- Bascom Palmer Eye Institute, Miller School of Medicine, University of Miami, Miami, FL, United States
Introduction: The application of artificial intelligence (AI) systems in ophthalmology is rapidly expanding. Early detection and management of keratoconus is important for preventing disease progression and the need for corneal transplant. We review studies regarding the utility of AI in the diagnosis and management of keratoconus and other corneal ectasias.
Methods: We conducted a systematic search for relevant original, English-language research studies in the PubMed, Web of Science, Embase, and Cochrane databases from inception to October 31, 2023, using a combination of the following keywords: artificial intelligence, deep learning, machine learning, keratoconus, and corneal ectasia. Case reports, literature reviews, conference proceedings, and editorials were excluded. We extracted the following data from each eligible study: type of AI, input used for training, output, ground truth or reference, dataset size, availability of algorithm/model, availability of dataset, and major study findings.
Results: Ninety-three original research studies were included in this review, with the date of publication ranging from 1994 to 2023. The majority of studies were regarding the use of AI in detecting keratoconus or subclinical keratoconus (n=61). Among studies regarding keratoconus diagnosis, the most common inputs were corneal topography, Scheimpflug-based corneal tomography, and anterior segment-optical coherence tomography. This review also summarized 16 original research studies regarding AI-based assessment of severity and clinical features, 7 studies regarding the prediction of disease progression, and 6 studies regarding the characterization of treatment response. There were only three studies regarding the use of AI in identifying susceptibility genes involved in the etiology and pathogenesis of keratoconus.
Discussion: Algorithms trained on Scheimpflug-based tomography seem promising tools for the early diagnosis of keratoconus that can be particularly applied in low-resource communities. Future studies could investigate the application of AI models trained on multimodal patient information for staging keratoconus severity and tracking disease progression.
1 Introduction
1.1 Keratoconus
Keratoconus is a progressive corneal ectasia characterized by stromal thinning and corneal steepening, ranging in severity from asymptomatic subclinical disease to severe corneal scarring requiring corneal transplantation for visual rehabilitation (1). As the condition progresses, patients may experience decreased visual acuity, photophobia, and image distortion (1). A meta-analysis study reported that younger patients and patients with a baseline maximum keratometry steeper than 55 D were more likely to experience disease progression (2). Patients with a medical history involving allergic eye disease or atopic conditions were also more likely to experience keratoconus progression (3). The pathophysiology of keratoconus involves reduced keratocyte density, loss of stromal lamellae with fibroblast degradation, redistribution of collagen, and increased proteolysis leading to a breakdown in structural integrity (4). This condition most commonly occurs between the second and third decade of life and has an estimated global prevalence of 1.38 per 1000 individuals (5, 6). The global prevalence of keratoconus varies across populations but has been previously estimated to range between 0.2-4790 per 100,000 individuals, with the lowest reported in Russia (5). The highest prevalence rates have been reported in Asian and Middle Eastern communities (5).
Multiple ancillary testing modalities have been used in conjunction with clinical examination for the diagnosis of keratoconus. Placido-based videokeratoscopy and ultrasonic central pachymetry were previously used in the diagnosis and severity staging of keratoconus (7). Modern Placido disc-based corneal topography devices are still popular, as they provide information about corneal curvature, surface irregularities, and aberrations by generating color-coded maps (1, 8). More recently, Scheimpflug-based corneal tomography imaging has allowed for greater analysis of the cornea by generating three-dimensional representations of the anterior segment to provide information about corneal thickness as well as the anterior and posterior cornea (7, 9). Corneal tomography is now the gold standard method for corneal ectasia diagnosis, and the Pentacam® (OCULUS, Arlington, WA, USA) is one of the most common Scheimpflug-based corneal tomography devices (9, 10). Another adjunct imaging technology used for evaluation of corneal ectasias is anterior-segment optical coherence tomography (AS-OCT), which generates cross-sectional corneal images that can be used to identify asymmetry in corneal thinning, posterior curvature, and epithelial/total corneal thickness via epithelial mapping (1). Corneal biomechanics, including corneal hysteresis and deformation amplitude, can also be used to diagnose early stages of keratoconus through an association with central corneal thickness (11).
Early keratoconus diagnosis is important for successful management and prevention of disease progression (12). Corneal cross-linking (CXL) is a procedure developed in 2003 that promotes bond formation between corneal collagen fibrils using riboflavin and ultraviolet-A light, which has demonstrated excellent long-term efficacy in reducing progression of keratoconus but has a threshold of procedural safety for the thinnest pachymetry value that can be treated (12). As CXL alone does not significantly improve visual outcomes, early detection and disease management can also allow for enhanced visual rehabilitation of patients without requiring keratoplasty, including options of hard contact lenses, intracorneal ring segment implantation, intraocular collamer lens implantation, and CXL combined with laser ablative procedures (e.g. photorefractive keratectomy) (12).
1.2 Models of artificial intelligence
The application of artificial intelligence (AI) in the diagnosis and management of ophthalmic diseases has been rapidly increasing since the 1970s (13). AI associates a particular outcome with variables of different weights, and trained models can be applied in establishing disease diagnosis, determining management, and predicting the prognosis of patients (14). Machine learning (ML) is a subset of AI that uses a training dataset for tasks but does not require programming (15). Features from the input data, which may include imaging and patient demographic information, are used to form a feature vector which then serves as the starting point for the ML model (16). Supervised ML is trained on a set of inputs with correct outcome labels available (17). In contrast, unsupervised ML models receive inputs without outcome labels (18). ML techniques include logistic regression, decision tree, random forest, support vector machine, and multilayer perceptron (Table 1) (15, 16).
Deep learning (DL) is a subset of machine learning that includes convolutional neural networks and does not require manual feature extraction from the research team (15, 29). DL models are composed of neural network layers that represent operations, and the output of one layer serves as the input of the next layer (30). Convolutional neural networks consist of convolution layers, pooling layers, and a fully connected network layer (16). Back propagation, normalization of input, dropout, and residual networks may be used to reduce error, reduce overfitting, and improve training (16).
The application of AI models is evaluated through several measures, which most commonly include accuracy, sensitivity, specificity, and area under the receiver operating curve (AUC or AUROC). The output of AI models in the majority of included studies is a class prediction (i.e. keratoconus versus healthy). Accuracy is the proportion of predictions that are true positives and negatives among all predictions. Sensitivity of a class is the proportion of true positives among all predictions of that class. Specificity describes the rate of true negatives (31). AUC ranges from 0 to 1 and is the area under the curve of the function modeled by sensitivity and 1-specificity. This provides a measure of diagnostic accuracy, with higher scores (closer to 1) representing greater accuracy (32).
1.3 Ophthalmic applications of artificial intelligence
The application of AI models in ophthalmology has been widely studied, particularly for posterior segment diseases including diabetic retinopathy, glaucoma, age-related macular degeneration, and retinopathy of prematurity (ROP) (33, 34). Ng et al. reported that AI algorithms for diabetic retinopathy may be closest to application in clinical medicine (33). DL systems are able to detect diabetic retinopathy, predict disease progression, and predict diabetic macular edema using fundus images (33). There also exist DL algorithms to detect glaucoma and predict progression using fundus photographs, optical coherence tomography, and Humphrey visual fields (33). AI systems are particularly useful for detecting ROP from fundus imaging given that there is grading variation among experts, and some low-resource countries have heavy disease burden with limited access to specialist care (33). DL algorithms have demonstrated high AUC, sensitivity, and specificity for identifying retinopathy of prematurity requiring further management (33).
There has also been an increase in reports evaluating the application of AI systems in detecting anterior segment diseases, including keratoconus, infectious keratitis, cataract, and pterygium using anterior segment photographs and AS-OCT images (35). AI has also been used to screen patients for post-corneal transplant rejection as well as to grade cataracts (35). However, several studies regarding the application of AI in anterior segment disease diagnosis and management were limited by a small sample size with low heterogeneity (35).
As shown, clinical utilization of AI models in ophthalmology has rapidly increased in the past 7 years since the first reports of DL systems trained for screening of retinal pathologies (36, 37). Due to the greater variety of anterior segment imaging and variable use in evaluating different conditions, widespread adoption of ML/DL algorithms for corneal pathology has not yet occurred. However, as imaging-based evaluation of keratoconus has now become standard of care due to improved technology, this study aims to provide a systematic review on the current state of utilizing AI and ML/DL platforms in the diagnosis, evaluation, management, and prognosis of keratoconus and corneal ectasias.
2 Methods of literature search
2.1 Search strategy
Using the Preferred Reporting Items for Systematic reviews and Meta-Analyses (PRISMA) framework as a guide, a systematic review technique was used to evaluate studies describing artificial intelligence and keratoconus. The search strategy was created based on the population, interventions, comparators, outcomes, and study design (PICOS) architecture, resulting in the study question: “Is artificial intelligence a sensitive and specific tool for the diagnosis and management of keratoconus or other corneal ectasias compared to clinical diagnosis and management led by ophthalmologists?” The systematic search was conducted using the PubMed, Web of Science, Embase, and Cochrane databases from inception to October 31, 2023, to select full-length, English articles in peer-reviewed journals. The MeSH keywords included in the search strategy were keratoconus, corneal ectasia, artificial intelligence, machine learning, and deep learning, with all combinations of these terms searched.
