- 1Department of Computer Science and Software Engineering, California Polytechnic State University, San Luis Obispo, CA, United States
- 2Department of Computer Science, San Francisco State University, San Francisco, CA, United States
- 3Scripps Institution of Oceanography, University of California, San Diego, San Diego, CA, United States
- 4National Ocean Service, National Oceanic and Atmospheric Administration, Silver Spring, MD, United States
- 5Department of Computer Science and Engineering, University of California, Santa Cruz, Santa Cruz, CA, United States
Rip currents present a significant safety risk to beach tourists and coastal communities, resulting in hundreds of annual drownings all over the world. A key contributing factor to this danger is the lack of awareness among beachgoers about recognizing and avoiding these rip currents. In response to this issue, we introduce RipFinder, a mobile app equipped with machine learning (ML) models trained to detect two types of rip currents. Users can leverage the app’s computer vision capabilities to use their phone’s camera to identify these hazardous rip currents in real time. The amorphous and ephemeral nature of rip currents makes it challenging to detect them with high accuracy using object detection models. To address this, we propose a client-server ML model-based computer vision system designed specifically to improve rip current detection accuracy. This novel approach enables the app to function with or without internet connectivity, proving particularly beneficial in regions without lifeguards or internet access. Additionally, the app serves as an educational resource, offering in-app information about rip currents. It also promotes citizen science involvement by encouraging users to contribute valuable information on detected rip currents. This paper presents the app’s overall design and discusses the challenges inherent to the rip current detection system.
1 Introduction
Rip currents are dangerous, strong, fast-moving currents that pull swimmers away from the shore, often leading to drownings and fatalities. They pose a significant hazard to beachgoers and can easily overpower even strong, experienced swimmers. Rip currents are a global issue, affecting coastlines around the world (Zhang et al., 2021; Retnowati et al., 2012; Mucerino et al., 2021). In the United States alone, they account for an estimated 100 drownings a year (Gensini and Ashley, 2010). Rip currents can form suddenly and without obvious signs, which can catch swimmers off guard. While there are general conditions that can lead to their formation, predicting exactly when and where they will appear is challenging. Furthermore, rip currents are created through various mechanisms and, as a result, exhibit different visual characteristics. This complexity of occurrence and variability in appearance makes them difficult to identify (Castelle et al., 2016). Consequently, many beachgoers lack the essential knowledge and awareness needed to recognize and avoid these perilous currents.
Rip current detection techniques are significantly important because of their potential to save lives. As a public safety issue, the implications extend beyond swimmers. Lifeguards, rescue teams, and even bystanders who try to help can also be put in danger. If rip currents could be detected reliably, then beachgoers and lifeguards could be alerted to the dangers in real-time. This would likely result in a significant decrease in the number of rip current-related incidents and fatalities. By providing more accurate information about rip currents, the general public could make more informed decisions about when it is safe to enter the water, thereby enhancing overall public safety. The development and deployment of tools, such as rip current prediction models (Dusek and Seim, 2013) or mobile apps that can detect and provide real-time alerts and tips about rip currents could be instrumental in these efforts.
While rip currents can often be visually identified by experienced swimmers, surfers, lifeguards, and coastal scientists, traditional detection and data collection methods typically involve in-situ instrumentation, such as GPS-equipped drifters and current meters (Leatherman, 2017; MacMahan et al., 2011). However, recent studies have demonstrated that images and video can also be used to detect rip currents. These approaches leverage computer vision and machine learning (ML) models for object detection to spot and identify these potentially dangerous phenomena (de Silva et al., 2021; Silva et al., 2023; Dumitriu et al., 2023; Maryan et al., 2019; Mori et al., 2022; Philip and Pang, 2016; Rampal et al., 2022; Rashid et al., 2021). However, detecting and segmenting rip currents with high accuracy using ML methods presents unique challenges due to their amorphous and ephemeral nature. Given the potentially fatal nature of dangerous rip currents, their detection is a matter of life and death. Thus, high accuracy and reliability are crucial for any rip current detection tool to issue warnings and take preventive actions to decrease the number of rip current-related incidents. Providing such capability for real-world use, i.e., on mobile platforms, adds another layer of technical challenge.
Many object detection ML models can detect rip currents, but the challenge lies in deploying these models in real-time on mobile devices with limited power and computational resources. More accurate yet computationally resource-intensive, ML models cannot run directly on mobile devices. By sending the visual input for object detection to a remote server, it can be achieved on mobile devices. However, this approach is not always feasible, especially in beach locations where server connectivity is unavailable. Alternatively, mobile-optimized ML models can feasibly run using the limited computational resources of portable devices without server connectivity but at the cost of sacrificing accuracy.
To address these challenges, we introduce a mobile application, or app, designed to detect rip currents using ML models for computer vision. Users can identify potential rip currents in real-time by simply aiming their phone’s camera toward the ocean. We propose a client-server system of object detection models to balance the trade-off between computational speed and accuracy. Depending on the mobile device’s available computational resources and internet connectivity, this app employs one or more ML models to identify rip currents. If the device is relatively new and has adequate computational resources, our app runs two different types of mobile-optimized ML models to enhance the reliability of rip current detection. For older, resource-constrained devices, only one ML model is used. Moreover, when internet connectivity is available, part of the visual data is transmitted to a server for further verification of the detection using a more accurate large model. Our system combines client-server architecture with multiple ML model-based computer vision to enhance the accuracy and reliability of rip current detection. The novelty of our solution lies in its implementation of this combined system, allowing the app to function both with and without internet connectivity. Our app’s versatility is especially invaluable in areas where lifeguards are absent or internet access is limited, establishing it as a crucial tool for public safety.
In addition to rip current detection, our app places a strong emphasis on educating users about the dangers of rip currents through informative in-app content and links to additional resources. Our aim is to empower beach enthusiasts with the knowledge necessary to make informed decisions, protecting themselves and others from these hazardous rip currents. Moreover, our app includes a citizen science feature, enabling users to contribute to scientific knowledge. This is done by encouraging them to record and share data, such as geotagged images and videos, along with additional information about detected rip currents. Harnessing the collective power of app users, we can gather valuable data that improves our understanding of rip currents and helps verify existing rip current forecast models. Ultimately, this leads to the development of more effective safety measures and strategies.
The contributions of this paper are as follows:
● Introduction of RipFinder: a mobile app designed for real-time, vision-based rip current detection.
● Development of a client-server system tailored for the ML models utilized in the rip current detection app.
● A comprehensive analysis and comparison of state-of-the-art ML models for rip detection.
2 Related work
2.1 Real-time object detection
Developing a mobile application for effectively and reliably identifying rip currents necessitates real-time object detection capabilities. Deep learning has revolutionized the field of object detection, as well as other computer vision tasks. Convolutional neural networks (CNNs) have become the standard method for these applications. Numerous large and intricate models, such as Faster R-CNN—a two-stage regionbased detector (Ren et al., 2015)—and DETR (Detection Transformers)—an object detector based on the Transformer architecture (Carion et al., 2020)—offer remarkable accuracy in object detection tasks. For instance, Faster R-CNN has been adeptly used for real-time object detection in drones by connecting to a remote GPU server (Lee et al., 2017). However, these detectors often bear significant computational complexity, rendering them difficult to deploy on mobile or embedded platforms for real-time performance. An earlier server-based system named Glimpse, offering continuous, real-time object recognition for mobile devices, was introduced by Chen et al. (2015). Nonetheless, server-reliant systems prove impractical in locations devoid of internet connectivity.
