- 1Observatoire Pelagis, UAR 3462, CNRS - La Rochelle Université, La Rochelle, France
- 2Share the Ocean, Larmor-Baden, France
- 3Biology Department and ECOMARE and CESAM, University of Aveiro, Aveiro, Portugal
- 4AZTI, Marine Research, Basque Research and Technology Alliance (BRTA), Pasaia, Spain
- 5Instituto Español de Oceanografía - Consejo Superior de Investigaciones Científicas (IEO-CSIC), Centro Oceanográfico de Vigo, Vigo, Spain
- 6Centre d’Etudes Biologiques de Chizé - La Rochelle, UMR 7372 CNRS – La Rochelle Université, Villiers-en-Bois, France
As apex predators, cetaceans play an essential ecological role in marine ecosystems. Fluctuations in the abundance of these top predators linked to human activities can have detrimental consequences for the entire ecosystem. Cetaceans face numerous anthropogenic threats that can have both short and long-term effects. To ensure their conservation, it is necessary to identify changes in seasonal distributions at small and large scales. We aimed to model the seasonal distribution of the most abundant cetacean species in the European Atlantic waters and the Mediterranean Sea by assembling datasets collected over 16 years of surveys using a standardised line-transect protocol. Data were homogenised, detection functions fitted and effective strip widths estimated. We extracted environmental variables integrated over the water column, which we transformed using a principal component analysis (PCA). The dimensions of the PCA were then integrated as explanatory variables in a generalised additive model, taking seasonal and spatial effects into account to predict the seasonal cetacean distribution. We were able to highlight changes in the spatial distribution and/or density of cetaceans throughout the year at a large scale, considering environmental extrapolation areas to predict where environmental variables were sampled during the surveys. For minke (Balaenoptera acutorostrata) and fin (B. physalus) whales, densities varied over the seasons but not the distribution, suggesting a seasonal migration outside the survey areas. For common dolphins (Delphinus delphis), bottlenose dolphins (Tursiops truncatus) and harbour porpoises (Phocoena phocoena), densities varied little but distributions did over the seasons. Finally, pilot whales (Globicephala spp), Risso’s (Grampus griseus) and striped (Stenella coeruleoalba) dolphins showed little seasonal variation in their distribution. Using monthly dynamic environmental variables at depth and PCA dimensions in habitat models, we produced maps of the seasonal distribution of cetaceans in the Mediterranean Sea and the European Atlantic waters to help fill gaps in our knowledge of cetacean distribution.
1 Introduction
As apex predators, cetaceans play an essential ecological role in marine ecosystems (Pirotta et al., 2019). They participate in transferring nutrients and biomass between the deep and surface environments through their excretions. They can sequester carbon and promote primary production (plankton) by releasing their excreta to the surface (Doughty et al., 2016). They are also involved in the top-down regulation of lower trophic levels (Hunt and McKinnell, 2006). A reduction in the abundance of cetaceans leads to an increase in the abundance of mesopredators and invertebrate predators, and an increase in this abundance reduces prey abundances (Baum and Worm, 2009). Variations in the abundance of these top predators linked to human activities can therefore have detrimental consequences for the whole ecosystem. Cetaceans face numerous anthropogenic threats that can have short-term effects by directly killing individuals (bycatch, Peltier et al., 2016, ship strikes, Schoeman et al., 2020) and long-term effects by reducing the fitness or physical condition of species (noise and chemical pollution, Murphy et al., 2018; Miller et al., 2022, ingestion of plastic debris, Unger et al., 2016).
Variations in the distribution of cetaceans expose them to different combinations of pressures, that should be considered when assessing their conservation status. It is, therefore, necessary to monitor cetacean populations and to have a good knowledge of their habitat use but also to identify changes in seasonal distribution at small and large scales, as some human activities have specific seasonal temporalities (e.g., fishing seasons, summer tourist activities). This knowledge can be used to identify high-risk areas and to implement appropriate mitigation measures (Breen et al., 2017; Pennino et al., 2017).
To identify and/or predict species geographical distributions, scientists commonly use species distribution models (SDMs), which relate species observation data to the ecological or spatial characteristics of the environment (Elith and Leathwick, 2009). By analysing the conditions under which a species is (or is not) observed, models allow the similarity of conditions at each site to be estimated and the potential species distribution to be predicted (Franklin, 2010). Surface environmental variables are commonly used as proxies for prey distribution in SDMs to explain cetacean distributions (Redfern et al., 2006; Elith and Leathwick, 2009; Roberts et al., 2016; Redfern et al., 2017; Rogan et al., 2017), and recently variables that characterise the water column have emerged as relevant factors in modelling species distributions (Becker et al., 2016; Brodie et al., 2018; Waggitt et al., 2020; Virgili et al., 2022). Dynamic variables that characterise the deep ocean layers are likely to have a greater influence on the deep prey fields on which cetaceans feed than surface parameters alone.
The line-transect distance sampling method, which involves counting animals using a moving platform along linear transects to estimate species densities (Buckland et al., 2001), is often used to collect sighting data implemented in SDMs. At fine scales, surveys typically use a single platform (a boat or aircraft), but at large scales, multiple platforms are often used to cover the entire area (one or more boats and/or one or more aircraft), so a standardised protocol must be implemented to ensure homogeneous and consistent coverage.
Unfortunately, due to financial and logistical constraints, large-scale surveys are rare (e.g., SCANS surveys in European waters in 2005, 2016, 2022, Hammond et al., 2013; Rogan et al., 2017; Hammond et al., 2021; Gilles et al., 2023) and generally conducted in summer (optimal season), whereas smaller surveys can be conducted over several seasons. Consequently, to study seasonal changes in species distributions at large scale, it is necessary to assemble datasets collected during various surveys at different spatial and temporal scales. This collation requires the homogenisation of data collected by different platforms (different equipment, observation heights, data collection software, etc) and the application of standardised protocols facilitates data processing.
Recently, data assembling has been useful for modelling species distributions in the North Atlantic Ocean (e.g., Roberts et al., 2016; Waggitt et al., 2020; Virgili et al., 2019). In particular, Roberts et al. (2016) aggregated 23 years (from 1992 to 2014) of aerial and ship-based cetacean surveys to model the cetacean distribution in the US Atlantic and Gulf of Mexico. Waggitt et al. (2020) aggregated aerial and ship surveys from 1980 to 2018 to model the cetacean distribution in the Northeast Atlantic Ocean. In our study, we assembled datasets collected over 16 years of surveys (from 2005 to 2021) using a standardised line-transect protocol to model the seasonal distribution of the most abundant cetacean species in the European Atlantic waters and the Mediterranean Sea. In the European Atlantic waters, we have extended the prediction zone, particularly in the south, and used more recent data than Waggitt et al. (2020), which could strengthen the results of their study by using both overlapping and different datasets and a different methodology. In the Mediterranean Sea, most of the data came from the ASI survey, we have not only used the aerial component as in Cañadas et al. (2023), but we have also aggregated data from the boat component and aerial and boat data collected off the French coast. We used monthly dynamic environmental variables that describe the water column to consider mechanisms that influence species distribution at depth. Following the methodology of Lambert et al. (2018), we performed a Principal Component Analysis (PCA) to describe the environment as a whole from the monthly dynamic environmental variables and integrated the PCA dimensions in Generalised Additive Models (GAMs) to predict the seasonal cetacean distributions. Highlighting seasonal changes or consistency in species distributions could help establish marine protected areas and Important Marine Mammal Areas, or manage human activities where species are concentrated during certain seasons.
2 Materials and methods
2.1 Study areas
This study focuses on two study areas, the European Atlantic waters (from 34° to 70°N and from 15°W to 17°E) and the Mediterranean Sea (all the Mediterranean basin from 30° to 46°N and from 6°W to 37°E; Figure 1).
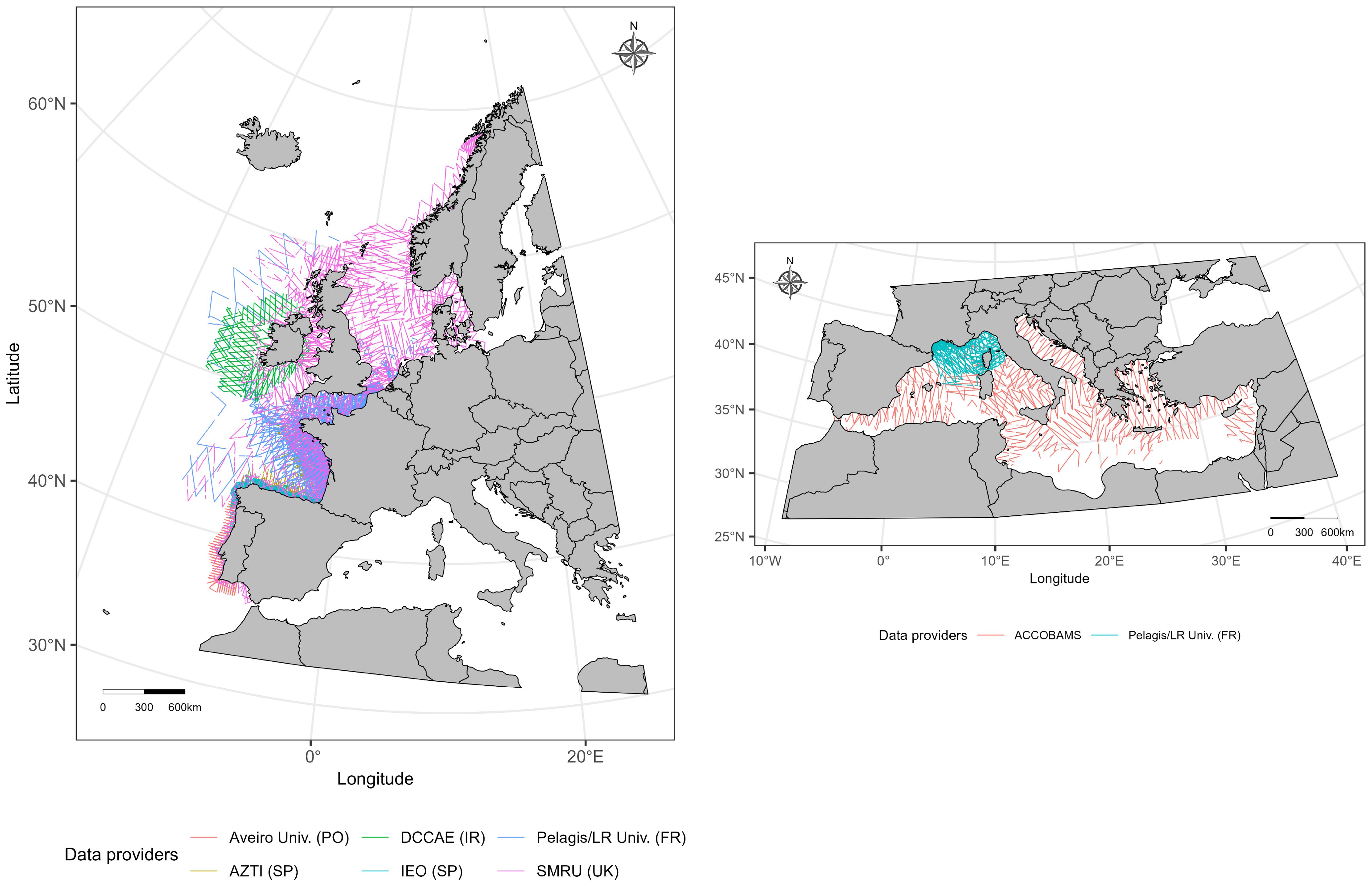
Figure 1. Total observation effort in the European Atlantic waters (left) and the Mediterranean Sea (right). The data comes from seven different organisations. Aveiro Univ. (PO): University of Aveiro (Portugal), DCCAE (IR): Department of Communications, Climate Action and Environment (Ireland), Pelagis/LR Univ. (FR): Pelagis/La Rochelle University (France), AZTI (SP): AZTI foundation (Spain), IEO (SP): Spanish Institute of Oceanography (Spain), SMRU (UK): Sea Mammal Research Unit (United Kingdom), ACCOBAMS: Agreement on the Conservation of Cetaceans of the Black Sea, Mediterranean Sea and contiguous Atlantic area. Survey effort by season and region is shown in Supplementary Figures S1, S2. Coordinate reference system: ETRS89-extended/LAEA Europe - EPSG:3035.
European Atlantic waters are characterised by a complex hydrography, with water masses flowing mainly from west to east, driven by the northern and southern branches of the North Atlantic Drift (Marzocchi et al., 2015). On the continental shelf, currents are mainly driven by tides and wind, but the main flow is from south to north. The area is also characterised by the presence of heterogeneous habitats, with shallow continental shelves along the European coast, steep continental slopes and deep canyons down to 5,000 m. The average temperature varies between 7 and 15°C at the surface and between 5.5 and 7.5°C at 1,000 m. Productivity is variable, it increases from south to north, with nutrient-rich deep waters rising to the surface in certain areas, such as off Galicia and west of Ireland (Raine, 1990; Bode et al., 2009).
The Mediterranean Sea is a semi-enclosed sea with a relatively small continental shelf. The Strait of Sicily connects the western and eastern basins. Most surface waters come from the Atlantic Ocean via the Strait of Gibraltar and flow in a cyclonic direction along the continental shelf at average depths between 0 and 200 m. Below, between 200 and 600 m depth, the intermediate Levantine water flows, which is the warmest and saltiest water mass within the Mediterranean Sea. This intermediate Levantine water is involved in the formation of all deep waters below 600 m (deep waters of the Adriatic, Aegean, Tyrrhenian and western Mediterranean Sea; Millot and Taupier-Letage, 2005). The average depth in the Mediterranean Sea is around 1,500 m and the maximum depth is around 5,100 m in the Ionian Sea. The water surface temperature varies from 10 to 15°C in winter and from 21 to 30°C in summer. The temperature hovers around 12°C at 1,000 m. It is one of the saltiest seas. The Mediterranean Sea is an oligotrophic sea except at the mouths of major rivers.
2.2 Data collation
To cover as much of the study areas as possible and to increase the amount of data that can be used in the habitat models, effort and observation data from different organisations were collated, in a non-exhaustive manner (following Virgili et al., 2019). The data were collected from surveys conducted by aircraft and boats between 2005 and 2021, i.e., 16 years of data, in spring (March to May coverage), summer (June to August), autumn (September to November) and winter (December to February). As a minimum, all the datasets had to record the Beaufort sea state along the transects. A single common dataset was created by aggregating all survey datasets with standardised units and formats. Effort data were linearised and divided into segments of approximately 10 km. Cetacean sightings were collected using a line-transect distance sampling protocol (Buckland et al., 2001). For each sighting, the species, the number of individuals, the observation conditions and the perpendicular distances from the transects were recorded. Sightings were assigned to the effort segments during which they were recorded.
The total distance covered in good conditions was approximately 422,400 km in the European Atlantic waters and 79,600 km in the Mediterranean Sea (Figure 1; Supplementary Table S1). In the European Atlantic waters, about 52% of the total effort was performed by aircraft and 48% by boat and in the Mediterranean Sea, 86% was performed by aircraft and 14% by boat (Table 1; Supplementary Figure S1). Observation height/altitude varied between surveys (Table 1). Most aircraft transects in both regions were conducted at altitudes between 150 and 250 m and speeds around 150 km/h (speed was not always provided). In the Mediterranean Sea, most boat transects were conducted with a platform at 5 m, while in the European Atlantic waters, the observation height was more variable, with the 16 m platform being the most common (speed was not always provided). The observation effort was not homogeneous between the different seasons. In the European Atlantic waters, 38% of the effort was performed in summer, 28% in spring, 18% in autumn and 16% in winter (Supplementary Figure S1). In the Mediterranean Sea, autumn data (4% of the effort) were combined with summer data (96% of the effort).
