- 1State Key Laboratory of Marine Geology, Tongji University, Shanghai, China
- 2Project Management Office of China National Scientific Seafloor Observatory, Tongji University, Shanghai, China
- 3Laoshan Laboratory, Qingdao, China
- 4School of Electronic and Information Engineering, Tongji University, Shanghai, China
Autonomous profiling floats, such as the Argo floats, predominantly rely on battery power for their energy supply. However, the limited energy storage capacity of batteries imposes significant constraints on their operational lifespan, observation frequency, and the integration of advanced sensors, which has emerged as a critical bottleneck hindering long-term autonomous observations. To address this issue, researchers have explored two primary technical routes: optimizing energy consumption and harvesting energy. This review first systematically analyzes the research progress concerning the energy consumption characteristics of autonomous profiling floats. It then summarizes the key technical strategies and advancements in current energy consumption optimization efforts across four domains: hydraulic system, sensor system, satellite communication system, and control algorithm. Subsequently, the paper reviews the developments and challenges associated with self-powered autonomous profiling floats, with a particular focus on the application of phase-change-material (PCM)-based thermal energy harvesting technology. Finally, the paper proposes that future endeavors should concentrate on advancing energy management and energy development technologies. These include the adoption of Edge Artificial Intelligence (Edge AI) technology for intelligent energy management, flexible solar cells and underwater photovoltaic technologies, Triboelectric Nanogenerator (TENG) technology for wave energy harvesting, novel thermal energy harvesting techniques, and hybrid energy harvesting solutions. By promoting energy diversification and enhancing efficiency, these innovations can strengthen the energy security for autonomous profiling floats and meet the increasing demands for scientific observation.
1 Introduction
Autonomous profiling floats (hereafter referred to as profiling floats) are compact, expendable disposable monitoring devices capable of autonomously collecting ocean profile data. These floats are lightweight, easily deployable, and operational all year round across most global oceans. Since its inception in the 1990s, the Argo (Array for Real-time Geostrophic Oceanography) program has significantly advanced the development of this float technology (Gould, 2005). As of March 2025, 4,153 Argo floats (the profiling floats used in the Argo program) are in operation worldwide, and more than three million data archives have been publicly released.1 These data provide extensive three-dimensional ocean observations for oceanographic research, climate studies, weather forecasting, and ecosystem monitoring (Riser et al., 2016; Johnson et al., 2022; Johnson and Fassbender, 2023; Liu et al., 2023c). Consequently, Argo has been recognized as the “crown jewel” of ocean observing systems (National Oceanic and Atmospheric Administration, 2024).
Most profiling floats exhibit similar design characteristics and operational principles (Swift and Riser, 1994; Langebrake et al., 2002; Schwithal and Roman, 2009; Ward et al., 2014; Zhu et al., 2015; Viswanathan and Taher, 2016; Leymarie et al., 2018; Le Mézo et al., 2020; Chen et al., 2022; Moum et al., 2023; Babić et al., 2024). Structurally, profiling floats are primarily cylindrical. However, those designed for deep-sea operations, typically beyond 4,000 meters, are often spherical to enhance pressure resistance while maintaining generally consistent internal components. Taking the core Argo float as an example, it can be divided into six components: satellite communication system, sensor system, control system, hydraulic system, battery, and shell (Figure 1). The float relies on a hydraulic system for movement, with an internal reservoir storing oil. A hydraulic pump enables the transfer of oil between the internal reservoir and the external bladder, thereby altering the bladder’s volume to control the float’s ascent or descent in seawater. An antenna facilitates satellite communication, enabling data transmission, position determination, and mission updates. High-precision oceanographic sensors, such as CTD sensors, are installed at the top of the float.
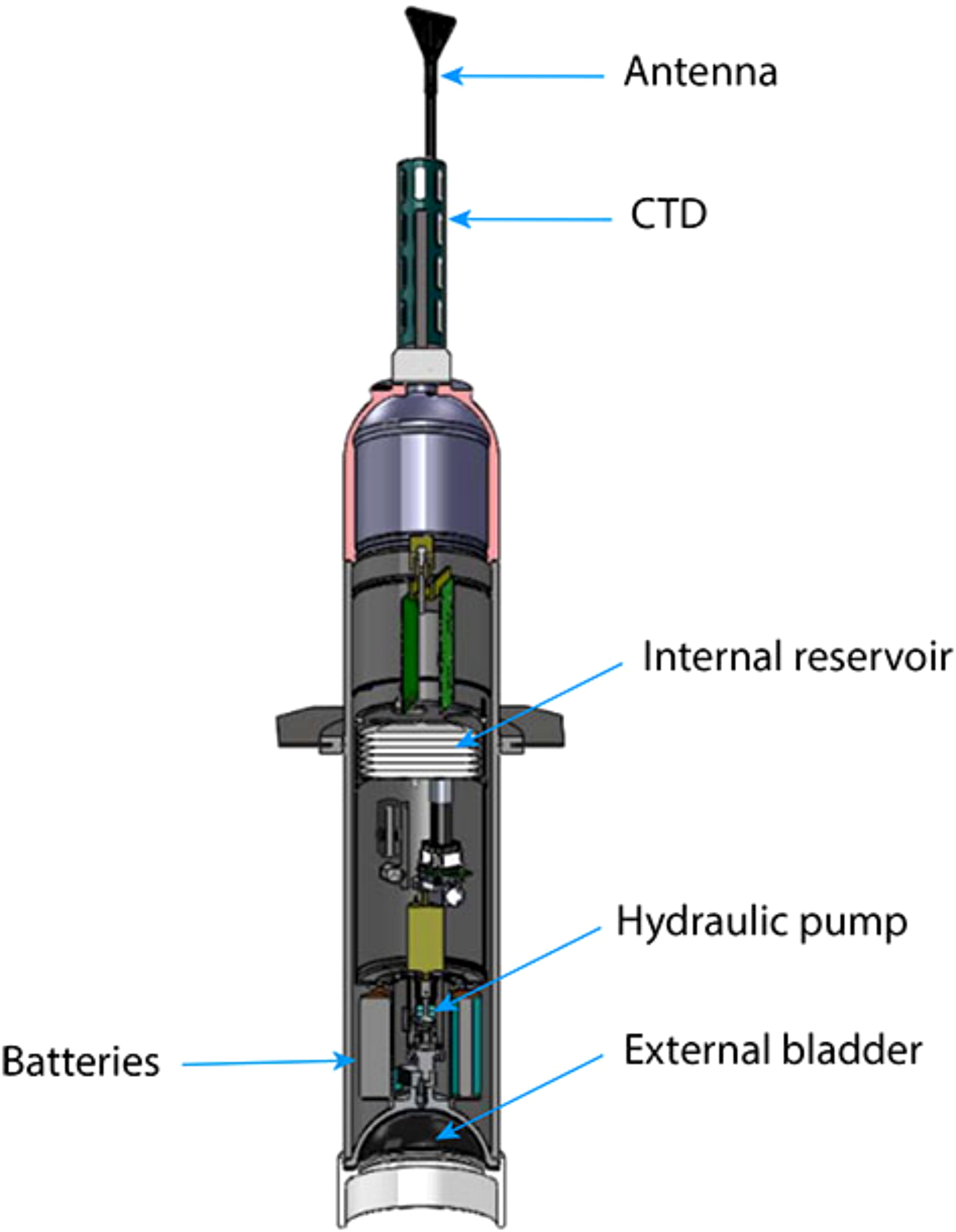
Figure 1. Schematic of the basic components of a core Argo float (Morris et al., 2024).
Profiling floats operate in a cyclical mode. For instance, a core Argo float completes a cycle in approximately ten days (Figure 2). It sinks to a drift depth of 1,000 meters for about 9 days, drifting with deep ocean currents. Subsequently, the float sinks to its profile depth of 2,000 meters or greater. During its ascent to the surface, it measures key environmental parameters, such as conductivity, temperature, and pressure. At the surface, the float performs satellite positioning and data transmission before sinking again to repeat the cycle.
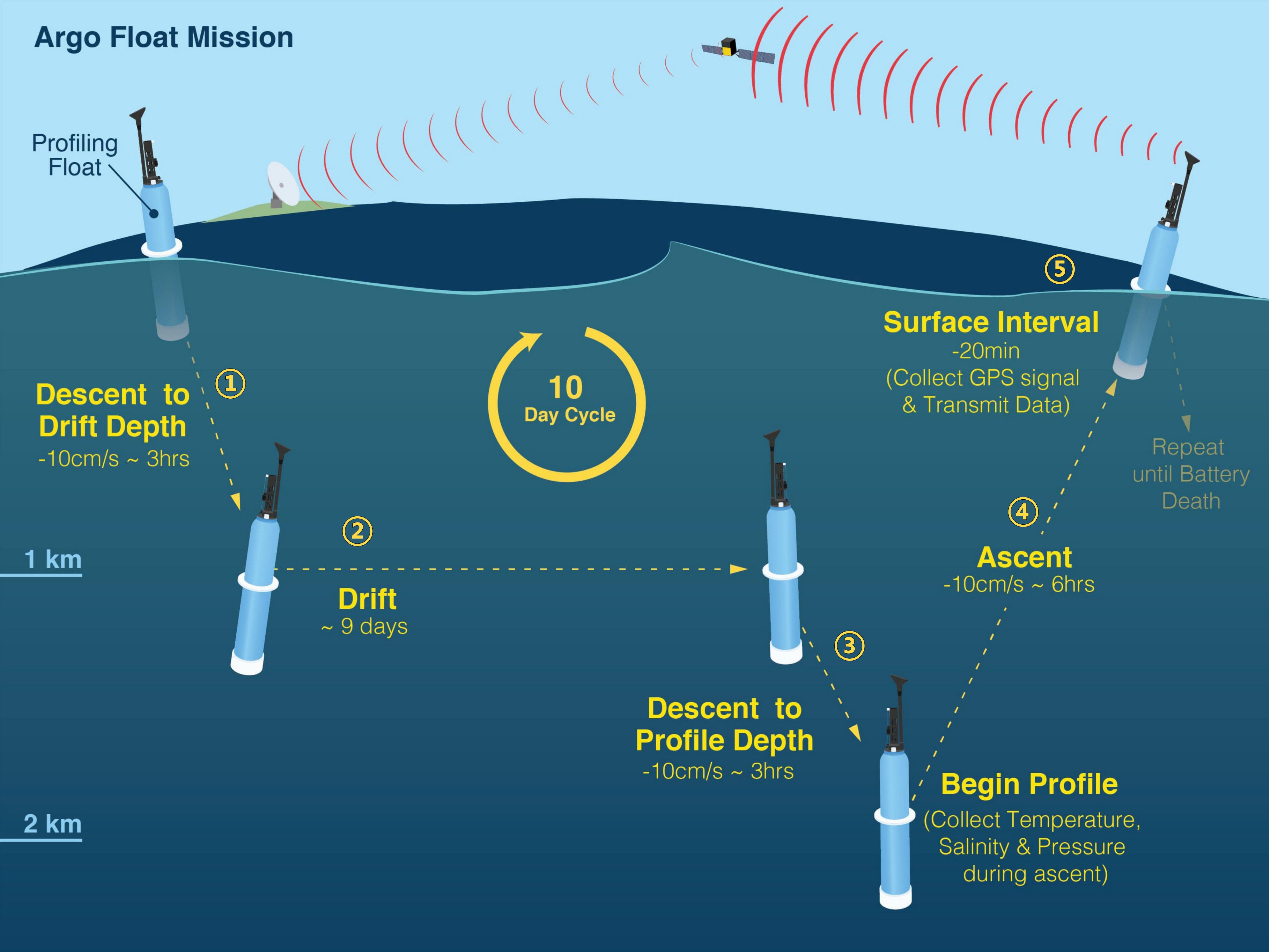
Figure 2. Operational workflow of a core Argo float (Wong et al., 2020).
Currently, most profiling floats rely entirely on battery power. Their limited energy capacity necessitates strategies such as reducing sampling frequency (Argo data management, 2022), restricting deep-sea profiling cycles (Le Reste et al., 2016), and extending drift durations to prolong operational lifespan. Given the high costs associated with deployment and recovery, the majority of profiling floats are not retrieved or maintained after deployment, and the floats cease to function once their battery power is exhausted. Argo floats typically operate for four to five years before their battery depletes, causing them to sink. To sustain the Argo observation network, international organizations deploy 500 to 600 additional floats annually. Each Argo float costs between $25,000 and $185,000, with the program’s annual expenditure exceeding $40 million.2
With the growing demand for scientific observations, the integration of additional sensors has become increasingly urgent, further intensifying energy consumption (Johnson et al., 2009; Roemmich and The Argo Steering, 2009; Johnson and Claustre, 2016). Consequently, minimizing the operational power consumption of profiling floats and enhancing their energy harvesting capabilities to ensure a reliable energy supply have emerged as critical challenges hindering advancements in profiling float technology. Thus, addressing these challenges is essential to support additional sensors and extend operational lifespan (Roemmich et al., 2019a). This paper provides a comprehensive review of current research on profiling float energy management, focusing on two primary aspects: optimizing energy consumption and harvesting energy. Based on the information presented, potential future directions for profiling float energy management and harvesting are discussed. In essence, ensuring a sufficient energy supply will enhance the observation capabilities (Muthuvel et al., 2018), reduce costs, and mitigate the environmental impact of float abandonment.
2 Research progress on low-power consumption strategies for autonomous profiling floats
2.1 Analysis of energy consumption in autonomous profiling floats
The work cycle of profiling floats can be divided into four phases: descent, drift, ascent, and communication (Figure 2, Table 1). During the drift phase, the control system transitions to sleep mode, while other systems are temporarily powered off. Consequently, power consumption during this phase is minimal and it is often omitted from motion energy analysis.