Achieving accurate and reliable real-time object detection on mobile devices without depending on servers presents inherent challenges. Numerous efforts have been directed toward integrating deep learning methods on mobile devices by creating compact, mobile-optimized ML models. Typically, streamlined architectures, like one-stage CNNs, render the models lightweight, allowing them to function swiftly on mobile devices—making them an ideal choice for real-time object detection. The primary compromise for such efficiency is a minor decrease in accuracy relative to their more elaborate counterparts (Huang et al., 2017). We scrutinized a range of mobile-optimized ML models to ascertain the best fit for our system. SSD-MobileNetV2 (Sandler et al., 2018) stood out as one of the earliest trustworthy models tailored for mobile platforms. Among the contemporary one-stage models refined for mobile devices are variants of RT-DETR (Lv et al., 2023), EfficientDet (Tan et al., 2020), and YOLO (Jocher et al., 2022). Our investigation encompassed a comprehensive evaluation of potential ML models suitable for real-time rip current detection using computer vision on mobile platforms.
2.2 Rip current detection with ML
Given its impact on public safety, the problem of automated rip current detection has been approached using various methods, some of which predate the emergence of deep learning techniques. For example, Philip and Pang (2016) utilized optical flow on video sequences to discern the predominant flow towards the sea, aiding human observers in rip current detection. Maryan et al. (2019) employed modified Haar cascade methods to detect rip currents from time-averaged images. The concept of rip current detection via deep learning-based methods is not entirely new either. de Silva et al. (2021) were among the early adopters of deep learning methods for rip current detection, employing Faster R-CNN, a large model that achieved high accuracy. They introduced a frame aggregation technique that bolstered detection accuracy for fixedposition cameras, but this technique was not suitable for moving cameras. Mori et al. (2022) offered a flow-based method to accentuate and depict rip currents for human observers. However, this approach also demands a stationary camera and serves as a visualization tool rather than an automated detection system. In recent years, there have been several scholarly works about new deep learning model-based rip current detection techniques. For instance, Rashid et al. (2021) and Zhu et al. (2022) presented RipDet and YOLO-Rip, respectively. These lightweight rip current detection models, rooted in Tiny-YOLOv3 and YOLOv5s, belong to the smaller members of the YOLO family and are adept for environments with limited computational power. Rampal et al. (2022) showcased that the mobile-optimized, single-stage model SSD-MobileNetV2 can achieve performance metrics comparable to Faster R-CNN. Furthermore, Dumitriu et al. (2023) explored and compared various iterations of YOLOv8 for rip current segmentation. Silva et al. (2023) unveiled RipViz, an innovation that examines 2D vector fields and interprets pathline behaviors to pinpoint rip currents. Like that of Dumitriu et al. (2023), this method highlights the rip region’s shape but identifies currents based on water movement rather than water appearance. Yet, while there is an assortment of effective rip current detection methods employing ML, a real-world application—such as a mobile app—primed for public safety and enhancing awareness for tangible societal impact remains elusive. This work endeavors to fill that void by devising a deployable mobile device-based real-time system for rip current detection.
3 System design and methods
3.1 System architecture
Figure 1 presents an overview of the RipFinder system architecture. Our comprehensive system, designed to effectively identify and alert users of rip currents, is organized into two primary components:
1. The client mobile app serves as the primary user interface. Within this app, we have integrated four ML models, each tailored specifically for mobile devices. As the device processes real-time visual input, these models evaluate the data and issue warnings if rip currents are detected. Depending on the device’s processing power, the app can deploy either one or two ML models for detection. More modern devices with substantial resources can utilize two types of mobile-optimized ML models simultaneously, enhancing the reliability of rip current detection. In contrast, older devices with limited resources might default to a single model. Nevertheless, the ultimate decision to use one or two models rests with the user. When feasible, the app suggests users employ two models for optimal detection, but they retain the freedom to choose only one from the available options if preferred.
2. Our system’s server-side employs complex ML models that demand significant computational resources and GPU capabilities, ensuring rip currents are detected with high accuracy. When a user captures an image or video via our mobile app, this data is sent to the server for in-depth analysis. After the server-side models process the data, the detection results are relayed back to the mobile app.
Additionally, we offer the option to execute multiple models on the server, depending on its capabilities (number of CPUs and GPUs, system memory, etc.), enhancing reliability through redundancy.
Our system attempts to improve the reliability of rip current detection in a two-fold way. The use of two models enhances detection reliability on the client app, even though it demands more computational resources. Server-side models, being complex and larger, boast superior accuracy, thus ensuring that server-aided rip current detection is more reliable when internet access is available. The client-side model, meanwhile, operates using the on-device computational resources without the need for an internet connection. The results section further elaborates on the justification behind these two design choices. Thus, our system’s design allows it to operate both online and offline.
Training datasets are essential for training both client-side and server-side ML models. We developed our dataset by utilizing the existing dataset from de Silva et al. (2021) and supplementing it with a large amount of our own data. Further details on the dataset and the ML model training process are explained in the implementation section.
3.2 Mobile apps
Figure 2 provides a visual representation of our mobile app’s user interface, offering an intuitive, user-friendly environment. We created both Android and iOS versions of the mobile app. The application’s design caters to a variety of user needs and includes the following features:
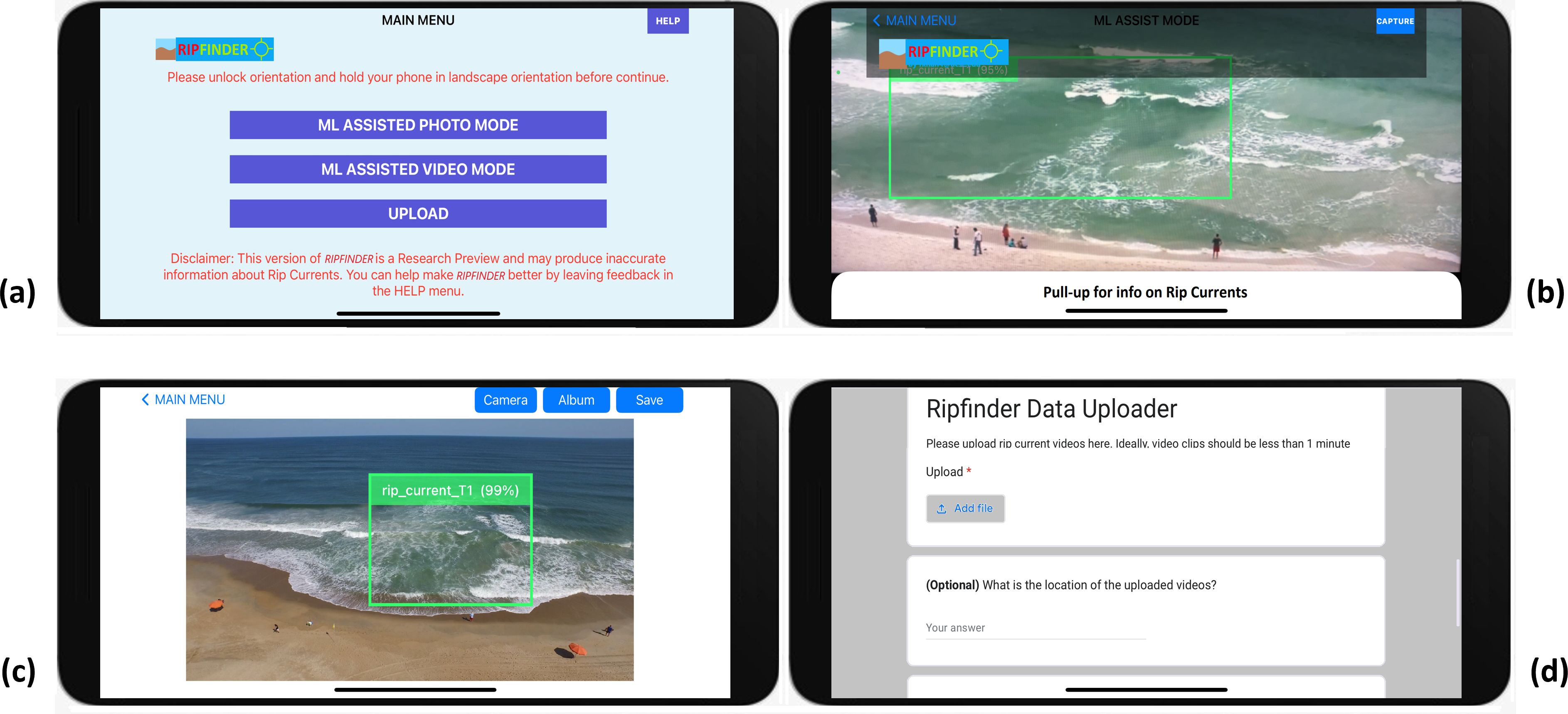
Figure 2. GUI of RipFinder App (a) Main menu, (b) Real-time detection from live camera view, (c) Detection from single image, (d) Data uploader for citizen science contribution.