Nine species or species groups of cetaceans were studied in the European Atlantic waters and four were studied in the Mediterranean Sea (Table 2). The number of sightings for the other species was insufficient to allow robust modelling of their distribution. Long- (Globicephala melas) and short- (G. macrorhynchus) finned pilot whales are encountered in the area (Sabatier et al., 2015) but difficult to identify at the species level, especially from the air; thus, they were grouped. Atlantic white-sided (Lagenorhynchus acutus) and white-beaked (L. albirostris) dolphins (hereafter called Lagenorhynchus) were also grouped together, mainly because the number of sightings was too low for each species and partly because Lagenorhynchus species are difficult to distinguish from the air.
The number of sightings was not consistent between seasons for all species (Table 2). The difference was particularly marked for certain species. Virgili et al. (2018) recommend a minimum of 50 sightings to fit habitat models, a number that should evolve depending on the size of the study area. To ensure that this number was reached, for some species, data from summer and autumn, and from winter and spring, were combined in the European Atlantic waters. Before combining the sighting data, we checked that there were no significant differences between the combined seasons in terms of sighting distributions (visually on the maps), but also in terms of group sizes (using Student’s t-tests). Analyses of variance were used to group the seasons with the closest medians and ranges for the environmental variables. This was done for fin whales (Balaenoptera physalus), minke whales (B. acutorostrata) and Risso’s dolphins (Grampus griseus). For Lagenorhynchus, the number of sightings was sufficient only in summer, so only the summer distribution was modelled. For striped dolphins (Stenella coeruleoalba), the number of sightings in winter was too low, so we did not model the winter distribution. The study did not include observations where common dolphins could not be distinguished from striped dolphins. For the group of pilot whales, the number of sightings in winter was low, but we still modelled their distribution because, as pilot whales are generally concentrated along continental slopes, their distribution is easier to model and the number of sightings may be lower. In the Mediterranean Sea, as with effort data, summer and autumn data were combined for all species as there was insufficient effort in autumn to allow modelling.
2.3 Detection functions and effective strip widths
To fit the detection functions and estimate the Effective Strip Widths (ESWs), we pooled the data from the two regions (European Atlantic waters and Mediterranean Sea). For each species, perpendicular distances between the sightings and the transects were used to fit detection functions. Platform and observation height/altitude influence cetacean detection, so we defined observation height classes for which detection functions were fitted. We defined three classes: two classes for boat platforms (5-10 m and 10-20 m) and one class for the aircraft platform. When the number of sightings in the boat surveys was insufficient, we pooled data from the two boat classes. The speed of the platform also affects detection, but this information was not available for all surveys, so we have not included it in the analyses.
For each species or species group and each observation height class, detection functions were fitted with or without the Beaufort sea state as a covariate using a multiple covariate distance sampling (MCDS) method (Marques and Buckland, 2003). The Beaufort sea state was the only detection variable common to all surveys, so it was the only detection variable tested in the models. Detection functions were fitted using the ‘dfuncEstim’ function of the ‘Rdistance’ R-package (McDonald et al., 2019) and half-normal or hazard rate distributions. The best models were selected using the Akaike information criterion (AIC; Buckland et al., 2015). Once detection functions were fitted, we estimated ESWs (Buckland et al., 2001).
2.4 Habitat-based density modelling
2.4.1 Environmental variables
To determine whether the cetacean distribution was related to surface or water column oceanographic processes, several depth classes were defined to extract environmental variables. These classes were defined according to the distribution of water masses in the European Atlantic waters and the Mediterranean Sea.
In the European Atlantic waters, the first class considered was the surface. The cetacean distribution may be related to processes at the surface, or these processes may influence those at depth. The second class was defined as the zone extending vertically from 0 to 600 m. The waters between 0 and 600 m have the characteristics of the central waters of the North Atlantic Ocean. The third class extended from 600 to 1,500 m. In the Bay of Biscay, between 600 and 1,500 m, the Mediterranean waters flow through Gibraltar. The fourth class extended from 1,500 to 3,500 m and corresponded to the deep waters of the Northeast Atlantic Ocean. The last class extended from 3,500 m to the bottom of the sea and corresponded to the Antarctic waters (Koutsikopoulos and Le Cann, 1996). This last class is inaccessible to cetaceans but can influence the upper layers by upwelling cold water.
In the Mediterranean Sea, four depth classes were considered: “surface”, “0-200 m”, “200-600 m” and “600 m-bottom”. Waters between 0 and 200 m depth come from the Atlantic Ocean and enter the Mediterranean Sea through the Strait of Gibraltar. Below, there is the intermediate Levantine water, averaging between 200 and 600 m, which is the warmest and saltiest in the Mediterranean Sea. Finally, below 600 m, the deep waters circulate, partly formed by the Levantine intermediate water (Millot and Taupier-Letage, 2005).
To model species distributions, we extracted static variables, which did not vary with time and were independent of depth classes, and dynamic variables, which varied with time, for each of the defined depth classes (Table 3).
For static variables, we extracted the bathymetry at a 0.02° resolution (cell size from 1.4 to 4.2 km2, https://www.emodnet-bathymetry.eu/) and then calculated the slope (inclination of the bottom) and roughness (difference between the maximum and minimum depths of the pixels surrounding the central pixel) from it (function ‘terrain’ from ‘raster’ R-package, Hijmans, 2023). The cells were then aggregated to match the cell size of the dynamic variables, i.e., 0.083° (cell size from 24 to 73 km2) in the European Atlantic waters and 0.042° in the Mediterranean Sea (cell size from 14.9 to 18.5 km2).
For dynamic variables, we extracted monthly variables for each year between 2000 and 2021. We obtained water temperature, current vectors, salinity, chlorophyll-a concentration and net primary production for each depth class from the Copernicus website (https://data.marine.copernicus.eu/products). We also extracted sea surface height, bottom temperature and mixed layer depth. The spatial resolution of the variables was 0.083° in the European Atlantic waters and 0.042° in the Mediterranean Sea, except for chlorophyll-a concentration and net primary production, which was 0.25° (cell size from 221 to 661 km2, not available at finer resolution at a large scale). From these variables, temperature gradients, eddy kinetic energy, chlorophyll-a concentration gradients and net primary production gradients were calculated (function ‘getGradients’ from ‘grec’ R-package, Lau-Medrano, 2024). For each dynamic variable, monthly climatological averages were calculated for each month over the 2000-2021 period and associated with the effort segments and prediction grids.
2.4.2 Principal component analysis
Based on Lambert et al. (2018), instead of using environmental variables directly in the SDMs, we transformed them using a PCA (Jongman et al., 1995). This technique allows transforming correlated variables into new variables (or dimensions) that are decorrelated with each other and summarising information by reducing the number of variables (thus limiting computational time). The environmental space is characterised by different PCA dimensions that aggregate several environmental variables. A PCA aims to reduce the number of variables while retaining as much information as possible. Each dimension explains a percentage of the total variability. To avoid losing too much information, we chose a number of dimensions that explained at least 70% of the total variability, i.e., five dimensions, which also corresponded to a slower decrease in the percentage of inertia. We finally reduced the 29 environmental variables to five dimensions.
2.4.3 Models
In each study area, habitat models were fitted for each species or species group using GAMs (Hastie and Tibshirani, 1987). These models establish functional relationships between the number of individuals observed and environmental variables, in this case, the dimensions of the PCA. We included each of the five PCA dimensions in the model, specifying a season factor for each dimension. In this way, we obtained one curve per season for each dimension, curves that may be different if the environment chosen by the species varies over the year or very similar if the relationship between the species and its environment varies little over time.
We also included a spatial effect in the model, as a predictor with an interaction between latitude and longitude, to account for variability that would not be explained by the variables. This spatial effect would represent some other mechanisms not captured by the environmental variables included in the modelling approach (Paradinas et al., 2023).
Models were fitted to the number of individuals per segment with a Tweedie distribution to account for overdispersion in the count data (Foster and Bravington, 2013). We set a maximum of three inflexion points (i.e., four degrees of freedom), which allows the model to fit the complexity of the curve to the data while avoiding overfitting. The number of individuals observed per sampling unit was corrected by the area sampled by including an offset in the model, calculated as 2 × segment length × ESW. The ‘mgcv’ R-package (Wood, 2017) was used to fit the GAMs. The explained deviance, expressed in %, reflects the goodness of fit of the model; the higher the deviance, the better the model.
2.4.4 Predictions
After fitting the models, we predicted seasonal species distributions in the European Atlantic waters and the Mediterranean Sea (0.1x0.1° spatial resolution, cell sizes between 45 and 100 km2 in the European Atlantic waters and between 87 and 106 km² in the Mediterranean Sea, depending on latitude). When there was sufficient data, we made a prediction for the spring, summer, autumn and winter seasons. When the amount of data was insufficient, we combined summer and autumn data and winter and spring data. In the European Atlantic waters, the prediction zone was defined by the maximum coverage of the surveys and applied to all seasons. The prediction zone extended from 35°N to 70°N and from 20°W to 10°E. In the Mediterranean Sea, the prediction zone in summer/autumn extended over the entire basin.
To quantify the uncertainty associated with the predictions, we calculated the coefficient of variation (CV) associated with each cell in the prediction grid, where CV = mean/standard deviation. We transformed the CV values into classes corresponding to the percentiles.
2.4.5 Gap analysis
As the prediction zones were not fully covered by the surveys (Figure 1), we performed a gap analysis of the environmental space not covered during the surveys to identify areas where extrapolation would not be representative of the sampled environmental space (Bouchet et al., 2020). The aim was to spatially extrapolate the predictions only in areas where the environmental variables were within the range of values of the environmental variables sampled during the surveys. The predictions (or extrapolations) made in gap areas are considered less reliable because the model had no information on the species presence in these environments. Analyses were performed by season in both study areas using the ‘dsmextra’ R-package (Bouchet et al., 2019). Finally, unreliable extrapolation areas were identified by black colours in the prediction maps, and therefore, no prediction was provided in these extrapolation areas. The extrapolation areas depended on the distribution of effort and not on the species, so they were the same for all species but varied by season.
3 Results
3.1 Species presence
In the European Atlantic waters, common dolphins (Delphinus delphis), bottlenose dolphins (Tursiops truncatus), fin whales, Risso’s dolphins, striped dolphins and pilot whales were recorded mainly in the Bay of Biscay, off the Portuguese coast and west of Ireland (Supplementary Figure S3). Lagenorhynchus were observed in the northern North Sea, northern Scotland and northern and western Ireland. Harbour porpoises (Phocoena phocoena) and minke whales were observed everywhere except in the oceanic waters of the Bay of Biscay. In the Mediterranean Sea, all species were mainly observed in the western Mediterranean Sea, with virtually no sighting data in the south and east of the Mediterranean Sea (Supplementary Figure S4). Striped and bottlenose dolphins were also observed in the Aegean and Adriatic Seas, and a few sightings of Risso’s dolphins were recorded in these two areas. Sightings of fin and pilot whales were mainly restricted to the Liguro-Provençal basin.
3.2 Detection functions and ESWs
The platform had a significant effect on the detection probability, with shorter distances observed in aircraft surveys than in boat surveys (Supplementary Figure S5). Detection probabilities varied by species, with larger animals being more visible at greater distances, except for minke whales, which are relatively cryptic. Detection functions were all fitted with the Beaufort sea state as a detection variable, except for minke whales and Lagenorhynchus, for which there were not enough sightings for all sea state classes. The detection probability decreased with increasing Beaufort sea state. It was therefore more difficult to detect animals when sea conditions deteriorated. This effect was more or less pronounced depending on the species; the closer the curves were, the less effect the Beaufort had on detection. For fin whales, for example, detection was relatively unaffected by the Beaufort sea state in aircraft surveys, but the effect was more pronounced in boat surveys. For bottlenose dolphins, the Beaufort sea state had a clear effect on detection for boats whose observation platform was under 10 m above the sea surface.
These detection functions were used to estimate ESWs (Figure 2). In general, ESWs were lower for aircraft than for boats because, in boat surveys, observations are made towards the horizon, whereas in aircraft surveys observations are performed below the platform. As the Beaufort sea state increased, the ESWs decreased. Small species such as the harbour porpoise, which is difficult to observe, had lower ESWs (less than 0.5 km by boat) than larger species such as the fin whale (up to 3.8 km by boat).
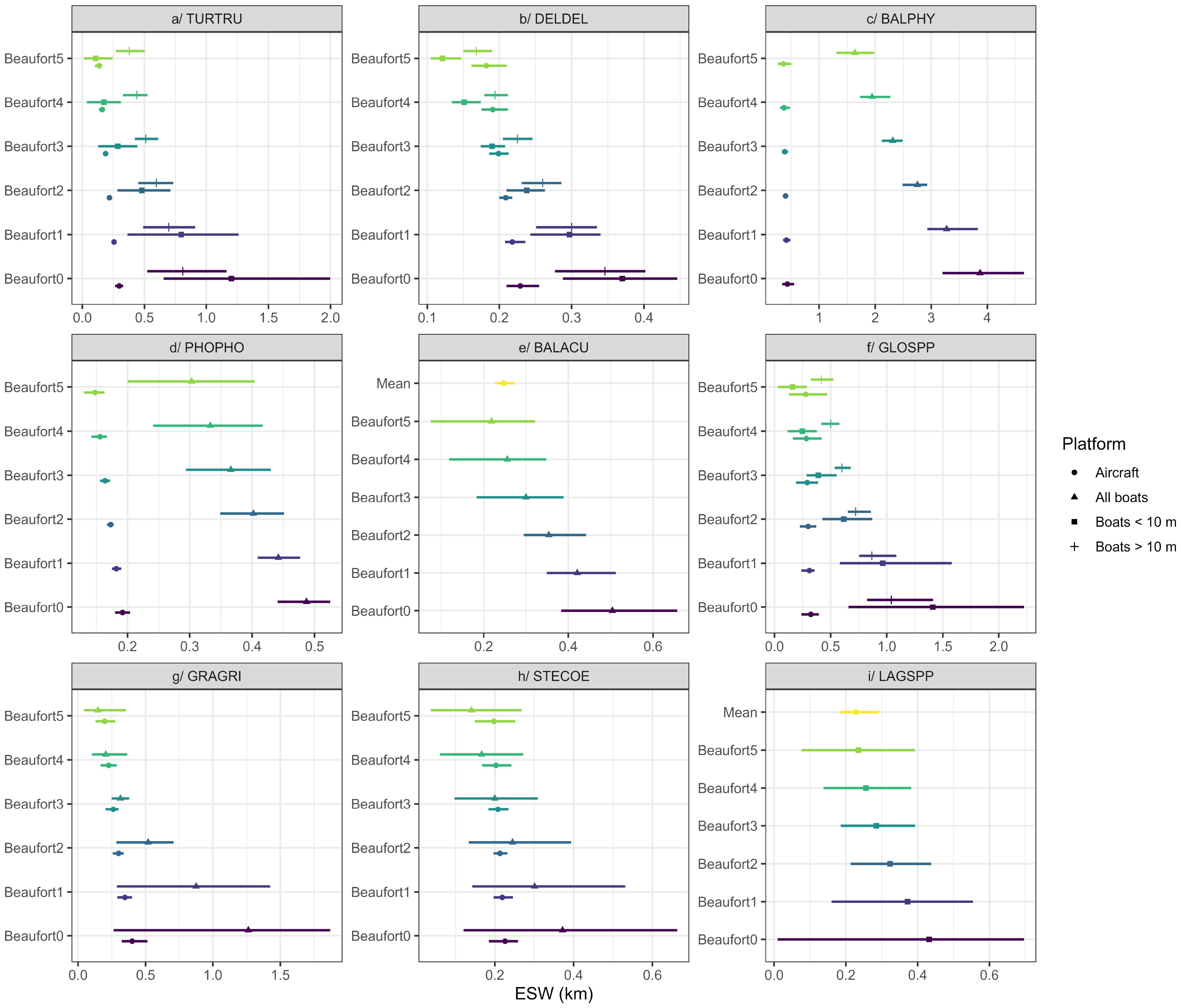
Figure 2. Effective strip widths (ESWs) estimated for each species and each Beaufort sea state class. Symbols represent mean values and horizontal bars represent confidence intervals. Beaufort sea state classes are represented from 0 (excellent conditions) to 5 (poor conditions). Large uncertainties reflect a low number of data for the sea state under consideration. This is particularly true for low Beaufort sea states in boat surveys, as few observations have been made in these conditions. Aircraft surveys are usually conducted in very good conditions, which reduces the confidence intervals. Mean: estimated mean value for all Beaufort sea states. Species codes are available in Table 2.
