Explore article hub
- 1Research Center “E. Piaggio”, University of Pisa, Pisa, Italy
- 2Department of Information Engineering, University of Pisa, Pisa, Italy
- 3Interuniversity Center for the Promotion of the 3R Principles in Teaching and Research, (Centro 3R), Pisa, Italy
A Viewpoint on the Frontiers in Science Lead Article
Organoid intelligence (OI): the new frontier in biocomputing and intelligence-in-a-dish
Key points
- Brain organoids are a unique template for engineering a new era of bio-inspired supercomputer technology.
- The power requirements for exploiting brain organoids may be higher than those of the most powerful computers.
- Further research is needed to accelerate sustainable solutions in brain organoid-leveraged supercomputing technologies.
Introduction
Since the inception of brain organoids in 2013, research and discussion on the topic have grown exponentially. Their ability to recapitulate anatomical and functional features of the human brain has aroused global interest. Between 2013 and 2022, on average more than 10 articles per month were published on brain organoids (1826), and over 1/3 of them were not original research but reviews (587, source PubMed). Scientists have exploited brain organoids as brain mimicry tools for basic research, toxicity, and drug testing and have pondered over their potential sentiency and consciousness. While these debated features of brain organoids have not been detected, the speculation of advanced applications beyond what is currently possible is an exciting prospect. Smironova et al. (1) make a convincing case for the supremacy of the human brain in terms of its computational and cognitive capacity and very low power consumption. These parameters are compared with the best computers, which are known to have a substantial carbon footprint. They also make a compelling argument for exploiting brain organoids as the next generation of machinery for biocomputing. Since these 3D constructs might perform as parts of a human brain, they reason, different constructs paralleled together might operate as efficiently and effectively as our brains do. Thus, a program of interdisciplinary research is envisioned for exploiting the –unknown and yet to be unleashed – computational power of brain organoids for memorizing and computing inputs and for interfacing them to machines to advance our understanding of cognition, memory, neuro-degenerative and developmental diseases, and for applications in biomedicine.
Energy considerations of organoid intelligence
The authors admit that brain organoids will consume more power than their human brain equivalents, owing to the infrastructure and resources required for their generation and maintenance. A key question is: how do the input energy requirements compare with those of a supercomputer? Reasonable estimates can be made starting from the energy requirements for tissue engineered or cultivated meat, which rely on incubators, media, hydrogel scaffolds, and perfusion systems – very similar to organoids. Assuming 30 days of culture, the carbon footprint (using conventional energy sources) for producing engineered meat is 13.6 kg CO2/kg. Most of this is electricity, with an energy equivalent of 200 MJ/kg or 55 kWh/kg (2). Current technology generates organoids weighing around 4.10-7 kg, which might provide computation for 30 days after maturation and training. Thus, a single organoid requires 3.10-5 W to run for its – rather short – lifetime, while its human brain equivalent (i.e., a piece of brain weighing the same) requires 6.10-6 W (i.e., five-fold less power than its organoid equivalent). Intriguingly, these numbers (summarized in Table 1) do suggest that the power needed to maintain an organoid is much less than for a computer with the same storage capacity – making a strong case for organoid intelligence (OI) over computer intelligence. However, these calculations are based on mass and numbers of neurons; we are not considering the real storage capacity or calculation speed of brain organoids, neither of which have yet been quantified. We note also that the brain and its approximately equivalent supercomputer survive much longer, while brain organoids have a shorter lifespan, thus they will need to be continuously generated and then trained to be useful, which adds to their total energetic cost.
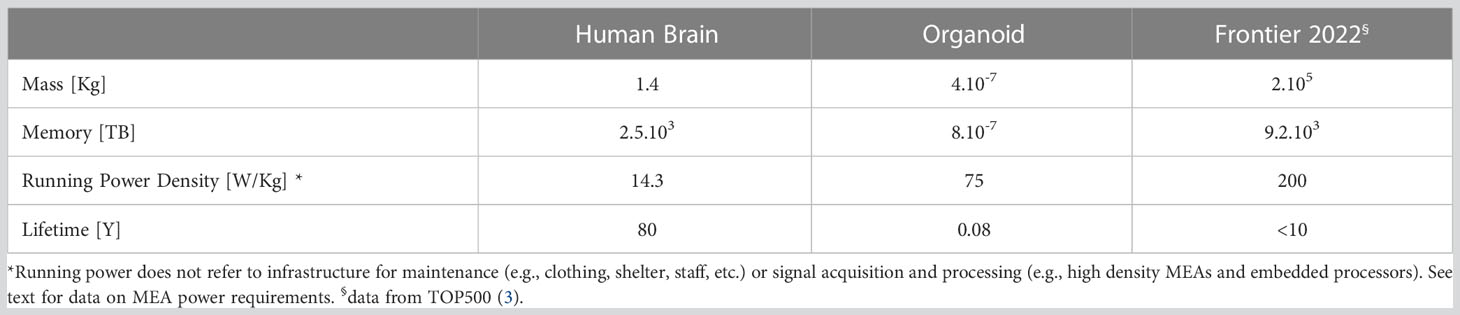
Table 1 Power requirements for maintenance of the average adult human brain, organoids, and the Frontier supercomputer the US Department of Energy’s Oak Ridge National Laboratory.
Besides maintenance requirements, exploiting OI requires additional power resources, specifically high-density microelectrode arrays (MEAs) and associated processing and storage. For example, the Neuropixels probe itself consumes only 36.5 mW (4), but this is only around 10% of the power needed for recording electrophysiological signals from high density arrays (Maxwell Biosystems MaxOne datasheet). Adding this to the data in Table 1 makes the total power density requirement for organoids a whopping 1 MW/kg. Finally, as prospected by Smirnova et al., appropriate “computational harnessing” would also necessitate big data and machine learning architectures for support, further adding to the total energy footprint.
