- 1Department of Hydrology & Water Resources, Hohai University, Nanjing, China
- 2Ministry of Water, Sanitation & Irrigation, Nairobi, Kenya
- 3Department of Earth and Climate Sciences, University of Nairobi, Nairobi, Kenya
- 4IGAD Climate Predictions and Applications Centre, Nairobi, Kenya
- 5Department of Water Conservancy and Hydropower, Hohai University, Nanjing, China
Flooding in riverine basins remains a recurring disaster, often leading to extensive property destruction and, in extreme scenarios, loss of lives. In recent years, the Shouchang River Basin in Zhejiang Province, China, has experienced increasing flood risks, driven by a combination of extreme weather events, urban expansion, and alterations in natural land use. Managing these events is becoming increasingly crucial to minimize the impact on vulnerable communities and critical infrastructure. This study develops an integrated framework for flood forecasting and hydrodynamic floodplain mapping using HEC-HMS and HEC-RAS 6.5 over a 10 km stretch of the Shouchang River upstream of Shouchang Town. The hydrological model (HEC-HMS) simulates rainfall-runoff processes across five sub-basins, using observed rainfall and streamflow data from four gauging stations, to capture key flow dynamics. Based on local plans for Shouchang Town, a total of 28 villages are situated within exposure areas of sub-basin 5. Out of villages only 22 rescue centers are found to be unaffected and thus effective for sheltering flood victims. Four rescue centers, Yongjiaqiao, Henanli, Ximen, and Datanbian would need relocation to higher grounds, including adding new resettlement sites and modifying transfer plans and routes. Simulations show that, while flood defenses protect most regions under upstream flows of 1,200 m3/s, the levees along Shili Shouchngjiang Ecology Leisure Greenway breach once this threshold is surpassed. The study highlights the need to review the existing flood evacuation and transfer analysis system, given that some evacuation centers could be exposed to flood associated risks.
1 Introduction
Floods are regarded as one of the most catastrophic disasters worldwide, second to earthquakes, being aggravated by a combination of several natural and man-made elements (https://science.nasa.gov/climate-change/extreme-weather/; Jonkman et al., 2024; Ritchie et al., n.d.; Yu et al., 2022). Hydro-meteorological disasters cause a great deal of death worldwide and have a profound effect, particularly on populations who are less resilient, usually being residents of low to middle income nations with inadequate flood protection infrastructure, and insufficient response and evacuation systems. Over 2 billion people are in danger of flooding globally, and these hazards are anticipated to rise as a result of uncontrolled development and climate change (Devitt et al., 2023; Rentschler et al., n.d.). Extreme rainfall events due to climate change (https://gpm.nasa.gov/) and urbanization-induced surface runoff are viewed to be two interconnected factors that have increasingly driven flooding cases globally (Rentschler et al., n.d.; Zhang et al., 2018; Du et al., 2019; Dharmarathne et al., 2024; Miller and Hutchins, 2017). Climate change has resulted in heavier and more erratic rainfall (https://gpm.nasa.gov/; United States Environmental Protection Agency (US EPA), n.d.), leading to greater river discharge. Simultaneously, urbanization has reduced the natural capacity of landscapes to absorb water by replacing permeable areas with impervious surfaces (Sohn et al., 2020; Ding et al., 2022), such as concrete and asphalt, significantly weakening natural evaporation (Toronto Region Conservation Authority, 2008). The combined effect results in higher runoff volumes, overwhelming rivers and drainage systems, increasing the frequency, and intensity of floods.
Extreme rainfall events in heavily urbanized regions are major triggers for urban floods since the traditional river systems cannot handle the surge of water. China is one of the countries where flooding has become a regular occurrence in its rivers and cities due to rapid urban expansion and climate change (Ding et al., 2022; Islam and Wang, 2024; Ma et al., 2022). The country has witnessed several major flood events, including the 2016 floods that affected over 60 million people, resulting in significant loss of life and economic damage (Jiang et al., 2018; Ritchie et al., n.d.). Rivers in Zhejiang Province, such as the Shouchang River experience seasonal flooding caused by heavy rainfall and urban expansion. Recently, a report published on 4th of July 2024 by China's State Flood Control and Drought Relief indicated that China's flood control efforts had entered a critical phase, with 33 rivers nationwide exceeding warning levels by June 24, 2024, according to the Ministry of Water Resources. Triggered by persistent heavy downpours, 22 rivers in Jiangxi, Hubei, Anhui, Zhejiang and Guizhou had reported floods exceeding alert marks that resulted in pelting of many areas by the torrential rains (Xinhua News Agency, n.d.). These rivers included Xin'an River which has one of its major tributaries as Shouchang river. As part of mitigative measures authorities had to initiate reservoirs to release water for flood control in cities and towns like Jiande and Shouchang, East China's Zhejiang province. Even with flood protection infrastructure, there still remains challenges in developing effective flood management strategies due to the dynamic interplay of physical, socio-economic, and hydrological factors (Awah et al., 2024; Mai et al., 2020). Major damages in urban, semi-urban, and rural environments have been experienced when hydrographic networks burst banks during severe floods (Boudreau et al., n.d.). In the domain of hydrological forecasting and disaster management, accurate prediction of floods can help mitigate potential risks and reduce the impact on communities and infrastructure (Kumar et al., 2023; Merz et al., 2020; Kioko and Ouya, n.d.).
