- 1Key Laboratory of Marine Ecosystem Dynamics, Ministry of Natural Resources & Second Institute of Oceanography, Ministry of Natural Resources, Hangzhou, China
- 2Ocean College, Zhejiang University, Zhoushan, China
- 3College of Life Sciences and Medicine, Zhejiang Sci-Tech University, Hangzhou, China
- 4Zhejiang Sci-Tech University Shaoxing Academy of Biomedicine Co., Ltd., Shaoxing, China
Mudflats and sandflats are two common types of coastal tidal flats, the structure and function differences of microbial communities between them are still underappreciated. Beibu Gulf is a diurnal tidal regime located in China, the differences between the two type of tidal flats could be more distinct. In this study, we collected a total of 6 samples from Beibu Gulf, consisting of 3 sandflats samples and 3 mudflats samples, classified based on clay and silt content. Generally, the mudflats samples exhibited higher levels of NH4+_N and TOC, but lower in ORP and pH. The microbial diversity of the two types of tidal flats was investigated, revealing great differences existed and sandflats had higher microbial richness and diversity than mudflats. Furthermore, we analyzed the association between microbial communities and environmental factors, finding NH4+_N to have the highest contribution to the total variation in microbial community structure, and microbial groups such as Desulfobacterota, Campilobacterota, Chloroflexota, Calditrichota, Spirochaetota, Zixibacteria, Latescibacterota and Sva0485 group in mudflats were positively associated with NH4+_N. The functions of microbial community were predicted using metagenomic sequences and metagenome assembled genome (MAG). Mudflats contained more genes for carbon fixation. Nitrate and nitrite reduction were widely existed in mudflats and sandflats, but nitrogen fixation was only existed in mudflats, and Campilobacterota, Desulfobacterota and Gammaproteobacteria MAGs were mainly responsible for it. Sandflats composed more genes for ammonium oxidation, but no MAG was found whether in sandflats or mudflats. Microbes in mudflats exhibited a greater abundance of genes related to sulfur cycling, especially in reduction process, unique MAGs in mudflats such as Calditrichota, Chloroflexota, Desulfobacterota and Zixibacteria MAGs are responsible for sulfate and sulfite reduction. Finally, we predicted functions of ammonium related microbes in mudflats based on MAGs and found Campilobacterota and Desulfobacterota MAGs were important for high accumulation of ammonium in mudflats. This study illuminated the structural and functional differences of microbial communities in mudflats and sandflats, providing new insights into the relationship of microbial communities and environment in the tidal flat.
1 Introduction
Tidal flats are important transitional areas that connect the ocean and land, characterized by strong dynamic interactions between tide and river runoff, and between surface water and groundwater (Osland et al., 2016). Tidal flats usually defined as ecosystems including sandflats and mudflats that undergo regular tidal inundation (Healy et al., 2002). These ecosystems play vital roles with biodiversity, ecological services (Murray et al., 2019). However, tidal flats are affected by human and natural activities such as coastal development (Sarukhán et al., 2005), sea-level rise (Passeri et al., 2015), and coastal erosion (Nicholls et al., 2007), which is interfering the ecological structure and function, with significant implications for their ecological services (Zhang et al., 2020).
Microorganisms play a crucial role in the tidal flat ecosystem influencing key ecological processes (Böer et al., 2009), such as energy flow, pollutant degradation, element transformation and migration (Wang et al., 2020a). The diversity and composition of microbial communities in sediment are primarily influenced by various environmental factors, including salinity (Zhang et al., 2021), pH (Zhang et al., 2017), total organic carbon (Du et al., 2011), temperature (Lv et al., 2016), nitrogen (Ki et al., 2018), and the content of silt and clay (Boey et al., 2021). This selective effect of the environment on microorganisms is a central concept in niche theory, which suggests that microbial dispersal is unlimited and community assembly is primarily driven by deterministic factors, such as environmental selection (Webb et al., 2002). Bass-Becking hypothesized that “everything is everywhere, but the environment selects,” due to the small size, high rates of population growth, and the vast abundance of microbes. Environmental and nutrient factors were fundamental for microbial growth and development and thus could clearly shape the pattern of microbial communities (Li et al., 2020). Understanding the structure of bacterial communities is crucial for studying tidal flat functions and their response to environmental changes.
Mudflats and sandflats, two common types of tidal flats, exhibit distinct differences in their physical, chemical, and biological characteristics (Thrush et al., 2004; Boey et al., 2021). Research has demonstrated that due to the greater cohesiveness and less permeability of terrigenous sediments (silt and clay, <63 μm on the Wentworth scale), the increase of silt and clay content significantly influences the environmental conditions and biogeochemical processes (Lohrer et al., 2004; Cummings et al., 2009). Compared with sandflat sediments, mudflat sediments are dominated by diffusive transport (Huettel et al., 2014), and may restrict nutrient processing (release, uptake or transformation of organic nutrients by the benthos) facilitating ecosystem shifts toward eutrophication (Douglas et al., 2018), which indicated that mudflat sediments have a higher level of anoxia (Boey et al., 2021). Furthermore, sandier, more permeable sediments are dominated by advective transport (Huettel et al., 2014), are more oxygenated, and metabolites are rapidly exchanged for new substrates, which facilitates higher rates of bacterial carbon uptake compared to mudflat sediments (Woulds et al., 2016). Microbial communities associated with sandy sediments are thus thought t have the ability to quickly adapt to changing biogeochemical conditions (Boey et al., 2021).
The Beibu Gulf, situated in northwest of the South China Sea, is a semi-enclosed tropical and subtropical gulf partially enclosed by the Leizhou Peninsula, Qiongzhou Strait, Hainan Island, Vietnam, and stretches to the Guangxi coast in the north (Ma et al., 2010). One notable characteristic of the Beibu Gulf coast is the presence of numerous estuaries, including the Red River in Vietnam and the Beilun, Maoling, and Dafeng Rivers in China, these rivers serve as significant potential sources of nutrients and pollution that enter the Gulf (Dou et al., 2013). Additionally, the Beibu Gulf experiences a diurnal tidal regime, which is uncommon in other regions of China. Compared with the common semi-diurnal tide, the diurnal tide has a longer continuous submerged time on a single tidal day, and the diurnal and semi-diurnal tidal zones are different in hydrology, climate and soil characteristics (He et al., 2007).
In this study, field investigations were carried out with two types of tidal flat along the coastline of the Beibu Gulf. We aimed to solve the following scientific issues: (1) to determine the differences in microbial structure and diversity between mudflats and sandflats in the Beibu Gulf; (2) to identify the key environmental factors that influence the distribution of microbes in both mudflats and sandflats; (3) to assess the interaction mechanisms between environmental factors and microbial communities.
2 Materials and methods
2.1 Samples collection and strain isolation
The study area encompassed the northern and eastern regions of the Beibu Gulf, spanning across Guangxi and Guangdong Provinces, China (Figure 1). Random collection of sediment samples was carried out from 6 stations along the tidal flats of the Beibu Gulf. The sampling positions were determined using a global positioning system by Ocean data view (Supplementary Table 1) (Schlitzer, 2021). Based on the observations at the sampling sites, the collected samples were classified into two types: mudflats-like sediment samples (M1, M2, M3) and sandflats-like sediment samples (S1, S2, S3). For each sampling site, three replicates of 0-5 cm soils from the sub-top layer were collected and thoroughly mixed to create a composite sample. The collected samples were transported to the laboratory in an ice box, with some stored at 4°C for strain isolation and culture experiments, while the remaining portion was stored in a -20°C freezer for subsequent sequencing and physical and chemical analysis.
2.2 Physicochemical analysis
Pore water in the sediment samples was extracted using Rhizon CSS pore water technique, followed by in situ analysis of salinity (SAL), pH, oxidation-reduction potential (ORP), dissolved oxygen (DO), and temperature (T) using a water quality analyzer (HQ40D, Hach) in combination with salinity (CDC401, Hach), pH (pHC108, Hach), oxidation-reduction potential (MTC101, Hach), and dissolved oxygen (LDO101, Hach) probes. The content of silt and clay (SC) was characterized using a laser particle size analyzer (MS2000, Malvern), in brief, sediments with diameters < 63 μm were defined as mud (a combination of clay and silt according to the Wentworth scale), and those with a mud content ≥10% were categorized as mudflats, while those with ≤10% were classified as sandflats (Folk et al., 1970). The total organic carbon (TOC) was determined using the potassium dichromate volumetric method, total nitrogen (TN) in the sediment was determined using the Kjeldahl method (Kjeldahl, 1883), and total phosphorus (TP) was analyzed using the acidic molybdate–ascorbic acid spectrophotometric method (Ames, 1966). The concentrations of ammonium nitrogen (NH4+_N) and nitrate nitrogen (NO3-_N) in the sediment were determined using the indophenol blue colorimetric method (Peterson, 1979) and the hydrazine sulfate method (Sims, 1961), respectively. Triplicate samples (n = 3) were analyzed, and the data were presented as mean values ± standard deviations (SD) on a dry weight basis. Statistical significance of the sediment properties was assessed using a two-sided analysis of Welch’s t-test (Welch, 1947), with a p-value less than 0.05 considered as significant.
2.3 DNA extraction and sequencing
DNA extraction from the sediment samples (0.5 g each) was performed using the Magnetic Soil and Stool DNA Kit (TIANGEN, China) following the manufacturer’s instructions. The extracted environmental DNA served as the template for polymerase chain reaction (PCR), with amplification of bacterial 16S rRNA genes targeting the V4 hypervariable region using Earth Microbiome Project (EMP) standard primers 515F (5′‐GTGCCAGCMGCCGCGGTAA‐3′) and 806R (5′‐GGACTACHVGGGTWTCTAAT‐3′) (Thompson et al., 2017). The amplified products were visualized by electrophoresis on a 2% (w/v) agarose gel. PCR products with clear bands were pooled in equal densities and purified using the GeneJET Gel Recovery Kit (Thermo Scientific, China). High-throughput sequencing was performed using the Illumina NovaSeq 6000 sequencing platform (Novogene, China) with paired-end reads. Each sediment sample was sequenced in triplicate.
The extracted environmental DNA was also used for high-throughput sequencing on the Illumina HiSeq platform, and paired-end reads were generated using Magigene (Shenzhen, P.R. China). Sequencing libraries were prepared using the NEBNext® UltraTM DNA Library Prep Kit for Illumina (NEB, Ipswich, U.S.A.) following the manufacturer’s recommendations, with index codes assigned to each sample for sequence attribution. The raw reads have been deposited into the NCBI Sequence Read Archive (SRA) database under the accession numbers PRJNA774167 and PRJNA967168.
