- 1School of Fishery, Zhejiang Ocean University, Zhoushan, China
- 2College of Marine Living Resource Sciences and Management, Shanghai Ocean University, Ihanghai, China
- 3The Key Laboratory of Sustainable Exploitation of Oceanic Fisheries Resources, Ministry of Education, Shanghai Ocean University, Shanghai, China
- 4National Distant-Water Fisheries Engineering Research Center, Shanghai Ocean University, Shanghai, China
- 5Key Laboratory of Oceanic Fisheries Exploration, Ministry of Agriculture and Rural Affairs, Shanghai, China
In the context of the growing demand for the sustainable development and conservation of fish stocks, artificial intelligence (AI) technologies are essential for supporting scientific fish stock management. Artificial intelligence technology provides an effective solution for the intelligent recognition of fish information. This study used bibliometric analysis to review a sample of 719 scientific articles from the WoSCC (Web of Science Core Collection) database from 2014-2024. The results revealed a significant increase in the number of publications from 2014-2024, with publications mainly from China, the USA (the United States) and other developed countries. The top three impactful journals are Ecological Informatics, Computers and Electronics in Agriculture and the ICES Journal of Marine Science. The most frequent keyword co-occurrence analysis was deep learning, and the best keyword clustering effect was computer vision. The findings indicate that this bibliometric evaluation provides a holistic visualization of the research frontier of AI in fish information identification, and our findings underscore the growing global importance of AI in fish information identification research and highlight publication trends, hotspots, and future research directions in this area. In conclusion, our findings provide valuable insights into the emerging frontiers of AI-based fish information identification.
1 Introduction
Fish are important food sources for humans, and fisheries constitute an important component of agricultural economies worldwide (Liu et al., 2023). The exploitation and protection of fish resources has become increasingly vital for meeting the growing global demand for animal protein and addressing food security challenges (Mandal and Ghosh, 2024a). Performing accurate taxonomic assessments of fish information is a persistent challenge for researchers and practitioners alike, especially in the exploitation and protection of fish resources (Campos et al., 2025). However, traditional methods often rely on manual analysis, which can be time-consuming, labor-intensive, and prone to human error (Ou et al., 2023). Additionally, these methods may not provide real-time data, limiting the ability to respond promptly to changing conditions and potential issues (Wu et al., 2022). In this context, the application of AI has shown great promise in revolutionizing FI-AI (the cross-application of fish information identification and artificial intelligence), thereby contributing to the sustainability of the exploitation and protection of fish resources. Therefore, automatically and intelligently identifying fish information has become a hot issue in marine and fishery science.
AI has emerged as a transformative technology with significant potential for revolutionizing marine and fishery science. AI refers to the development and application of computer systems capable of performing tasks that typically require human intelligence, such as learning, problem-solving, and decision-making (Mandal and Ghosh, 2024a). It encompasses a range of techniques that enable machines to mimic human intelligence, learn from data, and make autonomous decisions (Janiesch et al., 2021). The rapid development of AI has promoted the progress of marine and fishery science, especially in recent years, and the emergence of numerous AI methods has helped solve many problems in FI-AI (Saberioon et al., 2017). Machine learning (Support Vector Machine, k-Nearest Neighbor, Random Forest, etc.), a subset of AI, has the main function of solving problems that exist on the basis of algorithms and learning data to create mathematical models that improve the performance of systems on computers (Vo et al., 2021). It can analyze datasets, recognize patterns, and generate models that facilitate accurate identification via FI-AI. The identification method based on the combination of a machine learning algorithm and fish features has high interpretability and good robustness and can achieve excellent identification performance on small datasets (Ou et al., 2023). Deep learning (Visual Geometry Group Network, Residual Neural Network, You Only Look Once, etc.), a branch of machine learning, is a machine learning method based on neural networks that can effectively extract high-level target features for recognition and classification (Liu et al., 2023; Kaur et al., 2023). It has strong performance and robustness and extensive application prospects in FI-AI. The deep-learning-based fish information identification method allows the model to automatically learn features in a data-driven manner, thus achieving fish information acquisition, and this method has a more obvious effect on large datasets than does technology that relies on prior knowledge to design features (Kaur et al., 2023).
With the proposal of intelligent marine science and intelligent fishery science, many scholars have applied artificial intelligence to fish information identification. By using AI technology for analysis and processing, some shortcomings of traditional methods can be overcome. AI is widely used in the identification of fish information. At present, many researchers worldwide have published relevant research on FI-AIs, including studies on fish species (Li et al., 2022), fish behavior (Abangan et al., 2023), fish abundance (Ditria et al., 2020), fish length and fish weight (Bravata et al., 2020; Wu et al., 2024), fish otoliths (Stock et al., 2021) and fish sex (Barulin, 2019). In recent years, relevant articles on the application of FI-AIs are shown in Table 1. By analyzing FI-AIs, the use of artificial intelligence technology can achieve faster and more accurate identification of important information. Owing to its advantages of intellectualization, high accuracy, and high efficiency, AI technology provides new solutions for many bottleneck problems in traditional fish research (Liu et al., 2023). This makes AI technology better for the identification of fish information and shows the strong advantages of FI-AI applications (Wu et al., 2024; Stock et al., 2021; Barulin, 2019; Signaroli et al., 2022; Yu et al., 2023; Xun et al., 2024; Allken et al., 2021; Flores et al., 2024; Yu et al., 2021).
The research on artificial intelligence technology in FI-AI also faces certain challenges and potential limitations. For example, Campos et al. (2023) published a study demonstrating the risks associated with relying solely on images for documenting new species records, rediscoveries, taxonomic descriptions, and distribution expansions. They highlighted concerns regarding image authenticity, especially in cases where images may be altered, adulterated, or AI-generated, potentially leading to inaccuracies in biodiversity documentation. Another example of failure of using FI-AI was published by Campos et al. (2025). In this case Campos and collaborators demonstrated that a machine learning computer vision model created to identify Amazonian fish was unable to accurately identify fish photographs, resulting in 82% of misidentification, and did not outperform what would be expected by chance. Therefore, the research and application of AI technology in FI-AI needs to update and iterate on technologies and methods to further improve FI-AI.
Bibliometrics is a method that uses mathematical statistics to quantitatively study the results, impact, and trends of published publications (Xu et al., 2021; Xiao et al., 2025). It can identify publication patterns in specific fields, quantitatively evaluate citation data, understand research priorities, and predict future research directions (Huang et al., 2019). This methodological approach has been used by several authors, with excellent results (Santos de Moura and Vianna, 2020; Segaran et al., 2023). Although some studies have reviewed various aspects of identification research on fish information (Matley et al., 2023; Nalmpanti et al., 2023), a quantitative, scientometric outlook has been lacking for the substantial body of literature on FI-AI. This study employs scientometric analysis to provide a quantitative global research performance assessment of the existing publications pertaining to FI-AI. A quantitative analysis of the literature in this field will help improve the understanding of trends, key topics and journals available for FI-AI.
2 Materials and methods
2.1 Data source
The data used for analysis were obtained from the Web of Science Core Collection (WoSCC) database. The WoSCC database is one of the most commonly used comprehensive databases for bibliometric analysis, and it contains a multidisciplinary index of scholarly literature abstracts that include multiple datasets (Falagas et al., 2008). The retrieval conditions were Topic = (machine learning OR deep learning) and Topic = (fish identification OR fish recognition OR fish classification). The time span of the data was from 1 January 2014 to 22 November 2024, with “Article” or “Review Article” document types selected. To ensure the validity and reliability of the search results, we manually excluded some irrelevant documents on the basis of their titles, abstracts, methods, and results. In the end, 719 articles were selected, and a bibliometric database was established. The full records and citation references were exported in plain text for further analysis.
2.2 Introduction of bibliometric instruments
The visualization software used in this study includes CiteSpace, VOSviewer, and SCImago Graphica. Among them, CiteSpace (https://citespace.podia.com/) is scientometric software developed by Professor Chaomei Chen. It uses citation analysis, network algorithms, and other methods to mine, analyze, and visualize information contained in bibliometric data, revealing trends in relevant fields (Chen, 2017). VOSviewer (https://www.vosviewer.com/) is software developed by Leiden University in the Netherlands. It is used to visualize bibliometric data on the basis of distance, with more closely related terms appearing closer to the center in the visual display (Perianes-Rodriguez et al., 2016). Scimago Graphica (https://www.scimagojr.com/) is a powerful and easy-to-use graphical tool that can be used for bibliometric analysis (Bojesen et al., 2024; Hassan-Montero et al., 2022).