Although other research work has been done in this basin, this study addresses the need for an integrated approach, by marrying structural and non-structural mechanisms to manage flood risks on the Shouchang River in Zhejiang Province. The study aims at; (1) mapping flood-prone areas within the Shouchang River basin, (2) developing flood early warning system by the use of coupled HEC-HMS and AR models to predict both runoff and stream flow at the Yuankou hydrological station based on historical rainfall data, (3) providing actionable insights for flood mitigation by aligning flood forecasts with hydrodynamic models, and (4) constructing a flood evacuation transfer analysis model based on hazard zones and evacuation units, evacuation population, evacuation methods and designating resettlement areas. It is anticipated that the flood early warning system, together with the early actions related to evacuation and transfer, could be applied not only to the Shouchang River but also to other flood-prone regions.
The subsequent sections of the manuscript are arranged as follows; Section 2 presents the Study Area, Data and Methods are presented in Section 3, while the Results, Discussion and Conclusion follow in Sections 4–6, respectively.
2 Study area
Shouchang Town, located in the southwest-central part of Jiande City in Zhejiang Province, China, spans an area of 145.75 km2 and has a population of 45,000 people, as recorded by the Zhejiang Provincial Government in 2023. Geographically, the town lies between latitudes 29.000°N and 29.050°N, and longitudes 118.967°E and 119.250°E, with an elevation of ~73.55 m (241.31 ft) above sea level. The basin lies within the broader Qianjiang River system, which is a tributary of the larger Qiantang River. The basin is characterized by a mix of mountainous terrain and valleys. The surrounding mountains influence the flow of water into the basin, creating a network of streams and rivers that converge in the low-lying areas. The predominant soil type in the basin is typically red soil, which is common in southern China The region's topography features mountainous terrain, steep slopes, river basins, and low-lying areas, making it susceptible to natural hazards such as landslides, riverine and flash floods since land use here majorly consists of residential areas, farmlands, reservoirs, tourist attractions, and infrastructure such as roads, dikes, and power facilities.
In the central and low-hill regions of the basin, human activities have fragmented the evergreen broad-leaved forests over time, giving way to coniferous forests and plantations (Zhu et al., 1991). Common cultivated species include citrus, Chinese tallow, camellia, oleifera, and bamboo. The northern mountainous areas feature natural vegetation such as Pinus massoniana, Cunninghamia lanceolata, Phyllostachys pubescens, tung oil trees, and tea. Large tracts of artificial warm coniferous forests, including Cunninghamia lanceolata, Cryptomeria fortunei, and Huangshan pine, dominate the landscape, accounting for over 90% of the forested area (Zhu et al., 1991). The Shouchang River basin forms the basis of the study area for this research, with the most vulnerable subbasin being subbasin five (5) as illustrated in Figure 1. The river, originating from Shangmacun and Lijiazhen towns, flows for over 60 km downstream. This study focuses on a 10 km section of the river, chosen for its geographical and socio-economic significance. The total catchment area of the basin is ~690 km2, where higher elevations are particularly prone to landslides and lower areas are at risk of inundation.