3.3 Characterisation of the environment using PCA
Each dimension of the PCA was driven by different variables (Figure 3). In the European Atlantic waters, dimension 1 was mainly driven by variables characterising the deep layers of the water column, such as depth, temperature, temperature gradients and currents below 600 m; dimension 2, by surface and subsurface currents and eddies above 600 m; dimension 3, by surface and subsurface temperatures above 600 m; dimension 4, by primary production variables (chlorophyll-a concentration and net primary production) above 600 m; and dimension 5, by topography, in this case slope and roughness.
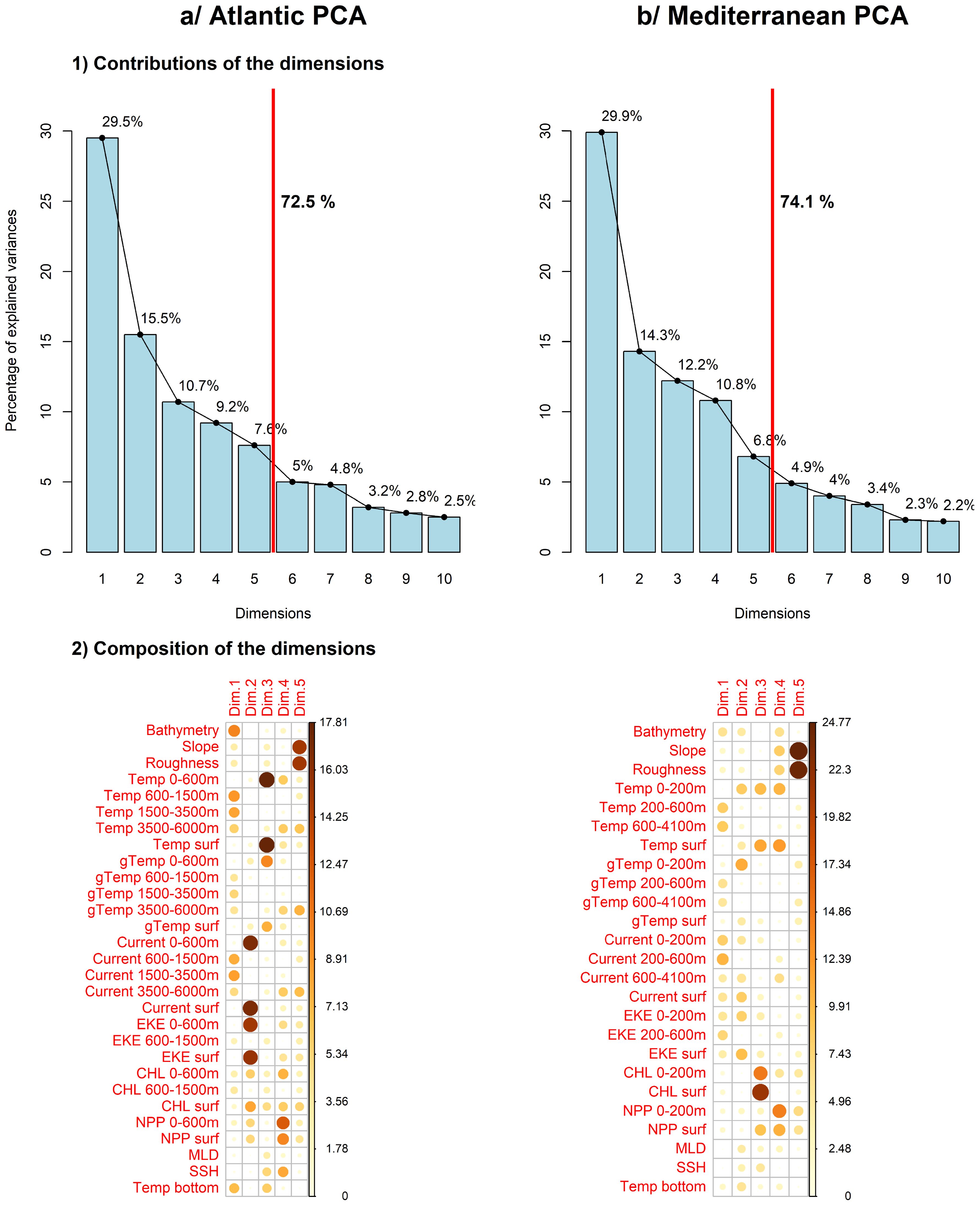
Figure 3. Results of the principal component analysis (PCA) in the European Atlantic waters (A) and the Mediterranean Sea (B). 1) Histograms represent the variance explained by each PCA dimension. We chose to keep the dimensions that explained at least 70% of the variance, i.e., 5 dimensions. 2) The correlation plots represent the variables that drove each dimension. The larger and darker the circle, the more important the variable. Dim: dimension, Temp: temperature, gTemp: temperature gradients, EKE: eddy kinetic energy, CHL: chlorophyll-a concentration, NPP: net primary production, MLD: mixed layer depth, SSH: water surface height, Temp bottom: bottom temperature, surf: surface.
In the Mediterranean Sea, dimension 1 was mainly driven by dynamic variables characterising the deep layers of the water column, such as temperature, currents and eddies below 200 m, dimension 2, by dynamic surface variables (temperature, temperature gradient, currents, eddy kinetic energy), dimension 3, by surface and subsurface chlorophyll-a concentration, dimension 4, by surface and subsurface net primary production and dimension 5, by slope and roughness, as in the European Atlantic waters.
3.4 Models and predictions
Extrapolation areas were the same for all species but varied by season. In the European Atlantic waters, extrapolation areas were larger in spring and winter, especially in the north of the prediction zone and off the Bay of Biscay and the Iberian Peninsula. In summer, a large part of the area was sampled during the surveys, so the extrapolation areas were very limited. In autumn, only the northern part of the prediction zone was considered as an extrapolation, so the surveys were sufficient to sample the range of the environmental variables. In the Mediterranean Sea, the extrapolation areas were very limited because almost the entire Mediterranean Sea was sampled in summer.
All prediction maps are available in the PrediWhales application (http://peladev.univ-lr.fr:3838/pelagis/PrediWhales_v2/).
3.4.1 European Atlantic waters
3.4.1.1 Bottlenose dolphin
The bottlenose dolphin model explained 26.7% of the deviance (Figure 4). All dimensions were significant. The functional relationships between density and explanatory variables varied greatly between seasons. For dimensions 1 (currents and bottom temperatures) and 3 (surface temperatures), the relationships were reversed in autumn compared to the other seasons, positive for dimension 1 and negative for dimension 3. The relationship with surface and subsurface currents and eddies (dimension 2) was strong and negative in spring compared to the other seasons. The biological variables (chlorophyll-a and net primary production, dimension 4) had a stronger negative effect in autumn than in the other seasons. The relationships with the static variables (slope and roughness, dimension 5) were similar and positive in summer and spring and negative in winter and autumn. These relationships resulted in a change in the distribution of bottlenose dolphins over the year, particularly in autumn (Figure 5). In spring and summer, the highest densities were predicted along the continental slope from the Spanish coast to western Ireland and to a lesser extent on the continental shelves. In winter, the highest densities were predicted on the continental shelves from Spain to western Ireland. In autumn, the highest densities were predicted beyond the continental slope, mostly in western Ireland. Bottlenose dolphins were predicted to avoid the shelf edge in autumn due to the low number of sightings in this area. The highest uncertainties were estimated in the northern part of the study area (beyond 55°N), but also off the Bay of Biscay and off the Portuguese coast, due to the low number of sightings in the southern part of the study area (Supplementary Figure S6).
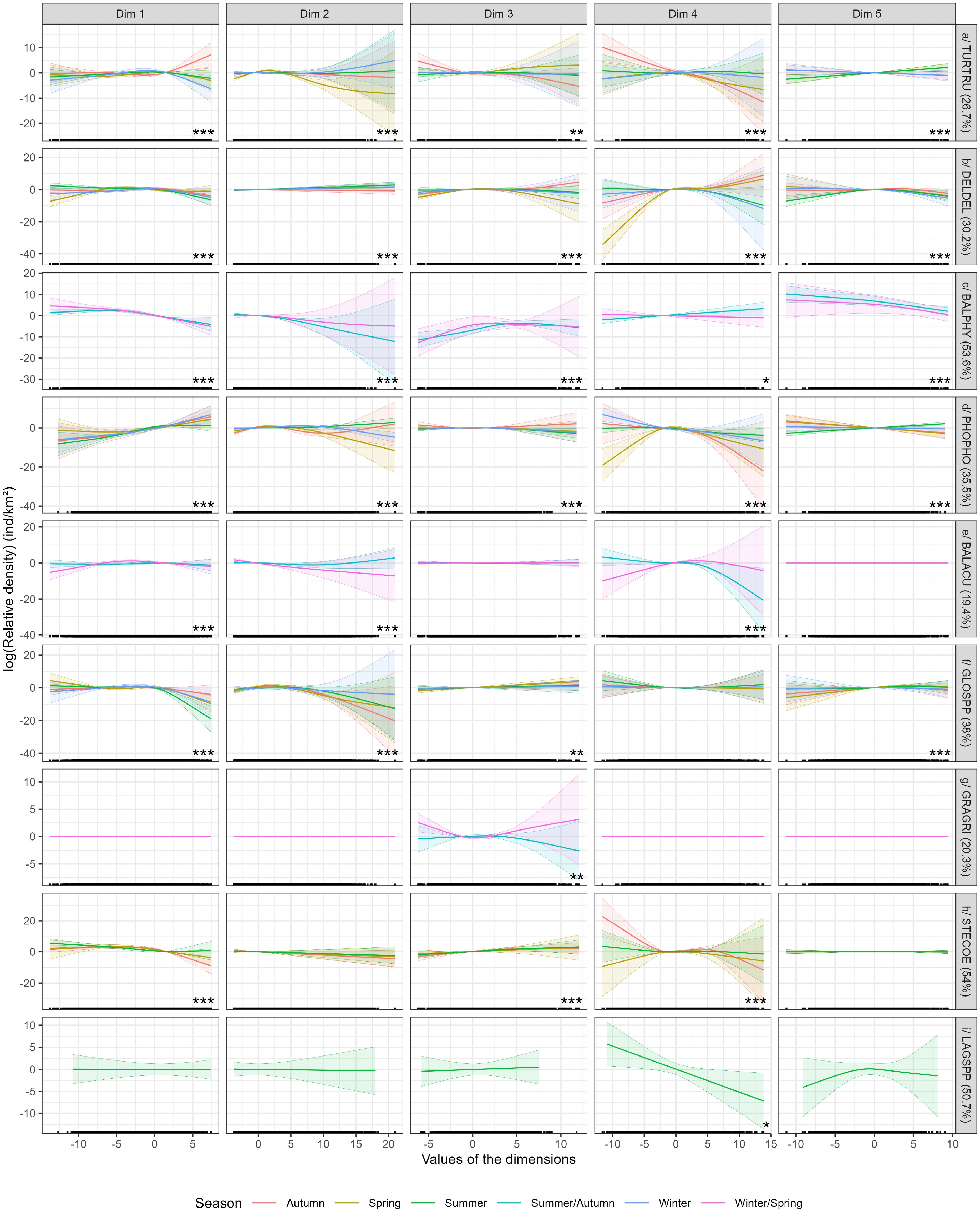
Figure 4. Relationships obtained for each model in the European Atlantic waters. The curves represent the relationships obtained between cetacean density and explanatory variables (the five dimensions of the PCA) for each season according to the different dimensions of the PCA (Figure 3). Explained deviances of the models fitted for each species are shown in percentages between brackets. The stars (*) represent the significance of the variable; the higher the number, the more significant the variable. If there is no star, the variable is not significant. Spring: March to May, Summer: June to August, Autumn: September to November, Winter: December to February.
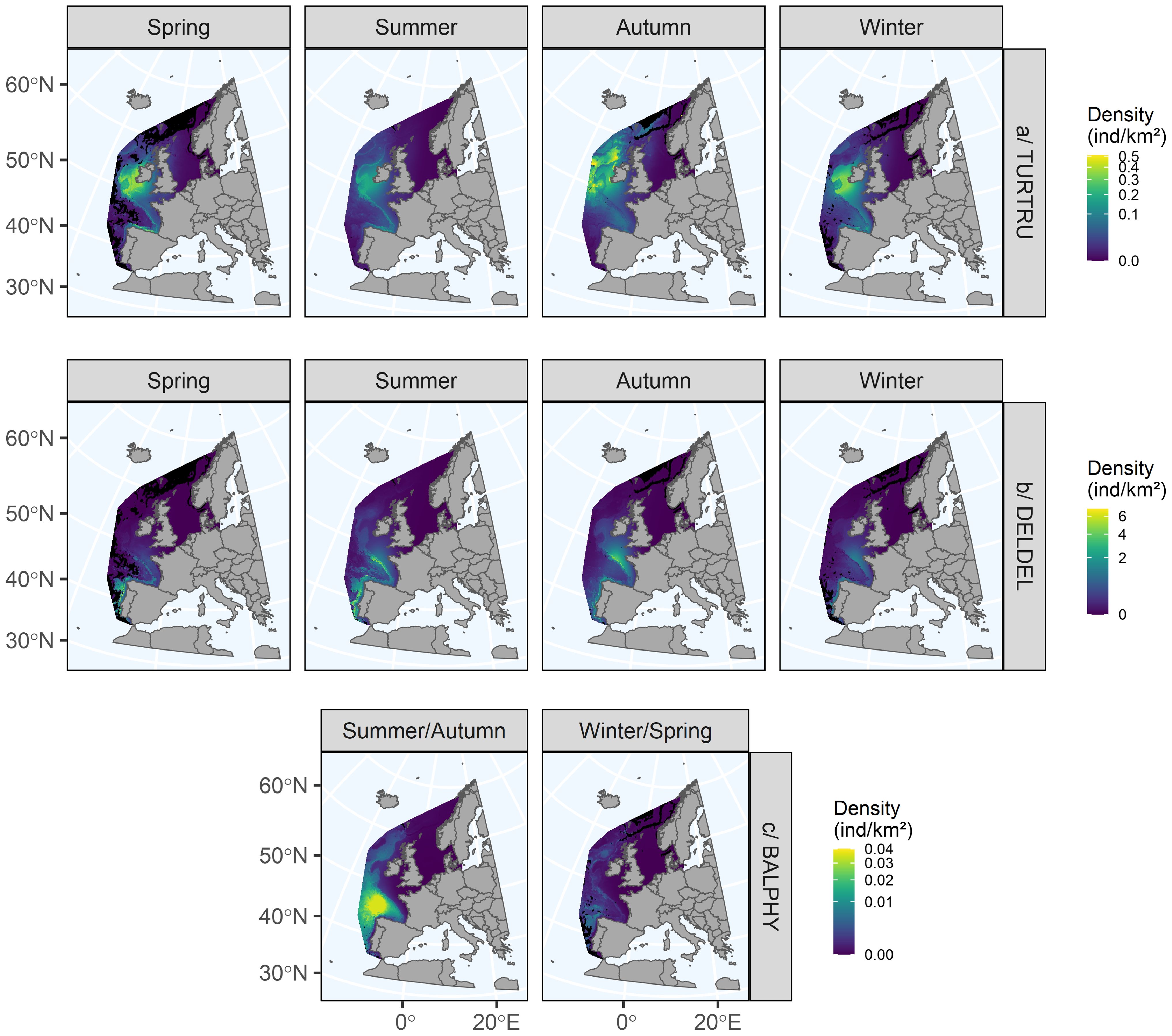
Figure 5. Seasonal distributions predicted for bottlenose dolphins (a), common dolphins (b) and fin whales (c) in the European Atlantic waters. Black cells represent extrapolation areas where the environmental space did not correspond to the environmental space sampled during the surveys. Uncertainty maps are available in Supplementary Figure S6. ind/km2: individuals per square kilometre, Spring: March to May, Summer: June to August, Autumn: September to November, Winter: December to February.
