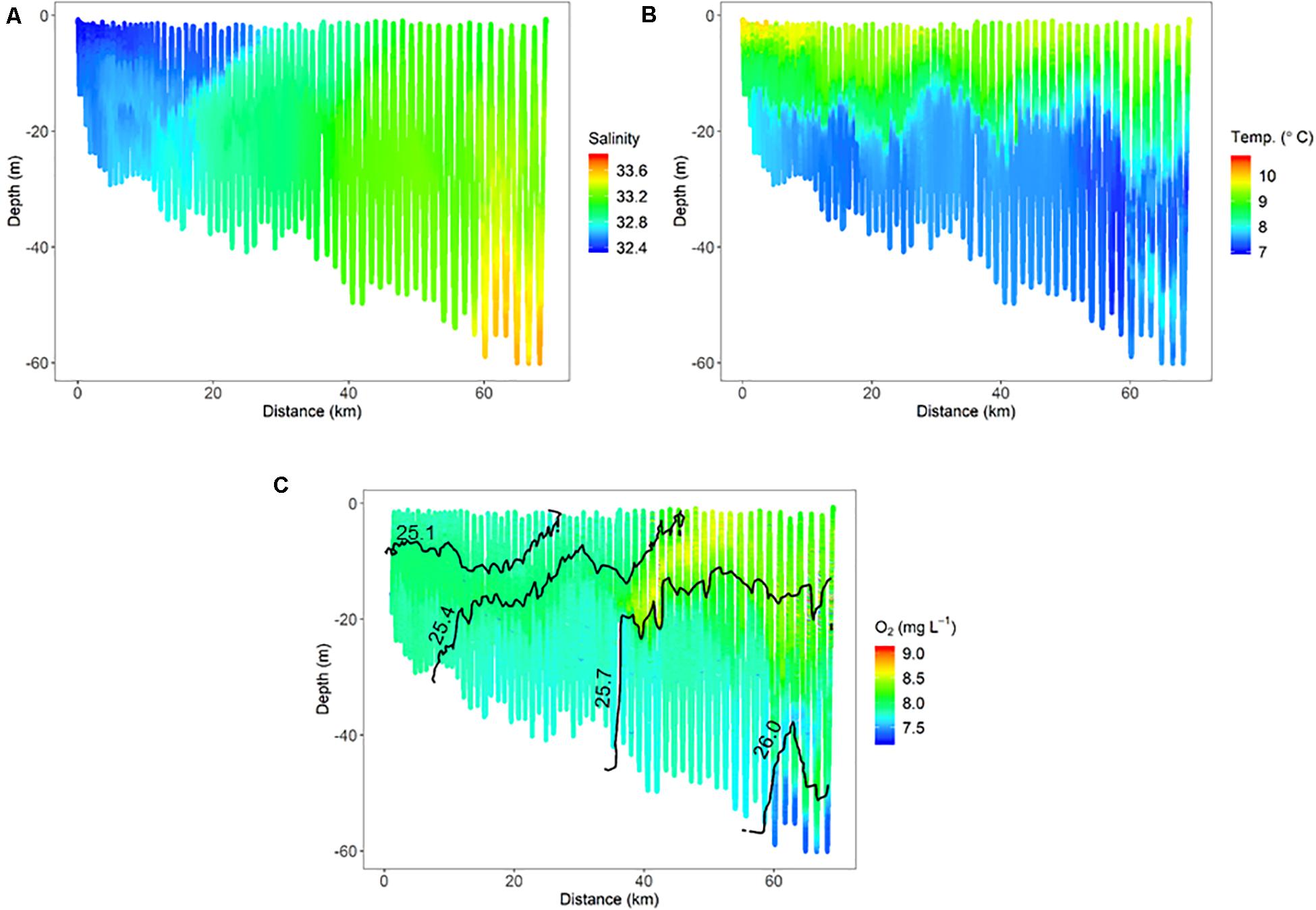
Figure 3. Physical oceanographic conditions from ISIIS tow #1 including, (A) salinity, (B) temperature, and (C) dissolved oxygen. Isopycnals located in panel C (1025.1, 1025.4, 1025.7, and 1026.0 kg m–3) are displayed as a reference to other figures.
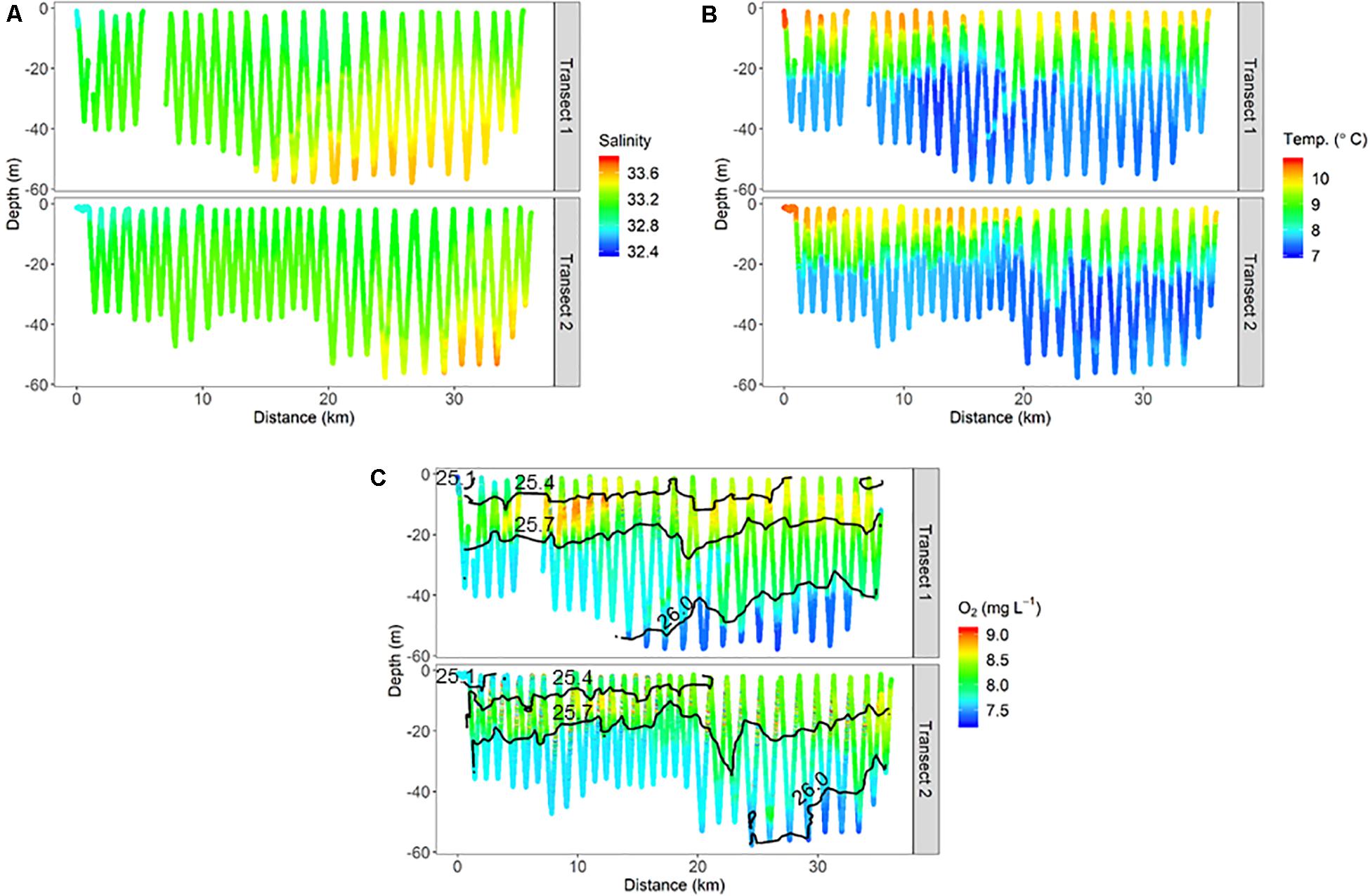
Figure 4. Physical oceanographic conditions from ISIIS tow #3 including, (A) salinity, (B) temperature, and (C) dissolved oxygen. Isopycnals located in panel C (1025.1, 1025.4, 1025.7, and 1026.0 kg m–3) are displayed as a reference.
Vertical Distribution and Size vs. Abundance From Imaging Systems
Vertical particle concentration distributions from HOLOCAM profiles (a, b, c, and e) at one station (I15) showed a consistent peak in concentrations between 18 and 23 m depth (Figure 5), with variations in the precise vertical location. The ciliate numbers varied between 76 and 83% of the manually identified particles in this size range, clearly indicating that the peak was driven by the enhanced ciliate concentrations at these depths (see Figure 6E for example of copepod with background full of ciliates).
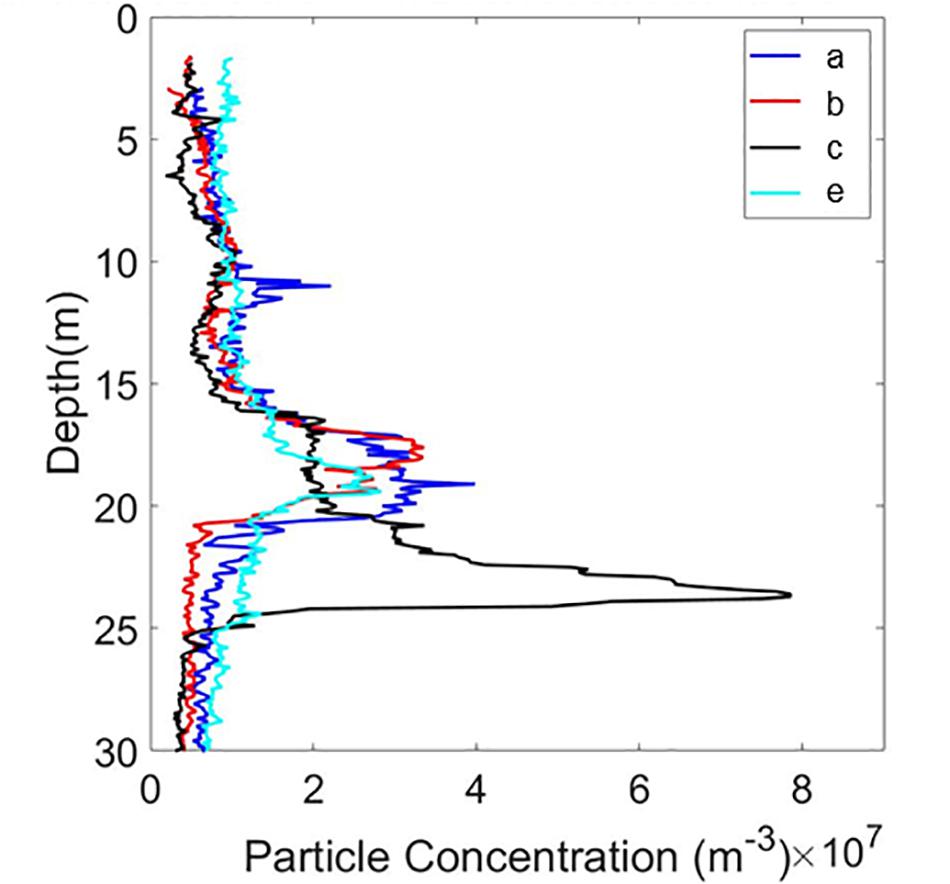
Figure 5. Vertical distribution of particles detected from 4 profiles at the same station (I15) by the HOLOCAM.