2.4 16S rRNA gene amplicon sequences and statistical analysis
The raw sequences obtained from Illumina sequencing were processed using QIIME2 version 2021.4 software (Liu et al., 2021). The QIIME2 cutadapt module was utilized to remove adapter and barcode sequences from the raw 16S rRNA gene sequences. Subsequently, the assembled sequences were filtered and de-replicated to obtain representative sequences known as Amplicon Sequence Variants (ASVs) and a feature table (ASVs table) by using DADA2 module in QIIME2. Taxonomy annotation was performed based on the SILVA 132 database (Quast et al., 2012), and the resulting representative sequences and feature tables with taxonomy annotation information were imported into QIIME2 for downstream analysis. To minimize the impact of sequencing depth on treatment effects, the sediment samples were randomly resampled to the same sequence depth based on the sample with the fewest number of sequences. Alpha-diversity indices, including observed_features, Faith’s phylogenetic diversity (faith_pd), Shannon index, and Chao1 value, were calculated for each sample using QIIME2. Principal coordinate analysis (PCoA) based on weighted UniFrac distance (Lozupone and Knight, 2005) and Bray-Curtis similarity (Bray and Curtis, 1957) were conducted to explore differences in bacterial community structures among the sampling sites. Differences in species abundances between the two groups were compared using Welch’s t-test with the Statistical Analysis of Metagenomic Profiles (STAMP version 2.1.3) software (Parks et al., 2014). Spearman’s rank correlation coefficient was employed to analyze the correlation between microbial communities and different environmental factors (Spearman, 1987). Redundancy analysis (RDA) was used to identify key factors influencing bacterial community structure (McArdle and Anderson, 2001), and envfit analysis in the ‘vagan’ package was performed to evaluate the explanatory ability of environmental factors on the community.
2.5 Metagenomic analysis
The total of 1,283,967,682 metagenomic raw reads (430,455,448 raw reads in sandflat samples and 853,512,234 raw reads in mudflat samples, Supplementary Table 5) were filtered and trimmed using Trimmomatic version 0.39 (Bolger et al., 2014) to generate clean reads. De novo assembly of metagenomes was performed for each sample (Supplementary Table 6) using the MEGAHIT module implemented in MetaWRAP pipeline software version 1.2.1 (Uritskiy et al., 2018). QUAST was used to evaluate the quality of each assembly (Gurevich et al., 2013). High- and medium-quality metagenome-assembled genomes (MAGs) were binned using modules implemented in MetaWRAP pipeline software version 1.2.1 (Uritskiy et al., 2018), with completeness and contamination thresholds set at >50% and <10%, respectively (Bowers et al., 2017). The abundance of MAGs in different samples was calculated using the quant_bins module in MetaWRAP pipeline software version 1.2.1 (Uritskiy et al., 2018). Duplicate MAGs with an average nucleotide identity (ANI) ≥95% were removed using the dereplicate module in dRep version 3.4.2 (Olm et al., 2017). Prior to detailed metabolic analysis, the MAGs were assigned initial taxonomy using the GTDB-Tk classify pipeline and a phylogenomic tree was constructed using the GTDB-Tk infer pipeline (Chaumeil et al., 2019). The final trees were visualized using Interactive Tree of Life (iTOL) v.5 (Letunic and Bork, 2021). Open reading frames (ORFs) were predicted using Prodigal v.2.6.3 (Hyatt et al., 2010) with the parameter “-p meta”, and then annotated using KOfam (Aramaki et al., 2020) and custom HMM profiles within METABOLIC v.4.0 (Zhou et al., 2022) with default settings. Functional genes first characterized by METABOLIC were additionally testified using the MEROPS database release 12.3 (Rawlings et al., 2018), CAZy database (Zhang et al., 2018; Drula et al., 2022), PSORTb v.3.0 (Yu et al., 2010), FeGenie (Garber et al., 2020), and HydDB (Søndergaard et al., 2016). All statistical analyses were performed using R software (version 4.0.4), SPSS Statistics (version 26.0), online cloud computing platform (https://www.bioincloud.tech/), and online drawing platform (https://www.chiplot.online/tvbot.html).
3 Results
3.1 Physicochemical properties of sediments
The main physical and chemical properties of sediment at different sampling points are presented in Supplementary Table 2. The silt and clay contents (<63 μm) in the mudflat-like sediment samples (M1-M3) ranged from 33.791% to 98.637%, while in the sandflat-like sediment samples (S1-S3), they ranged from 0% to 7.798%. According to Folk’s classification of sediments, the silt and clay contents indicate that samples M1-M3 correspond to mudflat sediments, while samples S1-S3 correspond to sandflat sediments (Supplementary Figure 1). Compared the environmental factors of sediments, NH4+_N was great significantly higher (p< 0.01) and TOC was significantly higher (p< 0.05) in mudflat than sandflat, on the other hand, ORP was great significantly higher (p< 0.01) and pH was significantly higher (p< 0.05) in sandflat than mudflat. Meanwhile, some factors were not different significantly including the content of TN in mudflats were higher and NO3-_N, TP, DO and SAL were lower compared to sandflat (Supplementary Table 3). Correlation analysis revealed that the content of silt and clay (SC) was positively correlated with TOC, NH4+_N, and TN (r> 0.5, p< 0.05), but negatively correlated with SAL, pH, ORP and DO (r< -0.5, p< 0.05) (Supplementary Table 4).
3.2 Microbial diversity and community structure
A total of 18 amplicon sequencing data (6 sediment samples, with 3 replicates for each sample) were retained after trimming and checking for chimera. In total, there were 959,821 sequences, with a mean sequence number of 53,323 in each sample. For each sample, four alpha diversity indices (shannon, observed_features, Faith’s phylogenetic diversity (faith_pd), and chao1) were analyzed. Except for the shannon index which show relative higher values in sandflat, other three indexes in sandflat were significantly higher than those in mudflat (Wilcox-test, p<0.05), indicated that sandflat had higher microbial richness and diversity than mudflat in the Beibu Gulf (Figure 2A). To assess the microbial community distributions, a PCoA based on weighted_unifrac distance analysis was performed. The results showed that all sediment samples were clearly separated into two groups. This suggested that the type of tidal flats in the Beibu Gulf region had an important influence on the microbial community composition (Figure 2B).
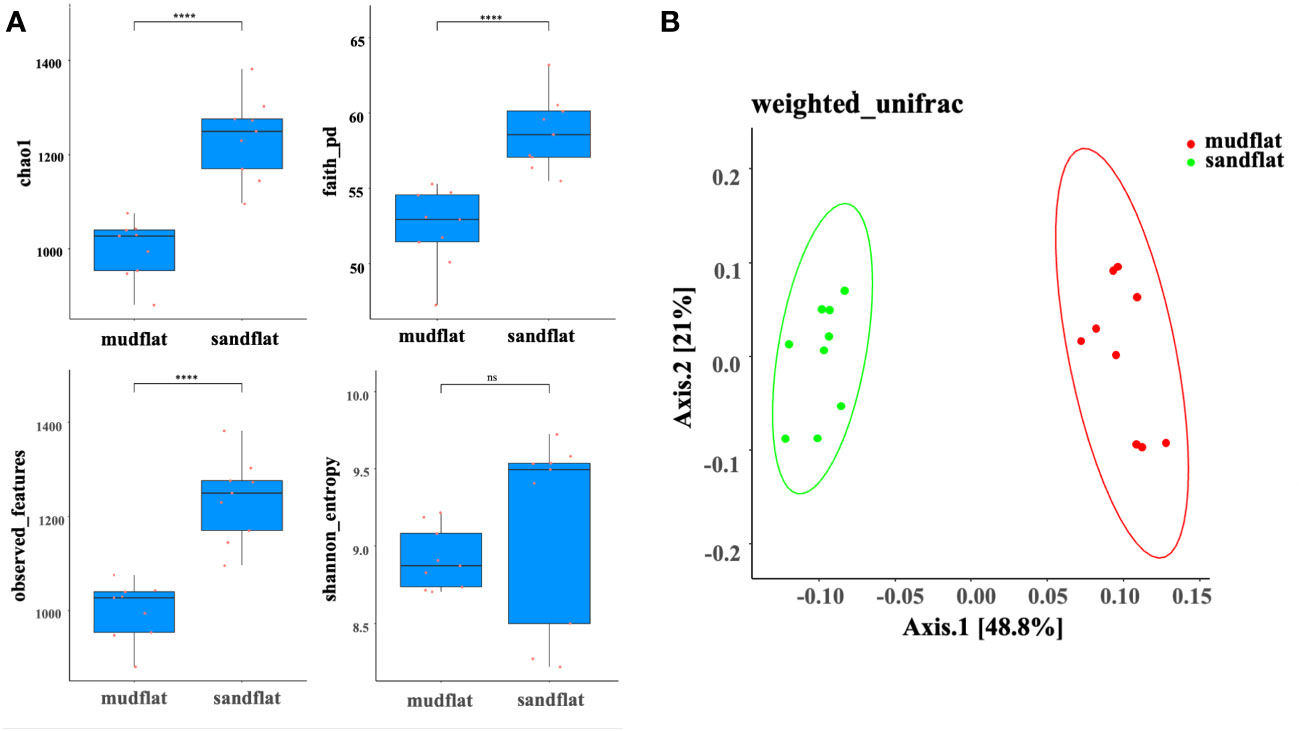
Figure 2 (A) Alpha diversity indexes of mudflat and sandflat sediments. The values of chao1, faith_pd, observed_features and Shannon_entropy. (B) Differences in microbial community distribution between mudflat and sandflat sediments assessed using principle coordinate analysis (PCoA) based on weighted_unifrac distance. ****: significance at the p < 0.05 level. ns, not significant.
A total of 2816 ASVs were identified from mudflat sediments, while 2716 ASVs were identified from sandflat sediments. Among these, 677 ASVs were unique to mudflats and 577 ASVs were unique to sandflats (Figure 3A). The dominant phyla in mudflats were Pseudomonadota, Desulfobacterota, Campilobacterota, Bacteroidota and Chloroflexota, whereas in sandflats, the dominant phyla were Pseudomonadota, Actinomycetota, Bacteroidota, Acidobacteria and Crenarchaeota (Figure 3B). A significant difference was observed in the distribution of phyla between the two sediment types, with higher abundance of Desulfobacterota, Campilobacterota, Chloroflexota, Calditrichota, Spirochaetota, Zixibacteria, Latescibacterota, Sva0485 and Deferrisomatota in mudflats, and higher abundance of Pseudomonadota, Actinomycetota, Crenarchaeota, Acidobacteriota, NB1_j, Planctomycetota, PAUC34f and AncK6 in sandflats (Supplementary Figure 2).
