- 1School of Natural Sciences, Macquarie University, North Ryde, NSW, Australia
- 2Department of Mathematics and Computer Science, Ursinus College, Collegeville, PA, United States
- 3Anderson Cabot Center for Ocean Life, New England Aquarium, Boston, MA, United States
- 4Department of Biology, Woods Hole Oceanographic Institution, Woods Hole, MA, United States
Abundance and population trends of Critically Endangered North Atlantic right whales (Eubalaena glacialis, NARW) have been estimated using mark-recapture analyses where an individual’s state is based upon set delineations of age, using historical estimates of age at first reproduction. Here we assigned individual females to states based upon their reproductive experience, rather than age. We developed a Bayesian mark-recapture-recovery model to investigate how survival, recapture, site-fidelity and dead-recovery probabilities vary for female NARW in different states, using data collected from 1977-2018. States were assigned as calves for individuals in their first year; pre-breeder for individuals greater than one year of age who had yet to produce a calf, or breeder if an individual had reproduced. A decline in abundance of female NARW was seen starting in 2014, with 185 females declining yearly to 142 by 2018. The largest decline was seen in breeding females, with 72 estimated to be alive at the beginning of 2018, while female pre-breeder abundance plateaued at around 70 between 2011- 2018. Females born from 2000 onwards had an average 4% (95% CI:0.03-0.06) chance of transitioning from pre-breeder to breeder, compared to 8% (95%CI:0.06-0.1) for females born prior. This reduction in transition rate from non-breeder to breeder for the current cohort resulted in breeding females declining to 51% of the female population by 2018. We show that a collapse in fecundity of breeding females, and the failure of pre-breeders to start breeding, is an important factor in understanding the current decline in abundance of the NARW.
Introduction
North Atlantic right whales (Eubalaena glacialis, NARW) are one of the most endangered species of extant whales (Cooke, 2020), with recovery post-whaling impaired by both acute and chronic human induced stressors often leading to mortality (Kraus, 1990; Knowlton and Kraus, 2001). Historically, there were two populations of NARW, an eastern population now believed extinct, and a western population found off the east coast of North America, which has been the subject of intensive research since the 1980’s (Notarbartolo di Sciara et al., 1998; Kraus et al., 2007). The most recent estimate for the entire western population is 366 individuals, and the species has declined in abundance since 2010 (Pace et al., 2017; Hayes et al., 2020). Environmental and anthropogenic stressors have been shown to impede the recovery of right whale populations by reducing calving rates (Christiansen et al., 2018; Christiansen et al., 2020). Recent work investigating the sub-lethal effects of ship strikes, entanglement and climate driven shifts in prey availability has shown that the lengths of individual right whales who have been entangled, and individuals whose mother was entangled during nursing, are shorter than non-entangled whales (Stewart et al., 2021). Entanglement was the one anthropogenic impact that could be definitively identified as causing stunting (Stewart et al., 2021), with per capita rates of entanglement initially peaking in 2000, though have been steadily increasing since 2007 (Knowlton et al., 2012; Stewart et al., 2021). There is a temporal component to stunting as well, with individuals born each year since 1981 becoming progressively smaller, with the difference now as large as a meter (Stewart et al., 2021). Stunted growth reduces available energy stores, resulting in individuals having less energy to invest into reproduction (Christiansen et al., 2020) as well as being less resilient to future entanglements (Stewart et al., 2021), and so increasing the risk of death. Adult female mortalities primarily caused by vessel strikes and entanglement in fishing gear also contribute to this decline, with few individuals left to contribute new members to the population (Corkeron et al., 2018).
Changes to a population, and its rate of increase, are well known to be influenced by the changes in fitness of different age groups or stage classes of individuals (Horvitz et al., 1997). In long-lived vertebrate species, such as the NARW, changes to juvenile survival are known to have a smaller influence on the population growth rate compared to an equivalent change in adult survival (Eberhardt, 1977; Gaillard et al., 2000). As such, in many large mammals, females appear to be selected to favor their own survival over reproductive events and are likely to only reproduce when in good condition (Gaillard and Yoccoz, 2003). However, temporal changes in the population growth rate can be more influenced by juvenile survival, used as a measure of recruitment into the breeding portion of the population, than by adult survival, because of the large variability that can occur within these traits (Gaillard et al., 1998). Understanding the influence of survival and the recruitment of breeding females on yearly variability in abundance is difficult, with elasticity analysis alone unable to separate the contributions, instead it must be combined with empirical estimates (Gaillard et al., 1998). Environmental variation can confound recruitment of breeders in ungulates, with unfavorable conditions leading to a large decrease in juvenile survival (Gaillard and Yoccoz, 2003). Therefore, understanding the relative influence of survival and recruitment of breeders is needed to elucidate the factors influencing changes in population trends. Changes in the survival and recruitment of individuals in different classes will influence the stage structure of a population leading to changes in the population growth rate (Baker et al., 2017). With recent declines in the abundance of the NARW, we require an understanding of how survival and recruitment of breeders have changed over time, and how these may have influenced the stage structure of the population, leading to the current state of the NARW population.
Due to the precarious state of the NARW, a number of mark-recapture models have been developed over the last 30 years aimed at improving our understanding of survival and abundance (Caswell et al., 1999; Fujiwara and Caswell, 2001; Fujiwara and Caswell, 2002; Pace et al., 2017). Historically, these mark-recapture models for the NARW imposed various life history state (referring to the assigned biological stage class, inferring the “true” biological state within the model) based upon the age and/or sighting history of an individual. Individuals were generally assigned as being juveniles if between one and nine years and had not previously bred, and adults if older than nine years, of unknown age and sighted for more than eight years, or if they had previously calved (Hamilton et al., 1998), or, in one study, as adults if 5 years of age or older (Pace et al., 2017). The life history based state used in these earlier studies approximated historic NARW life history, but more recent research indicates that female right whales are failing to calve despite being well beyond what was the presumed average age of first parturition (Kraus et al., 2007).
There are multiple factors contributing to this delay in first parturition, including intrinsic factors, such as failure to conceive and pregnancy loss (Browning et al., 2010). In addition, there are extrinsic factors, such as food limitation and stress from past entanglement, which can result in individuals having insufficient energy stores to reproduce (Moore et al., 2021). Imperfect observations could also confound the age of first parturition, with undetected calf mortalities biasing estimates for age at first parturition. Age at first parturition tends to be the most labile life history parameter in females of large mammals (Eberhardt, 1977; Gaillard et al., 2000). Photo-identification records indicate that in the past decade, female NARW that have previously calved have increased the years between having each calf, and females that have yet to calve are no longer doing so at around nine years of age. Shifts in breeding intervals and age at first parturition can influence the number of reproductively active individuals within the population, and influence the number of individual breeders recruited, resulting in shifts in abundance. NARW currently show greater heterogeneity around the age of first parturition than previously observed, suggesting the current approach of assigning adults as individuals nine years of age or older may no longer represent an accurate approximation of a stable stage structure (Jenouvrier et al., 2005; Jenouvrier et al., 2015). To account for this variability around age of maturation, we use three reproductive states where individuals are assigned as calves in their first year, pre-breeders for individuals older than one who are estimated to have not bred, and breeders for individuals who are known or estimated to have had one or more reproductive event.
Mark-recapture models use live sightings of individuals to investigate survival, and only incorporate known deaths as auxiliary data. In contrast, mark-recapture-recovery models include death as a known state into which an individual can transition. These models combine both live recapture and dead recovery data into a single model, which provides a more precise estimate for survival (Gilroy et al., 2012). Mark-recapture-recovery models also provide an estimate for fidelity by accounting for permanent emigrations from the study area, where fidelity is calculated as 1 – the probability of permanently emigrating the study area (Burnham, 1993; Kéry and Schaub, 2011). Emigration was included in the model to account for individuals within the population who disappear from the sighting history, though information is lacking about if the individual has died, or if they have moved to a poorly or unstudied area and are missed during survey efforts (Kraus et al., 1986; Right Whale Consortium, 2020). Traditional mark-recapture models cannot distinguish between mortality and emigration, and as such only estimate apparent survival, though by accounting for known deaths and fidelity, mark-recapture recovery models can provide a more precise estimate of survival, known as true survival (Lebreton et al., 1999). Mark-recapture-recovery analysis can improve parameter estimation and provide greater levels of precision with smaller sample sizes.
Using a multi-event framework, we fit a Bayesian mark-recapture-recovery model, which includes information on the known deaths of individuals (known as a ‘recovery’) as well as live re-sights, to investigate how survival varied with state for female NARW, and how state influenced the probability of females’ recapture and recovery. We focused on the influence of state to investigate how these parameters varied over the study period.