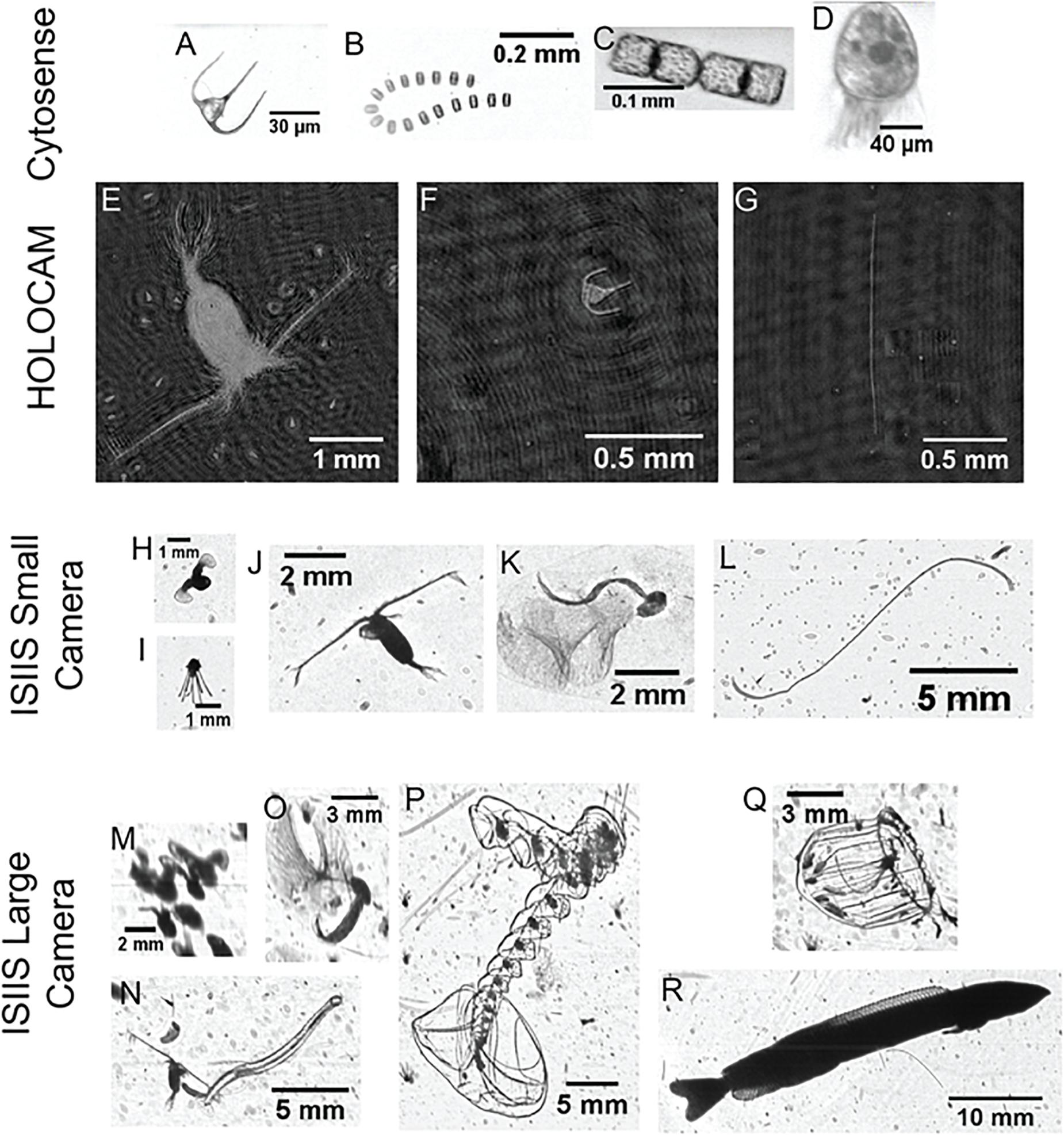
Figure 6. Example organisms detected by different imaging systems. For the Cytosense, (A) Ceratium spp. dinoflagellate, (B) Thalassiosira spp. diatom and (C) Cerataulina spp. diatom and (D) a ciliate (likely Strombidium spp.). For the HOLOCAM, (E) calanoid copepod (with numerous ciliates in the background), (F) Ceratium spp., and (G) diatom chain (genus and species not resolvable). For the ISIIS small camera, (H) veliger (early stage pteropod), (I) echinoderm larva, (J) calanoid copepod, (K) appendicularian, (L) diatom chain with numerous unresolved particles in the background. For the ISIIS large camera, (M) multiple veligers that appear to be touching, generating a large ‘particle’ (imaged through the 50-cm depth of field), (N) chaetognath and copepod, (O) appendicularian, (P) siphonophore (Sphaeronectes spp.), (Q) hydromedusa (Aglantha spp.), (R) juvenile sand lance (Ammodytes spp.).
The CytoSense analyzed small volumes of water from the Niskin bottle samples in a qualitative manner with regards to taxonomy but also enumerated and measured particle lengths. The CytoSense detected the smallest size class of organisms imaged (see Figure 6 for examples). Data pooled from all hydrographic stations on May 01, 2018 and later showed a steady decline in abundance with increasing particle length, but the particle lengths above 0.5 mm were likely not being quantified based on the data missing in some larger size bins. The smallest bin showed a strong spike in abundance possibly due to break up of fragile detritus in the Niskin bottle (Figure 7).
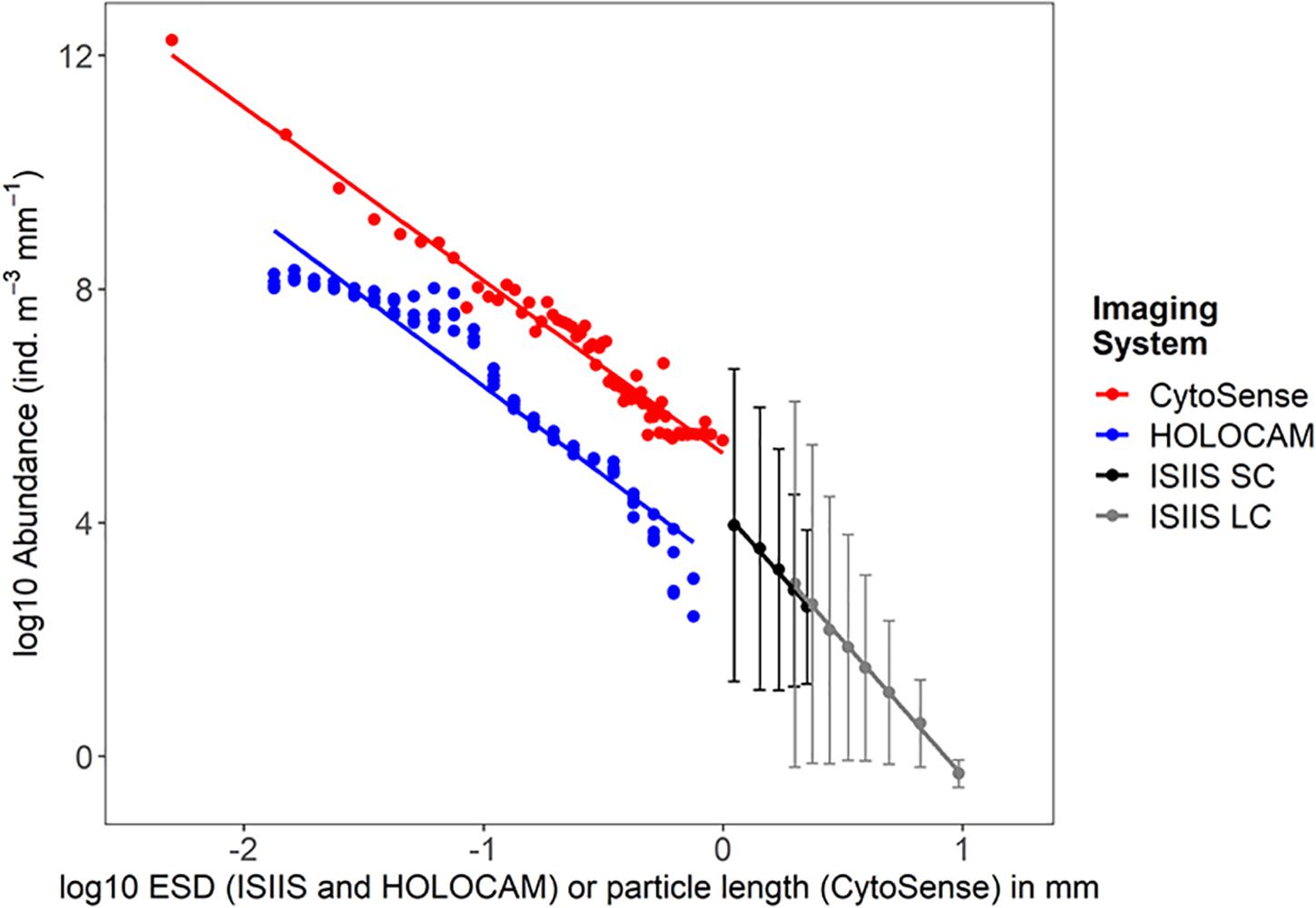
Figure 7. Particle size vs. abundance as detected by the CytoSense (for hydrographic stations visited on May 01, 2018 and afterward), 4 vertical profiles from the HOLOCAM, and the two cameras (SC, small camera; LC, large camera) from the ISIIS. Errorbars on the ISIIS data indicate the standard deviation of the particle concentrations from the different size classes.
The depth-averaged particle size spectra for the 4 HOLOCAM profiles showed a distinct peak in the ciliate size range (0.08–0.1 mm or −1.3 log10 mm ESD, Figure 7). The spike in this size abundance corresponded to depths associated with the peaks from Figure 7. From 0.1 to 0.4 mm, the particle size distribution spectra for each profile indicate that particle compositions did not change much over the duration of these profiles. Above 0.4 mm ESD, the data became more scattered as large particles/organisms (e.g., copepods) were much lower in concentration and less likely to be observed within the HOLOCAM sample volume. Slopes of the particle size vs. abundance plots between the HOLOCAM and CytoSense were similar (−3.05 and −2.97) despite the fact that they measured ESD and particle length, respectively.
Comparison of the small and large cameras on the ISIIS revealed similar patterns in size and abundance from the U-shaped tow on May 01 (Figure 7). From the small camera, a total of 1,001,264 particles were segmented between 0.95 and 2.37 mm ESD over a total imaged volume of water of 267.08 m3 (mean concentration of 3748.97 ind. m–3 for this size class). The large camera, which sampled 15.67 times more water volume along the same transect distance, imaged 1,705,535 particles ranging between 1.88 mm and ∼40 mm ESD. (Note that the large camera did not collect images for the final ∼6 km of transect 2 due to a malfunction in the image acquisition software). For both camera systems, relatively larger particles and plankton were rarer and had less variability in their small scale abundances compared to the smaller size classes (Figure 7). Linear regressions between log10 transformed abundances and sizes revealed nearly identical slopes between the two camera systems (−4.64 and −4.59 for the large and small cameras, respectively). The abundance offset between the most comparable size class ∼1.99 mm ESD (the smallest for the large camera and the second largest for the small camera) was 887.42 ind. m–3 mm–1 for the large camera versus 689.20 ind. m–3 mm–1 for the small camera. In other words, the large camera detected 28.8% higher abundances relative to the small camera in this size class, which is potentially a consequence of imaging through a larger distance of water (depth of field 50 cm vs. 8.9 cm), organism avoidance of the small camera sampling tube in the middle of the vehicle, or a combination of both.
For ISIIS tow #1, 1,243,482 particles were segmented in the large camera system over the 69.1 km transect (4151.41 m3 sampled, particles > 1.88 mm ESD). This corresponded to a mean particle concentration of 299.53 ind. m–3, which was substantially less than the mean abundances on ISIIS tow #3 for particles of the same size class (>1.83 mm ESD were 445.63 ind. m–3). Tow #1, however, transited through a large region of lower salinity water (Figures 3, 4), while Tow #3 started and ended in more offshore waters.
Size-Dependent Composition of Plankton From the ISIIS Cameras
The relative abundance of different types of plankton was dependent on the particle size, and, in some cases, on the camera systems used to measure them (Figure 8). The ISIIS small camera (8.9 cm depth of field) was dominated by diatoms and copepods across most size classes. Among the size classes that overlapped between the small and large camera systems, some plankton types exhibited little difference between systems (e.g., appendicularians, chaetognaths, gelatinous organisms, and shrimp), while several groups had much higher proportions in the large camera (50 cm depth of field), such as copepods, pteropod veligers, and marine snow aggregates. Segments associated with appendicularian houses were present in low numbers across a wide range of size classes in both cameras, while appendicularians (animal visible in the segment) were more common in the larger size range. The diatoms were the only plankton group that substantially increased in relative abundance in the small camera, jumping from 3.8% in the large camera to 29.0% in the small camera for the identical size class (∼2.0 mm ESD, Figure 8). Echinoderm larvae were found in dense aggregations but were only segmented consistently in the smallest size category. These organisms also occasionally dominated the plankton abundances in the nets (Table 2). Both cameras showed an increasing proportion of gelatinous zooplankton with increasing particle size, and the largest size category was the only one to detect fish larvae/juveniles in any substantial number (1.6%, data not shown). The relative peaks in ctenophores, siphonophores, and hydromedusae all occurred within the largest size class as well (ESD > 5.6 mm, 57.4%), with siphonophores comprising 2.3%. The proportion of image segments that could not be identified by an expert was fairly consistent across the size classes for the large camera but made up a slightly smaller proportion when transitioning to the data from the small camera.