3.2.1 Live camera and visualization tool
The app offers a live camera feature to capture the seashore and serves as a real-time visualizer, placing bounding boxes around detected rip currents in the view, thus acting as an immediate warning system (Figure 2b).
3.2.2 ML model selection
From the in-app menu, users can choose the ML model for real-time rip current detection. On devices with higher computational resources, users have the option to turn on or off the use of two models in parallel for increased reliability.
3.2.3 Image and video recording
The app enables real-time rip current detection and the recording of images and videos, letting users document and share potential rip currents with other beachgoers and rip current researchers.
3.2.4 Rip current detection tool for existing images
RipFinder app analyzes existing images on the phone to identify rip currents, offering retrospective insights to users (Figure 2c).
3.2.5 Educational resources
Our app features an educational hub with resources on rip currents, accessible via a pull-up menu and help menu, ensuring users always have information at hand (Figure 2b).
3.2.6 Data upload tool
We integrated a data upload tool (Figure 2d) for users to share geotagged rip current images and observations, fostering community collaboration and enhancing our dataset for improved algorithm refinement.
3.3 Client-side ML models
In our application, RipFinder, we integrate several mobile-optimized ML models, all trained on a rip current dataset for client-side detection. These models have been tailored to ensure swift and efficient performance on mobile devices, which facilitates real-time rip current detection. The current version of RipFinder incorporates the following models:
3.3.1 YOLOv8n and YOLOv8m
YOLOv8, the latest in the YOLO series known for fast object detection (Redmon et al., 2016; Jocher et al., 2023), includes variants like YOLOv8n (nano) and YOLOv8m (medium) optimized for mobile devices. Its architecture facilitates single-pass detections, making it ideal for real-time applications such as rip current detection.
3.3.2 EfficientDet D0 and EfficientDet D2
EfficientDet, known for its object detection prowess (Tan et al., 2020), has a unique scalable architecture that adjusts to computational resources, making it ideal for mobile use; it offers eight variants, D0 to D7, based on image size.
Of the four ML models at our disposal, the app selects one or two mobile-optimized models for rip current detection, contingent upon a device’s computational prowess and internet connectivity. Modern, high-end devices employ two models, while the older, resource-constrained devices resort to just one. YOLOv8n and EfficientDet D0, due to their lesser computational demand, are ideally deployed as standalone models or in conjunction with dated or less competent mobile devices. In contrast, YOLOv8m and EfficientDet D2 are better aligned with newer devices boasting significant computational strength.
3.4 Server-side ML models
Server-side, we engage a collection of high-performance ML models tailored for more resource-intensive computations. Given their demanding computational needs, these models are perfectly positioned for server-side deployment, capitalizing on robust hardware resources, including GPUs. For the server side, we’ve selected:
3.4.1 YOLOv8l and YOLOv8x
The YOLOv8 ‘l’ (large) and ‘x’ (extra-large) variants (Jocher et al., 2023) are more complex than their mobile-optimized versions, offering higher accuracy but requiring greater computational power, ideal for situations demanding utmost accuracy with ample resources.
3.4.2 Real-time detection transformer
RT-DETR, a real-time adaptation of the DETR transformer-based object detection model (Lv et al., 2023; Carion et al., 2020), maintains DETR’s accuracy while ensuring faster performance. We trained its large and extra-large versions, RT-DETR-L and RT-DETR-X, for server-side use.
By leveraging these server-side models that can deliver high accuracy, we bolster the final verification of detected rip currents, reinforcing the reliability of our rip current detection tool.
4 Implementation
Various components of our system were implemented using the latest available technology.
4.1 Dataset
Our training dataset distinguishes between two types of rip currents based on their visual features. The first, termed bathymetry-controlled rip, is characterized by areas devoid of breaking waves, presenting as darker and calmer regions flanked by brighter waves. The second, known as transient rip, is identified by water discoloration due to sediment plumes that extend beyond the breaking waves. Though both classes represent rip currents, their visual features differ significantly. Detecting one type of rip current with an ML model trained on data from another type is unfeasible. Treating these two types as a single class compromises the effectiveness of the trained model. The label correlograms in the Figure 3 illustrate the distinctions between the two classes based on the labeled regions of images from each class.
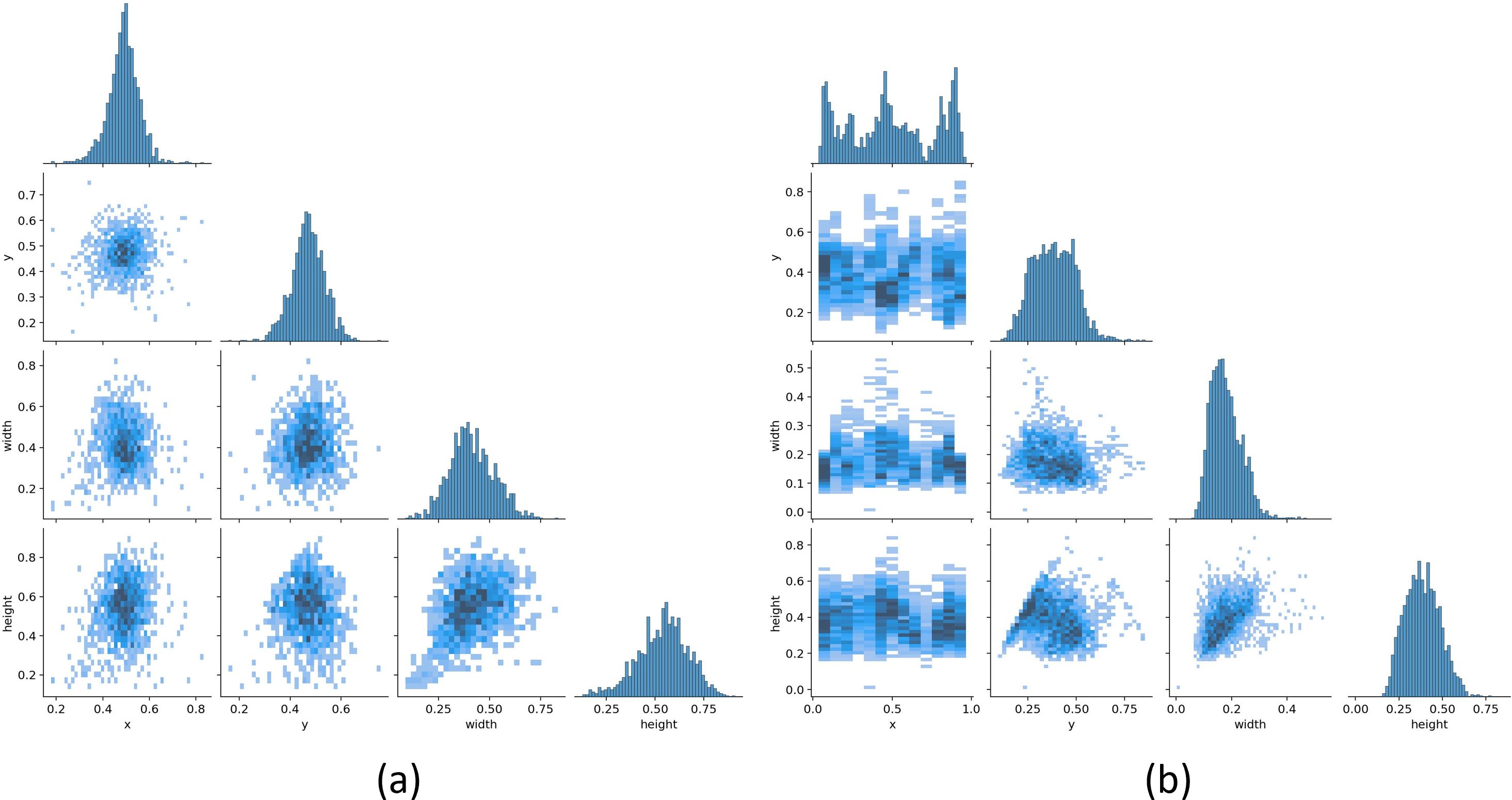
Figure 3. The label correlograms for (a) bathymetry controller rips and (b) transient rips, depicted in the figure, illustrate the distinctions between the two classes based on the labeled regions of images from each class.