3.4.1.2 Common dolphin
The model obtained for the common dolphin explained 30.2% of the deviance (Figure 4). All dimensions were significant. Although this was more pronounced for certain dimensions, the relationships differed between seasons. This suggested that animals did not have the same habitat preferences throughout the year. In spring, the relationship with bottom currents and temperatures (dimension 1) was reversed compared to the other seasons, but all were generally weak. Relationships with surface and subsurface currents and eddies (dimension 2) were positive, weak and similar between the four seasons. The relationship with surface and subsurface temperatures (dimension 3) was reversed in autumn compared to the other seasons. The relationships with chlorophyll-a concentration and primary production (dimension 4) were similar and negative in summer and winter and positive in spring and autumn, with strong variations. The relationships with slope and roughness (dimension 5) were partly different in summer compared to the other seasons, but all were weak. In autumn and winter, common dolphins were predicted mainly on the continental shelf of the Bay of Biscay and along the Spanish and Portuguese coasts, with the highest densities in autumn (Figure 5). In spring and summer, they were predicted mainly on the continental slope, with higher densities in Portuguese waters in spring, while in summer, the densities were similar in the Bay of Biscay and in Portuguese and Spanish waters, with a movement towards Irish waters. The uncertainties associated with the highest predictions were estimated mainly in the northern part of the study area and offshore in the oceanic zone due to the lower number of surveys and fewer sightings in our dataset (Supplementary Figure S6).
3.4.1.3 Fin whale
For fin whales, effort and sighting data were aggregated in summer and autumn, and in winter and spring. The model explained 53.6% of the deviance (Figure 4). All dimensions were significant. The relationships varied relatively little between the two seasons, except for dimension 4 (chlorophyll-a concentration and primary production), for which the relationships were reversed between the two seasons, positive in summer/autumn and negative in winter/spring. All relationships were strong and negative except for dimension 3. The distributions were very similar between summer/autumn and winter/spring (Figure 5). Fin whales were mainly concentrated in the oceanic area of the Bay of Biscay and off the west coast of Ireland, Spain and Portugal. The highest densities were predicted in the Bay of Biscay, with densities being much higher in summer/autumn than in winter/spring. Uncertainties were much higher in the north and east of the study area, where relatively few individuals were observed during the surveys we aggregated (Supplementary Figure S6).
3.4.1.4 Harbour porpoise
The harbour porpoise model explained 35.5% of the deviance (Figure 4). All dimensions were significant. For dimension 1 (bottom temperatures and currents), the relationships were strong, positive and similar between seasons. For dimensions 2 and 4 (surface and subsurface currents and eddies, biological production), the relationships differed between the four seasons with stronger variations in spring and autumn. For dimension 3 (surface and subsurface temperatures), the relationship in autumn was reversed (positive) compared to the other seasons, but all were weak. For dimension 5 (slope and roughness), the relationships were similar in autumn and spring, reversed in summer and almost flat in winter. The highest porpoise densities were predicted in the North Sea, especially in the south and west, and along the Norwegian coast (Figure 6). They were also predicted to occur on the continental shelf of the Bay of Biscay, in the Celtic Sea and west of Ireland. In winter, the highest densities were estimated in the south and east of the North Sea (mainly because survey effort in winter in the northern part of the North Sea was low), while at other times of the year, the densities were more homogeneous, although porpoises were mainly concentrated in the south and west of the North Sea. The uncertainties associated with the highest predictions were found in the oceanic zone beyond the continental slope, where the animals were very rarely observed (Supplementary Figure S7).
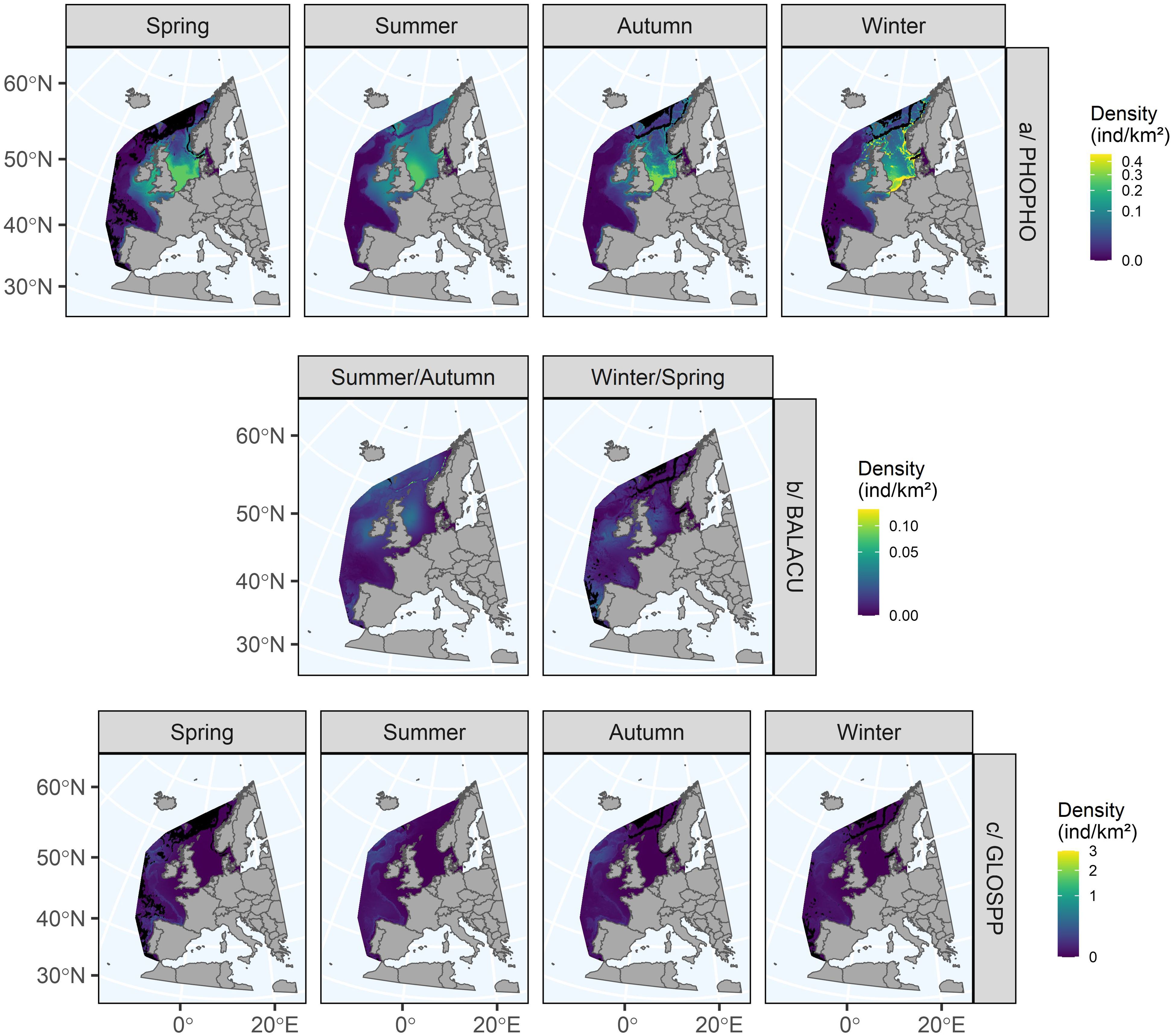
Figure 6. Seasonal distributions predicted for harbour porpoises (a), minke whales (b) and pilot whales (c) in the European Atlantic waters. Black cells represent extrapolation areas where the environmental space did not correspond to the environmental space sampled during the surveys. Uncertainty maps are available in Supplementary Figure S6. ind/km2: individuals per square kilometre, Spring: March to May, Summer: June to August, Autumn: September to November, Winter: December to February.
3.4.1.5 Minke whale
The minke whale model explained 19.4% of the deviance (Figure 4). Only dimensions 1, 2 and 4 were significant. For these dimensions (bottom temperatures and currents, surface and subsurface currents and eddies, biological production), the relationships differed seasonally and were essentially reversed and strong, while for dimensions 3 and 5, the relationships were flat. This suggested a change in distribution or density. Although the distributions were similar between the two seasons, densities were higher in summer/autumn than in winter/spring (Figure 6). In both seasons, minke whales were predicted to occur mainly in the north of the study area, beyond 50°N, in the North Sea, west of Ireland and in the Norwegian Sea, but also off Portugal. The densities in the Bay of Biscay were low. The very high densities predicted on the shelf edge in the north of the study area in summer/autumn were probably due to model overfitting but were not entirely inconsistent with the predictions of Waggitt et al. (2020) and were consistent with the offshore movement of animals in autumn (Risch et al., 2014). Uncertainties were much higher off the Bay of Biscay, in the eastern North Sea and the far north of the study area because minke whales were rarely observed in these areas (Supplementary Figure S7).
3.4.1.6 Pilot whales
The pilot whale model explained 38% of the deviance (Figure 4). All dimensions were significant except dimension 4. The relationships varied little between seasons, regardless of dimension, suggesting little change in their distribution. In fact, the distribution of pilot whales in the European Atlantic waters varied very little between seasons (Figure 7). Pilot whales were predicted mainly on the continental slope throughout the area from Portugal to northern Scotland. Densities were higher in spring than in summer and autumn, and even higher than in winter. In spring, there was a higher concentration of individuals in the north and west of Scotland and off the Iberian Peninsula. The highest uncertainties were found in the English Channel, the North Sea and off the coast of Spain, as these areas had virtually no observations and low sampling effort in the aggregated surveys (Supplementary Figure S8).
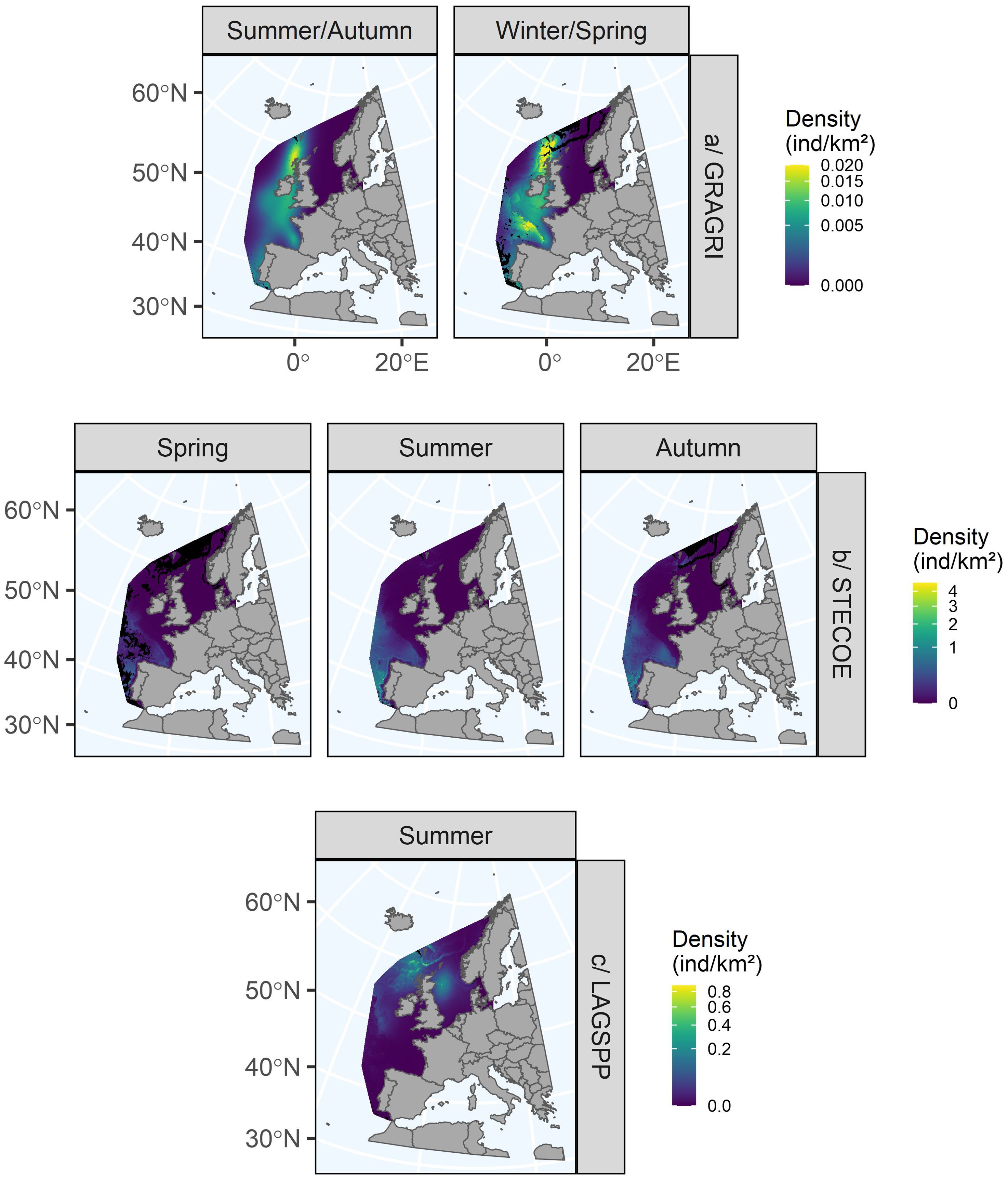
Figure 7. Seasonal distributions predicted for Risso’s dolphins (a), striped dolphins (b) and Lagenorhynchus species (c) in the European Atlantic waters. Black cells represent extrapolation areas where the environmental space did not correspond to the environmental space sampled during the surveys. Uncertainty maps are available in Supplementary Figure S7. ind/km2: individuals per square kilometre, Spring: March to May, Summer: June to August, Autumn: September to November, Winter: December to February.
3.4.1.7 Risso’s dolphin
The Risso’s dolphin model explained 20.3% of the deviance (Figure 4). Only dimension 3 was significant. The relationships differed only for dimension 3 (surface and subsurface temperatures), where they were strong, positive and then negative in summer/autumn and negative and then positive in winter/spring. For the other dimensions, relationships were all flat. Given the discrepancies in the relationships, we did not expect different predictions (Figure 7). The highest densities of Risso’s dolphins were predicted in western Scotland and the Celtic Sea in both seasons. In the Bay of Biscay and off Portugal and Spain, the predictions were slightly more divergent. In winter/spring, higher densities were predicted beyond the continental slope, while in summer/autumn, the highest densities were predicted on the continental slope and shelf. The uncertainties associated with the predictions were high in the north and east of the study area, as relatively few Risso’s dolphins were observed during the surveys due to the cryptic behaviour of Risso’s dolphins at the surface (Supplementary Figure S8).
3.4.1.8 Striped dolphin
The number of striped dolphin sightings in winter was not sufficient to give satisfactory results (cf. Table 2), so we decided to fit the model for the spring, summer and autumn seasons. The model explained 54% of the deviance (Figure 4). Only dimensions 1, 3 and 4 were significant. For dimensions 1, 2, 3 and 5, the relationships varied relatively little over the seasons and were generally weak. However, the relationships with biological production variables (dimension 4) varied considerably, with the autumn relationship being particularly strong. The distribution of striped dolphins varied relatively little between spring, summer and autumn (Figure 7). However, densities varied and were much higher in summer and autumn than in spring, which may indicate that animals arrive in the study area during the summer. Striped dolphins were predicted beyond the continental slope, with higher densities predicted off Portugal. The uncertainties associated with the highest predictions were mainly found in the north of the study area, where the number of surveys was low and there were virtually no sightings of striped dolphins (Supplementary Figure S8).