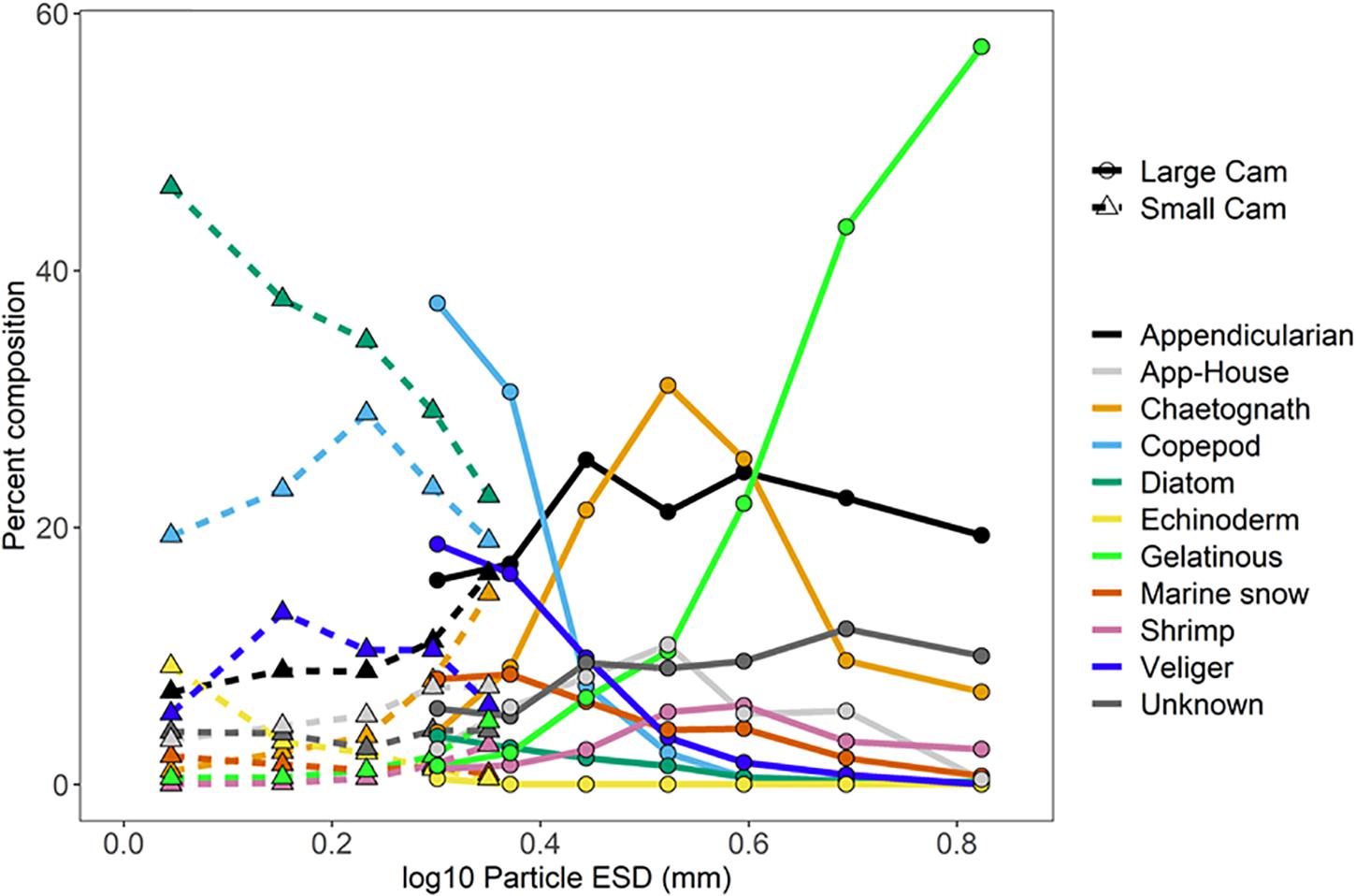
Figure 8. Measured particle sizes (log10 ESD) vs. percent composition for the ISIIS small and large cameras.
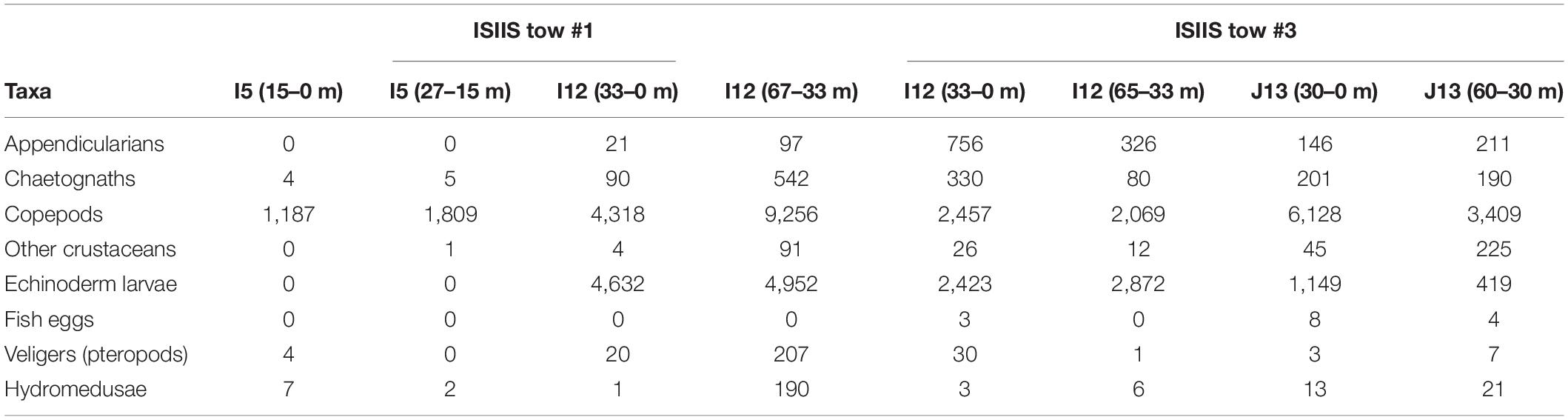
Table 2. Detected concentrations for plankton collected in the vertical net samples (ind. m–3) at the beginning and end of the ISIIS tows #1 and #3.
Two size classes of plankton (1.88–2.82 mm and 2.83–7.59 mm ESD) from the ISIIS large camera, with the larger size class roughly corresponding to the shift toward increasing dominance of gelatinous organisms, had slightly differing spatial patterns for both tows (Figure 9). For ISIIS tow #1, there were two highly-concentrated patches in shallower water, but most individuals tended to be aggregated just above the 1025.7 kg m–3 isopycnal further offshore (near the offshore end of ISIIS tow #3). The larger plankton size class had high concentrations in a similar region of the aggregations for the smaller size class, but there was another group of individuals in deeper waters that was absent in the smaller size class. ISIIS tow #3 abundances in the small size class were dominated by a patch of pteropod veligers in the inshore area of the tow on transect #2. The larger size class, although still represented in the dense patch on transect #2, tended to be abundant closer to the surface and further offshore compared to the smaller size class.
The physical conditions detected by the ISIIS instrumentation and the broader-scale observations suggested a biological response for different planktonic groups, as indicated by changes in abundances and distributions. Both ISIIS tows showed a predominance of organisms of all size classes residing within the shallowest 25 m of the water column, which also generally had higher concentrations of dissolved oxygen. For ISIIS tow #1, peak abundances formed around a distance of 40 km from the start of the transect (near I9), corresponding to an area where there was a transition from a water column with uniform salinity, to slightly higher salinities at depth (∼33.3). Salinity peaked at ∼60 km along the transect and corresponded spatially to a region with high abundances of large particles, including aggregations of juvenile sand lance (Ammodytes spp.) near the bottom (station I12). For ISIIS tow #3, aggregation of veligers occurred below a surface plume of fresher and warmer waters (transect #2), potentially originating from the mouth of Delaware Bay. High concentrations of veligers were also detected near the surface during the “turn” between ISIIS transects #1 and #2 (data not shown), indicating their presence within the fresher surface waters. Another near surface zooplankton aggregation occurred from 20 to 30 km along transect #1, also associated with a surface plume of warmer and slightly fresher waters. This aggregation, however, contained a larger variety of planktonic organisms (mainly appendicularians and gelatinous organisms) and lower abundances of veligers. Similar to ISIIS tow #1, this patch was located just above the 1025.7 kg m–3 isopycnal.
Comparison of Imagery-Derived Abundances to Plankton Nets
The net samples captured similar broad-scale patterns in the plankton abundances relative to the imaging systems but with differing detected community compositions. Copepods were the most dominant taxa in all net samples, comprising 98.6–99.6% of the taxa in the inshore station (I5) and 47.5–60.4% of the offshore station (I12, Table 2) that bracketed ISIIS tow #1. The offshore net sample (I12) contained appendicularians, albeit in relatively low concentrations compared to the ISIIS where they composed 10-30% of all particles across size classes. All plankton taxa were most abundant in the deeper sample from the offshore station, with some groups, such as chaetognaths increasing by an order of magnitude or more. Net samples from ISIIS tow #3 showed more echinoderm larvae compared to the tow #1 samples. However, copepods were generally the most abundant group, comprising >75% of the taxa from the final station after ISIIS tow #3 was completed. The mean ESD of the individuals collected in the nets corresponded roughly to what was detected by the ISIIS small camera but also included sizes slightly smaller than the ISIIS can reliably identify (data not shown). The echinoderm larvae and pteropod veligers that were patchy (according to the ISIIS data) and common in this small size class were detected in variable abundances in the net samples, with patches in horizontal space possibly missed due to the lower station-based spatial resolution of the nets.
A direct comparison of the quantified size classes that overlapped between the two ISIIS cameras (1.88–2.37 mm ESD) on tow #3 revealed changes in detected abundances and degree of plankton aggregation (i.e., patchiness). Although fine-scale concentrations from both cameras were highly correlated (Spearman’s ρ = 0.767), the large camera detected higher maximum concentrations compared to the small camera, and the aggregations qualitatively appeared to be more diffuse in the spatial distributions generated by the small camera (Figures 10A,B). The degree of spatial aggregation, described by the Lloyd’s patchiness index (1 = random distribution of plankton) across different size classes, showed a steady increase in patchiness with increasing size. In the size ranges that overlapped between the two camera systems, the large camera tended to detect higher patchiness, although the small camera data did show a sharp increase in patchiness for the largest size class (2.27–2.37 mm ESD, Figure 10C). Patchiness tended to be more variable toward the less abundant larger sizes. These different spatial distributions and patchiness metrics may have been related to the detected plankton composition differences between the two cameras. For example, diatom chains were a much higher percentage of the composition in the small camera system (Figure 8).