Statistical analysis indicates that the primary energy consumption of profiling floats during a work cycle (Figure 2) is mainly allocated to hydraulic drive, satellite communication, sensor measurement, and system control (Table 2). Accordingly, the research on low-power consumption strategies has been primarily focused on these four aspects (Figure 3). Among these, the hydraulic system constitutes the most energy-intensive component, thereby becoming a major focus of optimization efforts. For APEX floats, which are the most widely deployed type in the Argo program, the hydraulic system consumes approximately 28% of the total energy consumption, whereas, for Deep SOLO floats operating at depths of up to 6,000 meters, this proportion reaches as high as 78% (Roemmich et al., 2019b).
As an autonomous platform, the energy consumption of each component of the profiling float is interdependent to a certain extent (Figure 3). For example, increasing sensor sampling frequency not only raises the power consumption of individual sensors but also generates larger volumes of observational data. This increase in data volume imposes additional processing requirements on the control system (e.g., for data compression) and increases the burden on satellite communication. Given the limited bandwidth of satellite communications, transmitting large volumes of data may necessitate extended communication durations, thereby significantly increasing the energy consumption of the satellite communication system. Consequently, effective energy management requires a system-level approach to minimize overall power consumption.
To develop the low-power strategy, it is typically necessary to first analyze the motion model of the float and subsequently construct an energy consumption model to analyze the factors affecting energy consumption under different motion states of profiling floats (Yang et al., 2019). When constructing a motion model, most researchers tend to simplify the analysis by disregarding the influence of lateral currents or the vertical variation of seawater density, focusing primarily on the forces of gravity, buoyancy, and drag in the vertical direction (McGilvray and Roman, 2010; Barker, 2014; Dologlonyan and Grekov, 2018; Zou et al., 2018; Yang et al., 2019; Guo, 2020; Liu et al., 2020; Wang et al., 2020b; Wu, 2021; Wen et al., 2022). However, due to inhomogeneous mass distribution within the float, lateral flow perturbations induce an inevitable tilt angle. In this regard, Si et al. constructed a single-profile energy consumption model for the 4000-m Deep-Argo Otarriinae, incorporating 19 parameters (Si et al., 2020). It was found that the gliding angle, diving depth, and gliding speed had the greatest impact on energy consumption, while the influence of other parameters was relatively limited (Si et al., 2020). Therefore, applying a simplified model that neglects the effects of horizontal flow may lead to significant errors when researching optimizing float energy consumption.
2.2 Low-power consumption strategies for hydraulic systems
Commercially available profiling floats typically utilize a highly reliable hydraulic system as the buoyancy engine to achieve ascent and descent. This system mainly consists of an internal reservoir and external bladder, solenoid valves, a hydraulic pump, and pipelines. During the float’s movement, the hydraulic pump drives oil to flow between the internal reservoir and the external bladder, thereby changing the float’s volume to achieve ascent or descent. Due to the need for the hydraulic system to actively discharge oil to counteract the high pressure of the deep sea during the ascent phase, energy consumption during this phase is greater than in others. Therefore, researchers have often focused on modeling and analyzing the energy consumption of the hydraulic system during the ascent phase.
When constructing an energy consumption analysis model for hydraulic systems (Table 3), studies generally employ numerical analysis methods such as the Runge-Kutta method, to solve differential equations and obtain solutions that minimize power consumption (Chen et al., 2017; Zou et al., 2018; Wang et al., 2020b). Some researchers have also developed simulation models (Dologlonyan and Grekov, 2018; Yang et al., 2019; Guo, 2020) and applied the Non-dominated Sorting Genetic Algorithm II (NSGA-II) (Liu et al., 2019b) for energy consumption analysis.
After conducting an energy consumption analysis of the hydraulic system, researchers primarily focused on low-power technology in areas such as controlling operating speed, managing oil discharge frequency, and optimizing the hydraulic system’s structural design.
2.2.1 Optimal speed intervals-based low-power oil discharge strategy
According to the energy consumption analysis, factors such as gliding angle, diving depth, and gliding speed significantly impact the energy consumption of profiling floats (Si et al., 2020). The gliding angle is determined by the float’s mechanical properties and the environment, the diving depth by observation requirements, and the gliding speed mainly by the float’s oil discharge strategy. Therefore, researchers have often controlled operating speeds to achieve low-power movement.
Wen et al. used the calculus of variations method and found that in areas with small seawater density changes, uniform motion could achieve the best energy-saving effect when the initial and final speeds during ascent were the same, given the same ascent time and distance (Wen et al., 2022). However, in hydraulic systems, discharging oil requires a certain amount of time, resulting in a lag in speed changes, making it challenging to consistently maintain a specific speed (Dologlonyan and Grekov, 2018). Therefore, the design of low-power oil discharge strategies must ensure that oil discharge is regulated to maintain the float’s operating speed within the optimal speed interval [umin, umax] at all times (Chen et al., 2017; Wang et al., 2020b). It has been suggested that under conditions where the sensor’s normal operation for data acquisition is maintained, reducing the minimum speed threshold (umin) could decrease energy consumption (Wang et al., 2020b).
Upon the determination of the optimal speed interval, closed-loop feedback control for oil discharge is commonly utilized (Wen et al., 2022). In cases where speed dips below the minimum threshold (umin), oil discharge is boosted to reach maximum speed (umax), thus guaranteeing continuous operation within the set range. It has been demonstrated that a reasonable optimal speed interval can achieve a balance between energy saving and time saving. Chen et al.’s strategy reduced the motor energy consumption during the ascent phase of the deep-sea profiling float by approximately 51.16% (Chen et al., 2017). Nevertheless, an improper setting of the speed interval may result in the frequent activation of motors and pumps, heightening the risk of equipment wear (Wang et al., 2020b).
2.2.2 Optimal frequency-based low-power oil discharge strategy
Since the float’s movement is achieved through both oil discharge and return operations, directly controlling the change in oil volume is simpler than controlling the movement speed. In 2013, Petzrick proposed that pumping in small increments during ascent could minimize energy loss when pumping oil under high pressure in deep-sea conditions (Petzrick et al., 2013). Other researchers found that, for the same oil discharge volume, total power consumption exhibited a nonlinear relationship with the oil drainage frequency (Mu et al., 2018; Wang et al., 2020b, 2022). By employing numerical calculations (Mu et al., 2018), simulation analyses (Wang et al., 2020b; Wang, 2020), multi-objective optimization (Liu et al., 2019b), adaptive genetic algorithms (Zhi et al., 2021), and experimental measurements (Wang et al., 2022) to determine the optimal discharge frequency, significant reductions in power consumption were achieved. For instance, the staged oil discharge strategy established through simulation analysis saved approximately 24.2% more energy compared to a single discharge (Wang, 2020). Considering the increase in static energy consumption, the oil discharge strategy obtained through NSGA-II reduced dynamic energy consumption during the floating process by 28.9% within 2 hours (Liu et al., 2019b). Sea tests revealed that the discharge strategy determined through the reliability testing saved approximately 22.5% of the total energy consumption of the FUXING float (Wang et al., 2022).
It is worth noting that while controlling the frequency of oil discharges reduces the difficulty of control, each oil discharge causes a sudden increase followed by a decrease in the speed of the float. Additionally, variations in seawater pressure at different depths lead to differing speed changes. Therefore, in practical applications, it is essential to set an appropriate amount of oil discharge and discharge frequency to ensure that sampling during the ascent process remains as uniform as possible while avoiding equipment wear caused by frequent oil discharges.
2.2.3 Structural design optimization-based low-power consumption technology for hydraulic system
The installation of hydraulic accumulators is a key technology for energy saving in most hydraulic systems (Rydberg, 2005). Currently, the most commonly used type in hydraulic circuits is the hydropneumatic accumulator (Costa and Sepehri, 2023). When the system pressure is high, the accumulator converts hydraulic energy into the internal energy of the gas for storage. When the system pressure is low, it converts the internal energy of the gas back into hydraulic energy for release, thereby reducing the frequent start-up of the hydraulic pump and minimizing energy loss, which improves the overall energy efficiency of the system. In Argo floats such as the Webb N2 APEX and SOLO floats, similar principles of accumulators are employed, using air pumps or nitrogen gas canisters in conjunction with the hydraulic system to reduce energy consumption (Davis et al., 2001; Roemmich et al., 2009).
The hydraulic pump, as the core component of the hydraulic system, directly affects the performance and energy consumption of the entire system. Profiling floats primarily use plunger pumps as the power source. Improving the volumetric efficiency of plunger pumps can not only reduce energy waste but also extend the service life of the equipment. It has been found that hydraulic systems in deep-sea high-pressure environments are prone to airlock phenomena (i.e., small vacuum bubbles entering the circuit causing the pump to malfunction) and a significant decrease in the volumetric efficiency of plunger pumps, which can be overcome by introducing air pumps (Wang et al., 2022) or adjusting the hydraulic design parameters (Zhao, 2019). Additionally, it has been found that optimal buoyancy, determined by precise oil transfer through ballast weight, can lead to more effective energy consumption (Veeraragavan et al., 2022).
2.3 Low-power consumption strategies for sensor system and satellite communication system
The energy consumption of the sensor system and satellite communication system of profiling floats occurs during the processes of data generation, processing, and transmission. Thus far, low power consumption has been primarily achieved by reducing data volume and decreasing working time.
The sensors on profiling floats can be divided into ocean observation sensors and auxiliary operational sensors. The latter typically utilize mature micro-sensors, which consume minimal power. However, the continuous operation of ocean observation sensors during the ascent process can lead to increased energy consumption. For instance, the energy consumption ratio of the sensors on the SOCCOM APEX float, equipped with multiple biogeochemical sensors can reach as high as 41.4% (Riser et al., 2018). Furthermore, the volume of data collected during observations is directly related to the energy consumption of subsequent satellite communications.
The observation sampling frequency of profiling floats depends on their design, sensor type, and task requirements, and different floats may have different sampling frequencies at varying depths. To reduce power consumption, it was proposed that extracting a set of characteristic values from multiple groups of data measured within a certain depth interval to represent the true values within that interval would decrease the subsequent data transmission volume (Liu, 2020). However, reducing the volume of data may lead to the inability to observe high-frequency changes in the ocean and the omission of critical ocean data. To balance data recording and energy consumption, the Argo program suggests some sampling strategies for several float types (Argo data management, 2022). For the Provor Bio 5.0 Argo float equipped with a Seabird CTD and Wetlab Saraover optical sensor, the primary sampling (CTD sampling) is typically set to occur every 25 dbar from the bottom to 200 dbar and every 10 dbar from 200 dbar to the surface, while the secondary sampling (chlorophyll sampling) is typically set to occur every 10 dbar from 1000 to 300 dbar and every 1 dbar from 300 dbar to the surface (Argo data management, 2022).
Once the data collection of the profiling floats is completed, communication and positioning are conducted on the sea surface. During this phase, energy consumption is primarily influenced by the transmission efficiency of the satellite system, operational duration, and hardware power consumption. Initially, the profiling floats primarily utilized the ARGOS satellite system (0.06 kByte/min) to obtain location and transmit data. Its unidirectional transmission mechanism necessitated prolonged sea surface floating durations (6–12 hours) for data transmission and positioning operations, thereby significantly elevating the risk of encountering surface obstructions (such as ships, sea ice, or flotsam) by 3–6 times (Morris et al., 2024). Since 2005, the profiling floats have adopted GPS for location acquisition and have utilized Iridium satellites for bidirectional communication in either SBD (approximately 1 kByte/min) or RUDICS (approximately 12 kByte/min) mode (André et al., 2020). This significantly reduced communication time, with the transmission of 1000 temperature/salinity (T/S) standard profile data taking only about 7 minutes (Gruber et al., 2007). For instance, an APEX Argo (7553) float equipped with the Daytona 9522A Iridium module had a power consumption of 4.2 W during communication connection, was capable of transmitting 160 bytes per second, and its GPS module had a power consumption of 0.221 W, with a typical GPS positioning time of 120 seconds (Gordon, 2017). As of February 2025, the usage of the Iridium communication system in the global Argo observation network had reached 97.54%.3
The COPEX float, HM2000 float, and FUXING float developed in China all utilize the BeiDou Navigation Satellite System as their communication method, which features positioning, navigation, timing, and short message communication services (Lu et al., 2016; Liu et al., 2019a; Wang et al., 2022). Currently, the latest BeiDou-3 system provides a short message communication service capable of handling 10 million communications per hour for China and surrounding regions, with a receiver transmission power of 1–3 W, and supports a maximum of 14,000 bits per single communication, while the global short message communication service supports a maximum of 560 bits per single communication (China Satellite Navigation Office, 2019). Furthermore, a low-energy transmission terminal based on the BeiDou system was developed for Argo floats through strategic power-state modulation. By deactivating hardware components during non-transmission intervals and leveraging multi-stage STOP-mode transitions, the system demonstrated a 77.282% decrease in single communication energy demand compared to continuous power-dissipation configurations (Li et al., 2024).
Due to the limited satellite bandwidth, transmitting large volumes of data typically necessitates a prolonged transmission period, thereby leading to increased energy consumption. Researchers have frequently employed lossless data compression methods to enhance transmission efficiency while maintaining data integrity and accuracy. For example, a lossless compression rate of 26% was achieved using the LZSS method (Xie, 2020), and a 25.9% compression rate was realized with a Differential coding-Huffman method (Li et al., 2024). Additionally, a novel block-wise lossless compression method was developed for Argo floats data, integrating bidirectional LSTM networks, multi-head self-attention mechanisms, and multilayer perceptrons. This method achieved a compression rate of 12.11% on both PC and Jetson Nano platforms (Guo et al., 2024).