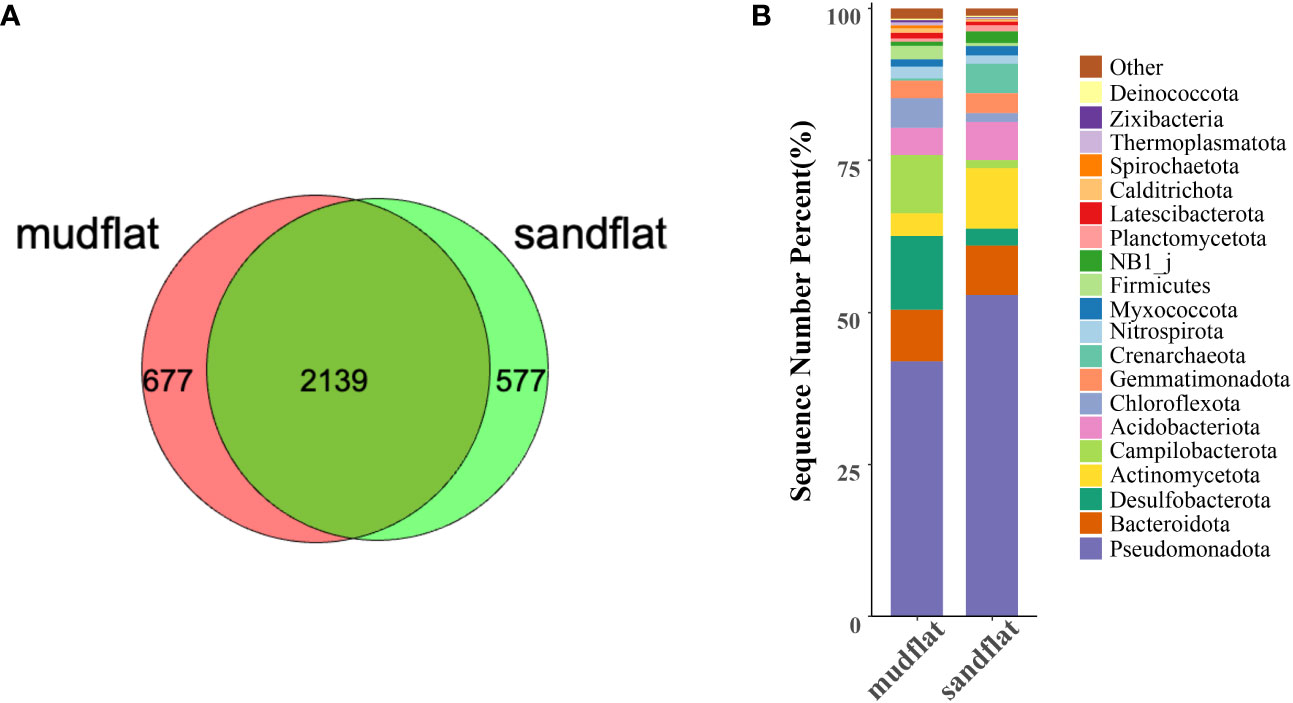
Figure 3 (A) The number of ASVs from mudflat and sandflat samples. (B) The microbial community structures and taxonomic compositions based on classifications at phylum level.
3.3 The association of microbial community structure and environmental factors
We used redundancy analysis (RDA) and envfit analysis to examine the contribution of environmental factors to variations in microbial community structure in tidal flats. The results of RDA revealed that the first two axes explained 69.35% of the total variables, with RDA1 accounting for 42.13% and RDA2 accounting for 18.9%. Between the two types of tidal flats, mudflats showed positive correlations between the abundance of microbial community and NH4+_N, TN, TOC and SC, while sandflats showed positive correlations between the abundance of microbial community and pH, ORP, and DO (Figure 4A). In which, NH4+_N (envfit analysis, r2 = 0.95), pH (envfit analysis, r2 = 0.90), TN (envfit analysis, r2 = 0.78), ORP (envfit analysis, r2 = 0.72), DO (envfit analysis, r2 = 0.68), TOC (envfit analysis, r2 = 0.67) and SC (envfit analysis, r2 = 0.60) made a significant (p< 0.01) contribution on the total variation of the microbial community structure. These findings highlighted the important role of the environment in shaping microbial communities, and the observed differences in environmental factors between mudflats and sandflats likely contributed to the variations in their microbial groups.
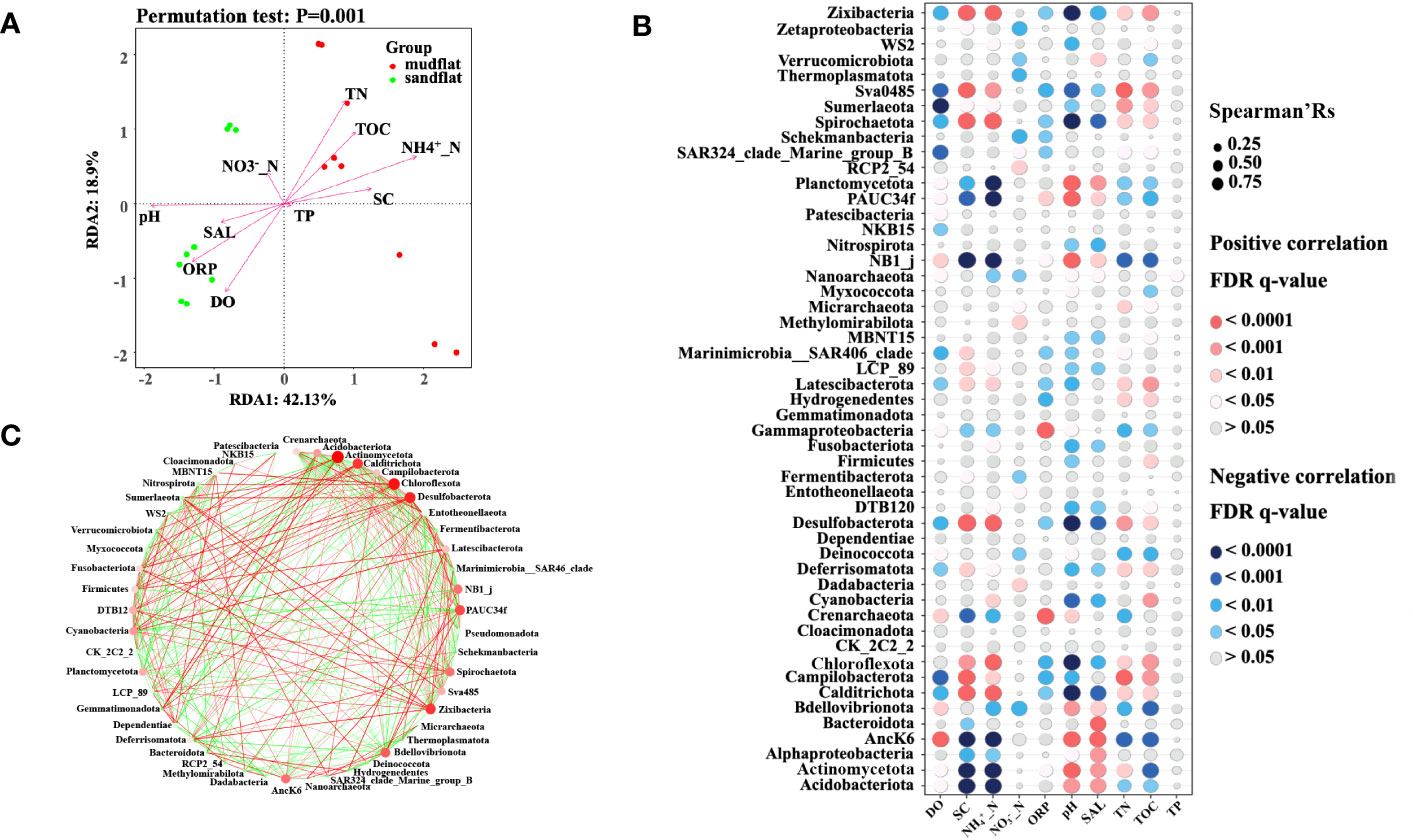
Figure 4 (A) The results of redundancy analysis (RDA) between environmental factors and microbial abundance/diversity in these two types of tidal flats. (B) The results of Spearman correlation analysis between microbial community and environmental factors. The Spearman correlation ecoefficiency is shown on the x axis and the bar are color coded by FDR q-value. The color of the bar represents the FDR (q-value) level. (C) Co-occurrence networks of the phyla in tidal flats. Notes: The size of each node indicates the abundances of the phylum, and the color of each node indicates different phyla taxa. Red and green lines represent positive and negative correlations, respectively (Spearman, p < 0.05, r > 0.5).
The correlation between microbial communities and environmental factors was analyzed using Spearman’s rank correlation coefficient. As shown in Figure 4B, the microbial groups including Desulfobacterota, Campilobacterota, Chloroflexota, Calditrichota, Spirochaetota, Zixibacteria, Latescibacterota and Sva0485, shown higher abundance in mudflat sediments, were positively associated with environmental factors including NH4+_N, TN, TOC and SC (Spearman’Rs > 0.5, q-value < 0.01), and negatively associated with pH, ORP and DO (Spearman’Rs < -0.5, q-value < 0.01), however, Chloroflexota was not negatively associated with DO (Spearman’Rs < -0.5, q-value > 0.05). Similarly, microbial groups with higher abundance in sandflat sediments, including Acidobacteriota, Crenarchaeota, Planctomycetota, Actinomycetota, NB1_j, Alphaproteobacteria and Gammaproteobacteria were positively associated with DO and pH (Spearman’Rs > 0.5, q-value < 0.05), but negatively associated with SC, NH4+_N, and TOC (Spearman’Rs < -0.5, q-value < 0.05), all of these groups, except Acidobacteriota and AncK6, showed a positively association with ORP (Spearman’Rs > 0.5, q-value < 0.05),
After evaluating the interpretive ability of environmental factors to the microbe community, we found that NH4+_N (envfit analysis, r2 = 0.95) played the most important role to the tidal flat samples. For instance, Desulfobacterota, Campilobacterota, Chloroflexota, Calditrichota, Spirochaetota, Zixibacteria, Latescibacterota and Sva0485 in mudflats were positively associated with NH4+_N (Spearman’Rs > 0.5, q-value < 0.01), on the contrary, Acidobacteriota, Crenarchaeota, Planctomycetota, Actinomycetota, NB1_j, Alphaproteobacteria and Gammaproteobacteria in sandflats were negatively associated with NH4+_N (Spearman’Rs < -0.5, q-value < 0.01). Network analysis was used to explore the co-occurrence pattern between microorganisms (Barberán et al., 2012). The network diagrams revealed 49 nodes (p < 0.05, r > 0.5) (Figure 4C). Furthermore, there were 181 edges with positive correlations and 164 edges with negative correlations. The phyla shown a significant correlation with NH4+_N, including Calditrichota, Campilobacterota, Chloroflexota, Desulfobacterota, Spirochaetota and Zixibacteria, exhibited positive correlations with each other but negative correlations with Acidobacteriota, Actinomycetota, Alphaproteobacteria and Gammaproteobacteria. The phylum Actinomycetota had the largest number of interactional nodes, with 9 positive correlations and 17 negative correlations. Pseudomonadota, the most abundant phylum, was only directly linked to the other 3 phyla. Therefore, there was a significant correlation among the interaction, the abundance of the microbial community, and environmental factors.
