- 1Exploration Technology Lab, National Geographic Society, Washington, DC, United States
- 2Pristine Seas, National Geographic Society, Washington, DC, United States
There is a growing need for marine biodiversity baseline and monitoring data to assess ocean ecosystem health, especially in the deep sea, where data are notoriously sparse. Baited cameras are a biological observing method especially useful in the deep ocean to estimate relative abundances of scavenging fishes and invertebrates. The National Geographic Society Exploration Technology Lab developed an autonomous benthic lander platform with a baited camera system to conduct stationary video surveys of deep-sea megafauna. The first-generation landers were capable of sampling to full ocean depth, however, the form factor, power requirements, and cost of the system limited deployment opportunities. Therefore, a miniaturized version (76 cm × 76 cm × 36 cm, 18 kg in air) was developed to provide a cost-effective method to observe ocean life to 6000 m depth. Here, we detail this next-generation deep-sea camera system, including the structural design, scientific payload, and the procedures for deployment. We provide an overview of NGS deep-sea camera system deployments over the past decade with a focus on the performance improvements of the next-generation system, which began field operations in 2017 and have performed 264 deployments. We present example imagery and discuss the strengths and limitations of the instrument in the context of existing complementary survey methods, and for use in down-stream data products. The key operational advantages of this new instrument are spatial flexibility and cost-efficiency. The instrument can be hand-deployed by a single operator from a small craft concurrent with other shipboard operations. The main limitation of the system is battery power, which allows for 6 h of continuous recording, and takes up to 8 h to recharge between deployments. Like many baited-camera methods, this instrument is specialized to measure the relative abundance of mobile megafauna that are attracted to bait, which results in a stochastic snapshot of the species at the deployment location and time. The small size and ease of deployment of this next-generation camera system allows for increased sample replication on expeditions, and presents a path forward to advance cost-effective biological observing and sustained monitoring in the deep ocean.
Introduction
There is a growing need for marine biodiversity baseline and monitoring data to assess ocean ecosystem health, especially in the deep sea, where data is notoriously sparse (Costello et al., 2010; Levin et al., 2019; Rogers et al., 2020). Emerging deep-sea methods and technologies, such as remote autonomous benthic landers with high-definition imaging capability, can aid international biodiversity monitoring networks (e.g., the Marine Biodiversity Observation Network), extend observing capacity into the deep ocean (Muller-Karger et al., 2018; Moltmann et al., 2019; Rogers et al., 2020), and aid scientific networks (e.g., Biology and Ecosystems Panel of the Global Ocean Observing System; Deep Ocean Observing Strategy) to incorporate cost-effective biological observing capabilities into integrated deep ocean assessments (Levin et al., 2019). Harnessing emerging technologies for deep-sea biological observation is critical to creating a global knowledge base of this ecosystem about which very little is known (Levin et al., 2019).
New biological data obtained from high definition imaging can be used to build the marine Essential Biodiversity Variables (EBVs) (Pereira et al., 2013; Muller-Karger et al., 2018) and biological Essential Ocean Variables (EOVs) (Miloslavich et al., 2018; Bax et al., 2019). These variables are necessary for ecosystem indicator development and reporting over the Ocean Decade (Moltmann et al., 2019; Ryabinin et al., 2019; Tanhua et al., 2019), which help to build a better understanding of the health of ocean ecosystems. However, it is critical that the strengths and limitations inherent to emerging observing methods are transparent. This enables observers and users to converge on agreed data standards and practices that complement existing approaches (Pearlman et al., 2019). Such transparency and data standards are necessary to ensure that data obtained from emerging methods contribute to the development of ecosystem indicators that address policy and management requirements (e.g., suitable for building EBV/EOV data products) (Hardisty et al., 2019; Jetz et al., 2019).
Autonomous free-fall lander vehicles (untethered instrumented seafloor platforms) for deep-sea research were developed in the 1930s (Ewing and Vine, 1938), and have since been used successfully to sample full ocean depth (Jamieson et al., 2009; Hardy et al., 2013), and for a variety of deep-sea research purposes (Phleger and Soutar, 1971; Smith et al., 1976). Landers offer many advantages over human-operated submersible vehicles, and ship-tethered equipment such as Remote Operated Vehicles (ROVs), towed camera sleds, and trawls (Isaacs and Schick, 1960; Worcester et al., 1997; Priede and Bagley, 2000). The main advantage is that autonomous landers enable simultaneous deployments to obtain spatial replication of surveys, and can be conducted while the ship performs concurrent operations, significantly optimizing expensive ship time (Brandt et al., 2016). To unlock the potential to sample the deep ocean at scale, autonomous lander vehicles offer a promising direction for the future of deep-sea research with improved sampling efficiency compared to ship-tethered equipment (Brandt et al., 2016).
Landers equipped with baited cameras have proven to be an especially useful tool for biological observation in the deep ocean where food is limited (Willis and Babcock, 2000; Trenkel et al., 2004; Bailey et al., 2007; Drazen and Sutton, 2017). The bait attracts mobile fishes and invertebrates to the camera, and data extracted from the footage can be used to estimate relative abundances of these scavenging taxa (Farnsworth et al., 2007; Harvey et al., 2007; Yeh and Drazen, 2011; Linley et al., 2016; Leitner et al., 2017). Therefore, baited cameras are commonly used to study top predator communities in the deep sea (Priede and Bagley, 2000; Bailey et al., 2007; Yeh and Drazen, 2009; Drazen and Sutton, 2017), and share many common sampling features with shallow-water Baited Remote Underwater Video Station (BRUVS) techniques (Langlois et al., 2018), so that standards can be shared and data could be comparable among methodologies (Whitmarsh et al., 2017).
However, due to their size and weight, many lander vehicles still require large ships with winch capabilities to operate them (e.g., Hardy et al., 2013; but see Phillips et al., 2019; Gallo et al., 2020). In 2009, the National Geographic Society (NGS) Exploration Technology Lab developed a hand-deployable deep-sea lander and camera system that has since been deployed opportunistically across the world’s ocean, largely on NGS Pristine Seas expeditions (e.g., Friedlander et al., 2019; Giddens et al., 2019; Table 1). The instrument is a remote autonomous benthic lander with a baited camera system, and is used for stationary video surveys of deep-sea demersal scavenging megafauna. The first-generation landers were capable of sampling to full ocean depth (Turchik et al., 2015) however the form factor (size and weight), power requirements, and cost of the system limited deployment opportunities. Therefore a next-generation miniaturized version was developed to optimize the physical design and scientific payload, providing improved efficiency and a cost-effective method to observe life in the deep sea to 6000 m depth.
In this paper we detail the novel next-generation NGS deep-sea camera system, including the structural design and scientific payload, and describe the methodological procedures, including set up, deployment, recovery, and data management. We describe instrument uses over a decade of operation (2010–2020) and discuss the performance of the next-generation system, which was used from 2017 onward for improved efficiency in deep-ocean benthic observing. Example imagery is presented, and discussed in terms of the strengths and limitations of the observing method in relationship to existing complementary BRUVS survey methods. The resulting data dimensions and attributes from the observing instrument and sampling approach are discussed in terms of suitability for use in downstream EBV/EOV data products. This work represents a significant step forward in improved efficiency and cost-reduction to conduct stationary video surveys in the deep ocean, increase opportunities for sampling, and obtain marine biodiversity baseline and monitoring data to assess ocean ecosystem health.