For the bathymetry-controlled rip current category, we utilized a dataset consisting of 1780 images made publicly available by de Silva et al. (2021). For the transient rip current category, we curated a new dataset comprising 7565 labeled images. These were selectively extracted from videos captured by a drone, which focused on the visual signature of transient rip currents, and a Wi-Fi camera set up specifically for monitoring rip currents. We combined both datasets to train our model in the detection of the two rip current types. This dataset was then divided into an 80:20 split for training and validation, with 80% allocated for training purposes and the remaining 20% used for validation. The efficacy of the trained models was assessed using a series of test videos. Figure 4 showcases a selection of images from our dataset.
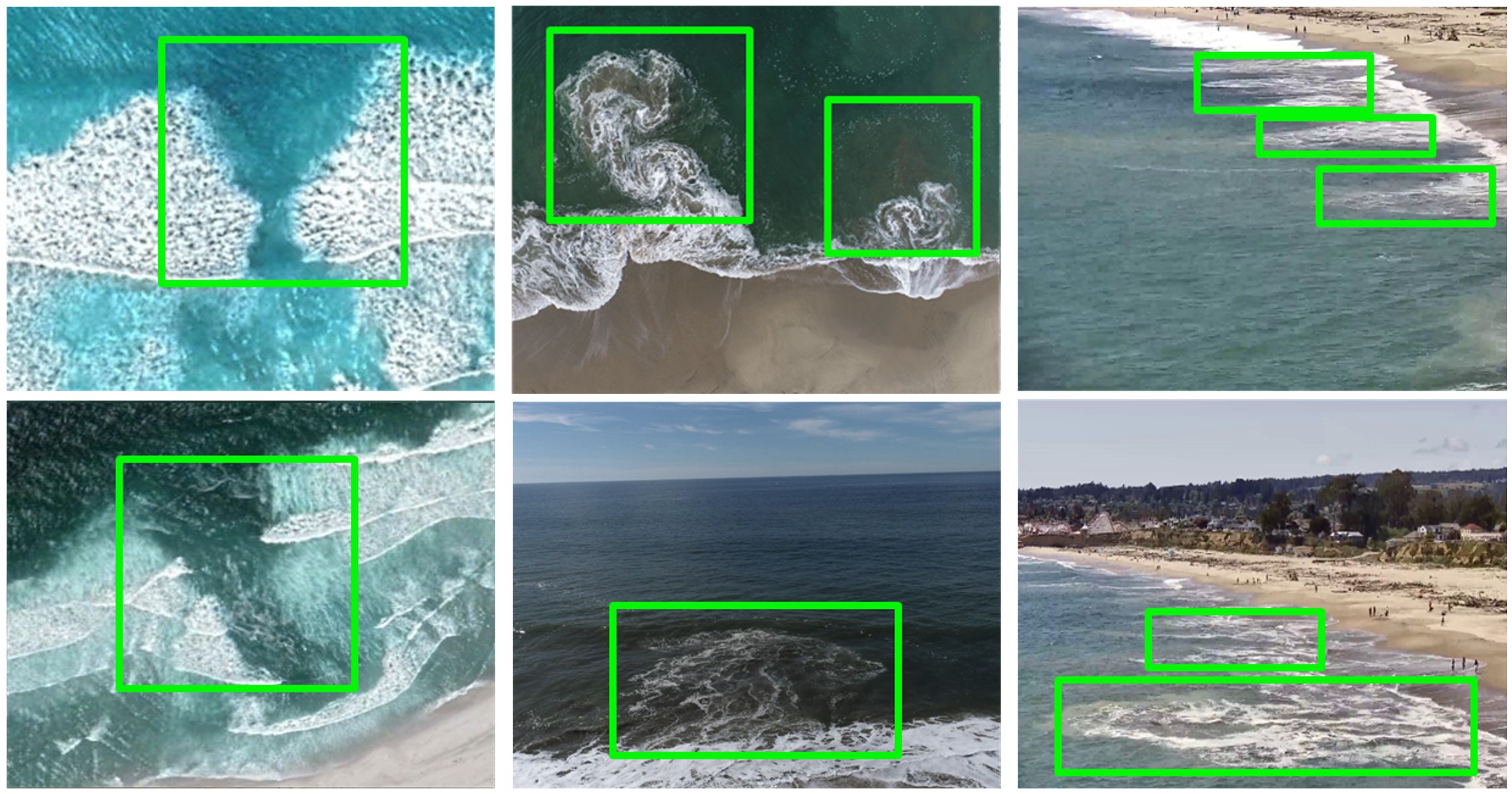
Figure 4. Some examples from our training dataset. The images on the first column are from the dataset by de Silva et al. (2021). The images on the second and third columns are from the dataset we collected using a drone and a wireless rip activity monitoring camera, respectively.
It is important to include imagery from diverse geographic regions and environmental conditions to enhance model robustness. Our dataset includes images from publicly available sources, drone footage, and fixed-location cameras. We incorporated the de Silva et al. (2021) dataset, which features satellite imagery from diverse regions. To enhance generalization, we are collaborating with coastal research partners to expand data collection across varied wave conditions, lighting, and water characteristics. Additionally, our citizen science initiative allows users to contribute images, enriching the dataset. While expanding the dataset and refining models is an ongoing effort, it remains independent of RipFinder’s core architecture, as the ML models can be continuously updated with improved datasets.
4.2 ML model training and evaluation
We conducted ML model training on an AWS cloud server equipped with eight vCPUs, 61 GB of memory, and an NVIDIA Tesla V100 GPU boasting 16 GB of video memory. The EfficientDet models were trained using the TensorFlow library, while the YOLOv8 and RT-DETR models were trained with the Ultralytics library, which is based on PyTorch. All model trainings were initialized with a maximum of 500 epochs. For all versions of YOLOv8 and RT-DETR, a patience parameter of 50 was set. The patience parameter defines the number of epochs to wait before halting training via early stopping if there’s no improvement in performance on a validation dataset. Since the EfficientDet models do not allow for the definition of a patience parameter, we monitored convergence through TensorBoard and manually terminated the training once convergence was observed. All models converged within 300 epochs. We trained all models from scratch, instead of using transfer learning with MS COCO pretrained models from the ML libraries, to prevent negative transfer (Wang et al., 2019). This decision was made because our rip current class data domain is distinct from any of the classes in the MS COCO2017 dataset (Lin et al., 2014).
4.3 Client apps and server
We developed the iOS version of the app in Swift using Xcode, and wrote the Android version in Java with Android Studio. To ensure broad accessibility, we tested the RipFinder app on a wide range of mobile devices, including both high-end and low-end models. While Table 1 presents results from the iPhone 12 Pro (2020) and Google Pixel 6 (2021), which served as our primary development devices, we also validated the app’s performance on older and more budget-friendly models such as the Samsung A50 (2019), Samsung S23 (2023), LG G3 (2014), LG G5 (2016), and Xiaomi Redmi 10A (2022), and older iPhones such as the iPhone XR (2018). This extensive testing confirms that the app performs efficiently across a diverse spectrum of hardware, greatly enhancing its real-world applicability.