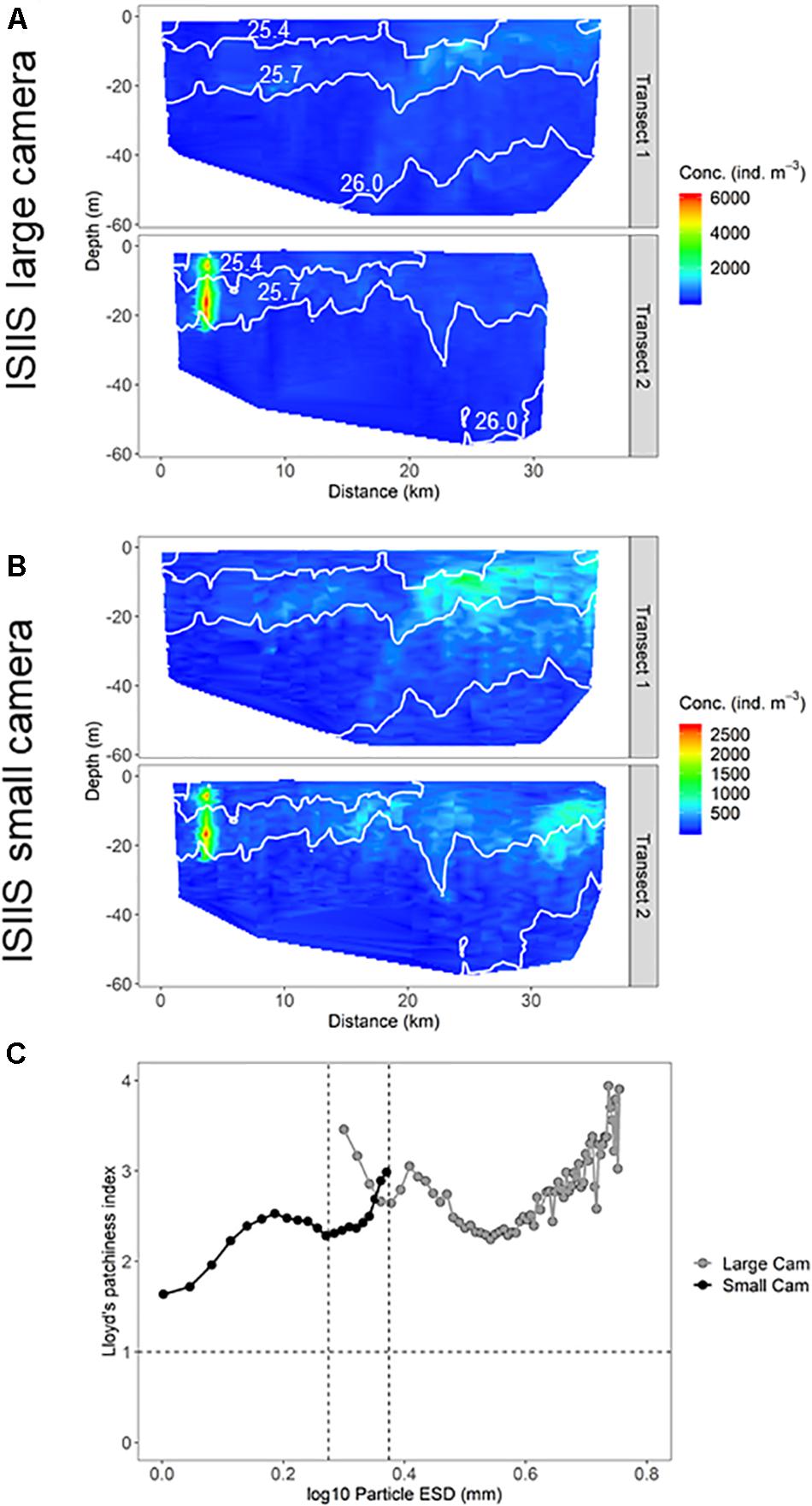
Figure 10. Distribution of particles in the ISIIS (A) large and (B) small cameras that overlap in size (1.88–2.37 mm ESD). (C) Lloyd’s patchiness index (random distribution = 1. Higher values indicate more patchiness.) for particles vs. size detected by the two cameras on the ISIIS. The two vertical dotted lines indicate the size range where the two cameras overlap.
Hydroacoustic Backscatter in Relation to Organisms Detected by the ISIIS
Acoustic data from the 18 and 38 kHz transducers indicated acoustic backscatter was relatively low inshore (<40 km along the transect, Figure 11). However, a thin scattering layer was consistently observed at 18 kHz between 10–15 m depth along the transect (ISIIS tow #1). Water column backscatter increased substantially beyond 50 km along the transect, and beyond 60 km, a strong scattering layer was observed at both 18 and 38 kHz extending from the seabed into the water column. These observations reflect the patterns observed in the ISIIS for the >7.59 mm ESD size class (dominated by gelatinous zooplankton), particularly the increased scattering in the 18 kHz echosounder and large particle abundance near the offshore end of the cross-shelf transect (Figure 11C). This high scattering region also corresponded to the highest detected zooplankton abundances in the nets (dominated by copepods). There was a significant positive correlation between the ISIIS particle abundance (>4.6 mm ESD) and NASC (Spearman’s rank correlation coefficient, ρ = 0.52), but the relationship was weaker for the largest size organisms (>9.4 mm ESD) that were sampled more sporadically by the ISIIS (Spearman’s ρ = 0.37).
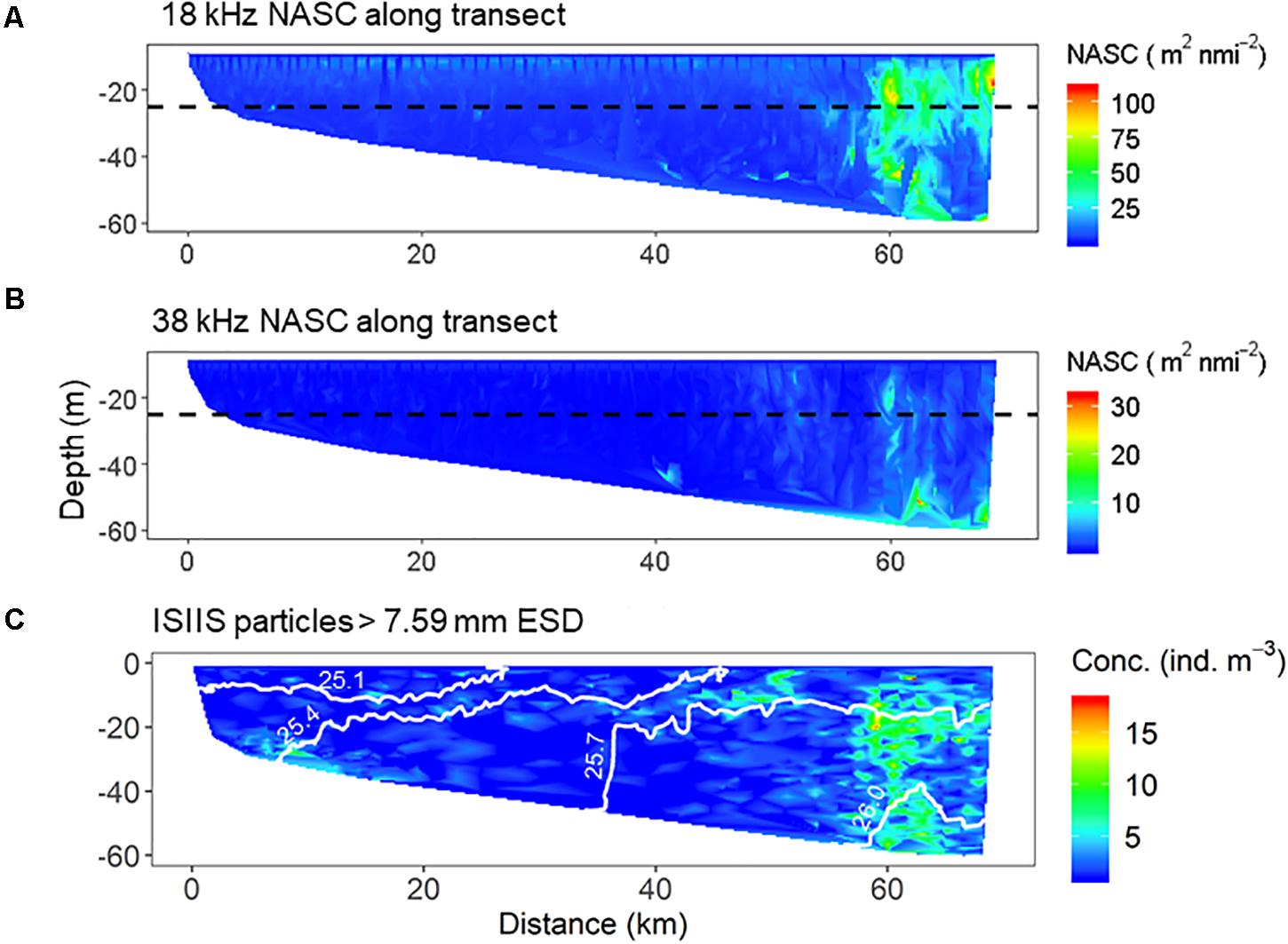
Figure 11. Distribution of NASC (acoustic backscatter) for the (A) 18 kHz and (B) 38 kHz transducers. (C) Abundance of particles larger than 7.59 mm ESD as detected by the ISIIS along the same transect.
Nutrient and Phytoplankton Concentration and Composition
Nitrate concentrations in surface waters from all 33 samples at stations examined during this study ranged from 0 to 4.6 mmol m–3, with highest surface concentrations occurring at lowest salinity (28.6) near the coast. Generally, at intermediate salinities from 29.5 to 33, nitrate was near 0 in surface waters, whereas at surface salinity > 33, nitrate was approximately 2 mmol m–3. Nitrate concentrations increased with depth. This pattern was observed at the stations at the beginning (station I5) and end (station I12) of ISIIS tow #1 (Figure 12). Nitrite concentrations (not shown) were, on average, about 10% of the nitrate and had a similar spatial pattern to the nitrate. Ammonium concentrations were also lower than nitrate and had similar spatial patterns (Figure 12C). Phosphate concentrations in surface waters ranged from 0.3 to 0.7 mmol m–3, and silicate concentrations were between 0.4 and 4.3 mmol m–3. At the beginning of ISIIS tow #1 at station I5, the surface layer chl-a concentration measured by HPLC was 0.8 mg m–3 (Figure 12F). At the end of ISIIS tow #1 at station I12, chl-a concentration was higher at 2.8 mg m–3. Accompanying the increase in chl-a from I5 to I12, there was a shift in the dominant size fraction of the phytoplankton (Figure 12) as calculated from the HPLC pigment data and the size fraction equations of Uitz et al. (2006). Microplankton dominated at I5, comprising 52% of the chl-a but decreasing to 34% of the chl-a at I12. In contrast, nanoplankton comprised 36% of the chl-a at I5 but increased to 59% at I12. Picoplankton decreased from 13% of the chl-a at I5 to 7% at I12 (Figure 12G).