Materials and Equipment
The NGS Deep-Sea Camera System
While the first iteration of the deep-sea camera system was developed to reach full ocean depth (Turchik et al., 2015), subsequent improvements in design and economy present increased opportunities for deployment and use for the marine biodiversity observing community. Below we describe the next generation of the “mini” NGS deep-sea camera system (rated to 6000 m depth), which is small (76 cm × 76 cm × 36 cm), lightweight (18 kg in air), and low-cost (∼ $10,000 USD to manufacture) compared to many existing technologies. Details of build (mechanical illustration, electrical block diagrams, firmware state machine diagram) are available at https://github.com/NGS-Exploration-Technology/Deep-Sea-Camera-System.
Structural Design
The physical layout of the deep-sea camera system consists of a glass housing with external lights, a shaft that holds a bait canister, and the release mechanism that attaches to the anchor (Figure 1). The glass pressure housing is a 33 cm diameter, 1.2 cm thick borosilicate glass sphere (Vitrovex, Nautilus Marine, GmbH). The upper and lower hemispheres are sealed at the center with butyl rubber tape followed by vinyl tape, to maintain 0.6 atm inside the sphere during operation. The bottom hemisphere houses a high definition (HD) camera, the camera controller, and batteries (details below) (Figure 2A). The upper hemisphere contains the Argos satellite beacon and VHF beacon used for recovery (details below), and the electrical bulkhead for data download and battery charging (Figure 2B). A set of magnet switches activates the camera controller and the recovery beacon (Figure 2B).
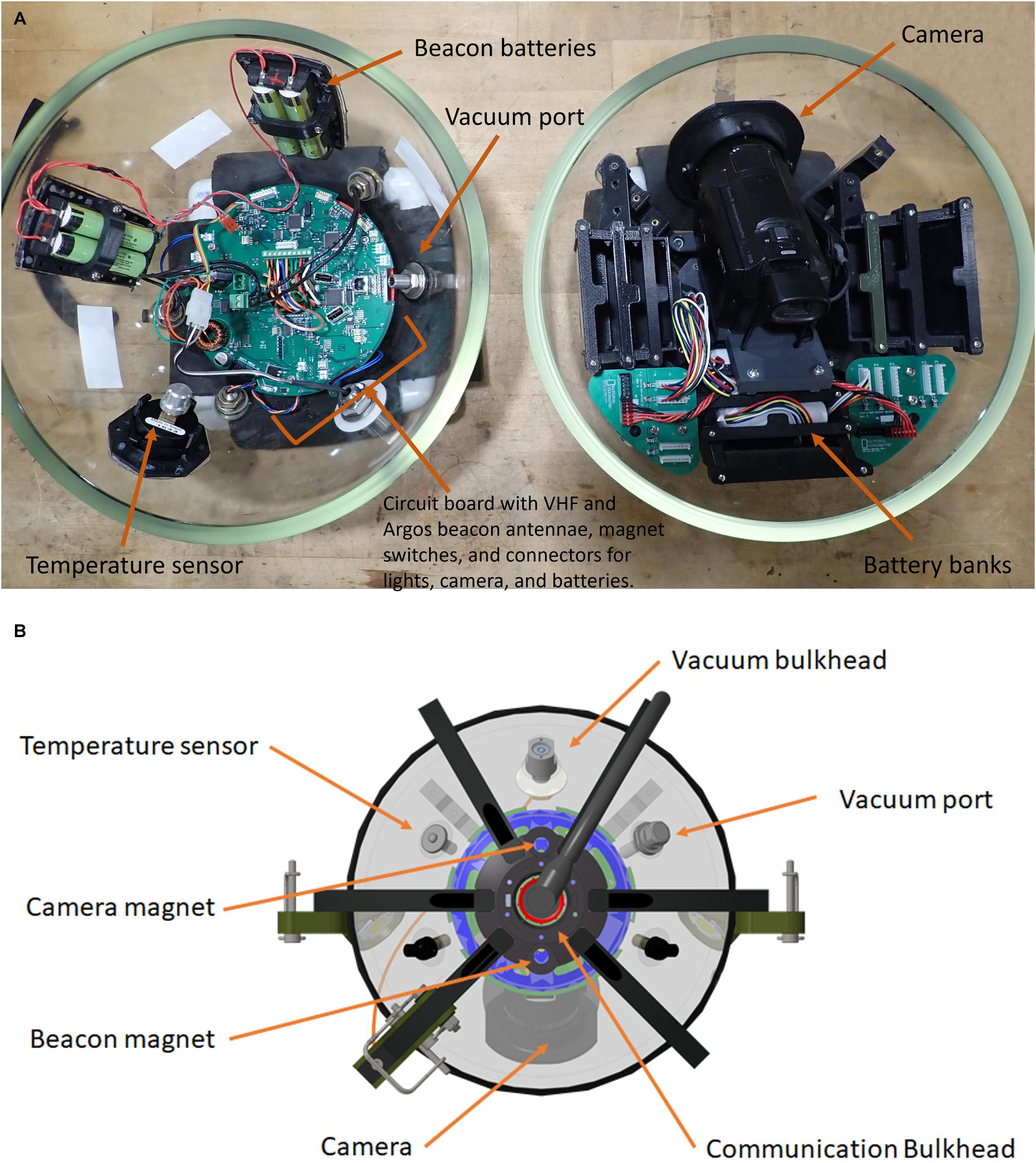
Figure 2. (A) Two hemispheres of the NGS deep-sea camera system; lower hemisphere (right) contains the high definition (HD) camera; upper hemisphere (left) contains the Argos satellite beacon and VHF beacon, and the electrical bulkhead for data download and battery charging; (B) outside view diagram of upper hemisphere; communication bulkhead in the center, along with magnets, sensors, and vacuum port.
The stainless steel detachable bottom shaft (4.5 cm diameter, 41 cm long) holds the bait canister, which is a removable PVC pipe perforated with 1.3 cm holes (3.2 cm horizontal distance between holes, 2.5 cm vertical distance between holes) to allow the odor of the bait to disperse without loss of bait. At the bottom of the shaft is an attachment point for the release system (details below) and temporary anchor (Figure 1). The anchor is comprised of a piece of biodegradable line tied to a 100% cotton biodegradable pillowcase filled with local sand (measured to weigh 10 kg). With a 10 kg anchor, the instrument descends at ∼ 1.1 m/s. The anchor line extends 2 m from the seafloor to optimize the field of view to both capture large mobile scavenging megafauna and allow for identification of organisms on or above the benthos.
The release system consists of a single-use burn-wire and a back-up Galvanic Time Release (GTR) (Neptune Marine Supply) (Figure 1). The primary burn-wire system consists of a stainless steel nylon-coated wire with a 6 mm section of coating removed. The exposed wire is connected to a bolt. At the user-designated pre-programmed time, these components are energized via the camera controller and pressure-housing bulkhead, which causes the exposed wire to disintegrate through electrolytic erosion. This releases the anchor, and the camera system ascends to the surface under its own buoyancy (9.28 N) at ∼ 1 m/s. Should the primary release mechanism fail, the GTR serves a back-up function. The GTR (secured between the shaft/burn-wire and the anchor system) begins corroding upon immersion in salt water, and takes between 24 and 36 h to completely disintegrate, depending on seawater temperature and salinity.