2.4 Control algorithm optimization-based low-power consumption strategy
Profiling floats are autonomously operated instruments, and control algorithms are required to achieve precise buoyancy adjustment, motion control, data collection and transmission management, and energy allocation.
The most classic control algorithm employed is proportional-integral-derivative (PID) control (Borase et al., 2021), especially in control systems that require maintaining a set point. It is simple and easy to use, thus widely utilized in the depth control of underwater vehicles (McGilvray and Roman, 2010; Morales-Aragón et al., 2025). However, the PID algorithm parameters are fixed, while the float experiences fluctuations in pressure and flow during the diving process. Therefore, a fixed-parameter PID controller may not be able to adjust the control signal in some situations, leading to slower system response, overshoot, and increased energy loss (Mu et al., 2019; Li et al., 2023). In addition, there is a trade-off between power consumption optimization and operational performance metrics during the hydrodynamic adjustment phases (Carneiro et al., 2024). The PID controller is typically optimized for a single objective, making it difficult to achieve global optimization and thus unable to attain the best energy-saving effect.
Some research efforts have focused on developing control algorithms to further reduce energy consumption. Studies have shown that advanced control algorithms based on PID have yielded limited improvements in energy efficiency (Mu et al., 2019; Yang et al., 2019). Therefore, alternative control algorithms have been proposed. For example, motion planning based on genetic algorithms was implemented for a 4000-meter profiling float, reducing energy consumption to 1/50 of that of dual closed-loop fuzzy PID control and RBF-PID methods, while maintaining the same accuracy (Zheng et al., 2021). A speed closed-loop control strategy was proposed for a 4000-m profiling float, resulting in an 18% improvement in energy efficiency compared to the traditional control strategy when the set speed was 1 m/s (Liu et al., 2020).
3 Research progress in energy harvesting technology for autonomous profiling floats
While low-power strategies can extend the operational lifespan of floats, the inherent limitations of battery-based energy supplies remain. Traditional alkaline batteries in Argo floats have been replaced with lithium batteries (Johnson and Fassbender, 2023), achieving an average lifespan of 1,396 days by March 1, 2025.4 The development of environmental energy harvesting technologies and self-powered autonomous profiling floats represents a key strategy to overcome these limitations, integrate additional observation sensors, and further extend the float’s lifespan.
Currently, self-powered autonomous profiling floats are not widely commercially available, with most research still in the prototype stage. Energy harvesting technologies primarily focus on ocean thermal energy (Chao, 2016), ocean current energy (Wu et al., 2018), and energy recovery (Xue et al., 2020). Among these, ocean thermal recharging profiling floats, which exploit the substantial temperature gradients between surface and deep ocean layers to generate power, have become a major research focus due to their high seasonal and diurnal stability (Wang et al., 2020a). Over the past two decades, phase-change-material (PCM)-based thermal recharging profiling floats have been predominantly developed and studied. The technology’s increasing maturity is demonstrated through practical implementations such as SOLO-TREC and Navis-SL1.
3.1 Developments in PCM-based thermal recharging profiling floats
The PCM system stores thermal energy through phase transitions: at the ocean surface, warmer seawater causes the PCM to transition from solid to liquid, thereby storing thermal energy. As the float descends into colder waters, the PCM returns to its solid state. This cyclical phase change drives a hydroelectric generator through pressure differentials created by volume changes, facilitating the conversion of thermal to electrical energy (Wang et al., 2019b). Alkanes are extensively adopted as PCMs due to their superior volumetric latent heat capacity, substantial solid-to-liquid volumetric expansion coefficients, and inherent chemical inertness, which eliminates phase segregation risks and material corrosion concerns (Wang et al., 2020a).
3.1.1 Historical evolution of PCM-based thermal recharging profiling floats
Under the leadership of Yi Chao et al. at the Jet Propulsion Laboratory, a thermoelectric generator was developed based on the buoyancy engine concept of the Slocum glider (Chao, 2016). This system harnesses pressure from PCM phase-change expansion and two composite nitrogen bottles to drive 840 mL of high-pressure oil through a 1.5 cm3 hydraulic motor (Figure 4A). A gearbox amplifies the rotational speed fourfold, enabling the attached generator to produce approximately 200 W over 30 seconds. By integrating this technology into a SOLO profiling float, SOLO-TREC, the world’s first thermal recharging profiling float prototype, was developed. SOLO-TREC is 50% longer and has a weight/volume ratio approximately twice that of battery-powered SOLO floats, incorporating 10 heat exchange tubes (Buis, 2010; Chao, 2016). During sea trials from November 2009 to June 2011, SOLO-TREC completed three daily profiling measurements, generating 1.7 Wh per dive and collecting more than one thousand vertical profiles of temperature and salinity (Chao, 2016).
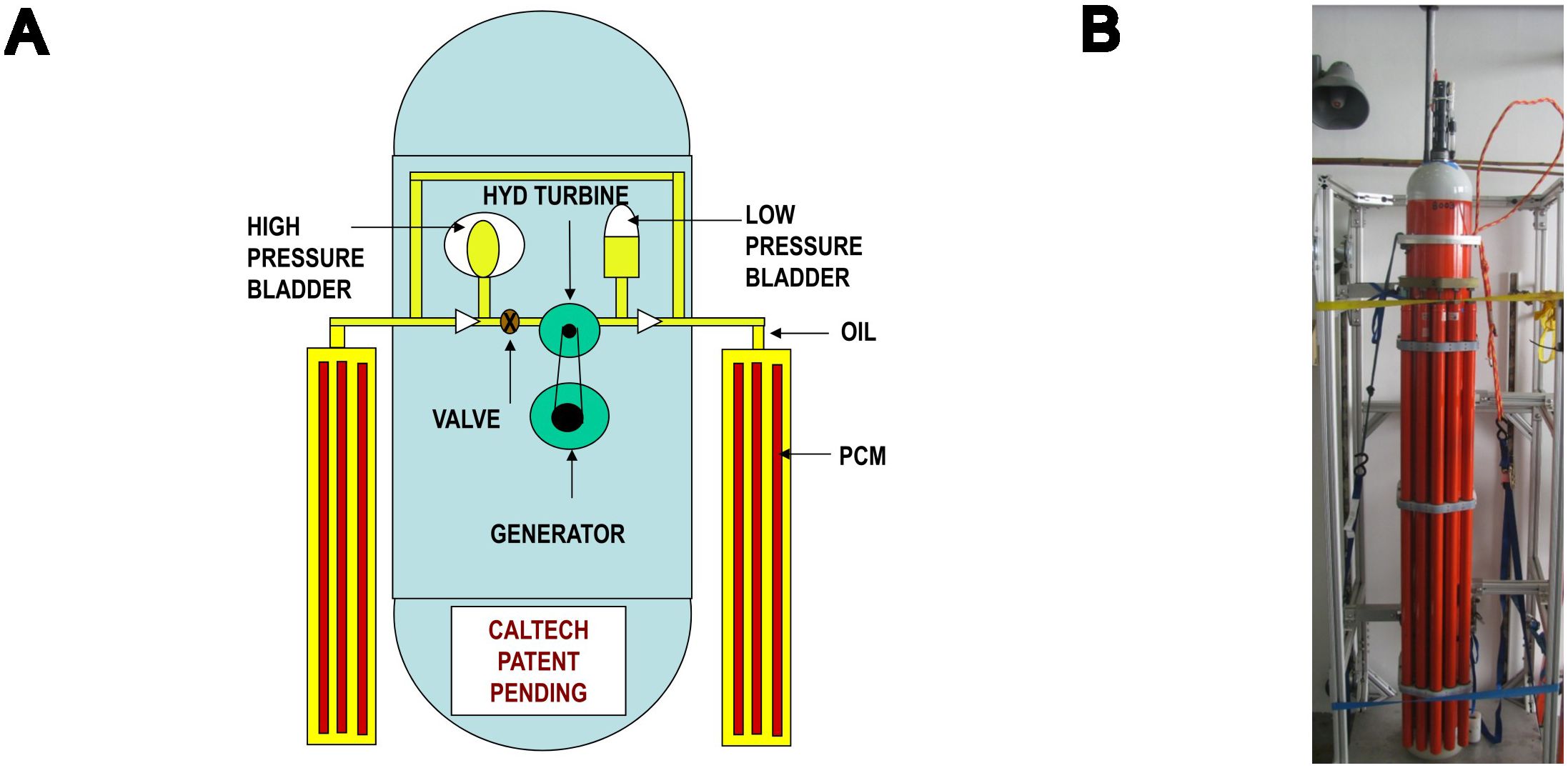
Figure 4. SOLO-TREC and commercial thermal recharging profiling floats: (A) Power generation principle of SOLO-TREC (Valdez et al., 2011); (B) SOLO-TREC prototype (Valdez et al., 2011).
Building on SOLO-TREC, Seatrec Inc. released its first commercial product, the Navis-SL1 thermal recharging profiling float, in 2019. This float can generate 2.2 Wh of energy per dive at a maximum operating depth of 1,000 meters (Seatrec Inc, 2020). In 2023, Seatrec launched the infiniTE™ thermal recharging profiling float, a modular platform with “plug-and-play” sensors, such as an echosounder, hydrophone, and CTD sensor. The infiniTE™ can conduct three profiling analyses per day at a depth of 1,000 meters and generate more than 3 Wh of energy per dive (Seatrec Inc, 2023).
Currently, there is a significant disparity in the technological maturity of thermal recharging profiling floats. SOLO-TREC, a representative achievement in this field, has undergone extensive sea trials and achieved commercial upgrades, while most other prototypes remain at the conceptual design or short-term sea trial verification stage (Figure 5). Several innovative designs have been proposed to improve energy conversion efficiency, such as optimizing the heat exchange system parameters (Wang et al., 2017a, 2018), refining key configuration parameters (Tian et al., 2014; Wang et al., 2024), enhancing the buoyancy driving system (Xia et al., 2021), and developing external power generation modules (Zhang et al., 2022a). The feasibility of these designs has been demonstrated through simulation analysis and experimental validation. However, most studies lack long-term sea trial verification and require further optimization to enhance stability and reliability in real marine environments (Tian et al., 2014; Xia et al., 2021; Wang et al., 2024).
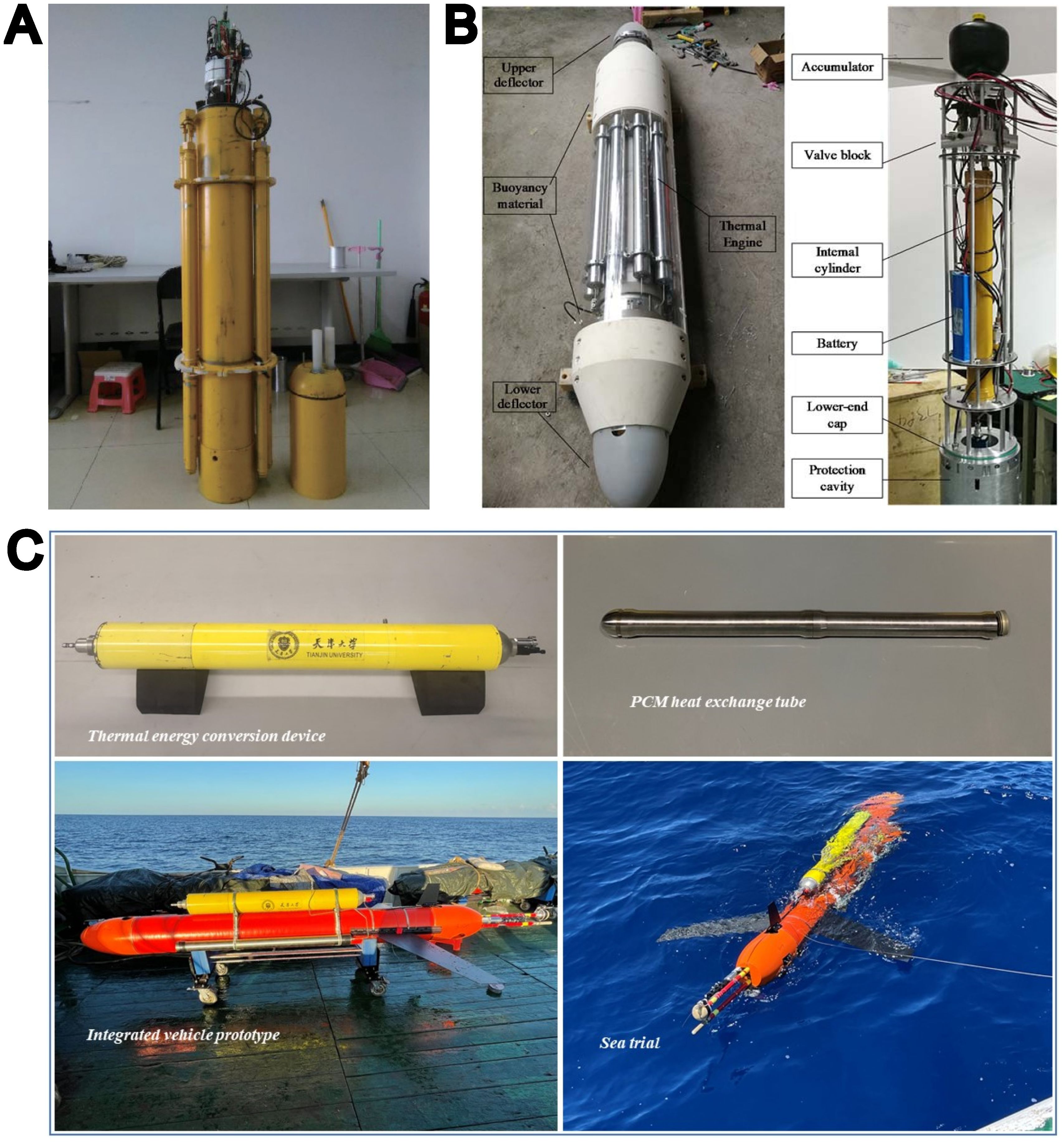
Figure 5. Thermal recharging profiling float prototype: (A) Small ocean thermal energy conversion device (Wang et al., 2018a); (B) Self-driven profiler with a buoyancy-adjusting system for ocean thermal energy (Xia et al., 2021); (C) External power generation module developed for Smart Float (Zhang et al., 2022a).