2.3 Statistical analysis and visualization
Scientometrics of publications can clearly and succinctly summarize the development process, hot spots, and future trends of a certain field (Chen, 2017; Perianes-Rodriguez et al., 2016; Bojesen et al., 2024). Hence, the research steps of this paper are as follows (Figure 1). First, the research theme of this paper was defined: “Application of artificial intelligence in fish information identification: a scientometric perspective”. A preliminary literature database was subsequently searched according to the topic, and after excluding publications from unrelated research areas, the relevant data were exported through publication selection. Finally, the data analysis and data visualization were performed via bibliometric instruments.
The bibliometric analysis and visualization in this study were conducted mainly via CiteSpace (6.4. R1) VOSviewer (1.6.20), and SCImago Graphica Beta (1.0.46). Multiple units extracted from the dataset, including authors, institutions, countries, journals, and keywords, were used to conduct the bibliometric analysis. In this study, the CiteSpace parameters were set as follows: the time slices were set from 2014-2024, with each slice representing one year. The node types were selected on the basis of the content of the analysis. The G-index, top N, and top N% thresholds were set to 35, 50, and 10.0, respectively, while the other parameters were left at their default settings. The VOSviewer parameters are set to default values. SCImago Graphica Beta parameters This is also the default.
3 Results
3.1 Publications characteristics
The number of published papers is an important indicator of trends in this field. In the realm of global scholarly output, a comprehensive review of literature from 2014-2024 yielded 719 publications aligning with the established search parameters. The number of publications and trends in this field are shown in Figure 1A. The annual number of publications significantly increased from 16 papers in 2014 to 135 papers in 2024. This increasing trend in publication volume intensified, particularly in stage II (2020–2024), as illustrated in Figure 2A. Figure 2B lists the top 10 categories, and Figure 2C further displays the publication trends for the top 5 categories. These categories have gained significant attention in recent years, particularly after 2020. The Fisheries and Marine Freshwater Biology category has been gaining popularity since 2020. The publications depicted in Figure 1 indicate that research on fish identification is receiving considerable attention. This also reflects the increasing focus on artificial intelligence and fish identification fields across research.
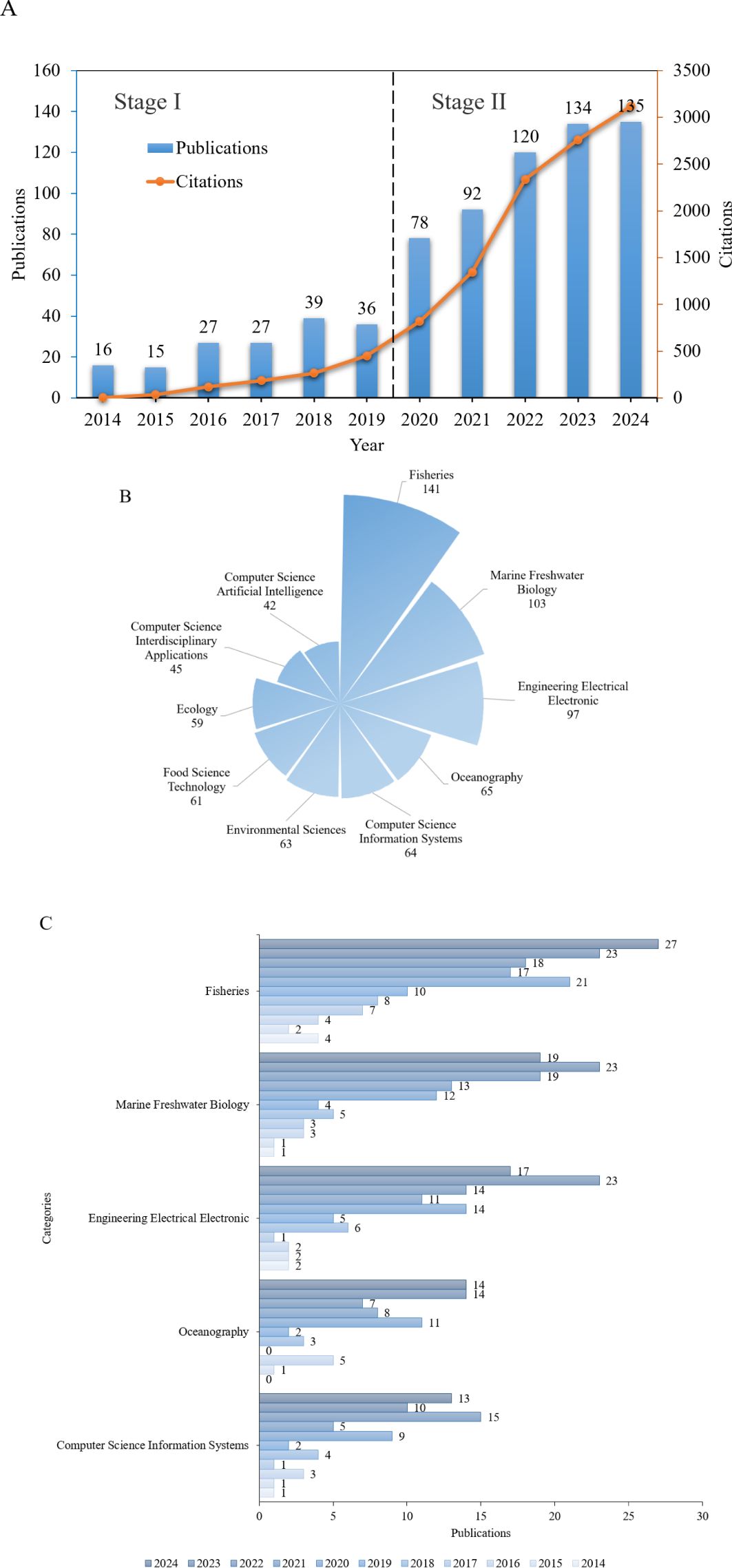
Figure 2. (A) The number of publications from 2014-2024. (B) The top 10 Web of Science categories. (C) Publication trends of the top 5 Web of Science categories.
3.2 Analysis of the contributions of authors, institutions, and countries
3.2.1 Analysis of the contributions of authors and institutions
Among the 3081 authors, 31 reached the threshold of at least 5 publications (Figure 3). The larger the circle and font in the network diagram are, the stronger the link and the greater the number of publications. The color of a circle indicates the cluster to which it belongs. The one with the largest circle is Li, Daoliang. He has published up to 28 publications on the application of FI-AI (Table 2). The second largest circle is Chen, Yingyi and Yang, Xinting. The largest group of relevant authors is 11, which are concentrated in Figure 3A. The colors in Figure 3B represent the author’s active period: “light yellow” indicates that researchers have published fish identification research recently (2024), and “dark blue” indicates that they were published approximately 2014.