The recovery beacon is activated with a magnet switch, and consists of a 401 MHz Argos satellite transmitter (Telonics), for global tracking, and a 150 MHz VHF transmitter (Telonics) (for short-range tracking). The beacon is powered by four parallel 3.6 V, 3.4 Ah lithium ion primary batteries, with 1-year battery life in continuous operation. The camera controller consists of a custom-designed PCB based on the Microchip Technology PIC24 series microcontroller. A single waterproof bulkhead (BH10FTI, SubConn), externally located on the upper hemisphere, provides an electrical connection for charging and data retrieval and can be accessed through this bulkhead. The camera system is powered by a bank of 14.4 V, 2.6 Ah lithium ion batteries providing 337 Wh., with the capacity for 6 h of video recording with illumination (see Supplementary Table 1 for battery-duration field-testing results).
The deep-sea camera system uses a high efficiency LED lighting system (Cree XLamp CMT1930 LED), which consists of two lights each placed approximately 318 mm from the center of the sphere, with a viewing angle of 115°. The lights are angled downward at a constant 45° from the horizontal plane, with an illumination of 1530 lm and a color temperature of 4000 K.
Scientific Payload
The camera is a Sony Handycam, FDR-AX33 4K Ultra-High Definition (3840 × 2160) video camera with a 20.6 megapixel still image capability. The camera is fixed at a 45° declination from the horizontal plane and has a 35 mm equivalent focal length of 29.8 mm, which results in a horizontal 62.27° and a vertical 37.53° angular field-of-view. The camera has a wide-angle adapter, which provides a 0.70 nominal magnification factor. Video is stored as an mp4 file. Typically the camera is programmed to record 2 out of 10 min, and turns on 10 s before the lights, though mission parameters are fully customizable depending on user needs, programmed through the externally located electrical bulkhead. Additionally, onboard sensors measure temperature (Texas Instruments, LM34CAZ) and pressure (Keller, 7LY HP) for each deployment.
Methods
The objective of this method is to conduct stationary video surveys of bathyal demersal mobile scavenging megafauna, and to contribute fish and invertebrate abundance and distribution observations to the global ocean and biodiversity observing community. The deep-sea camera system deployment process has four stages: setup (∼ 30 min.), deployment (∼ 15 min.), recovery (depends on sea state), and data management (30 min download time for 1 h of video footage). Battery-charging time can take up to 8 h. The set up process includes testing and loading the mission, setting up the release mechanism, attaching the anchor, and loading the bait. Further details for each step are found in the Users Manual at https://github.com/NGS-Exploration-Technology/Deep-Sea-Camera-System.
Deployment Process
Deploying the camera system takes one or two people, and can be done from a range of craft sizes, from small rigid inflatables to a large research vessel. If the water line is not directly accessible from a vessel, a slip line can be used to lower the instrument slowly into the water, however, it is preferable to remove any unnecessary lines as they present opportunity for entanglement.
The video survey time is programmed prior to deployment. It is likely that deep-sea camera deployments should last a minimum of 6 h, even at the shallow depth-range (e.g., 200 m), based on the bait plume detection (Priede and Merrett, 1998; Jamieson, 2016). Just prior to deployment, the beacon and camera system are activated by removing magnets that otherwise keep those systems off. A GPS location and time (UTC) is marked upon deployment. Based on the pre-programmed deployment time, and the calculation of ascent rate, the operator can estimate the expected time of surfacing, and arrive to the deployment area for device recovery, ready to retrieve the camera upon surfacing.
For instrument recovery, if the operator is within several hundred meters of the device, it can be detected visually by the high-visibility orange flag (during daylight hours) or reflective bands on the flag (during nighttime hours). Additionally, external lights flash every 10 s once the mission timer has elapsed, aiding visual detection at night. If the operator on the open ocean is within 10 km of the device at the surface, the bearing for navigation can be determined using a directional Yagi antenna with a radio receiver tuned to the specific frequency of the instrument’s VHF beacon. The radio signal is, however, inhibited by landmasses and should be considered when deploying in settings such as fjord lands or around small islands. Beyond 10 km distance, the operator can track the device using location fixes from the Argos satellite network forwarded to an operator’s satellite phone. Fair seas and frequent Argos satellite passing can provide a location fix with an accuracy of within 250 m. However, the accuracy of the location coordinates can vary greatly (e.g., sea state, broadcast signal strength, etc.) therefore this system should be used only in case of unforeseen circumstances (e.g., early release, deteriorated sea state prohibiting retrieval when the device first reaches the surface).
Following retrieval, video footage and sensor data are downloaded from the device to an external hard drive and the system batteries are recharged. All of this is executed through the single waterproof bulkhead, thus the hemispheres do not need to be separated between deployments, and barring unforeseen complications, should be able to remain intact throughout the duration of an expedition. The same bulkhead and cabling used for data download is also used to set the next mission’s parameters.
Data Management
To efficiently process and manage the data, the video files are stored in “Tator,” which is a cloud-based collaborative video annotation platform developed by CVisionAI1. Annotations are made in Tator and proceed from a standard annotation methodology currently in development for the NGS deep-sea camera system’s remote video surveys (Marsh et al., in prep). This annotation protocol is Darwin Core compliant with standardized taxonomic nomenclature according to the World Register of Marine Species (WoRMS), and adheres to the Ocean Biodiversity Information System (OBIS) data standard formats for image-based marine biology (Costello et al., 2013; De Pooter et al., 2017; Provoost et al., 2017). Uncertainty classification is provided with controlled vocabulary according to the “Open Nomenclature” standard identification qualifiers (e.g., comments on video quality and taxonomic identification resolution) (Sigovini et al., 2016).
Metrics and Biodiversity Analyses
In the video analysis stage, annotations are made from the video footage to identify species to the highest taxonomic resolution, and obtain a relative abundance metric (maximum number per frame, or MaxN). The maximum number of individuals of each species in a video frame, rather than a total tally per deployment, is recorded to ensure that individuals are not double counted (Langlois et al., 2018). Other variables that may be quantified from this approach include time of first arrival of a taxon, and time at which the maximum number of a taxon is observed, time being relative to the landing of the device on the seafloor (e.g., Leitner et al., 2017; Linley et al., 2018).
To assess biodiversity across sites, Hill numbers (the effective number of species) can be used as a unified way to compare measures of biodiversity with varying sample coverage (Chao et al., 2014). Coverage-based rarefaction (interpolation) and extrapolation (prediction) curves with a bootstrap routine can be produced in R (iNEXT package; Hsieh et al., 2016), and used to estimate asymptotic diversity. For example, Giddens et al. (2019) used data from the deep-sea camera system to produced fish family accumulation curves for four sites in the Tropical Eastern Pacific, and found that sample completeness ranged from 84.3% at Clipperton (13 deployments) to 100% at Malpelo (19 deployments). Further, multivariate statistics and generalized hierarchical models can be used to compare community structure between depths and habitat types and, coupled with publicly available oceanographic data, across regions to evaluate environmental drivers of biodiversity (e.g., Giddens et al., 2019).
Results
NGS Deep-Sea Camera System Uses
With the NGS deep-sea camera system, 595 deployments have been conducted on 39 expeditions from 2010 to 2020 (Table 1). For the next-generation version, which was used from 2017 onward, 264 deployments were conducted on 17 expeditions. Deployments for the next-generation system ranged in depth from 31 to 5296 m, and in duration from 7 to 94 h per expedition. Deployment length ranged from 1 to 12 h, and the average video length obtained per deployment ranged from 1 h 21 min to 4 h 33 min per deployment (Table 1). Example imagery is shown in Figure 3, illustrating a diversity of taxa, habitats, depths, and geographies imaged during expeditions. Complete annotations for all previous expeditions are still in process, thus the number of organisms observed in this dataset are not reported here. However, previous studies have assessed local biodiversity and community composition from shallow to deep ecosystems (e.g., Friedlander et al., 2019) performed regional comparisons in bathyal demersal scavenging megafauna (e.g., Tropical Eastern Pacific; Giddens et al., 2020), published new species records (e.g., Galapagos; Buglass et al., 2020), and described novel behavioral observations (e.g., Eastern Pacific Black Ghost Shark [Hydrolagus melanophasma]; Giddens et al., 2020).