3.1.2 Challenges in the development of PCM-based thermal recharging profiling floats
Although the PCM-based thermal recharging profiling float has been successfully commercialized, the energy collection system of the most advanced SOLO-TREC series float generates only 1.7 to 3 Wh of electricity per dive (Chao, 2016; Seatrec Inc, 2023), which is insufficient to power multiple observation sensors and enable deeper observation. Furthermore, several challenges have emerged during the research process, hindering the advancement of PCM-based thermal harvesting technologies. These challenges are discussed below.
3.1.2.1 Limited temperature gradients in the marine environment
The ocean is characterized by relatively small temperature gradients. In high-latitude regions beyond 60° north and south, sea surface temperatures typically fall below 5°C, while in low-latitude areas between 20° north and south, they can exceed 25°C (Herbert et al., 2016). Except for certain marginal seas (e.g., the Mediterranean Sea, Red Sea, and Sulu Sea), temperatures in most deep-sea areas below 2,000 meters are generally below 4°C (Yasuhara and Danovaro, 2016). Consequently, the distribution of thermal energy in the ocean is uneven. Even in tropical regions, the vertical temperature gradient is typically less than 30°C, resulting in low thermal conversion efficiency. This limitation restricts the applicability of thermoelectric systems that require a temperature gradient greater than 20°C.
3.1.2.2 Low energy conversion efficiency
The energy conversion process in PCM-based thermal energy harvesting systems involves three phases: thermal-to-hydraulic (η1 ≈ 0.7%), hydraulic-to-kinetic (η2 ≈ 40%-50%), and kinetic-to-electrical energy conversion (η3 ≈ 50%-60%) (Brown et al., 2014; Haldeman et al., 2015; Shi et al., 2016; Kim et al., 2018; Wang et al., 2019a). As a result, the overall energy conversion efficiency of PCM-based unmanned underwater vehicles (UUVs) remains below 0.6% (Jung et al., 2022), constrained primarily by material properties, structural design, and energy harvesting strategies.
3.1.2.2.1 Thermodynamic modeling of PCM-Based energy conversion
To identify efficiency-limiting factors, a thermodynamic model for PCM-based thermal energy conversion was developed, focusing on the solid-liquid phase change process (Wang et al., 2018b), revealing that environments with small temperature gradients benefit from stiffer structures and PCMs with higher solid/liquid density ratios (Wang et al., 2018b). An innovative theoretical model utilizing the effective heat capacity method was proposed, incorporating the effects of PCM porosity in the solid state (Xia et al., 2018). This model addresses the limitations of traditional approaches in describing the phase change process of PCM mixed with an insoluble liquid by analyzing the volumetric change rate and melting/solidification times (Xia et al., 2018).
3.1.2.2.2 Limitations in the thermal properties of PCM materials
Alkanes, widely used as PCMs, exhibit limitations such as low thermal conductivity and thermal delamination, which hinder volumetric expansion and reduce energy conversion efficiency (Wang et al., 2020a). Strategies such as structural optimization, the addition of lightweight high-conductivity materials, and the development of PCM composites have been proposed to accelerate phase transitions and enhance thermal performance (Fan and Khodadadi, 2011; Xia et al., 2018; Wu et al., 2020; Muhammad et al., 2024; Prasad et al., 2024). For example, a recent study demonstrated that a PCM composite reduced the phase change temperature by 0.9–1.6°C and the volumetric change rate by 16–40% (Muhammad et al., 2024). Structurally, dividing the heat exchanger into smaller segments enabled nearly independent internal circulation, reducing melting time by 34% over the original design (Yao et al., 2024).
3.1.2.2.3 Inadequate thermal-mechanical coupling design optimization
The simultaneous occurrence of PCM thermal conversion and float motion creates a coupling between thermal cycling and kinematic behavior (Wang et al., 2020a). To enhance the performance of the thermal recharging profiling float, optimization studies have focused on its shape design, structure design, and control system. For instance, a genetic algorithm was developed for the multi-objective optimization of the float’s shape, resulting in an 8.9% improvement in motion performance at maximum speed (Zhou, 2023). A teardrop-shaped thermal recharging profiling float employing a neural-network-assisted genetic algorithm reduced hydrodynamic resistance by 9.2% at a speed of 0.5 m/s (Zhao et al., 2024).
Hydraulic accumulators have also been employed to accumulate gradual PCM expansion and release it collectively upon phase change completion, thereby enhancing both the volume change rate and thermal conversion efficiency (Wang et al., 2017b; Wang, 2018; Wang et al., 2019b). A hydraulic accumulator with a PCM-based energy collector achieved a 0.6% conversion efficiency within a 1-20°C temperature range without increased pressure (Wang et al., 2019b). Increasing the accumulator’s volume and pre-charge pressure was shown to improve energy storage power (Zhang et al., 2022b; Chen et al., 2024). Additionally, employing a multiple energy storage strategy in the accumulator has been demonstrated to accelerate the melting process, increasing maximum storage power by 41.52% compared to the single storage system (Chen et al., 2024).
To ensure the optimal operation of the power generation system, the application of the maximum efficiency point tracking (MEPT) control strategy is critical. A MEPT strategy designed using the grey wolf algorithm enhanced energy conversion efficiency by 25.89% under a 0.5Ω load (Zhou, 2023). An RBFNN-PSO-PID hybrid method further improved system efficiency from below 19.05% to over 34.3% while maintaining stability under variable loads (Xia et al., 2020). Furthermore, system behavior, including the mechanical efficiency of the hydraulic motor, overall system efficiency, and DC generator load current, is influenced by the pressure gradient and rotational speed when the motor and outlet pressure are fixed (Chen et al., 2020). Therefore, speed can be regulated via current to maintain peak operating efficiency (Chen et al., 2020).
In summary, overcoming the intrinsic limitations of PCM-based thermal recharging profiling floats necessitates a system-level approach that addresses interdependent material, structural, and control factors. Future research should prioritize a comprehensive investigation of heat transfer mechanisms, including improvements in PCM properties and the structural optimization of thermal-to-hydraulic energy converters, to increase thermal conversion efficiency. Developing an integrated energy conversion efficiency model is also essential for guiding component-level optimization, minimizing energy losses across all conversion phases, and establishing a theoretical foundation for more effective system design. Additionally, the use of advanced materials and technologies, such as high thermal conductivity lightweight additives or PCM composites, should be explored to accelerate phase transition dynamics and enhance volumetric expansion. Optimizing thermal-mechanical coupling designs or incorporating active control of the energy conversion process can further improve the efficiency of hydraulic-to-electrical energy transfer. Finally, integrating buoyancy regulation with energy harvesting and storage systems may enable more efficient utilization of thermal energy in marine environments (Haldeman et al., 2015).
3.2 Recent advances in alternative energy harvesting technologies
In addition to thermal energy harvesting technology, the integration of other energy harvesting technologies with profiling floats has also been explored. However, unlike PCM-based thermal recharging profiling floats, these technologies remain in the conceptual and preliminary validation phases.
In recent years, wave energy has been recognized as a high-density (2–3 kW/m²) renewable energy source that is readily accessible (Lopez et al., 2013). Several patents have been granted for the integration of hydraulic turbines (Liu et al., 2023b) and inertial electromagnetic power generation technology (Ren et al., 2019b) with profiling floats to capture energy from their wave-induced oscillations on the sea surface. An innovative profiling float design utilizing near-surface wave energy has been proposed (Figure 6A). Unlike traditional profiling floats, which rely primarily on hydraulic systems for buoyancy adjustment, this design harnesses wave-induced motion through a heave plate connected to turbines via stiff and elastic tethers (Shomberg et al., 2022). These components generate power through the flow induced by the pod’s oscillations and enable depth adjustment by modulating drag to facilitate data collection (Shomberg et al., 2024). This concept has been experimentally validated, demonstrating an electrical output of 10 W under simulated conditions (Shomberg et al., 2024).
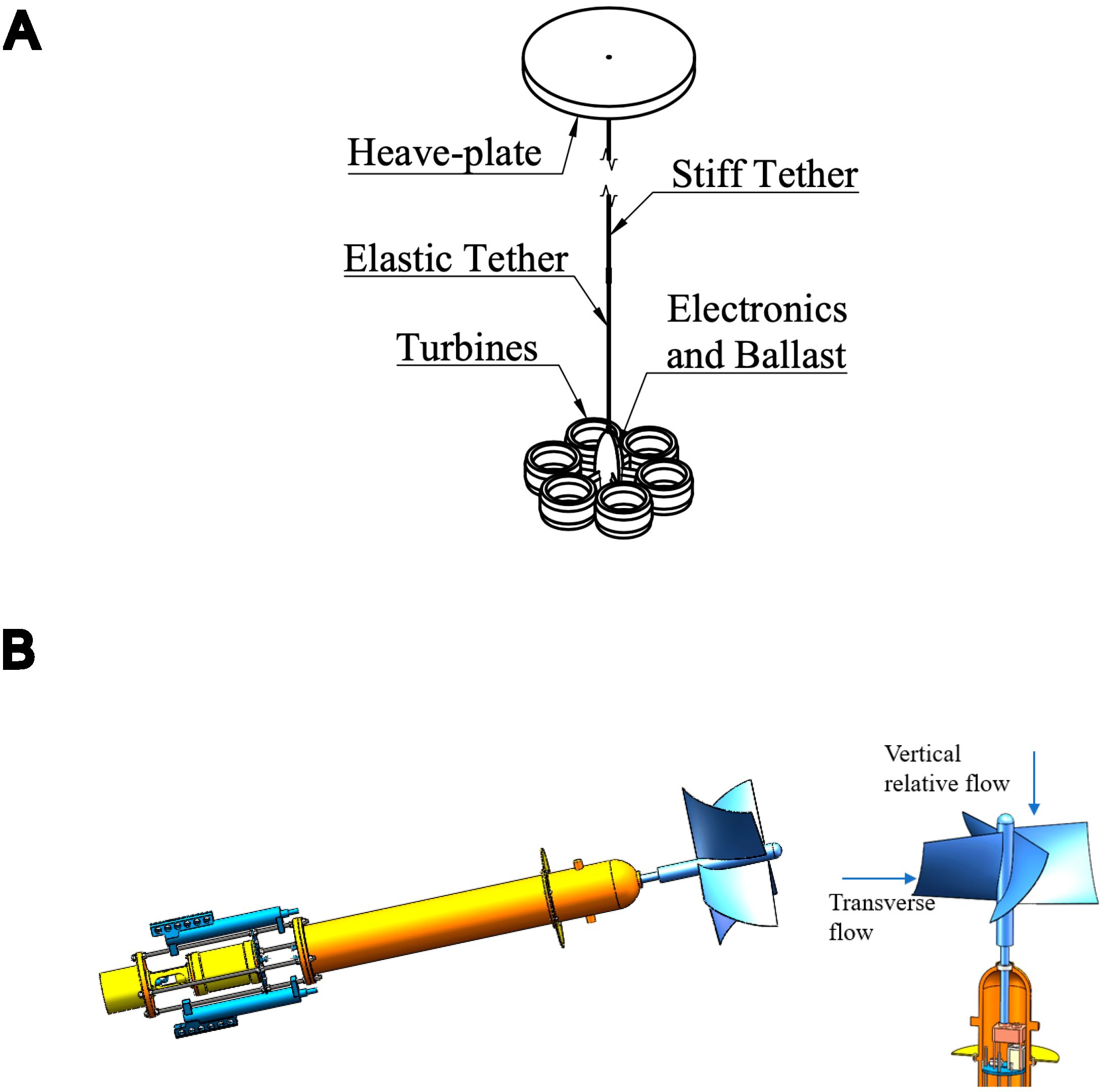
Figure 6. Conceptual designs of environmental energy-powered profiling floats: (A) Near-surface wave energy-powered profiling float (Shomberg et al., 2024); (B) Marine current energy-powered profiling float (Wu et al., 2018).
In addition to harnessing wave energy at the sea surface, a current energy converter was designed for deep-sea profiling floats (Figure 6B). This system employs a spiral involute blade to capture radial current energy in shallow waters and axial relative current energy generated by the float’s autonomous vertical motion (Wu et al., 2018). The turbine’s minimum self-starting flow speed is approximately 0.3 m/s, while stable operation requires speeds greater than 0.6 m/s. Higher flow speeds, smaller load factors, and smaller buoy inclinations could improve performance, but they also amplify load fluctuations and increase the probability of damage (Wu et al., 2018). Therefore, load and speed must be optimized to ensure stable self-starting and sustained operation (Wu et al., 2018).
As a different development direction, multiple patents have described conceptual designs for solar-powered profiling floats (McCoy, 1993; Wang et al., 2006; Ren et al., 2019a). For instance, McCoy proposed a self-powered autonomous profiling float that integrates solar and thermal energy harvesting (McCoy, 1993). In this design, solar cells are installed within the float’s transparent housing to facilitate solar energy collection. Additionally, a thermoelectric device is positioned inside the float, with one side in thermal contact with a thermal mass(e.g., water or ethylene glycol) and the other in thermal contact with the surrounding water medium, creating a temperature gradient that facilitates electricity generation through the Peltier effect (McCoy, 1993). However, to date, there are no existing reports documenting the actual fabrication of solar-powered profiling floats.