Methods
Photo-identification database
Photo-identification studies for North Atlantic right whales began in the 1960’s, and since the 1980’s there have been substantial aerial and boat surveys that focus on the species’ known habitats (Kraus et al., 1986; Pettis et al., 2004; Hamilton et al., 2007). The whales are individually identifiable using callosity patterns on their heads, lips, and chins, as well as unusual skin colorations and scars, making it possible to track individuals over time (Kraus et al., 1986). Surveys collect information on the sightings of individuals as well as data on location captured, entanglement history, calving events, body condition, and known deaths (referred to as recovered), forming an extensive database on the majority of animals in the western population (Right Whale Consortium, 2020). Sighting histories consisted of individuals along the full extent of their known habitat throughout the western North Atlantic. State determination was based upon the first year of sighting (Hamilton et al., 1998). Calving season is typically from December-February, so for this study we considered a year to be 1st November till 31st October to account for early births. Data on 318 female North Atlantic right whales, of which 199 were of known state (calf, pre-breeder, breeder) and 119 were of unknown or partially known state, photographically identified between 1977-2018 (Right Whale Consortium, 2020) were used for this study.
Capture histories
Capture histories were constructed as part of an open Jolly-Seber framework, by taking the observations of an individual in a given year and compressing them into a single value (1: seen as a calf, 2: seen as a pre-breeder, 3: seen as a breeder, 4: seen in unknown state, 5: recovered as a calf, 6: recovered as a pre-breeder, 7: recovered as a breeder, or 8: neither seen nor recovered). The occurrence of each whale during each of the 41 sample periods constitutes the capture history. The capture history is then combined with a period before the first year where all individuals were assigned the observation value of eight (neither seen nor recovered). After that initial period, individuals were assigned states, either calves for their first year following birth, pre-breeder for individuals of one or more years of age who had not calved, or breeders, who were individuals who had calved at some point over their lifespan. Once an individual was assigned as a breeder they could not transition back to pre-breeder. Individuals who could not be assigned to a state, were assigned as unknown state individuals. The unknown state was comprised of individuals who were not first sighted in their birth year, and individuals who had a sighting gap of one year or more after the age of five until sighted with a calf.
Mark-recapture-recovery model
We used a multi-event Jolly-Seber model (George, 1992; Schwarz and Arnason, 1996; Brooks et al., 2002; King, 2012) for the joint analysis of mark-recapture-recovery data (Lebreton et al., 1999; Kéry and Schaub, 2011). In this model, we considered ten true biological states: not yet entered (NE), calf alive within study site (CAI), pre-breeder alive within study site (PAI), breeder alive within study site (BAI), pre-breeder alive outside study site (PAO), breeder alive outside study site (BAO), calf recently dead (CRD), pre-breeder recently dead (PRD), breeder recently dead (BRD), and dead (†). When an individual is encountered it can be difficult to correctly identify the state, especially for things like reproductive status, leading to the use of Hidden Markov Models to analyse mark-recapture data in order to distinguish between the observation and the latent state (Gimenez et al., 2012). Numerous approaches to parameterize this type of uncertainty have been developed (Nichols et al., 2004; Conn and Cooch, 2009; Sanz-Aguilar et al., 2011). Here we use an approach based upon that described in Gimenez et al. (2012), where unknown state assignment (δ) probability for individuals whose state could not be ascertained is estimated as one minus the probability of correctly assigning an individual’s state for both pre-breeders and breeders (Gimenez et al., 2012). The focal parameters for this model are presented in Table 1.
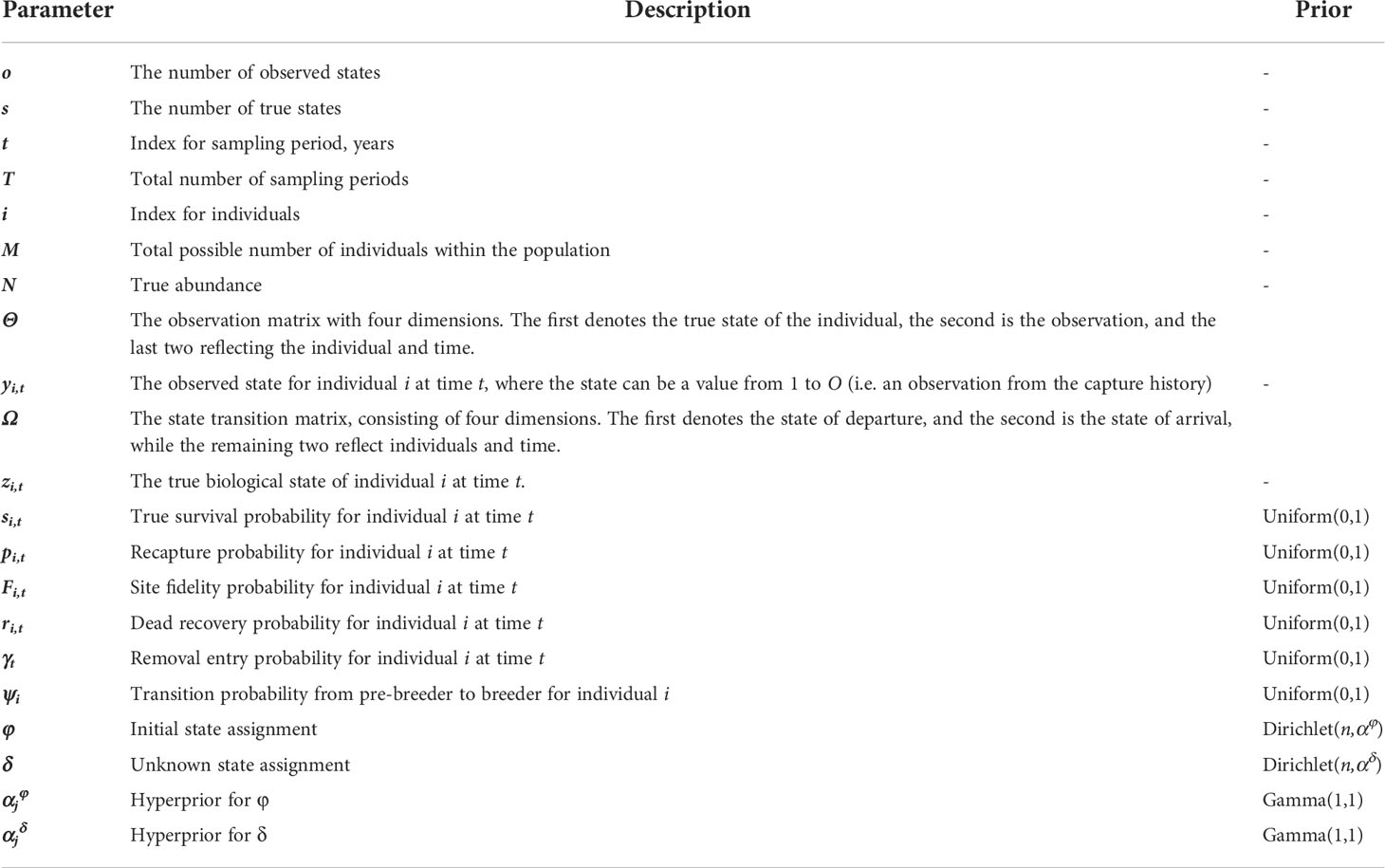
Table 1 List of notations and focal parameters estimated, brief description and priors used within the mark-recapture-recovery model.
The state of all individuals i at the first occasion (zi,1) was set such that they were considered to have not yet entered the population (state one):
The state of individual i at the second occasion (zi,2) is:
While subsequent states are conditional on the state at the previous time period, thus we denote the state model as
where Zi,t specifies the state of individual i at time t, and Ωzi,t,1…s,i,t describes state membership over time, where s is the number of true states (Kéry and Schaub, 2011).
The observation model is denoted as
where Yi,t specifies the observation of individual i at time t, and Θzi,t,1…o,i,t describes the observational process, linking the true states to the observed states, where o is the number of observed states (Kéry and Schaub, 2011).
Transition probability was modelled with the individual specific coefficient of cohort, where an individual was assigned a: 1) if they were sighted pre-2000; 2) if they were born in 2000 or later; and NA) if they entered the population after 2000 in an unknown state, or younger than five, due to their inability to transition, as well as individuals added in data augmentation. Cohorts were divided at the year 2000 due to the increase in the per capita rates of entanglement (Knowlton et al., 2012). To illustrate the modeling process, multinomial tree diagrams are presented showing the stage structure and a visual representation of the model (Figure 1).