3.4 The differences among mudflats and sandflats in microbial biogeochemical cycles and antibiotic resistance genes
The metagenomic sequencing data was used to detect the biogeochemical functions of microbial communities in two different sediment types: mudflats and sandflats. Key genes involved in sulfur, nitrogen, and carbon cycling were annotated to analyze these functions. Although both mudflats and sandflats had similar overall functions, there were notable differences in the specific processes of sulfur, nitrogen, and carbon cycling (Figure 5). In the carbon cycling, it was observed that mudflats exhibited a significantly higher presence of genes associated with carbon fixation. The primary carbon sequestration pathways identified were the Wood-Ljungdahl pathway and the 3-Hydroxypropionate cycle. When considering fermentation, sandflats displayed a greater abundance of genes related to alcohol utilization (adh) and acetate to acetyl-CoA (acs). On the other hand, mudflats exhibited a higher prevalence of genes involved in acetate synthesis (acdA, ack, pta), pyruvate oxidation (porA) and the breakdown/synthesis of pyruvate (pflD). In the nitrogen cycling, the genes responsible for nitrogen fixation (nifD/K/H) were only found in mudflats and not in sandflats. This suggested that the process of nitrogen fixation (N2 to NH4+) only occurs in mudflats, potentially leading to higher levels of ammonium input in mudflats compared to sandflats. Conversely, the genes for ammonium oxidation (amoA/B/C) were more abundant in sandflats, indicating that microbes in sandflats might prefer to oxidize ammonium to nitrite (NH4+ to NO2-). Regarding the processes of nitrite oxidation (NO2- to NO3-) and nitrate reduction (NO3- to NO2-), the abundance of corresponding genes (nxrA/B for nitrite oxidation; napA/B and narG/H for nitrate reduction) in mudflats and sandflats were similar. The abundance of genes involved in nitrite ammonification (NO2- to NH4+) (nrfA/D/H and nirB/D) were also similar in both sandflats and mudflats. However, nrfA/D/H genes were relatively more abundant in mudflats, while nirB/D genes were relatively more abundant in sandflats. In the sulfur cycling, microbes in mudflats exhibited a higher abundance of functional genes in all processes, particularly in sulfur oxidation (sdo and dsr A/B), sulfate reduction (apr A and sat), sulfite reduction (dsrD) and thiosulfate disproportionation (phsA). In summary, sandflats showed a greater potential for ammonium oxidation, while mudflats demonstrated a stronger potential for sulfur metabolism, nitrogen fixation and carbon fixation.
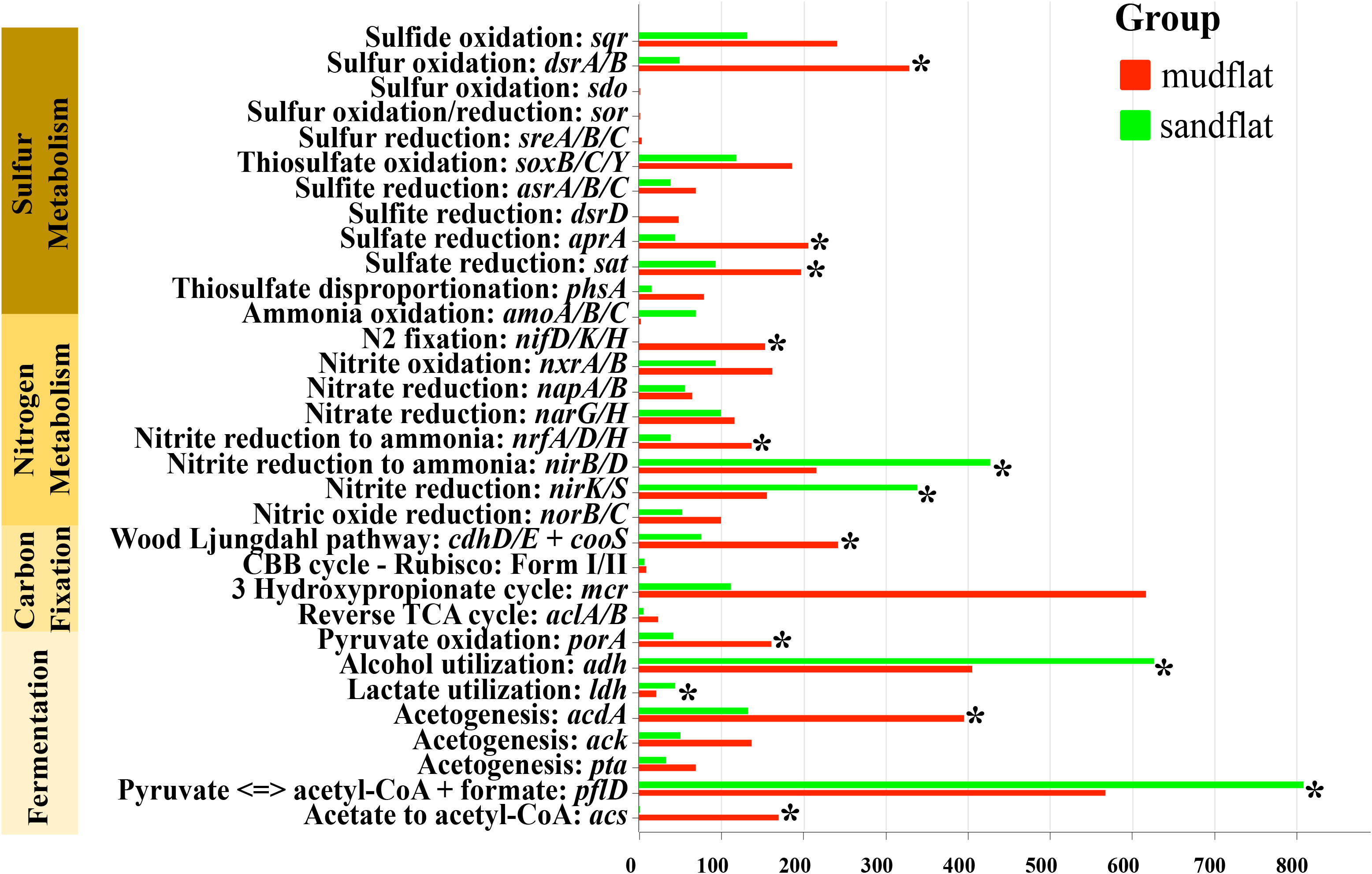
Figure 5 The abundance (TPM) of metabolic genes for these two types of tidal flats. *Significant differences (p< 0.05).
The metagenomic assemblies data was used to identify and define the ARGs in these two types of tidal flats (Supplementary Figure 3). A total of 129 ARGs subtypes (50 ARGs subtypes in sandflats samples and 79 ARGs subtypes in mudflat samples) were retained and those ARGs belonged to 14 different ARG types. Multidrug, Fluoroquinolone and Tetracycline antibiotic were the main ARG types in these two types of tidal flat. Elfamycin, Cephem, Acridinedye, Rifamycin, Triclosan, Phenicol and Diaminopyrimidine antibiotic were unique ARG types in sandflats, in which, Phenicol and Diaminopyrimidine antibiotic were abundant ones. The resistance mechanisms in both sandflats and mudflats were mainly based on antibiotic efflux (resistance-nodulation-cell division (RND) antibiotic efflux pump), antibiotic target alteration and antibiotic target protection,but antibiotic inactivation was only found in sandflats.
3.5 Phylogenetic, metabolic and functional diversity of metagenome-assembled genomes in tidal flats
In this study, we obtained 185 bacterial and 17 archaeal draft MAGs (58 MAGs from sandflat sediments, 144 MAGs from mudflat sediments). After dereplication, we had a total of 197 MAGs (180 bacterial and 17 archaeal draft MAGs) for further analysis. The MAGs were initially characterized phylogenetically using GTDB-TK, compared with the result of 16S rRNA amplicon, all of them could be assigned to a known phylum, but some archaeal MAGs like Aenigmatarchaeota and Asgardarchaeota were only found by metagenomic assembled analysis from mudflat samples (Figure 6A; Supplementary Table 7). As shown in Figure 6B, sandflat and mudflat were not very similar in MAGs profiles under phyla level, which illuminated their difference in microbial diversity. To explore the metabolic and functional diversity of the MAGs, we summarized the functions of each MAG at the phylum level. As the results, we found genes involved in carbon, nitrogen and sulfur metabolism were widespread and shared in MAGs across all tidal flats, but distinct features also existed between different sediment types (Figure 7; Supplementary Table 8; Supplementary Figures 4–6). In the carbon cycle, the majority of MAGs contain pathways for oxidative phosphorylation and oxygen metabolism. Additionally, some MAGs had the potential for substrate-level phosphorylation through acetate formation. All samples exhibit heterotrophy, autotrophy, and mixotrophy metabolism. Notably, MAGs from mudflat samples showed potential for carbon fixation, particularly in Campilobacterota, Chloroflexota, Desulfobacterota, Gammaproteobacteria, Asgardarchaeota, Micrarchaeota, Thermoproteota and Thermoplasmatota. In the nitrogen cycle, nitrogen fixation was only identified in the mudflat, with Campilobacterota, Desulfobacterota and Gammaproteobacteria MAGs being primarily responsible for this process. Ammonium oxidation was only observed in Thermoproteota MAG from sandflat samples. Nitrate reduction was found to be widespread in both the mudflat and sandflat, with Bacteroidota, Calditrichota, Campilobacterota, Chloroflexota and Desulfobacterota MAGs being specific workers in the mudflat, while Alphaproteobacteria and Actinomycetota MAGs were specific workers in the sandflat. Nitrite reduction to ammonia was also found to be widespread in both the mudflat and sandflat, with additional specific workers including Acidobacteriota, Bacteroidota, Calditrichota, Chloroflexota, Campilobacterota, Desulfobacterota, Myxococcota and Zixibacteria MAGs being identified in the mudflat. In the sulfur cycle, the reduction process was more prevalent in mudflats. Specifically, sulfite reduction was only found in mudflats, while sulfate reduction occurs in both sediments. However, there was a greater diversity and abundance of MAGs associated with sulfate reduction in mudflats. Alphaproteobacteria, Gammaproteobacteria, Bacteroidota and Thermoproteota were common MAGs playing these functions in both sandflats or mudflats. Notably, unique MAGs like Calditrichota, Chloroflexota, Desulfobacterota and Zixibacteria MAGs were found to perform both functions in mudflats.