3.4.1.9 Lagenorhynchus
We were only able to fit a model for Lagenorhynchus in summer, as the number of sightings in the other seasons was insufficient. The model explained 50.7% of the deviance. Only dimension 4 was weakly significant. The linear relationships obtained for dimensions 1 to 4 showed little complexity, suggesting fairly direct relationships with the environmental variables. The relationships for dimensions 1, 2 and 3 were almost flat, that for dimension 4 was negative, and that for dimension 5 was positive and then negative. Lagenorhynchus were predicted only in the north of the study area, beyond 50°N (Figure 6). They were predicted to occur mainly offshore close to the shelf edge in the north-west of Scotland and on the continental shelf in the western North Sea. Unlike the previous species, the areas of greatest uncertainty were predicted in the south of the area and in the far north because Lagenorhynchus had not been observed in these areas (Supplementary Figure S7).
3.4.2 Mediterranean Sea
3.4.2.1 Bottlenose dolphin
The Mediterranean bottlenose dolphin model explained 18.2% of the deviance (Figure 8). Only dimensions 2, 4 and 5 were significant. All relationships were linear, positive for dimension 1 (dynamic variables at depth) and negative and strong for dimensions 2 to 5 (dynamic surface variables, surface and subsurface biological productions, topography). In summer/autumn, bottlenose dolphins were predicted mainly in the Alborán, Ligurian, Tyrrhenian, Sicilian, Adriatic and Aegean Seas, east of the Balearic Islands, as well as on the continental slope off the French coast and in the Gulf of Lion (Figure 9). Very few animals were predicted in the centre of the western basin and in the south and east of the eastern basin. Uncertainties were very high in the south and east of the Mediterranean Sea (Supplementary Figure S9).
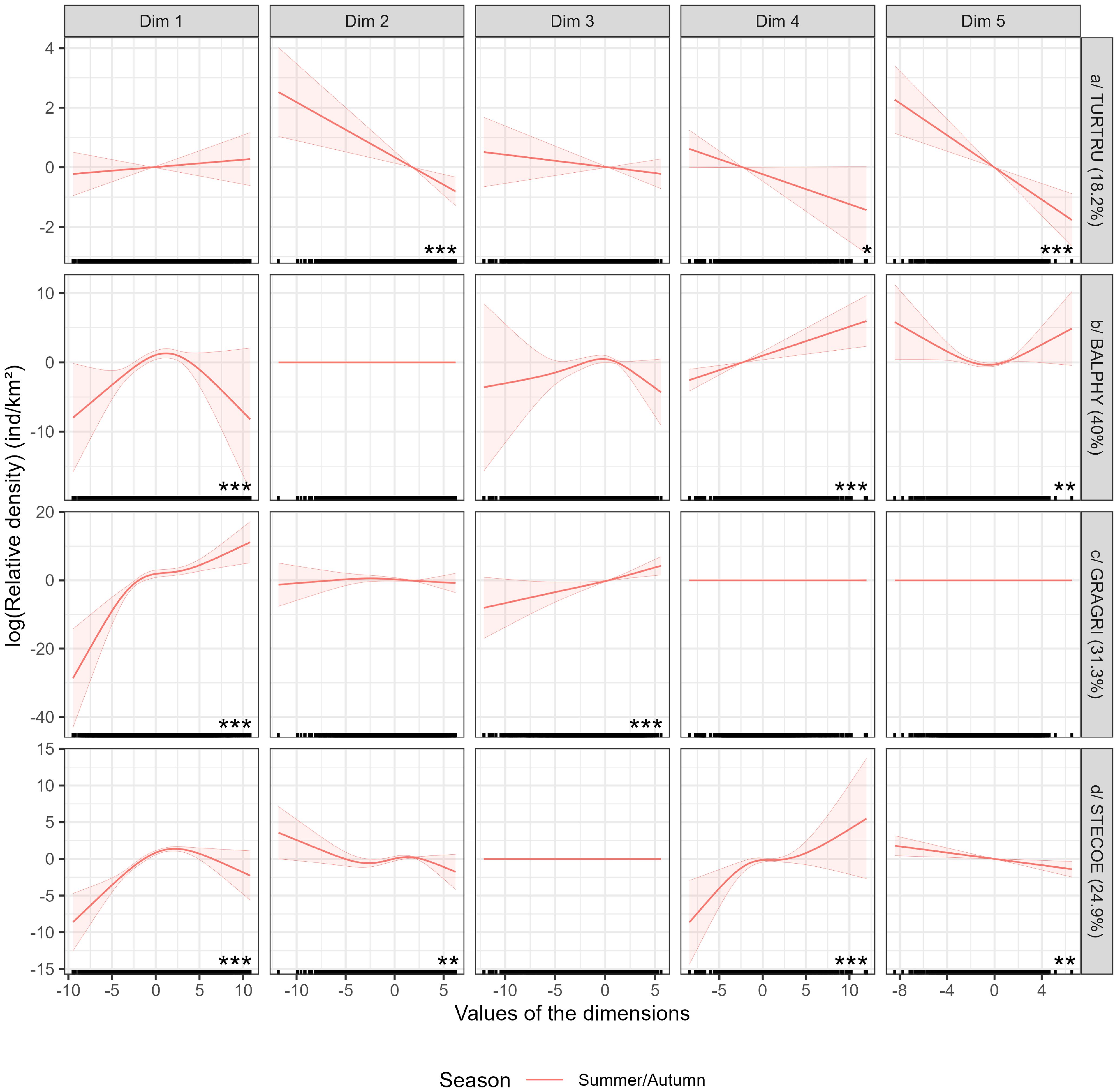
Figure 8. Relationships obtained for each model in the Mediterranean Sea. The curves represent the relationships obtained between cetacean density and explanatory variables (the five dimensions of the PCA) for each season according to the different dimensions of the PCA (Figure 3). Explained deviances of the models fitted for each species are shown in percentages between brackets. The stars (*) represent the significance of the variable; the higher the number, the more significant the variable. If there is no star, the variable is not significant. Summer: June to August, Autumn: September to November.
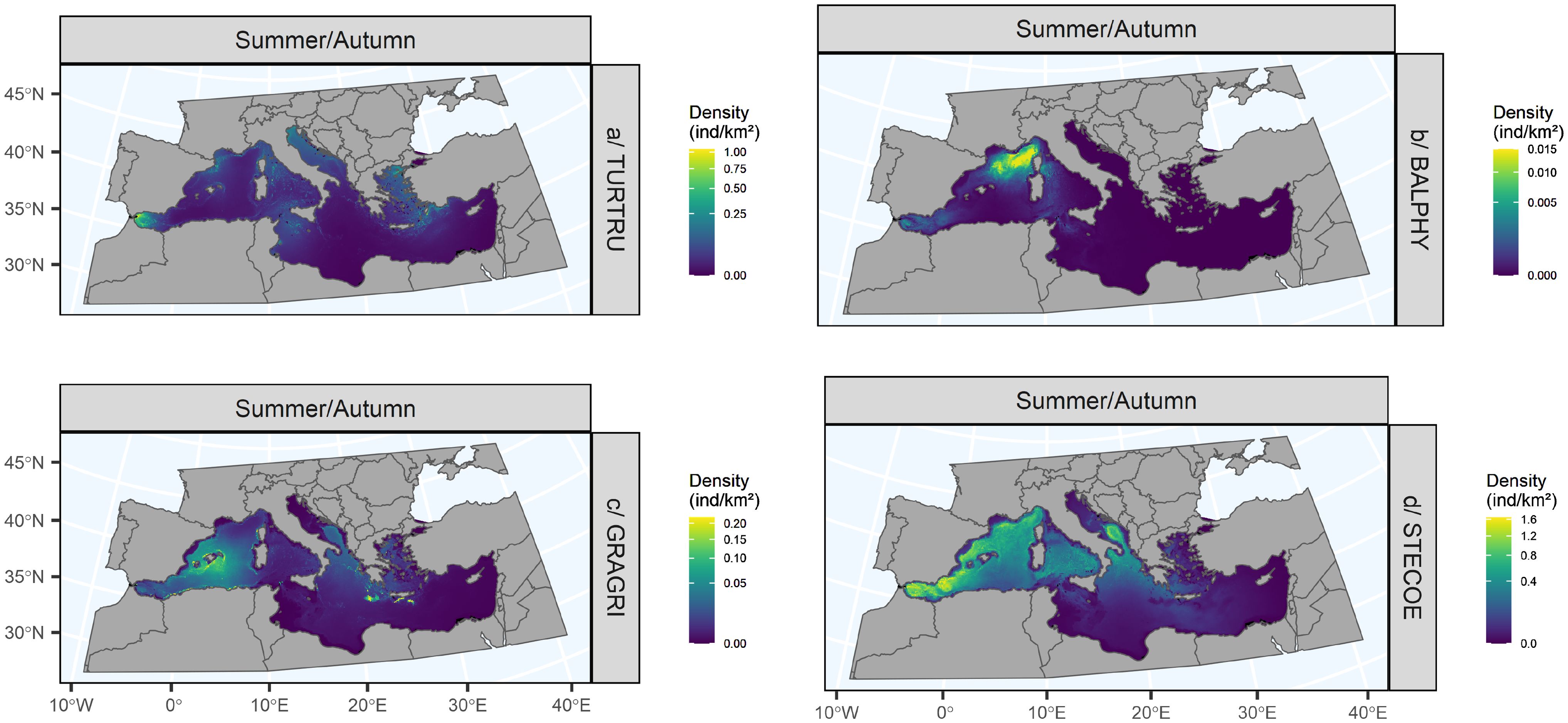
Figure 9. Summer/Autumn distributions predicted for bottlenose dolphins (A), fin whales (B), Risso’s dolphins (C) and striped dolphins (D) in the Mediterranean Sea. Black cells represent extrapolation areas where environmental variables were not sampled during the surveys. Uncertainty maps are available in Supplementary Figure S9. ind/km2: individuals per square kilometre, Summer: June to August, Autumn: September to November.
3.4.2.2 Fin whale
The fin whale model explained 40% of the deviance (Figure 8). Only dimensions 1, 3 and 5 were significant. The relationships were more complex than those for bottlenose dolphins except for dimension 2 (dynamic surface variables), where the relationship was flat. The relationships were strong, positive and then negative for dimensions 1 and 3 (dynamic variables at depth and surface and subsurface chlorophyll-a concentration), positive for dimension 4 (surface and subsurface net primary production), and negative then positive for dimension 5 (slope and roughness). The highest densities were predicted off the French coast, in the Liguro-Provençal basin (Figure 9). Individuals were also predicted in the Alborán Sea and the Tyrrhenian Sea. No individuals were predicted in the eastern basin beyond 11°E. However, the highest uncertainties were estimated in the eastern basin, where there was less survey effort (Supplementary Figure S9).
3.4.2.3 Risso’s dolphin
The Risso’s dolphin model explained 31.3% of the deviance (Figure 8). Only dimensions 1 and 3 were significant. The relationships obtained for dimensions 4 and 5 (surface and subsurface net primary production and topography) were flat and almost flat for dimension 2 (dynamic surface variables). The relationships for dimensions 1 and 3 (dynamic variables at depth and surface and subsurface chlorophyll-a concentrations) were strong and positive. Risso’s dolphins were predicted mainly in the western Mediterranean Sea, the southern Adriatic and the Ionian Sea (Figure 9). The highest densities were predicted around the Balearic Islands, west of Corsica, along the Algerian coast and south of Greece. In contrast, densities were extremely low in the south and east of the Mediterranean basin. Areas of high uncertainty were predicted in the Alborán Sea, the Adriatic Sea and the southern and eastern Mediterranean Sea, as very few individuals had been observed in these areas (Supplementary Figure S9).
3.4.2.4 Striped dolphin
The striped dolphin model explained 24.9% of the deviance (Figure 8). All dimensions except dimension 3 were significant. Except for dimension 3 (surface and subsurface chlorophyll-a concentration) where the relationship was flat, all relationships were strong, positive and then negative for dimension 1 (dynamic variables at depth), negative for dimensions 2 and 5 (dynamic surface variables and topography) and positive for dimension 4 (by surface and subsurface net primary production). In summer/autumn, dolphins were mainly distributed in the western basin and in oceanic habitats (Figure 9). They were also predicted to occur in the southern Adriatic and the Ionian Sea. However, densities were very low in the southern and eastern Mediterranean Sea, partly explained by the low sampling effort. Uncertainties were high in the south and east of the Mediterranean Sea (Supplementary Figure S9).
4 Discussion
In this study, we aimed to predict the seasonal distribution of the most abundant cetacean species in the European Atlantic waters and the Mediterranean Sea by considering processes that may influence their distribution in the water column. To do this, we collated sighting and effort data from several boat and aircraft surveys using a standardised line-transect protocol. The data were homogenised, and spatial and seasonal effects were included in the models to predict seasonal distributions. By including environmental variables describing the water column in a PCA, we were able to describe the environment in three dimensions and not just the surface environment. For species such as cetaceans, which generally feed at depth, it is essential to consider these variables in the models to be able to identify the mechanisms that influence species distributions. Depending on the species, we highlighted either similar or different habitat uses between seasons, resulting in changes in the large-scale spatial distribution and/or density of cetaceans throughout the year. The distributions were predicted considering the extrapolation areas, and we only predicted in zones where the ranges of environmental variables were sampled during the surveys. When predicting outside the areas sampled during the surveys, it is essential to take these extrapolation areas into account to avoid false predictions in uninformed zones.
4.1 Methodological considerations
In this study, we faced a spatially and temporally uneven sampling effort. Most surveys were conducted in the summer when the weather conditions were the most favourable, but there were gaps during the other seasons. Mannocci et al. (2018) identified other surveys that could have been used in the Mediterranean Sea, but most of them were carried out in summer and would not have filled the gaps in winter. The northern and western Mediterranean Sea were well surveyed in summer, but the south of the basin was not, limiting the number of sightings available for modelling the distribution. Waggitt et al. (2020) faced the same disparity in data in the European Atlantic waters, although they collected a greater number of surveys with different protocols (ESAS, strip transect and line-transect protocols). Most of the effort they collected was between May and September, highlighting the need for more sampling, particularly in winter. Predictions made in areas with low coverage are necessarily more uncertain than those made in areas with high coverage.
In the European Atlantic waters, except for Lagenorhynchus, we obtained distribution maps for two or four seasons, which allowed us to highlight possible changes in distribution over the year. We defined a time scale of 3 or 6 months, although a monthly scale might be more appropriate for understanding species distributions, as it was done in Waggitt et al. (2020). Here, the number of sightings per month was insufficient for most species and the model would not have been able to fit relationships across all months. Virgili et al. (2018) have shown that at least 50 sightings should be used to model species distributions, depending on the size of the study area. Therefore, for the less common species, we had to combine data collected in summer and autumn, and in winter and spring. This certainly had an impact on models and predictions, especially for species whose distribution changes in mid-season or for migratory species, such as the minke and fin whales (Risch et al., 2014; Gauffier et al., 2018, 2020). Combining data from two seasons may obscure changes in behaviour and be confounded by seasonal variations in survey effort. Spring and autumn are transitional months for migratory species, and combining them with months when changes in distribution are more limited, such as in summer, may obscure some of the patterns. More sampling outside the summer months would be very beneficial, but in the absence of more consistent effort between seasons, we have aggregated it.