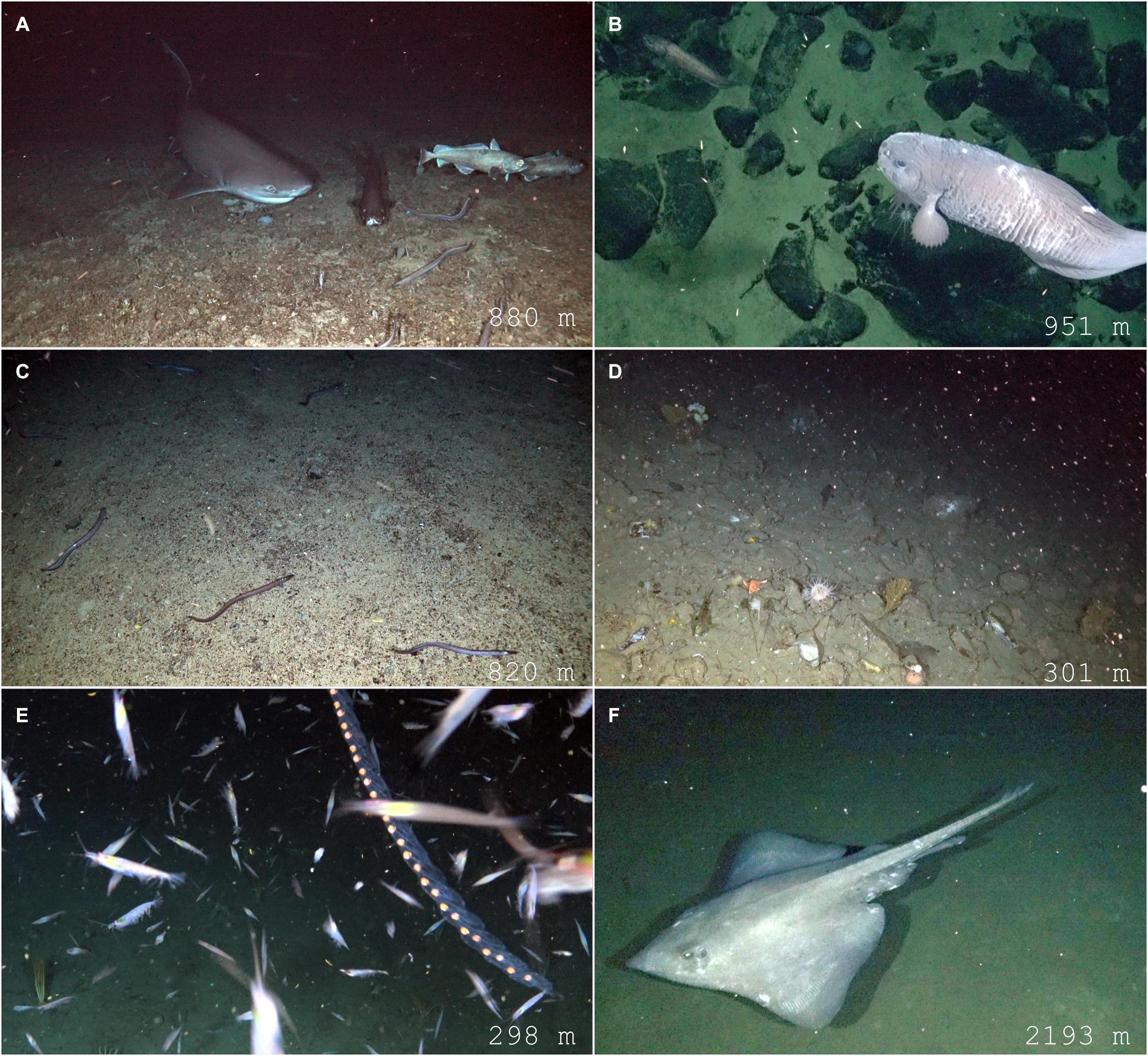
Figure 3. Example imagery from NGS deep-sea camera system: (A) Hexanchus griseus, Dalatias licha, Synaphobranchidae, and Mora moro, at 880 m on a seamount in the Azores; (B) Liparid at 951 m at Malpelo Island; (C) Elongate taxa, Synaphobranchus sp., at 820 m in the Azores; (D) Benthic taxa in background of a frame can be difficult or impossible to confidently identify; 301 m on the Antarctic shelf; (E) Siphonophora and light-attracted krill (Eupahsiidae); 298 m on the Antarctic shelf; (F) Bathyraja spinosissima, at 2193 m at Malpelo Island.
The NGS deep-sea camera system has been used across a variety of different geomorphological zones and habitats (Table 1). It has been used extensively on seamounts (e.g., Easton et al., 2017; Buglass et al., 2020), in oceanic island/archipelagos (e.g., Giddens et al., 2020), polar shelf areas (e.g., Friedlander et al., 2020) and fjordlands (Chile, Southeast Alaska; Table 1). While the first-generation landers made exploratory deployments to hadal zones in ocean trenches (e.g., Puerto Rico, Tonga and Marianas Trenches) up to 10,641 m depth, the majority of deployments made with the next-generation deep-sea camera system have been conducted in <3000 m (Table 1). The current deployment dataset represents 19 of the 37 different Ecological Marine Units (EMUs; Sayre et al., 2017), with deployments in water masses of varying temperatures, oxygen levels, and chemical properties (nitrate, phosphate, silicate) (Sayre et al., 2017). Most expeditions represented 4–6 different EMUs, with some expeditions in the Pacific Ocean sampling up to 8 different EMUs (e.g., Desventuradas, Palau, Niue, Malpelo, Costa Rica) (Table 1).
The deep-sea camera system is usually deployed and recovered once per day from the main ship or a support vessel (e.g., zodiac), while other operations are carried out simultaneously. The device can be hand-deployed and requires just one operator. Typically, 2–4 instruments are brought on expeditions to allow for simultaneous deployments. This spatial flexibility and cost-efficiency in deployment operations, including reduced ship and personnel time required, are the key operational advantages of this method over larger lander systems and ship-tethered equipment.
The main limitations of the method are battery power, which limits recording time to 6 h in total per deployment, and the battery charging time between deployments, which can take up to 8 h. However, because of the single bulkhead for data retrieval, battery charging, and mission programming, the pressure housing remains sealed over the course of the expedition, which makes the deployment turnaround process simple and relatively time efficient. Thus, the system can be in near continuous operation over the course of an expedition, factoring in time for data retrieval and battery charging.
NGS Deep-Sea Lander Performance
The next-generation NGS deep-sea camera system performed successfully over the 264 deployments conducted since 2017 (Table 1). The instrument has been recovered on 100% of its deployments, though it has relied on the back-up release mechanism (GTR) and back-up Argos satellite tracking system on occasion. Release issues were most commonly due to flawed electrical connection to the burn-wire (user error in set up), or sharks biting the burn-wire release cable causing an early release. In the Seychelles, surface currents were strong enough that after a shark-induced early release, the system was carried away from the deployment site. In this case, the Argos satellite tracking provided device location after surfacing. On one occasion in the Galapagos (Tropical Eastern Pacific), the device became entangled with the substrate after release, but eventually freed itself and was recovered using the Argos satellite tracking system on the surface. The first-generation system suffered two losses in ocean trenches and abyssal plains >6000 m, surmised to be from defects in the glass that caused a catastrophic implosion (Turchik et al., 2015). However, for the next-generation system described here, no structural errors persist and the instrument has performed successfully on its 264 deployments above 6000 m.