In addition to harvesting external energy, the integration of a hydraulic motor into the buoyancy-driven system has been proposed to enable energy recovery for Deep Argo (Xue et al., 2020). It has been demonstrated that this design can recover 11.35% of the energy consumed by the hydraulic pump and reduce its energy consumption by 2.77% (Xue et al., 2020). However, several challenges remain, including mitigating inertia-induced fluctuations in the hydraulic motor’s rotational speed and output torque during energy recovery (Xue et al., 2020). Given the limited amount of energy recoverable by profiling floats in this process, extending its operational lifespan through energy recovery may be less effective than through energy harvesting.
4 Outlook
To address energy supply constraints in autonomous profiling floats, extensive research on low-power technologies has been conducted, focusing on the hydraulic system, sensor system, satellite communication system, and control algorithms to enhance the sensor-carrying capacity and extend service life. For the hydraulic system, which accounts for the highest energy usage, consumption was reduced by 22.5%–51.16% through optimized design and low-power oil discharge strategies (Chen et al., 2017; Wang et al., 2022). To minimize communication duration and reduce satellite system energy consumption, lossless data compression algorithms have been employed, achieving compression rates of 12.11%–26% (Xie, 2020; Guo et al., 2024). Regarding the control system, various low-power control algorithms that balance energy efficiency and motion have been proposed. However, most studies to date have focused primarily on battery-powered profiling floats, employing idealized motion and energy consumption models that often disregard horizontal ocean currents and variations in seawater pressure. Additionally, studies have largely centered on individual systems, modules, or factors, overlooking the complex coupling relationships between different components in the energy consumption process. Consequently, most low-power technologies remain in the preliminary validation phase, with limited practical implementation, highlighting the need for system-level energy optimization and broader application across various profiling floats.
To address the limitations of battery power, Yi Chao’s team developed PCM-based thermal energy harvesting technology, advancing the commercialization of thermal recharging profiling floats. This innovation enables the generation of over 3 Wh of electricity per dive to depths of 1,000 m, enhancing energy supply and operational capability (Seatrec Inc, 2023). Nevertheless, several challenges persist, such as the small temperature gradient in the marine environment and the relatively low energy conversion efficiency. Other types of environmental energy harvesting technologies remain largely in the conceptual design stage when it comes to their application in profiling floats.
The increasing demand for ocean observation intensifies the need for higher sensor-carrying capacity and enhanced energy supply in profiling floats. Therefore, optimizing energy management strategies and integrating emerging energy harvesting technologies are essential to overcoming these challenges.
4.1 Leverage edge AI for advanced intelligent energy management
Edge Artificial Intelligence (Edge AI) enables intelligent management in resource-constrained devices such as profiling floats. Unlike traditional AI, which relies on cloud-based data processing, Edge AI deploys algorithms and models directly onto low-power microcontrollers and edge devices (e.g., Nvidia Jetson Nano), utilizing techniques such as model compression, pruning, and optimization (Gibbs and Kanjo, 2023), thereby ensuring efficient operation on constrained hardware. For more demanding tasks, dedicated AI accelerators can be integrated. For instance, an RNN architecture implemented on a Lattice ICE40UP5K FPGA was shown to consume only 360 µW at a clock frequency of 146 kHz (Bartels et al., 2023). The combination of Edge AI’s computational efficiency and low power consumption makes it particularly suitable for energy management, data processing, and intelligent decision-making in profiling floats, with significant potential for advancing autonomous ocean observation.
In intelligent energy consumption prediction and management, Edge AI enables real-time forecasting of energy usage, dynamically adjusting computing resource allocation and power distribution based on environmental variations and data collection frequency (Nammouchi et al., 2021; Lv et al., 2023). This capability facilitates the optimal scheduling of component operation and energy consumption in profiling floats. For data processing, Edge AI can locally analyze seawater parameters such as temperature, salinity, and dissolved oxygen, enabling real-time anomaly detection and accurate trend identification (Tran et al., 2022; Zhao et al., 2022). Furthermore, in response to the complex and variable marine environment, Edge AI can enhance autonomous decision-making by integrating multi-sensor data to adjust sampling depth, data transmission frequency, and other parameters, allowing profiling floats to operate independently under diverse conditions (Wang et al., 2019c). These capabilities not only enhance system responsiveness and stability but also significantly reduce communication costs and energy consumption, providing a viable approach for the long-term, efficient, and autonomous operation of profiling floats with broad application potential.
4.2 Facilitate research and applications of flexible solar cells and underwater photovoltaic technologies
Traditional solar cells face challenges in profiling float applications due to their large space requirements and susceptibility to corrosion (McLeod and Ringwood, 2022). However, recent advancements in flexible solar cell technology have opened new possibilities for integration into profiling floats. These novel solar cells are lightweight, portable, and flexible while exhibiting good bending cycle stability (Liu et al., 2023a). In addition, an autonomous underwater vehicle (AUV) design incorporating flexible solar cells has been proposed (Byford and Wood, 2019). Power generation performance was estimated, with outputs ranging from 424-1700Wh/m2/day at a depth of 1 ft below the surface, thereby validating the feasibility of this conceptual approach (Byford and Wood, 2019).
However, traditional solar energy harvesting methods remain predominantly constrained to surface or near-surface operations, thereby limiting their efficacy for underwater applications. Indeed, recent studies have demonstrated that solar energy is still accessible underwater, with irradiance values reaching approximately 130 W/m² even at a depth of 20 m (Qian et al., 2024; Lv et al., 2025). Consequently, underwater photovoltaic (PV) technology has garnered increasing attention. Accordingly, extensive research has been conducted on the selection and fabrication of solar cells optimized for underwater light conditions (Enaganti et al., 2020; Enaganti and Goel, 2021; Röhr et al., 2023). It has been demonstrated that wide-bandgap solar cells, particularly gallium indium phosphorus (GaInP) and organic solar cells with bandgaps exceeding 1.5 eV, outperform silicon solar cells (1.1 eV) (Röhr et al., 2023). These cells have exhibited operational efficiencies of 14% or higher even under low-light underwater conditions (Röhr et al., 2020, 2022; Samantaray et al., 2023; Qian et al., 2024). Furthermore, their low cost, high efficiency, and flexibility make them well-suited for underwater environments. However, at present, underwater photovoltaic technology has predominantly garnered attention within the field of photovoltaic materials. Future research will need to further explore its potential for powering underwater marine equipment, with particular emphasis on shallow-water applications.
4.3 Advance wave energy harvesting technology based on triboelectric nanogenerator principles
Wave energy harvesting technology enables the conversion of mechanical energy from seawater vibrations into electrical energy through various mechanisms, including electromagnetic, electrostatic, and piezoelectric methods. Among these, electromagnetic generators (EMGs) are the most widely used, characterized by high power output and operational stability (Xu et al., 2022). However, EMGs generally exhibit low efficiency under mild wave conditions at sea (Xu et al., 2022).
Given that profiling floats are generally characterized by their small size and limited oscillation range, these constraints highlight the need for more compact and efficient systems. Triboelectric Nanogenerators (TENGs), characterized by their potential for miniaturization, simple design, affordability, high power density, and strong adaptability to low-frequency waves (Choi et al., 2023), have emerged as a promising solution for profiling floats. TENGs operate on the principle of the triboelectric effect, where charge transfer occurs between two distinct materials, generating an electric potential that can be harnessed as they make contact and subsequently separate (Shan et al., 2024).
In 2019, a high-output multilayered TENG was integrated into a self-powered intelligent buoy system to harvest wave energy on the surface, successfully providing a steady DC voltage of 2.5 V to the load (Xi et al., 2019). Additionally, a hybridized energy harvesting system integrating TENGs and EMGs was designed and evaluated on a buoy in the Jialing River and an AUV in the Huanghai Sea (Gao et al., 2020). It was found that the device’s resonance frequency was reached exclusively at a water wave frequency of 1 Hz, yielding peak voltages of 11.15 V for the TENG and 3.1 V for the EMG, respectively (Gao et al., 2020). Moreover, TENG units demonstrated the ability to sense angular attitude changes based on variations in output energy (Gao et al., 2020). However, most TENGs remain at the prototype stage and are currently undergoing laboratory examination.
4.4 Explore novel thermal energy harvesting technologies
PCM-based thermal recharging profiling floats have been commercially introduced. However, challenges remain, including low energy conversion efficiency, the poor thermal conductivity of PCM, and its substantial weight (Wang et al., 2020a). In addition, the power generation process requires the coordinated operation of multiple modules, including the hydraulic system, mechanical system, and batteries, which increases system complexity and vulnerability to malfunctions (Wang et al., 2020a). Additionally, the reliance on temperature gradients for phase transitions imposes operational limitations on PCM-based thermal recharging profiling floats, particularly in shallow waters and high-latitude regions.
In response to these limitations, alternative thermal energy harvesting technologies, such as shape memory alloys (SMAs), thermoelectric generators (TEGs), and thermodynamic cycles, have been actively explored. These technologies, along with their potential applications in UUVs, have been extensively reviewed (Wang et al., 2020a; Jung et al., 2022). Key features of these technologies are summarized as follows:
(1) SMA-based thermal energy harvesting technology capitalizes the ability of SMAs to deform at low temperatures and return to their original shape upon heating. This approach involves fewer energy conversion steps, offering higher theoretical reliability (Wang et al., 2020a), which makes it suitable for compact and reliable systems. However, the technology remains at the laboratory or conceptual design stage, with high material costs posing a significant barrier.
(2) TEG-based thermal energy harvesting technology directly converts thermal energy into electrical energy through the Seebeck effect (Tang et al., 2016). Although this method provides smooth and straightforward thermoelectric conversion (Amara-Madi et al., 2013; Lee et al., 2018), its efficiency is limited by the relatively small oceanic temperature gradient, which constrains its applicability to early-stage research and development.
(3) Thermodynamic cycle-based thermal energy harvesting technology theoretically achieves higher efficiencies, with reported values reaching up to 4% (Yoon et al., 2017). While primarily implemented in large-scale thermal power plants (Wang et al., 2015), this technology remains at the theoretical research stage for UUV applications (Yuan et al., 2013). The complexity of the system and high costs continue to hinder its practical implementation (Wang et al., 2019a).
In summary, while these technologies offer promising alternatives, they are still in the conceptual and theoretical stages for UUVs. To date, no studies have explored their application in profiling floats. Consequently, further research and development are required to evaluate their potential in this context.
4.5 Engineer hybrid power generation systems for enhanced energy security and reliability
Given the complexity and variability of the marine environment, power generation technologies based on a single energy source or operating principle often exhibit limited effectiveness under fluctuating sea conditions. For instance, the performance of solar cells is significantly diminished during cloudy weather and at night, while the efficiency of thermal energy conversion is constrained by the magnitude of the temperature gradient. To address these limitations, the integration of multiple energy harvesting technologies(e.g., solar, wave, and thermal energy) can offer a more stable and continuous power supply, independent of transient environmental conditions (Wang et al., 2020a). Furthermore, as the spatial distribution of energy resources varies across different marine regions, with some areas being more suitable for wave energy harvesting while some for thermal energy, a multi-source power generation system can optimize energy harvesting by dynamically selecting the most appropriate energy source based on geographic and environmental characteristics, thereby enhancing overall efficiency.
In 2022, a hybrid nanogenerator (TPEPT-HG) was proposed, integrating TENG, PV, EMG, piezoelectric nanogenerator (PENG), and thermal energy (TG) units (Xue et al., 2022). This system was deployed on an intelligent ocean buoy for sustainable energy generation. Laboratory evaluations demonstrated that at an excitation frequency of 2.4 Hz, the maximum peak-to-peak power outputs of the TENG, PENG, and EMG reached 0.25 mW, 1.58 mW, and 13.8 mW, respectively (Xue et al., 2022). Additionally, the PV unit achieved a maximum open-circuit voltage of 1.33 V and a short-circuit current of 49 mA, while the TG unit produced 5 V and 15 mA (Xue et al., 2022).
Compared to single-source energy conversion systems, hybrid power generation technologies mitigate the temporal and environmental constraints associated with individual energy sources, thereby significantly improving overall energy harvesting efficiency. However, the practical deployment of such systems necessitates addressing key challenges related to energy integration, system coordination, and real-time power management. The development of advanced energy management strategies is crucial for ensuring efficient regulation, seamless switching among different power sources, and the reliable operation of hybrid power generation systems in complex marine environments.
In summary, the energy limitations of autonomous profiling floats are expected to be addressed through advancements in system-level energy consumption optimization, the integration of intelligent technologies, and breakthroughs in solar, thermal, and wave energy harvesting, coupled with the enhanced capabilities of hybrid power generation technologies. These developments will drive ocean observation toward greater efficiency, intelligence, and sustainability.
Author contributions
YY: Visualization, Investigation, Writing – review & editing, Writing – original draft. QY: Visualization, Funding acquisition, Conceptualization, Project administration, Writing – review & editing, Writing – original draft, Supervision. FJ: Project administration, Conceptualization, Writing – original draft, Writing – review & editing. WZ: Supervision, Writing – review & editing, Conceptualization.
Funding
The author(s) declare that financial support was received for the research and/or publication of this article. This research was sponsored by the National Key Research and Development Program (2018YFC1405803), the National Natural Science Foundation of China (40976025), the China National Scientific Seafloor Observatory (2017-000030-73-01-002437), the Ocean Negative Carbon Emissions (ONCE) Program, and the Interdisciplinary Collaborative Research Project at Tongji University (2023-1-ZD-03 and 2023-1-ZD-04).