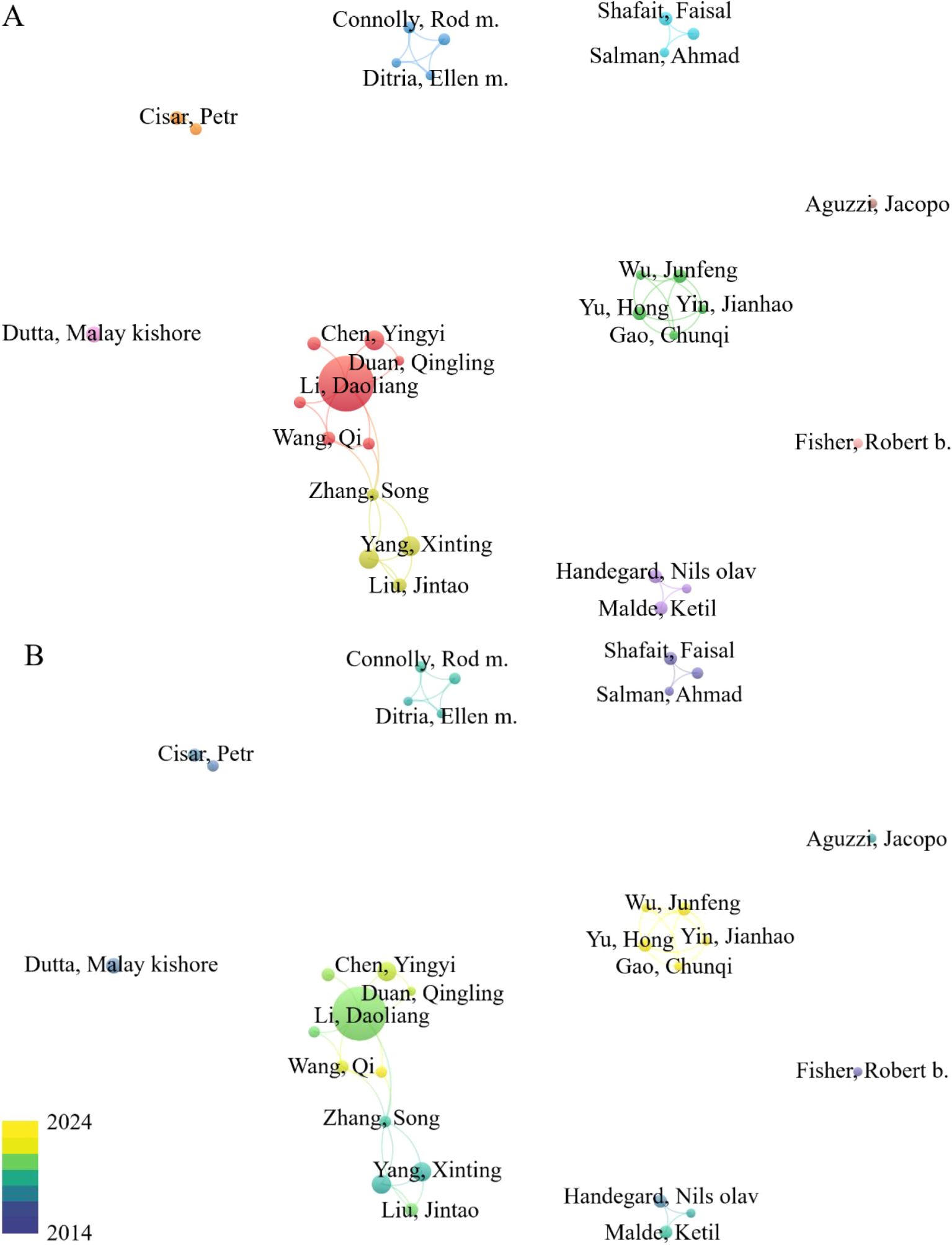
Figure 3. A co-authorship network visualization (A) and overlay visualization (B) map of 31 authors with more than 5 publications.
A total of 46 organizations met the threshold of a minimum of 5 publications per organization (Figure 4). There are fewer connections between organizations (Figure 4A). The group represented by China Agricultural University is the most obvious organizational link. This suggests that research in this field is conducted by multiple independent groups, reflecting a diverse range of research directions but also highlighting the potential for increased collaboration. Figures 4B, 3B have similar meanings. The color changes indicate time changes. Among them, China Agricultural University has published the most 49 publications in FI-AI field (Table 3).
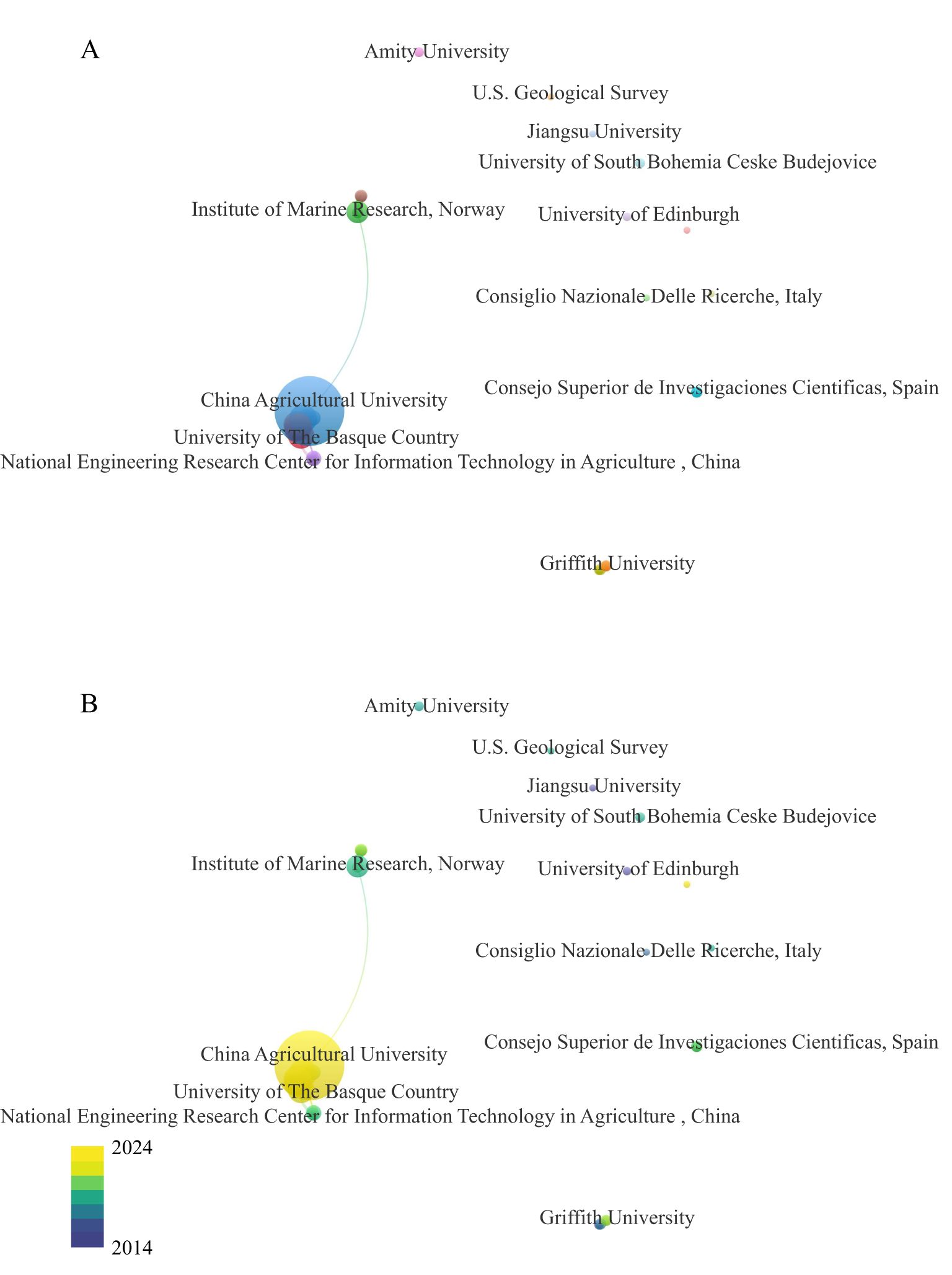
Figure 4. Network visualization of cooperation among academic institutes (A) and overlay visualization (B) map of 46 organizations with more than 5 publications.
3.2.2 Distribution and cooperation of the contributing countries
The artificial intelligence development of FI-AIs has consistently garnered international attention. The top 10 countries with the most publications include China, the USA, Spain, England, India, Australia, Norway, France, Italy, and Japan (Figure 5A). The world map that visualizes the distribution of countries worldwide and their collaborations is presented in a network format (Figure 5B). The red lines indicate the strength of collaboration. The links between countries are primarily concentrated between North America and Europe and between Oceania and Europe, with strong connections between East Asia and North America. It portrays the cooperative relationships between countries in research, emphasizing notable collaboration (Figure 5C). Joint research involving China, the USA, Spain, England, India and Australia ranks among the top six countries. Among these, the connections between China and the United States, China and Spain, and China and England are particularly close.