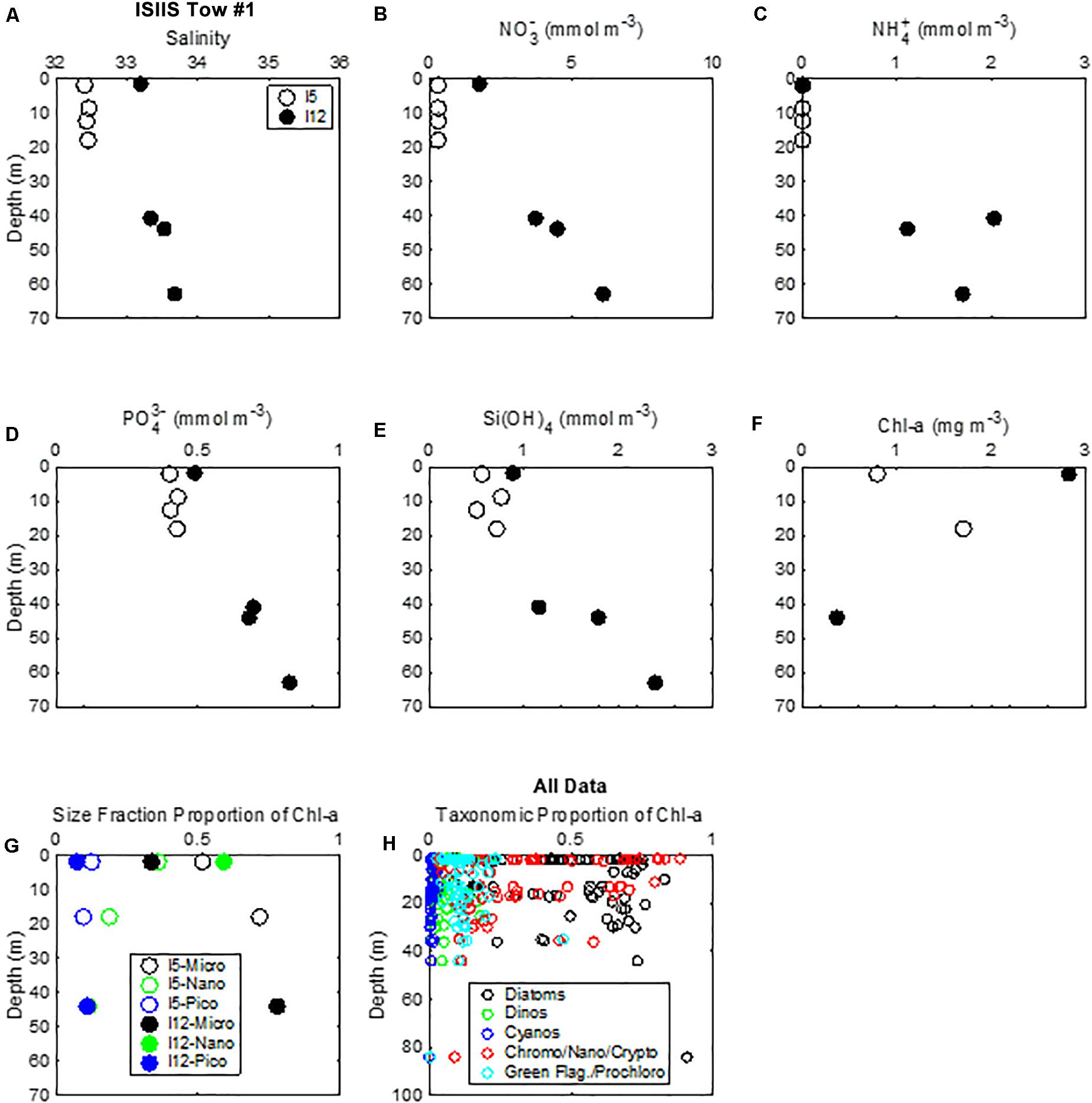
Figure 12. Vertical distribution of (A) salinity, nutrients (B) nitrate, (C) ammonium, (D) phosphate, (E) silica), and (F) chlorophyll-a at the beginning and end of ISIIS tow #1. (G) Size fraction of chlorophyll-a for the same stations as the nutrients. (H) Total taxonomic proportion of chlorophyll-a determined by HPLC.
Taxonomically, phytoplankton biomass across the shelf was dominated by diatoms, representing, on average, 47% of the chl-a concentration (Figure 12H). Cryptophytes/nanoflagellates/chromophytes were the next most abundant group, representing an average of 35% of the chl-a concentration. The remainder of the phytoplankton biomass contained green flagellates/prochlorophytes (11%), dinoflagellates (6%), and cyanobacteria (1%).
Discussion
By deploying several systems in the same shelf environment, and in some cases directly comparing the fine-scale spatial distribution and composition of similar size classes, we described detailed spatial patterns of an unprecedented size range of different organisms in connection to the physical oceanographic environment. The aggregations detected by the different systems were variable depending on organism size and composition. For the smallest size classes, aggregations of ciliates detected by the HOLOCAM were confined to a relatively narrow portion of the water column. For the smaller size classes captured by the ISIIS, dense aggregations were vertically dispersed, tended to cross isopycnals (e.g., pteropod veligers), and were associated with near surface fresher waters. Larger size classes tended to inhabit offshore water masses and were dominated by gelatinous zooplankton whose spatial distributions were tightly coupled to isopycnals. The acoustics detected a general trend toward higher backscatter further offshore where larger particles tended to reside in the ISIIS, and zooplankton abundances were generally higher in the net samples from this area. Although the samples encompass a relatively short time period, the instruments, combined, describe detailed environmental conditions for different taxa and size classes, while also providing new lessons for interpreting the size and composition data from imaging systems.
Influence of Sampling Method on Detected Abundances
Our evaluation of the imagery data demonstrated that, for systems that were directly comparable, the optical setup influenced the detected abundances, which supported our first hypothesis. Small changes in pixel resolution or sampling volume can also impact which organisms are detected, as demonstrated by the direct comparison between the ISIIS small and large cameras. The effect of pixel resolution was most apparent for the diatom chains imaged by the ISIIS; diatoms were quite rare in the large camera (59-μm pixel resolution) but became a majority of the segmented particles in the small camera (42-μm pixel resolution). These differences in diatom detection are likely related to their thin, chain-forming morphology – a trait that also influences their interactions with grazers (Kenitz et al., 2020). Diatom chains in the ISIIS images are typically only ∼1–3 pixels wide (50–150 μm) but can be >1 cm long. A slight increase in pixel resolution, therefore, effectively makes them double the number of pixels in area because their area is approximately equal to their perimeter. Such a dramatic increase in particle area from slight enhancement of pixel resolution would not occur with more round particles due to this simple fact of morphology. In other words, when particle thickness is a limiting factor for detection, small changes in pixel resolution can be the difference between particles being abundant or not detected at all. This should be considered for future studies utilizing automated image processing, as the perimeter to area ratio could indicate which particles may experience different detection rates under varied camera pixel resolutions and image processing settings. Diatoms were also relatively rare in the HOLOCAM imagery, probably due to relatively low abundances of diatoms at the particular station where the system was deployed (I15), as indicated by HPLC data from offshore sites, and the relatively small hologram volume relative to the ISIIS small camera images (∼3.7 mL per hologram and ∼165 mL per image, respectively).
The net samples served as a “ground-truth” for the imagery and showed copepods as the dominant zooplankton group for most stations (occasionally surpassed by echinoderm larvae), which appears to contrast strongly with the imagery data. The percent composition for copepods found at the stations greatly differed from that found in the ISIIS for the relevant size classes (maximum of 10 to 30% copepods). Although the trajectory of the ISIIS sampling differed from where the nets were deployed (in addition to the vast differences in spatial scale), a combination of the total particle abundance and the percent composition in the ISIIS compares favorably with the plankton nets. Because the copepods made up ∼30% of the organisms in the ISIIS from 1 mm to 3 mm ESD (Figure 8), and the mean concentration of organisms in that size range was ∼10,000 individuals m–3 mm–1 (Figure 7), that would correspond to ∼6,000 ind. m–3, which is similar to copepod abundance found in the nets (water column average ranged from 1,000 to 9,000 ind. m–3). Similar calculations for other taxa, however, would reveal stark differences, particularly for soft-bodied organisms. These discrepancies are likely due to biases of net systems toward robust zooplankton body compositions and against gelatinous organisms, which has been described previously using more thorough direct comparisons (e.g., Båmstedt et al., 2003; Remsen et al., 2004). The relative abundances of these fragile organisms in the net samples, however, did match the broad-scale patterns detected by the ISIIS (higher abundances offshore).
Even at relatively fast tow speeds (∼2.5 m s–1 for the ISIIS), avoidance behaviors likely explain some discrepancies in patchiness between the two cameras, as well as some consistencies in the percentage of unidentifiable organisms for different size classes. The composition of the plankton clearly shifted toward more gelatinous organisms for larger sizes. Surprisingly, the percentage of unknown organisms was relatively consistent across size classes for the large camera. With more pixels per object, larger particles should have a higher probability of identification to a “known” category. The reason for an “unknown” identification, however, appears to change with size. Larger particles, particularly shrimps and small fishes, have good swimming ability and sometimes attempt to avoid the imager (indicated by blurriness or an obvious startle response). Toward the larger end of the size spectrum, there is more avoidance, and toward the smaller size classes, the pixel resolution becomes the limiting factor, which generates a relatively consistent rate of unknowns across size classes. This hypothesis is further supported by the fact that the unknowns were less frequent in the smaller camera data (higher pixel resolution), particularly for the size classes that overlapped with the large camera. Along similar lines of thinking, organism avoidance could be one factor driving to the reduced patchiness detected by the small camera.
Persistent Hurdles for Assessing Size and Composition With Imagery
Organism body composition differences present a challenge for extraction (i.e., segmentation), identification, and sizing with imaging systems. Appendicularians were common across the size classes, and they are often surrounded by mucous “houses” that can collect marine snow aggregates on their surfaces. Because of the differences in pixel gray level between the organism body (dark) and the mucous house (faint), the body is often segmented alone. However, when the house contains marine snow aggregates, or the optical path goes through a particularly dark portion of the house, the mucous house may be segmented. We identified marine snow segments associated with a house and classified these as an “appendicularian house.” This approach can artificially expand size range of “houses” because they are often detected after being discarded by the organism and in various stages of degradation. It is difficult to determine if segmentation was inaccurate when looking at individual segments, and it highlights the somewhat philosophical question of what constitutes the appropriate “size” of marine particles and organisms with complex morphologies. These “house” segments could also be classified as marine snow and, in fact, might be with automated image processing algorithms. The rate at which this error occurs, however, has not been systematically evaluated. One approach to mitigate the over-segmentation issue includes detecting and joining adjacent segments, which is effective for chains of diatom cells (e.g., Nayak et al., 2018), yet in other contexts, this approach can introduce problems, such as determining the identification for a segment with more than one organism type present. More experimentation with segmentation algorithms and methods of classification is needed, particularly for closely associated organisms that are frequently detected when deploying in situ imaging systems (e.g., Möller et al., 2012; Takahashi et al., 2013; Greer et al., 2018).
Dense aggregations of organisms can also introduce errors with regards to measuring size and abundance [i.e., under-segmentation, see Greer et al. (2014) for an example regarding copepods]. Pteropod veligers were so highly concentrated that they were under-segmented within patches on ISIIS tow #3, but they were also abundant in other areas. This under-segmentation issue is directly related to the depth of field for the optical setup. For larger depths of field, which is necessary to have for quantifying rarer organisms, high concentrations of plankton will lead to increased probability of overlap in the images. Addressing the relationships between overlap probability, particle size, abundance, and depth of field should be examined through simulation, which would be useful for applying correction factors to size and abundance estimates (e.g., Luo et al., 2018). In some ecosystems, the overlap problem may not influence quantified abundances; however, evidence regarding this issue is elusive, as patchiness is only recently being described with the level of taxonomic and spatial detail needed to assess this kind of problem (Greer et al., 2016).
As automated algorithms continue to be applied to imagery datasets for examining taxonomic patterns (e.g., Faillettaz et al., 2016; Luo et al., 2018; Ellen et al., 2019), these hurdles for accurate analysis may be difficult to detect with typical validation workflows. The intensity of aggregations and rapid shifts in composition found in our study suggests that these issues with image processing deserve more attention. For the vast majority of studies utilizing plankton imagery, there is a size-detection threshold or specified size classes, and understanding general composition (i.e., dominance of diatom chains or appendicularians) is key for determining if these errors may influence detected patterns.
Relationships Between Biological and Physical Variables and Organism Distributions
There was a clear relationship between organism size and broad-scale (1–10 km) abundance that may have been related to the phytoplankton community structure. Chlorophyll-a and nutrients tended to be higher offshore near the shelf break, and the plankton community structure shifted between inshore and offshore stations. Other than fresher surface waters that were irregular and often connected to aggregations of zooplankton (e.g., pteropod veligers), temperature and salinity did not change dramatically across the shelf. The nearshore environment was dominated by microplankton, particularly diatoms (>50% of total composition), whereas the offshore zone was dominated by nanoplankton (non-diatom) size fraction (>50% of total composition). In relation to this pattern, there were substantial changes in the size classes of zooplankton represented in the imagery, which were related to the taxonomic composition (larger sizes tended to include more gelatinous organisms). The offshore region with more nanoplankton had large numbers of gelatinous zooplankton, including appendicularians, and the inshore region tended to have patches of hard-bodied zooplankton and generally smaller-sized organisms relative to offshore. The net samples, although few in number, reflected this broad-scale pattern in that more appendicularians and hydromedusae were captured offshore.