2.2 Inclusion and exclusion criteria
Original, English-language research articles published in peer-reviewed journals regarding the use of any AI, ML or DL model in the evaluation of the diagnosis, pathophysiology, severity, clinical progression, or evaluation of the response to management of keratoconus and other corneal ectasias were included in this study. Case reports, editorials, commentaries, conference abstracts, and literature reviews were excluded. Non-English-language articles were also excluded. Publications were not restricted by year. Figure 1 displays PRISMA diagram of the study selection methodology (38).
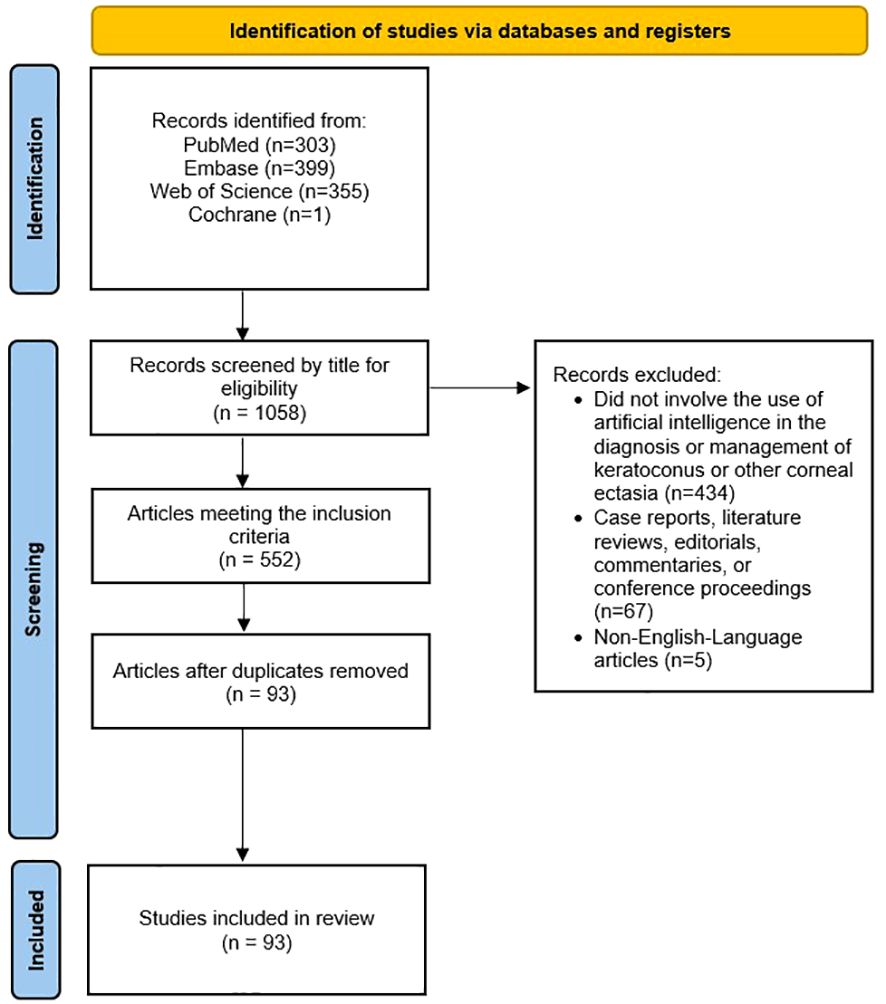
Figure 1 Preferred Reporting Items for Systematic Reviews and Meta-Analyses (PRISMA) diagram of the study selection methodology for this review.
2.3 Data extraction
The following variables were collected for each included study:
● Type of artificial intelligence
● Input used for training
● Output
● Ground truth or reference standard
● Dataset size (number of images, eyes, and patients, when applicable and available)
● Availability of algorithm/model
● Source and availability of dataset
● Major study findings
2.4 Quality assessment
The Quality Assessment of Diagnostic Accuracy Studies-2 (QUADAS-2) tool was used to assess the risk of bias and applicability concerns of studies regarding the diagnosis, severity, and clinical grading of keratoconus and other corneal ectasias (39). The Quality Assessment of Prognostic Accuracy Studies (QUAPAS) tool was used to assess the risk of bias and applicability concerns of studies regarding the prediction of disease progression and response to treatment/management (40). A quality assessment for studies regarding the etiology and pathogenesis was not completed due to the absence of a validated assessment tool for this type of study.
3 Results
The search strategy yielded 93 original research studies. These studies are summarized in Table 2, and we discuss some key studies in the following Results section. There were no concerns regarding the applicability of any studies given the broad nature of our review question and strict application of the inclusion and exclusion criteria.
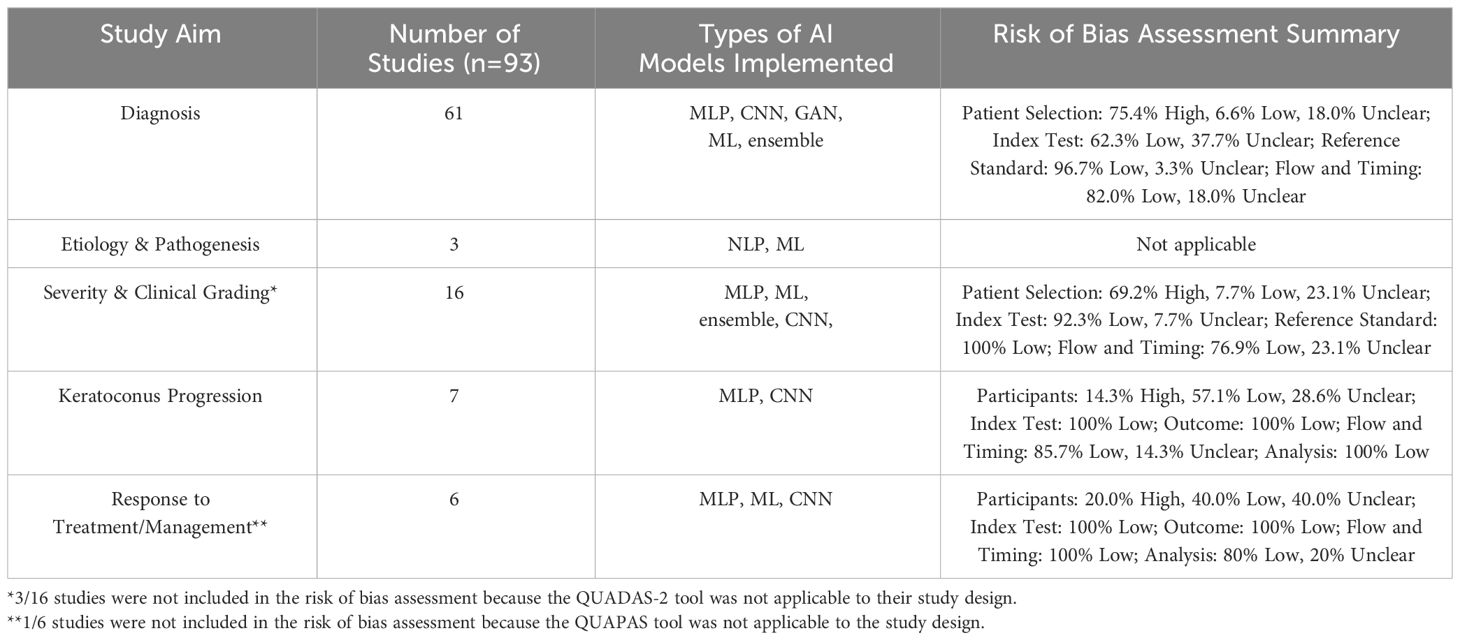
Table 2 Overview of the number of studies, types of AI models implemented for each study aim, and quality assessment of studies included in this review. The types of AI models include multilayer perceptron (MLP), convolutional neural network (CNN), generative adversarial network (GAN), traditional machine learning (ML), ensemble, and natural language processing (NLP).
3.1 Diagnosis of keratoconus
Among the included studies, AI was most frequently used for the diagnosis of keratoconus and other ectasias, particularly in subclinical cases (n = 61, Supplementary Table 1) (31, 41–100). The earliest study using artificial intelligence to detect keratoconus was published in 1994 by Maeda et al. (65) This study used eight indices from TMS-1 videokeratoscope data (Computed Anatomy Inc., New York City, NY, USA) to detect keratoconus among a set of eyes with normal corneas and corneas with various diagnoses (65). The linear discriminant function generated a Keratoconus Prediction Index value that was used to classify eyes as “keratoconus” or “nonkeratoconus” based on a cutoff value (65, 100). The model demonstrated sensitivities of 100% and 89% with three false-positives and one false-positive in the training and validation set, respectively (65). More recently, Silverman et al. evaluated the use of stepwise linear discriminant analysis and neural networks in detecting keratoconus using corneal epithelial and stromal thickness maps from Artemis 1® (StarFish Medical, Victoria, BC, Canada) very high frequency ultrasound arc-scans (96). Eyes with keratoconus were reported to have focal epithelial and stromal thinning with a surrounding ring of epithelial thickening (96, 101).