The server-side components were programmed in Python. We evaluated the server-side ML models on a desktop server equipped with a 16-core Intel Core i9 3.2 GHz CPU, 30 GB of memory, and an NVIDIA RTX3080 GPU with 10 GB of video memory.
4.4 Data privacy and security measures
Ensuring data privacy and security is a core aspect of RipFinder, particularly for citizen science contributions. All uploaded images, videos, and metadata are encrypted to prevent unauthorized access. Personally identifiable information is anonymized before storage, and location data is collected only with user consent, then obscured or aggregated to prevent tracking. We adhere to institutional ethical guidelines and restrict data access to authorized researchers who validate contributions. Users receive clear terms of use and can request data removal. Our retention policies prevent unnecessary long-term storage, ensuring responsible data handling while supporting rip current research.
4.5 Quality control and validation of user-uploaded data
To ensure the accuracy and reliability of citizen science contributions, RipFinder employs a multi-step validation process combining automated filtering, metadata verification, and expert review.
4.5.1 Automated screening and metadata verification
All user-uploaded images and videos first undergo computer vision-based pre-screening, which filters out irrelevant or low-quality submissions. Additionally, metadata, such as location, timestamp, and environmental conditions, is cross-referenced with rip current forecasts from NOAA and other sources. Any inconsistencies flag submissions for further review.
4.5.2 Expert validation and continuous improvement
Flagged submissions undergo manual review by rip current specialists, including NOAA scientists and coastal researchers, ensuring only verified data is incorporated into the dataset. A continuous feedback loop refines detection accuracy by improving machine learning models over time. Verified contributors may also receive recognition, fostering quality participation.
By integrating automated detection with expert validation, RipFinder ensures that only high-confidence, research-grade data supports scientific analysis and rip current safety efforts.
5 Results and discussion
5.1 Performance analysis of ML models
In this section, we present a performance analysis and comparison of state-of-the-art (SOTA) object detection models tailored for rip current detection. We compared ML models including EfficientDet D0, EfficientDet D1, EfficientDet D2, YOLOv8n, YOLOv8s, YOLOv8m, YOLOv8l, YOLOv8x, RT-DETR-l, and RT-DETR-x. To gauge the accuracy of these models, we utilized nine test videos annotated with ground truth data. To ensure model generalization and robustness, we validated RipFinder using diverse test videos from independent sources. Four of these videos were selected for their relevance to our rip current detection objectives from the test set introduced by de Silva et al. (2021). Additionally, three videos were drone-captured by us, while the last two originated from a wireless camera at webcoos.org dedicated to rip current monitoring.
While our model validation primarily utilized video data captured from elevated perspectives, we acknowledge that real-world user applications will often involve videos recorded at ground level. However, rip currents exhibit distinct visual characteristics that remain detectable even from a beach-level viewpoint. Lifeguards and experienced swimmers routinely identify rip currents using precisely these visual cues. With appropriate training datasets, ML models can similarly leverage these visual indicators to detect rip currents.
To evaluate the effectiveness of our object detection models, we use Intersection over Union (IoU) as the primary performance metric. Our evaluation methodology follows the object detection benchmarking approach outlined by Padilla et al. (2020), which provides a standardized toolbox for computing IoU. This toolbox calculates the ratio of overlap between the predicted and ground truth bounding boxes, allowing for a precise and objective assessment of detection accuracy.
Unlike classification tasks that rely on confusion matrices, object detection inherently requires spatial accuracy in addition to detection presence. IoU directly accounts for true positives, false positives, and localization precision, making it a more suitable metric for this study. Given the established use of IoU in object detection benchmarks, additional metrics such as precision, recall, and F1 score are not required to support our results and would be redundant in this context.
Our accuracy assessment followed the methodology described by de Silva et al. (2021), where:
Frames were considered classified as correct if the detected bounding boxes had an Intersection over Union (IoU) score versus ground truth bounding boxes above 0.3. IoU is calculated as:
The comparison results are presented in Table 2, and some examples of detected rip currents are shown in Figure 5. Based on these results, we can justify the following two design choices we made.
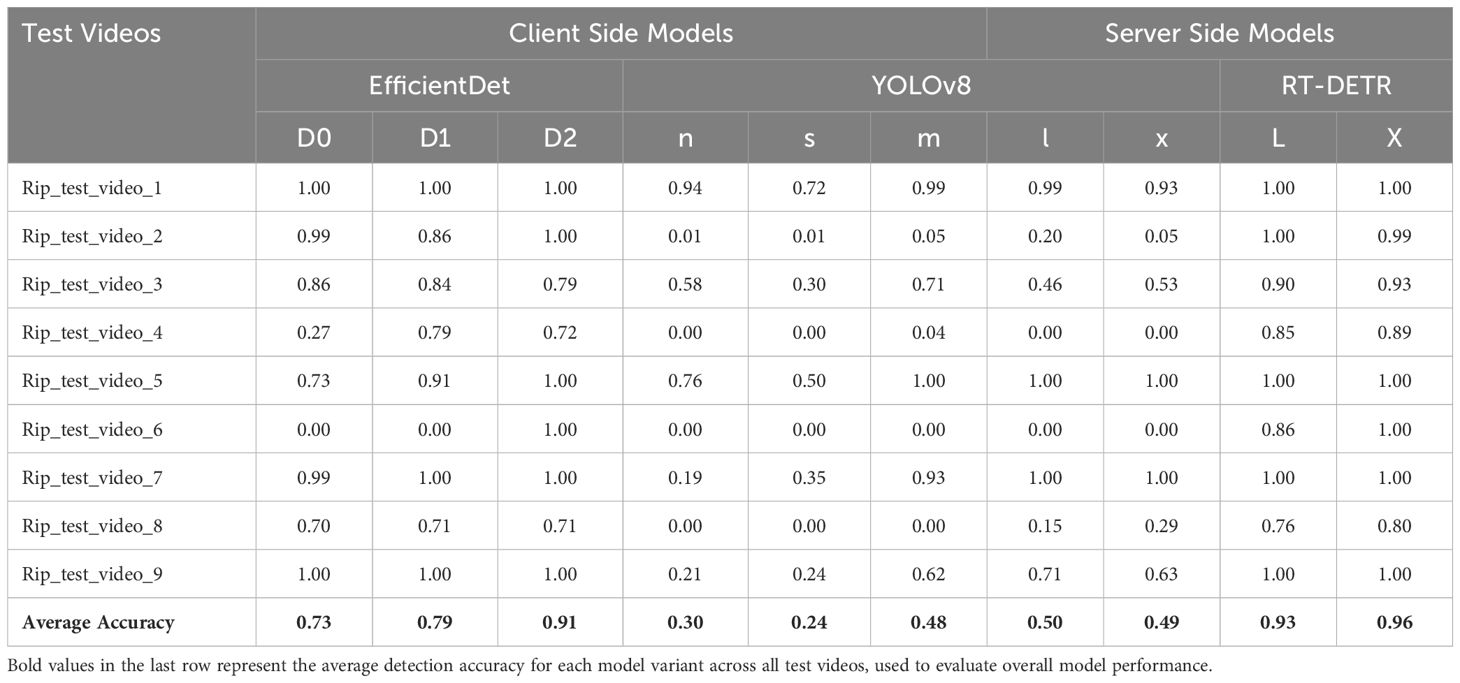
Table 2. We compared the detection accuracy of the SOTA methods to select the best options for the client and server application.
5.2 Statistical analysis of model performance
While the per-video accuracy results in Table 2 offer a general comparison, we further examined whether the observed accuracy differences among models are statistically meaningful. We treated each of the nine test videos as repeated measurements, one “sample” per model, yielding paired accuracy data for each model across the same videos. Below, we illustrate a straightforward method using 95% confidence intervals (CIs) for each model’s mean accuracy. These intervals help gauge whether model performance truly differs on average or if apparent differences could be due to sampling variability Rainio et al. (2024).