While the phytoplankton community may have influenced some of the larger scale patterns of zooplankton abundance, the in situ imagery showed that organism distributions had differing spatial relationships to physical oceanographic structure, depending on both size and body composition. Thus, the support for our second hypothesis, that organism aggregations would occur at density gradients, was mixed. Locally high abundances, particularly for the larger size classes of organisms, were often just above the 1025.7 kg m–3 isopycnal for both ISIIS tows (Figure 9). These results are consistent with other high-resolution observations of gelatinous organisms, which dominated the larger size classes and can display tight spatial correlation with certain isotherms or isopycnals (e.g., Jacobsen and Norrbin, 2009; Frost et al., 2010; Luo et al., 2014; Suzuki et al., 2018). Patches of hard-bodied zooplankton, on the other hand, can show similar coupling to isopycnals (Möller et al., 2012) or be relatively untethered to physical discontinuities in the water column (Baumgartner et al., 2013; Greer et al., 2014). Further inshore on May 1 (ISIIS tow #3), the large aggregation of pteropod veligers (most apparent in the smaller size classes) was perpendicular to the isopycnal trajectory, but was associated with the edge of a fresher water parcel near the surface. High abundances of veligers were also imaged within these fresher waters while turning the ISIIS near the surface (data not shown). Although few high-resolution observations of pteropods exist, Gallager et al. (1996) found that pteropods concentrated near the center of the water parcels, rather than near water parcel boundaries. This pattern is generally consistent with our findings: gelatinous organisms aggregated near the physical transitions, while the pteropod veligers did not appear to have the same affinities for water mass boundaries or isopycnals. Although the precise ecological interactions producing these distributions are not known, controlled experiments offer promise for resolving interactions between size- or taxon-specific zooplankton behavior and the entrained prey communities near density discontinuities (e.g., True et al., 2018).
Fine-scale acoustic backscatter showed strong spatial overlap with the abundances of large organisms detected by the ISIIS, especially beyond ∼50 m depth. However, the majority of organisms observed by the ISIIS in this size category (i.e., gelatinous) are not likely to be significant sources of backscatter at 18 and 38 kHz. Hydroacoustic surveys conducted with similar frequencies to the ones used in this study can detect small fishes and siphonophores, which have gas-filled body parts (Proud et al., 2019), and post-processing techniques can be used to classify distinct taxonomic groups (e.g., fishes with and without swim bladders, crustaceans, etc.) based on their unique frequency-dependent response (Jech and Michaels, 2006; De Robertis et al., 2010; McQuinn et al., 2013; D’Elia et al., 2016). According the subsample of the largest imaged organisms, juvenile fishes (Ammodytes spp.) were confined to the deeper waters offshore and could have produced some portion of the acoustic backscatter. Approximately 2.3% of the organisms in the largest size class (>5.57 mm ESD) were siphonophores, including many from the genus Nanomia, which have pneumatophores that contribute to acoustic backscatter (Davison et al., 2015). The siphonophores were slightly more common than the fishes that comprised 1.6% of this size class. It seems likely, based on the concentration of the large particles offshore, that fishes and larger gelatinous organisms are spatially co-located, implying that more detailed analyses are required to determine the contribution of different taxa to the acoustic backscatter. In other instances, however, avoidance of the ISIIS by potential scatterers (e.g., fishes >5 cm) may prohibit robust identifications of the contributors to acoustic backscatter at the frequencies used in this study. Determining the identity of the scatters is particularly difficult when multiple target organisms are present (Stanton, 2012; Wiebe et al., 2017) and capable of movement throughout the water column with changing orientation depending on their ecology and environmental cues (Benfield et al., 2000; Parra et al., 2019; Boswell et al., 2020).
Conclusion
With the increasing use of high-frequency sampling systems to understand how organisms aggregate in the ocean, it is important to consider which organisms or patterns are sampled quantitatively with a particular sampling technology. Depending on the actual organism taxonomic composition, concentrations, and the tow speed or optical setup, a system can produce misleading results. Although imaging systems detect fine-scale patterns for fragile and hard-bodied zooplankton, there are many caveats with the relationship between detected size and organism morphology that are also influenced by the optical properties of the system. Deploying multiple high-resolution systems showed size-dependent patterns that differed in their associations with the physical oceanographic conditions. This intense patchiness likely has implications for the ecology of the planktonic groups examined here, but these relatively new technologies are just scraping the surface of what remains to be discovered. Our approach allowed us to better understand the strengths and weaknesses of each system in terms of what kinds of patterns they can detect, but more thorough evaluations of sampling tradeoffs among systems are still needed. Achieving this goal of understanding these quantitative samplers is critical for assimilating zooplankton data into ecological models (Everett et al., 2017).
Walter Munk referred to the state of oceanography in the 20th century as the “century of undersampling” (Munk, 2000). Although he was mostly referring to the lack of spatiotemporal resolution for various physical processes, the same or more extreme statements could be made about the previous century of sampling the ocean’s biological constituents. High-resolution biological sampling in coastal, open ocean, and within the context of global surveys is now possible across a range of size classes, including both hard- and soft-bodied zooplankton (Lombard et al., 2019). Characterizing the distributions and properties of these organisms or particles has many implications for the functioning of trophic food webs (Heneghan et al., 2016; Everett et al., 2017) and the global biological pump (Guidi et al., 2016; Fender et al., 2019). Future deployments of these systems, along with a robust evaluation of the sampling trade-offs, will increase their utility for describing critical biological processes and understanding how these systems may operate together to “see” previously unresolvable phenomena.
Data Availability Statement
The raw data supporting the conclusions of this manuscript will be made available by the authors, without undue reservation, to any qualified researcher.
Author Contributions
AG collected the ISIIS data, analyzed the data, and wrote the manuscript. JL analyzed the Niskin bottle samples, wrote relevant sections, and edited the manuscript. BB collected and analyzed the acoustic backscatter data and wrote relevant sections. AN collected and analyzed the HOLOCAM data, wrote relevant sections, and edited the manuscript. RB analyzed HOLOCAM data and edited the manuscript. AR designed the field study, analyzed physical oceanographic data, and edited the manuscript. JC analyzed the plankton net data, wrote relevant sections, and edited the manuscript. MM collected the HOLOCAM data and edited the manuscript. AH collected the Niskin bottle samples, analyzed the phytoplankton and nutrient data, and edited the manuscript. NS collected the HOLOCAM data and edited the manuscript. KB analyzed the acoustic data and edited the manuscript. IS analyzed the HF radar data and physical oceanographic data and edited the manuscript. SR analyzed physical data and edited the manuscript. BP designed the field study, coordinated ship data collection efforts, analyzed CytoSense data, wrote relevant sections, and edited the manuscript. All authors contributed to the article and approved the submitted version.
Funding
This research was funded by the Naval Research Laboratory project InTro: Intermediate Trophic Levels - Interconnections with Fronts, Eddies and Primary Production (Program Element 61153N). The holographic data collection and processing efforts were also supported by NSF grants OCE-1634053 and OCE-1657332.
Conflict of Interest
The authors declare that the research was conducted in the absence of any commercial or financial relationships that could be construed as a potential conflict of interest.
Acknowledgments
We would like to thank the captain and crew of the RV Hugh R. Sharp. Stephanie Anderson assisted in generating some of the figures. Ian Martens supported all aspects of cruise logistics and was instrumental in making for a successful field sampling expedition. Frank Hernandez provided guidance with the identification of the juvenile fish imaged by the ISIIS. We would also like to thank Laura Treible and two reviewers who provided thorough comments and edits that helped improve this manuscript.
Footnotes
References
Andersen, K. H., Berge, T., Goncalves, R. J., Hartvig, M., Heuschele, J., Hylander, S., et al. (2016). Characteristic sizes of life in the oceans, from bacteria to whales. Annu. Rev. Mar. Sci. 8, 217–241. doi: 10.1146/annurev-marine-122414-034144
Båmstedt, U., Kaartvedt, S., and Youngbluth, M. (2003). An evaluation of acoustic and video methods to estimate the abundance and vertical distribution of jellyfish. J. Plankton Res. 25, 1307–1318. doi: 10.1093/plankt/fbg084
Baumgartner, M. F., Lysiak, N. S. J., Esch, H. C., Zerbini, A. N., Berchok, C. L., and Clapham, P. J. (2013). Associations between North Pacific right whales and their zooplanktonic prey in the southeastern Bering Sea. Mar. Ecol. Prog. Ser. 490, 267–284. doi: 10.3354/meps10457
Benfield, M. C., Davis, C. S., and Gallager, S. M. (2000). Estimating the in-situ orientation of Calanus finmarchicus on Georges Bank using the Video Plankton Recorder. Plankton Biol. Ecol. 47, 69–72.
Benoit-Bird, K. J., and McManus, M. A. (2012). Bottom-up regulation of a pelagic community through spatial aggregations. Biol. Lett. 8, 813–816. doi: 10.1098/rsbl.2012.0232
Bez, N. (2000). On the use of Lloyd’s index of patchiness. Fish. Oceanogr. 9, 372–376. doi: 10.1046/j.1365-2419.2000.00148.x
Biard, T., Stemmann, L., Picheral, M., Mayot, N., Vandromme, P., Hauss, H., et al. (2016). In situ imaging reveals the biomass of giant protists in the global ocean. Nature 532, 504–507. doi: 10.1038/nature17652
Blanchard, J. L., Heneghan, R. F., Everett, J. D., Trebilco, R., and Richardson, A. J. (2017). From bacteria to whales: using functional size spectra to model marine ecosystems. Trends Ecol. Evol. 32, 174–186. doi: 10.1016/j.tree.2016.12.003
Boswell, K. M., D’Elia, M., Johnston, M. W., Mohan, J. A., Warren, J. D., Wells, R. J., et al. (2020). Oceanographic structure and light levels drive patterns of sound scattering layers in a low-latitude oceanic system. Front. Mar. Sci 7:51. doi: 10.3389/fmars.2020.00051
Broughton, E. A., and Lough, R. G. (2006). A direct comparison of MOCNESS and Video Plankton Recorder zooplankton abundance estimates: Possible applications for augmenting net sampling with video systems. Deep Sea Res. II 53, 2789–2807. doi: 10.1016/j.dsr2.2006.08.013
Buskey, E. J., Baker, K. S., Smith, R. C., and Swift, E. (1989). Photosensitivity of the oceanic copepods Pleuromamma gracilis and Pleuromamma xiphias and its relationship to light penetration and daytime depth distribution. Mar. Ecol. Prog. Ser. 55, 207–216. doi: 10.3354/meps055207
Conley, K. R., Lombard, F., and Sutherland, K. R. (2018). Mammoth grazers on the ocean’s minuteness: a review of selective feeding using mucous meshes. Proc. R. Soc. B-Biol. Sci. 285:20180056. doi: 10.1098/rspb.2018.0056
Cowen, R. K., Greer, A. T., Guigand, C. M., Hare, J. A., Richardson, D. E., and Walsh, H. J. (2013). Evaluation of the In Situ Ichthyoplankton Imaging System (ISIIS): comparison with the traditional (bongo net) sampler. Fish. Bull. 111, 1–12. doi: 10.7755/FB.111.1.1
Cowen, R. K., and Guigand, C. M. (2008). In situ ichthyoplankton imaging system (ISIIS): system design and preliminary results. Limnol. Oceanogr. Methods 6, 126–132. doi: 10.4319/lom.2008.6.126
Dadon-Pilosof, A., Lombard, F., Genin, A., Sutherland, K. R., and Yahel, G. (2019). Prey taxonomy rather than size determines salp diets. Limnol. Oceanogr. 64, 1996–2010. doi: 10.1002/lno.11165
Davis, C. S., and McGillicuddy, D. J. Jr. (2006). Transatlantic abundance of the N2-fixing colonial cyanobacterium Trichodesmium. Science 312, 1517–1520. doi: 10.1126/science.1123570
Davis, C. S., Thwaites, F. T., Gallager, S. M., and Hu, Q. (2005). A three-axis fast-tow digital Video Plankton recorder for rapid surveys of plankton taxa and hydrography. Limnol. Oceanogr. Methods 3, 59–74. doi: 10.4319/lom.2005.3.59
Davison, P. C., Koslow, J. A., and Kloser, R. J. (2015). Acoustic biomass estimation of mesopelagic fish: backscattering from individuals, populations, and communities. ICES J. Mar. Sci. 72, 1413–1424. doi: 10.1093/icesjms/fsv023
De Robertis, A., McKelvey, D. R., and Ressler, P. H. (2010). Development and application of an empirical multifrequency method for backscatter classification. Can. J. Fish. Aquat. Sci. 67, 1459–1474. doi: 10.1139/F10-075
D’Elia, M., Warren, J. D., Rodriguez-Pinto, I., Sutton, T. T., Cook, A., and Boswell, K. M. (2016). Diel variation in the vertical distribution of deep-water scattering layers in the Gulf of Mexico. Deep-Sea Res. Part I-Oceanogr. Res. Pap 115, 91–102. doi: 10.1016/j.dsr.2016.05.014
Demer, D. A., Berger, L., Bernasconi, M., Bethke, E., Boswell, K., Chu, D., et al. (2015). Calibration of acoustic instruments. ICES Cooperat. Res. Rep. 326:133.