The majority of the included studies regarding keratoconus or corneal ectasia diagnosis with AI used data from corneal topography (47, 51–53, 56, 57, 67, 73, 74, 77, 78, 83, 91, 92, 94, 100), Scheimpflug-based tomography (43, 45, 49, 54, 55, 59, 60, 62, 64, 66, 70, 76, 79, 80, 84, 86–88, 90, 95, 99), or optical coherence tomography (OCT) (31, 46, 50, 53, 68, 69, 72, 80, 84) as the input. For example, de Almeida et al. utilized 52 parameters from Pentacam® (OCULUS, Arlington, WA, USA) tomography to generate the Corneal Tomography Multivariate Index (CTMVI) using paraconsistent feature engineering and a support vector machine classifier (90). CTMVI was then used to discriminate between very asymmetric ectasia with normal topography and healthy corneas. When using these 52 features, CTMVI demonstrated a sensitivity, specificity, and AUC of 0.844, 0.874, and 0.926, respectively (90). After combining CTMVI and the Pentacam Random Forest Index, the model demonstrated a sensitivity and specificity of 0.99 and 0.84, respectively. Other input for AI-based keratoconus or corneal ectasia diagnosis included data from corneal deformation videos (42, 55, 71, 97), air-puff tonometry (50), corneal endothelial images from specular microscopy (81), and lateral segment photographs (93).
Beyond diagnosing subclinical keratoconus or keratoconus, Ahn et al. demonstrated that AI can be used to screen patients in a primary care setting that may need further evaluation with corneal topography for keratoconus. Using input parameters of subjective visual impairment (based on a patient survey), visual acuity, intraocular pressure, and autokeratometry parameters, they compared the performance of five previously-reported AI models (102–106). The ensemble model with soft voting method demonstrated superior performance with this task with a sensitivity of 90.5% and 96.4% in the internal and external test datasets, respectively (44). Intraocular pressure and mean corneal power were the most highly ranked in the feature importance analysis (44). A soft voting ensemble classifier has been previously used to predict major adverse cardiovascular events among patients with acute coronary system (107).
In the absence of a large dataset of eyes with keratoconus, Lavric et al. and Subramanian et al. used SyntEyes KTC to generate a sufficiently large set of corneal topography images for model training (57, 97). The SyntEyes KTC model was developed by Rozema et al. using Scheimpflug tomography, ocular biometry, and wavefront data of 145 eyes with keratoconus (108). Abdelmotaal et al. used a conditional generative adversarial network (CGAN) called pix2pix to produce images of keratoconus eyes (95). The pix2pix CGAN is composed of a Generator that uses the input image to form the output image, and a Discriminator that determines the similarity of the generated image to an image from the original dataset or an image from the Generator (95). The authors reported that the model produced subjectively and objectively plausible images of keratoconus, early keratoconus, and normal eyes (95). They trained a VGG-16 deep convolutional neural network on combinations of original images and images synthesized by the pix2pix CGAN. The VGG-16 model trained with all original images and synthesized images demonstrated the highest accuracy of 99.56% in discriminating early keratoconus, keratoconus, and normal eyes (95).
3.2 Etiology & pathogenesis of keratoconus
Only three studies have investigated the utility of AI in exploring the genetic etiology and mechanical pathogenesis of keratoconus (Supplementary Table 2) (109–111). Hosoda et al. conducted a genome-wide association study (GWAS) of central corneal thickness using IBM’s Watson for Drug Discovery AI technology. They found the STON2 rs2371597 and SMAD3 rs12913547 loci to be involved in keratoconus development. STON2 and SMAD3 have roles in extracellular matrix (ECM) remodeling, so these variants may contribute to the stromal ECM changes described in keratoconus (109, 112). The authors noted that additional GWAS may be used to identify pathways driving keratoconus development (109). Wang et al. identified 8 differentially expressed genes (AREG, BBC3, DUSP2, MAP3K8, SMAD7, CDKN1A, JUN, and LIF) between patients with and without keratoconus using the random forest model, support vector machine model, and generalized linear model (110). These genes may affect cell mitosis (AREG), macrophage dysfunction (BBC3), cell cycle arrest (CDKN1A), apoptosis (DUSP2, CDKN1A), and proliferation, differentiation, and death (JUN) (110). The authors concluded that abnormal cell proliferation, differentiation, and autophagy pathways may be involved in keratoconus development (110).
Given the reported association between mechanical eye rubbing and pathogenesis of keratoconus, Nokas et al. built a wrist-mounted sensor that used an accelerometer, gyroscope, and machine learning algorithms to detect eye rubbing activity and remind the user to cease such activity (111). With limited AI-based studies on this topic, future research could further investigate susceptibility genes and utility of behavior modification in keratoconus pathogenesis.
3.3 Severity & clinical grading of keratoconus
Sixteen original research articles identified in this review assessed severity or other clinical features of patients with keratoconus (Supplementary Table 3) (104, 113–127). Among studies evaluating keratoconus severity, the ground truth included staging based on topography findings (104, 113, 118, 120, 125, 127), tomography findings (116), the Ectasia Screening Index (114), Keratoconus Severity Index (119), and Amsler-Krumeich criteria (120, 121, 126). Chen et al. compared six convolutional neural network models trained with one or a combination of four color-coded corneal tomography maps (axial, anterior elevation, posterior elevation, and pachymetry) as well as a majority voting strategy model to predict the presence and stage of keratoconus (121). The model trained with all four maps demonstrated the best AUC in distinguishing healthy from keratoconus eyes (121). Interestingly, the majority voting model and the model using the back elevation map demonstrated the highest AUC for discriminating healthy and stage 1 keratoconus eyes, as well as stage 1 and stage 2 keratoconus eyes, respectively (121).
Dong et al. and Dos Santos et al. both used deep learning models to segment corneal OCT scans (122, 123). Dong et al. used a corneal segmentation algorithm to measure the thickness of epithelial and stromal tissue with an error of less than 4 microns (123). The OCT images were taken by the Optovue RTVUE 100 device (Optovue, Inc., Fremont, CA, USA). They reported that as keratoconus progressed, total corneal thickness decreased, particularly temporal and inferior to the pupil center (123). Epithelial thickness also decreased as keratoconus severity increased (123). However, keratoconic eyes with stromal scarring demonstrated a larger epithelial thickness with irregular variations (123). Dos Santos et al. developed CorneaNet, a neural network to segment OCT images of eyes with and without keratoconus and produce thickness maps of the epithelium, Bowman layer, and stroma (122). The image scans were acquired on a custom-built, ultra-high resolution OCT system (128). CorneaNet achieved an accuracy of 99.56% with this task, but the authors noted that accuracy may be limited by network architecture, image noise, as well as insufficient and incorrect training data (122).
3.4 Keratoconus progression
Seven studies investigated AI-based prediction of disease progression in eyes with keratoconus (Supplementary Table 4) (129–135). The majority of these studies only provided binary categorization of keratoconus eyes as progressive or nonprogressive. Kundu et al. developed two AI models, based on either tomographic changes or clinical risk factors, to identify risk factors underlying keratoconus progression and label patients with either likely disease “progression” or “no progression.” (129) They found that elevated serum immunoglobin E (IgE), systemic allergies, eye rubbing, and serum vitamin D level were important characteristics in the evaluation for the risk of keratoconus progression (129). Patients categorized into the progression group had a significantly higher serum IgE compared to those categorized into the no progression group (129). Eye rubbing may also be due to ocular irritation, fatigue, and stress (129). Eye rubbing reduces keratocyte density and modifies intraocular pressure, which can contribute to keratoconus development (129). The results of this study were limited in their generalizability given that the study was based only on an Asian Indian group of patients (129).
Alternatively, Kamiya et al. used a deep learning model to predict keratoconus progression with color-coded maps from AS-OCT (133). They reported that after adjusting for age, the accuracy of their algorithm improved from 0.794 to 0.849 (133). However, this study was limited by the absence of external validation and confirmation of repeatability, small sample size, possibly inaccurate keratoconus diagnosis, and the effect of contact lenses (133).
3.5 Response to treatment/management
Six studies featured the use of AI in characterizing the response to different treatment modalities, such as by predicting postoperative outcomes or the need for future intervention (Supplementary Table 5) (136–141). Both Valdés-Mas et al. and Lyra et al. employed machine learning to predict postoperative refractive outcomes after intracorneal ring implantation, including corneal curvature, astigmatism, asphericity, and keratometry (138, 140). Liu et al. utilized machine learning to predict postoperative visual acuity and keratometry two years after corneal crosslinking (139). While these studies demonstrate the potential of AI models to aid in disease prognostication after different treatments, validation studies are necessary prior to their widespread generalization and adoption.
4 Discussion
Keratoconus is a corneal ectatic disease that can be diagnosed through several imaging modalities, including corneal topography and tomography (1). Furthermore, early detection of keratoconus and other corneal ectasias with prompt management can help slow disease progression and reduce the risk for vision loss or need for a corneal transplant (12). Therefore, AI systems that can process images and generate predictions may be used to reduce the rate of missed or delayed diagnoses, thereby improving patient outcomes. We conducted a systematic review to evaluate the state of AI systems that have been applied in the diagnosis and management of keratoconus and other corneal ectasias among adult patients.
We found that the majority of original research studies involving the clinical application of AI algorithms among patients with keratoconus are based on disease diagnosis. The most current and highest impact studies in the field include Al-Timemy et al., Tan et al., Ambrosio et al., Kuo et al., Kamiya et al., and Chen et al. (42, 52, 72, 82, 88, 121) The majority of these studies used a convolutional neural network AI model, which is a DL method that does not require manual feature extraction from the research team unlike ML algorithms (15, 29). Most of these studies also used Scheimpflug-based corneal tomography scans or indices as their model’s input. This may be explained by the ability of Scheimpflug-based corneal tomography to provide additional information about the posterior cornea, which has been shown to exhibit ectatic changes earlier than the anterior cornea in keratoconus, allowing for earlier diagnosis (142). Additionally, the Belin ABCD classification system (A: anterior radius of curvature, B: back (posterior) radius of curvature, C: minimal corneal thickness, and D: best spectacle distance visual acuity) was developed to monitor disease progression evident on the posterior corneal surface in the absence of anterior corneal surface changes (142). Given that the aforementioned studies demonstrated relatively high diagnostic accuracy of DL algorithms trained on tomography input, further refinement and validation of AI systems evaluating information from Scheimpflug-based tomography may be adopted in clinical practice to streamline the identification of patients with subclinical keratoconus that may benefit from close monitoring for progression to clinical disease. Additionally, a mobile-based application with this technology can be used to develop a screening program for subclinical keratoconus and other corneal ectasia in low-resource countries.