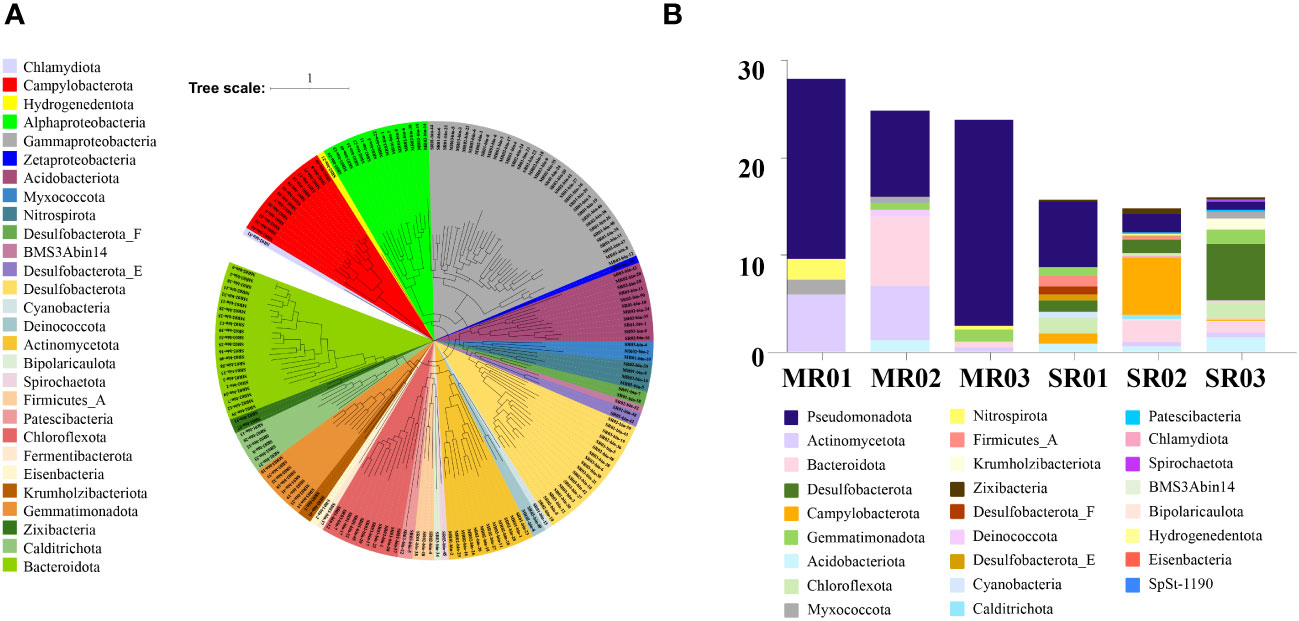
Figure 6 (A) Maximum-likelihood phylogenomic reconstruction of bacterial metagenome-assembled genomes generated in GTDB-Tk. The tree was generated with 120 bacterial marker genes. Taxa are shown at the phylum level, except for the Pseudomonadota shown at the class level. (B) Relative abundance of MAG phyla, based on normalized read coverage.
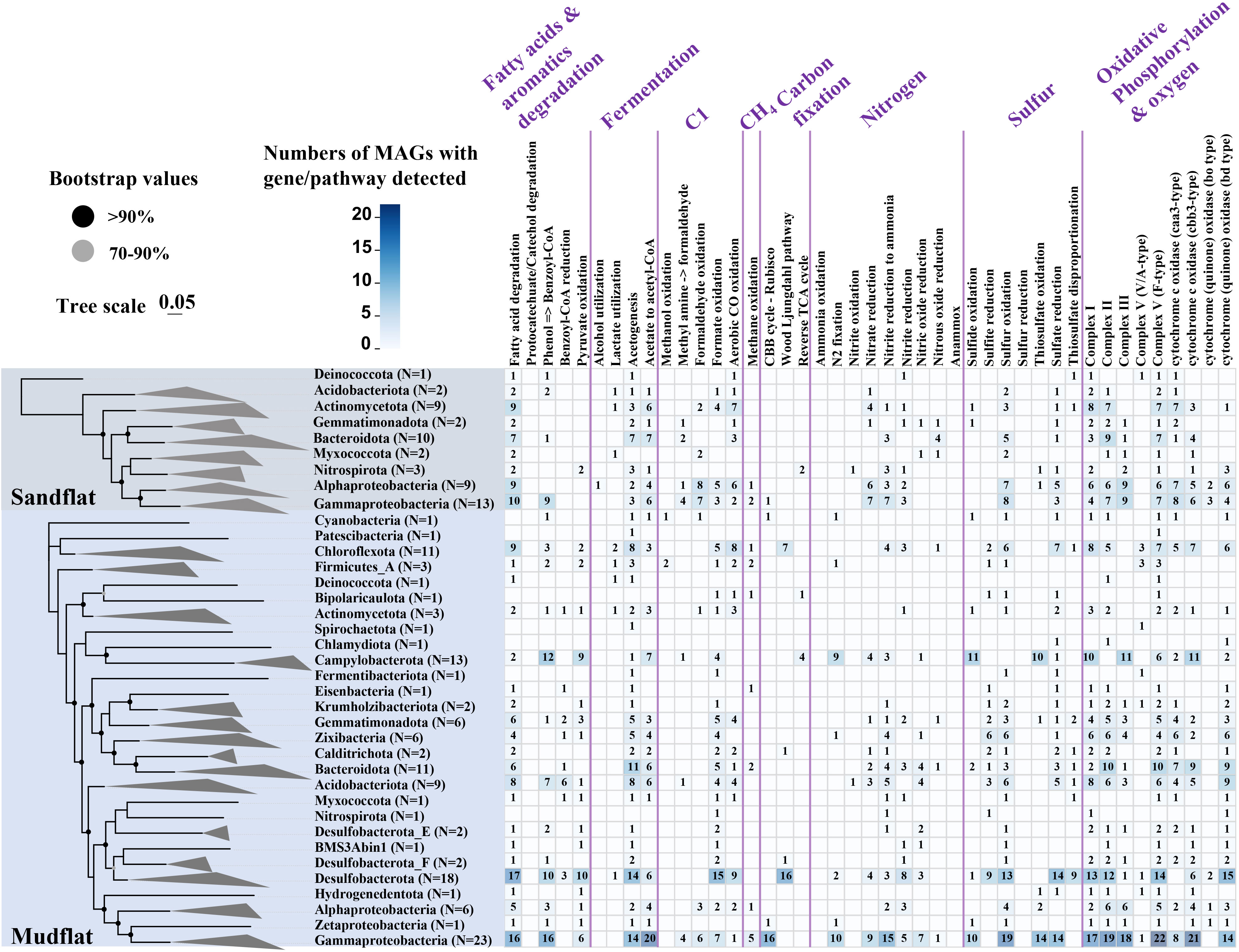
Figure 7 Core metabolic gene presence across phylogenetic clusters in these two types of tidal flats. The number of MAGs in each clade is shown in parentheses. Bacterial clades are shown at the phylum level expect for Pseudomonadota shown in class. Nodes with ultrafast bootstrap support ≥ 70% are shown with filled circles.
3.6 The predicted function of ammonium related microbes in mudflats based on MAGs
Based on the Spearman’s rank correlation analysis shown in Figure 4B, some microbes assembled in MAGs including Calditrichota, Campylobacterota, Chloroflexota, Desulfobacterota and Zixibacteria exhibited a higher abundance in the mudflat and were significantly positively correlated with NH4+_N. We selected these MAGs for further analysis to investigate their relationship with NH4+_N and their specific functions (Figure 8). As for the sulfur cycle, the reduction of sulfite (SO32- to H2S) was observed in Calditrichota (2/2 MAGs), Desulfobacterota (9/18 MAGs) and Zixibacteria (6/6 MAGs). Additionally, sulfite was produced by the process of sulfur oxidation (S to SO32-), sulfate reduction (SO42- to SO32-) and thiosulfate disproportionation (S2O32- to SO32- and H2S) and the main microbes responded for these processes above were Calditrichota (1/2, 2/2 and 1/2 MAGs, respectively), Chloroflexota (6/11, 7/11 and 1/11 MAGs, respectively), Desulfobacterota (13/18, 14/18 and 9/18 MAGs, respectively) and Zixibacteria (6/6, 1/6 and 1/6 MAGs, respectively). Campylobacterota (10/13 MAGs) predominantly participated in thiosulfate oxidation (S2O32- to SO42-). Sulfur reduction (S to H2S) was not observed in the identified MAGs, however, certain microbes like Campylobacterota (11/13 MAGs) might capable of oxidizing sulfide (H2S to S), leading to a decrease in H2S levels. For the nitrogen cycle, nitrogen fixation (N2 to NH4+) was found in Campylobacterota (9/13 MAGs), Desulfobacterota (2/18 MAGs) and Zixibacteria (1/6 MAGs). Nitrite reduction to ammonia (NO2- to NH4+) was detected in Calditrichota (1/2 MAGs), Campylobacterota (3/13 MAGs), Chloroflexota (3/11 MAGs), Desulfobacterota (3/18 MAGs) and Zixibacteria (4/6 MAGs). Nitrate reduction (NO3- to NO2-) was found in Calditrichota (1/2 MAGs), Campylobacterota (4/13 MAGs) and Desulfobacterota (4/18 MAGs). Nitrite reduction (NO2- to NO) and nitric oxide reduction (NO to N2O) were both found in Desulfobacterota (8/18 and 3/18 MAGs, respectively). The genes for nitrous oxide reduction (N2O to N2) was only found in Chloroflexota (1/13 MAGs). However, no MAGs were found to be involved in nitrification (NH4+ to NO3-/NO2-).
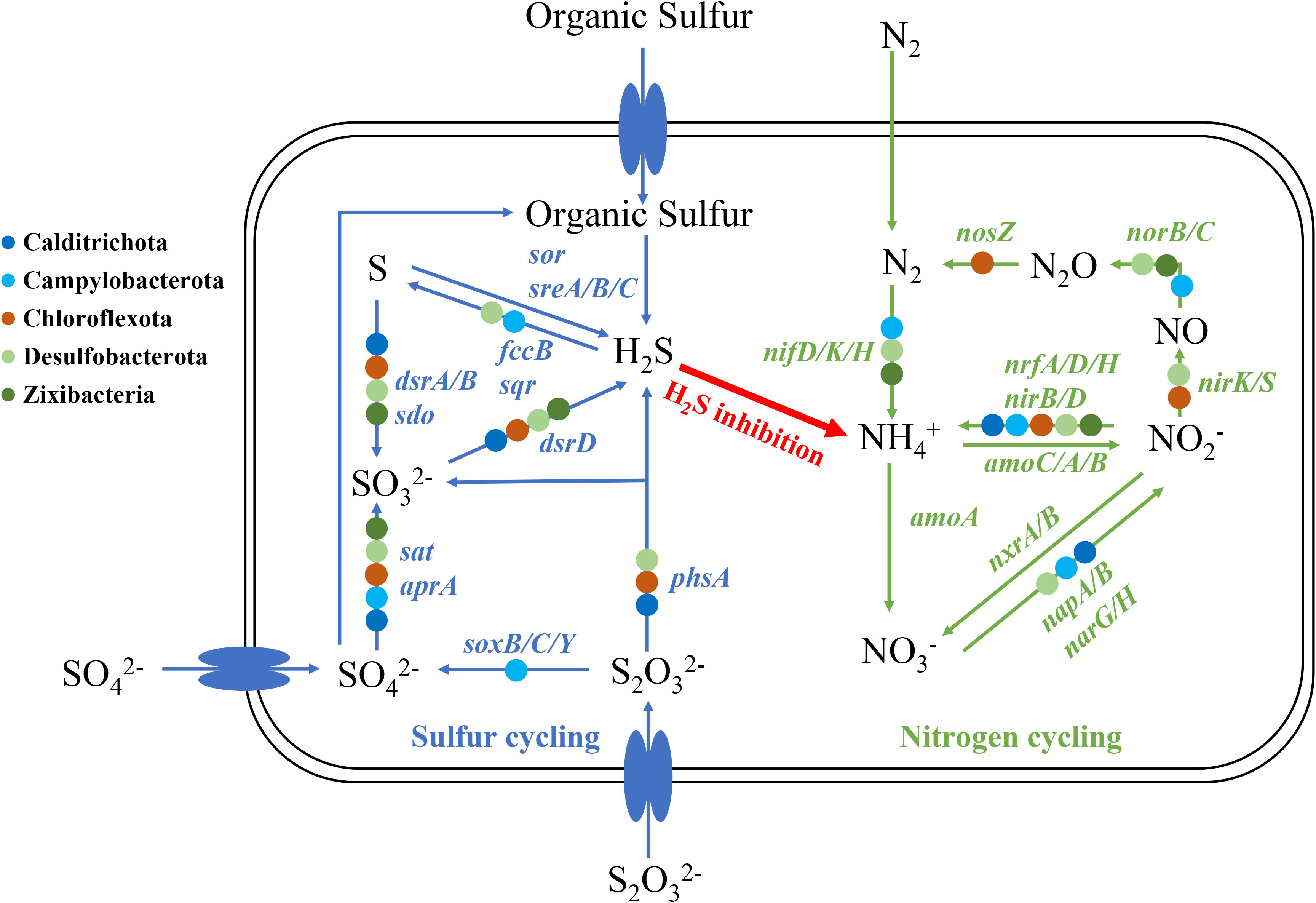
Figure 8 Potential scheme of microbial nitrogen and sulfur metabolisms and associated interactions in mudflats.