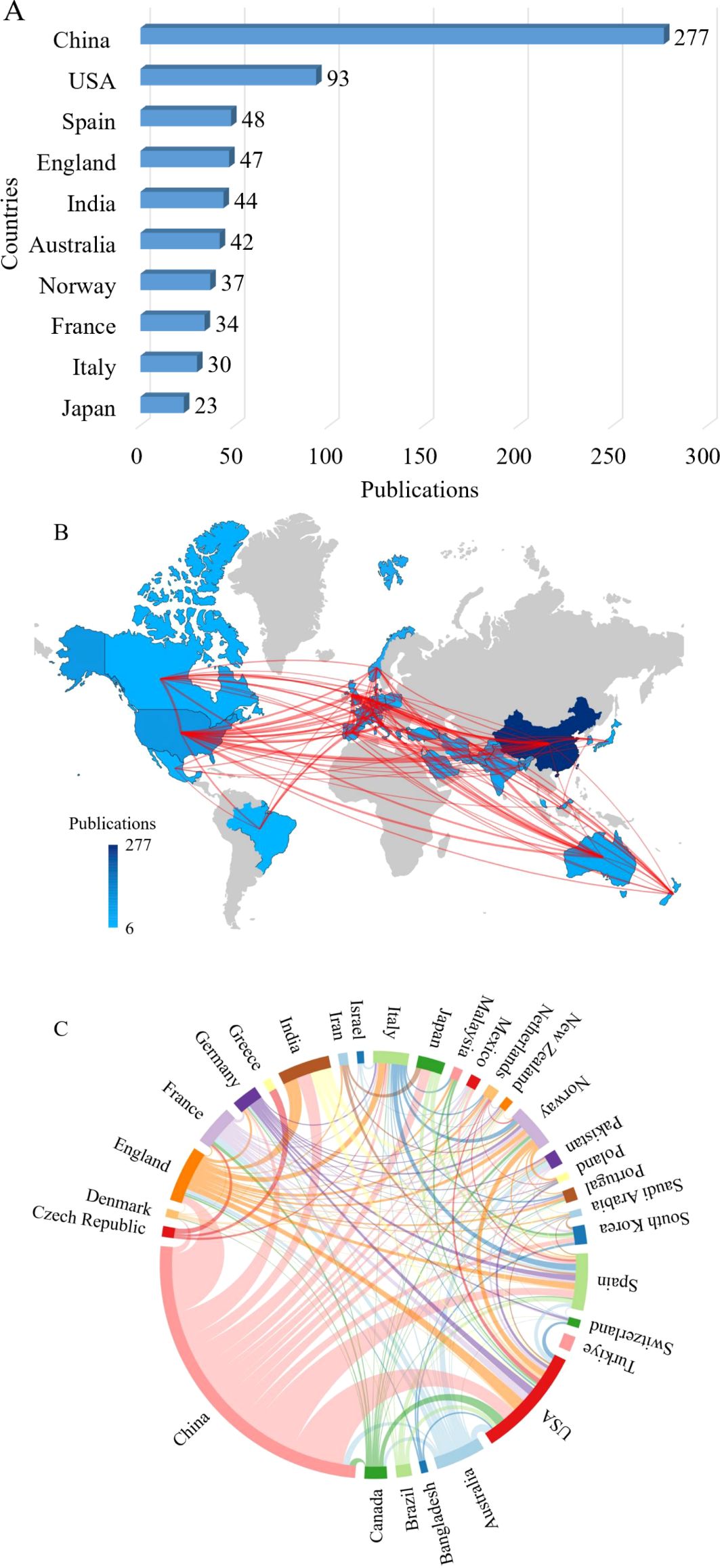
Figure 5. (A) The number of publications of the top 10 countries from 2014-2024. (B) Country collaboration map generated via Scimago Graphica. (C) A network diagram that illustrates the cooperative relationships between countries.
3.3 Impactful journals and co-citation analysis
We performed a systematic analysis of influential journals and co-cited journals. The journals with the most production and co-citation are Ecological informatics (Figure 6A, circle maximum) and Proceedings of the IEEE conference on computer vision and pattern recognition (Figure 6C, circle maximum), respectively. Ecological informatics leads the cohort with a commendable tally of 35 publications, followed closely by the Computers and electronics in agriculture with 34 publications, ICES Journal of Marine Science with 27, IEEE access with 24, and Fisheries research contributing 22 articles (Figure 6B). The first three citations are Proceedings of the IEEE conference on computer vision and pattern recognition, the ICES Journal of Marine Science, and Computers and electronics in agriculture (Figure 6D).
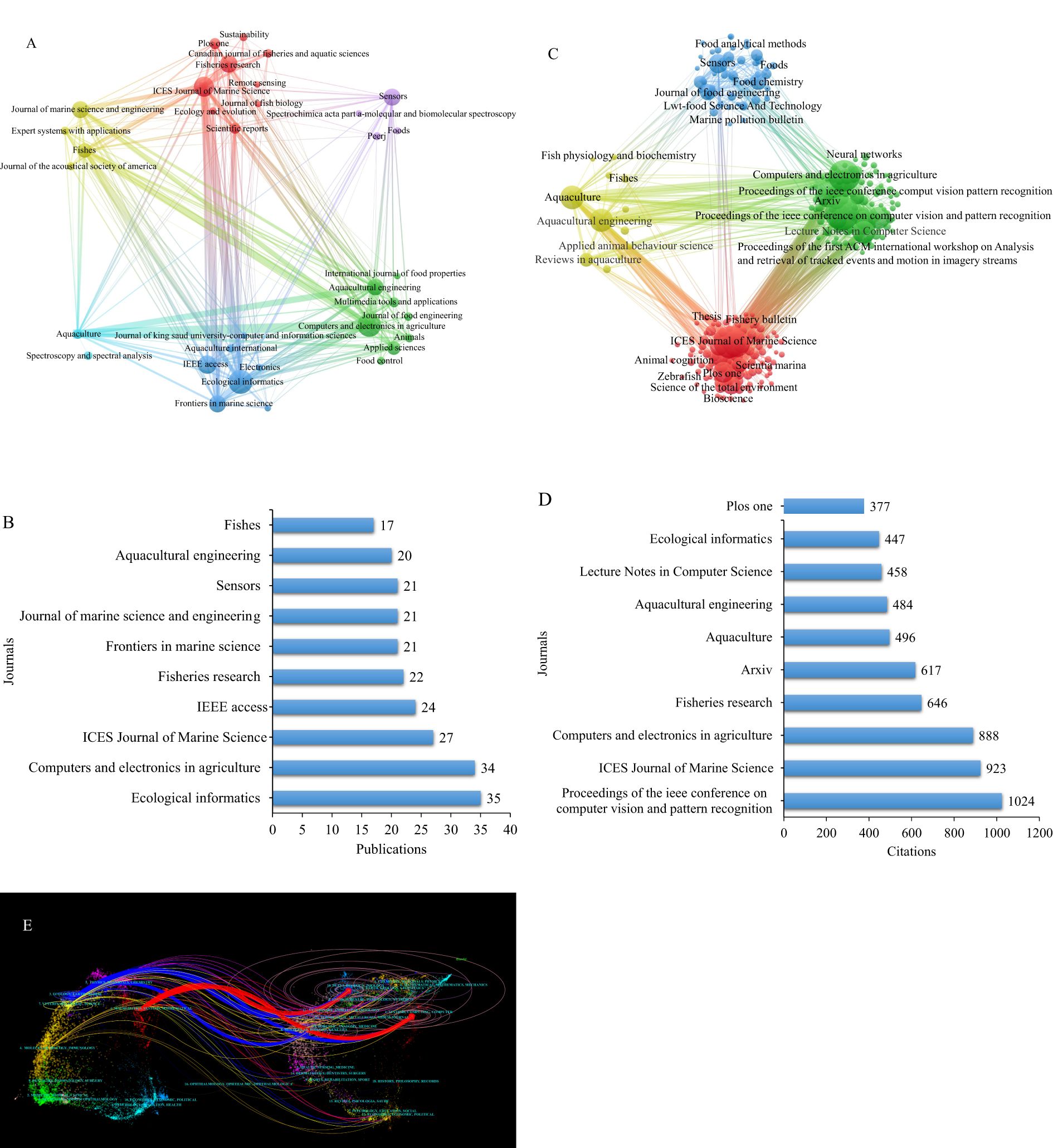
Figure 6. Analysis of influential journals and co-cited journals. (A) Network visualization maps of the most influential journals produced with VOSviewer. (B) The top 10 influential journals in the field of FI-AI. (C) Network visualization maps of the most co-cited journals produced with VOSviewer. (D) The top 10 co-cited journals in the field of FI-AI. (E) Dual-map overlay for publications related to FI-AI via CiteSpace.
A dual map overlay revealed the correlation of research disciplines and the citation relationships among the influential journals related to FI-AI (Figure 6E). The map on the left is the map of the citing journal, and the map on the right is the map of the cited journal. The curve is the citation path of the publication, which can indirectly reflect the disciplinary connection between the citing publication and the cited publication. The diagram consists of five main paths. The blue line represents the subject link from “ecology, earth, marine” to “systems, computing, computer”, “molecular, biology, genetics”, and “plant, ecology, zoology”. The red line indicates subject associations from “mathematics, systems, mathematical” to “systems, computing, computer” and “plant, ecology, zoology”. The relevant publications indicating the FI-AI field are mainly concentrated in the fields of “ecology, earth, marine” and “mathematics, systems, mathematical “. The publications cited are mainly in the fields of “systems, computing, computer”, “molecular, biology, genetics”, and “plant, ecology, zoology”.