AI models have been less commonly used in other contexts, such as identifying genetic susceptibility and predicting disease progression. Unlike disease diagnosis, these contexts may rely on model input that involves more subjective elements, such as demographic, environmental risk factors, and other clinical factors. For example, Kundu et al. recently developed an AI model using the random forest algorithm to predict progressive keratoconus using clinical and ocular surface risk factors determined from a patient questionnaire (129). Their system demonstrated an AUC of 0.81, and 76% of cases classified as progressive by an AI model trained on tomographic changes were also classified as progressive by the clinical risk factors AI model (129). Within each nondiagnostic context, the AI systems demonstrated relative variability in their performance in completing classification or prediction tasks, and further research is needed to draw conclusions regarding the application of AI models in nondiagnostic contexts for keratoconus. In the future, validated AI models trained on environmental and clinical risk factors could be particularly useful in predicting disease progression to identify patients at risk for severe disease. These identified patients may potentially benefit from prophylactic corneal crosslinking to strengthen corneal integrity and reduce risk of progression.
Some studies included in this review were limited by their design. For example, only one specialist determined the presence of keratoconus for the reference standard of some diagnostic accuracy studies, including Almeida Jr. et al., Lucena et al., Lopes et al., Chandapura et al., Cohen et al., Zéboulon et al., Mosa et al., and Zaki et al. (45, 47, 49, 53, 54, 75, 87, 93) This could have resulted in biased classification of eyes. Additionally, some studies included a relatively low sample size of study groups, which reduced the power of the study. Ahn et al. included only 69, 39, and 43 patients in the subclinical keratoconus study group in the training, internal, and external datasets, respectively (44). Other studies, such as Cohen et al., do not include parameters for demographic information, such as age, sex, or race/ethnicity which can affect the baseline corneal curvature and thickness (54, 143–145).
The strengths of this study lie in the comprehensiveness of clinical contexts included in this systematic review. While previously published reviews regarding the application of AI in keratoconus and other corneal ectasia focus on diagnostic accuracy (146–149), we also included studies regarding grading disease severity, predicting disease progression, understanding etiology and pathogenesis, and predicting response to treatment or management. However, our review has several limitations. To begin with, except for nine studies published prior to 2013, the majority of studies included in our review were published within the last decade. This may be explained by the fact that the application of ML/DL in medicine, including ophthalmology, has grown in popularity more recently (150). Furthermore, we searched a limited number of medical databases, including PubMed, Embase, Web of Science, and Cochrane. Studies meeting our eligibility criteria but not indexed in these databases may have been excluded from this review. While we completed a qualitative data extraction among included studies, we were unable to conduct a meta-analysis with statistical methodology given the contextual and methodological variation between studies and their AI systems. Lastly, some studies included in this review were based on specific patient populations with low diversity, which decreases the generalizability of this review’s findings.
With the incorporation of ML/DL AI algorithms and advancements in corneal imaging technology, the potential ability of clinicians to detect and treat keratoconus at earlier stages to prevent disease progression is promising. AI can also be used to determine ectasia severity, identify susceptibility genes, categorize the keratoconus as progressive or non-progressive, and predict response to surgical management. These applications are particularly important for low-resource nations which may have a scarcity of cornea specialists. In these underserved areas, the incorporation of validated AI models could help reduce the rate of missed or delayed corneal ectasia diagnoses. For example, validated smartphone applications could allow for longitudinal at-home screening of keratoconus among patients with risk factors for the condition. Looking ahead, some clinical applications of AI include using multimodal patient data (a combination of corneal images, demographic information, and environmental risk factors) as the input to determine the keratoconus stage and track disease progression.
Additionally, AI can be used in the surgical planning of some therapeutic options for keratoconus that are growing in popularity, such as ray-tracing-guided transepithelial photorefractive keratectomy with accelerated crosslinking, which may help reduce refractive overcorrection and stromal tissue ablation (151). Mazzotta et al. used a tissue-preservation algorithm involving ray tracing among 38 patients with stable keratoconus undergoing this procedure (151). They reported that the algorithm and surgical treatment significantly improved visual outcomes in their cohort (151). This demonstrates that AI-based algorithms could personalize surgical planning to improve postoperative outcomes, so the implementation of these algorithms for other surgical procedures for visual rehabilitation in keratoconus patients should be investigated.
Data availability statement
The original contributions presented in the study are included in the article/Supplementary Material. Further inquiries can be directed to the corresponding author.
Author contributions
DG: Investigation, Writing – original draft, Writing – review & editing. AYZ: Conceptualization, Methodology, Supervision, Writing – original draft, Writing – review & editing.
Funding
The author(s) declare that no financial support was received for the research, authorship, and/or publication of this article.
Conflict of interest
The authors declare that the research was conducted in the absence of any commercial or financial relationships that could be construed as a potential conflict of interest.
Publisher’s note
All claims expressed in this article are solely those of the authors and do not necessarily represent those of their affiliated organizations, or those of the publisher, the editors and the reviewers. Any product that may be evaluated in this article, or claim that may be made by its manufacturer, is not guaranteed or endorsed by the publisher.
Supplementary material
The Supplementary Material for this article can be found online at: https://www.frontiersin.org/articles/10.3389/fopht.2024.1380701/full#supplementary-material
References
1. Asimellis G, Kaufman EJ. Keratoconus. Treasure Island (FL: StatPearls Publishing Copyright © 2023, StatPearls Publishing LLC (2023).
2. Ferdi AC, Nguyen V, Gore DM, Allan BD, Rozema JJ, Watson SL. Keratoconus natural progression: A systematic review and meta-analysis of 11 529 eyes. Ophthalmology. (2019) 126:935–45. doi: 10.1016/j.ophtha.2019.02.029
3. Antunes-Foschini R, Doná H, de Mello PHSA, Pereira RB, Marqueis IM, Rocha EM, et al. Natural history and predictors for progression in pediatric keratoconus. Sci Rep. (2023) 13:4940. doi: 10.1038/s41598-023-32176-5
4. Mas Tur V, MacGregor C, Jayaswal R, O’Brart D, Maycock N. A review of keratoconus: Diagnosis, pathophysiology, and genetics. Survey Ophthalmol. (2017) 62:770–83. doi: 10.1016/j.survophthal.2017.06.009
5. Santodomingo-Rubido J, Carracedo G, Suzaki A, Villa-Collar C, Vincent SJ, Wolffsohn JS. Keratoconus: An updated review. Contact Lens Anterior Eye. (2022) 45:101559. doi: 10.1016/j.clae.2021.101559
6. Hashemi H, Heydarian S, Hooshmand E, Saatchi M, Yekta A, Aghamirsalim M, et al. The prevalence and risk factors for keratoconus: A systematic review and meta-analysis. Cornea. (2020) 39:263–70. doi: 10.1097/ICO.0000000000002150
7. Belin MW, Ambrósio R. Scheimpflug imaging for keratoconus and ectatic disease. Indian J Ophthalmol. (2013) 61:401–6. doi: 10.4103/0301-4738.116059
8. Cavas-Martínez F, de la Cruz Sánchez E, Nieto Martínez J, Fernández Cañavate FJ, Fernández-Pacheco DG. Corneal topography in keratoconus: state of the art. Eye Vis (Lond). (2016) 3:5. doi: 10.1186/s40662-016-0036-8
9. Hashemi H, Mehravaran S. Day to day clinically relevant corneal elevation, thickness, and curvature parameters using the orbscan II scanning slit topographer and the pentacam scheimpflug imaging device. Middle East Afr J Ophthalmol. (2010) 17:44–55. doi: 10.4103/0974-9233.61216
10. Alghamdi A, Khan MS, Dakhil TA. Understanding corneal epithelial thickness mapping. Middle East Afr J Ophthalmol. (2022) 29:147–55. doi: 10.4103/meajo.meajo_207_22
11. Masiwa LE, Moodley V. A review of corneal imaging methods for the early diagnosis of pre-clinical Keratoconus. J Optom. (2020) 13:269–75. doi: 10.1016/j.optom.2019.11.001
12. Bui AD, Truong A, Pasricha ND, Indaram M. Keratoconus diagnosis and treatment: recent advances and future directions. Clin Ophthalmol. (2023) 17:2705–18. doi: 10.2147/OPTH.S392665
13. Honavar SG. Artificial intelligence in ophthalmology - Machines think! Indian J Ophthalmol. (2022) 70:1075–9. doi: 10.4103/ijo.IJO_644_22
14. Siddiqui AA, Ladas JG, Lee JK. Artificial intelligence in cornea, refractive, and cataract surgery. Curr Opin Ophthalmol. (2020) 31:253–60. doi: 10.1097/ICU.0000000000000673
15. Rampat R, Deshmukh R, Chen X, Ting DSW, Said DG, Dua HS, et al. Artificial intelligence in cornea, refractive surgery, and cataract: basic principles, clinical applications, and future directions. Asia Pac J Ophthalmol (Phila). (2021) 10:268–81. doi: 10.1097/APO.0000000000000394
16. Erickson BJ. Basic artificial intelligence techniques: machine learning and deep learning. Radiol Clin North Am. (2021) 59:933–40. doi: 10.1016/j.rcl.2021.06.004
17. Sidey-Gibbons JAM, Sidey-Gibbons CJ. Machine learning in medicine: a practical introduction. BMC Med Res Methodol. (2019) 19:64. doi: 10.1186/s12874-019-0681-4
18. Singh Pathania Y, Budania A. Artificial intelligence in dermatology: “unsupervised” versus “supervised” machine learning. Int J Dermatol. (2021) 60:e28–e9. doi: 10.1111/ijd.15288
19. Shi S, An X, Li Y. Ultrasound radiomics-based logistic regression model to differentiate between benign and Malignant breast nodules. J Ultrasound Med. (2023) 42:869–79. doi: 10.1002/jum.16078
20. Baştanlar Y, Ozuysal M. Introduction to machine learning. Methods Mol Biol. (2014) 1107:105–28. doi: 10.1007/978-1-62703-748-8_7
21. Venkatasubramaniam A, Wolfson J, Mitchell N, Barnes T, JaKa M, French S. Decision trees in epidemiological research. Emerg Themes Epidemiol. (2017) 14:11. doi: 10.1186/s12982-017-0064-4
22. Sankari ES, Manimegalai D. Predicting membrane protein types using various decision tree classifiers based on various modes of general PseAAC for imbalanced datasets. J Theor Biol. (2017) 435:208–17. doi: 10.1016/j.jtbi.2017.09.018
24. Jin Y, Lan A, Dai Y, Jiang L, Liu S. Development and testing of a random forest-based machine learning model for predicting events among breast cancer patients with a poor response to neoadjuvant chemotherapy. Eur J Med Res. (2023) 28:394. doi: 10.1186/s40001-023-01361-7
25. Zhang S, Li Z, Xin XG. [Support vector machine?assisted diagnosis of human Malignant gastric tissues based on dielectric properties]. Nan Fang Yi Ke Da Xue Xue Bao. (2017) 37:1637–42. doi: 10.3969/j.issn.1673-4254.2017.12.13
26. Abirami S, Chitra P. Chapter Fourteen - Energy-efficient edge based real-time healthcare support system. In: Raj P, Evangeline P, editors. Advances in computers, vol. 117 . Cambridge, Massachusetts: Elsevier (2020). p. 339–68.