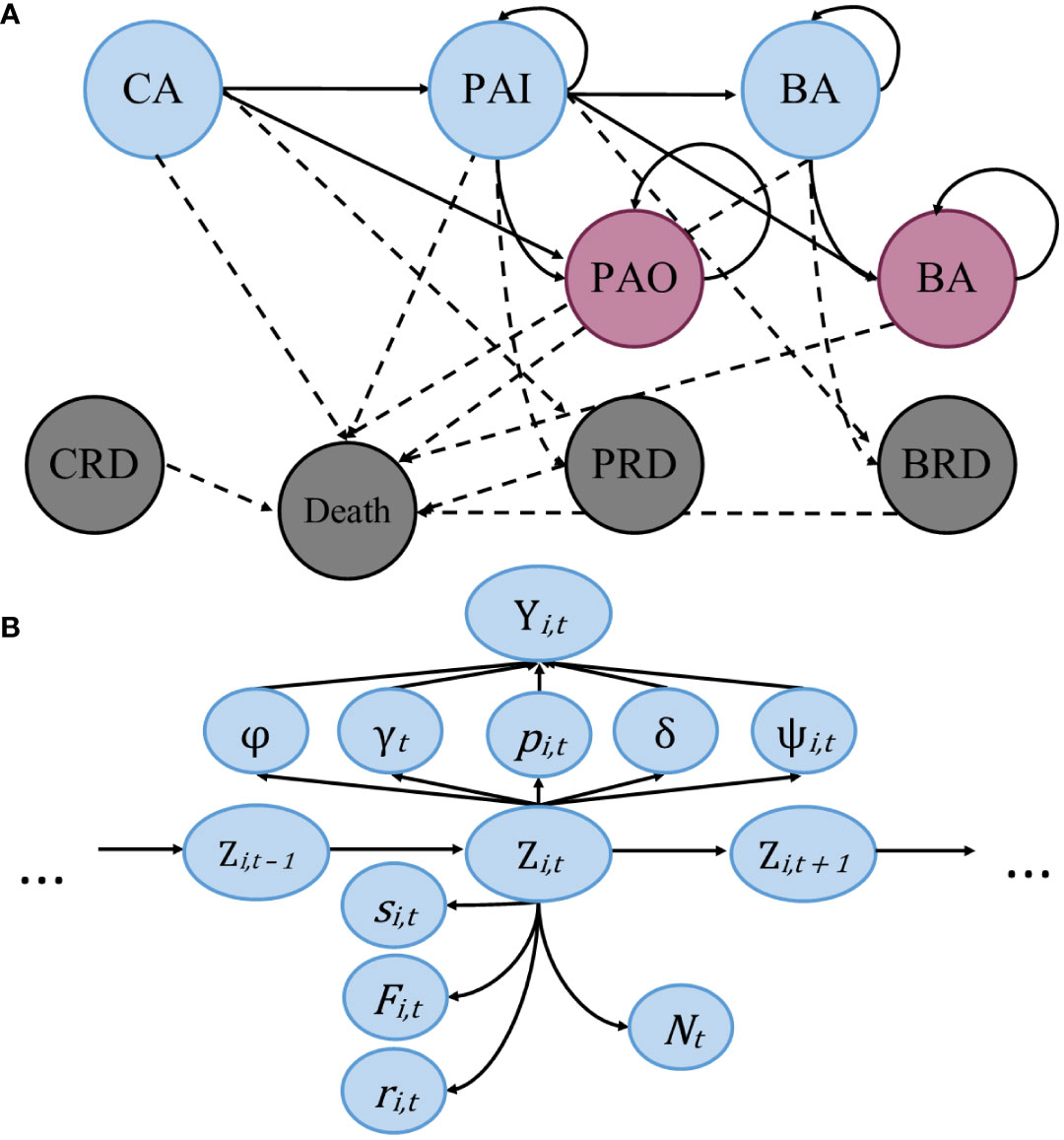
Figure 1 Multinomial tree diagrams showing the transitions between states and the model structure. (A) Simplified stage structure of the model, with circles representing states of an individual, blue circles represent individuals alive within the study area, red represents individuals alive outside the study area, and grey represents dead individuals. Solid lines represent the movement of individuals between states from time t to t+1, and dotted lines representing possible transitions to mortality. (B) Graphical depiction of the statistical model. State Z is dependent upon removal entry probability (γ), initial state assignment (φ), transition probability from pre-breeder to breeder (ψ), unknown state assignment (δ), and recapture probability (p), all of which are dependent upon the observation (Y). Lastly, we have true survival probability (s), fidelity probability (F), recovery probability (r), and abundance (N), which are estimated as a function of individual state, informed by the available data.
Therefore, the general structure of joint posterior for this multi-state mark-recapture-recovery model is:
Vague Uniform prior distributions were used to allow for a natural parametrization of the model, as per Royle and Dorazio (2008). Dirichlet priors were used for initial state and unknown state assignment to ensure they were in the interval [0,1] and sum to 1, followed by the relation:
Initial values were provided for the assignment of unknown individuals within the observation matrix. Different initial values were provided for each of the three chains, with one assigning all unknowns as breeders, another assigning all unknowns as pre-breeders and finally, one with half unknowns randomly assigned as breeders and half as pre-breeders.
Model fitting was done using the program JAGS (version 4.3) (Plummer, 2003), run in R (R Core Team, 2017), via the package r2jags (version 0.6-1) (Su and Yajima, 2020). The model was run for 200000 iterations, consisting of three chains, with 100000 iterations discarded as burn-in, and no thinning. Fitting the model was computationally expensive, taking ~11 days to run on an Intel Core i5-8400 processor with 8GB RAM. Convergence was assessed both visually using trace plots, as well as by the statistic (Brooks, 1998; Brooks and Gelman, 1998; Korner-Nievergelt et al., 2015). Posterior predictive checks were used to assess the fit of the model. Further detail on the model structure and R code can be found in the SI.
Fecundity
Detection of calving events occurs when a female is sighted accompanying a calf in the southern calving grounds. The calving grounds are intensively surveyed, with the number of individuals into the catalog who are of unknown age decreasing since 1990, suggesting that calving events are rarely missed (Right Whale Consortium, 2020). Using the annual calving data and estimates for females in the breeding population, we calculated fecundity as:
Where the number of reproductively available females is the number of breeders in a given year minus the number of calves born in the previous two years. We used this definition of reproductively available to account for the three year reproductive cycle of NARW’s, thus excluding individuals who would be unavailable to calf in a given year.
An annual per capita productivity index (API) was also calculated as:
Where Nt is the estimated female abundance for time t from the MRR output. We compared annual estimates for survival, fecundity and API to help understand trends in abundance and productivity over time.
Results
Abundance steadily increased until 2010, then remained relatively stable with minor fluctuations until 2013, after which estimated abundance decreased (Figure 2A). In 2018 total abundance of female right whales was estimated at 142 individuals (95% CI: 135-150). The population saw a peak in female abundance in 2010/11, estimated at 185 (95% CI: 183-188) in 2010 and 184 (95% CI: 183-187) in 2011 (Figure 2A). In 2018 breeding females made up just slightly more than half of the female population (73 individuals, 95% CI: 67-81), compared to a peak in proportional abundance in 2010, when they were approximately 60% of the female population (112 individuals, 95% CI: 108-116) (Figure 2B). Estimates for mean abundance of pre-breeders remained relatively stable from 1990 until 2009 (range: 29-47), after which it increased in 2010 and plateaued until 2018 (range 49-73) (Figure 2B). Peaks in the number of female calves were seen in 2001 and 2005 with 13 calves born each year, and again in 2009 with 18 calves born (Figure 2B).