NGS Deep-Sea Imaging System Performance
The NGS deep-sea camera system has produced high-quality imagery of the deep-benthos (Figure 3). The system is designed to optimize the survey field of view and the ability to identify distinguishing characteristics of mobile taxa (e.g., pectoral and anal fins, head characteristics) (Figures 3A,B,F). This optimization is achieved with the oblique camera angle, which permits larger areas of seafloor to be visible compared with downward-facing (vertical) photography (Jamieson, 2016). This combination of camera and angle (Ultra-High Definition at 45°), LED lighting (1530 lm), and altitude (2 m above the seafloor) provides sufficient field of view (horizontal = 62.27°, vertical = 37.53°) and lighting to identify large mobile taxa. However, small sessile fauna (e.g., meiofanua, infauna) in the background of a frame can be difficult or impossible to confidently identify (e.g., Figure 3D), therefor this method focuses on assessments of mobile megafauna.
The NGS deep-sea camera system is free to spin in full 360-degree rotation around the anchor line axis, typically due to current, or an animal coming into physical contact with the camera. One disadvantage of this design compared to downward facing cameras, is that if the angled camera is facing away from where the animal is approaching, the video could miss the exact time of arrival (Jamieson, 2016). To capture the variability of survey area observed, rotation degree is recorded in the annotation process, quantified as a portion of a full 360-degree view (e.g., 90, 180, 270, and 360) represented during the deployment.
One challenge in animal identification with the current system is that the bait canister is situated below the camera and outside the field of view (Figure 1). Initially designed to keep the video frame unobstructed, this placement can, however, make taxonomic identification more challenging, as some animals approach the bait and obscure the camera lens. Future design improvements are planned to modify this feature so that the bait is within the field of view to aid in species identification to the highest possible taxonomic resolution.
Discussion
The next-generation NGS deep-sea camera system and deployment process has proven to be a robust and efficient deep-sea stationary video survey method. To ensure that data obtained is suitable for downstream use in EBV/EOV data product development, and interoperable with existing survey methods, we discuss the strengths and limitations of the method for consideration in future use.
Advantages and Limitations of Method
A major advantage of the NGS deep-sea camera system method, as with other BRUVS, is that it is a non-extractive technique, and can be used in delicate habitats (e.g., deep-sea coral gardens) (Jamieson, 2016; Whitmarsh et al., 2017; Levin et al., 2019). Further, for fish communities, an advantage of video rather than physical sampling, is that slender bodied species (e.g., Synaphobranchus sp.) (Figure 3C) can escape through mesh used in physical sampling, creating a sampling artifact based on body-size and form (Clark et al., 2016). However, as a BRUVS survey method, the NGS deep-sea camera system is specialized to assess a target group (mobile predators with scavenging tendencies that are attracted to the bait), and is not intended to gather data on the full biological community (Farnsworth et al., 2007; Yeh and Drazen, 2011) as this method cannot consistently image small sessile fauna (e.g., meiofanua, infauna) (Bailey et al., 2007; e.g., Figure 3D). Further, chance encounters with non-attracted species (e.g., Siphonophora, Figure 3E) are not uncommon, but should be analyzed separately from bait-attracted taxa. Similarly, some animals are attracted not by the bait, but by some other disturbance introduced by the camera system, such as light (e.g., Eupahsiidae, Figure 3E). These non-bait-attracted animal observations are noted during video review and annotation, and filtered accordingly in statistical analyses and inference of the mobile scavenging megafaunal assemblage. This limitation should be transparent in any presentation or discussion of the interpreted data.
Measures of density per unit volume are not obtained directly from survey footage because the current speed and direction affect the bait plume area of detection (Priede and Merrett, 1998). Therefore, any measure of density from baited cameras is considered theoretical based on models of the bait plume over distance and time (Priede and Merrett, 1998; Jamieson, 2016). The odor detection threshold is unknown for most species, however theoretical models predict a plume area of 0.6 km2 after 6 h, and 2.4 km2 after 12 h (assuming an average current speed of 0.05 ms–1) (Jamieson, 2016). Relative (rather than absolute) measures of abundance (MaxN) from the resultant footage is emphasized in data products and metadata to acknowledge the systemic bias of the method, not only for bait-attracted fauna in general, but also for varying behavior effects within the scavenging and predator assemblage (e.g., behavior to different bait-types, and species-specific reactions to artificial illumination). Species-specific reactions are expected to be consistent across sampling, so relative estimates are achievable with a consistent survey method. With these caveats noted, this method is specialized to measure the relative abundance and distribution for target taxa.
Avoidance Behavior
Many deep-sea species are highly sensitive to artificial illumination, and so are repelled rather than attracted to the survey area (Fitzpatrick et al., 2013). While there is no evidence for absence of species due to artificial illumination, visual impairment can result in behavior change and thus uncertainty in baited camera results (Jamieson, 2016). A variety of animal responses to light are noted in the literature and range from blindness for some species to no effect for others (Jamieson, 2016). For example, deep-water eels (Synaphobranchus kaupii) decreased in numbers with illumination (Bailey et al., 2005) and sablefish (Anoplopoma fimbria) showed a flight response with white, but not far red light (Raymond and Widder, 2007). The Eastern Pacific Black Ghost shark (Hydrolagus melanophasma) was deterred from un-baited ROV surveys (James et al., 2009), however we saw no flight response in this species when using the NGS deep-sea camera system at Clipperton Atoll in the Tropical eastern Pacific (Giddens et al., 2020). As baited cameras typically use lower intensity and less-sustained illumination, compared to ROV and submersible surveys, the BRUVS method is less likely to disturb deep-sea fauna (Jamieson, 2016). Because of the systemic bias of artificial light introduced to a dark environment during deep-sea deployments, it is important that lighting intensity remains consistent among video surveys, in order to produce comparative relative estimates of biodiversity and community composition among locations (Hammerschlag et al., 2017).
For bait-selection, fishes that are native to the experimental site are more representative of the natural setting (Jamieson, 2016). Therefore, the specific bait type will vary among sampling campaigns specific to the region (Langlois et al., 2018). However, the use of deep-sea fish as bait may cause avoidance in conspecifics (Barry and Drazen, 2007), so surface-dwelling or pelagic fishes of the region should be used.
Sampling Approach
The current NGS deep-sea camera system dataset (from years 2010 to 2020) consists of baseline observations and opportunistic sampling with point video surveys, producing species inventories for bait-attracted fauna. However, given the opportunistic model, past sampling campaigns rarely sampled evenly among depth strata and habitat types. Given variation in detectability among species and habitats, species-absences may be less-reliably measured by opportunistic point video surveys (Jetz et al., 2019). Especially for mobile groups such as predators and scavenging megafauna, larger or longer-lasting survey campaigns offer more reliable evidence of taxon absence. Repeated surveys that use a standardized protocol for sampling presence-absence at multiple time points are currently in development. Metadata reporting for each campaign, past and planned, will provide information about the extent, resolution, measurement units, and uncertainties of spatial, temporal, and taxonomic data dimensions (Jetz et al., 2019).