27. Choi RY, Coyner AS, Kalpathy-Cramer J, Chiang MF, Campbell JP. Introduction to machine learning, neural networks, and deep learning. Transl Vis Sci Technol. (2020) 9:14. doi: 10.1167/tvst.9.2.14
28. Nistal-Nuño B. Outcome prediction for critical care patients with respiratory neoplasms using a multilayer perceptron neural network. Einstein (Sao Paulo). (2023) 21:eAO0071. doi: 10.31744/einstein_journal/2023AO0071
29. Sarker IH. Deep learning: A comprehensive overview on techniques, taxonomy, applications and research directions. SN Comput Sci. (2021) 2:420. doi: 10.1007/s42979-021-00815-1
30. Heilbroner SP, Miotto R. Deep learning in medicine. Clin J Am Soc Nephrol. (2023) 18:397–9. doi: 10.2215/CJN.0000000000000080
31. Elsawy A, Abdel-Mottaleb M. A novel network with parallel resolution encoders for the diagnosis of corneal diseases. IEEE Trans BioMed Eng. (2021) 68:3671–80. doi: 10.1109/TBME.2021.3082152
32. Zou KH, O’Malley AJ, Mauri L. Receiver-operating characteristic analysis for evaluating diagnostic tests and predictive models. Circulation. (2007) 115:654–7. doi: 10.1161/CIRCULATIONAHA.105.594929
33. Ng Wei Y, Zhang S, Wang Z, Ong Charles Jit T, Gunasekeran Dinesh V, Lim Gilbert Yong S, et al. Updates in deep learning research in ophthalmology. Clin Sci. (2021) 135:2357–76. doi: 10.1042/CS20210207
34. Ting DSW, Pasquale LR, Peng L, Campbell JP, Lee AY, Raman R, et al. Artificial intelligence and deep learning in ophthalmology. Br J Ophthalmol. (2019) 103:167–75. doi: 10.1136/bjophthalmol-2018-313173
35. Ting DSJ, Foo VH, Yang LWY, Sia JT, Ang M, Lin H, et al. Artificial intelligence for anterior segment diseases: Emerging applications in ophthalmology. Br J Ophthalmol. (2021) 105:158–68. doi: 10.1136/bjophthalmol-2019-315651
36. Gulshan V, Peng L, Coram M, Stumpe MC, Wu D, Narayanaswamy A, et al. Development and validation of a deep learning algorithm for detection of diabetic retinopathy in retinal fundus photographs. Jama. (2016) 316:2402–10. doi: 10.1001/jama.2016.17216
37. Ting DSW, Cheung CY, Lim G, Tan GSW, Quang ND, Gan A, et al. Development and validation of a deep learning system for diabetic retinopathy and related eye diseases using retinal images from multiethnic populations with diabetes. Jama. (2017) 318:2211–23. doi: 10.1001/jama.2017.18152
38. Page MJ, Moher D, Bossuyt PM, Boutron I, Hoffmann TC, Mulrow CD, et al. PRISMA 2020 explanation and elaboration: updated guidance and exemplars for reporting systematic reviews. BMJ. (2021) 372:n160. doi: 10.1136/bmj.n160
39. Whiting PF, Rutjes AW, Westwood ME, Mallett S, Deeks JJ, Reitsma JB, et al. QUADAS-2: a revised tool for the quality assessment of diagnostic accuracy studies. Ann Intern Med. (2011) 155:529–36. doi: 10.7326/0003-4819-155-8-201110180-00009
40. Lee J, Mulder F, Leeflang M, Wolff R, Whiting P, Bossuyt PM. QUAPAS: an adaptation of the QUADAS-2 tool to assess prognostic accuracy studies. Ann Intern Med. (2022) 175:1010–8. doi: 10.7326/M22-0276
41. Mohammadpour M, Heidari Z, Hashemi H, Yaseri M, Fotouhi A. Comparison of artificial intelligence-based machine learning classifiers for early detection of keratoconus. Eur J Ophthalmol. (2022) 32:1352–60. doi: 10.1177/11206721211073442
42. Tan Z, Chen X, Li K, Liu Y, Cao H, Li J, et al. Artificial intelligence-based diagnostic model for detecting keratoconus using videos of corneal force deformation. Transl Vis Sci Technol. (2022) 11:32. doi: 10.1167/tvst.11.9.32
43. Xu Z, Feng R, Jin X, Hu H, Ni S, Xu W, et al. Evaluation of artificial intelligence models for the detection of asymmetric keratoconus eyes using Scheimpflug tomography. Clin Exp Ophthalmol. (2022) 50:714–23. doi: 10.1111/ceo.14126
44. Ahn H, Kim NE, Chung JL, Kim YJ, Jun I, Kim TI, et al. Patient selection for corneal topographic evaluation of keratoconus: A screening approach using artificial intelligence. Front Med (Lausanne). (2022) 9:934865. doi: 10.3389/fmed.2022.934865
45. Almeida GC Jr., Guido RC, Balarin Silva HM, Brandão CC, de Mattos LC, Lopes BT, et al. New artificial intelligence index based on Scheimpflug corneal tomography to distinguish subclinical keratoconus from healthy corneas. J Cataract Refract Surg. (2022) 48:1168–74. doi: 10.1097/j.jcrs.0000000000000946
46. Kundu G, Shetty R, Khamar P, Mullick R, Gupta S, Nuijts R, et al. Universal architecture of corneal segmental tomography biomarkers for artificial intelligence-driven diagnosis of early keratoconus. Br J Ophthalmol. (2023) 107:635–43. doi: 10.1136/bjophthalmol-2021-319309
47. Lucena AR, Araújo MO, Carneiro RFL, Cavalcante TDS, Ribeiro ABN, Anselmo FJM. Development of an application for providing corneal topography reports based on artificial intelligence. Arq Bras Oftalmol. (2021) 85:351–8. doi: 10.5935/0004-2749.20220051
48. Issarti I, Consejo A, Jiménez-García M, Hershko S, Koppen C, Rozema JJ. Computer aided diagnosis for suspect keratoconus detection. Comput Biol Med. (2019) 109:33–42. doi: 10.1016/j.compbiomed.2019.04.024
49. Lopes BT, Ramos IC, Salomão MQ, Guerra FP, Schallhorn SC, Schallhorn JM, et al. Enhanced tomographic assessment to detect corneal ectasia based on artificial intelligence. Am J Ophthalmol. (2018) 195:223–32. doi: 10.1016/j.ajo.2018.08.005
50. Lu NJ, Elsheikh A, Rozema JJ, Hafezi N, Aslanides IM, Hillen M, et al. Combining spectral-domain OCT and air-puff tonometry analysis to diagnose keratoconus. J Refract Surg. (2022) 38:374–80. doi: 10.3928/1081597X-20220414-02
51. Lavric A, Valentin P. KeratoDetect: keratoconus detection algorithm using convolutional neural networks. Comput Intell Neurosci. (2019) 2019:8162567. doi: 10.1109/ECAI46879.2019
52. Kuo BI, Chang WY, Liao TS, Liu FY, Liu HY, Chu HS, et al. Keratoconus screening based on deep learning approach of corneal topography. Transl Vis Sci Technol. (2020) 9:53. doi: 10.1167/tvst.9.2.53
53. Chandapura R, Salomao MQ, Ambrosio R Jr., Swarup R, Shetty R, Sinha Roy A. Bowman’s topography for improved detection of early ectasia. J Biophotonics. (2019) 12:e201900126. doi: 10.1002/jbio.201900126
54. Cohen E, Bank D, Sorkin N, Giryes R, Varssano D. Use of machine learning to achieve keratoconus detection skills of a corneal expert. Int Ophthalmol. (2022) 42:3837–47. doi: 10.1007/s10792-022-02404-4
55. Ambrósio R Jr., Lopes BT, Faria-Correia F, Salomão MQ, Bühren J, Roberts CJ, et al. Integration of scheimpflug-based corneal tomography and biomechanical assessments for enhancing ectasia detection. J Refract Surg. (2017) 33:434–43. doi: 10.3928/1081597X-20170426-02
56. Gao HB, Pan ZG, Shen MX, Lu F, Li H, Zhang XQ. KeratoScreen: early keratoconus classification with zernike polynomial using deep learning. Cornea. (2022) 41:1158–65. doi: 10.1097/ICO.0000000000003038
57. Subramanian P, Ramesh GP. Keratoconus classification with convolutional neural networks using segmentation and index quantification of eye topography images by particle swarm optimisation. BioMed Res Int. (2022) 2022:8119685. doi: 10.1155/2022/8119685