3.4 Keywords analysis
3.4.1 Keyword co-occurrence
We used CiteSpace software to conduct a co-occurrence analysis of the keywords collected from the literature from 2014-2024. The co-occurrence analysis generated a network map in CiteSpace, visualizing the relationships between keywords and highlighting the scientific hotspots in FI-AI research. In the keyword co-occurrence network, each node represents a keyword, and the larger the node is, the higher the frequency of keyword occurrence (Figure 7A). The thickness of the links between nodes represents the co-occurrence frequency, with thicker links indicating more frequent occurrences. The color of the nodes changes from dark blue to dark pink, indicating that the year in which the keyword appears in the article is becoming increasingly close. The purple square around the node indicates that the intermediate centrality of the keyword is greater than 0.1. Keywords such as “neural networks”, “system”, “abundance”, “behavior”, and “size” exhibit intermediate centrality in the network. This indicates that the research objects of publications in the field of fish identification generally focus on biological characteristics and deep learning networks. Furthermore, keywords such as “system”, “behavior”, “neural network”, “quality”, and “convolutional neural network” clearly have a higher frequency of occurrence, in addition to those closely related to the search terms (Table 4). This suggests that these keywords serve as bridges within the network, indicating that numerous publications on FI-AI incorporate these keywords in their content.
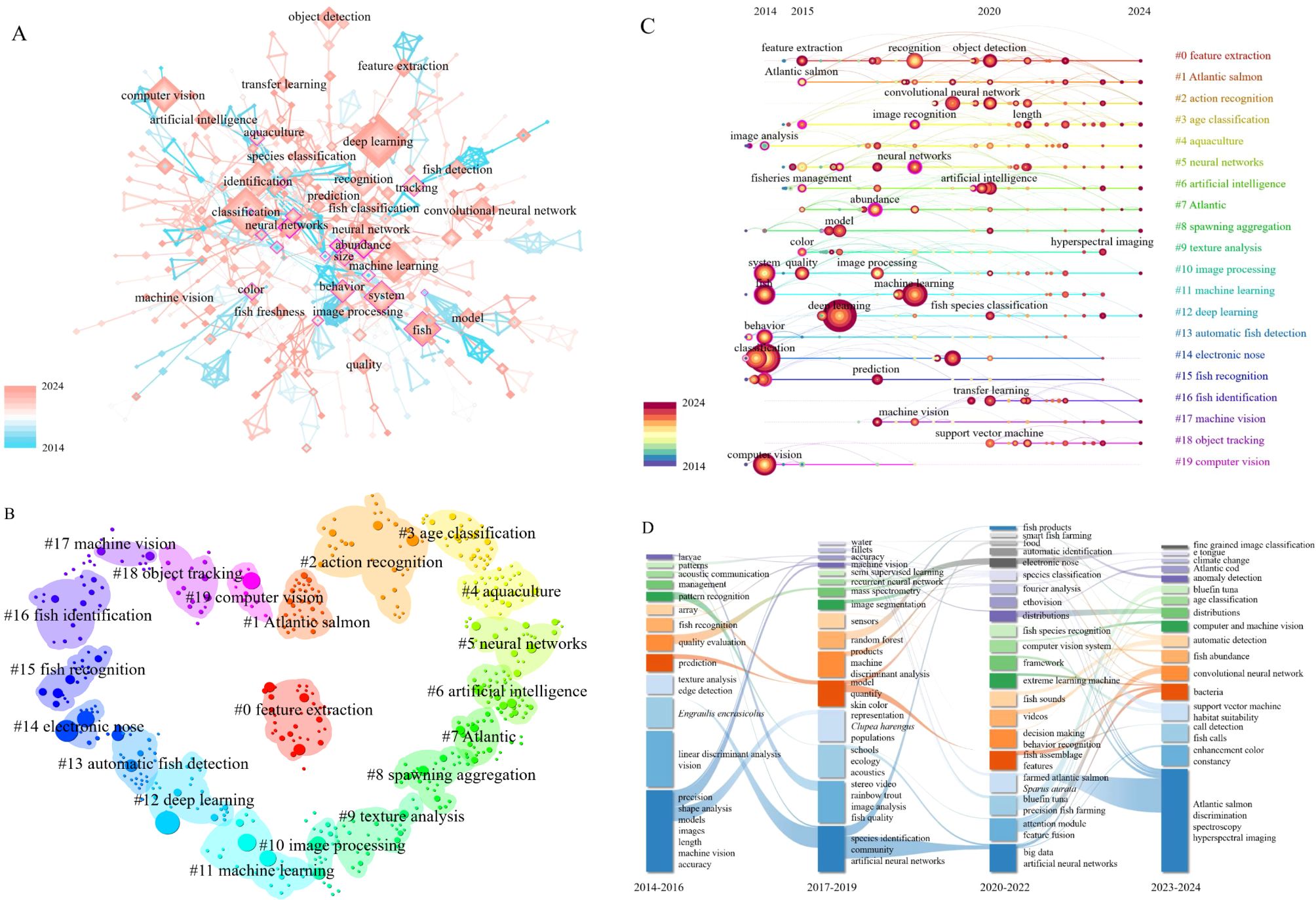
Figure 7. (A) The keyword co-occurring network using CiteSpace. (B) Cluster analysis of the keywords related to FI-AI. (C) Visualization map of the timeline view of keyword analysis via CiteSpace. (D) Sankey diagram illustrating the occurrence frequency of keywords over time.
3.4.2 Keywords clustering
Through cluster analysis of keywords in the field of FI-AI, a large field can be scientifically divided into several small fields according to the research content (Figure 7B). It includes a total of 20 different clusters (Figure 7B), and the main keyword information contained in the clusters is shown in Table 5. The silhouette value in the table is used to evaluate the clustering effect. When the value is greater than 0.7, the clustering results are highly reliable. The closer the value is to 1, the better the clustering effect is. The top 20 clusters are all greater than 0.7, among which cluster ID 19 is equal to 1.
3.4.3 Keywords vicissitude
The evolution of the keyword clusters of FI-AI publications can be observed through the timeline graph (Figure 7C). Research published between 2014 and 2024 was selected for analysis, with a time slice length of 1 year. All the keywords can be divided into twenty subclusters with excellent homogeneity. Clusters #10, #11, and #12 have remained active over a long period, underscoring their representation of enduring research topics. The largest circle appears in the largest cluster, deep learning, indicating that the keyword “deep learning” had the highest number of publications and was closely associated with “fish species classification”, highlighting significant attention to the biological species and artificial intelligence during this cluster.
We analyze the occurrence frequency of keywords over time. Articles published between 2014 and 2016 focused on precision, shape analysis, models, images, length, machine vision, and accuracy. Articles published between 2017 and 2019 focused on species identification, communities, and artificial neural networks. The papers published between 2020 and 2022 focus on the module of species identification and artificial neural networks. Recent publications have focused on Atlantic salmon, discrimination, spectroscopy, and hyperspectral imaging (Figure 7D).
3.4.4 Keywords burstiness
This study uses CiteSpace’s burst detection algorithm to analyze keyword burstiness, with a minimum burst duration set at 5 years, and the top 10 keywords with the strongest citation bursts are identified (Figure 8). The blue lines in the graph represent the keyword timelines, with red segments indicating the burst duration. The keyword ‘image analysis’ emerged as the most intense (strength = 4.33), closely followed by ‘image processing’ (4.22) and ‘species classification’ (3.94). Remarkably, ‘patterns’ had the longest burst duration, spanning 6 years from 2015-2021, reflecting its enduring relevance in the field.
4 Discussion
4.1 Publications distribution and change in the field of FI-AI
The aim of this study was to analyze the current status and trends of FI-AI publications from 2014-2024 via the WoSCC database and bibliometric tools. The results help with understanding recent FI-AI research progress. The results show that from 2014-2024, the global publishing situation underwent two phases. In the first phase (2014-2019), FI-AI was still at a low level. In the second stage, from 2020-2024, FI-AI research developed rapidly, and the identification application of AI in fish information has received increasing attention. These findings indicate a promising future for FI-AI research. Authors are primarily likely to pay increasing attention to the top 5 research categories in this field, including studies on fisheries, marine freshwater biology, engineering electrical electronics, oceanography, and computer science information systems (Figure 1). This shows that marine science and fishery science are significantly affected by AI, which further promotes the intelligent development of fish information (Bojesen et al., 2024; Bonnichsen et al., 2025; Lilkendey et al., 2024).