Deployment Time per Depth
Generally, as depth increases, faunal density decreases over the vast expanse of the seafloor (Schiaparelli et al., 2016). Therefore, it is expected that with greater depth, a longer sampling time is needed to assess biodiversity and scavenger community composition with baited camera surveys (Whitmarsh et al., 2017). Sampling methods for shallow-water BRUVS (e.g., 0–100 m) are well established, and consistently use 1-hour deployment times (Langlois et al., 2018). However, the optimum sampling time in bathyal and abyssal depths are still unknown (Whitmarsh et al., 2017). To optimize deep BRUVS deployment protocols, a measure of the minimum time for video deployments per depth strata is needed to ensure adequate sampling for MaxN without diminishing returns on recording time invested (Giddens et al., in prep). Further, as the density of organisms is expected to vary with levels of particulate organic carbon (POC) reaching the deep-sea from the surface waters (Levin et al., 2001) measures of time-to-MaxN should be determined for an array of seascape regimes (Giddens et al., in prep).
Conclusion
The deep ocean presents a unique challenge for biological observing. While the density of any kind of data in the deep ocean is low, this is especially true for biological observations (Levin et al., 2019). Routine observations over time will be essential for environmental monitoring of the deep ocean, especially for Ecosystem Impact Assessments associated with resource extraction, area-based management, and climate change studies (Levin et al., 2019). To attain this data requirement efficiently, there is a need to incorporate emerging technology, such as low-cost imaging, into observing strategy plans (Canonico et al., 2019; Levin et al., 2019).
The next-generation NGS deep-sea camera system described here is a low-cost, lightweight device that can be deployed from nearly any vessel and added to an existing monitoring program to extend biological observing capacity into the deep sea. By detailing the deep-sea camera system elements and deployment protocols, along with the strengths and limitations of the method, our intention is to connect this information with ocean observers using similar methods and sampling approaches, and work toward common data standards to enable interoperability. Harmonized data streams can be used in conjunction to measure and report on the status of benthic fish assemblages at a global scale, and advance decadal goals for an integrated ocean observing system (Pearlman et al., 2019; Ryabinin et al., 2019).
Data Availability Statement
The original contributions presented in the study are included in the article/Supplementary Material, further inquiries can be directed to the corresponding author.
Author Contributions
JG and AT conceived the manuscript. JG coordinated the author contributions, wrote the text, edited, and contributed to the figures. WG and MR contributed to the manuscript figures and text. DD and AT contributed to the manuscript ideas and text. All authors approved the submission.
Funding
This work was funded by the National Geographic Society.
Conflict of Interest
The authors declare that the research was conducted in the absence of any commercial or financial relationships that could be construed as a potential conflict of interest.
Acknowledgments
We thank Enric Sala, Alan Friedlander, and the Pristine Seas team for their support of the NGS Deep-Sea Research Project. We also thank Alex Moen and the Explorer Engagement Program at NGS, and Deep-Sea Research team members Benjamin Woodward, Leigh Marsh, Jeffrey Drazen, Virginia Moriwake, and Katherine Croff Bell. We would like to thank Kasie Coccaro, Kyler Abernathy, and the Exploration Technology Lab members past and present, including Eric Berkenpas, Mike Shepard, Brad Henning, Jessica Elfadl, and Fabien Laurier. Finally we thank the editor and the two reviewers, whose comments have greatly improved this manuscript.
Supplementary Material
The Supplementary Material for this article can be found online at: https://www.frontiersin.org/articles/10.3389/fmars.2020.601411/full#supplementary-material
Footnotes
References
Bailey, D. M., Genard, B., Collins, M. A., Rees, J. F., Unsworth, S. K., Battle, E. J., et al. (2005). High swimming and metabolic activity in the deep-sea eel Synaphobranchus kaupii revealed by integrated in situ and in vitro measurements. Physiol. Biochem. Zool. 78, 335–346. doi: 10.1086/430042
Bailey, D. M., King, N. J., and Priede, I. G. (2007). Cameras and carcasses: historical and current methods for using artificial food falls to study deep-water animals. Mar. Ecol. Prog. Ser. 350, 179–191. doi: 10.3354/meps07187
Barry, J. P., and Drazen, J. C. (2007). Response of deep-sea scavengers to ocean acidification and the odor from a dead grenadier. Mar. Ecol. Prog. Ser. 350, 193–207. doi: 10.3354/meps07188
Bax, N. J., Miloslavich, P., Muller-Karger, F. E., Allain, V., Appeltans, W., Batten, S. D., et al. (2019). A response to scientific and societal needs for marine biological observations. Front. Mar. Sci. 6:395. doi: 10.3389/fmars.2019.00395
Brandt, A., Gutt, J., Hildebrandt, M., Pawlowski, J., Schwendner, J., Soltwedel, T., et al. (2016). Cutting the umbilical: new technological perspectives in benthic deep-sea research. J. Mar. Sci. Eng. 4:36. doi: 10.3390/jmse4020036
Buglass, S., Nagy, S., Ebert, D., Sepa, P., Turchik, A., Bell, K. L., et al. (2020). First records of the seven−gilled Notorynchus cepedianus and six−gilled Hexanchus griseus sharks (Chondrichthyes: Hexanchiformes: Hexanchidae) found in the Galápagos marine reserve. J. Fish Biol. 97, 926–929. doi: 10.1111/jfb.14447
Canonico, G., Buttigieg, P. L., Montes, E., Stepien, C. A., Wright, D., Benson, A., et al. (2019). Global observational needs and resources for marine biodiversity. Front. Mar. Sci. 6:367. doi: 10.3389/fmars.2019.00367
Chao, A., Gotelli, N. J., Hsieh, T. C., Sander, E. L., Ma, K. H., Colwell, R. K., et al. (2014). Rarefaction and extrapolation with Hill numbers: a framework for sampling and estimation in species diversity studies. Ecol. Monogr. 84, 45–67. doi: 10.1890/13-0133.1
Clark, M. R., Bagley, N. W., and Harley, B. (2016). “Trawls,” in Biological Sampling in the Deep Sea, eds M. R. Clark, M. Consalvey, and A. A. Rowden (Oxford: Wiley Blackwell), 16–35.