Organoids: Supercomputers of the future?
In addition to energetic considerations, considerable improvements must be made for assessment of cerebral organoids as the supercomputers of the future. Crucially, we assume that brain organoids function as downscaled models of the brain (or parts of it), so running them in parallel should result in something that works like a whole brain. But we still have no means of extrapolating or upscaling to determine their fidelity. Furthermore, and in relation to their uncharted fidelity, they still suffer from ‘batch syndrome’: they are noisy, with little or no reproducibility. Humans and their brains are all different, and it is not clear whether the huge variability in cerebral organoids is attributable to their ability to resemble the intrinsic heterogeneities and diversity of living organisms, which may be due to genetic makeup or environmental fluctuations, or if they are just plain noisy and unreliable. Such variability is likely to be reflected in the organoid neural networks, which may lead to unwanted, unexpected, and non-linear or unpredictable outcomes when using them to memorize and compute inputs.
So it makes more sense to make better computers that run on sustainable energy sources and that work more like brains inspired by the human brain’s architecture, with all its diversity. In this scenario, leveraging OI and looking beyond, we envisage the digitization of brain organoids as a first step to reverse engineering brain intelligence in-silico (5). To start, we need to decipher the organoid connectome using multiscale imaging and volumetric reconstruction (6). Then the organoid connectome could be implemented as a neural network, whose neurons are described by their morphology and metabolic and electrophysiological dynamics, and whose cell-to-cell connections (in terms of number, strength, and plasticity) mimic the ability of biological neural networks to store memory, learn tasks, etc. In this way, we can avoid the many disadvantages of culturing brain organoids for computational purposes, namely the need for highly skilled personnel and high energy costs (Table 1), a sterile environment, incubators, pumps, and continuous interfacing with electronics for signal recording and network stimulation. Moreover, we can leverage a truly bioinspired architecture, with an efficiency closer to our brains, running on ‘classical’ hardware, which is easier to maintain and has a longer lifespan.
That is not to say that brain organoids do not serve any purpose: besides giving us the unprecedented opportunity to study the brain up close, they are also exquisite tools for understanding brain development and disease, and instruments for personalized and precision medicine, reducing – and we hope one day, replacing – the use of animals in biomedical science. The program outlined by Smirnova et al. could help achieve major milestones in medicine, science, and technology, spearheading groundbreaking research. It could also provide the tools to go beyond the limits of biological computing (using biological components to build computers) and to engineer new hybrid systems which perform both bio-inspired computing (including machine learning and artificial intelligence) and classical computation.
Author contributions
All authors contributed to the conception and writing of the manuscript.
Funding
Work supported by funding from the European Union’s HORIZON EIC program under grant agreement No 101099310 (NAP) and by the Italian Ministry of Education and Research (MIUR) in the framework of the FoReLab project (Departments of Excellence).
Conflict of interest
The authors declare that the research was conducted in the absence of any commercial or financial relationships that could be construed as a potential conflict of interest.
Publisher’s note
All claims expressed in this article are solely those of the authors and do not necessarily represent those of their affiliated organizations, or those of the publisher, the editors and the reviewers. Any product that may be evaluated in this article, or claim that may be made by its manufacturer, is not guaranteed or endorsed by the publisher.
References
1. Smirnova L, Caffo BS, Gracias DH, Huang Q, Morales Pantoja IE, Tang B, et al. Organoid intelligence (OI): the new frontier in biocomputing and intelligence-in-a-dish. Front Sci (2023) 1:1017235. doi: 10.3389/fsci.2023.1017235
2. Sinke P, Swartz E, Sanctorum H, van der Giesen C, Odegard I. Ex-ante life cycle assessment of commercial-scale cultivated meat production in 2030. Int J Life Cycle Assess. (2023). doi: 10.1007/s11367-022-02128-8
3. TOP500 (2022). Top 500 List. Available at: https://www.top500.org/lists/top500/2022/11/ [Accessed 11 January 2023].
4. Steinmetz NA, Aydin C, Lebedeva A, Okun M, Pachitariu M, Bauza M, et al. Neuropixels 2.0: A miniaturized high-density probe for stable, long-term brain recordings. Science (2021) 372:6539. doi: 10.1126/science.abf4588
5. Cauwenberghs G. Reverse engineering the cognitive brain. PNAS (2013) 110:39. doi: 10.1073/pnas.1313114110
Keywords: brain organoid, intelligence, power requirements, supercomputers, biological computing
Citation: Magliaro C and Ahluwalia A. To brain or not to brain organoids. Front Sci (2023) 1:1148873. doi: 10.3389/fsci.2023.1148873
Received: 20 January 2023; Accepted: 24 January 2023;
Published: 28 February 2023.
Edited and Reviewed by:
Richard S. Frackowiak, Swiss Federal Institute of Technology Lausanne, SwitzerlandCopyright © 2023 Magliaro and Ahluwalia. This is an open-access article distributed under the terms of the Creative Commons Attribution License (CC BY). The use, distribution or reproduction in other forums is permitted, provided the original author(s) and the copyright owner(s) are credited and that the original publication in this journal is cited, in accordance with accepted academic practice. No use, distribution or reproduction is permitted which does not comply with these terms.
*Correspondence: Arti Ahluwalia, YXJ0aS5haGx1d2FsaWFAdW5pcGkuaXQ=