4.2 Highly contributing authors, institutions, countries, and their cooperation
Scholars with many citations or many publications play a leading role in the research direction in this field, so understanding these high-impact scholars is equivalent to understanding who leads the research in this field (Zhang and Xie, 2023). This bibliometric study revealed that Li, Daoliang, is a leader in FI-AI. Analyzing the contributions of countries and institutions to research in this field will help scholars seek cooperation between universities or international institutions, thereby promoting the development of the field (Ding et al., 2022). In this study, China Agricultural University published the most publications in this field, indicating that the university is at the leading level of research in this field. In terms of cooperation between countries, the connections between China and the United States, China and Spain, and China and England are particularly close. Notably, China and the USA exhibit the closest collaboration and dominate the field, collectively contributing to more than half of the global publications (Figure 4).
In addition, this study revealed that while there is little communication and cooperation between authors or institutions, there is considerable communication and cooperation between countries. Therefore, there is a need to strengthen communication between different authors and institutions through technical cooperation and the academic exchange of FI-AI. According to the different contents of different authors or different institutions, the research bottleneck can be broken through by complementary advantages.
4.3 Research frontiers and hotspots of FI-AI
4.3.1 Impact journals and cited journals
We analyzed impact journals (top 10) and co-cited journals (top 10) and found that FI-AI research is concentrated in journals related to marine and fisheries sciences. We also analyzed the dual-map overlay for publications related to FI-AI. The path from the left to the right (from citing publications to cited publications) in the figure becomes significantly more fragmented, indicating a broader range of disciplines in the cited literature. The fact that FI-AI publications cite publications from a wider range of disciplines further illustrates the trend toward diversification in FI-AI research. Relevant studies have shown that AI technology can identify a variety of types of fish information (fish species, fish behavior and fish feeding, etc.) to contribute to technological breakthroughs in aquaculture (Liu et al., 2023). In addition, according to a study of the electronic monitoring (EM) of global capture fisheries, there are 100 EM trials and 12 fully implemented programs worldwide, and EM research uses AI technology to identify fish information (fish species and catch quantity, etc.) (van Helmond et al., 2020).
4.3.2 Keyword trends and their correlation with FI-AI
In this study, the co-occurrence of keywords via FI-AI was analyzed. The keywords of the publication summarized the core content of the FI-AI and reflected a large amount of information. The analysis of keywords in the field of FI-AI is helpful for further understanding the research hotspots in this field (Mao et al., 2018). The most frequent co-occurrence of keywords is deep learning, and in recent years, the research hotspot of FI-AI has been dominated by deep learning. Furthermore, keyword cluster analysis scientifically divides the research content of FI-AI into several small fields. The statistical clustering algorithm is used to simplify the co-occurrence network of FI-AI into a relatively simple intergroup relationship (Segaran et al., 2023). The top 15 clusters are all greater than 0.9 (silhouette value), among which cluster ID 19 (computer vision) is equal to 1. The main keywords corresponding to cluster ID 19 is fish recognition, welfare, neural network, artificial intelligence, and quality evaluation. The second in terms of the silhouette value is cluster ID 15 (fish recognition), whose main keywords are object recognition, vector machine, imbalanced data, fish identification, and fish recognition. It not only refines the research hotspot of FI-AI but also contributes to researchers’ overall understanding of the research hotspot. Each cluster of FI-AIs also reflects major research advances in the field of study, which amounts to a summary of the major elements of the field of FI-AIs.
By analyzing the keyword burstiness of FI-AIs, this study obtains the keywords frequently mentioned by FI-AIs from 2014-2024. The two keywords with the highest intensity are image analysis and image processing, and the top ten keywords with the highest intensity are also images, color and shape. The burstiness of these keywords indicates that FI-AI focuses mainly on visual perception (Liu et al., 2023; Mandal and Ghosh, 2024a; Li et al., 2022). The changes in the keyword clusters of FI-AI publications were analyzed via a timeline graph. We also analyze the frequency of keyword occurrences over time. From 2014–2024, FI-AI research was focused mainly on fish biology, and different biological characteristics were studied through AI technology. Furthermore, research on fish biology is constantly innovating intelligent technology, indicating continuous maturation and improvement in research within this field FI-AIs (Saleh et al., 2022).
4.4 Future development trends for FI-AI
As AI continues to evolve, FI-AI offers multiple benefits in fish research. FI-AI is highly automated and intelligent: FI-AI can leverage the integration of diverse data sources, such as computer vision, underwater acoustics, sensor networks, and genetic data, to provide a holistic view of fish information (Mandal and Ghosh, 2024b). By combining data from various sources, AI models can uncover complex relationships, identify real-time fish information, and generate comprehensive assessments of fish information. FI-AI research contributes to the automation of fisheries, reducing the need for manual labor and improving operational efficiency (Khokher et al., 2022). FI-AI can also help further analyze fish behavior patterns (Lopez-Marcano et al., 2021), detect early signs of fish disease (Chiu et al., 2022), enable precision fisheries (Gladju et al., 2022), and provide insights for decision-making (Barreto et al., 2022).
Hence, FI-AI has great potential in the future. Based on the bibliometric analysis of FI-AI research trends and research frontiers in this study, we believe that the further development trends of FI-AI in the future are: (1) the development of FI-AI integration systems for different fish information can optimize the utilization of fish resources and contribute to the scientific decision-making of fish resource management; (2) The combination of FI-AI and AI-driven robots can simplify the operation tasks of fish-related industries, improve the identification accuracy and reduce human labor and human error; (3) The integration of FI-AI with generative AI can offer enhanced data and technical support, thereby further improving identification accuracy and expanding practical applications; (4) Build a comprehensive FI-AI monitoring platform for fish information resources, which will further realize the responsible and sustainable development and protection of fish resources.
4.5 Suggestions for FI-AI
4.5.1 Technical advice for FI-AI
In the process of identifying fish information, although machine learning has high requirements for feature extraction, it has strong interpretability, which is of great significance in future FI-AI research (Li et al., 2022). Through the bibliometric analysis of FI-AI in this study, it is found that deep learning is the main hotspot in this field in recent years. Fish information identification models based on deep learning can automatically extract features, and deep learning also has high identification accuracy when analyzing fish information tasks. Deep learning can provide a more effective method for researchers in the identification process of fish information in the future. However, there are some shortcomings in the deep learning of FI-AI, such as the amount of fish information data, network model generalization and parameter selection. Therefore, for the further development of FI-AI. Our recommendations are as follows: First, effective solutions to the lack of informative data in fish are provided by means of data augmentation, transfer learning, active learning, and the use of generative adversarial networks (Liu et al., 2023; Shin et al., 2016; Kong et al., 2022). Secondly, for the network generalization ability of deep learning, the relationship between training accuracy and validation accuracy needs to be further determined. Parameter selection for deep learning can be solved by using hyperparameter optimization algorithms to assist decision making (Bergstra and Bengio, 2012; Snoek et al., 2012). Finally, the practical application of FI-AI can be further optimized by model improvement (adjusting the structure and parameters of the model) and multimodal technology (Zhao et al., 2022).
4.5.2 Suggestions on the application promotion of FI-AI
Currently, AI technology has not yet achieved widespread implementation of intelligent applications for fish information, and relevant practitioners lack a profound understanding of AI technology. The effectiveness of FI-AI largely depends on the availability and reliability of fish information data (Aryai et al., 2021). FI-AI needs to be effectively trained on domain-specific data, and its models and techniques have not been popularized (van Helmond et al., 2020). Owing to its technical difficulty, FI-AI requires a high interdisciplinary level (Liu et al., 2023). Additionally, the refinement of AI technology (specifically, the identification technology for particular fish information) and the integration of systems (into practical application products) remain inadequate. Consequently, the involvement of scientists, industry stakeholders, and regulatory agencies in FI-AI research plays a critical role in overcoming technological barriers and establishing practical applications for fish information system integration and the sustainable development of fish resources. Cultivating interdisciplinary talents will also help promote the further development of the field. In summary, AI-based identification of fish information can contribute significantly to our understanding of FI-AI, aid in effective conservation efforts for fish, and support sustainable management practices to ensure the long-term health and resilience of our fish resources.