4 Discussion and conclusion
4.1 Two types of tidal flats in Beibu Gulf were distinct in environmental factors including TOC, NH4+_N and ORP
Mudflats and sandflats, two common types of tidal flats, are distinguished by their grain size and sediment composition, low-grain mudflat sediments and large-grain sandflat sediments are easily formed by the influence of erosion and sedimentation processes (Thrush et al., 2004). During sampling, we found that mangroves Avicennia marina were present near all sampled mudflats, and the presence of seedlings indicated that the mangroves were actively expanding. This suggested that the mudflats we studied primarily consist of terrestrial sediments with a high sedimentation rate, while in the sandflats, tidal processes and the deposition of coarse-grained sediments dominated (Lovelock et al., 2007; Swales et al., 2007; Flemming, 2011). The contents of TOC (p < 0.05) and NH4+_N (p < 0.01) in mudflats were significantly higher than those in sandflats (Supplementary Table 3). The high concentration of TOC was consistent with the previous results (Trimmer et al., 2000; Rauch and Denis, 2008), and the high concentration of TOC was, on the one hand, due to the input of terrestrial sediments and carbon fixation by plants, algae and microbes, on the other hand, as the result of the small charged particles enhanced the adsorption of TOC (Yang et al., 2015; Arias-Ortiz et al., 2021; Boey et al., 2021). Meanwhile, increasingly anaerobic, cohesive and impermeable conditions in mudflat sediments also encouraged the accumulation of NH4+_N (Gooday et al., 2009; Moseman-Valtierra et al., 2010; Douglas et al., 2018). In contrast, our result that ORP was higher in sandflat (Supplementary Table 3), indicating mudflat sediments exhibit strong reducibility, the reason for this differences could be due to high permeability and aerobic environments in sandflat (Woulds et al., 2016).
4.2 Mudflats had lower microbial diversity than sandflats
The environment is a significant factor in the selection of microorganisms (Webb et al., 2002). Various environmental factors, such as sediment properties and nutrient availability, can either promote or inhibit bacterial community structure and function to some extent (Buyer et al., 2010; Wang et al., 2020b). Our results showed that in the alpha diversity, the species abundance and diversity in sandflat were higher than those in mudflat, mudflats and sandflats was different in microbial diversity and microbial community structure (Figure 2). This result is not consistent with previous studies which mudflat had higher microbial diversity than sandflat (Boey et al., 2021). This discrepancy may be attributed to the higher oxygen content and ORP found in the sandflat, which is a result of its stronger permeability and heterogeneous physicochemical gradients (Huettel et al., 2014; Woulds et al., 2016). Higher oxygen content and ORP could increase heterotrophic activity and boost higher biomass such as Actinomycetota, Bacteroidota and Pseudomonadota (Hou et al., 2017). On the contrary, the lower oxygen content and ORP would lead to the environment in a state of hypoxia/anoxia, resulting in changes in microbial community structure, some anaerobic bacteria like Desulfobacterota would produce H2S through sulfate reduction and interrupt the growth of other microorganisms (Pett-Ridge and Firestone, 2005; Sinkko et al., 2019). Meanwhile, the higher diversity in the sandflat may be attributed to the influence of tides, the occurrence of a large number of transient species and/or the continuous aerobic degradation of organic carbon providing broader ecological niche (Boey et al., 2021).
4.3 The differences of microbial functions between sandflat and mudflat in Beibu Gulf
The distinct environmental factors between sandflat and mudflat result in significant differences in biodiversity, which in turn leads to variations in microbial functions. Through the analysis of metagenome sequences, it was observed that mudflats had a higher abundance of genes related to carbon fixation through the Wood-Ljungdahl pathway and 3-Hydroxypropionate cycle (Figure 5). The Wood-Ljungdahl pathway was identified in Calditrichota, Chloroflexota and Desulfobacterota MAGs, while no MAGs were found to be responsible for the 3-Hydroxypropionate cycle (Figure 7). Consequently, further study should focus on the strains responsible for the 3-Hydroxypropionate cycle. Furthermore, nitrogen fixation was only existed in mudflat and annotated in Campilobacterota, Desulfobacterota, Firmicutes, Gammaproteobacteria, Zetaproteobacteria and Zixibacteria, combined with the proportion of most of these microbes were also higher in mudflat (Figure 3B), indicating that these microbes might mainly contribute to the high NH4+_N shown in the mudflat. The ratio of genes for ammonium oxidation were higher in sandflat, even MAGs responsible for it was not found, it was also illuminated that ammonium oxidation was the unique feature of sandflat (Figures 5, 7). In addition, mudflats had a stronger potential in sulfur metabolism, Acidobacteriota, Bacteroidota, Campilobacterota, Calditrichota, Chloroflexota, Desulfobacterota, Gammaproteobacteria, Gemmatimonadota and Zixibacteria MAGs were mainly participated in it (Figures 5, 7). Mudflats were dominated by diffusion transport, which promoted the fermentation of organic matter (Douglas et al., 2018), the traditional biogeochemical processes such as microbial fermentation were related to the rapid metabolism of sulfur in sediments (Bowles et al., 2014). It had also shown that the sulfur cycle was mainly driven by sulfate reduction (Wasmund et al., 2017), and among the oxidants consumed by reoxidation and reduction, microorganisms in mudflats tended to use sulfate and metal ions as electron acceptors (Braker et al., 2001). These illuminated sulfur related MAGs may also function in fermentation and metal cycle of mudflats.
4.4 The unique microbial community in mudflats played an important role in high ammonium accumulation
Based on the result of Spearman’s rank correlation, RDA and envfit analysis, the content of NH4+_N was the most important environmental factor to the microbial community in mudflats, this feature could be attributed to several potential factors. On the one hand, mudflat was an anoxic, organic-matter-rich environment with strong reducible property. In such environment, the nitrification would be inhibited due to the lack of oxygen, and the sufficient organic matter would be oxidized and stimulate sulfate reduction to produce H2S, which was then passed through blocking the necessary intermediate step of nitrification and separated nitrogen regeneration from denitrification (Lovley and Klug, 1986). In addition, some of the microbes like nitrate reducing and nitrite ammoniating microbial groups prefer high-reduction anoxic environments would not grow well in it (Gao et al., 2010). On the other hand, it relied on the unique microbial community in mudflats. For example, Desulfobacterota, typically occurred in hypoxic place, traditionally regarded as bacteria that both reduce and oxidize sulfates in wetland systems, it could produce H2S through sulfite reduction and thiosulfate disproportionation and high concentration of H2S would inhibit the oxidation of NH4+_N (Joye and Hollibaugh, 1995; Joye and Anderson, 2008). In addition, Calditrichota and Zixibacteria could also be attributed to the production of H2S. Campilobacterota in mudflats encoded genes including nitrogen fixation, nitrate reduction, nitrite reduction to ammonium, and thiosulfate oxidation, it produced NH4+_N by nitrogen fixation, nitrate reduction and nitrite reduction to ammonium, and helped other microbial groups like Desulfobacterota by providing sulfate which could link and regulate the S and N cycles, and stratify the microbial communities (Huang et al., 2021). In summary, the high accumulation of ammonium in mudflat would be the result of strong reductive and anoxic conditions, and the unique microbial groups such as Calditrichota, Campilobacterota, Desulfobacterota and Zixibacteria.
Data availability statement
The original contributions presented in the study are publicly available. This data can be found here: NCBI-BioProject, PRJNA774167 and PRJNA967168.
Author contributions
Y-LY: Data curation, Formal Analysis, Investigation, Methodology, Software, Writing – original draft. K-JM: Data curation, Investigation, Methodology, Software, Writing – review & editing. Y-HF: Formal Analysis, Investigation, Writing – review & editing. Z-CW: Data curation, Investigation, Software, Writing – review & editing, Conceptualization, Formal Analysis. G-YF: Project administration, Supervision, Writing – review & editing. CS: Conceptualization, Funding acquisition, Methodology, Project administration, Supervision, Writing – review & editing, Writing – original draft. X-WX: Conceptualization, Funding acquisition, Project administration, Supervision, Writing – review & editing.
Funding
This study was supported by National Science and Technology Fundamental Resources Investigation Program of China (2019FY100700), Zhejiang Provincial Natural Science Foundation of China (LDT23D06025D06), the Key R&D Program of Zhejiang (#2023C03011), National Natural Science Foundation of China (No. 31900003) and the Fundamental Research Funds of Zhejiang Sci-Tech University (22042315-Y).
Acknowledgments
We appreciate the help of Dr. Lin Xu in the guide of metagenome analysis.
Conflict of interest
CS was employed by Zhejiang Sci-Tech University Shaoxing Academy of Biomedicine Co., Ltd.
The remaining authors declare that the research was conducted in the absence of any commercial or financial relationships that could be construed as a potential conflict of interest.
The authors declared that they were an editorial board member of Frontiers, at the time of submission. This had no impact on the peer review process and the final decision
Publisher’s note
All claims expressed in this article are solely those of the authors and do not necessarily represent those of their affiliated organizations, or those of the publisher, the editors and the reviewers. Any product that may be evaluated in this article, or claim that may be made by its manufacturer, is not guaranteed or endorsed by the publisher.