The mean accuracy of each model was computed by averaging the detection accuracy across all test videos. The standard deviation was calculated to measure the variability in performance across different video samples. The standard error of the mean was calculated as:
To quantify the uncertainty in these estimates, we determined the 95% confidence interval (CI). We used a two-sided t-distribution (given the small sample size) with 8 degrees of freedom (n − 1 = 9 − 1) and a critical value of ≈ 2.306 for 95% confidence:
A higher-variance model yields a wider interval, possibly overlapping intervals of both stronger and weaker performers. Large differences in means with minimal interval overlap typically point to genuine performance gaps, but borderline cases call for further pairwise statistical testing (e.g., with multiplecomparison corrections).
Table 3 reports the mean accuracy, standard deviation, and 95% CIs for each model. Although the average accuracies match Table 2, the confidence intervals offer insight into the consistency of each model’s performance across videos.
5.2.1 Findings and interpretation
From the statistical analysis, the RT-DETR-X model achieved the highest mean accuracy (µ = 0.96) with a very narrow confidence interval (CI = [0.91,1.00]), indicating consistent and highly reliable performance. Similarly, RT-DETR-L (µ = 0.93) and EffDet-D2 (µ = 0.91) demonstrated high accuracy with relatively low variability, confirming their robustness for rip current detection. Conversely, YOLOv8n, YOLOv8s, and YOLOv8m exhibited the lowest mean accuracies and the widest confidence intervals, reflecting high variability and inconsistent detection performance. The EffDet-D0 and EffDet-D1 models, while moderately accurate, showed greater performance fluctuations due to their wider confidence intervals.
5.2.2 Implications for model selection
The statistical findings reinforce the rationale behind selecting EffDet-D2 and YOLOv8n for mobile deployment, as they balance accuracy and efficiency. Meanwhile, RT-DETR-L and RT-DETR-X were the most reliable server-side models, offering superior accuracy with minimal variability. These insights confirm that our chosen client-server hybrid approach effectively optimizes both computational efficiency and real-time detection performance. By incorporating statistical validation, we ensure that model selection is based on empirical evidence rather than raw accuracy alone. This strengthens the reliability of RipFinder as a robust and scientifically validated rip current detection tool.
5.3 Other considerations
5.3.1 Running two ML models to increase accuracy
While running multiple models demands more computational resources, it enhances reliability. This design decision stems from the understanding that ML models with varying architectures possess distinct strengths and shortcomings. Research by Mekhalfi et al. (2022) indicates that models from the YOLO family tend to identify more objects, even if their precision varies. In contrast, EfficientDet provides more stable and accurate detection. In many cases, one of the models might not detect specific instances of rip currents, even if they were trained using the same data. For instance, although the rip current in “Rip test video 6” can be detected by EfficientDet D2, it isn’t identified by any other mobile models. Thus, deploying two models ensures that a challenging-to-detect rip current is more likely to be detected on a more capable device. Additionally, since rip current detection pertains to safety, minimizing false negatives is more crucial than avoiding excessive false positives. Therefore, while employing two models might seem redundant for general applications, it is beneficial for the purpose of rip current detection.
5.3.2 Two models vs. three or more
The decision to use two models on the client side balanced accuracy, computational demands, and processing time. Although running more than two models could improve detection accuracy through ensemble techniques, the benefits were minimal compared to the significant increase in resource consumption and latency.
Additional models would heavily strain server CPU and GPU resources, leading to higher costs and potential delays during peak usage. Increased latency from more models would compromise real-time detection, critical for user safety. The two-model setup already offers robust redundancy, ensuring reliable detection even if one model underperforms. The combination of YOLOv8l for broad detection and RT-DETR-L for detailed analysis provides a well-rounded solution.
After evaluating various models, EfficientDet-D2 and YOLOv8n were selected for mobile deployment due to their optimal balance of speed, accuracy, and compact size. For server-side operations, YOLOv8l and RT-DETR-L were chosen to maximize accuracy and reliability, enabling effective online and offline functionality. The findings, summarized in Table 1, highlight models that meet both hardware constraints and application needs for proficient rip current detection.
5.3.3 Running ML models on both the client and server side
More advanced and complex models, such as RT-DETR-L and RT-DETR-X, achieve higher accuracy but are limited to server execution. Thus, when an internet connection is available, server-assisted rip current detection becomes more reliable. The client-side models serve as the primary object detection mechanism, ensuring that rip current detection operates at the highest possible accuracy both with and without internet connectivity.
5.4 Evaluation and model selection
5.4.1 Addressing detection bias
Different machine learning models exhibit varying performance across rip current types, leading to detection bias in some cases. For example, EfficientDet-D0 struggles with transient rip currents, showing a higher false-negative rate. This discrepancy arises due to differences in model architectures, feature extraction capabilities, and training data distribution. Models optimized for certain visual cues, such as wave breaks in bathymetry-controlled rips, may not generalize as well to transient rips, which often exhibit diffuse, sediment-laden water patterns.
Rather than refining a single model, RipFinder employs a multi-model strategy to balance detection accuracy and computational efficiency. This approach ensures adaptability, allowing the system to leverage mobile-optimized models for real-time detection while utilizing more powerful server-side models when internet access is available. Table 2 compares model performance, highlighting trade-offs between accuracy, speed, and resource constraints.
While this work prioritizes flexibility over single-model optimization, we recognize the importance of improving individual model performance. Future efforts will focus on fine-tuning models using more diverse datasets and reducing false negatives in challenging conditions. By continuously integrating improved architectures and expanded training data, RipFinder will further enhance detection reliability for all rip current types.
5.4.2 Evaluation
Among the ten (10) models highlighted in Table 2, we chose eight (8) for further evaluation. From the less accurate EfficientDet D0 and D1 variants, we selected only D0 because of smaller size. YOLOv8s was similarly excluded due to its poor accuracy. We evaluated the chosen models on a server equipped with a single GPU, an iPhone 12 Pro, and a Google Pixel 6 to determine the best-fit models for each platform (Table 1). Our benchmarking of each model’s performance focused on two primary metrics:
1. We evaluated the real-time responsiveness of each model by measuring the frames processed per second (FPS). This metric offers insights into the model’s speed and its ability to detect rip currents in real-time scenarios. EfficientDet-D0 and YOLOv8n exhibited higher FPS on mobile devices, marking them as optimal choices for devices with limited computational capabilities. Meanwhile, the enhanced accuracy of EfficientDet-D2 makes it a reliable option while still maintaining real-time performance.
2. Each model’s storage footprint needs to be considered for embedding them in a mobile app, given that mobile devices have diverse storage capabilities and may also be running other apps simultaneously. Assessing a model’s storage needs ensures that the application remains streamlined and does not overtax the device’s memory. While the compactness of EfficientDet-D0 and YOLOv8n makes them as ideal for devices with resource constraints, the relatively small size and superior performance of EfficientDet-D2 make it a trustworthy option.
To further validate the practical applicability of our system, we extended our device testing to include low-end and older Android models. While certain high-end devices demonstrated superior performance, models such as the Xiaomi Redmi 10A and Samsung A50 successfully ran RipFinder, demonstrating that the app is not solely dependent on flagship devices.
5.5 Model performance evaluation
5.5.1 EfficientDet-D0 and D2
EfficientDet-D0 was notable for its high FPS, making it responsive on mobile devices, but it sometimes struggled with detecting transient rip currents in complex backgrounds, leading to occasional false negatives. On the other hand, EfficientDet-D2, while slightly slower, offered higher accuracy in distinguishing rip currents from similar water patterns, making it a more reliable choice for detailed analysis despite its larger storage requirements.
5.5.2 YOLOv8 variants
YOLOv8n excelled in real-time performance due to its compact size and speed, effectively detecting well-defined rip currents but occasionally missing subtler ones. YOLOv8m balanced speed and accuracy, handling both bathymetry-controlled and transient rip currents consistently, making it suitable for mobile deployment. The larger YOLOv8l and YOLOv8x models used server-side provided superior accuracy, detecting even faint rip currents, though their size and computational demands restricted them to server environments. YOLOv8s was excluded due to poor accuracy, particularly in complex scenarios.