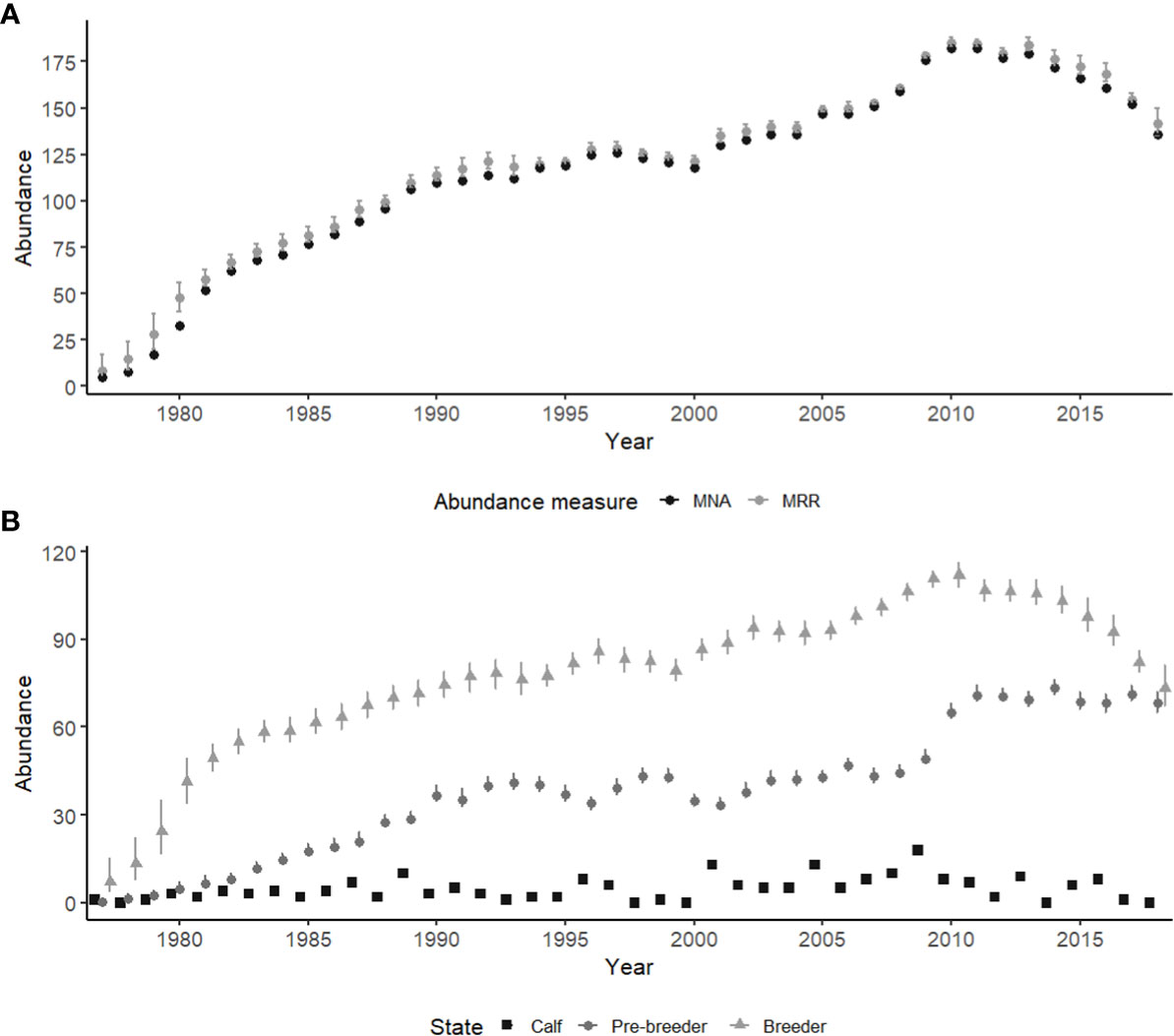
Figure 2 Abundance estimates for female North Atlantic right whales from 1977-2018. (A) Abundance estimates for female North Atlantic right whales, mark-recapture-recovery (MRR) model estimates (grey), compared to minimum number alive (MNA) for each year (black). (B) The breakdown of abundance into state with calf (squares), pre-breeders (circles) and breeders (triangles). Error bars indicate the 95% credible intervals. Calf abundance was derived from counts of female calves sighted yearly, and are not estimated.
Estimated true survival, i.e., accounting for permanent emigration as opposed to apparent survival [see (Kéry and Schaub, 2011)] for pre-breeders and breeders showed little fluctuation between years from the mid 1980’s until 2018, with a mean survival estimate of 0.96 (range: 0.92-0.98) and 0.97 (range: 0.94-0.99) respectively (Figure 3A). Yearly fluctuations in the estimates of true survival were greatest for calves, with a mean true survival of 0.79 (range: 0.50-0.92) (Figure 4). Estimates for calf true survival showed greater uncertainty in years with fewer individuals born (Figures 2B, 3A) (SI). Fidelity estimates for both breeders and pre-breeders remained consistently high from the mid 1980’s until 2015, after which uncertainty in the estimates for breeders increased (Figure 3B).
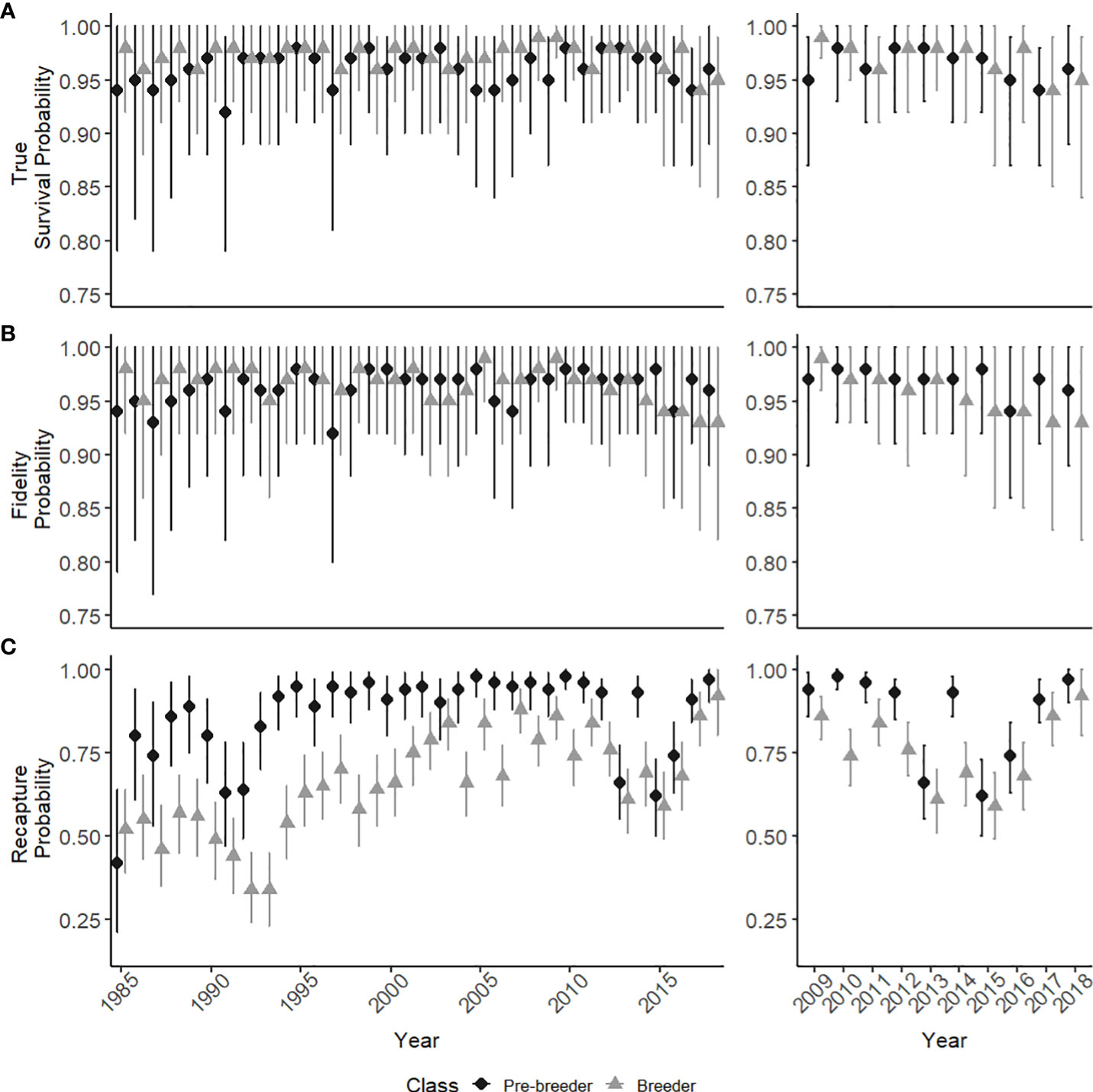
Figure 3 State specific parameter estimates from 1985-2018 with 95% credible intervals (95% CI), for pre-breeders (dark circles) and breeders (light triangles). (A) The true survival probability (B) Fidelity probability (C) The recapture probability. Windows show a detailed view of the last ten modelled years.