Supplementary material
The Supplementary Material for this article can be found online at: https://www.frontiersin.org/articles/10.3389/fmars.2023.1256393/full#supplementary-material
References
Ames B. N. (1966). Assay of inorganic phosphate, total phosphate and phosphatase. Meth. Enzymol. 8, 115–118. doi: 10.1016/0076-6879(66)08014-5
Aramaki T., Blanc-Mathieu R., Endo H., Ohkubo K., Kanehisa M., Goto S., et al. (2020). KofamKOALA: KEGG ortholog assignment based on profile HMM and adaptive score threshold. Bioinformatics 36 (7), 2251–2252. doi: 10.1093/bioinformatics/btz859
Arias-Ortiz A., Masqué P., Glass L., Benson L., Kennedy H., Duarte C. M., et al. (2021). Losses of soil organic carbon with deforestation in mangroves of Madagascar. Ecosystems 24 (1), 1–19. doi: 10.1007/s10021-020-00500-z
Barberán A., Bates S. T., Casamayor E. O., Fierer N. (2012). Using network analysis to explore co-occurrence patterns in soil microbial communities. ISME J. 6 (2), 343–351. doi: 10.1038/ismej.2011.119
Böer S. I., Hedtkamp S. I., Van Beusekom J. E., Fuhrman J. A., Boetius A., Ramette A. (2009). Time-and sediment depth-related variations in bacterial diversity and community structure in subtidal sands. ISME J. 3 (7), 780–791. doi: 10.1038/ismej.2009.29
Bolger A. M., Lohse M., Usadel B. (2014). Trimmomatic: a flexible trimmer for illumina sequence data. Bioinformatics 30 (15), 2114–2120. doi: 10.1093/bioinformatics/btu170
Boey J. S., Mortimer R., Couturier A., Worrallo K., Handley K. M. (2021). Estuarine microbial diversity and nitrogen cycling increase along sand–mud gradients independent of salinity and distance. Environ. Microbiol. 24, 50–65. doi: 10.1111/1462-2920.15550
Bowers R. M., Kyrpides N. C., Stepanauskas R., Harmon-Smith M., Doud D., Reddy T., et al. (2017). Minimum information about a single amplified genome (MISAG) and a metagenome-assembled genome (MIMAG) of bacteria and archaea. Nat. Biotechnol. 35 (8), 725–731. doi: 10.1038/nbt.3893
Bowles M. W., Mogollón J. M., Kasten S., Zabel M., Hinrichs K.-U. (2014). Global rates of marine sulfate reduction and implications for sub–sea-floor metabolic activities. Science 344(6186), 889–891. doi: 10.1126/science.1249213
Braker G., Ayala-del-Rıío H. L., Devol A. H., Fesefeldt A., Tiedje J. M. (2001). Community structure of denitrifiers, Bacteria, and Archaea along redox gradients in Pacific Northwest marine sediments by terminal restriction fragment length polymorphism analysis of amplified nitrite reductase (nirS) and 16S rRNA genes. Appl. Environ. Microbiol. 67 (4), 1893–1901. doi: 10.1128/AEM.67.4.1893-1901.2001
Bray J. R., Curtis J. T. (1957). An ordination of the upland forest communities of southern wisconsin. Ecol. Monogr. 27 (4), 326–349. doi: 10.2307/1942268
Buyer J. S., Teasdale J. R., Roberts D. P., Zasada I. A., Maul J. E. (2010). Factors affecting soil microbial community structure in tomato cropping systems. Soil Boil Biochem. 42 (5), 831–841. doi: 10.1016/j.soilbio.2010.01.020
Chaumeil P.-A., Mussig A. J., Hugenholtz P., Parks D. H. (2019). GTDB-Tk: a toolkit to classify genomes with the Genome Taxonomy Database. Bioinformatics 36 (6), 1925–1927. doi: 10.1093/bioinformatics/btz848
Cummings V., Vopel K., Thrush S. (2009). Terrigenous deposits in coastal marine habitats: influences on sediment geochemistry and behaviour of post-settlement bivalves. Mar. Ecol. Prog. Ser. 383, 173–185. doi: 10.3354/meps07983
Dou Y., Li J., Zhao J., Hu B., Yang S. (2013). Distribution, enrichment and source of heavy metals in surface sediments of the eastern Beibu Bay, South China Sea. Mar. pollut. Bull. 67 (1-2), 137–145. doi: 10.1016/j.marpolbul.2012.11.022
Douglas E. J., Pilditch C. A., Lohrer A. M., Savage C., Schipper L. A., Thrush S. F. (2018). Sedimentary environment influences ecosystem response to nutrient enrichment. Estuar. Coast. 41 (7), 1994–2008. doi: 10.1007/s12237-018-0416-5
Drula E., Garron M.-L., Dogan S., Lombard V., Henrissat B., Terrapon N. (2022). The carbohydrate-active enzyme database: functions and literature. Nucleic Acids Res. 50 (D1), D571–D577. doi: 10.1093/nar/gkab1045
Du J., Xiao K., Huang Y., Li H., Tan H., Cao L., et al. (2011). Seasonal and spatial diversity of microbial communities in marine sediments of the South China Sea. Anton Leeuw 100 (3), 317–331. doi: 10.1007/s10482-011-9587-9
Flemming B. (2011). 3.02-geology, morphology, and sedimentology of estuaries and coasts. Waltham: Acad. Press 3, 7–38. doi: 10.1016/B978-0-12-374711-2.00302-8
Folk R. L., Andrews P. B., Lewis D. W. (1970). Detrital sedimentary rock classification and nomenclature for use in New Zealand. New Zealand J. Geol. Geophys. 13 (4), 937–968. doi: 10.1080/00288306.1970.10418211
Gao H., Schreiber F., Collins G., Jensen M. M., Kostka J. E., Lavik G., et al. (2010). Aerobic denitrification in permeable wadden sea sediments. ISME J. 4 (3), 417–426. doi: 10.1038/ismej.2009.127
Garber A. I., Nealson K. H., Okamoto A., McAllister S. M., Chan C. S., Barco R. A., et al. (2020). FeGenie: a comprehensive tool for the identification of iron genes and iron gene neighborhoods in genome and metagenome assemblies. Front. Microbiol. 37. doi: 10.3389/fmicb.2020.00037
Gooday A., Jorissen F., Levin L., Middelburg J., Naqvi S., Rabalais N., et al. (2009). Historical records of coastal eutrophication-induced hypoxia. Biogeosciences 6 (8), 1707–1745. doi: 10.5194/bg-6-1707-2009
Gurevich A., Saveliev V., Vyahhi N., Tesler G. (2013). QUAST: quality assessment tool for genome assemblies. Bioinformatics 29 (8), 1072–1075. doi: 10.1093/bioinformatics/btt086
He B., Lai T., Fan H., Wang W., Zheng H. (2007). Comparison of flooding-tolerance in four mangrove species in a diurnal tidal zone in the Beibu Gulf. Estuar. Coast. Shelf S 74 (1-2), 254–262. doi: 10.1016/j.ecss.2007.04.018
Healy T., Wang Y., Healy J.-A. (2002). Muddy coasts of the world: processes, deposits and function (Amsterdam: Elsevier).
Hou Z., Nelson W. C., Stegen J. C., Murray C. J., Arntzen E., Crump A. R., et al. (2017). Geochemical and microbial community attributes in relation to hyporheic zone geological facies. Sci. Rep. 7 (1), 12006. doi: 10.1038/s41598-017-12275-w
Huang L., Bae H. S., Young C., Pain A. J., Martin J. B., Ogram A. (2021). Campylobacterota dominate the microbial communities in a tropical karst subterranean estuary, with implications for cycling and export of nitrogen to coastal waters. Environ. Microbiol. 23 (11), 6749–6763. doi: 10.1111/1462-2920.15746
Huettel M., Berg P., Kostka J. E. (2014). Benthic exchange and biogeochemical cycling in permeable sediments. Annu. Rev. Mar. Sci. 6, 23–51. doi: 10.1146/annurev-marine-051413-012706
Hyatt D., Chen G.-L., LoCascio P. F., Land M. L., Larimer F. W., Hauser L. J. (2010). Prodigal: prokaryotic gene recognition and translation initiation site identification. BMC Bioinf. 11 (1), 1–11. doi: 10.1186/1471-2105-11-119
Joye S. B., Anderson I. C. (2008). Nitrogen cycling in coastal sediments. Nitrogen Mar. Environ. 2, 868–915. doi: 10.1016/B978-0-12-372522-6.00019-0
Joye S. B., Hollibaugh J. T. (1995). Influence of sulfide inhibition of nitrification on nitrogen regeneration in sediments. Science 270, 623–625. doi: 10.1126/science.270.5236.62
Ki B.-M., Huh I. A., Choi J.-H., Cho K.-S. (2018). Relationship of nutrient dynamics and bacterial community structure at the water–sediment interface using a benthic chamber experiment. J. Environ. Sci. Heal A 53 (5), 482–491. doi: 10.1080/10934529.2017.1412191
Kjeldahl J. (1883). A new method for the determination of nitrogen in organic matter. Z. für Analytische Chemie 22, 366–382 . doi: 10.1007/BF01338151
Letunic I., Bork P. (2021). Interactive tree of life (iTOL) v5: an online tool for phylogenetic tree display and annotation. Nucleic Acids Res. 49 (W1), W293–W296. doi: 10.1093/nar/gkab301
Li N., Chen X., Zhao H., Tang J., Jiang G., Li Z., et al. (2020). Spatial distribution and functional profile of the bacterial community in response to eutrophication in the subtropical Beibu Gulf, China. Mar. pollut. Bull. 161, 111742. doi: 10.1016/j.marpolbul.2020.111742
Liu Y.-X., Qin Y., Chen T., Lu M., Qian X., Guo X., et al. (2021). A practical guide to amplicon and metagenomic analysis of microbiome data. Protein Cell 12 (5), 315–330. doi: 10.1007/s13238-020-00724-8
Lohrer A. M., Thrush S. F., Hewitt J. E., Berkenbusch K., Ahrens M., Cummings V. J. (2004). Terrestrially derived sediment: response of marine macrobenthic communities to thin terrigenous deposits. Mar. Ecol. Prog. Ser. 273, 121–138. doi: 10.3354/meps273121
Lovelock C. E., Feller I. C., Ellis J., Schwarz A. M., Hancock N., Nichols P., et al. (2007). Mangrove growth in New Zealand estuaries: the role of nutrient enrichment at sites with contrasting rates of sedimentation. Oecologia 153 (3), 633–641. doi: 10.1007/s00442-007-0750-y
Lovley D. R., Klug M. J. (1986). Model for the distribution of sulfate reduction and methanogenesis in freshwater sediments. Geochimica Cosmochimica Acta 50 (1), 11–18. doi: 10.1016/0016-7037(86)90043-8
Lozupone C., Knight R. (2005). UniFrac: a new phylogenetic method for comparing microbial communities. Appl. Environ. Microbiol. 71 (12), 8228–8235. doi: 10.1128/AEM.71.12.8228-8235.2005
Lv X., Ma B., Yu J., Chang S. X., Xu J., Li Y., et al. (2016). Bacterial community structure and function shift along a successional series of tidal flats in the Yellow River Delta. Sci. Rep-UK 6 (1), 1–10. doi: 10.1038/srep36550
Ma F., Wang Y., Li Y., Ye C., Xu Z., Zhang F. (2010). The application of geostatistics in grain size trend analysis: a case study of eastern Beibu Gulf. J. Geogr. Sci. 20 (1), 77–90. doi: 10.1007/s11442-010-0077-1
McArdle B. H., Anderson M. J. (2001). Fitting multivariate models to community data: a comment on distance-based redundancy analysis. Ecology 82 (1), 290–297. doi: 10.1890/00129658(2001)082[0290:FMMTCD]2.0.CO;2
Moseman-Valtierra S., Armaiz-Nolla K., Levin L. (2010). Wetland response to sedimentation and nitrogen loading: diversification and inhibition of nitrogen-fixing microbes. Ecol. Appl. 20 (6), 1556–1568. doi: 10.1890/08-1881.1
Murray N. J., Phinn S. R., DeWitt M., Ferrari R., Johnston R., Lyons M. B., et al. (2019). The global distribution and trajectory of tidal flats. Nature 565 (7738), 222–225. doi: 10.1038/s41586-018-0805-8
Nicholls R. J., Wong P., Burkett V., Codignotto J., Hay J., McLean R., et al. (2007). Climate change 2007: impacts, adaptation and vulnerability. Contribution of working group II to the fourth assessment report of the intergovernmental panel on climate change (Cambridge: Cambridge University Press).