We collected data over 16 years, assuming that cetacean distributions were stable over time and only varied seasonally. This was a strong assumption that can be refuted. For example, the SCANS surveys, conducted in the summers of 1994, 2005 and 2016, respectively, showed changes in summer distribution for most of the species studied. For the harbour porpoise, for example, Hammond et al. (2013) showed that its distribution shifted from the north to the south of the North Sea in the interval between the two surveys in 1994 and 2005. For the common dolphin, a shift was observed between the 2005 and 2016 surveys, indicating interannual variation in the species distributions. However, to detect a change in distribution between years, it would have been necessary to have equivalent spatial coverage in each year, which would have allowed a year effect to be introduced into the model. As the annual spatial coverage of the surveys was highly variable, the inclusion of a year effect would have reflected the distribution of the surveys rather than the actual annual species distribution.
It is important to note that, unlike Waggitt et al. (2020), we did not fully account for detection biases (availability and perception biases) associated with cetacean observations. Detection biases are difficult to estimate and depend on the region, the species and the height and speed of the observation platform (boat vs. plane, sailboat vs. motorboat, high vs. low observation platform, etc.). This bias should be estimated for each species and each survey by setting up specific double platform protocols (e.g., Cañadas et al., 2004; Lambert et al., 2019; Hammond et al., 2021), which is rarely done. Perception biases were estimated when fitting the detection functions and estimating the ESWs by taking into account the platform, the observation height/altitude and that animals were less detectable when observation conditions were unfavourable. For example, ESWs were larger for boat surveys with an elevated platform than for aerial surveys, because sightings are generally recorded below the aircraft. However, we did not attempt to account for availability bias, i.e. biases related to the availability of animals at the surface or subsurface for observation.
In this study, the prediction zone was defined according to the maximum spatial coverage of the surveys, i.e. according to the spatial coverage of the summer surveys. The prediction zone should have varied according to the season, but the aim of the study was to predict the species distributions at the same scale for the different seasons. We therefore extrapolated geographically but were careful to predict only in the environmental interpolation zones, i.e. the zones where the ranges of environmental variables were within the range of values sampled during the surveys (Bouchet et al., 2020). It is important to consider these extrapolation areas because SDMs tend to predict extreme densities in unsampled areas. However, it should be borne in mind that even if the environmental extrapolation zones are taken into account, certain areas remain poorly sampled, such as the southern and eastern Mediterranean Sea, the North Sea and the north of the British Isles, and predictions are therefore more uncertain.
Using different methodologies and partially shared common datasets, we identified distribution patterns common to Waggitt et al. (2020) in the European Atlantic waters and Cañadas et al. (2023) in the Mediterranean Sea. With a larger number of datasets, Waggitt et al. (2020) was able to predict species distributions on a monthly scale. The number of sightings in our dataset was more limited and we were forced to gather data on a seasonal scale. Comparing the distribution maps between the different studies allowed the results to be validated, but also to identify areas that differed and that would benefit from more sampling to validate the predictions. Getting generally similar broad patterns was really encouraging. As pointed out by Waggitt et al. (2020), GAMs can tend to overfit the results and lead to overdispersion, which is visible on certain maps (e.g., the map of minke whales in summer/autumn). However, the consistency of the results between the two studies shows the relevance of using GAMs with a Tweedie distribution, as shown, for example, in the studies of Redfern et al. (2017); Virgili et al. (2019) and Cañadas et al. (2023).
4.2 Modelling species distribution from variables at depth
Cetacean distribution is generally driven by prey distribution, which in turn is driven by oceanographic and biological processes such as currents and primary production (Astarloa et al., 2021; García-Barón et al., 2020; Louzao et al., 2019; Orphanides et al., 2023). Most cetacean prey is found either in the water column or close to the sea bed, or is changing distribution. For example, whiting (Merlangius merlangus) and sandeels (Ammodytes spp) have benthic/demersal and pelagic phases. Minke whales, fin whales and common dolphins feed largely on pelagic prey (Olsen and Holst, 2001; Aguilar and García-Vernet, 2018; Dool and Bosker, 2022), while pilot whales and Risso’s dolphins feed on benthic prey (Spitz et al., 2011; Santos et al., 2014), and harbour porpoises, Lagenorhynchus, bottlenose dolphins and striped dolphins feed on pelagic and demersal prey (Santos and Pierce, 2003; Ringelstein et al., 2006; Hernandez-Milian et al., 2016; Dool and Bosker, 2022). Surface variables are therefore not the only ones to influence the distribution of prey at depth. The strongest and most significant relationships were obtained with the dimensions carried by the dynamic variables characterising the water column and the biological production at the surface, subsurface and depth, regardless of the habitat used by the cetacean prey, except for Risso’s and Lagenorhynchus in the European Atlantic waters. This may reflect that all oceanographic and biological processes, both at the surface and at depth, influence the distribution of cetaceans and that it is necessary to consider all three dimensions of space to predict their distribution (Becker et al., 2016; Waggitt et al., 2020; Virgili et al., 2022). In addition, the significance of the variables differed between the two major study areas, which may indicate a difference in habitat use by species between the Mediterranean Sea and the European Atlantic waters. As the dimensions in the two regions were not supported by the same variables, a more detailed analysis or even a PCA common to both regions would allow a better comparison of the significance of the variables. The significance of the variables is also likely to vary within a region, particularly in European Atlantic waters, especially where there are large areas of epicontinental sea (e.g. the North Sea), compared to seas more influenced by the Atlantic (e.g. the Celtic Sea).
The development and increasing availability of environmental variables that describe the ocean in three dimensions is leading to a better understanding of species distributions, and we can only encourage their use in SDMs. Indeed, Becker et al. (2016) and Virgili et al. (2022) showed a model improvement when depth variables were included in the modelling of cetacean distributions. The constraint associated with this use of variables that characterise the water column is the very high number of variables. In this study, we divided the water column into different water masses and calculated the mean values of the variables in the different classes defined, but we could have considered a different division (every 100 m, for example), which would have considerably increased the number of variables. To overcome this problem, instead of using environmental variables directly in the models, we transformed all the variables into five dimensions using a PCA, following the methodology of Lambert et al. (2018). The PCA dimensions were linear combinations of the raw environmental variables and offered two main advantages for habitat modelling: (i) the number of variables included in the models was reduced, and (ii) the problem of collinearity between environmental variables was eliminated (e.g., between chlorophyll-a concentration and net primary production or between depth and slope). We were not able to fully explain the species distributions using depth variables (explained deviances less than 100%), but the results obtained were very satisfactory (explained deviances between 18% and 54%) and very consistent with sightings collected during the surveys and with their known distribution (e.g., Lambert et al., 2017a, b; Waggitt et al., 2020). The main limitation associated with the use of PCA was the interpretation of the models. Here, we were only able to identify the variables that drove the PCA dimensions and to compare the relationships between seasons but not to interpret the results in terms of habitat preferences. However, as our main objective was to predict the species distributions, this method proved to be efficient.
4.3 Seasonal cetacean distributions
Distribution maps must be interpreted with caution, as they represent relative (not absolute) densities and the species distribution at large spatial scales and over several decades. Density surface models fitted to large-scale data cannot predict the distribution of subpopulations such as bottlenose dolphin coastal populations either in the Atlantic Ocean or in the Mediterranean Sea (Louis, 2014; Gnone et al., 2011). For these populations, fine-scale monitoring is more appropriate and the predictions obtained in our study mostly relate to the offshore ecotypes, particularly for bottlenose dolphins.
In the European Atlantic waters, we predicted three general distribution patterns. Some species showed seasonal variations in density but similar distribution patterns. We might assume that these variations may be due to the migration of animals, as a significant decrease in density necessarily leads to a departure of animals. This was the case for minke and fin whales. The distribution maps were very similar to those of Waggitt et al. (2020) in the north of the study area but, we also predicted relatively high densities off Spain and Portugal for both species in the two seasons. In the Bay of Biscay, as for Waggitt et al. (2020), fin whale densities were high in summer/autumn and lower in winter/spring and minke whale densities were low in summer/autumn but in contrast, minke whale densities in winter/spring were higher. In the European Atlantic waters, these species, mainly fin whales, have been shown to migrate seasonally between high latitude summer feeding grounds and low latitude winter breeding grounds (Risch et al., 2014; Aguilar and García-Vernet, 2018). In the case of fin whales, the fact that similar curves were obtained for the two seasons (Figure 4) supports the idea of possible migration. Physiological processes such as feeding and reproduction underlie these changes in density.
For other species, we predicted changes in distribution, while overall densities remained stable. For the common dolphin, we showed that animals were predicted to be mainly on the continental slope in spring and summer, whereas they were distributed over the continental shelf in autumn and winter. These results confirm those of Murphy et al. (2013) and Lambert et al. (2017a). This seasonal change could be related to the migration of pelagic prey (Pusineri et al., 2007; Meynier et al., 2008), moving from the edges of the continental shelf in summer to more coastal habitats in winter. This migration can vary from year to year, both in terms of how far north they move seasonally and the extent to which they occupy shelf seas. The fact that we obtained different curves in autumn and spring (Figure 4) supports this hypothesis. These could be transitional periods, possibly associated with either a change in diet or a shift in available prey. For harbour porpoises, we have little data from the North Sea, but the predictions obtained were consistent with the recent distribution obtained by Gilles et al. (2016), with a westward concentration of animals in spring and summer, consistent with the distribution of whiting and cod (Gadus morhua, Ransijn et al., 2021). In autumn and winter, the predictions were more consistent with those of Waggitt et al. (2020), with a concentration of animals to the east and south of the North Sea, consistent with the distribution of sprat (Sprattus sprattus) and herring (Clupea harengus, Ransijn et al., 2021). This change in distribution may be partly related to a change in the distribution of harbour porpoise prey such as sandeels (Santos and Pierce, 2003; Gilles et al., 2016), but other prey or factors may also be involved. In French waters, harbour porpoise densities were lower in summer and much higher in spring, with a more offshore distribution in summer than in winter. These results were consistent with and supported those of Lambert et al. (2017a) and changes in distribution may be related to prey availability. For bottlenose dolphins, we predicted a shift between the continental shelf and the shelf edge during the year, while Waggitt et al. (2020) predicted consistently high densities on the shelf edge. The strong annual changes in the distribution in certain areas, such as the Celtic Sea, are not visible here (Rogan et al., 2018). The diet of bottlenose dolphins is highly diversified, including gadoids and cephalopods, and they can forage in different habitats (Spitz et al., 2006; Hernandez-Milian et al., 2015). This shift may therefore be related to a change in diet or a shift in prey availability.
Pilot whales, Risso’s dolphins and striped dolphins showed little change in their distribution over the seasons, which was very consistent with Waggitt et al. (2020)’s results. We also showed a concentration of species off Spain and Portugal where environmental conditions are favourable. Distributions may change little because their diets are more adaptable than those of other species and they manage to find their food in all seasons. Pilot whales were mainly concentrated offshore and along the shelf edge, as previously shown by Rogan et al. (2017). According to Spitz et al. (2011), unlike other deep-diving species, pilot whales can forage on both the continental shelf and in the oceanic habitat and they can exploit different trophic levels depending on the season (de Stephanis et al., 2008), so they are highly adaptable. Risso’s dolphins were not restricted to shelf edges, they were also predicted on the continental shelf and in oceanic waters as previously shown by Jefferson et al. (2014). The stomach contents of Risso’s dolphins vary geographically, with mainly benthic squid found in the Bay of Biscay (Spitz et al., 2011), while octopus (Eledone cirrhosa) were also found in the UK, with fish and crustaceans in smaller proportions (MacLeod et al., 2014). These species are largely distributed in the Northeast Atlantic Ocean, which explains the wide distribution of Risso’s dolphins. Striped dolphins were mainly predicted in the south of the study area with the highest densities predicted off Portugal. Contrary to Waggitt et al. (2020), we did not predict seasonal variations in species densities. Striped dolphin distribution is mainly driven by their prey distribution. Stomach content analysis revealed the presence of lanternfish (Notoscopelus kroeyeri and Lobianchia gemellarii) and squid (Teuthowenia megalops and Histioteuthis spp) in the stomachs, which are all oceanic species (Ringelstein et al., 2006).
For Lagenorhynchus, we only had enough data for modelling during the summer, so we were unable to predict their seasonal distribution. Their distribution range lay to the north of the study area. The predicted distribution combined the distribution of the two species included in the group, the distribution of the demersal feeder white-beaked dolphin in shelf waters (Reid et al., 2003; Canning et al., 2008; Jansen et al., 2010; Evans and Waggitt, 2020) and the distribution of the pelagic feeder Atlantic white-sided dolphin near the shelf edge (Reid et al., 2003; Wall et al., 2013; Hernandez-Milian et al., 2016; Evans and Waggitt, 2020). Our results were very consistent with their known seasonal distribution in the Scottish waters (Canning et al., 2008; Wall et al., 2013) and with the summer predictions of Waggitt et al. (2020).
In the Mediterranean Sea, we only predicted the summer/autumn species distribution. Effort was fairly homogeneous, but a clear west-east gradient in the sightings was observed (Supplementary Figures S2, S4). The predictions were consistent with those of Cañadas et al. (2023), mainly in the western and northern central basins, and we both found lower densities in the eastern basin. Bottlenose dolphins showed a preference for coastal and shelf waters. They are a generalist species whose diet and foraging behaviour appear to be area-dependent (Bearzi et al., 2008), moving inshore during the summer to calve, which explains the absence of the species in oceanic areas. Fin whales were predicted only in the western basin, mainly in the Liguro-Provençal Basin, an area known to concentrate fin whales and krill in summer (Geijer et al., 2016; Notarbartolo di Sciara et al., 2016). No individuals were observed in the eastern basin while few individuals have already been observed (Stephens et al., 2021). Risso’s dolphins were widely distributed in oceanic waters, except in the south and east where little or no observation effort has been performed, with the highest densities predicted on continental slopes and near offshore islands, as previously shown by Bearzi et al. (2011). They feed mainly on mesopelagic squid, which explains their concentration in slope areas. Striped dolphins were mainly concentrated in the western and north-central basins in oceanic waters. They are generally found in different habitats due to their opportunistic feeding habits (Azzellino et al., 2008).
4.4 Application for species conservation
The distribution of cetaceans is thought to be mainly related to the distribution and availability of their prey, but human activities such as offshore wind farms, shipping and fishing can also affect cetacean distribution as they often overlap (Avila et al., 2018; Evans et al., 2021). The influence of these threats on species distribution is difficult to model and incorporate into SDMs, but the distribution predicted in the study can be used to inform conservation, in particular by allowing human activities to be planned in areas where they will have less impact because of lower concentrations of animals. Not all human activities have the same impact on species. Small species such as harbour porpoises, striped dolphins and especially common dolphins in the Bay of Biscay are vulnerable to bycatch in fishing gear (Peltier et al., 2016; IJsseldijk et al., 2021; Marçalo et al., 2021). Larger cetacean species, such as fin whales, are more sensitive to collisions with vessels (ferries, cargos, etc.; Evans et al., 2011; Schoeman et al., 2020). For example, the area off the Bay of Biscay in summer/autumn would be particularly sensitive for fin whales, yet it is an area frequently crossed by commercial and passenger vessels (Halpern et al., 2015; Pirotta et al., 2019). Routing vessels away from the concentration area would certainly be beneficial for fin whales. For common dolphins, the prediction maps clearly showed a concentration of individuals on the continental shelf of the Bay of Biscay in autumn and winter. Peltier et al. (2021) showed that this is an area where common dolphins stranded on the French coast and showing signs of bycatch are likely to have been caught. For example, the use of noncatching fishing gear in this area would be preferable. The prediction maps also show a concentration of harbour porpoises in the North Sea, one of the most anthropised seas in the world, with intensive development of offshore wind farms, accidental captures and intense shipping (Halpern et al., 2015). Activities need to be regulated to limit the impact on this species.