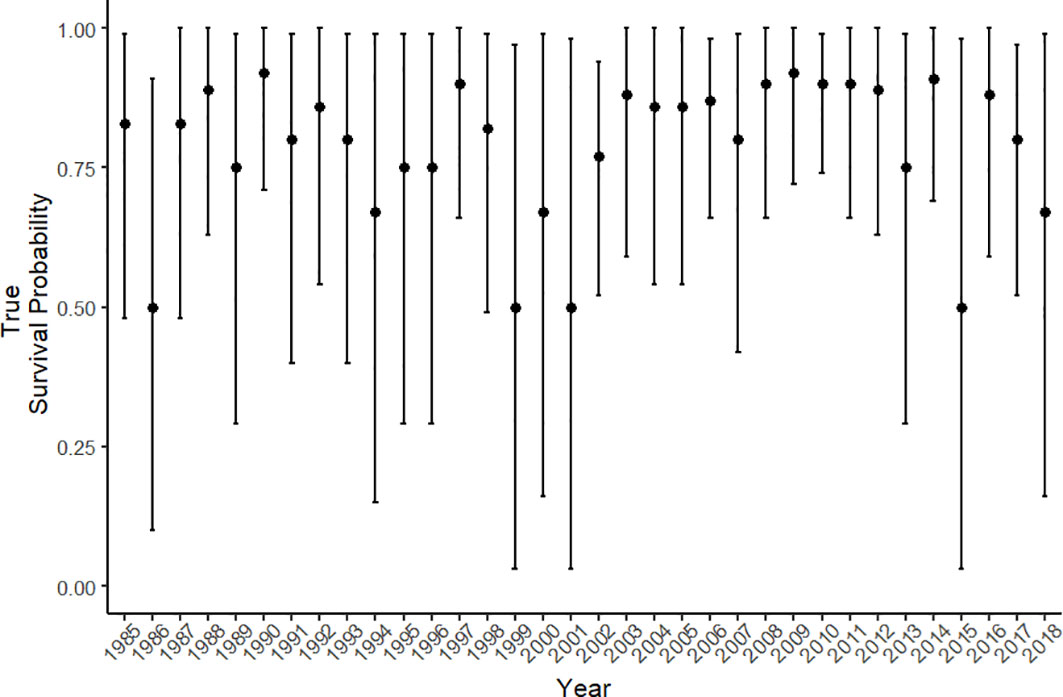
Figure 4 The true survival probability for calves from 1985 – 2018. Error bars represent the 95% credible intervals.
Recapture probability was consistently higher for pre-breeders compared to breeders from the mid 1980’s until 2010 (Figure 3C). In 2013, the recapture probability declined for both pre-breeders (0.66, 95% CI: 0.55-0.77) and breeders (0.61, 95% CI: 0.51-0.70), with a gradual increase since 2016 (Figure 3C). Individual heterogeneity in recapture probabilities can lead to underestimation of abundance estimates when not accounted for (Kéry and Schaub, 2011). Additional models were analyzed, which included individual heterogeneity to account for the potential bias. Abundance estimates did not differ between the two versions of the model, with the model accounting for individual heterogeneity discarded due to overparameterization and computational time.
Individuals assigned as pre-breeders who were born between 2000 and 2018 had an average probability of 0.04 (95% CI: 0.03-0.06) of transitioning to a breeder per year, while individuals born pre-2000 had an average transition probability of 0.08 (95%CI: 0.06-0.1). Fecundity, or the proportion of calves born per reproductively available female per year, was seen to decline post 2011 to a low of 0.04 in 2017, following a relatively stable period from 2001-2011 (range: 0.15-0.30) (Figure 5A). Calf production, measured as the annual per capita productivity index (API), showed large variability over the study period with a mean of 0.11 (range: 0.00-0.23) (Figure 5B).
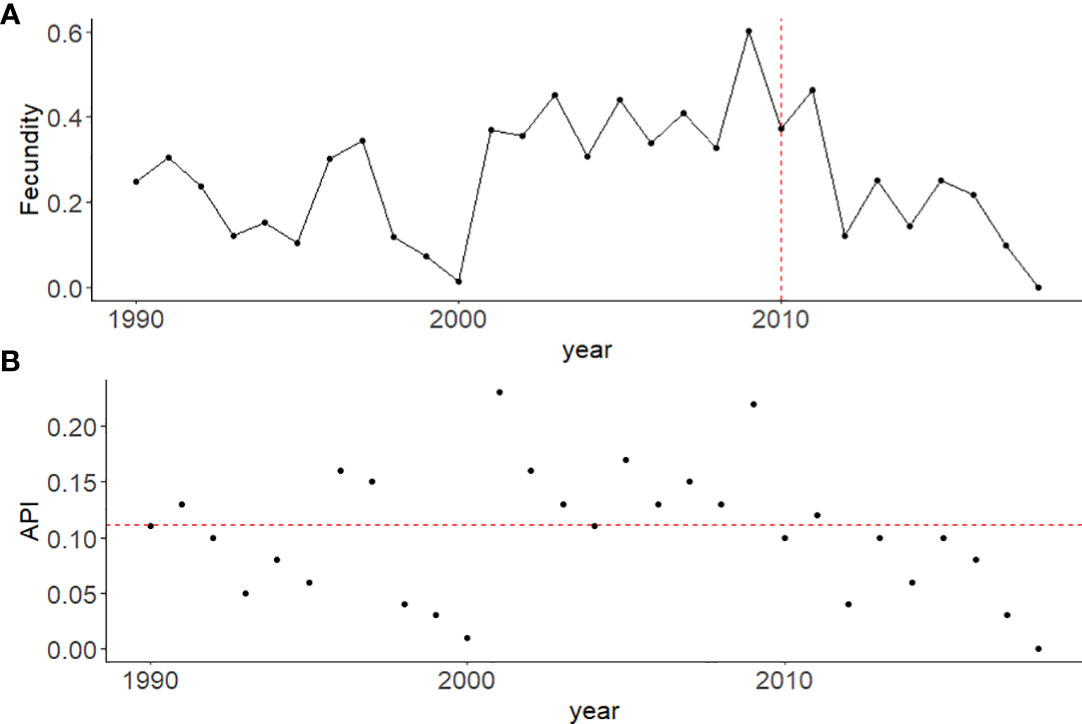
Figure 5 Reproductive measures for population growth for female North Atlantic right whales from 1990 – 2018. (A) The yearly fecundity rate, measured as the proportion of calves detected to the number of reproductively available females in the population per year. The vertical dotted line (red) indicates 2010, after which abundance of female breeders started to decline. (B) Annual productivity index (API) calculated as the total number of calves detected in a given year/average abundance estimate per year (Nt). The horizontal dotted line (red) indicates the average API of 0.11 for this population.
Individuals whose state could not be ascertained were more likely to be assigned as breeders with a probability of 0.53 (95% CI: 0.51-0.56) than to pre-breeder. Initial state probability, i.e., the probability of any individual being captured for the first time, and assigned to a particular state when first observed was highest for calves with average of 0.60 individuals assigned as calves (95% CI: 0.55-0.66), followed by breeders with an average of 0.35 (95% CI: 0.29-0.41), pre-breeders at 0.03 (95% CI: 0.01-0.08) and calves recovered dead at 0.01 (95% CI: 0.002-0.02).
Discussion
North Atlantic right whales live in one of the most industrialized habitats of any whale species (Kraus et al., 2007), and so there are a number of biological and anthropogenic factors influencing survival, including reproductive events, food availability, vessel strikes, and entanglement (Kraus, 1990; Knowlton and Kraus, 2001; van der Hoop et al., 2017; Corkeron et al., 2018; Christiansen et al., 2020). Understanding how demographic parameters have changed over time, and the influence of anthropogenic and intrinsic pressures on these changes, is important to understand how the parameters influence trends in population structure and size, which in turn can help inform management strategies. The recent reclassification of NARW to Critically Endangered on the IUCN Red List (Cooke, 2020) highlights the importance of a robust understanding of population demographics. With any free-ranging populations, obtaining reliable detections and sightings of enough individuals to collect this demographic information is difficult. Here, by combining multiple sources of information -re-sightings, and dead recoveries - we provide the most unbiased estimates yet available for survival, recapture probabilities, and abundance estimates for different states of NARWs (Barker, 1995; Barker, 1997).