Olm M. R., Brown C. T., Brooks B., Banfield J. F. (2017). dRep: a tool for fast and accurate genomic comparisons that enables improved genome recovery from metagenomes through de-replication. ISME J. 11 (12), 2864–2868. doi: 10.1038/ismej.2017.126
Osland M. J., Enwright N. M., Day R. H., Gabler C. A., Stagg C. L., Grace J. B. (2016). Beyond just sea-level rise: Considering macroclimatic drivers within coastal wetland vulnerability assessments to climate change. Global Change Biol. 22 (1), 1–11. doi: 10.1111/gcb.13084
Parks D. H., Tyson G. W., Hugenholtz P., Beiko R. G. (2014). STAMP: statistical analysis of taxonomic and functional profiles. Bioinformatics 30 (21), 3123–3124. doi: 10.1093/bioinformatics/btu494
Passeri D. L., Hagen S. C., Medeiros S. C., Bilskie M. V., Alizad K., Wang D. (2015). The dynamic effects of sea level rise on low-gradient coastal landscapes: A review. Earths Future 3 (6), 159–181. doi: 10.1002/2015EF000298
Peterson G. L. (1979). Review of the folin phenol protein quantitation method of lowry, rosebrough, farr and randall. Anal. Biochem. 100 (2), 201–220. doi: 10.1016/0003-2697(79)90222-7
Pett-Ridge J., Firestone M. (2005). Redox fluctuation structures microbial communities in a wet tropical soil. Appl. Environ. Microbiol. 71 (11), 6998–7007. doi: 10.1128/AEM.71.11.6998-7007.2005
Quast C., Pruesse E., Yilmaz P., Gerken J., Schweer T., Yarza P., et al. (2012). The SILVA ribosomal RNA gene database project: improved data processing and web-based tools. Nucleic Acids Res. 41 (D1), D590–D596. doi: 10.1093/nar/gks1219
Rauch M., Denis L. (2008). Spatio-temporal variability in benthic mineralization processes in the eastern English Channel. Biogeochemistry 89 (2), 163–180. doi: 10.1007/s10533-008-9191-x
Rawlings N. D., Barrett A. J., Thomas P. D., Huang X., Bateman A., Finn R. D. (2018). The MEROPS database of proteolytic enzymes, their substrates and inhibitors in 2017 and a comparison with peptidases in the PANTHER database. Nucleic Acids Res. 46 (D1), D624–D632. doi: 10.1093/nar/gkx1134
Sarukhán J., Whyte A., Hassan R., Scholes R., Ash N., Carpenter S., et al. (2005). Millenium ecosystem assessment: Ecosystems and human well-being (Washington, DC: Island Press).
Schlitzer R. (2021) Ocean data view. Available at: https://odv.awi.de.
Sims R. P. A. (1961). Formation of heteropoly blue by some reduction procedures used in the micro-determination of phosphorus. Analyst 86 (1026), 584–590. doi: 10.1039/AN9618600584
Sinkko H., Hepolehto I., Lyra C., Rinta-Kanto J. M., Villnäs A., Norkko J., et al. (2019). Increasing oxygen deficiency changes rare and moderately abundant bacterial communities in coastal soft sediments. Sci. Rep. 9 (1), 1–14. doi: 10.1038/s41598-019-51432-1
Søndergaard D., Pedersen C. N., Greening C. (2016). HydDB: a web tool for hydrogenase classification and analysis. Sci. Rep. 6 (1), 1–8. doi: 10.1038/srep34212
Spearman C. (1987). The proof and measurement of association between two things. Am. J. Psychol. 100 (3/4), 441–471. doi: 10.2307/1422689
Swales A., Bentley S. J., Lovelock C., Bell R. G. (2007). “Sediment processes and mangrove-habitat expansion on a rapidly-prograding muddy coast, New Zealand,” in Coastal sediments (Virginia: Amer Society of Civil Engineers), 1441–1454.
Thompson L. R., Sanders J. G., McDonald D., Amir A., Ladau J., Locey K. J., et al. (2017). A communal catalogue reveals Earth’s multiscale microbial diversity. Nature 551 (7681), 457–463. doi: 10.1038/nature24621
Thrush S., Hewitt J., Cummings V., Ellis J., Hatton C., Lohrer A., et al. (2004). Muddy waters: elevating sediment input to coastal and estuarine habitats. Front. Ecol. Environ. 2 (6), 299–306. doi: 10.2307/3868405
Trimmer M., Nedwell D. B., Sivyer D. B., Malcolm S. J. (2000). Seasonal benthic organic matter mineralisation measured by oxygen uptake and denitrification along a transect of the inner and outer River Thames estuary, UK. Mar. Ecol. Prog. Ser. 197, 103–119. doi: 10.3354/meps197103
Uritskiy G. V., DiRuggiero J., Taylor J. (2018). MetaWRAP–a flexible pipeline for genome-resolved metagenomic data analysis. Microbiome 6 (1), 1–13. doi: 10.1186/s40168-018-0541-1
Wang X., Yang J., Xie X., Chen X., Pu L., Zhang X. (2020b). Soil microbial succession with soil development since costal reclamation. Catena 187, 104393. doi: 10.1016/j.catena.2019.104393
Wang W., Yang Y., Zhou Y., Zhang S., Wang X., Yang Z. (2020a). Impact of anthropogenic activities on the sediment microbial communities of Baiyangdian shallow lake. Int. J. Sediment Res. 35 (2), 180–192. doi: 10.1016/j.ijsrc.2019.10.006
Wasmund K., Mußmann M., Loy A. (2017). The life sulfuric: microbial ecology of sulfur cycling in marine sediments. Environ. Microbiol. Rep. 9 (4), 323–344. doi: 10.1111/1758-2229.12538
Webb C. O., Ackerly D. D., McPeek M. A., Donoghue M. J. (2002). Phylogenies and community ecology. Annu. Rev. Ecol. Evol. S 33 (1), 475–505. doi: 10.1146/annurev.ecolsys.33.010802.150448
Welch B. L. (1947). The generalization of ‘student’s’ problem when several different population varlances are involved. Biometrika 34 (1-2), 28–35. doi: 10.1093/biomet/34.1-2.28
Woulds C., Bouillon S., Cowie G. L., Drake E., Middelburg J. J., Witte U. (2016). Patterns of carbon processing at the seafloor: the role of faunal and microbial communities in moderating carbon flows. Biogeosciences 13 (15), 4343–4357. doi: 10.5194/bg-13-4343-2016
Yang B., Cao L., Liu S.-M., Zhang G.-S. (2015). Biogeochemistry of bulk organic matter and biogenic elements in surface sediments of the Yangtze River Estuary and adjacent sea. Mar. pollut. Bull. 96 (1-2), 471–484. doi: 10.1016/j.marpolbul.2015.05.003
Yu N. Y., Wagner J. R., Laird M. R., Melli G., Rey S., Lo R., et al. (2010). PSORTb 3.0: improved protein subcellular localization prediction with refined localization subcategories and predictive capabilities for all prokaryotes. Bioinformatics 26 (13), 1608–1615. doi: 10.1093/bioinformatics/btq249
Zhang H., Yohe T., Huang L., Entwistle S., Wu P., Yang Z., et al. (2018). dbCAN2: a meta server for automated carbohydrate-active enzyme annotation. Nucleic Acids Res. 46 (W1), W95–W101. doi: 10.1093/nar/gky418
Zhang G., Bai J., Tebbe C. C., Zhao Q., Jia J., Wang W., et al. (2021). Salinity controls soil microbial community structure and function in coastal estuarine wetlands. Environ. Microbiol. 23 (2), 1020–1037. doi: 10.1111/1462-2920.15281
Zhang Z., Guo Y., Guo L., Hu F., Zhao Y., Jin C., et al. (2020). Elucidating salinity adaptation and shock loading on denitrification performance: focusing on microbial community shift and carbon source evaluation. Bioresour. Technol. 305, 123030. doi: 10.1016/j.biortech.2020.123030
Zhang H., Zheng S., Ding J., Wang O., Liu F. (2017). Spatial variation in bacterial community in natural wetland-river-sea ecosystems. J. Basic Microbiol. 57 (6), 536–546. doi: 10.1002/jobm.201700041
Keywords: tidal flats, microbial diversity, ammonium, mudflat, metagenome, Beibu Gulf
Citation: Ye Y-L, Ma K-J, Fu Y-H, Wu Z-C, Fu G-Y, Sun C and Xu X-W (2023) The heterogeneity of microbial diversity and its drivers in two types of sediments from tidal flats in Beibu Gulf, China. Front. Mar. Sci. 10:1256393. doi: 10.3389/fmars.2023.1256393
Received: 10 July 2023; Accepted: 08 September 2023;
Published: 27 September 2023.
Edited by:
Zhongzheng Yan, East China Normal University, ChinaReviewed by:
Bao-zhu Fang, Chinese Academy of Sciences (CAS), ChinaZhaobin Huang, Quanzhou Normal University, China
Da Huo, Chinese Academy of Sciences (CAS), China
Copyright © 2023 Ye, Ma, Fu, Wu, Fu, Sun and Xu. This is an open-access article distributed under the terms of the Creative Commons Attribution License (CC BY). The use, distribution or reproduction in other forums is permitted, provided the original author(s) and the copyright owner(s) are credited and that the original publication in this journal is cited, in accordance with accepted academic practice. No use, distribution or reproduction is permitted which does not comply with these terms.
*Correspondence: Xue-Wei Xu, eHV4d0BzaW8ub3JnLmNu; Cong Sun, bWljaGFlbF9zY0BzaW5hLmNvbQ==