Ellen, J. S., Graff, C. A., and Ohman, M. D. (2019). Improving plankton image classification using context metadata. Limnol. Ocenogr. Methods 17, 439–461. doi: 10.1002/lom3.10324
Everett, J. D., Baird, M. E., Buchanan, P., Bulman, C., Davies, C., Downie, R., et al. (2017). Modeling what we sample and sampling what we model: challenges for zooplankton model assessment. Front. Mar. Sci 4:77. doi: 10.3389/fmars.2017.00077
Faillettaz, R., Picheral, M., Luo, J. Y., Guigand, C., Cowen, R. K., and Irisson, J. (2016). Imperfect automatic image classification successfully describes plankton distribution patterns. Methods Oceanogr. 15-16, 60–77. doi: 10.1016/j.mio.2016.04.003
Fender, C. K., Kelly, T. B., Guidi, L., Ohman, M. D., Smith, M. C., and Stukel, M. R. (2019). Investigating particle size-flux relationships and the biological pump across a range of plankton ecosystem states from coastal to oligotrophic. Front. Mar. Sci. 6:603. doi: 10.3389/fmars.2019.00603
Feunteun, A., de Schrevel, C., Verhaegen, M., Chevallier, D., Duchemin, M., Ziani, N., et al. (2018). First evaluation of the cookie-cutter sharks (Isistius sp.) predation pattern on different cetacean species in Martinique. Environ. Biol. Fishes 101, 749–759. doi: 10.1007/s10641-018-0735-1
Frost, J. R., Jacoby, C. A., and Youngbluth, M. J. (2010). Behavior of Nemopsis bachei L. Agassiz, 1849 medusae in the presence of physical gradients and biological thin layers. Hydrobiologia 645, 97–111. doi: 10.1007/s10750-010-0213-z
Gallager, S. M., Davis, C. S., Epstein, A. W., Solow, A., and Beardsley, R. C. (1996). High-resolution observations of plankton spatial distributions correlated with hydrography in the Great South Channel. Georges Bank. Deep Sea Res. Part II Top. Stud. Oceanogr. 43, 1627–1663. doi: 10.1016/s0967-0645(96)00058-6
Giering, S. L. C., Hosking, B., Briggs, N., and Iversen, M. H. (2020). The interpretation of particle size, shape, and carbon flux of marine particle images is strongly affected by the choice of particle detection algorithm. Front. Mar. Sci 7:564. doi: 10.3389/fmars.2020.00564
Gorsky, G., Ohman, M. D., Picheral, M., Gasparini, S., Stemmann, L., and Romagnan, J. B. (2010). Digital zooplankton image analysis using the ZooScan integrated system. J. Plankton Res. 32, 285–303. doi: 10.1093/plankt/fbp124
Greer, A. T., Chiaverano, L. M., Luo, J. Y., Cowen, R. K., and Graham, W. M. (2018). Ecology and behaviour of holoplanktonic scyphomedusae and their interactions with larval and juvenile fishes in the northern Gulf of Mexico. ICES J. Mar. Sci. 75, 751–763. doi: 10.1093/icesjms/fsx168
Greer, A. T., Cowen, R. K., Guigand, C. M., Hare, J. A., and Tang, D. (2014). The role of internal waves in larval fish interactions with potential predators and prey. Prog. Oceanogr. 127, 47–61. doi: 10.1016/j.pocean.2014.05.010
Greer, A. T., Woodson, C. B., Smith, C. E., Guigand, C. M., and Cowen, R. K. (2016). Examining mesozooplankton patch structure and its implications for trophic interactions in the northern Gulf of Mexico. J. Plankton Res. 38, 1115–1134. doi: 10.1093/plankt/fbw033
Guidi, L., Chaffron, S., Bittner, L., Eveillard, D., Larhlimi, A., Roux, S., et al. (2016). Plankton networks driving carbon export in the oligotrophic ocean. Nature 532:465. doi: 10.1038/nature16942
Heneghan, R. F., Everett, J. D., Blanchard, J. L., and Richardson, A. J. (2016). Zooplankton are not fish: improving zooplankton realism in size-spectrum models mediates energy transfer in food webs. Front. Mar. Sci 3:201. doi: 10.3389/fmars.2016.00201
Henschke, N., Everett, J. D., Richardson, A. J., and Suthers, I. M. (2016). Rethinking the role of salps in the ocean. Trends Ecol. Evol. 31, 720–733. doi: 10.1016/j.tree.2016.06.007
Hooker, S. B., Heukelem, L., Thomas, C., Claustre, H., Ras, J., Barlow, R., et al. (2005). The second SeaWiFS HPLC analysis round-robin experiment (SeaHARRE-2). NASA Tech. Mem. 1–112.
Hoving, H., Christiansen, S., Fabrizius, E., Hauss, H., Kiko, R., Linke, P., et al. (2019). The pelagic in situ observation system (PELAGIOS) to reveal biodiversity, behavior, and ecology of elusive oceanic fauna. Ocean Sci. 15, 1327–1340. doi: 10.5194/os-15-1327-2019
Jacobsen, H. P., and Norrbin, M. F. (2009). Fine-scale layer of hydromedusae is revealed by Video Plankton Recorder (VPR) in a semi-enclosed bay in northern Norway. Mar. Ecol. Prog. Ser. 380, 129–135. doi: 10.3354/meps07954
Jech, J. M., and Michaels, W. L. (2006). A multifrequency method to classify and evaluate fisheries acoustics data. Can. J. Fish. Aquat. Sci. 63, 2225–2235. doi: 10.1139/F06-126
Jennings, S., Pinnegar, J., Polunin, N., and Boon, T. (2001). Weak cross-species relationships between body size and trophic level belie powerful size-based trophic structuring in fish communities. J. Anim. Ecol. 70, 934–944. doi: 10.1046/j.0021-8790.2001.00552.x
Katz, J., and Sheng, J. (2010). Applications of holography in fluid mechanics and particle dynamics. Annu. Rev. Fluid Mech. 42, 531–555. doi: 10.1146/annurev-fluid-121108-145508
Kelley, D., Richards, C., and WG127 S. (2017). gsw: Gibbs Sea Water Functions. Available online at: https://CRAN.R-project.org/package=gsw.
Kenitz, K. M., Orenstein, E. C., Roberts, P. L. D., Franks, P. J. S., Jaffe, J. S., Carter, M. L., et al. (2020). Environmental drivers of population variability in colony-forming marine diatoms. Limnol. Oceanogr. 65, 2515-2528. doi: 10.1002/lno.11468
Kiørboe, T. (2008). A Mechanistic Approach to Plankton Ecology. Princeton: Princeton University Press.
Kiørboe, T., Visser, A. P., and Andersen, K. H. (2018). A trait-based approach to ocean ecology. ICES J. Mar. Sci. 75, 1849–1863. doi: 10.1093/icesjms/fsy090
Korneliussen, R. J., Diner, N., Ona, E., Berger, L., and Fernandes, P. G. (2008). Proposals for the collection of multifrequency acoustic data. ICES J. Mar. Sci 65, 982–994. doi: 10.1093/icesjms/fsn052
Koslow, J. A. (2009). The role of acoustics in ecosystem-based fishery management. ICES J. Mar. Sci. 66, 966–973. doi: 10.1093/icesjms/fsp082
Letcher, B. H., and Rice, J. A. (1997). Prey patchiness and larval fish growth and survival: inferences from an individual-based model. Ecol. Model. 95, 29–43. doi: 10.1016/s0304-3800(96)00015-4
Lombard, F., Boss, E., Waite, A. M., Vogt, M., Uitz, J., Stemmann, L., et al. (2019). Globally consistent quantitative observations of planktonic ecosystems. Front. Mar. Sci. 6:196. doi: 10.3119/fmars.2019.00196
Luo, J. Y., Grassian, B., Tang, D., Irisson, J. O., Greer, A. T., Guigand, C. M., et al. (2014). Environmental drivers of the fine-scale distribution of a gelatinous zooplankton community across a mesoscale front. Mar. Ecol. Prog. Ser. 510, 129–149. doi: 10.3354/meps10908
Luo, J. Y., Irisson, J. O., Graham, B., Guigand, C., Sarafraz, A., Mader, C., et al. (2018). Automated plankton image analysis using convolutional neural networks. Limnol. Oceanogr. Methods. 16, 814–827. doi: 10.1002/lom3.10285
McQuinn, I. H., Dion, M., and St Pierre, J. F. (2013). The acoustic multifrequency classification of two sympatric euphausiid species (Meganyctiphanes norvegica and Thysanoessa raschii), with empirical and SDWBA model validation. ICES J. Mar. Sci. 70, 636–649. doi: 10.1093/icesjms/fst004
Möller, K. O., St John, M., Temming, A., Floeter, J., Sell, A. F., Herrmann, J., et al. (2012). Marine snow, zooplankton and thin layers: indications of a trophic link from small-scale sampling with the Video Plankton Recorder. Mar. Ecol. Prog. Ser. 468, 57–69. doi: 10.3354/meps09984
Moore, T. S., Churnside, J. H., Sullivan, J. M., Twardowski, M. S., Nayak, A. R., McFarland, M. N., et al. (2019). Vertical distributions of blooming cyanobacteria populations in a freshwater lake from LIDAR observations. Remote Sens. Environ. 225, 347–367. doi: 10.1016/j.rse.2019.02.025
Munk, W. (2000). Oceanography before, and after, the advent of satellites. Elsevier Oceanogr. Ser. 63, 1–4. doi: 10.1016/S0422-9894(00)80002-1
Nayak, A. R., McFarland, M. N., Sullivan, J. M., and Twardowski, M. S. (2018). Evidence for ubiquitous preferential particle orientation in representative oceanic shear flows. Limnol. Oceanogr. 63, 122–143. doi: 10.1002/lno.10618
Ohman, M. D., Davis, R. E., Sherman, J. T., Grindley, K. R., Whitmore, B. M., Nickels, C. F., et al. (2019). Zooglider: an autonomous vehicle for optical and acoustic sensing of zooplankton. Limnol. Oceanogr. Methods 17, 69–86. doi: 10.1002/lom3.10301
Parra, S. M., Greer, A. T., Book, J. W., Deary, A. L., Soto, I. M., Culpepper, C., et al. (2019). Acoustic detection of zooplankton diel vertical migration behaviors on the northern Gulf of Mexico shelf. Limnol. Oceanogr. 64, 2092–2113. doi: 10.1002/lno.11171
Peacock, E. E., Olson, R. J., and Sosik, H. M. (2014). Parasitic infection of the diatom Guinardia delicatula, a recurrent and ecologically important phenomenon on the New England Shelf. Mar. Ecol. Prog. Ser. 503, 1–10. doi: 10.3354/meps10784
Picheral, M., Guidi, L., Stemmann, L., Karl, D. M., Iddaoud, G., and Gorsky, G. (2010). The Underwater Vision Profiler 5: an advanced instrument for high spatial resolution studies of particle size spectra and zooplankton. Limnol. Oceanogr. Methods 8, 462–473. doi: 10.4319/lom.2010.8.462
Priyadarshi, A., Smith, S. L., Mandal, S., Tanaka, M., and Yamazaki, H. (2019). Micro-scale patchiness enhances trophic transfer efficiency and potential plankton biodiversity. Sci. Rep. 9:17243. doi: 10.1038/s41598-019-53592-6
Proud R., Handegard, N. O., Kloser, R. J., Cox, M. J., and Brierley, A. S. (2019). From siphonophores to deep scattering layers: uncertainty ranges for the estimation of global mesopelagic fish biomass. ICES J. Mar. Sci. 76, 718–733. doi: 10.1093/icesjms/fsy037
Remsen, A., Hopkins, T. L., and Samson, S. (2004). What you see is not what you catch: a comparison of concurrently collected net, Optical Plankton Counter, and Shadowed Image Particle Profiling Evaluation Recorder data from the northeast gulf of mexico. Deep-Sea Res. Part I-Oceanogr. Res. Pap. 51, 129–151. doi: 10.1016/j.dsr.2003.09.008
Samson, S., Hopkins, T., Remsen, A., Langebrake, L., Sutton, T., and Patten, J. (2001). A system for high-resolution zooplankton imaging. IEEE J. Ocean. Eng. 26, 671–676. doi: 10.1109/48.972110
Sanders, T., and Garvine, R. (2001). Fresh water delivery to the continental shelf and subsequent mixing: an observational study. J. Geophys. Res. Oceans 106, 27087–27101. doi: 10.1029/2001JC000802
Schmid, M. S., Cowen, R. K., Robinson, K., Luo, J. Y., Briseño-Avena, C., and Sponaugle, S. (2020). Prey and predator overlap at the edge of a mesoscale eddy: fine-scale, in-situ distributions to inform our understanding of oceanographic processes. Sci. Rep. 10:921. doi: 10.1038/s41598-020-57879-x
Schneider, C. A., Rasband, W. S., and Eliceiri, K. W. (2012). NIH Image to ImageJ: 25 years of image analysis. Nat. Methods 9, 671–675. doi: 10.1038/nmeth.2089
Sevadjian, J. C., McManus, M. A., Ryan, J., Greer, A. T., Cowen, R. K., and Woodson, C. B. (2014). Across-shore variability in plankton layering and abundance associated with physical forcing in Monterey Bay, California. Cont. Shelf Res. 72, 138–151. doi: 10.1016/j.csr.2013.09.018
Simmonds, J., and MacLennan, D. (2005). Observation and Measurement of Fish: Fisheries Acoustics: Theory and Practice. Hoboken, NJ: Wiley.