5.5.3 RT-DETR variants
RT-DETR-L and RT-DETR-X, designed for server use, offered high accuracy and reliability, excelling in differentiating rip currents from similar patterns like wave shadows and sandbars. Their complex architecture required substantial computational resources, making them suitable only for server-side deployment.
6 Limitations and future work
While RipFinder is designed to improve rip current detection using diverse datasets and a hybrid clientserver architecture, certain limitations remain.
6.1 Dataset scope and generalization
Our dataset includes rip current images from multiple independent sources, such as NOAA, coastal research partners, and public sources (de Silva et al., 2021; Mori et al., 2022). However, we recognize that geographic and environmental variations may still impact model generalization, particularly in detecting rip currents under unique wave conditions or in less studied coastal regions. To mitigate these effects and improve generalization, we are:
● Expanding the dataset by incorporating images from diverse geographic locations and environmental conditions.
● Using data augmentation techniques, such as lighting adjustments, resolution scaling, and viewpoint shifts, to simulate different acquisition conditions.
● Leveraging citizen science contributions to introduce more real-world variability, ensuring models encounter a wider range of rip current appearances.
Future work will include a systematic evaluation of model generalization across different data sources and acquisition methods to further reduce bias and improve detection accuracy in real-world applications.
6.2 Server dependency and offline functionality
The client-server hybrid architecture enhances detection accuracy by leveraging more powerful models on the server. However, we acknowledge that server dependency may limit real-time detection in areas with poor or no internet connectivity. To mitigate this, RipFinder is designed to function independently using on-device models, ensuring continued usability in offline scenarios, although with a trade-off in detection accuracy.
6.3 Potential biases in model training
Training data biases may influence model performance, particularly in detecting less common rip current types. To improve fairness and generalizability, we plan to conduct further bias analysis, integrate domain adaptation techniques, and continuously refine the dataset to address potential imbalances.
6.4 Robustness in complex marine environments
Rip current detection is inherently challenging in extreme conditions, such as strong waves, light variations, and surface reflections. While multi-model detection improves reliability, some edge cases remain difficult to classify. Detection failures often occur when transient rips blend into background wave activity, making them harder to distinguish. As RipFinder is model-agnostic, future iterations can integrate more advanced models specifically trained for challenging marine conditions. Additionally, ongoing data collection through citizen science contributions will help refine model generalization, ensuring greater robustness over time.
6.5 Real-world usability from beach-level perspective
Another key consideration is the real-world usability of the app when deployed by users at beach level rather than from an elevated viewpoint. Although our current dataset primarily includes images captured from drones and other high vantage points, we recognize the importance of validating detections from ground-level perspectives. Future work will involve expanding our dataset to incorporate user-submitted images and videos captured at beach level, enabling the machine learning models to generalize more effectively across various viewing angles. Additionally, we plan to implement citizen-science feedback loops to continuously refine model accuracy based on real-world user data.
7 Conclusion
In this paper, we introduce Ripfinder, a mobile app equipped with an ML-based computer vision tool designed to mitigate the safety hazards associated with rip currents, which are a leading cause of drownings globally. Ripfinder features a sophisticated system that ensures rip current detection even in the absence of internet connectivity, making it indispensable in regions without lifeguards or reliable internet coverage. This capability is crucial for enhancing beach safety in remote and underserved areas.
Beyond its detection capabilities, Ripfinder enriches user knowledge with in-app informational content and videos about rip currents, helping users understand the dangers and how to avoid them. This educational component is vital for raising awareness and promoting safe behaviors at the beach. A standout feature of Ripfinder is its inclusion of citizen science. By inviting users to share data about identified rip currents, the app not only enhances scientific understanding but also fosters community engagement. This participatory approach leverages the collective efforts of users to contribute valuable data that can be used for further research and analysis, ultimately improving the overall understanding of rip current patterns and behaviors.
Ripfinder’s integration of public safety, education, and scientific progress underscores its multifaceted approach to ensuring safer beach outings. By combining advanced technology with user engagement and educational resources, Ripfinder aims to create a comprehensive solution that addresses both immediate safety concerns and long-term scientific goals. The app exemplifies how modern technology can be harnessed to address real-world problems, making beaches safer and more enjoyable for everyone.
Data availability statement
The raw data supporting the conclusions of this article will be made available by the authors, without undue reservation.
Author contributions
FK: Conceptualization, Data curation, Formal analysis, Investigation, Methodology, Resources, Software, Validation, Visualization, Writing – original draft, Writing – review & editing. AdS: Conceptualization, Data curation, Validation, Writing – original draft, Writing – review & editing. AsP: Funding acquisition, Project administration, Resources, Supervision, Validation, Writing – original draft, Writing – review & editing. GD: Funding acquisition, Investigation, Project administration, Resources, Supervision, Writing – original draft, Writing – review & editing. JD: Conceptualization, Formal analysis, Investigation, Supervision, Visualization, Writing – original draft, Writing – review & editing. AlP: Conceptualization, Data curation, Formal analysis, Funding acquisition, Investigation, Methodology, Project administration, Resources, Supervision, Validation, Writing – original draft, Writing – review & editing.
Funding
The author(s) declare that financial support was received for the research and/or publication of this article. This work is partially funded by the following grants: Southeast Coastal Ocean Observing Regional Association (SECOORA) sub-award from NOAA award number NA20NOS0120220, the US Coastal Research Program (USCRP) through a Sea Grant award number NA23OAR4170121, and a grant from the UCSC Center for Coastal Climate Resilience.
Acknowledgments
We are grateful for the support and permissions granted by the California State Parks System and the Monterey Bay National Marine Sanctuary to operate our drone for this and related research. We also thank the Santa Cruz Port District, the California State Park Lifeguards and the other beta testing participants for their invaluable assistance in evaluating our system and providing feedback to help us improve RipFinder. We also acknowledge the support from the Google Cloud Research Credits program and AWS Cloud Credit for Research.
Conflict of interest
The authors declare that the research was conducted in the absence of any commercial or financial relationships that could be construed as a potential conflict of interest.
Generative AI statement
The author(s) declare that Generative AI was used in the creation of this manuscript. Grammarly and GPT-4 was used in order to improve readability and language during the preparation of this work. After using these tools, we reviewed and edited the content as needed and take full responsibility for the content of the publication.
Publisher’s note
All claims expressed in this article are solely those of the authors and do not necessarily represent those of their affiliated organizations, or those of the publisher, the editors and the reviewers. Any product that may be evaluated in this article, or claim that may be made by its manufacturer, is not guaranteed or endorsed by the publisher.
Author disclaimer
The statements, findings, conclusions, and recommendations are those of the authors and do not necessarily reflect the views of SECOORA, NOAA, or UCSC. The content of the information provided in this publication does not necessarily reflect the position or the policy of the government, and no official endorsement should be inferred.
References
Carion N., Massa F., Synnaeve G., Usunier N., Kirillov A., and Zagoruyko S. (2020). “End-to-end object detection with transformers,” in European conference on computer vision (Glasgow, UK: Springer), 213–229.
Castelle B., Scott T., Brander R., and McCarroll R. (2016). Rip current types, circulation and hazard. Earth Sci. Rev. 163, 1–21. doi: 10.1016/j.earscirev.2016.09.008
Chen T. Y.-H., Ravindranath L., Deng S., Bahl P., and Balakrishnan H. (2015). “Glimpse: Continuous, real-time object recognition on mobile devices,” in Proceedings of the 13th ACM Conference on Embedded Networked Sensor Systems (Association for Computing Machinery, SenSys ‘15, New York, NY, USA), 155–168. doi: 10.1145/2809695.2809711
de Silva A., Mori I., Dusek G., Davis J., and Pang A. (2021). Automated rip current detection with region based convolutional neural networks. Coastal Eng. 166, 103859. doi: 10.1016/j.coastaleng.2021.103859
Dumitriu A., Tatui F., Miron F., Ionescu R. T., and Timofte R. (2023). “Rip current segmentation: A novel benchmark and YOLOv8 baseline results,” in Proceedings of the IEEE/CVF conference on computer vision and pattern recognition. (Vancouver, BC, Canada: IEEE) 1261–1271.