Working on a large scale enables to meet international conservation objectives for marine megafauna, such as those set by the OSPAR Convention in the North-East Atlantic Ocean or ACCOBAMS in the Mediterranean Sea, or to identify Important Marine Mammal Areas. The benefits are even more obvious when we focus on mobile species such as cetaceans. It is then possible to work at a population scale. The study of seasonality could also allow the establishment of dynamic marine protected areas according to the seasons. Distribution maps alone are not enough to identify high-risk areas. Other parameters, such as the concentration of ships, the time the animals spend in an area and the species life cycle, need to be considered, but this work can contribute to more targeted measures for conservation of cetacean species.
5 Conclusion
By combining 16 years of surveys, we predicted the seasonal distribution of the most abundant cetacean species in the European Atlantic waters and the Mediterranean Sea using environmental variables integrated over the water column instead of the usual surface variables. We identified areas of species concentration where human activities should be restricted. For example, this study has confirmed previously identified areas with high densities of common dolphins in the Bay of Biscay to limit bycatches, or areas with high densities of fin whales in the European Atlantic waters and the Mediterranean Sea, to limit collisions with shipping (commercial or passenger). The results presented here reinforce the results obtained from earlier studies using different datasets or methodologies and demonstrate the need for adequate survey effort in space and time. At present, these data are not available in winter in most areas, there are still large gaps where survey effort is low, for example in the southern and south-eastern Mediterranean Sea, the northern and north-western parts of the study area in European Atlantic waters, and in many areas off the shelf. Improved seasonal coverage will allow us to better predict where species occur.
Data availability statement
The raw data supporting the conclusions of this article will be made available by the authors upon request. Note that availability of final results is subject to approval from the funders.
Author contributions
AV: Writing – original draft, Methodology, Investigation, Formal analysis, Conceptualization. HA: Writing – review & editing, Data curation. AA: Writing – review & editing, Data curation. GD: Writing – review & editing, Data curation. IG: Writing – review & editing, Data curation. CE: Writing – review & editing, Data curation. ML: Writing – review & editing, Data curation. SL: Writing – review & editing, Data curation. CS: Writing – review & editing, Data curation. OV: Writing – review & editing, Data curation. VR: Writing – review & editing, Validation, Supervision, Conceptualization.
Funding
The author(s) declare financial support was received for the research, authorship, and/or publication of this article. This study was funded by the Direction Générale de l’Armement Techniques Navales.
Acknowledgments
We are grateful to all the observers and staff (ships’ captains, crews and pilots) who have participated in collecting the data used in this study. We thank the Sea Marine Mammal Unit for providing SCANS and CODA survey data. We acknowledge the ObSERVE project undertaken by the Department of Communications, Climate Action and Environment and the National Parks and Wildlife Service, Department of Culture, Heritage and the Gaeltacht for providing ObSERVE data. We thank the Spanish Ministry of Ecological Transition and Demographic Challenge for partially funding the collection of the data collected by Spain, as well as the scientific institutions IEO and AZTI for supporting these programs. We also thank the Agreement on the Conservation of Cetaceans of the Black Sea, Mediterranean Sea and contiguous Atlantic area (ACCOBAMS) for providing ASI data. We thank the Direction Générale de l’Armement Techniques Navales for funding this study. We would particularly like to thank Matthieu Authier and Floriane Plard for their advice in carrying out the analyses. Finally, AV personally thanks the project Share the Ocean, which aims to reduce collisions between ships and cetaceans, for giving her the opportunity to write this paper.
Conflict of interest
The authors declare that the research was conducted in the absence of any commercial or financial relationships that could be construed as a potential conflict of interest.
Publisher’s note
All claims expressed in this article are solely those of the authors and do not necessarily represent those of their affiliated organizations, or those of the publisher, the editors and the reviewers. Any product that may be evaluated in this article, or claim that may be made by its manufacturer, is not guaranteed or endorsed by the publisher.
Supplementary material
The Supplementary Material for this article can be found online at: https://www.frontiersin.org/articles/10.3389/fmars.2024.1319791/full#supplementary-material
References
Aguilar A., García-Vernet R. (2018). “Fin whale: Balaenoptera physalus,” in Encyclopedia of Marine Mammals (Third Edition), 3rd ed. Eds. Würsig B., Thewissen J., Kovacs K. M. (London, UK: Academic Press), 368–371. doi: 10.1016/B978-0-12-804327-1.00128-X
Astarloa A., Louzao M., Andrade J., Babey L., Berrow S., Boisseau O., et al. (2021). The role of climate, oceanography, and prey in driving decadal spatio-temporal patterns of a highly mobile top predator. Front. Mar. Sci. 8, 665474. doi: 10.3389/fmars.2021.665474
Avila I. C., Kaschner K., Dormann C. F. (2018). Current global risks to marine mammals: Taking stock of the threats. Biol. Conserv. 221, 44–58. doi: 10.1016/j.biocon.2018.02.021
Azzellino A., Gaspari S., Airoldi S., Nani B. (2008). Habitat use and preferences of cetaceans along the continental slope and the adjacent pelagic waters in the western Ligurian Sea. Deep Sea Res. Part I: Oceanographic Res. Papers 55, 296–323. doi: 10.1016/j.dsr.2007.11.006
Baum J. K., Worm B. (2009). Cascading top-down effects of changing oceanic predator abundances. J. Anim. Ecol. 78, 699–714. doi: 10.1111/j.1365-2656.2009.01531.x
Bearzi G., Fortuna C. M., Reeves R. R. (2008). Ecology and conservation of common bottlenose dolphins Tursiops truncatus in the Mediterranean Sea. Mammal Rev. 39, 92. doi: 10.1111/j.1365-2907.2008.00133.x
Bearzi G., Reeves R. R., Remonato E., Pierantonio N., Airoldi S. (2011). Risso’s dolphin Grampus griseus in the Mediterranean Sea. Mamm. Biol. 76, 385–400. doi: 10.1016/j.mambio.2010.06.003
Becker E. A., Forney K. A., Fiedler P. C., Barlow J., Chivers S. J., Edwards C. A., et al. (2016). Moving towards dynamic ocean management: How well do modeled ocean products predict species distributions? Remote Sens. 8, 149. doi: 10.3390/rs8020149
Bode A., Alvarez-Ossorio M., Cabanas J., Miranda A., Varela M. (2009). Recent trends in plankton and upwelling intensity off Galicia (nw Spain). Prog. Oceanography 83, 342–350. doi: 10.1016/j.pocean.2009.07.025
Bouchet P., Miller D., Mannocci L., Roberts J., Harris C., Thomas L. (2019). dsmextra: A Toolkit for Extrapolation Assessments in Density Surface Models. R package version 1.1.5.
Bouchet P. J., Miller D. L., Roberts J. J., Mannocci L., Harris C. M., Thomas L. (2020). dsmextra: Extrapolation assessment tools for density surface models. Methods Ecol. Evol. 11, 1464–1469. doi: 10.1111/2041-210X.13469
Breen P., Brown S., Reid D., Rogan E. (2017). Where is the risk? integrating a spatial distribution model and a risk assessment to identify areas of cetacean interaction with fisheries in the northeast Atlantic. Ocean Coast. Manage. 136, 148–155. doi: 10.1016/j.ocecoaman.2016.12.001
Brodie S., Jacox M. G., Bograd S. J., Welch H., Dewar H., Scales K. L., et al. (2018). Integrating dynamic subsurface habitat metrics into species distribution models. Front. Mar. Sci. 5, 219. doi: 10.3389/fmars.2018.00219
Buckland S., Anderson D., Burnham K., Laake J., Borchers D., Thomas L. (2001). Introduction to Distance Sampling: Estimating Abundance of Biological Populations (Oxford, UK: Oxford University Press). doi: 10.1093/oso/9780198506492.001.0001
Buckland S. T., Rexstad E. A., Marques T. A., Oedekoven C. S. (2015). Distance sampling: methods and applications (New York, USA: Springer International Publishing). doi: 10.1007/978-3-319-19219-2
Canning S. J., Santos M. B., Reid R. J., Evans P. G., Sabin R. C., Bailey N., et al. (2008). Seasonal distribution of white-beaked dolphins (Lagenorhynchus albirostris) in UK waters with new information on diet and habitat use. J. Mar. Biol. Assoc. United Kingdom 88, 1159–1166. doi: 10.1017/S0025315408000076
Cañadas A., Desportes G., Borchers D. (2004). The estimation of the detection function and g(0) for shortbeaked common dolphins (Delphinus delphis), using doubleplatform data collected during the NASS-95 Faroese survey. J. Cetacean Res. Manage. 6, 191–198. doi: 10.47536/jcrm.v6i2.782
Cañadas A., Pierantonio N., Araújo H., David L., Di Meglio N., Dorémus G., et al. (2023). Distribution patterns of marine megafauna density in the Mediterranean Sea assessed through the ACCOBAMS Survey Initiative (ASI). Front. Mar. Sci. 10. doi: 10.3389/fmars.2023.1270917
de Stephanis R., García-Tíscar S., Verborgh P., Esteban-Pavo R., Pérez S., Minvielle-Sebastia L., et al. (2008). Diet of the social groups of long-finned pilot whales (Globicephala melas) in the strait of Gibraltar. Mar. Biol. 154, 603–612. doi: 10.1007/s00227-008-0953-8
Dool T., Bosker T. (2022). Predicted microplastic uptake through trophic transfer by the shortbeaked common dolphin (Delphinus delphis) and common bottlenose dolphin (Tursiops truncatus) in the northeast Atlantic Ocean and Mediterranean Sea. Mar. pollut. Bull. 180, 113745. doi: 10.1016/j.marpolbul.2022.113745
Doughty C. E., Roman J., Faurby S., Wolf A., Haque A., Bakker E. S., et al. (2016). Global nutrient transport in a world of giants. Proc. Natl. Acad. Sci. 113, 868–873. doi: 10.1073/pnas.1502549112
Elith J., Leathwick J. R. (2009). Species distribution models: Ecological explanation and prediction across space and time. Annu. Rev. Ecology Evolution Systematics 40, 677–697. doi: 10.1146/annurev.ecolsys.110308.120159
Evans P., Baines M., Anderwald P. (2011). Risk assessment of potential conflicts between shipping and cetaceans in the ASCOBANS region. ASCOBANS AC18/Doc 6–04, 32 pp.
Evans P. G. H., Carrington C. A., Waggitt J. J. (2021). Risk Mapping of Bycatch of Protected Species in Fishing Activities. Sea Watch Foundation & Bangor University, UK. European Commission Contract No. 09029901/2021/844548/ENV.D.3. 212pp.
Evans P., Waggitt J. (2020). “chap. Cetaceans,” in Atlas of the Mammals of Great Britain and Northern Ireland (Exeter, UK: Pelagic Publishing), 134–184. doi: 10.53061/XTWI9286
Foster S. D., Bravington M. V. (2013). A Poisson-Gamma model for analysis of ecological nonnegative continuous data. Environ. Ecol. Stat 20, 533–552. doi: 10.1007/s10651-012-0233-0
Franklin J. (2010). Mapping species distributions: spatial inference and prediction (Cambridge, UK: Cambridge University Press). doi: 10.1017/CBO9780511810602
García-Barón I., Santos M. B., Saavedra C., Astarloa A., Valeiras J., García Barcelona S., et al. (2020). Essential ocean variables and high value biodiversity areas: Targets for the conservation of marine megafauna. Ecol. Indic. 117, 106504. doi: 10.1016/j.ecolind.2020.106504
Gauffier P., Borrell A., Silva M. A., Víkingsson G. A., López A., Giménez J., et al. (2020). Wait your turn, north Atlantic fin whales share a common feeding ground sequentially. Mar. Environ. Res. 155, 104884. doi: 10.1016/j.marenvres.2020.104884
Gauffier P., Verborgh P., Giménez J., Esteban R., Sierra J. S., de Stephanis R. (2018). Contemporary migration of fin whales through the strait of Gibraltar. Mar. Ecol. Prog. Ser. 588, 215–228. doi: 10.3354/meps12449
Geijer C. K., Notarbartolo di Sciara G., Panigada S. (2016). Mysticete migration revisited: are Mediterranean fin whales an anomaly? Mammal Rev. 46, 284–296. doi: 10.1111/mam.12069
Gilles A., Authier M., Ramirez-Martinez N. C., Araújo H., Blanchard A., Carlström J., et al. (2023). Estimates of cetacean abundance in European Atlantic waters in summer 2022 from the SCANS-IV aerial and shipboard surveys. Final report published 29 September 2023. 64 pp. Available at: https://tinyurl.com/3ynt6swa.
Gilles A., Viquerat S., Becker E. A., Forney K. A., Geelhoed S. C. V., Haelters J., et al. (2016). Seasonal habitat-based density models for a marine top predator, the harbor porpoise, in a dynamic environment. Ecosphere 7 (6), e01367. doi: 10.1002/ecs2.1367
Gnone G., Bellingeri M., Dhermain F., Dupraz F., Nuti S., Bedocchi D., et al. (2011). Distribution, abundance, and movements of the bottlenose dolphin (Tursiops truncatus) in the Pelagos sanctuary MPA (north-west Mediterranean Sea). Aquat. Conservation: Mar. Freshw. Ecosyst. 21, 372–388. doi: 10.1002/aqc.1191
Halpern B. S., Frazier M., Potapenko J., Casey K. S., Koenig K., Longo C., et al. (2015). Spatial and temporal changes in cumulative human impacts on the world’s ocean. Nat. Commun. 6, 7615. doi: 10.1038/ncomms8615
Hammond P., Lacey C., Gilles A., Viquerat S., Börjesson P., Herr H., et al. (2021). Estimates of cetacean abundance in European Atlantic waters in summer 2016 from the SCANS-III aerial and shipboard surveys. Tech. rep. Wageningen Mar. Res. 41.
Hammond P. S., Macleod K., Berggren P., Borchers D. L., Burt L., Cañadas A., et al. (2013). Cetacean abundance and distribution in European Atlantic shelf waters to inform conservation and management. Biol. Conserv. 164, 107–122. doi: 10.1016/j.biocon.2013.04.010
Hastie T., Tibshirani R. (1987). Generalized additive models: Some applications. J. Am. Stat. Assoc. 82, 371–386. doi: 10.1080/01621459.1987.10478440
Hernandez-Milian G., Berrow S., Santos M. B., Reid D., Rogan E. (2015). Insights into the trophic ecology of bottlenose dolphins (Tursiops truncatus) in Irish waters. Aquat. Mammals 41, 226–239. doi: 10.1578/AM.41.2.2015.226
Hernandez-Milian G., Santos M. B., Reid D. G., Rogan E. (2016). Insights into the diet of Atlantic white-sided dolphins (Lagenorhynchus acutus) in the northeast Atlantic. Mar. Mamm. Sci. 32 (2), 735–742. doi: 10.1111/mms.12272
Hunt G. L., McKinnell S. (2006). Interplay between top-down, bottom-up, and wasp-waist control in marine ecosystems. Prog. Oceanography 68, 115–124. doi: 10.1016/j.pocean.2006.02.008
IJsseldijk L. L., Scheidat M., Siemensma M. L., Couperus B., Leopold M. F., Morell M., et al. (2021). Challenges in the assessment of bycatch: Postmortem findings in harbor porpoises (Phocoena phocoena) retrieved from gillnets. Veterinary Pathol. 58, 405–415. doi: 10.1177/0300985820972454
Jansen O. E., Leopold M. F., Meesters E. H., Smeenk C. (2010). Are white-beaked dolphins Lagenorhynchus albirostris food specialists? Their diet in the southern North Sea. J. Mar. Biol. Assoc. United Kingdom 90, 1501–1508. doi: 10.1017/S0025315410001190
Jefferson T. A., Weir C. R., Anderson R. C., Ballance L. T., Kenney R. D., Kiszka J. J. (2014). Global distribution of Risso’s dolphin Grampus griseus: a review and critical evaluation. Mammal Rev. 44, 56–68. doi: 10.1111/mam.2014.44.issue-1
Jongman R. H. G., Braak C. J. F. T., van Tongeren O. F. R. (1995). Data Analysis in Community and Landscape Ecology (Cambridge, UK: Cambridge University Press). doi: 10.1017/CBO9780511525575
Koutsikopoulos C., Le Cann B. (1996). Physical processes and hydrological structures related to the Bay of Biscay anchovy. Scientia Marina 60, 9–19.