58. Lu NJ, Koppen C, Hafezi F, Ni Dhubhghaill S, Aslanides IM, Wang QM, et al. Combinations of Scheimpflug tomography, ocular coherence tomography and air-puff tonometry improve the detection of keratoconus. Cont Lens Anterior Eye. (2023) 46:101840. doi: 10.1016/j.clae.2023.101840
59. Cao K, Verspoor K, Sahebjada S, Baird PN. Evaluating the performance of various machine learning algorithms to detect subclinical keratoconus. Transl Vis Sci Technol. (2020) 9:24. doi: 10.1167/tvst.9.2.24
60. Smadja D, Touboul D, Cohen A, Doveh E, Santhiago MR, Mello GR, et al. Detection of subclinical keratoconus using an automated decision tree classification. Am J Ophthalmol. (2013) 156:237–46 e1. doi: 10.1016/j.ajo.2013.03.034
61. Karimi A, Meimani N, Razaghi R, Rahmati SM, Jadidi K, Rostami M. Biomechanics of the healthy and keratoconic corneas: A combination of the clinical data, finite element analysis, and artificial neural network. Curr Pharm Des. (2018) 24:4474–83. doi: 10.2174/1381612825666181224123939
62. Ruiz Hidalgo I, Rozema JJ, Saad A, Gatinel D, Rodriguez P, Zakaria N, et al. Validation of an objective keratoconus detection system implemented in a scheimpflug tomographer and comparison with other methods. Cornea. (2017) 36:689–95. doi: 10.1097/ICO.0000000000001194
63. Maeda N, Klyce SD, Smolek MK. Comparison of methods for detecting keratoconus using videokeratography. Arch Ophthalmol. (1995) 113:870–4. doi: 10.1001/archopht.1995.01100070044023
64. Cao K, Verspoor K, Chan E, Daniell M, Sahebjada S, Baird PN. Machine learning with a reduced dimensionality representation of comprehensive Pentacam tomography parameters to identify subclinical keratoconus. Comput Biol Med. (2021) 138:104884. doi: 10.1016/j.compbiomed.2021.104884
65. Maeda N, Klyce SD, Smolek MK, Thompson HW. Automated keratoconus screening with corneal topography analysis. Invest Ophthalmol Vis Sci. (1994) 35:2749–57.
66. Feng R, Xu Z, Zheng X, Hu H, Jin X, Chen DZ, et al. KerNet: A novel deep learning approach for keratoconus and sub-clinical keratoconus detection based on raw data of the pentacam HR system. IEEE J BioMed Health Inform. (2021) 25:3898–910. doi: 10.1109/JBHI.2021.3079430
67. Al-Timemy AH, Mosa ZM, Alyasseri Z, Lavric A, Lui MM, Hazarbassanov RM, et al. A hybrid deep learning construct for detecting keratoconus from corneal maps. Transl Vis Sci Technol. (2021) 10:16. doi: 10.1167/tvst.10.14.16
68. Elsawy A, Eleiwa T, Chase C, Ozcan E, Tolba M, Feuer W, et al. Multidisease deep learning neural network for the diagnosis of corneal diseases. Am J Ophthalmol. (2021) 226:252–61. doi: 10.1016/j.ajo.2021.01.018
69. Fassbind B, Langenbucher A, Streich A. Automated cornea diagnosis using deep convolutional neural networks based on cornea topography maps. Sci Rep. (2023) 13:6566. doi: 10.1038/s41598-023-33793-w
70. Kovacs I, Mihaltz K, Kranitz K, Juhasz E, Takacs A, Dienes L, et al. Accuracy of machine learning classifiers using bilateral data from a Scheimpflug camera for identifying eyes with preclinical signs of keratoconus. J Cataract Refract Surg. (2016) 42:275–83. doi: 10.1016/j.jcrs.2015.09.020
71. Leao E, Ing Ren T, Lyra JM, MaChado A, Koprowski R, Lopes B, et al. Corneal deformation amplitude analysis for keratoconus detection through compensation for intraocular pressure and integration with horizontal thickness profile. Comput Biol Med. (2019) 109:263–71. doi: 10.1016/j.compbiomed.2019.04.019
72. Kamiya K, Ayatsuka Y, Kato Y, Fujimura F, Takahashi M, Shoji N, et al. Keratoconus detection using deep learning of colour-coded maps with anterior segment optical coherence tomography: a diagnostic accuracy study. BMJ Open. (2019) 9:e031313. doi: 10.1136/bmjopen-2019-031313
73. Maeda N, Klyce SD, Smolek MK. Neural network classification of corneal topography. Preliminary demonstration. Invest Ophthalmol Vis Sci. (1995) 36:1327–35.
74. Prakash G, Perera C, Jhanji V. Comparison of machine learning-based algorithms using corneal asymmetry vs. single-metric parameters for keratoconus detection. Graefes Arch Clin Exp Ophthalmol. (2023) 261:2335–42. doi: 10.1007/s00417-023-06049-6
75. Zeboulon P, Debellemaniere G, Bouvet M, Gatinel D. Corneal topography raw data classification using a convolutional neural network. Am J Ophthalmol. (2020) 219:33–9. doi: 10.1016/j.ajo.2020.06.005
76. Ruiz Hidalgo I, Rodriguez P, Rozema JJ, ND S, Zakaria N, Tassignon MJ, et al. Evaluation of a machine-learning classifier for keratoconus detection based on scheimpflug tomography. Cornea. (2016) 35:827–32. doi: 10.1097/ICO.0000000000000834
77. Souza MB, Medeiros FW, Souza DB, Garcia R, Alves MR. Evaluation of machine learning classifiers in keratoconus detection from orbscan II examinations. Clinics (Sao Paulo). (2010) 65:1223–8. doi: 10.1590/S1807-59322010001200002
78. Accardo PA, Pensiero S. Neural network-based system for early keratoconus detection from corneal topography. J BioMed Inform. (2002) 35:151–9. doi: 10.1016/S1532-0464(02)00513-0
79. Castro-Luna G, Jimenez-Rodriguez D, Castano-Fernandez AB, Perez-Rueda A. Diagnosis of subclinical keratoconus based on machine learning techniques. J Clin Med. (2021) 10:4281. doi: 10.3390/jcm10184281
80. Shi C, Wang M, Zhu T, Zhang Y, Ye Y, Jiang J, et al. Machine learning helps improve diagnostic ability of subclinical keratoconus using Scheimpflug and OCT imaging modalities. Eye Vis (Lond). (2020) 7:48. doi: 10.1186/s40662-020-00213-3
81. Wan Q, Wei R, Ma K, Yin H, Deng YP, Tang J. Deep learning-based automatic diagnosis of keratoconus with corneal endothelium image. Ophthalmol Ther. (2023) 12:3047–65. doi: 10.1007/s40123-023-00795-w
82. Al-Timemy AH, Alzubaidi L, Mosa ZM, Abdelmotaal H, Ghaeb NH, Lavric A, et al. A deep feature fusion of improved suspected keratoconus detection with deep learning. Diagnostics (Basel). (2023) 13:1689. doi: 10.3390/diagnostics13101689
83. Castro-Luna GM, Martinez-Finkelshtein A, Ramos-Lopez D. Robust keratoconus detection with Bayesian network classifier for Placido-based corneal indices. Cont Lens Anterior Eye. (2020) 43:366–72. doi: 10.1016/j.clae.2019.12.006
84. Abdelmotaal H, Mostafa MM, Mostafa ANR, Mohamed AA, Abdelazeem K. Classification of color-coded scheimpflug camera corneal tomography images using deep learning. Transl Vis Sci Technol. (2020) 9:30. doi: 10.1167/tvst.9.13.30
85. Twa MD, Parthasarathy S, Roberts C, Mahmoud AM, Raasch TW, Bullimore MA. Automated decision tree classification of corneal shape. Optom Vis Sci. (2005) 82:1038–46. doi: 10.1097/01.opx.0000192350.01045.6f
86. Fırat M, Çankaya C, Çınar A, Tuncer T. Automatic detection of keratoconus on Pentacam images using feature selection based on deep learning. Int J Imaging Syst Technol. (2022) 32:1548–60. doi: 10.1002/ima.22717
87. Mosa ZM, Ghaeb NH, Ali AH. Detecting keratoconus by using SVM and decision tree classifiers with the aid of image processing. Baghdad Sci J. (2019) 16:1022–9. doi: 10.21123/bsj.2019.16.4(Suppl.)