Earlier studies have applied stage-structured models to NARW (Fujiwara and Caswell, 2002), however, these matrix population models assume the population has a stable stage structure (Hoy et al., 2020), which is no longer the case for NARW, making their continued application inappropriate. Recent work on several vertebrate species found that age structure dynamics are often non-stable, and can be an important mechanism influencing fluctuations in abundance (Hoy et al., 2020). Ignoring non-stable population structures can result in the failure of management efforts, due to substantial differences in the estimated growth rate when compared to models that assume a stable age or stage structure (Hoy et al., 2020). Changes in the population structure for female NARW highlights that many females are delaying reproduction, or failing to reproduce, indicating that assuming maturity based upon age, as used in earlier studies (Fujiwara and Caswell, 2002; Pace et al., 2017; Meyer-Gutbrod and Greene, 2018; Linden, 2020), no longer appropriately represents reproduction for this species. Therefore, projections on future population trajectories for NARWs based on these models (e.g (Meyer-Gutbrod and Greene, 2018; Linden, 2020) are likely to be incorrect, including those projections that underpin NOAA’s recent Biological Opinion (NOAA Fisheries, 2021a) on the impact of fisheries on NARW (Linden, 2020).
Our abundance estimates show subtle but important differences from previous work (Pace et al., 2017). We found that the abundance of female right whales increased over the 41 year study period from 81 in 1985, peaking at 185 individuals (95% CI: 183-187) in 2010 (Figure 2A), after which their abundance plateaued to 2013, then declined from 184 individuals in 2013 to 142 individuals at the beginning of 2018 (95% CI: 135-150). This coincides with a post-2010 change in the general distribution of NARW (Davis et al., 2017), and with an influx of NARW into the Gulf of St Lawrence post-2014 (Simard et al., 2019), and increased use of a foraging area in offshore, southern New England in recent years, particularly 2017-2018 (Quintana-Rizzo et al., 2021). Both of these sites, when NARW started using them, lacked spatially-explicit management programs to minimize NARW morbidity and mortality. While management measures aimed at mitigating human impacts have been instituted in Canadian waters in the Gulf of St Lawrence (Davies and Brillant, 2019), minimal actions have occurred in the US waters off southern New England (NOAA Fisheries, 2021b).
In 2018, pre-breeders composed an estimated 49% of the population and breeding females 51%. Moreover, females compose less than half the population of NARWs, with a male biased sex ratio (Pace et al., 2017; Harcourt et al., 2019). The reduction in the number of breeding females has two contributing factors, a decline in the number breeding females post-2010, and a decline in the transition rate for non-breeding individuals to becoming breeding individuals. Female survival has been considered the key driving factor contributing to the recent decline (Corkeron et al., 2018); these results show that lack of pre-breeders starting to calve and so recruiting into the breeding cohort is also driving the decline, a point that has been missing from consideration of NARW conservation efforts, with current abundance estimates and population growth projections assuming maturity based upon an individual’s age (Pace et al., 2017; Meyer-Gutbrod and Greene, 2018). By assuming maturity by age, individuals who delay first reproduction or never reproduce are grouped with breeding individuals which can bias projections by assuming a higher reproductive potential for the species. The sublethal stressors causing stunting (Stewart et al., 2021) and failure to start calving require as much attention as do anthropogenic causes of NARW mortality.
True survival estimates from our model remained relatively stable across years for both pre-breeders and breeders with a mean true survival estimate of 0.96 (range: 0.92-0.98) and 0.97 (range: 0.94-0.99) respectively between 1985 and 2018. Estimates for true survival of calves was more variable with an average of 0.79 (range: 0.50-0.92) between 1985 and 2018. These survival estimates for NARW are low compared to the congeneric southern right whale (Eubaleana australis), with adult survival estimates of 0.99 (95% CI: 0.98-0.99) in South Africa (Best et al., 2001), and another balaenid, the bowhead whale (Balaena mysticetus) with estimated survival at 0.98 (95% CI: 0.95-1.00) (Zeh et al., 2002). Estimates for the southern right whale and the bowhead whale represent apparent survival rather than true survival. Apparent survival is likely to be biased low due to unaccounted emigration, and is lower than true survival unless fidelity is equal to one (Schaub and Royle, 2014).
Recent work (Pace et al., 2017) estimated apparent survival, not true survival, for females 5+ years (considered adults in that model) at 0.968 (± 0.0073), and 0.955 (± 0.0127) for calves (which included both sexes, and were defined as up to 4 years of age). These estimates, being of different cohorts of whales from our model, are not directly comparable. Our survival estimates, likes those in (Pace et al., 2017) have substantial uncertainty around them, but our point estimates of true survival show greater fluctuation. Calf survival estimates were robust, given the level of survey effort of the calving and nursing grounds, missed calving events are unlikely (Kraus et al., 1986; Corkeron et al., 2018; Record et al., 2019). Estimates for calf survival show yearly variability with no notable declines consistent with the decline in abundance, suggesting consistent variability in calf survival for this species. Calf survival approximates to only four out of five calves surviving their first year of life, adding to the conservation dilemma posed by poor calving. First-year (and so, pre-breeder) mortalities were more likely to be missed, as individuals would not be added to the photo-identification catalogue, possibly biasing pre-breeder survival slightly high. No discernible difference in the true survival estimates of pre-breeder and breeder states over this time were seen in our estimates, suggesting that survival has less of an influence on the current decline of the NARW population when compared to recruitment of breeding females.
The decline in the abundance of breeding females is caused by a mix of mortality (of both breeders and pre-breeders that die before they can transition to breeding), and a failure to start breeding by pre-breeders. We show that individuals born from 2000 onward who were assigned as pre-breeders have a mean probability of 0.04 of transitioning to a breeder annually, half that of individuals born pre-2000, whose mean probability of transitioning was 0.08. Individual NARW born now are smaller than they were in past decades due, at least in part, to entanglements in fishing gear, with per capita rates of entanglement initially peaking in 2000 then declining until 2006, with rates steadily increasing since 2007 (Knowlton et al., 2012). Individuals who have been entangled in their life, or whose mother was entangled during nursing are, on average 0.64 m and 0.69 m shorter respectively than those who have not been affected by entanglement (Stewart et al., 2021). This helps explain the failure of females to gain sufficient condition to start calving, as smaller female right whales have less capacity to gain sufficient condition to calve than larger females (Christiansen et al., 2020). So, while the death of breeding females is driving the decline in the female population more than the death of pre-breeders, it is the lack of females joining the breeding cohort, with a plateau in pre-breeders abundance since 2010, that is a contributing factor to the population’s decline in recent years.
The change in the rate of recruitment into the breeding population has important implications for our understanding of anthropogenic impacts on NARW. The number of calves born per reproductively available female was seen to decrease post 2011, suggesting a reduction in fecundity in recent years (Figure 5A). Since 2012, the calving rate has also been depressed (Figure 5B), demonstrating that the failure to breed is not restricted solely to the lack of pre-breeders approaching maturity (Figure 2B). Reproduction is energetically expensive, with the failure to breed or the undernourishment of offspring being linked to poor body condition of mothers (Gaillard and Yoccoz, 2003). Female NARW that calve are in poorer body condition than congeneric southern right whales (Christiansen et al., 2020). The body condition of juvenile NARW is also poorer than their southern counterpart, which can lead to reduced energy for growth and delayed sexual maturity (Christiansen et al., 2020). Stunting of NARWs caused by sub-lethal effects is likely the biological driver for this shift in recruitment of breeding females shown by our model, with smaller individuals having less energy stores to invest in reproduction (Stewart et al., 2021).
Mortality and reproductive failure caused by entanglement can be eliminated by appropriate fisheries management, but failure caused by poor foraging quality due to climate change (Meyer-Gutbrod et al., 2021) is more intractable. The stunted growth of the individuals alive now can never be reversed. With the current finding of this model, combined with the new insights into the sub-lethal effects of entanglement, the importance of mitigating these effects is critical for the recruitment of breeding females, and the growth of the population. Half of the non-calf females have yet to calve, and with three males for every two females (Pace et al., 2017), reproductive females currently comprise only one-fifth of the species. This proportion of calving females will only decrease unless the impacts of both lethal and sub-lethal entanglements can be stopped. Failure to reverse this trend will leave a species whose numbers may remain at best a few hundred, but which will be functionally extinct within our lifetime.
Data availability statement
The data analyzed in this study is subject to the following licenses/restrictions: Data used in this study was provided and managed by the North Atlantic Right Whale Consortium. Requests to access these datasets should be directed to http://www.narwc.org.
Author contributions
JR wrote the manuscript and conducted the modeling. LN and PC aided with model design. PC and RH provided guidance on species biology. LN, PC, and RH reviewed and revised the manuscript. All authors designed the study. All authors read and approved the final manuscript.
Funding
JR is supported by a Macquarie University Research Excellence Scholarship.
Acknowledgments
We would like to thank the North Atlantic Right Whale Consortium for the use of their sightings and identification databases. We thank Nigel Yoccoz for his review of a draft of this manuscript.
Conflict of interest
The authors declare that the research was conducted in the absence of any commercial or financial relationships that could be construed as a potential conflict of interest.