Acknowledgments
Argo data were collected and made freely available by the International Argo Program and the national programs that contribute to it. (https://argo.ucsd.edu, https://www.ocean-ops.org). The Argo Programme is part of the Global Ocean Observing System. We thank all those who helped write this article and the editors and reviewers of this paper for their constructive feedback.
Conflict of interest
The authors declare that the research was conducted in the absence of any commercial or financial relationships that could be construed as a potential conflict of interest.
Generative AI statement
The author(s) declare that no Generative AI was used in the creation of this manuscript.
Publisher’s note
All claims expressed in this article are solely those of the authors and do not necessarily represent those of their affiliated organizations, or those of the publisher, the editors and the reviewers. Any product that may be evaluated in this article, or claim that may be made by its manufacturer, is not guaranteed or endorsed by the publisher.
Footnotes
- ^ https://argo.ucsd.edu/about/status/.
- ^ https://argo.ucsd.edu/about/.
- ^ https://www.ocean-ops.org/board?t=argo.
- ^ https://www.ocean-ops.org/board?t=argo.
References
Amara-Madi S., Price C. A., Bensaoula A., and Boukadoum M. (2013). “Autonomous sensor system for deep-sea pipeline monitoring,” in 2013 IEEE 11th International New Circuits and Systems Conference (NEWCAS), Paris, France. 1–4 (Piscataway, NJ: IEEE). doi: 10.1109/NEWCAS.2013.6573669
André X., Le Traon P. Y., Le Reste S., Dutreuil V., Leymarie E., Malardé D., et al. (2020). Preparing the new phase of Argo: technological developments on profiling floats in the NAOS project. Front. Mar. Sci. 7. doi: 10.3389/fmars.2020.577446
Argo data management team (2022). Argo user’s manual (Version 3.41.1). Brest, France: Ifremer. doi: 10.13155/29825
Babić A., Oreč M., Mišković N., and Diamant R. (2024). “SOUND: a swarm of low-cost floaters for sustainable fishing,” in Proceedings of the OCEANS 2024 - Singapore, Singapore, Singapore. (Piscataway, NJ: IEEE), 1–5. doi: 10.1109/OCEANS51537.2024.10682243
Barker L. (2014). Closed-loop buoyancy control for a coastal profiling float. Available online at: https://www.mbari.org/wp-content/uploads/2015/10/Barker_Laughlin.pdf (Accessed March 1, 2025).
Bartels J., Hagihara A., Minati L., Tokgoz K. K., and Ito H. (2023). An integer-only resource-minimized RNN on FPGA for low-frequency sensors in edge-AI. IEEE Sens. J. 23, 17784–17793. doi: 10.1109/jsen.2023.3286580
Bittig H. C., Maurer T. L., Plant J. N., Schmechtig C., Wong A. P. S., Claustre H., et al. (2019). A BGC-Argo guide: planning, deployment, data handling and usage. Front. Mar. Sci. 6. doi: 10.3389/fmars.2019.00502
Borase R. P., Maghade D. K., Sondkar S. Y., and Pawar S. N. (2021). A review of PID control, tuning methods and applications. Int. J. Dynam. Control 9, 818–827. doi: 10.1007/s40435-020-00665-4
Brown T. L., Atluri V. P., and Schmiedeler J. P. (2014). A low-cost hybrid drivetrain concept based on compressed air energy storage. Appl. Energy 134, 477–489. doi: 10.1016/j.apenergy.2014.07.111
Buis A. (2010). NASA demonstrates novel ocean-powered underwater vehicle. Available online at: https://www.jpl.nasa.gov/news/nasa-demonstrates-novel-ocean-powered-underwater-vehicle/ (Accessed February 20, 2025).
Byford B. and Wood S. (2019). “Onboard renewable energy charging methods to improve mAUV deployment life,” in Proceedings of the OCEANS 2019 MTS/IEEE SEATTLE, Seattle, WA, USA. 1–10 (Piscataway, NJ: IEEE). doi: 10.23919/OCEANS40490.2019.8962748
Carneiro J. F., Pinto J. B., de Almeida F. G., and Cruz N. A. (2024). Depth control of an underwater sensor platform: comparison between variable buoyancy and propeller actuated devices. Sensors 24, 3050. doi: 10.3390/s24103050
Chao Y. (2016). “Autonomous underwater vehicles and sensors powered by ocean thermal energy,” in Proceedings of the OCEANS 2016 - Shanghai, Shanghai, China. (Piscataway, NJ: IEEE), 1–4. doi: 10.1109/OCEANSAP.2016.7485367
Chen B., Chen Y., He M., Zhang C., Ma X., Xia Q., et al. (2020). “Experimental study of maximum electrical generating for ocean thermal profiler,” in Proceedings of the Global OCEANS Singapore - U.S. Gulf Coast Conference, Biloxi, MS, USA. (Piscataway, NJ: IEEE), 1–5. doi: 10.1109/ieeeconf38699.2020.9389411
Chen L., Cui W., and Pan B. (2017). Study on energy saving for deep-sea profiling float. Ship Build. China 58, 128–135. doi: 10.3969/j.issn.1000-4882.2017.03.013
Chen D., Martinez D., and Taylor T. H. (2022). “Design of a low-cost autonomous epipelagic profiling system for oceanic research,” in Proceedings of the 2022 Systems and Information Engineering Design Symposium (SIEDS), Charlottesville, VA, USA. (Piscataway, NJ: IEEE), 79–84. doi: 10.1109/SIEDS55548.2022.9799420
Chen B., Yang C., Yao Z., Xia Q., and Chen Y. (2024). Research on coupling enhanced heat transfer with energy storage in ocean thermal engine systems. Appl. Energy 360, 122712. doi: 10.1016/j.apenergy.2024.122712
China Satellite Navigation Office (2019). Development of the BeiDou navigation satellite system(Version 4.0). Available online at: http://en.beidou.gov.cn/SYSTEMS/Officialdocument/202001/P020200116329195978690.pdf (Accessed March 1, 2025).
Choi D., Lee Y., Lin Z. H., Cho S., Kim M., Ao C. K., et al. (2023). Recent advances in triboelectric nanogenerators: from technological progress to commercial applications. ACS Nano 17, 11087–11219. doi: 10.1021/acsnano.2c12458
Costa G. K. and Sepehri N. (2023). Hydraulic accumulators in energy efficient circuits. Front. Mech. eng. 9. doi: 10.3389/fmech.2023.1163293
Davis R. E., Sherman J. T., and Dufour J. (2001). Profiling ALACEs and other advances in autonomous subsurface floats. J. Atmos. Oceanic Technol. 18, 982–993. doi: 10.1175/1520-0426(2001)018<0982:PAAOAI>2.0.CO;2
Dologlonyan A. V. and Grekov A. N. (2018). “Improving autonomous operation of profiling floats,” in Proceedings of the 2018 International Russian Automation Conference (RusAutoCon), Sochi, Russia. 1–7 (Piscataway, NJ: IEEE). doi: 10.1109/RUSAUTOCON.2018.8501658
Enaganti P. K., Dwivedi P. K., Sudha R., Srivastava A. K., and Goel S. (2020). Underwater characterization and monitoring of amorphous and monocrystalline solar cells in diverse water settings. IEEE Sens. J. 20, 2730–2737. doi: 10.1109/JSEN.2019.2952428
Enaganti P. K. and Goel S. (2021). Investigation of silicon solar cells under submerged conditions with the influence of various parameters: a comparative study. Energy Technol. 9, 2100018. doi: 10.1002/ente.202100018
Fan L. and Khodadadi J. M. (2011). Thermal conductivity enhancement of phase change materials for thermal energy storage: a review. Renew. Sustain. Energy Rev. 15, 24–46. doi: 10.1016/j.rser.2010.08.007
Gao L., Lu S., Xie W., Chen X., Wu L., Wang T., et al. (2020). A self-powered and self-functional tracking system based on triboelectric-electromagnetic hybridized blue energy harvesting module. Nano Energy 72, 104684. doi: 10.1016/j.nanoen.2020.104684
Gibbs M. and Kanjo E. (2023). “Realising the power of edge intelligence: addressing the challenges in AI and tinyML applications for edge computing,” in Proceedings of the 2023 IEEE International Conference on Edge Computing and Communications (EDGE), Chicago, IL, USA. (Piscataway, NJ: IEEE), 337–343. doi: 10.1109/edge60047.2023.00056
Gordon L. (2017). Recommendations for reports about argo float batteries. Available online at: https://argo.ucsd.edu/wp-content/uploads/sites/361/2020/04/Argo-battery-report.pdf (Accessed March 1, 2025).
Gould W. J. (2005). From Swallow floats to Argo—the development of neutrally buoyant floats. Deep-sea Res. Pt. II 52, 529–543. doi: 10.1016/j.dsr2.2004.12.005
Gruber N., Doney S. C., Emerson S. R., Gilbert D., Kobayashi T., Körtzinger A., et al. (2007). The Argo-oxygen program: a white paper to promote the addition of oxygen sensors to the international Argo float program. Available online at: https://www.ioccg.org/groups/Oxygen_Argo_whitepaper_15feb07_r.pdf (Accessed March 1, 2025).
Guo F. (2020). Research on the hydraulic system and energy consumption of the 4000-meter Argo profiling floats. Shandong University, Jinan(Shandong. doi: 10.27272/d.cnki.gshdu.2020.002206
Guo S., Zhang W., Zheng Y., Li H., Yang Y., and Xu J. (2024). Research on the lossless data compression system of the Argo buoy based on BiLSTM-MHSA-MLP. J. Mar. Sci. Eng. 12, 2298. doi: 10.3390/jmse12122298
Haldeman C. D., Schofield O., Webb D. C., Valdez T. I., and Jones J. A. (2015). “Implementation of energy harvesting system for powering thermal gliders for long duration ocean research,” in Proceedings of the OCEANS 2015 - MTS/IEEE Washington, Washington, DC, USA. (Piscataway, NJ: IEEE), 1–5. doi: 10.23919/OCEANS.2015.7404559
Herbert T. D., Lawrence K. T., Tzanova A., Peterson L. C., Caballero-Gill R., and Kelly C. S. (2016). Late Miocene global cooling and the rise of modern ecosystems. Nat. Geosci. 9, 843–847. doi: 10.1038/ngeo2813
Johnson K., Berelson W., Boss E., Chase Z., Claustre H., Emerson S., et al. (2009). Observing biogeochemical cycles at global scales with profiling floats and gliders: prospects for a global array. Oceanography 22, 216–225. doi: 10.5670/oceanog.2009.81
Johnson K. and Claustre H. (2016). Bringing biogeochemistry into the Argo age. Eos. 97. doi: 10.1029/2016EO062427
Johnson G. C. and Fassbender A. J. (2023). After two decades, Argo at PMEL, looks to the future. Oceanography. 36, 54–59. Available at: https://www.jstor.org/stable/27257880 (Accessed March 1, 2025).
Johnson G. C., Hosoda S., Jayne S. R., Oke P. R., Riser S. C., Roemmich D., et al. (2022). Argo—two decades: global oceanography, revolutionized. Annu. Rev. Mar. Sci. 14, 379–403. doi: 10.1146/annurev-marine-022521-102008
Jung H., Subban C. V., McTigue J. D., Martinez J. J., Copping A. E., Osorio J., et al. (2022). Extracting energy from ocean thermal and salinity gradients to power unmanned underwater vehicles: state of the art, current limitations, and future outlook. Renew. Sustain. Energy Rev. 160, 112283. doi: 10.1016/j.rser.2022.112283
Kim H., Choi J. Y., Park J., Ruda M., Prasad R., Chao Y., et al. (2018). Towards the optimal operation of a thermal-recharging float in the ocean. Ocean Eng. 156, 381–395. doi: 10.1016/j.oceaneng.2018.02.043
King B., Riser S., and Wijffels S. (2017). Report from the 2nd float and CTD technical workshop. Available online at: https://argo.ucsd.edu/wp-content/uploads/sites/361/2022/05/Report_floattechnicalworshop_2017.pdf (Accessed March 2, 2025).