Lambert C., Authier M., Doray M., Dorémus G., Spitz J., Ridoux V. (2018). Decadal stability in top predator habitat preferences in the Bay of Biscay. Prog. Oceanography 166, 109–120. doi: 10.1016/j.pocean.2018.03.007
Lambert C., Authier M., Dorémus G., Gilles A., Hammond P., Laran S., et al. (2019). The effect of a multi-target protocol on cetacean detection and abundance estimation in aerial surveys. R. Soc. Open Sci. 6, 190296. doi: 10.1098/rsos.190296
Lambert C., Laran S., David L., Dorémus G., Pettex E., Canneyt O. V., et al. (2017a). How does ocean seasonality drive habitat preferences of highly mobile top predators? Part I: The north-western Mediterranean Sea. Deep Sea Res. Part II: Topical Stud. Oceanography 141, 115–132. doi: 10.1016/j.dsr2.2016.06.012
Lambert C., Pettex E., Dorémus G., Laran S., Stéphan E., Van Canneyt O., et al. (2017b). How does ocean seasonality drive habitat preferences of highly mobile top predators? Part II: The eastern north-Atlantic. Deep Sea Res. Part II: Topical Stud. Oceanography 141, 133–154. doi: 10.1016/j.dsr2.2016.06.011
Lau-Medrano W. (2024). grec: Gradient-Based Recognition of Spatial Patterns in Environmental Data. R package version 1.6.0.
Louis M. (2014). Social, ecological and genetic structures of bottlenose dolphins, Tursiops truncatus, in the Normano-Breton gulf and in the North-East Atlantic (La Rochelle, France: Ph.D. thesis, La Rochelle Université).
Louzao M., Valeiras J., García-Barcelona S., González-Quirós R., Nogueira E., Iglesias M., et al. (2019). Marine megafauna niche coexistence and hotspot areas in a temperate ecosystem. Continental Shelf Res. 186, 77–87. doi: 10.1016/j.csr.2019.07.013
MacLeod C. D., Santos M. B., Burns F., Brownlow A., Pierce G. J. (2014). Can habitat modelling for the octopus Eledone cirrhosa help identify key areas for Risso’s dolphin in Scottish waters? Hydrobiologia 725, 125–136. doi: 10.1007/s10750-013-1555-0
Mannocci L., Roberts J. J., Halpin P. N., Authier M., Boisseau O., Bradai M. N., et al. (2018). Assessing cetacean surveys throughout the Mediterranean Sea: a gap analysis in environmental space. Sci. Rep. 8, 3126. doi: 10.1038/s41598-018-19842-9
Marçalo A., Giménez J., Nicolau L., Frois J., Ferreira M., Sequeira M., et al. (2021). Stranding patterns and feeding ecology of striped dolphins, Stenella coeruleoalba, in western Iberia, (1981–2014). J. Sea Res. 169, 101996. doi: 10.1016/j.seares.2021.101996
Marques F. F., Buckland S. T. (2003). Incorporating covariates into standard line transect analyses. Biometrics 59, 924–935. doi: 10.1111/j.0006-341X.2003.00107.x
Marzocchi A., Hirschi J. J.-M., Holliday N. P., Cunningham S. A., Blaker A. T., Coward A. C. (2015). The north Atlantic subpolar circulation in an eddy-resolving global ocean model. J. Mar. Syst. 142, 126–143. doi: 10.1016/j.jmarsys.2014.10.007
McDonald T., Carlisle J., McDonald A. (2019). Rdistance: Distance-Sampling Analyses for Density and Abundance Estimation. R package version 2.1.3.
Meynier L., Pusineri C., Spitz J., Santos M., Pierce G., Ridoux V. (2008). Intraspecific dietary variation in the short-beaked common dolphin Delphinus delphis in the Bay of Biscay: importance of fat fish. Mar. Ecol. Prog. Ser. 354, 277–287. doi: 10.3354/meps07246
Miller P. J., Isojunno S., Siegal E., Lam F.-P. A., Kvadsheim P. H., Curé C. (2022). Behavioral responses to predatory sounds predict sensitivity of cetaceans to anthropogenic noise within a soundscape of fear. Proc. Natl. Acad. Sci. 119, e2114932119. doi: 10.1073/pnas.2114932119
Millot C., Taupier-Letage I. (2005). “chap. Circulation in the Mediterranean Sea: Updated description and schemas of the circulation of the water masses in the whole Mediterranean Sea,” in Handbook of Environmental Chemistry: The Mediterranean Sea, vol. 5-K. (Berlin, Germany: Springer Berlin Heidelberg), 29–66. doi: 10.1007/b107143
Murphy S., Law R. J., Deaville R., Barnett J., Perkins M. W., Brownlow A., et al. (2018). “chap. Organochlorine contaminants and reproductive implication in cetaceans: a case study of the common dolphin” in Marine Mammal Ecotoxicology. (Cambridge, UK: Academic Press), 3–38. doi: 10.1016/B978-0-12-812144-3.00001-2
Murphy S., Pinn E. H., Jepson P. D. (2013). The short-beaked common dolphin (Delphinus delphis) in the north-east Atlantic: distribution, ecology, management and conservation status. Oceanography Mar. biology: Annu. Rev. 51, 193–280.
Notarbartolo di Sciara G., Castellote M., Druon J.-N., Panigada S. (2016). Fin whales, Balaenoptera physalus: at home in a changing Mediterranean Sea? Adv. Mar. Biol. 75, 75–101. doi: 10.1016/bs.amb.2016.08.002
Olsen E., Holst J. C. (2001). A note on common minke whale (Balaenoptera acutorostrata) diets in the Norwegian Sea and the North Sea. J. Cetacean Res. Manage. 3, 179–183. doi: 10.47536/jcrm.v3i2.888
Orphanides C. D., Jech J. M., Palka D. L., Collie J. (2023). Relating marine mammal distribution to water column prey structure derived from echosounding. Mar. Ecol. Prog. Ser. 711, 101–119. doi: 10.3354/meps14290
Paradinas I., Illian J., Smout S. (2023). Understanding spatial effects in species distribution models. PloS One 18, e0285463. doi: 10.1371/journal.pone.0285463
Peltier H., Authier M., Caurant F., Dabin W., Daniel P., Dars C., et al. (2021). In the wrong place at the wrong time: Identifying spatiotemporal co-occurrence of bycaught common dolphins and fisheries in the Bay of Biscay (NE Atlantic) from 2010 to 2019. Front. Mar. Sci. 8. doi: 10.3389/fmars.2021.617342
Peltier H., Authier M., Deaville R., Dabin W., Jepson P. D., van Canneyt O., et al. (2016). Small cetacean bycatch as estimated from stranding schemes: the common dolphin case in the northeast Atlantic. Environ. Sci. Policy 63, 7–18. doi: 10.1016/j.envsci.2016.05.004
Pennino M. G., Arcangeli A., Prado Fonseca V., Campana I., Pierce G. J., Rotta A., et al. (2017). A spatially explicit risk assessment approach: Cetaceans and marine traffic in the Pelagos sanctuary (Mediterranean Sea). PloS One 12, e0179686. doi: 10.1371/journal.pone.0179686
Pirotta V., Grech A., Jonsen I. D., Laurance W. F., Harcourt R. G. (2019). Consequences of global shipping traffic for marine giants. Front. Ecol. Environ. 17, 39–47. doi: 10.1002/fee.1987
Pusineri C., Magnin V., Meynier L., Spitz J., Hassani S., Ridoux V. (2007). Food and feeding ecology of the common dolphin (Delphinus delphis) in the oceanic northeast Atlantic and comparison with its diet in neritic areas. Mar. Mammal Sci. 23, 30–47. doi: 10.1111/j.1748-7692.2006.00088.x
Ransijn J. M., Hammond P. S., Leopold M. F., Sveegaard S., Smout S. C. (2021). Integrating disparate datasets to model the functional response of a marine predator: a case study of harbour porpoises in the southern North Sea. Ecol. Evol. 11, 17458–17470. doi: 10.1002/ece3.8380
Redfern J., Ferguson M. C., Becker E., Hyrenbach K., Good C., Barlow J., et al. (2006). Techniques for cetacean–habitat modeling. Mar. Ecol. Prog. Ser. 310, 271–295. doi: 10.3354/meps310271
Redfern J. V., Moore T. J., Fiedler P. C., de Vos A., Brownell R. L., Forney K. A., et al. (2017). Predicting cetacean distributions in data-poor marine ecosystems. Diversity Distributions 23, 394–408. doi: 10.1111/ddi.12537
Reid J., Evans P., Northridge S. (2003). Atlas of cetacean distribution in north-west European waters (Peterborough, UK: Joint Nature Conservation Committee, Peterborough), 76pp.
Ringelstein J., Pusineri C., Hassani S., Meynier L., Nicolas R., Ridoux V. (2006). Food and feeding ecology of the striped dolphin, Stenella coeruleoalba, in the oceanic waters of the northeast Atlantic. J. Mar. Biol. Assoc. United Kingdom 86, 909–918. doi: 10.1017/S0025315406013865
Risch D., Castellote M., Clark C. W., Davis G. E., Dugan P. J., Hodge L. E., et al. (2014). Seasonal migrations of north Atlantic minke whales: novel insights from large-scale passive acoustic monitoring networks. Movement Ecol. 2, 24. doi: 10.1186/s40462-014-0024-3
Roberts J. J., Best B. D., Mannocci L., Fujioka E., Halpin P. N., Palka D. L., et al. (2016). Habitat-based cetacean density models for the US Atlantic and gulf of Mexico. Sci. Rep. 6, 22615. doi: 10.1038/srep22615
Rogan E., Breen P., Mackey M., Cañadas A., Scheidat M., Geelhoed S., et al. (2018). Aerial Surveys of Cetaceans and Seabirds in Irish waters: Occurrence, distribution and abundance in 2015-2017 (Dublin, Ireland: Tech. rep., Department of Communications, Climate Action and Environment and National Parks and Wildlife Service (NPWS), Department of Culture, Heritage and the Gaeltacht), 297pp.
Rogan E., Cañadas A., Macleod K., Santos M. B., Mikkelsen B., Uriarte A., et al. (2017). Distribution, abundance and habitat use of deep diving cetaceans in the north-east Atlantic. Deep Sea Res. Part II: Topical Stud. Oceanography 141, 8–19. doi: 10.1016/j.dsr2.2017.03.015
Sabatier E., Pante E., Dussud C., Van Canneyt O., Simon-Bouhet B., Viricel A. (2015). Genetic monitoring of pilot whales, Globicephala spp.(cetacea: Delphinidae), stranded on French coasts. Mammalia 79, 111–114. doi: 10.1515/mammalia-2013-0155
Santos M. B., Monteiro S. S., Vingada J. V., Ferreira M., López A., Martínez Cedeira J. A., et al. (2014). Patterns and trends in the diet of long-finned pilot whales (Globicephala melas) in the northeast Atlantic. Mar. Mammal Sci. 30, 1–19. doi: 10.1111/mms.12015
Santos M., Pierce G. (2003). The diet of harbour porpoise (Phocoena phocoena) in the northeast Atlantic. Oceanography Mar. Biology: an Annu. Rev. 41, 355–390.
Schoeman R. P., Patterson-Abrolat C., Plön S. (2020). A global review of vessel collisions with marine animals. Front. Mar. Sci. 7. doi: 10.3389/fmars.2020.00292
Spitz J., Cherel Y., Bertin S., Kiszka J., Dewez A., Ridoux V. (2011). Prey preferences among the community of deep-diving odontocetes from the Bay of Biscay, northeast Atlantic. Deep Sea Res. Part I: Oceanographic Res. Papers 58, 273–282. doi: 10.1016/j.dsr.2010.12.009
Spitz J., Rousseau Y., Ridoux V. (2006). Diet overlap between harbour porpoise and bottlenose dolphin: An argument in favour of interference competition for food? Estuarine Coast. Shelf Sci. 70, 259–270. doi: 10.1016/j.ecss.2006.04.020
Stephens G., Bas A. A., Hardy J., Awbery T., Rudd L., Abbiss L., et al. (2021). Sightings and stranding reports of fin whales (Balaenoptera physalus) in the Levantine Sea, with recent sightings from Turkey. J. Cetacean Res. Manage. 22, 55–60. doi: 10.47536/jcrm.v22i1.212
Unger B., Rebolledo E. L. B., Deaville R., Gröne A., IJsseldijk L. L., Leopold M. F., et al. (2016). Large amounts of marine debris found in sperm whales stranded along the North Sea coast in early 2016. Mar. pollut. Bull. 112, 134–141. doi: 10.1016/j.marpolbul.2016.08.027
Virgili A., Authier M., Boisseau O., Cañadas A., Claridge D., Cole T., et al. (2019). Combining multiple visual surveys to model the habitat of deep-diving cetaceans at the basin scale. Global Ecol. Biogeography 28, 300–314. doi: 10.1111/geb.12850
Virgili A., Authier M., Monestiez P., Ridoux V. (2018). How many sightings to model rare marine species distributions. PloS One 13, e0193231. doi: 10.1371/journal.pone.0193231
Virgili A., Teillard V., Dorémus G., Dunn T. E., Laran S., Lewis M., et al. (2022). Deep ocean drivers better explain habitat preferences of sperm whales Physeter macrocephalus than beaked whales in the Bay of Biscay. Sci. Rep. 12, 9620. doi: 10.1038/s41598-022-13546-x
Waggitt J. J., Evans P. G. H., Andrade J., Banks A. N., Boisseau O., Bolton M., et al. (2020). Distribution maps of cetacean and seabird populations in the north-east Atlantic. J. Appl. Ecol. 57, 253–269. doi: 10.1111/1365-2664.13525
Wall D., Murray C., O’Brien J., Kavanagh L., Wilson C., Ryan C., et al. (2013). Atlas of the Distribution and Relative Abundance of Marine Mammals in Irish Offshore Waters 2005-2011 (Merchants Quay, Kilrush, Co Clare, Ireland: Irish Whale & Dolphin Group).
Keywords: species distribution models, bottlenose dolphin Tursiops truncatus, common dolphin Delphinus delphis, fin whale Balaenoptera physalus, harbour porpoise Phocoena phocoena, Lagenorhynchus spp, minke whale Balaenoptera acutorostrata, pilot whales Globicephala spp
Citation: Virgili A, Araújo H, Astarloa Diaz A, Dorémus G, García-Barón I, Eira C, Louzao Arsuaga M, Laran S, Saavedra C, Van Canneyt O and Ridoux V (2024) Seasonal distribution of cetaceans in the European Atlantic and Mediterranean waters. Front. Mar. Sci. 11:1319791. doi: 10.3389/fmars.2024.1319791
Received: 11 October 2023; Accepted: 07 October 2024;
Published: 12 November 2024.
Edited by:
Mark Meekan, University of Western Australia, AustraliaReviewed by:
Peter Gausmann, Ruhr University Bochum, GermanyRoberto Carlucci, University of Bari Aldo Moro, Italy
Copyright © 2024 Virgili, Araújo, Astarloa Diaz, Dorémus, García-Barón, Eira, Louzao Arsuaga, Laran, Saavedra, Van Canneyt and Ridoux. This is an open-access article distributed under the terms of the Creative Commons Attribution License (CC BY). The use, distribution or reproduction in other forums is permitted, provided the original author(s) and the copyright owner(s) are credited and that the original publication in this journal is cited, in accordance with accepted academic practice. No use, distribution or reproduction is permitted which does not comply with these terms.
*Correspondence: Auriane Virgili, YXVyaWFuZUBzaGFyZXRoZW9jZWFuLmVhcnRo