5 Conclusion
Our comprehensive bibliometric and visual study underscores the burgeoning interest and remarkable advancements in the field of FI-AI from 2014-2024. This analysis, which spans more than a decade, reveals an upward trend in global research efforts in the field of FI-AI, and our analysis identifies prominent scholars such as Li Daoliang, Chen, Yingyi and Yang, Xinting who are actively researching FI-AI, whereas China and the United States lead the world in terms of the number of publications. The journal analysis by FI-AI revealed that AI technology has become more widely used in marine and fisheries science and that the application of AI technology is becoming more diverse. Among them, there is a strong correlation between influential journals and co-cited journals, and we find that AI technology has a significant impact on marine and fishery science. Through keyword analysis, the primary technologies of AI, specifically machine learning and deep learning, have been intelligently applied to fish information, primarily in the area of visual perception. In recent years, the use of deep learning technology to investigate the biological characteristics of fish has emerged as a major research focus. As FI-AI applications continue to evolve, their role in revolutionizing the intelligent identification of fish information is becoming increasingly apparent, paving the way for new and more effective AI applications.
Data availability statement
The original contributions presented in the study are included in the article/supplementary material. Further inquiries can be directed to the corresponding author/s.
Ethics statement
The manuscript presents research on animals that do not require ethical approval for their study.
Author contributions
LO: Conceptualization, Data curation, Investigation, Methodology, Project administration, Resources, Software, Writing – original draft, Writing – review & editing, Funding acquisition. LL: Investigation, Methodology, Writing – original draft. WQ: Investigation, Project administration, Supervision, Writing – review & editing. BL: Investigation, Project administration, Supervision, Writing – review & editing.
Funding
The author(s) declare that financial support was received for the research and/or publication of this article. This work was supported by the General Scientific Research Project of Education Department of Zhejiang Province (Y202353969), the Zhejiang Ocean University Talent Introduction Scientific Research Fund Project (JX6311033624), the Research Project of Shanghai Ocean University (SF202400175), The National Key R&D Program of China (2023YFD2401302), Program on the Survey, Monitoring and Assessment of Global Fishery Resources (Comprehensive scientific survey of fisheries resources at the high seas)sponsored by the Ministry of Agriculture and Rural Affairs, Follow-up program for Professor of Special Appointment (Eastern Scholar) at Shanghai Institutions of Higher Learning (GZ2022011), and The National Key R&D Program of China (2024YFD2400605).
Conflict of interest
The authors declare that the research was conducted in the absence of any commercial or financial relationships that could be construed as a potential conflict of interest.
Generative AI statement
The author(s) declare that no Generative AI was used in the creation of this manuscript.
Publisher’s note
All claims expressed in this article are solely those of the authors and do not necessarily represent those of their affiliated organizations, or those of the publisher, the editors and the reviewers. Any product that may be evaluated in this article, or claim that may be made by its manufacturer, is not guaranteed or endorsed by the publisher.
References
Abangan A. S., Kopp D., Faillettaz R. (2023). Artificial intelligence for fish behavior recognition may unlock fishing gear selectivity. Front. Mar. Sci. 10. doi: 10.3389/fmars.2023.1010761
Allken V., Rosen S., Handegard N. O., Malde K. (2021). A deep learning-based method to identify and count pelagic and mesopelagic fishes from trawl camera images. ICES J. Mar. Sci. 78, 3780–3792. doi: 10.1093/icesjms/fsab227
Aryai V., Abbassi R., Abdussamie N., Salehi F., Garaniya V., Asadnia M., et al. (2021). Reliability of multi-purpose offshore-facilities: Present status and future direction in Australia. Process. Saf. Environ. 148, 437–461. doi: 10.1016/j.psep.2020.10.016
Barreto M. O., Rey Planellas S., Yang Y., Phillips C., Descovich K. (2022). Emerging indicators of fish welfare in aquaculture. Rev. Aquacult. 14, 343–361. doi: 10.1111/raq.12601
Barulin N. V. (2019). Using machine learning algorithms to analyse the scute structure and sex identification of sterlet Acipenser ruthenus (Acipenseridae). Aquac. Res. 50, 2810–2825. doi: 10.1111/are.14233
Bergstra J., Bengio Y. (2012). Random search for hyper-parameter optimization. J. Mach. Learn. Res. 13, 281–305.
Bojesen T. A., Denechaud C., Malde K. (2024). Annotating otoliths with a deep generative model. ICES J. Mar. Sci. 81, 55–65. doi: 10.1093/icesjms/fsad170
Bonnichsen R., Nielsen G. G. B., Dam J. S., Schrøder-Petersen D., Buchmann K. (2025). AI-driven realtime monitoring of early indicators for ichthyophthirius multifiliis infection of rainbow trout. J. Fish. Dis. 48 (1), e14027. doi: 10.1111/jfd.14027
Campos D. S., de Oliveira R. F., de Oliveira Vieira L., de Bragança P. H. N., Guimarães E. C., Katz A. M., et al. (2025). Well-intentioned initiatives hinder understanding biodiversity conservation: an essay on a recent deep-learning image classifier for Amazonian fishes. Rev. Fish. Biol. Fisher. 35, 187–200. doi: 10.1007/s11160-024-09901-y
Campos D. S., Oliveira R. F. D., Vieira L. D. O., Bragança P. H. N. D., Nunes J. L. S., Guimarães E. C., et al. (2023). Revisiting the debate: documenting biodiversity in the age of digital and artificially generated images. Web. Ecol. 23, 135–144. doi: 10.5194/we-23-135-2023
Chen C. (2017). Science mapping: a systematic review of the literature. J. Data. Info. Sci. 2, 1–40. doi: 10.1515/jdis-2017-0006
Chiu M. C., Yan W. M., Bhat S. A., Huang N. F. (2022). Development of smart aquaculture farm management system using IoT and AI-based surrogate models. J. Agric. Food Res. 9, 100357. doi: 10.1016/j.jafr.2022.100357
Ding Q., Song X., Yuan M., Sun R., Zhang J., Yin L., et al. (2022). Removal of microcystins from water and primary treatment technologies–A comprehensive understanding based on bibliometric and content analysis 1991–2020. J. Environ. Manage. 305, 114349. doi: 10.1016/j.jenvman.2021.114349
Ditria E. M., Lopez-Marcano S., Sievers M., Jinks E. L., Brown C. J., Connolly R. M. (2020). Automating the analysis of fish abundance using object detection: optimizing animal ecology with deep learning. Front. Mar. Sci. 7. doi: 10.3389/fmars.2020.00429
Falagas M. E., Pitsouni E. I., Malietzis G. A., Pappas G. (2008). Comparison of PubMed, Scopus, web of science, and Google scholar: strengths and weaknesses. FASEB J. 22, 338–342. doi: 10.1096/fj.07-9492LSF
Flores A., Wiff R., Donovan C. R., Gálvez P. (2024). Applying machine learning to predict reproductive condition in fish. Ecol. Inform. 80, 102481. doi: 10.1016/j.ecoinf.2024.102481
Gladju J., Kamalam B. S., Kanagaraj A. (2022). Applications of data mining and machine learning framework in aquaculture and fisheries: A review. Smart Agric. Technol. 2, 100061. doi: 10.1016/j.atech.2022.100061
Hassan-Montero Y., De-Moya-Anegón F., Guerrero-Bote V. P. (2022). SCImago Graphica: a new tool for exploring and visually communicating data. Prof Inform 31 (5), e310502. doi: 10.3145/epi.2022.sep.02
Huang X., Fan X., Ying J., Chen S. (2019). Emerging trends and research foci in gastrointestinal microbiome. J. Transl. Med. 17, 1–11. doi: 10.1186/s12967-019-1810-x
Janiesch C., Zschech P., Heinrich K. (2021). Machine learning and deep learning. Electron. Mark. 31, 685–695. doi: 10.1007/s12525-021-00475-2
Kaur G., Adhikari N., Krishnapriya S., Wawale S. G., Malik R. Q., Zamani A. S., et al. (2023). Recent advancements in deep learning frameworks for precision fish farming opportunities, challenges, and applications. J. Food Quality. 2023, 4399512. doi: 10.1155/2023/4399512