Costello, M. J., Bouchet, P., Boxshall, G., Fauchald, K., Gordon, D., Hoeksema, B. W., et al. (2013). Global coordination and standardisation in marine biodiversity through the World Register of Marine Species (WoRMS) and related databases. PLoS One 8:e51629. doi: 10.1371/journal.pone.0051629
Costello, M. J., Coll, M., Danovaro, R., Halpin, P., Ojaveer, H., and Miloslavich, P. (2010). A census of marine biodiversity knowledge, resources, and future challenges. PLoS One 5:e12110. doi: 10.1371/journal.pone.0012110
De Pooter, D., Appeltans, W., Bailly, N., Bristol, S., Deneudt, K., Eliezer, M., et al. (2017). Toward a new data standard for combined marine biological and environmental datasets-expanding OBIS beyond species occurrences. Biodivers. Data J. 5:e10989. doi: 10.3897/bdj.5.e10989
Drazen, J. C., and Sutton, T. T. (2017). Dining in the deep: the feeding ecology of deep-sea fishes. Ann. Rev. Mar. Sci. 9, 337–366. doi: 10.1146/annurev-marine-010816-060543
Easton, E. E., Sellanes, J., Gaymer, C. F., Morales, N., Gorny, M., and Berkenpas, E. (2017). Diversity of deep-sea fishes of the Easter Island Ecoregion. Deep Sea Res. 2 Top. Stud. Oceanogr. 137, 78–88. doi: 10.1016/j.dsr2.2016.12.006
Ewing, M., and Vine, A. (1938). Deep−sea measurements without wires or cables. Eos Trans. Am. Geophys. Union 19, 248–251. doi: 10.1029/tr019i001p00248
Farnsworth, K. D., Thygesen, U. H., Ditlevsen, S., and King, N. J. (2007). How to estimate scavenger fish abundance using baited camera data. Mar. Ecol. Prog. Ser. 350, 223–234. doi: 10.3354/meps07190
Fitzpatrick, C., McLean, D., and Harvey, E. S. (2013). Using artificial illumination to survey nocturnal reef fish. Fish. Res. 146, 41–50. doi: 10.1016/j.fishres.2013.03.016
Friedlander, A. M., Giddens, J., Ballesteros, E., Blum, S., Brown, E. K., Caselle, J. E., et al. (2019). Marine biodiversity from zero to a thousand meters at Clipperton Atoll (Île de La Passion), Tropical Eastern Pacific. PeerJ 7:e7279. doi: 10.7717/peerj.7279
Friedlander, A. M., Goodell, W., Salinas-de-León, P., Ballesteros, E., Berkenpas, E., Capurro, A. P., et al. (2020). Spatial patterns of continental shelf faunal community structure along the Western Antarctic Peninsula. PLoS One 15:e0239895. doi: 10.1371/journal.pone.0239895
Gallo, N. D., Hardy, K., Wegner, N. C., Nicoll, A., Yang, H., and Levin, L. A. (2020). Characterizing deepwater oxygen variability and seafloor community responses using a novel autonomous lander. Biogeosciences 17, 3943–3960. doi: 10.5194/bg-17-3943-2020
Giddens, J., Goodell, W., Friedlander, A., Salinas-de-León, P., Shepard, C., Henning, B., et al. (2019). Patterns in bathyal demersal biodiversity and community composition around archipelagos in the Tropical Eastern Pacific. Front. Mar. Sci. 6:388. doi: 10.3389/fmars.2019.00388
Giddens, J., Salinas-de-León, P., Friedlander, A., Ebert, D. A., Henning, B., and Turchik, A. (2020). First observation of a Hydrolagus melanophasma (Chondrichthyes, Chimaeriformes, Holocephali) aggregation with egg cases extruding from a female. Mar. Biodivers. 50:91. doi: 10.1007/s12526-020-01122-3
Hammerschlag, N., Meyer, C. G., Grace, M. S., Kessel, S. T., Sutton, T. T., Harvey, E. S., et al. (2017). Shining a light on fish at night: an overview of fish and fisheries in the dark of night, and in deep and polar seas. Bull. Mar. Sci. 93, 253–284. doi: 10.5343/bms.2016.1082
Hardisty, A. R., Michener, W. K., Agosti, D., García, E. A., Bastin, L., Belbin, L., et al. (2019). The Bari Manifesto: an interoperability framework for essential biodiversity variables. Ecol. Inform. 49, 22–31. doi: 10.1016/j.ecoinf.2018.11.003
Hardy, K., Cameron, J., Herbst, L., Bulman, T., and Pausch, S. (2013). “Hadal landers: the DEEPSEA CHALLENGE ocean trench free vehicles,” in Proceedings of the Oceans 2013 MTS/IEEE- San Diego, (New York, NY: IEEE), 1–10.
Harvey, E. S., Cappo, M., Butler, J. J., Hall, N., and Kendrick, G. A. (2007). Bait attraction affects the performance of remote underwater video stations in assessment of demersal fish community structure. Mar. Ecol. Prog. Ser. 350, 245–254. doi: 10.3354/meps07192
Hsieh, T. C., Ma, K. H., and Chao, A. (2016). iNEXT: an R package for rarefaction and extrapolation of species diversity (Hill numbers). Methods Ecol. Evol. 7, 1451–1456. doi: 10.1111/2041-210x.12613
Isaacs, J. D., and Schick, G. B. (1960). Deep-sea free instrument vehicle. Deep Sea Res. (1953) 7, 61–67. doi: 10.1016/0146-6313(60)90009-5
James, K. C., Ebert, D. A., Long, D. J., and Didier, D. A. (2009). A new species of chimaera, Hydrolagus melanophasma sp. nov.(Chondrichthyes: Chimaeriformes: Chimaeridae), from the eastern North Pacific. Zootaxa 2218, 59–68. doi: 10.11646/zootaxa.2218.1.3
Jamieson, A. J. (2016). “Landers baited cameras and traps,” in Biological Sampling in the Deep Sea, eds M. R. Clark, M. Consalvey, and A. A. Rowden (Hoboken, NJ: John Wiley & Sons), 228–259. doi: 10.1002/9781118332535.ch11
Jamieson, A. J., Solan, M., and Fujii, T. (2009). Imaging deep-sea life beyond the abyssal zone. Sea Technol. 50, 41–46.
Jetz, W., McGeoch, M. A., Guralnick, R., Ferrier, S., Beck, J., Costello, M. J., et al. (2019). Essential biodiversity variables for mapping and monitoring species populations. Nat. Ecol. Evol. 3, 539–551. doi: 10.1038/s41559-019-0826-1
Langlois, T., Williams, J., Monk, J., Bouchet, P., Currey, L., Goetze, J., et al. (2018). “Marine sampling field manual for benthic stereo BRUVS (Baited Remote Underwater Videos),” in Field Manuals for Marine Sampling to Monitor Australian Waters, eds R. Przeslawski and S. Foster (Canberra, ACT: National Environment Science Programme Marine Biodiversity Hub), 82–104.
Leitner, A. B., Neuheimer, A. B., Donlon, E., Smith, C. R., and Drazen, J. C. (2017). Environmental and bathymetric influences on abyssal bait-attending communities of the Clarion Clipperton Zone. Deep Sea Res. 1 Oceanogr. Res. Pap. 125, 65–80. doi: 10.1016/j.dsr.2017.04.017
Levin, L. A., Bett, B. J., Gates, A. R., Heimbach, P., Howe, B. M., Janssen, F., et al. (2019). Global observing needs in the deep ocean. Front. Mar. Sci. 6:241. doi: 10.3389/fmars.2019.00241
Levin, L. A., Etter, R. J., Rex, M. A., Gooday, A. J., Smith, C. R., Pineda, J., et al. (2001). Environmental influences on regional deep-sea species diversity. Annu. Rev. Ecol. Syst. 32, 51–93. doi: 10.1146/annurev.ecolsys.32.081501.114002
Linley, T. D., Craig, J., Jamieson, A. J., and Priede, I. G. (2018). Bathyal and abyssal demersal bait-attending fauna of the Eastern Mediterranean Sea. Mar. Biol. 165:159.