Dusek G. and Seim H. (2013). A probabilistic rip current forecast model. J. Coastal Res. 29, 909–925. doi: 10.2112/JCOASTRES-D-12-00118.1
Gensini V. A. and Ashley W. S. (2010). An examination of rip current fatalities in the United States. Natural Hazards 54, 159–175. doi: 10.1007/s11069-009-9458-0
Huang J., Rathod V., Sun C., Zhu M., Korattikara A., Fathi A., et al. (2017). “Speed/accuracy trade-offs for modern convolutional object detectors,” in Proceedings of the IEEE Conference on Computer Vision and Pattern Recognition (CVPR). (Honolulu, Hawaii: IEEE), 7310–7311.
Jocher G., Chaurasia A., Stoken A., Borovec J., Kwon Y., Michael K., et al. (2022). ultralytics/yolov5: v7.0 - YOLOv5 SOTA Realtime Instance Segmentation (Geneva, Switzerland: Zenodo).
Leatherman S. B. (2017). Rip current measurements at three south florida beaches. J. Coastal Res. 33, 1228–1234. doi: 10.2112/JCOASTRES-D-16-00124.1
Lee J., Wang J., Crandall D., Šabanović S., and Fox G. (2017). “Real-time, cloud-based object detection for unmanned aerial vehicles,” in 2017 First IEEE International Conference on Robotic Computing (IRC) (Taichung, Taiwan: IEEE), 36–43. doi: 10.1109/IRC.2017.77
Lin T.-Y., Maire M., Belongie S., Hays J., Perona P., Ramanan D., et al. (2014). “Microsoft coco: Common objects in context,” in European conference on computer vision (Zurich, Switzerland: Springer), 740–755.
Lv W., Xu S., Zhao Y., Wang G., Wei J., Cui C., et al. (2023). DETRs beat YOLOs on real-time object detection. (Seattle, Washington: IEEE).
MacMahan J., Reniers A., Brown J., Brander R., Thornton E., Stanton T., et al. (2011). An introduction to rip currents based on field observations. J. Coastal Res. 27, iii–ivi. doi: 10.2112/JCOASTRES-D-11-00024.1
Maryan C., Hoque M. T., Michael C., Ioup E., and Abdelguerfi M. (2019). Machine learning applications in detecting rip channels from images. Appl. Soft Comput. 78, 84–93. doi: 10.1016/j.asoc.2019.02.017
Mekhalfi M. L., Nicolò C., Bazi Y., Rahhal M. M. A., Alsharif N. A., and Maghayreh E. A. (2022). Contrasting YOLOv5, Transformer, and EfficientDet detectors for crop circle detection in desert. IEEE Geosci. Remote Sens. Lett. 19, 1–5. doi: 10.1109/LGRS.2021.3085139
Mori I., de Silva A., Dusek G., Davis J., and Pang A. (2022). Flow-based rip current detection and visualization. IEEE Access 10, 6483–6495. doi: 10.1109/ACCESS.2022.3140340
Mucerino L., Carpi L., Schiaffino C. F., Pranzini E., Sessa E., and Ferrari M. (2021). Rip current hazard assessment on a sandy beach in liguria, nw mediterranean. Natural Hazards 105, 137–156. doi: 10.1007/s11069-020-04299-9
Padilla R., Netto S. L., and Da Silva E. A. (2020). “A survey on performance metrics for object-detection algorithms,” in 2020 international conference on systems, signals and image processing (IWSSIP) (IEEE). (Niteroi, Brazil: IEEE), 237–242.
Philip S. and Pang A. (2016). “Detecting and visualizing rip current using optical flow,” in EuroVis (Short Papers) (Groningen, The Netherlands: Eurographics Association), 19–23.
Rainio O., Teuho J., and Klén R. (2024). Evaluation metrics and statistical tests for machine learning. Sci. Rep. 14, 6086. doi: 10.1038/s41598-024-56706-x
Rampal N., Shand T., Wooler A., and Rautenbach C. (2022). Interpretable deep learning applied to rip current detection and localization. Remote Sens. 14, 91–9. doi: 10.3390/rs14236048
Rashid A. H., Razzak I., Tanveer M., and Robles-Kelly A. (2021). “RipDet: A fast and lightweight deep neural network for rip currents detection,” in International Joint Conference on Neural Networks (IJCNN). (Shenzhen, China: IEEE), 1–6.
Redmon J., Divvala S., Girshick R., and Farhadi A. (2016). “You only look once: Unified, real-time object detection,” in IEEE Conference on Computer Vision and Pattern Recognition (CVPR). (Las Vegas, NV, USA: IEEE), 779–788.
Ren S., He K., Girshick R., and Sun J. (2015). Faster R-CNN: Towards real-time object detection with region proposal networks. Adv. Neural Inf. Process. Syst. 28, 6048–6070. doi: 10.1109/TPAMI.2016.2577031
Retnowati A., Marfai M. A., and Sumantyo J. S. (2012). Rip currents signatures zone detection on alos palsar image at parangtritis beach, Indonesia. Indonesian J. Geogr. 43, 12–27.
Sandler M., Howard A., Zhu M., Zhmoginov A., and Chen L.-C. (2018). “MobileNetV2: Inverted residuals and linear bottlenecks,” in 2018 IEEE/CVF Conference on Computer Vision and Pattern Recognition (IEEE Computer Society, Los Alamitos, CA, USA), 4510–4520. doi: 10.1109/CVPR.2018.00474
Silva A. d., Zhao M., Stewart D., Hasan F., Dusek G., Davis J., et al. (2023). RipViz: Finding rip currents by learning pathline behavior. 2020 IEEE/CVF Conference on Computer Vision and Pattern Recognition (CVPR) 2023 (Seattle, WA, USA: IEEE), 1–13. doi: 10.1109/TVCG.2023.3243834
Tan M., Pang R., and Le Q. V. (2020). “EfficientDet: Scalable and efficient object detection,” in Proceedings of the IEEE/CVF Conference on Computer Vision and Pattern Recognition (CVPR). (Long Beach, CA, USA: IEEE), 10781–10790.
Wang Z., Dai Z., Poczos B., and Carbonell J. (2019). “Characterizing and avoiding negative transfer,” in Proceedings of the IEEE/CVF Conference on Computer Vision and Pattern Recognition (CVPR). (Long Beach, CA, USA: IEEE) 11293–11302.
Zhang Y., Huang W., Liu X., Zhang C., Xu G., and Wang B. (2021). Rip current hazard at coastal recreational beaches in China. Ocean Coastal Manage. 210, 105734. doi: 10.1016/j.ocecoaman.2021.105734
Keywords: rip current detection, data collection, citizen science, coastal observation, computer vision, deep learning, mobile application
Citation: Khan F, de Silva A, Palinkas A, Dusek G, Davis J and Pang A (2025) RipFinder: real-time rip current detection on mobile devices. Front. Mar. Sci. 12:1549513. doi: 10.3389/fmars.2025.1549513
Received: 21 December 2024; Accepted: 07 April 2025;
Published: 20 May 2025.
Edited by:
Muhammad Yasir, China University of Petroleum (East China), ChinaReviewed by:
Zhiqiang Li, Guangdong Ocean University, ChinaSurisetty Kumar, Space Applications Centre (ISRO), India
Copyright © 2025 Khan, de Silva, Palinkas, Dusek, Davis and Pang. This is an open-access article distributed under the terms of the Creative Commons Attribution License (CC BY). The use, distribution or reproduction in other forums is permitted, provided the original author(s) and the copyright owner(s) are credited and that the original publication in this journal is cited, in accordance with accepted academic practice. No use, distribution or reproduction is permitted which does not comply with these terms.
*Correspondence: Fahim Khan, ZmtoYW40QHVjc2MuZWR1; ZmtoYW4xOUBjYWxwb2x5LmVkdQ==