Langebrake L. C., Lembke C. E., Weisberg R. H., Byrne R. H., Russell D. R., Tilbury G., et al. (2002). “Design and initial results of a bottom stationing ocean profiler,” in Proceedings of the OCEANS ‘02 MTS/IEEE, Biloxi, MI, USA. (Piscataway, NJ: IEEE), 98–103. doi: 10.1109/OCEANS.2002.1193254
Lee W. K., Schubert M. J., Ooi B. Y., and Ho S. J. Q. (2018). Multi-source energy harvesting and storage for floating wireless sensor network nodes with long range communication capability. IEEE Trans. Ind. Appl. 54, 2606–2615. doi: 10.1109/TIA.2018.2799158
Le Mézo T., Le Maillot G., Ropert T., Jaulin L., Ponte A., and Zerr B. (2020). Design and control of a low-cost autonomous profiling float. Mech. Ind. 21, 512. doi: 10.1051/meca/2020037
Le Reste S., Dutreuil V., André X., Thierry V., Renaut C., Le Traon P.-Y., et al. (2016). Deep-Arvor”: a new profiling float to extend the Argo observations down to 4000-m depth. J. Atmos. Oceanic Technol. 33, 1039–1055. doi: 10.1175/JTECH-D-15-0214.1
Leymarie E., Penkerc’h C., Vellucci V., Lerebourg C., Antoine D., Boss E., et al. (2018). ProVal: a new autonomous profiling float for high quality radiometric measurements. Front. Mar. Sci. 5. doi: 10.3389/fmars.2018.00437
Li H., Fan Y., Wen Y., Zou Y., Ma Q., and Yang S. (2024). Communication management and data compression algorithm design of BeiDou transparent transmission terminal for Argo buoy. J. Mar. Sci. Eng. 12, 173. doi: 10.3390/jmse12010173
Li R., Zhang Y., Feng Z., Xu J., Wu X., Liu M., et al. (2023). Review of the progress of energy saving of hydraulic control systems. Processes 11, 3304. doi: 10.3390/pr11123304
Liu J. (2020). Design and implementation of the deep-sea profiling float monitoring system based on BeiDou navigation satellite system. Tianjin University, Tianjin. doi: 10.27356/d.cnki.gtjdu.2020.002591
Liu Y., Guo F., Zhai X., Li Z., Zhao H., and Xue G. (2020). Research on the operational stability and energy consumption of the profiling float. J. Coastal Res. 99), 21–30. doi: 10.2112/SI99-004.1
Liu J., Li X., Yang S., Li H., and Xu J. (2019a). Research status and progress of the “FUXING” autonomous profiling float. J. Ocean Technol. 38, 17–23. doi: 10.3969/j.issn.1003-2029.2019.06.003
Liu W., Liu Y., Yang Z., Xu C., Li X., Huang S., et al. (2023a). Flexible solar cells based on foldable silicon wafers with blunted edges. Nature 617, 717–723. doi: 10.1038/s41586-023-05921-z
Liu Y., Qin J., Han J., Xue G., and Huang S. (2023b). Argo buoy with water wheel energy collecting system. CN Patent ZL 202310830471.4 (Beijing: China National Intellectual Property Administration).
Liu Z., Xing X., Chen Z., Lu S., Wu X., Li H., et al. (2023c). Twenty years of ocean observations with China Argo. Acta Oceanologica Sin. 42, 1–16. doi: 10.1007/s13131-022-2076-3
Liu M., Yang S., Li H., Xu J., and Li X. (2019b). Energy consumption analysis and optimization of the deep-sea self-sustaining profile buoy. Energies 12, 2316. doi: 10.3390/en12122316
Lopez I., Andreu J., Ceballos S., Alegria I. M. D., and Kortabarria I. (2013). Review of wave energy technologies and the necessary power-equipment. Renew. Sustain. Energy Rev. 27, 413–434. doi: 10.1016/j.rser.2013.07.009
Lu S., Sun C., Liu Z., and Xu J. (2016). Comparative testing and data quality evaluation for COPEX, HM2000 and APEX profiling buoys. J. Ocean Technol. 35, 84–92. doi: 10.3969/j.issn.1003-2029.2016.01.014
Lv S., Lu M., Liu W., Li X., Lv W., Liu Z., et al. (2025). Recent advances in longitudinal spatial area marine photovoltaics. Renew. Sustain. Energy Rev. 208, 115036. doi: 10.1016/j.rser.2024.115036
Lv W., Yang P., Zheng T., Yi B., Ding Y., Wang Q., et al. (2023). Energy consumption and QoS-aware co-offloading for vehicular edge computing. IEEE Internet Things J. 10, 5214–5225. doi: 10.1109/JIOT.2022.3221966
McCoy K. (1993). Autonomous oceanographic profiler. U.S. Patent No 5283767 (Washington, DC: U.S. Patent and Trademark Office).
McGilvray B. and Roman C. (2010). “Control system performance and efficiency for a mid-depth Lagrangian profiling float,” in Proceedings of the OCEANS’10 IEEE SYDNEY, Sydney, NSW, Australia. (Piscataway, NJ: IEEE), 1–10. doi: 10.1109/OCEANSSYD.2010.5603906
McLeod I. and Ringwood J. V. (2022). Powering data buoys using wave energy: a review of possibilities. J. Ocean Eng. Mar. Energy 8, 417–432. doi: 10.1007/s40722-022-00240-3
Morales-Aragón I. P., Giménez-Gallego J., Gilabert J., Torres-Sánchez R., and Soto-Valles F. (2025). Design and application of depth control methods for autonomous underwater profilers in challenging marine environments. Results Eng. 25, 104031. doi: 10.1016/j.rineng.2025.104031
Morris T., Scanderbeg M., West-Mack D., Gourcuff C., Poffa N., Bhaskar T. V. S. U., et al. (2024). Best practices for core Argo floats - part 1: getting started and data considerations. Front. Mar. Sci. 11. doi: 10.3389/fmars.2024.1358042
Moum J. N., Rudnick D. L., Shroyer E. L., Hughes K. G., Reineman B. D., Grindley K., et al. (2023). Flippin’ xSOLO, an upper-ocean autonomous turbulence-profiling float. J. Atmospheric Oceanic Technol. 40, 629–644. doi: 10.1175/JTECH-D-22-0067.1
Mu W., Zou Z., Liu G., Yang Y., and Shi L. (2019). Depth control method of profiling float based on an improved double PD controller. IEEE Access 7, 43258–43268. doi: 10.1109/ACCESS.2019.2904626
Mu W., Zou Z., Sun H., and Yang Y. (2018). A control strategy with low power consumption for buoyancy regulation system of submersibles. J. Xi’an Jiaotong Univ. 52, 44–49. doi: 10.7652/xjtuxb201812007
Muhammad G., Yao Z., Chen Y., Xia Q., and Yang C. (2024). Experimental investigation on thermal performance of phase change materials - metal foam composites in ocean thermal engine. Case Stud. Therm. Eng. 60, 104693. doi: 10.1016/j.csite.2024.104693
Muthuvel P., Maurya S., and Sudhakar T. (2023). Development of autonomous underwater profiling drifter (AUPD) and field results. J. Earth Syst. Sci. 132, 11. doi: 10.1007/s12040-022-02029-2
Muthuvel P., Sudhakar T., Verma N., Thangavel C., Maurya S., Arumugam P., et al. (2018). “Development of deep sea profiling float: challenges and technology demand,” in Proceedings of the 2018 OCEANS - MTS/IEEE Kobe Techno-Oceans (OTO), Kobe, Japan. (Piscataway, NJ: IEEE), 1–4. doi: 10.1109/OCEANSKOBE.2018.8558832
Nammouchi A., Aupke P., Kassler A., Theocharis A., Raffa V., and Felice M. D. (2021). “Integration of AI, IoT, and edge computing for smart microgrid energy management,” in Proceedings of the 2021 IEEE International Conference on Environment and Electrical Engineering and 2021 IEEE Industrial and Commercial Power Systems Europe (EEEIC/I&CPS Europe ), Bari, Italy. (Piscataway, NJ: IEEE), 1–6. doi: 10.1109/EEEIC/ICPSEurope51590.2021.9584756
National Oceanic and Atmospheric Administration (2024). Argo, the ‘crown jewel’ of ocean observing systems, turns 25. Available online at: https://www.noaa.gov/news/argo-crown-jewel-of-ocean-observing-systems-turns-25 (Accessed March 1, 2025).
Petzrick E., Truman J., and Fargher H. (2013). “Profiling from 6,000 meters with the APEX-Deep float,” in Proceedings of the 2013 OCEANS - San Diego, SanDiego, CA, USA. (Piscataway, NJ: IEEE), 1–3. doi: 10.23919/OCEANS.2013.6741074
Prasad N. V. K., Naidu K. C. B., and Basha D. B. (2024). phase change materials for thermal energy storage: a concise review. Nano 19, 2430010. doi: 10.1142/s179329202430010x
Qian F., Yuan S., Zhang T., Wang L., Li X., Zheng H., et al. (2024). Highly transparent anti-reflection coating enhances the underwater efficiency and stability of perovskite solar modules. Nano Res. 17, 8126–8133. doi: 10.1007/s12274-024-6810-3
Ren C., Zhu X., Tian H., and Wang T. (2019a). Profiling Measurement Buoy Capable of Solar Charging. CN Patent ZL 201821759556.9 (Beijing: China National Intellectual Property Administration).
Ren C., Zhu X., Zhao X., Zhang D., and Chen P. (2019b). An ARGO buoy based on wave energy. CN Patent ZL 201820270140.4 (Beijing: China National Intellectual Property Administration).
Riser S. C., Freeland H. J., Roemmich D., Wijffels S., Troisi A., Belbéoch M., et al. (2016). Fifteen years of ocean observations with the global Argo array. Nat. Clim. Change 6, 145–153. doi: 10.1038/nclimate2872
Riser S. C., Swift D., and Drucker R. (2018). Profiling floats in SOCCOM: technical capabilities for studying the southern ocean. J. Geophys. Res. Oceans 123, 4055–4073. doi: 10.1002/2017JC013419
Roemmich D., Alford M. H., Claustre H., Johnson K., King B., Moum J., et al. (2019a). On the future of Argo: a global, full-depth, multi-disciplinary array. Front. Mar. Sci. 6. doi: 10.3389/fmars.2019.00439
Roemmich D., Johnson G., Riser S., Davis R., Gilson J., Owens W. B., et al. (2009). The Argo program: observing the global oceans with profiling floats. Oceanography 22, 34–43. doi: 10.5670/oceanog.2009.36
Roemmich D., Sherman J. T., Davis R. E., Grindley K., McClune M., Parker C. J., et al. (2019b). Deep SOLO: a full-depth profiling float for the Argo program. J. Atmos. Oceanic Technol. 36, 1967–1981. doi: 10.1175/JTECH-D-19-0066.1
Roemmich D. and The Argo Steering T. (2009). Argo: the challenge of continuing 10 years of progress. Oceanography 22, 46–55. doi: 10.5670/oceanog.2009.65
Röhr J. A., Lipton J., Kong J., Maclean S. A., and Taylor A. D. (2020). Efficiency limits of underwater solar cells. Joule 4, 840–849. doi: 10.1016/j.joule.2020.02.005
Röhr J. A., Sartor B. E., Duenow J. N., Qin Z. L., Meng J., Lipton J., et al. (2022). Identifying optimal photovoltaic technologies for underwater applications. iScience 25, 104531. doi: 10.1016/j.isci.2022.104531
Röhr J. A., Sartor B. E., Lipton J., and Taylor A. D. (2023). A dive into underwater solar cells. Nat. Photonics 17, 747–754. doi: 10.1038/s41566-023-01276-z
Rydberg K. E. (2005). “Hydraulic accumulators as key components in energy efficient mobile systems,” in Proceedings of the Sixth International Conference on Fluid Power Transmission and Control (ICFP’ 2005), Hangzhou, China. (Berlin, Germany: Springer), 12–15.
Samantaray M. R., Rana N. K., Ghosh D. S., and Chander N. (2023). Underwater performance analysis of perovskite solar cells. Phys. Status Solidi Rapid Res. Lett. 17, 2200208. doi: 10.1002/pssr.202200208
Schwithal A. and Roman C. (2009). “Development of a new Lagrangian float for studying coastal marine ecosystems,” in Proceedings of the OCEANS 2009-EUROPE, Bremen, Germany. (Piscataway, NJ: IEEE), 1–6. doi: 10.1109/OCEANSE.2009.5278296
Seatrec Inc (2020). Navis-SL1 data sheet. Available online at: https://seatrec.com/wp-content/uploads/2020/08/SEATREC-DATA-SHEET-NAVIS-SL1-061720.pdf (Accessed March 1, 2025).
Seatrec Inc (2023). InfiniTE brochure. Available online at: https://seatrec.com/wp-content/uploads/2023/05/InfiniTE-Brochure-Web.pdf (Accessed March 1, 2025).
Shan B., Ai T., and Wang K. (2024). Triboelectric nanogenerator for ocean energy harvesting: a review of technological advances and future perspectives. Int. J. Electrochem. Sci. 19, 100694. doi: 10.1016/j.ijoes.2024.100694
Shi Y., Wu T., Cai M., Wang Y., and Xu W. (2016). Energy conversion characteristics of a hydropneumatic transformer in a sustainable-energy vehicle. Appl. Energy 171, 77–85. doi: 10.1016/j.apenergy.2016.03.034
Shomberg R., Jakuba M., and Yoerger D. (2022). “Feasibility of a near-surface wave-powered profiling float for extended fully submerged autonomous deployments,” in Proceedings of the OCEANS 2022, Hampton Roads, Hampton Roads, VA, USA. (Piscataway, NJ: IEEE), 1–10. doi: 10.1109/OCEANS47191.2022.9977078
Shomberg R., Jakuba M., and Yoerger D. (2024). Design proposal and feasibility analysis for a near-surface wave-powered profiling float. J. Mar. Sci. Eng. 12, 137. doi: 10.3390/jmse12010137
Si W., Xue Y., Xue G., Liu Y., and Li Z. (2020). Energy consumption modeling and sensitivity analysis for Deep-Argo Otarriinae profiling float. Math. Probl. Eng. 2020, 1–14. doi: 10.1155/2020/6035369
Sohn N. (2013). Developing parameter estimation techniques for controller optimization of a coastal profiling float. Available online at: https://www.mbari.org/wp-content/uploads/2015/10/Sohn.pdf (Accessed March 1, 2025).