Khokher M. R., Little L. R., Tuck G. N., Smith D. V., Qiao M., Devine C., et al. (2022). Early lessons in deploying cameras and artificial intelligence technology for fisheries catch monitoring: where machine learning meets commercial fishing. Can. J. Fish. Aquat. Sci. 79, 257–266. doi: 10.1139/cjfas-2020-0446
Kong Q., Du R., Duan Q., Zhang Y., Chen Y., Li D., et al. (2022). A recurrent network based on active learning for the assessment of fish feeding status. Comput. Electron. Agr. 198, 106979. doi: 10.1016/j.compag.2022.106979
Li D., Wang Q., Li X., Niu M., Wang H., Liu C. (2022). Recent advances of machine vision technology in fish classification. ICES J. Mar. Sci. 79, 263–284. doi: 10.1093/icesjms/fsab264
Lilkendey J., Barrelet C., Zhang J., Meares M., Larbi H., Subsol G., et al. (2024). Herbivorous fish feeding dynamics and energy expenditure on a coral reef: Insights from stereo-video and AI-driven 3D tracking. Ecol. Evol. 14, e11070. doi: 10.1002/ece3.11070
Liu C., Wang Z., Li Y., Zhang Z., Li J., Xu C., et al. (2023). Research progress of computer vision technology in abnormal fish detection. Aquacult. Eng. 103, 102350. doi: 10.1016/j.aquaeng.2023.102350
Lopez-Marcano S., Jinks E. L., Buelow C. A., Brown C. J., Wang D., Kusy B., et al. (2021). Automatic detection of fish and tracking of movement for ecology. Ecol. Evol. 11, 8254–8263. doi: 10.1002/ece3.7656
Mandal A., Ghosh A. R. (2024a). Role of artificial intelligence (AI) in fish growth and health status monitoring: A review on sustainable aquaculture. Aquacult. Int. 32, 2791–2820. doi: 10.1007/s10499-023-01297-z
Mandal A., Ghosh A. R. (2024b). AI-driven surveillance of the health and disease status of ocean organisms: a review. Aquacult. Int. 32, 887–898. doi: 10.1007/s10499-023-01192-7
Mao G., Huang N., Chen L., Wang H. (2018). Research on biomass energy and environment from the past to the future: A bibliometric analysis. Sci. Total. Environ. 635, 1081–1090. doi: 10.1016/j.scitotenv.2018.04.173
Matley J. K., Klinard N. V., Larocque S. M., McLean M. F., Brownscombe J. W., Raby G. D., et al. (2023). Making the most of aquatic animal tracking: a review of complementary methods to bolster acoustic telemetry. Rev. Fish. Biol. Fisher. 33, 35–54. doi: 10.1007/s11160-022-09738-3
Nalmpanti M., Chrysafi A., Meeuwig J. J., Tsikliras A. C. (2023). Monitoring marine fishes using underwater video techniques in the Mediterranean Sea. Rev. Fish. Biol. Fisher. 33, 1291–1310. doi: 10.1007/s11160-023-09799-y
Ou L., Liu B., Chen X., He Q., Qian W., Li W., et al. (2023). Automatic classification of the phenotype textures of three Thunnus species based on the machine learning SVM algorithm. Can. J. Fish. Aquat. Sci. 80, 1221–1236. doi: 10.1139/cjfas-2022-0270
Perianes-Rodriguez A., Waltman L., Van Eck N. J. (2016). Constructing bibliometric networks: A comparison between full and fractional counting. J. Informetr. 10, 1178–1195. doi: 10.1016/j.joi.2016.10.006
Saberioon M., Gholizadeh A., Cisar P., Pautsina A., Urban J. (2017). Application of machine vision systems in aquaculture with emphasis on fish: state-of-the-art and key issues. Rev. Aquacult. 9, 369–387. doi: 10.1111/raq.12143
Saleh A., Sheaves M., Rahimi Azghadi M. (2022). Computer vision and deep learning for fish classification in underwater habitats: A survey. Fish 23, 977–999. doi: 10.1111/faf.12666
Santos de Moura M., Vianna M. (2020). A new threat: assessing the main interactions between marine fish and plastic debris from a scientometric perspective. Rev. Fish. Biol. Fisher. 30, 623–636. doi: 10.1007/s11160-020-09621-z
Segaran T. C., Aouissi H. A., Noor M. I. M., Wahid M. E. A., Lananan F., Petrisor A. I., et al. (2023). Assessing the state of seahorse research through scientometric analysis: an update. Rev. Fish. Biol. Fisher. 33, 1237–1262. doi: 10.1007/s11160-023-09794-3
Shin H. C., Roth H. R., Gao M., Lu L., Xu Z., Nogues I., et al. (2016). Deep convolutional neural networks for computer-aided detection: CNN architectures, dataset characteristics and transfer learning. IEEE T. Med. Imaging. 35, 1285–1298. doi: 10.1109/TMI.2016.2528162
Signaroli M., Lana A., Martorell-Barceló M., Sanllehi J., Barcelo-Serra M., Aspillaga E., et al. (2022). Measuring inter-individual differences in behavioural types of gilthead seabreams in the laboratory using deep learning. PeerJ 10, e13396. doi: 10.7717/peerj.13396
Snoek J., Larochelle H., Adams R. P. (2012). Practical bayesian optimization of machine learning algorithms. Adv. Neural Inf. Process. Syst. 25, 1–9.
Stock M., Nguyen B., Courtens W., Verstraete H., Stienen E., De Baets B. (2021). Otolith identification using a deep hierarchical classification model. Comput. Electron. Agr. 180, 105883. doi: 10.1016/j.compag.2020.105883
van Helmond A. T., Mortensen L. O., Plet-Hansen K. S., Ulrich C., Needle C. L., Oesterwind D., et al. (2020). Electronic monitoring in fisheries: lessons from global experiences and future opportunities. Fish. Fish. 21, 162–189. doi: 10.1111/faf.12425
Vo T. T. E., Ko H., Huh J. H., Kim Y. (2021). Overview of smart aquaculture system: Focusing on applications of machine learning and computer vision. Electronics 10, 2882. doi: 10.3390/electronics10222882
Wu X., Chu Y., Wang Z., Lou X., Dong Y., Wang B., et al. (2024). Quality non-destructive sorting of large yellow croaker based on image recognition. J. Food Eng. 383, 112227. doi: 10.1016/j.jfoodeng.2024.112227
Wu Y., Duan Y., Wei Y., An D., Liu J. (2022). Application of intelligent and unmanned equipment in aquaculture: A review. Comput. Electron. Agr. 199, 107201. doi: 10.1016/j.compag.2022.107201
Xiao G., Pan L., Lai F. (2025). Application, opportunities, and challenges of digital technologies in the decarbonizing shipping industry: A bibliometric analysis. Front. Mar. Sci. 1. doi: 10.3389/fmars.2025.1523267
Xu P., Xie M., Zhou W., Suo A. (2021). Research on fishery resource assessment and sustainable utilization (FRASU) during 1990–2020: a bibliometric review. Glob. Ecol. Conserv. 29, e01720. doi: 10.1016/j.gecco.2021.e01720
Xun Z., Wang X., Xue H., Zhang Q., Yang W., Zhang H., et al. (2024). Deep machine learning identified fish flesh using multispectral imaging. Curr. Res. Food Sci. 9, 100784. doi: 10.1016/j.crfs.2024.100784
Yu C., Hu Z., Han B., Wang P., Zhao Y., Wu H. (2021). Intelligent measurement of morphological characteristics of fish using improved U-Net. Electronics 10, 1426. doi: 10.3390/electronics10121426
Yu G., Zhang J., Chen A., Wan R. (2023). Detection and identification of fish skin health status referring to four common diseases based on improved YOLOv4 Model. Fishes 8, 186. doi: 10.3390/fishes8040186
Zhang H., Xie P. (2023). The mechanisms of microcystin-LR-induced genotoxicity and neurotoxicity in fish and mammals: Bibliometric analysis and meta-analysis. Sci. Total. Environ. 905, 167018. doi: 10.1016/j.scitotenv.2023.167018
Keywords: bibliometrics, computer vision, deep learning, ichthyology, visualization
Citation: Ou L, Lu L, Qian W and Liu B (2025) Application of artificial intelligence in fish information identification: a scientometric perspective. Front. Mar. Sci. 12:1575523. doi: 10.3389/fmars.2025.1575523
Received: 12 February 2025; Accepted: 18 March 2025;
Published: 15 April 2025.
Edited by:
Stephen J. Newman, Western Australian Fisheries and Marine Research Laboratories, AustraliaReviewed by:
Felipe Polivanov Ottoni, Federal University of Maranhão, BrazilGuangnian Xiao, Shanghai Maritime University, China
Copyright © 2025 Ou, Lu, Qian and Liu. This is an open-access article distributed under the terms of the Creative Commons Attribution License (CC BY). The use, distribution or reproduction in other forums is permitted, provided the original author(s) and the copyright owner(s) are credited and that the original publication in this journal is cited, in accordance with accepted academic practice. No use, distribution or reproduction is permitted which does not comply with these terms.
*Correspondence: Liguo Ou, bGdvdUB6am91LmVkdS5jbg==; Weiguo Qian, cWlhbi13ZWlndW9Ac29odS5jb20=; Bilin Liu, YmwtbGl1QHNob3UuZWR1LmNu