Publisher’s note
All claims expressed in this article are solely those of the authors and do not necessarily represent those of their affiliated organizations, or those of the publisher, the editors and the reviewers. Any product that may be evaluated in this article, or claim that may be made by its manufacturer, is not guaranteed or endorsed by the publisher.
Supplementary Material
The Supplementary Material for this article can be found online at: https://www.frontiersin.org/articles/10.3389/fmars.2022.994481/full#supplementary-material
References
Baker J., Swanson D.A., Tayman J., Tedrow L.M.. (2017). “Stable population theory,” in Cohort change ratios and their applications (Cham, Switzerland: Springer), 173–189.
Barker R. J. (1995). Open population mark-recapture models including ancillary sightings: a thesis presented in partial fulfilment of the requirements for the degree of doctor of philosophy in statistics at Massey university (Doctoral dissertation: Massey University).
Barker R. J. (1997). Joint modeling of live-recapture, tag-resight, and tag-recovery data. Biometrics 53 (2), 666–677. doi: 10.2307/2533966
Best P. B., Brsndão A., Butterworth D. S. (2001). Demographic parameters of southern right whales off south Africa. J. Cetacean Res. Manage. 2 (2), 161–169. doi: 10.47536/jcrm.vi.296
Brooks S. (1998). Markov Chain Monte Carlo method and its application. J. R. Stat. society: Ser. D (the Statistician) 47 (1), 69–100. doi: 10.1111/1467-9884.00117
Brooks S. P., Catchpole E.A., Morgan B.J.T., Harris M.P.. (2002). Bayesian Methods for analysing ringing data. J. Appl. Stat 29 (1-4), 187–206. doi: 10.1080/02664760120108683
Brooks S. P., Gelman A. (1998). General methods for monitoring convergence of iterative simulations. J. Comput. graphical Stat 7 (4), 434–455. doi: 10.1080/10618600.1998.10474787
Browning C. L., Rolland R. M., Kraus S. D. (2010). Estimated calf and perinatal mortality in western north Atlantic right whales (Eubalaena glacialis). Mar. Mammal Sci. 26 (3), 648–662. doi: 10.1111/j.1748-7692.2009.00361.x
Burnham K. P. (1993). A theory for combined analysis of ring recovery and recapture data. marked individuals in the study of bird population. (Switzerland, Birkhäuser-Verlag, Basel). 199–213.
Caswell H., Fujiwara M., Brault S. (1999). Declining survival probability threatens the north Atlantic right whale. Proc. Natl. Acad. Sci. 96 (6), 3308–3313. doi: 10.1073/pnas.96.6.3308
Christiansen F., Vivier F., Charlton C., Ward R., Amerson A., Burnell S., et al. (2018). Maternal body size and condition determine calf growth rates in southern right whales. Mar. Ecol. Prog. Ser. 592, 267–281. doi: 10.3354/meps12522
Christiansen F., et al. (2020). Population comparison of right whale body condition reveals poor state of the north Atlantic right whale. Mar. Ecol. Prog. Ser. 640, 1–16. doi: 10.3354/meps13299
Conn P. B., Cooch E. G. (2009). Multistate capture–recapture analysis under imperfect state observation: an application to disease models. J. Appl. Ecol. 46 (2), 486–492. doi: 10.1111/j.1365-2664.2008.01597.x
Cooke J. G. (2020). “Eubalaena glacialis,” in The IUCN red list of threatened species 2020. Downloaded on 10 July 2020. doi: 10.2305/IUCN.UK.2020-2.RLTS.T41712A178589687.en
Corkeron P., Hamilton P., Bannister J., Best P., Charlton C., Groch K.R., et al. (2018). The recovery of north Atlantic right whales, eubalaena glacialis, has been constrained by human-caused mortality. R. Soc. Open Sci. 5 (11), 180892. doi: 10.1098/rsos.180892
Davies K. T., Brillant S. W. (2019). Mass human-caused mortality spurs federal action to protect endangered north Atlantic right whales in Canada. Mar. Policy 104, 157–162. doi: 10.1016/j.marpol.2019.02.019
Davis G. E., Baumgartner M.F., Bonnell J.M., Bell J., Berchok C., Bort Thornton J., et al. (2017). Long-term passive acoustic recordings track the changing distribution of north Atlantic right whales (Eubalaena glacialis) from 2004 to 2014. Sci. Rep. 7 (1), 13460. doi: 10.1038/s41598-017-13359-3
Eberhardt L. (1977). "optimal" management policies for marine mammals. Wildlife Soc. Bull. 5 (4), 162–169. http://www.jstor.org/stable/3781901
Fujiwara M., Caswell H. (2001). Demography of the endangered north Atlantic right whale. Nature 414 (6863), 537–541. doi: 10.1038/35107054
Fujiwara M., Caswell H. (2002). Estimating population projection matrices from multi-stage mark-recapture data. Ecology 83 (12), 3257–3265. doi: 10.1890/0012-9658(2002)083[3257:Eppmfm]2.0.Co;2
Gaillard J.-M., Festa-Bianchet M., Yoccoz N., Loison A., Toigo C.. (2000). Temporal variation in fitness components and population dynamics of large herbivores. Annu. Rev. Ecol. Systemat 31 (1), 367–393. doi: 10.1146/annurev.ecolsys.31.1.367
Gaillard J.-M., Festa-Bianchet M., Yoccoz N. G. (1998). Population dynamics of large herbivores: variable recruitment with constant adult survival. Trends Ecol. Evol. 13 (2), 58–63. doi: 10.1016/S0169-5347(97)01237-8
Gaillard J.-M., Yoccoz N. G. (2003). Temporal variation in survival of mammals: a case of environmental canalization? Ecology 84 (12), 3294–3306. doi: 10.1890/02-0409
George E. I. (1992). Capture–recapture estimation via Gibbs sampling. Biometrika 79 (4), 677–683. doi: 10.1093/biomet/79.4.677
Gilroy J. J., Virzi T., Boulton R.L., Lockwood J.L.. (2012). A new approach to the “apparent survival” problem: estimating true survival rates from mark–recapture studies. Ecology 93 (7), 1509–1516. doi: 10.1890/12-0124.1
Gimenez O., Lebreton J.-D., Gaillard J.-M., Choquet R., Pradel R.. (2012). Estimating demographic parameters using hidden process dynamic models. Theor. population Biol. 82 (4), 307–316. doi: 10.1016/j.tpb.2012.02.001
Hamilton P. K., Knowlton A.R., Marx M.K., Kraus S.D.. (1998). Age structure and longevity in north Atlantic right whales eubalaena glacialis and their relation to reproduction. Mar. Ecol. Prog. Ser. 171, 285–292. doi: 10.3354/meps171285
Hamilton P., Knowlton A., Marx M. (2007). “Right whales tell their own stories: the photo-identification catalog,” in The urban whale: North Atlantic right whales at the crossroads (Cambridge, MA: Harvard University Press), 75–104.
Harcourt R., Van Der Hoop J., Kraus S., Carroll E.L.. (2019). Future directions in eubalaena spp.: comparative research to inform conservation. Front. Mar. Sci. 5, 530. doi: 10.3389/fmars.2018.00530
Hayes S. A., Josephson E., Maze-Foley K., Rosel P.E.. (2020). “US Atlantic And gulf of Mexico marine mammal stock assessments - 2019,” in NOAA Technical memorandum NMFS-NE-264. Washington, DC: NOAA
Horvitz C., Schemske D. W., Caswell H. (1997). “The relative “importance” of life-history stages to population growth: prospective and retrospective analyses,” in Structured-population models in marine, terrestrial, and freshwater systems (Boston, MA: Springer), 247–271.
Hoy S. R., MacNulty D.R., Smith D.W., Stahler D.R., Lambin X., Peterson R.O., et al. (2020). Fluctuations in age structure and their variable influence on population growth. Funct. Ecol. 34 (1), 203–216. doi: 10.1111/1365-2435.13431
Jenouvrier S., Barbraud C., Cazelles B., Weimerskirch H. (2005). Modelling population dynamics of seabirds: Importance of the effects of climate fluctuations on breeding proportions. Oikos 108 (3), 511–522. doi: 10.1111/j.0030-1299.2005.13351.x
Jenouvrier S., Péron C., Weimerskirch H. (2015). Extreme climate events and individual heterogeneity shape life-history traits and population dynamics. Ecol. Monogr. 85 (4), 605–624. doi: 10.1890/14-1834.1
Kéry M., Schaub M. (2011). Bayesian Population analysis using WinBUGS: a hierarchical perspective (New York, NY: Academic Press).