Linley, T. D., Gerringer, M. E., Yancey, P. H., Drazen, J. C., Weinstock, C. L., and Jamieson, A. J. (2016). Fishes of the hadal zone including new species, in situ observations and depth records of Liparidae. Deep Sea Res. 1 Oceanogr. Res. Pap. 114, 99–110. doi: 10.1016/j.dsr.2016.05.003
Miloslavich, P., Bax, N. J., Simmons, S. E., Klein, E., Appeltans, W., Aburto−Oropeza, O., et al. (2018). Essential ocean variables for global sustained observations of biodiversity and ecosystem changes. Glob. Change Biol. 24, 2416–2433. doi: 10.1111/gcb.14108
Moltmann, T., Zhang, H. M., Turton, J. D., Nolan, G., Gouldman, C. C., Griesbauer, L., et al. (2019). A Global Ocean Observing System (GOOS), delivered through enhanced collaboration across regions, communities, and new technologies. Front. Mar. Sci. 6:291. doi: 10.3389/fmars.2019.00291
Muller-Karger, F. E., Miloslavich, P., Bax, N. J., Simmons, S., Costello, M. J., Sousa Pinto, I., et al. (2018). Advancing marine biological observations and data requirements of the complementary essential ocean variables (EOVs) and essential biodiversity variables (EBVs) frameworks. Front. Mar. Sci. 5:211. doi: 10.3389/fmars.2018.00211
Pearlman, J., Bushnell, M., Coppola, L., Karstensen, J., Buttigieg, P. L., Pearlman, F., et al. (2019). Evolving and sustaining ocean best practices and standards for the next decade. Front. Mar. Sci. 6:277. doi: 10.3389/fmars.2019.00277
Pereira, H. M., Ferrier, S., Walters, M., Geller, G. N., Jongman, R. H. G., Scholes, R. J., et al. (2013). Essential biodiversity variables. Science 339, 277–278.
Phillips, B. T., Licht, S., Haiat, K. S., Bonney, J., Allder, J., Chaloux, N., et al. (2019). DEEPi: a miniaturized, robust, and economical camera and computer system for deep-sea exploration. Deep Sea Res. 1 Oceanogr. Res. Pap. 153:103136. doi: 10.1016/j.dsr.2019.103136
Phleger, C. F., and Soutar, A. (1971). Free vehicles and deep-sea biology. Am. Zool. 11, 409–418. doi: 10.1093/icb/11.3.409
Priede, I. G., and Bagley, P. M. (2000). In situ studies on deep-sea demersal fishes using autonomous unmanned lander platforms. Oceanogr. Mar. Biol. 38, 357–392.
Priede, I. G., and Merrett, N. R. (1998). The relationship between numbers of fish attracted to baited cameras and population density: studies on demersal grenadiers Coryphaenoides (Nematonurus) armatus in the abyssal NE Atlantic Ocean. Fish. Res. 36, 133–137. doi: 10.1016/s0165-7836(98)00105-2
Provoost, P., De Pooter, D., Appeltans, W., Bailly, N., Bristol, S., Deneudt, K., et al. (2017). Expanding the Ocean Biogeographic Information System (OBIS) beyond species occurrences. Biodivers. Inform. Sci. Stand. 1:e20515. doi: 10.3897/tdwgproceedings.1.20515
Raymond, E. H., and Widder, E. A. (2007). Behavioral responses of two deep-sea fish species to red, far-red, and white light. Mar. Ecol. Prog. Ser. 350, 291–298. doi: 10.3354/meps07196
Rogers, A., Aburto-Oropeza, O., Appeltans, W., Assis, J., Ballance, L. T., Cury, P., et al. (2020). Critical Habitats and Biodiversity: Inventory, Thresholds and Governance. Washington, DC: World Resources Institute.
Ryabinin, V., Barbière, J., Haugan, P., Kullenberg, G., Smith, N., McLean, C., et al. (2019). The UN decade of ocean science for sustainable development. Front. Mar. Sci. 6:470. doi: 10.3389/fmars.2019.00470
Sayre, R. G., Wright, D. J., Breyer, S. P., Butler, K. A., Van Graafeiland, K., Costello, M. J., et al. (2017). A three-dimensional mapping of the ocean based on environmental data. Oceanography 30, 90–103. doi: 10.5670/oceanog.2017.116
Schiaparelli, S., Rowden, A. A., and Clark, M. R. (2016). “Deep-sea fauna,” in Biological Sampling in the Deep Sea, eds M. R. Clark, M. Consalvey, and A. A. Rowden (Oxford: Wiley Blackwell), 16–35.
Sigovini, M., Keppel, E., and Tagliapietra, D. (2016). Open nomenclature in the biodiversity era. Methods Ecol. Evol. 7, 1217–1225. doi: 10.1111/2041-210x.12594
Smith, K. L. Jr., Clifford, C. H., Eliason, A. H., Walden, B., Rowe, G. T., and Teal, J. M. (1976). A free vehicle for measuring benthic community metabolism 1. Limnol. Oceanogr. 21, 164–170. doi: 10.4319/lo.1976.21.1.0164
Tanhua, T., McCurdy, A., Fischer, A., Appeltans, W., Bax, N., Currie, K., et al. (2019). What we have learned from the framework for ocean observing: evolution of the global ocean observing system. Front. Mar. Sci. 6:471. doi: 10.3389/fmars.2019.00471
Trenkel, V. M., Lorance, P., and Mahévas, S. (2004). Do visual transects provide true population density estimates for deepwater fish? ICES J. Mar. Sci. 61, 1050–1056. doi: 10.1016/j.icesjms.2004.06.002
Turchik, A. J., Berkenpas, E. J., Henning, B. S., and Shepard, C. M. (2015). “The deep ocean dropcam: a highly deployable benthic survey tool,” in Proceedings of the OCEANS 2015-MTS/IEEE Washington, (New York, NY: IEEE), 1–8. doi: 10.1016/j.dsr2.2014.07.011
Whitmarsh, S. K., Fairweather, P. G., and Huveneers, C. (2017). What is big BRUVver up to? Methods and uses of baited underwater video. Rev. Fish Biol. Fish. 27, 53–73. doi: 10.1007/s11160-016-9450-1
Willis, T. J., and Babcock, R. C. (2000). A baited underwater video system for the determination of relative density of carnivorous reef fish. Mar. Freshw. Res. 51, 755–763. doi: 10.1071/mf00010
Worcester, P. F., Hardy, K. R., Horwitt, D. D., and Peckham, D. A. (1997). A deep ocean data recovery module. Oceanogr. Lit. Rev. 8:901.
Yeh, J., and Drazen, J. C. (2009). Depth zonation and bathymetric trends of deepsea megafaunal scavengers of the Hawaiian Islands. Deep Sea Res. 1 Oceanogr. Res. Pap. 56, 251–266. doi: 10.1016/j.dsr.2008.08.005
Keywords: BRUVS, Bathyal, autonomous vehicle, remote imaging, Essential Biodiversity Variables, Essential Ocean Variables, benthic lander, scavenging megafauna
Citation: Giddens J, Turchik A, Goodell W, Rodriguez M and Delaney D (2021) The National Geographic Society Deep-Sea Camera System: A Low-Cost Remote Video Survey Instrument to Advance Biodiversity Observation in the Deep Ocean. Front. Mar. Sci. 7:601411. doi: 10.3389/fmars.2020.601411
Received: 31 August 2020; Accepted: 07 December 2020;
Published: 11 January 2021.
Edited by:
Enrique Montes, University of South Florida, United StatesReviewed by:
Emmanuel Boss, The University of Maine, United StatesNatalya D. Gallo, University of California, San Diego, United States
Copyright © 2021 Giddens, Turchik, Goodell, Rodriguez and Delaney. This is an open-access article distributed under the terms of the Creative Commons Attribution License (CC BY). The use, distribution or reproduction in other forums is permitted, provided the original author(s) and the copyright owner(s) are credited and that the original publication in this journal is cited, in accordance with accepted academic practice. No use, distribution or reproduction is permitted which does not comply with these terms.
*Correspondence: Jonatha Giddens, am9uYXRoYUBoYXdhaWkuZWR1