Skjoldal, H. R., Wiebe, P. H., Postel, L., Knutsen, T., Kaartvedt, S., and Sameoto, D. D. (2013). Intercomparison of zooplankton (net) sampling systems: results from the ICES/GLOBEC sea-going workshop. Prog. Oceanogr. 108, 1–42. doi: 10.1016/j.pocean.2012.10.006
Stanton, T. K. (2012). 30 years of advances in active bioacoustics: a personal perspective. Methods Oceanogr. 1-2, 49–77. doi: 10.1016/j.mio.2012.07.002
Sutherland, K. R., Madin, L. P., and Stocker, R. (2010). Filtration of submicrometer particles by pelagic tunicates. Proc. Natl. Acad. Sci. U.S.A. 107, 15129–15134. doi: 10.1073/pnas.1003599107
Suzuki, K. S., Niida, Y., Tsubono, T., Takimoto, H., Kumakura, E., Ishii, H., et al. (2018). Mechanisms underlying heterogeneous distribution of moon jellyfish Aurelia aurita s.l. across a sharp pycnocline. Mar. Ecol. Prog. Ser. 591, 229–239. doi: 10.3354/meps12357
Takahashi, K., Ichikawa, T., Saito, H., Kakehi, S., Sugimoto, Y., Hidaka, K., et al. (2013). Sapphirinid copepods as predators of doliolids: their role in doliolid mortality and sinking flux. Limnol. Oceanogr. 58, 1972–1984. doi: 10.4319/lo.2013.58.6.1972
Trevorrow, M. V., Mackas, D. L., and Benfield, M. C. (2005). Comparison of multifrequency acoustic and in situ measurements of zooplankton abundances in Knight Inlet, British Columbia. J. Acoust. Soc. Am. 117, 3574–3588. doi: 10.1121/1.1920087
True, A. C., Webster, D. R., Weissburg, M. J., and Yen, J. (2018). Copepod avoidance of thin chemical layers of harmful algal compounds. Limnol. Oceanogr. 63, 1041–1055. doi: 10.1002/lno.10752
Uitz, J., Claustre, H., Morel, A., and Hooker, S. B. (2006). Vertical distribution of phytoplankton communities in open ocean: an assessment based on surface chlorophyll. J. Geophys. Res. -Oceans 111:C08005. doi: 10.1029/2005JC003207
Wakabayashi, K., Sato, R., Hirai, A., Ishii, H., Akiba, T., and Tanaka, Y. (2012). Predation by the phyllosoma larva of Ibacus novemdentatus on various kinds of venomous jellyfish. Biol. Bull. 222, 1–5. doi: 10.1086/bblv222n1p1
Whitmore, B. M., Nickels, C. E., and Ohman, M. D. (2019). A comparison between Zooglider and shipboard net and acoustic mesozooplankton sensing systems. J. Plankton Res. 41, 521–533. doi: 10.1093/plankt/fbz033
Whitney, M., and Garvine, R. (2006). Simulating the Delaware Bay buoyant outflow: comparison with observations. J. Phys. Oceanogr. 36, 3–21. doi: 10.1175/JPO2805.1
Wiebe, P. H., and Benfield, M. C. (2003). From the Hensen net toward four-dimensional biological oceanography. Prog. Oceanogr. 56, 7–136. doi: 10.1016/s0079-6611(02)00140-4
Wiebe, P. H., Bucklin, A., and Benfield, M. C. (2017). “Sampling, preservation and counting of samples II: Zooplankton,” in Marine Plankton, eds C. Castellani and M. Edwards (Oxford: Oxford University Press), 104–135.
Woodson, C. B., and Litvin, S. Y. (2015). Ocean fronts drive marine fishery production and biogeochemical cycling. Proc. Natl. Acad. Sci. U.S.A. 112, 1710–1715. doi: 10.1073/pnas.1417143112
Appendix I: Description of Imaging Systems
CytoSense
The CytoSense (CytoBuoy, b.v., NL) scanning flow cytometer uses a 75 mW, 488 nm laser to enumerate and characterize individual particles in whole seawater (∼0.2 to 700 μm diameter and up to 10 mm in length). Sample water is injected into the center of a sheath fluid stream at a rate that allows single particles to be resolved individually. The sheath fluid is obtained by filtering 2 L of seawater (0.2-μm pore size) from the same location as the sample from the same Niskin bottle. An auto-ranging sample dosing pump and programmable detection thresholds allow a range of particle concentrations from 102 to 1010 to be analyzed. During the entire passage of the particles across the laser beam, photodiodes detect near-forward and sideward scattering of the laser (and depolarization of a second, 20 mW red laser), and photomultipliers detect fluorescence in 3 spectral bands (red, yellow-green, and orange). The amplitude, duration, and shape of the resulting ‘pulse’ signals of the various detectors provide information about the particles. Targeted imaging-in-flow allows select particles to be photographed with an in-line 1,280 pixel × 1,024 pixel CMOS camera (Pixelink PL-A741-BL).
Holocam
The digital holographic imaging technique involves illuminating a sample volume with a coherent light source (i.e., laser beam). A portion of the light passing through the volume gets scattered by particles in its path, while the remaining fraction passes through undisturbed. The hologram represents the recording of the interference pattern of the scattered and undisturbed light fields (Katz and Sheng, 2010). Subsequently, numerical reconstruction techniques can be used to get in-focus 2-D planes or sections within the 3-D sample volume, thus enabling accurate characterization of 3-D spatial distributions of particles at different depths. Further details on holography and applications can be found elsewhere (Vikram, 1992; Schnars and Jueptner, 2005; Katz and Sheng, 2010).
The HOLOCAM uses a 660 nm Nd-YAG laser as the source of illumination. Most aquatic organisms are minimally sensitive to this portion of the visible light spectrum, thus helping us record their behavior in the least obtrusive manner (Buskey et al., 1989). The unit images a sample volume of 3.69 mL per hologram at a frequency of 15 Hz. Further details about the HOLOCAM and methods of deployment can be found in Nayak et al. (2018). (See Table 1 for a comparison to other imaging systems). A Seabird Electronics 49 Fastcat CTD, strapped to the HOLOCAM, facilitated recording of co-located salinity, temperature and depth profiles during deployment. The timestamps of the CTD and HOLOCAM data were matched, and the CTD data were resampled at the HOLOCAM sampling frequency, thus providing depth information for each recorded hologram. Numerical reconstruction of holograms obtained in-focus particles at different depths using the Fresnel diffraction formula (Katz and Sheng, 2010). Post-processing steps, using established algorithms, have been described elsewhere (Nayak et al., 2018; Moore et al., 2019). The steps include image segmentation and binarization to isolate all particles in each hologram, thus populating a data table with relevant parameters for each particle, including major and minor axis length, aspect ratio, and equivalent size diameter, among others.
In situ Ichthyoplankton Imaging System
The In Situ Ichthyoplankton Imaging System (ISIIS) is a towed instrument that generates a continuous image of planktonic organisms while simultaneously collecting measurements of water column properties (Cowen and Guigand, 2008). The current version of the ISIIS has two cameras designed to sample different volumes of water (Table 1). The large camera has field of view of 12 cm (2,048 pixels) and uses a collimated light source projected across a 50 cm depth of field into a line scan camera (Dalsa Piranha 2 P2-22-02k40). The large camera is set toward the front of the vehicle near torpedo-shaped pods that minimize water disturbance. The small camera has a similar optical setup but a smaller field of view (4.3 cm, 1,024 pixels) with an 8.9 cm depth of field. This camera is set further toward the middle of the vehicle and about 50 cm higher (shallower). For both of these line scan cameras, a continuous “strip” of non-overlapping imagery is collected, but the image acquisition software breaks up the continuous image into roughly square images based on the field of view. For this reason, the constant speed of the ship (2.5 m s–1) and relatively stationary particles are key for obtaining accurate size measurements. Under high particle concentrations, multiple overlapping particles that can lead to erroneously low abundance calculations and inflated particle sizes (Greer et al., 2014) are less of an issue with the smaller camera setup due to the shorter optical path through the sampled water. Thin features on different organisms will also appear thicker (due to higher camera resolution in the small camera), which can affect the organisms’ detectability after image processing to extract regions of interest (i.e., image segmentation).
The ISIIS (including both cameras and oceanographic sensors) vertical position is controlled by motor-actuated wings that move the vehicle up and down throughout the water column from near-surface to 2–5 m from the bottom at a target vertical speed (0.2 m s–1). While collecting images from the two cameras, the ISIIS also measures salinity, temperature, depth (SBE 49 CTD, Seabird Electronics, Inc.), and dissolved oxygen (SBE 43, Seabird Electronics, Inc.) at a rate of 6 Hz, which, along with the image data, are transmitted via a fiber optic cable onto the shipboard computer.
Keywords: in situ imaging, zooplankton, acoustics, size distribution, community composition, patchiness, phytoplankton, sampling systems
Citation: Greer AT, Lehrter JC, Binder BM, Nayak AR, Barua R, Rice AE, Cohen JH, McFarland MN, Hagemeyer A, Stockley ND, Boswell KM, Shulman I, deRada S and Penta B (2020) High-Resolution Sampling of a Broad Marine Life Size Spectrum Reveals Differing Size- and Composition-Based Associations With Physical Oceanographic Structure. Front. Mar. Sci. 7:542701. doi: 10.3389/fmars.2020.542701
Received: 13 March 2020; Accepted: 27 November 2020;
Published: 22 December 2020.
Edited by:
Christian Grenz, UMR7294 Institut Méditerranéen d’Océanographie (MIO), FranceReviewed by:
Fabien Lombard, Sorbonne Universités, FranceAnna Metaxas, Dalhousie University, Canada
Copyright © 2020 Greer, Lehrter, Binder, Nayak, Barua, Rice, Cohen, McFarland, Hagemeyer, Stockley, Boswell, Shulman, deRada and Penta. This is an open-access article distributed under the terms of the Creative Commons Attribution License (CC BY). The use, distribution or reproduction in other forums is permitted, provided the original author(s) and the copyright owner(s) are credited and that the original publication in this journal is cited, in accordance with accepted academic practice. No use, distribution or reproduction is permitted which does not comply with these terms.
*Correspondence: Adam T. Greer, YXRncmVlckB1Z2EuZWR1