Swift D. D. and Riser S. C. (1994). RAFOS floats: defining and targeting surfaces of neutral buoyancy. J. Atmos. Oceanic Technol. 11, 1079–1092. doi: 10.1175/1520-0426(1994)011<1079:RFDATS>2.0.CO;2
Tang G., Rabeek S., and Arasu M. A. (2016). “Thermal design and temperature gradient analysis for a thermoelectric energy harvest device in off-shore and marine application,” in Proceedings of the 2016 IEEE 18th Electronics Packaging Technology Conference (EPTC), Singapore. (Piscataway, NJ: IEEE), 648–652. doi: 10.1109/EPTC.2016.7861560
Tian Z., Zhou Y., and Liu J. (2014). Modeling and simulating of thermal recharging(TREC) and power unit. J. Sichuan Ord. 35, 31–33. doi: 10.11809/scbgxb2014.01.009
Tran D. H., Nguyen V. L., Nguyen H., and Jang Y. M. (2022). “Short-term solar power generation forecasting using edge AI,” in 2022 13th International Conference on Information and Communication Technology Convergence (ICTC), Jeju Island, Korea. (Piscataway, NJ: IEEE), 341–343. doi: 10.1109/ICTC55196.2022.9952746
Valdez T. I., Jones J. A., Leland R. S., Aintablian H., Stell C. B., Jackson S. P., et al. (2011). “A self-powered underwater robot for ocean exploration and beyond,” in JPL von Karmen Lecture Series (JPL Open Repository, Pasadena, CA). Available at: https://hdl.handle.net/2014/43561 (Accessed March 3, 2025).
Veeraragavan S., Sarojani M., Suresh G., Muthuvel P., Tata S., and Ramadass G. A. (2022). “Optimization of deep-sea profiling float based on ballasting methodology,” in Proceedings of the OCEANS 2022 - Chennai, Chennai, India. (Piscataway, NJ: IEEE), 1–5. doi: 10.1109/OCEANSChennai45887.2022.9775131
Viswanathan V. and Taher T. (2016). “Buoyancy driven autonomous profiling float for shallow waters,” in Proceedings of the OCEANS 2016 MTS/IEEE Monterey, Monterey, CA, USA. (Piscataway, NJ: IEEE), 1–6. doi: 10.1109/OCEANS.2016.7761176
Wang R. (2018). System design and experiment of the thermal exchanger for underwater vehicles. Tianjin University, Tianjin. doi: 10.27356/d.cnki.gtjdu.2018.000449
Wang Q. (2020). Research on key issues of a deep-sea self-sustaining intelligent profile buoy system. Tianjin University, Tianjin. doi: 10.27356/d.cnki.gtjdu.2020.002675
Wang G., Ha D., and Wang K. (2018b). Harvesting environmental thermal energy using solid/liquid phase change materials. J. Intell. Mater. Syst. Struct. 29, 1632–1648. doi: 10.1177/1045389x17742733
Wang G., Ha D., and Wang K. (2019b). A scalable environmental thermal energy harvester based on solid/liquid phase-change materials. Appl. Energy 250, 1468–1480. doi: 10.1016/j.apenergy.2019.05.100
Wang X., Han Y., Wang C., Zhao Q., Chen X., and Chen M. (2019c). In-edge AI: enhancing mobile edge computing, caching, and communication via federated learning. IEEE Network 33, 156–165. doi: 10.1109/MNET.2019.1800286
Wang Q., Qiu Z., Yang S., Li H., and Li X. (2022). Design and experimental research of a novel deep-sea self-sustaining profiling float for observing the northeast off the Luzon Island. Sci. Rep. 12, 18885. doi: 10.1038/s41598-022-23208-7
Wang S., Wang Y., Xie C., Huang W., and Wang X. (2006). Compound energy source driven under-water vertical section buoy. CN Patent ZL 200610013379.5 (Beijing: China National Intellectual Property Administration).
Wang B., Wang G., Zhang W., Liu H., Li M., and Duan Y. (2017a). Design and testing of the heat exchange system for small ocean thermal energy power devices. J. Ocean Technol. 36, 47–52. doi: 10.3969/j.issn.1003-2029.2017.04.009
Wang H., Xu J., Yang X., Miao Z., and Yu C. (2015). Organic Rankine cycle saves energy and reduces gas emissions for cement production. Energy 86, 59–73. doi: 10.1016/j.energy.2015.03.112
Wang G., Yanan Y., Wang S., Zhang H., and Wang Y. (2019a). Efficiency analysis and experimental validation of the ocean thermal energy conversion with phase change material for underwater vehicle. Appl. Energy 248, 475–488. doi: 10.1016/j.apenergy.2019.04.146
Wang G., Yang Y., and Wang S. (2020a). Ocean thermal energy application technologies for unmanned underwater vehicles: a comprehensive review. Appl. Energy 278, 115752. doi: 10.1016/j.apenergy.2020.115752
Wang X., Yang Y., Yin S., and Meng X. (2024). An energy self-sufficient underwater profiling buoy powered by ocean thermal energy. Polish Marit. Res. 31, 43–58. doi: 10.2478/pomr-2024-0049
Wang L., Yao B., Wei Z., and Lian L. (2020b). Study of multi-step buoyancy regulation strategy for profiling float. Ships Sci. Technol. 42, 98–102. doi: 10.3404/j.issn.1672-7649.2020.05.019
Wang B., Zhang W., and Duan Y. (2018a). Analysis and test of power generating characteristics of small ocean thermal energy conversion device. Acta Energ. Sol. Sin. 39, 3302–3310. doi: 10.19912/j.0254-0096.2018.12.002
Wang R., Zhang H., Wang G., and Ma Z. (2017b). “Design of thermal power generation system based on underwater vehicles,” in 10th International Conference on Intelligent Robotics and Applications (ICIRA). Eds. Huang Y., Wu H., Liu H., and Yin Z. (Springer, Wuhan, China), 857–866. doi: 10.1007/978-3-319-65289-4_79
Ward B., Fristedt T., Callaghan A. H., Sutherland G., Sanchez X., Vialard J., et al. (2014). The air-sea interaction profiler (ASIP): an autonomous upwardly rising profiler for microstructure measurements in the upper ocean. J. Atmos. Oceanic Technol. 31, 2246–2267. doi: 10.1175/JTECH-D-14-00010.1
Wen F., Zhou H., Zhao X., Fu J., and Yao B. (2022). Optimal control strategy of energy consumption for the floating motion of deep-sea section buoys. Ship Sci. Technol. 44, 72–76. doi: 10.3404/j.issn.1672-7649.2022.04.015
Wong A. P. S., Wijffels S. E., Riser S. C., Pouliquen S., Hosoda S., Roemmich D., et al. (2020). Argo data 1999-2019: two million temperature-salinity profiles and subsurface velocity observations from a global array of profiling floats. Front. Mar. Sci. 7. doi: 10.3389/fmars.2020.00700
Wu X. (2021). Design and motion simulation of a profile float driven by ocean thermal energy. Harbin Engineering University, Harbin. doi: 10.27060/d.cnki.ghbcu.2021.001696
Wu S., Liu Y., and An Q. (2018). Hydrodynamic analysis of a marine current energy converter for profiling floats. Energies 11, 2218. doi: 10.3390/en11092218
Wu S., Yan T., Kuai Z., and Pan W. (2020). Thermal conductivity enhancement on phase change materials for thermal energy storage: a review. Energy Storage Mater. 25, 251–295. doi: 10.1016/j.ensm.2019.10.010
Xi F., Pang Y., Liu G., Wang S., Li W., Zhang C., et al. (2019). Self-powered intelligent buoy system by water wave energy for sustainable and autonomous wireless sensing and data transmission. Nano Energy 61, 1–9. doi: 10.1016/j.nanoen.2019.04.026
Xia Q., Chen Y., Yang C., Chen B., Muhammad G., and Ma X. (2020). Maximum efficiency point tracking for an ocean thermal energy harvesting system. Int. J. Energy Res. 44, 2693–2703. doi: 10.1002/er.5055
Xia Q., Chen Y., Yang C., Zhang T., and Zang Y. (2018). A new model of phase change process for thermal energy storage. Int. J. Energy Res. 42, 3877–3887. doi: 10.1002/er.4120
Xia Q., Muhammad G., Chen B., Zhang F., Zhang Z., Zhang S., et al. (2021). Investigation of a self-driven profiler with buoyancy adjusting system for ocean thermal energy. Appl. Sci. 11, 7086. doi: 10.3390/app11157086
Xie T. (2020). Design of communication scheme of ocean profiling float based on Beidou short message. Tianjin University, Tianjin. doi: 10.27356/d.cnki.gtjdu.2020.004522
Xu R., Wang H., Xi Z., Wang W., and Xu M. (2022). Recent progress on wave energy marine buoys. J. Mar. Sci. Eng. 10, 566. doi: 10.3390/jmse10050566
Xue F., Chen L., Li C., Ren J., Yu J., Hou X., et al. (2022). A static-dynamic energy harvester for a self-powered ocean environment monitoring application. Sci. China Technol. Sci. 65, 893–902. doi: 10.1007/s11431-021-1974-8
Xue G., Liu Y., Si W., Ji C., Guo F., and Li Z. (2020). Energy recovery and conservation utilizing seawater pressure in the working process of Deep-Argo profiling float. Energy 195, 116845. doi: 10.1016/j.energy.2019.116845
Yang Y., Yang Y., Mu W., and Xing S. (2019). Control strategy of low energy consumption rising and diving motion based on buoyancy actuation system. J. Huazhong Univ. Sci. Technol. (Nat. Sci. Ed.) 47, 57–62. doi: 10.13245/j.hust.190611
Yao Z., Chen Y., Muhammad G., Xia Q., and Yang C. (2024). Comprehensive investigation of a novel multi-segment heat exchanger for PCM/MF based ocean thermal engine: numerical analysis, model reduction and experimental work. Appl. Therm. Eng. 248, 123103. doi: 10.1016/j.applthermaleng.2024.123103
Yasuhara M. and Danovaro R. (2016). Temperature impacts on deep-sea biodiversity. Biol. Rev. 91, 275–287. doi: 10.1111/brv.12169
Yoon J. I., Seol S. H., Son C. H., Jung S. H., Kim Y. B., Lee H. S., et al. (2017). Analysis of the high-efficiency EP-OTEC cycle using R152a. Renew. Energy 105, 366–373. doi: 10.1016/j.renene.2016.12.019
Yuan H., Mei N., Hu S., Wang L., and Yang S. (2013). Experimental investigation on an ammonia-water based ocean thermal energy conversion system. Appl. Therm. Eng. 61, 327–333. doi: 10.1016/j.applthermaleng.2013.07.050
Zhang H., Ma X., and Yang Y. (2022a). An external ocean thermal energy power generation modular device for powering smart float. Energies 15, 3747. doi: 10.3390/en15103747
Zhang Y., Zhou Z., Shi W., and Li S. (2022b). Modeling and performance analysis of electro-mechanical systems for ocean thermal-driven buoys. J. Phys. Conf. Ser. Kunming. 2351, 012037. doi: 10.1088/1742-6596/2351/1/012037
Zhao Y. (2019). Design and optimization of buoyancy drive system of self-supported deep-sea profile buoy. Tianjin: Tianjin University. doi: 10.27356/d.cnki.gtjdu.2019.004522
Zhao D., Li S., Shi W., Zhou Z., and Guo F. (2024). Design and optimization of the teardrop buoy driven by ocean thermal energy. J. Mar. Sci. Eng. 12, 661. doi: 10.3390/jmse12040661
Zhao Q., Pei S., Zou Y., Zhao X., Yang R., Hu C., et al. (2022). “Low energy consumption power inspection solution based on cloud-fog-edge ladder collaboration technology,” in Proceedings of the 2022 2nd International Conference on Electrical Engineering and Control Science (IC2ECS), Nanjing, China. (Piscataway, NJ: IEEE), 615–618. doi: 10.1109/IC2ECS57645.2022.10087986
Zheng D., Xu J., Li X., and Li H. (2021). Low energy consumption depth control method of self-sustaining intelligent buoy. J. Meas. Sci. Instrum. 12, 74–82. doi: 10.3969/j.issn.1674-8042.2021.01.010
Zhi H., Zhou P., Chen Y., Zhao X., Hong Y., Lin M., et al. (2021). Buoyancy regulation strategy for underwater profiler based on adaptive genetic algorithm. J. Mar. Sci. Eng. 9, 53. doi: 10.3390/jmse9010053
Zhou Z. (2023). Research on optimization and control strategy of the buoy driven by ocean thermal energy. Shandong University, Jinan(Shandong. doi: 10.27272/d.cnki.gshdu.2023.001011
Zhu Y., Xu X., Wang J., Yang C., Li Q., and Cai M. (2015). “A hybrid underwater profiler used for persistent monitoring,” in Proceedings of the OCEANS 2015 - MTS/IEEE Washington, Washington, DC. (Piscataway, NJ: IEEE), 1–5. doi: 10.23919/OCEANS.2015.7401913
Keywords: autonomous profiling floats, Argo, low-power strategy, energy harvesting technology, blue energy, in situ oceanic observations
Citation: Yu Y, Yang Q, Ji F and Zhou W (2025) Research advances in energy management and harvesting technologies for autonomous profiling floats. Front. Mar. Sci. 12:1598701. doi: 10.3389/fmars.2025.1598701
Received: 23 March 2025; Accepted: 21 April 2025;
Published: 16 May 2025.
Edited by:
Oscar Schofield, Rutgers, The State University of New Jersey, United StatesReviewed by:
Chengbo Wang, University of Science and Technology of China, ChinaJoseph Gradone, Rutgers University, United States
Copyright © 2025 Yu, Yang, Ji and Zhou. This is an open-access article distributed under the terms of the Creative Commons Attribution License (CC BY). The use, distribution or reproduction in other forums is permitted, provided the original author(s) and the copyright owner(s) are credited and that the original publication in this journal is cited, in accordance with accepted academic practice. No use, distribution or reproduction is permitted which does not comply with these terms.
*Correspondence: Qunhui Yang, eWFuZ3FoQHRvbmdqaS5lZHUuY24=; Fuwu Ji, amlmdXd1QHRvbmdqaS5lZHUuY24=
†These authors have contributed equally to this work