King R. (2012). A review of Bayesian state-space modelling of capture-recapture-recovery data. Interface Focus 2 (2), 190–204. doi: 10.1098/rsfs.2011.0078
Knowlton A. R., Hamilton P.K., Marx M.K., Pettis H.M., Kraus S.D.. (2012). Monitoring north Atlantic right whale eubalaena glacialis entanglement rates: a 30 yr retrospective. Mar. Ecol. Prog. Ser. 466, 293–302. doi: 10.3354/meps09923
Knowlton A. R., Kraus S. D. (2001). Mortality and serious injury of northern right whales (Eubalaena glacialis) in the western north Atlantic ocean. J. Cetacean Res. Manage. 2, 193–208. doi: 10.47536/jcrm.vi.288
Korner-Nievergelt F., Roth T., Von Felten S., Guélat J., Almasi B., Korner-Nievergelt P.. (2015). Bayesian Data analysis in ecology using linear models with r, BUGS, and Stan (New York, NY: Academic Press).
Kraus S. D. (1990). Rates and potential causes of mortality in north Atlantic right whales (Eubalaena glacialis). Mar. Mammal Sci. 6 (4), 278–291. doi: 10.1111/j.1748-7692.1990.tb00358.x
Kraus S. D., Moore K.E., Price C.A., Crone M.J., Watkins W.A., Winn H.E., et al. (1986). The use of photographs to identify individual north Atlantic right whales (Eubalaena glacialis). Rep. Int. Whaling Commission 10, 145–151.
Kraus S. D., Rolland R., Rolland S. S. R. M. (2007). The urban whale: North Atlantic right whales at the crossroads (Cambridge, MA: Harvard University Press).
Lebreton J.-D., Almeras T., Pradel R. (1999). Competing events, mixtures of information and multistratum recapture models. Bird Study 46 (sup1), S39–S46. doi: 10.1080/00063659909477230
Linden D. (2020). Population projections of north Atlantic right whales under varying human-caused mortality risk and future uncertainty In: National Marine Fisheries Service Endangered Species Act Section 7 Biological Opinion Consultation (NOAA/NMFS/GARFO), 41, 492–537. doi: 10.25923/h9hz-3c72
Meyer-Gutbrod E. L., Greene C.H., Davies K.T.A., Johns D.G.. (2021). Ocean regime shift is driving collapse of the north Atlantic right whale population. Oceanography 34 (3), 22–31. doi: 10.5670/oceanog.2021.308
Meyer-Gutbrod E. L., Greene C. H. (2018). Uncertain recovery of the north Atlantic right whale in a changing ocean. Global Change Biol. 24 (1), 455–464. doi: 10.1111/gcb.13929
Moore M. J., Rowles T.K., Fauquier D.A., Baker J.D., Biedron I., Durban J.W., et al. (2021). REVIEW assessing north Atlantic right whale health: threats, and development of tools critical for conservation of the species. Dis. Aquat. Organisms 143, 205–226. doi: 10.3354/dao03578
Nichols J. D., Kendall W.L., Hines J.E., Spendelow J.A.. (2004). Estimation of sex-specific survival from capture–recapture data when sex is not always known. Ecology 85 (12), 3192–3201. doi: 10.1890/03-0578
NOAA Fisheries (2021a). Endangerd species act secttion 7 consultation - biological opinion. Greater Altantic Regional Fisher Office. doi: 10.25923/cfsq-qn06. Available at: https://repository.library.noaa.gov/view/noaa/30648
NOAA Fisheries (2021b) New and existing restricted areas: 2021 Atlantic Large whale take reduction plan modifications. Available at: https://www.fisheries.noaa.gov/resource/map/new-and-existing-restricted-areas-2021-atlantic-large-whale-take-reduction-plan.
Notarbartolo di Sciara G., Politi E., Bayed A., Beaubrun P.-C., Knowlton A.. (1998). A winter cetacean survey off southern Morocco, with a special emphasis on right whales. Rep. Int. Whaling Commission 48, 547–550.
Pace R.M. III, Corkeron P. J., Kraus S. D. (2017). State–space mark–recapture estimates reveal a recent decline in abundance of north Atlantic right whales. Ecol. Evol. 7 (21), 8730–8741. doi: 10.1002/ece3.3406
Pettis H. M., Rolland R.M., Hamilton P.K., Brault S., Knowlton A.R., Kraus S.D.. (2004). Visual health assessment of north Atlantic right whales (Eubalaena glacialis) using photographs. Can. J. Zool 82 (1), 8–19. doi: 10.1139/z03-207
Hornik K., Leisch F., Zeileis A., Plummer M. (2003). “JAGS: A program for analysis of Bayesian graphical models using Gibbs sampling,” in Proceedings of the 3rd international workshop on distributed statistical computing, Vienna, Austria 2 (1).
Quintana-Rizzo E., Leiter S., Cole T., Hagbloom M., Knowlton A., Nagelkirk P., et al. (2021). Residency, demographics, and movement patterns of north Atlantic right whales eubalaena glacialis in an offshore wind energy development in southern new England, USA. Endangered Species Res. 45, 251–268. doi: 10.3354/esr01137
R Core Team (2017). R: A language and environment for statistical computing (Vienna, Austria: R Foundation for Statistical Computing).
Record N. R., Runge J.A., Pendleton D.E., Balch W.M., Davies K.T.A., Pershing A.J., et al. (2019). Rapid climate-driven circulation changes threaten conservation of endangered north Atlantic right whales. Oceanography 32 (2), 162–169. doi: 10.5670/oceanog.2019.201
Right Whale Consortium (2020) North Atlantic Right Whale Consortium in North Atlantic Right Whale Consortium Identification and Sightings Database, New England Aquarium, Boston, MA, U.S.A, 01/20/2020.
Royle J. A., Dorazio R. M. (2008). Hierarchical modeling and inference in ecology: the analysis of data from populations, metapopulations and communities (London, UK: Elsevier).
Sanz-Aguilar A., Tavecchia G., Genovart M., Igual J.M., Oro D., Rouan L., et al. (2011). Studying the reproductive skipping behavior in long-lived birds by adding nest inspection to individual-based data. Ecol. Appl. 21 (2), 555–564. doi: 10.1890/09-2339.1
Schaub M., Royle J. A. (2014). Estimating true instead of apparent survival using spatial cormack–Jolly–Seber models. Methods Ecol. Evol. 5 (12), 1316–1326. doi: 10.1111/2041-210X.12134
Schwarz C. J., Arnason A. N. (1996). A general methodology for the analysis of capture-recapture experiments in open populations. Biometrics 52 (3), 860–873. doi: 10.2307/2533048
Simard Y., Roy N., Giard S., Aulanier F. (2019). North Atlantic right whale shift to the gulf of st. Lawrence in 2015, revealed by long-term passive acoustics. Endangered Species Res. 40, 271–284. doi: 10.3354/esr01005
Stewart J. D., Durban J.W., Knowlton A.R., Lynn M.S., Fearnbach H., Barbaro J., et al. (2021). Decreasing body lengths in north Atlantic right whales. Curr. Biol 31 (14), pp. 3174–3179. doi: 10.1016/j.cub.2021.04.067
van der Hoop J., Corkeron P., Moore M. (2017). Entanglement is a costly life-history stage in large whales. Ecol. Evol. 7 (1), 92–106. doi: 10.1002/ece3.2615
Keywords: Bayesian, mark-recapture-recovery, recruitment, endangered species, Eubalaena glacialis
Citation: Reed J, New L, Corkeron P and Harcourt R (2022) Multi-event modeling of true reproductive states of individual female right whales provides new insights into their decline. Front. Mar. Sci. 9:994481. doi: 10.3389/fmars.2022.994481
Received: 14 July 2022; Accepted: 20 September 2022;
Published: 06 October 2022.
Edited by:
Phil J. Bouchet, University of St Andrews, United KingdomReviewed by:
Nadine Stewart Lysiak, University of Massachusetts Boston, United StatesDoug Sigourney, Integrated Statistics, United States
Paul Kinas, Federal University of Rio Grande, Brazil
Copyright © 2022 Reed, New, Corkeron and Harcourt. This is an open-access article distributed under the terms of the Creative Commons Attribution License (CC BY). The use, distribution or reproduction in other forums is permitted, provided the original author(s) and the copyright owner(s) are credited and that the original publication in this journal is cited, in accordance with accepted academic practice. No use, distribution or reproduction is permitted which does not comply with these terms.
*Correspondence: Joshua Reed, am9zaHVhLnJlZWRAc3R1ZGVudHMubXEuZWR1LmF1