88. Ambrosio R Jr., MaChado AP, Leao E, Lyra JMG, Salomao MQ, Esporcatte LGP, et al. Optimized artificial intelligence for enhanced ectasia detection using scheimpflug-based corneal tomography and biomechanical data. Am J Ophthalmol. (2023) 251:126–42. doi: 10.1016/j.ajo.2022.12.016
89. Lavric A, Popa V, Takahashi H, Yousefi S. Detecting keratoconus from corneal imaging data using machine learning. IEEE Access. (2020) 8:149113–21. doi: 10.1109/Access.6287639
90. de Almeida GC Jr., Guido RC, Neto JS, Rosa JM, Castiglioni L, de Mattos LC, et al. Corneal Tomography Multivariate Index (CTMVI) effectively distinguishes healthy corneas from those susceptible to ectasia. Biomed Signal Process Control. (2021) 70. doi: 10.1016/j.bspc.2021.102995
91. Zeboulon P, Debellemaniere G, Gatinel D. Unsupervised learning for large-scale corneal topography clustering. Sci Rep. (2020) 10:16973. doi: 10.1038/s41598-020-73902-7
92. Al-Timemy AH, Ghaeb NH, Mosa ZM, Escudero J. Deep transfer learning for improved detection of keratoconus using corneal topographic maps. Cogn Computation. (2021) 14:1627–42. doi: 10.1007/s12559-021-09880-3
93. Zaki WMD W, Mat Daud M, Saad AH, Hussain A. Abdul mutalib H. A mobile solution for lateral segment photographed images based deep keratoconus screening method. Int J Integrated Eng. (2021) 13:18–27. doi: 10.30880/ijie.2021.013.05.003
94. Alshammari G, Hamad AA, Abdullah ZM, Alshareef AM, Alhebaishi N, Alshammari A, et al. Applications of deep learning on topographic images to improve the diagnosis for dynamic systems and unconstrained optimization. Wireless Commun Mobile Computing. (2021) 2021:1–7. doi: 10.1155/2021/4672688
95. Abdelmotaal H, Abdou AA, Omar AF, El-Sebaity DM, Abdelazeem K. Pix2pix conditional generative adversarial networks for scheimpflug camera color-coded corneal tomography image generation. Transl Vis Sci Technol. (2021) 10:21. doi: 10.1167/tvst.10.7.21
96. Silverman RH, Urs R, Roychoudhury A, Archer TJ, Gobbe M, Reinstein DZ. Epithelial remodeling as basis for machine-based identification of keratoconus. Invest Ophthalmol Vis Sci. (2014) 55:1580–7. doi: 10.1167/iovs.13-12578
97. Abdelmotaal H, Hazarbassanov RM, Salouti R, Nowroozzadeh MH, Taneri S, Al-Timemy AH, et al. Keratoconus detection-based on dynamic corneal deformation videos using deep learning. Ophthalmol Sci. (2024) 4:100380. doi: 10.1016/j.xops.2023.100380
98. Francis M, Shetty R, Padmanabhan P, Vinciguerra R, Vinciguerra P, Lippera M, et al. New simulation software to predict postoperative corneal stiffness before laser vision correction. J Cataract Refract Surg. (2023) 49:620–7. doi: 10.1097/j.jcrs.0000000000001169
99. Velazquez-Blazquez JS, Bolarin JM, Cavas-Martinez F, Alio JL. EMKLAS: A new automatic scoring system for early and mild keratoconus detection. Transl Vis Sci Technol. (2020) 9:30. doi: 10.1167/tvst.9.2.30
100. Santhiago MR, Araujo DC, Stival LR, Smadja D, Veloso AA. Ectasia risk model: A novel method without cut-off point based on artificial intelligence improves detection of higher-risk eyes. J Refract Surg. (2022) 38:716–24. doi: 10.3928/1081597X-20221018-01
101. Reinstein DZ, Archer TJ, Gobbe M. Corneal epithelial thickness profile in the diagnosis of keratoconus. J Refract Surg. (2009) 25:604–10. doi: 10.3928/1081597X-20090610-06
102. Li Y, Li M, Li C, Liu Z. Forest aboveground biomass estimation using Landsat 8 and Sentinel-1A data with machine learning algorithms. Sci Rep. (2020) 10:9952. doi: 10.1038/s41598-020-67024-3
103. Arik SÖ, Pfister T. TabNet: attentive interpretable tabular learning. ArXiv. (2019). doi: 10.48550/arXiv.1908.07442
104. Ghaderi M, Sharifi A, Jafarzadeh Pour E. Proposing an ensemble learning model based on neural network and fuzzy system for keratoconus diagnosis based on Pentacam measurements. Int Ophthalmol. (2021) 41:3935–48. doi: 10.1007/s10792-021-01963-2
105. Saikia MJ, Kuanar S, Mahapatra D, Faghani S. Multi-modal ensemble deep learning in head and neck cancer HPV sub-typing. Bioengineering (Basel). (2023) 11:13. doi: 10.3390/bioengineering11010013
106. Xie X, Xie M, Moshayedi AJ, Noori Skandari MH. A hybrid improved neural networks algorithm based on L2 and dropout regularization. Math Problems Engineering. (2022) 2022:8220453. doi: 10.1155/2022/8220453
107. Sherazi SWA, Bae J-W, Lee JY. A soft voting ensemble classifier for early prediction and diagnosis of occurrences of major adverse cardiovascular events for STEMI and NSTEMI during 2-year follow-up in patients with acute coronary syndrome. PloS One. (2021) 16:e0249338. doi: 10.1371/journal.pone.0249338
108. Rozema JJ, Rodriguez P, Ruiz Hidalgo I, Navarro R, Tassignon MJ, Koppen C. SyntEyes KTC: higher order statistical eye model for developing keratoconus. Ophthalmic Physiol Opt. (2017) 37:358–65. doi: 10.1111/opo.12369
109. Hosoda Y, Miyake M, Meguro A, Tabara Y, Iwai S, Ueda-Arakawa N, et al. Keratoconus-susceptibility gene identification by corneal thickness genome-wide association study and artificial intelligence IBM Watson. Commun Biol. (2020) 3:410. doi: 10.1038/s42003-020-01137-3
110. Wang L, Wang Y, Liu J, Zhao W. Identification of important genes of keratoconus and construction of the diagnostic model. Genet Res (Camb). (2022) 2022:5878460. doi: 10.1155/2022/5878460
111. Nokas G, Kotsilieris T. Preventing keratoconus through eye rubbing activity detection: A machine learning approach. Electronics. (2023) 12:1028. doi: 10.3390/electronics12041028
112. Kenney MC, Nesburn AB, Burgeson RE, Butkowski RJ, Ljubimov AV. Abnormalities of the extracellular matrix in keratoconus corneas. Cornea. (1997) 16:345–51. doi: 10.1097/00003226-199705000-00016
113. Herber R, Pillunat LE, Raiskup F. Development of a classification system based on corneal biomechanical properties using artificial intelligence predicting keratoconus severity. Eye Vis (Lond). (2021) 8:21. doi: 10.1186/s40662-021-00244-4
114. Yousefi S, Yousefi E, Takahashi H, Hayashi T, Tampo H, Inoda S, et al. Keratoconus severity identification using unsupervised machine learning. PloS One. (2018) 13:e0205998. doi: 10.1371/journal.pone.0205998
115. Mahmoud HAH, Mengash HA. Automated keratoconus detection by 3D corneal images reconstruction. Sensors (Basel). (2021) 21:2326. doi: 10.3390/s21072326
116. Issarti I, Consejo A, Jiménez-García M, Kreps EO, Koppen C, Rozema JJ. Logistic index for keratoconus detection and severity scoring (Logik). Comput Biol Med. (2020) 122:103809. doi: 10.1016/j.compbiomed.2020.103809
117. Aatila M, Lachgar M, Hamid H, Kartit A. Keratoconus severity classification using features selection and machine learning algorithms. Comput Math Methods Med. (2021) 2021:9979560. doi: 10.1155/2021/9979560
118. Malyugin B, Sakhnov S, Izmailova S, Boiko E, Pozdeyeva N, Axenova L, et al. Keratoconus diagnostic and treatment algorithms based on machine-learning methods. Diagnostics (Basel). (2021) 11:1933. doi: 10.3390/diagnostics11101933
119. Tai HY, Lin JJ, Huang YH, Shih PJ, Wang IJ, Yen JY. Correlation between corneal dynamic responses and keratoconus topographic parameters. J Int Med Res. (2022) 50:3000605221108100. doi: 10.1177/03000605221108100
120. Kamiya K, Ayatsuka Y, Kato Y, Shoji N, Mori Y, Miyata K. Diagnosability of keratoconus using deep learning with placido disk-based corneal topography. Front Med (Lausanne). (2021) 8:724902. doi: 10.3389/fmed.2021.724902
121. Chen X, Zhao J, Iselin KC, Borroni D, Romano D, Gokul A, et al. Keratoconus detection of changes using deep learning of colour-coded maps. BMJ Open Ophthalmol. (2021) 6:e000824. doi: 10.1136/bmjophth-2021-000824
122. Dos Santos VA, Schmetterer L, Stegmann H, Pfister M, Messner A, Schmidinger G, et al. CorneaNet: fast segmentation of cornea OCT scans of healthy and keratoconic eyes using deep learning. BioMed Opt Express. (2019) 10:622–41. doi: 10.1364/BOE.10.000622
123. Dong Y, Li D, Guo Z, Liu Y, Lin P, Lv B, et al. Dissecting the profile of corneal thickness with keratoconus progression based on anterior segment optical coherence tomography. Front Neurosci. (2021) 15:804273. doi: 10.3389/fnins.2021.804273
124. Lavric A, Anchidin L, Popa V, Al-Timemy AH, Alyasseri Z, Takahashi H, et al. Keratoconus severity detection from elevation, topography and pachymetry raw data using a machine learning approach. IEEE Access. (2021) 9:84344–55. doi: 10.1109/ACCESS.2021.3086021
125. Bolarín JM, Cavas F, Velázquez JS, Alió JL. A machine-learning model based on morphogeometric parameters for RETICS disease classification and GUI development. Appl Sci. (2020) 10:1874. doi: 10.3390/app10051874
126. Zorto AD, Sharif MS, Wall J, Brahma A, Alzahrani AI, Alalwan N. An innovative approach based on machine learning to evaluate the risk factors importance in diagnosing keratoconus. Inf Med Unlocked. (2023) 38. doi: 10.1016/j.imu.2023.101208
127. Smolek MK, Klyce SD. Current keratoconus detection methods compared with a neural network approach. Invest Ophthalmol Vis Sci. (1997) 38:2290–9.
128. Werkmeister RM, Sapeta S, Schmidl D, Garhöfer G, Schmidinger G, Aranha Dos Santos V, et al. Ultrahigh-resolution OCT imaging of the human cornea. BioMed Opt Express. (2017) 8:1221–39. doi: 10.1364/BOE.8.001221
129. Kundu G, Shetty N, Shetty R, Khamar P, D’Souza S, Meda TR, et al. Artificial intelligence-based stratification of demographic, ocular surface high-risk factors in progression of keratoconus. Indian J Ophthalmol. (2023) 71:1882–8. doi: 10.4103/IJO.IJO_2651_22
130. Shetty R, Kundu G, Narasimhan R, Khamar P, Gupta K, Singh N, et al. Artificial intelligence efficiently identifies regional differences in the progression of tomographic parameters of keratoconic corneas. J Refract Surg. (2021) 37:240–8. doi: 10.3928/1081597X-20210120-01
131. Reddy JC, Bhamidipati P, Dwivedi S, Dhara KK, Joshi V, Hasnat Ali M, et al. KEDOP: Keratoconus early detection of progression using tomography images. Eur J Ophthalmol. (2022) 32:2554–64. doi: 10.1177/11206721221087566
132. Jiménez-García M, Issarti I, Kreps EO, ND S, Koppen C, Varssano D, et al. Forecasting progressive trends in keratoconus by means of a time delay neural network. J Clin Med. (2021) 10:3238. doi: 10.3390/jcm10153238
133. Kamiya K, Ayatsuka Y, Kato Y, Shoji N, Miyai T, Ishii H, et al. Prediction of keratoconus progression using deep learning of anterior segment optical coherence tomography maps. Ann Transl Med. (2021) 9:1287. doi: 10.21037/atm
134. Kato N, Masumoto H, Tanabe M, Sakai C, Negishi K, Torii H, et al. Predicting keratoconus progression and need for corneal crosslinking using deep learning. J Clin Med. (2021) 10:844. doi: 10.3390/jcm10040844
135. Cao K, Verspoor K, Chan E, Daniell M, Sahebjada S, Baird PN. Stratification of keratoconus progression using unsupervised machine learning analysis of tomographical parameters. Intelligence-Based Med. (2023) 7:100095. doi: 10.1016/j.ibmed.2023.100095
136. Fariselli C, Vega-Estrada A, Arnalich-Montiel F, Alio JL. Artificial neural network to guide intracorneal ring segments implantation for keratoconus treatment: a pilot study. Eye Vis (Lond). (2020) 7:20. doi: 10.1186/s40662-020-00184-5
137. Yousefi S, Takahashi H, Hayashi T, Tampo H, Inoda S, Arai Y, et al. Predicting the likelihood of need for future keratoplasty intervention using artificial intelligence. Ocul Surf. (2020) 18:320–5. doi: 10.1016/j.jtos.2020.02.008
138. Valdés-Mas MA, Martín-Guerrero JD, Rupérez MJ, Pastor F, Dualde C, Monserrat C, et al. A new approach based on Machine Learning for predicting corneal curvature (K1) and astigmatism in patients with keratoconus after intracorneal ring implantation. Comput Methods Programs Biomed. (2014) 116:39–47. doi: 10.1016/j.cmpb.2014.04.003
139. Liu Y, Shen D, Wang HY, Qi MY, Zeng QY. Development and validation to predict visual acuity and keratometry two years after corneal crosslinking with progressive keratoconus by machine learning. Front Med (Lausanne). (2023) 10:1146529. doi: 10.3389/fmed.2023.1146529
140. Lyra D, Ribeiro G, Torquetti L, Ferrara P, MaChado A, Lyra JM. Computational models for optimization of the intrastromal corneal ring choice in patients with keratoconus using corneal tomography data. J Refract Surg. (2018) 34:547–50. doi: 10.3928/1081597X-20180615-01
141. Firat M, Cinar A, Cankaya C, Firat IT, Tuncer T. Prediction of Pentacam image after corneal cross-linking by linear interpolation technique and U-NET based 2D regression model. Comput Biol Med. (2022) 146:105541. doi: 10.1016/j.compbiomed.2022.105541
142. Belin MW. Chapter 15 - scheimpflug imaging for keratoconus and ectatic disease. In: Izquierdo L, Henriquez M, Mannis M, editors. Keratoconus. Elsevier, New Delhi (2023). p. 203–20.
143. Hashemi H, Asgari S, Emamian MH, Mehravaran S, Fotouhi A. Age-related changes in corneal curvature and shape: the shahroud eye cohort study. Cornea. (2015) 34:1456–8. doi: 10.1097/ICO.0000000000000595
144. Baboolal SO, Smit DP. South African Eye Study (SAES): ethnic differences in central corneal thickness and intraocular pressure. Eye. (2018) 32:749–56. doi: 10.1038/eye.2017.291
145. Alsaqr AM, Fagehi R, Sharha AA, Alkhudair M, Alshabrami A, Muammar AB, et al. Ethnic differences of corneal parameters: A cross-sectional study. Open Ophthalmol J. (2021) 15:13–20. doi: 10.2174/1874364102115010013
146. Pagano L, Posarelli M, Giannaccare G, Coco G, Scorcia V, Romano V, et al. Artificial intelligence in cornea and ocular surface diseases. Saudi J Ophthalmol. (2023) 37:179–84. doi: 10.4103/sjopt.sjopt_52_23
147. Shanthi S, Aruljyothi L, Balasundaram MB, Janakiraman A, Nirmaladevi K, Pyingkodi M. Artificial intelligence applications in different imaging modalities for corneal topography. Surv Ophthalmol. (2022) 67:801–16. doi: 10.1016/j.survophthal.2021.08.004
148. Zhang Z, Wang Y, Zhang H, Samusak A, Rao H, Xiao C, et al. Artificial intelligence-assisted diagnosis of ocular surface diseases. Front Cell Dev Biol. (2023) 11. doi: 10.3389/fcell.2023.1133680
149. Hashemi H, Doroodgar F, Niazi S, Khabazkhoob M, Heidari Z. Comparison of different corneal imaging modalities using artificial intelligence for diagnosis of keratoconus: a systematic review and meta-analysis. Graefes Arch Clin Exp Ophthalmol. (2023) 11:1133680. doi: 10.1007/s00417-023-06154-6
150. Li Z, Wang L, Wu X, Jiang J, Qiang W, Xie H, et al. Artificial intelligence in ophthalmology: The path to the real-world clinic. Cell Rep Med. (2023) 4:101095. doi: 10.1016/j.xcrm.2023.101095
Keywords: keratoconus, corneal ectasia, artificial intelligence, machine learning, deep learning
Citation: Goodman D and Zhu AY (2024) Utility of artificial intelligence in the diagnosis and management of keratoconus: a systematic review. Front. Ophthalmol. 4:1380701. doi: 10.3389/fopht.2024.1380701
Received: 02 February 2024; Accepted: 23 April 2024;
Published: 17 May 2024.
Edited by:
Namrata Sharma, All India Institute of Medical Sciences, IndiaReviewed by:
Davide Borroni, Riga Stradiņš University, LatviaMahmoud T. KhalafAllah, University of Alabama at Birmingham, United States
Copyright © 2024 Goodman and Zhu. This is an open-access article distributed under the terms of the Creative Commons Attribution License (CC BY). The use, distribution or reproduction in other forums is permitted, provided the original author(s) and the copyright owner(s) are credited and that the original publication in this journal is cited, in accordance with accepted academic practice. No use, distribution or reproduction is permitted which does not comply with these terms.
*Correspondence: Angela Y. Zhu, YXpodUBtaWFtaS5lZHU=
†These authors have contributed equally to this work