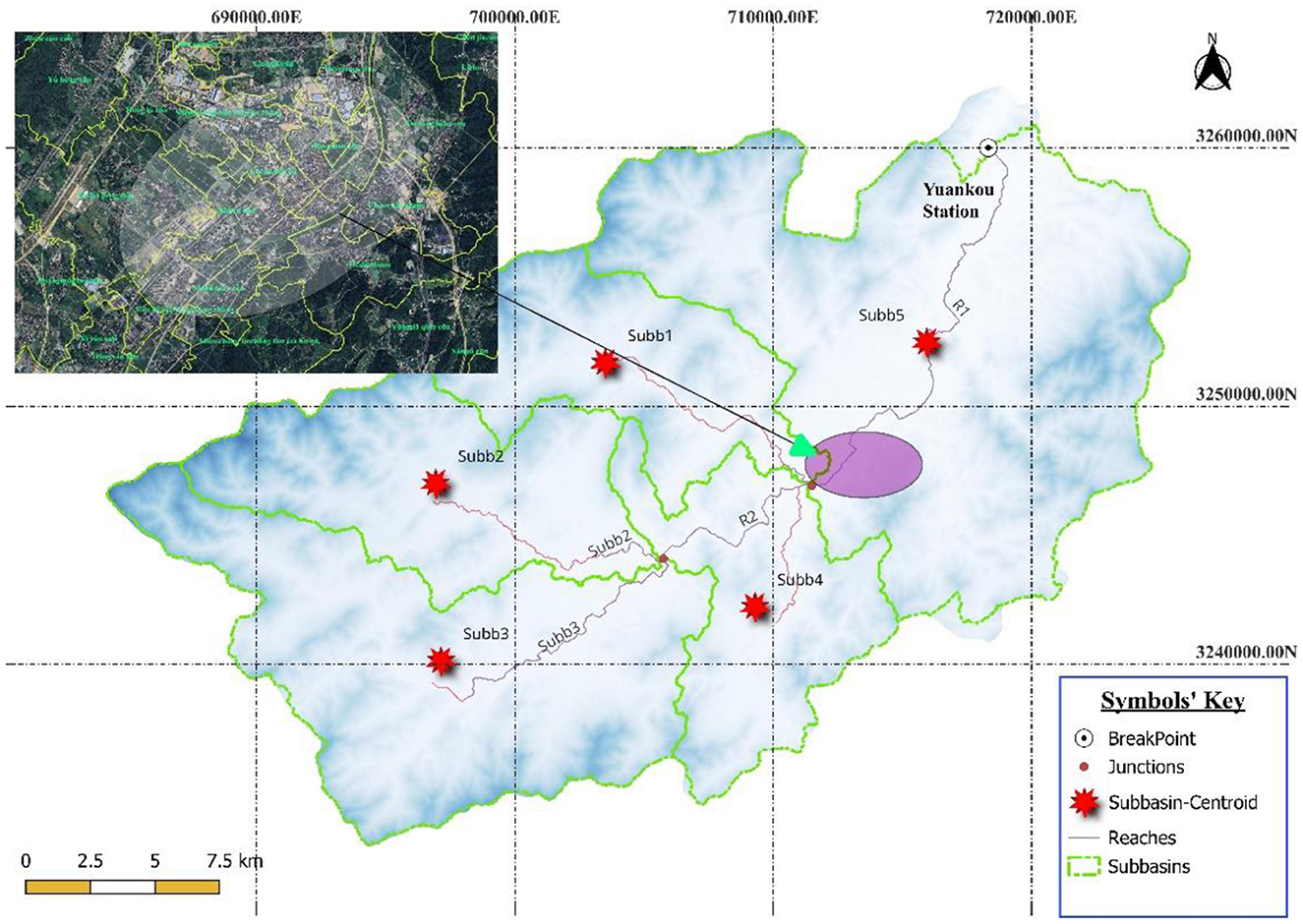
Figure 1. The five sub-basins within the study area forming part of the Shouchang River Basin as delineated for this study. The highlight in purple indicates the focus area, with the topographic map presented on the top-right inset. R1 and R2 presents the main river outlines, with tributaries joining at the points labeled Junctions.
The catchment generally experiences a humid subtropical climate (Cfa) with an average annual temperature of 18.63°C (65.53°F), which is about 0.18°C warmer than China's national average. The region receives about 167.64 mm (6.6 in) of precipitation annually, distributed about 178 rainfall days, making up 48.87% of the year. The combination of frequent rainfall, low elevation, and steep terrain increases the likelihood of flooding, water-logging, and landslides. Given these geographic and climatic conditions, comprehensive flood control measures are essential to protect the town's population and infrastructure. To address the flood risks effectively, grid-based monitoring and disaster preparedness plans are implemented alongside hydrodynamic floodplain mapping.
3 Data and methods
This section presents the various datasets and techniques utilized to achieve the objectives of the study.
3.1 Terrain model, land use and meteorological datasets
Digital terrain model (DTM) is a critical component in hydrological and hydraulic modeling as it governs the river channel network and water routing both within and outside the channels. This study utilized the 12.5 m resolution DTM from the Alaska Satellite Facility (https://search.asf.alaska.edu/). The 12.5 m resolution provides a balance between computational efficiency and spatial detail, enabling accurate representation of topographic features such as slopes, depressions, and drainage patterns, which are essential for flood prediction. The vertical accuracy of the DTM is reported to be within ±2 m, which is suitable for large-scale flood modeling studies.
To further enhance the terrain representation, cross-sections are defined and adjusted within the model to achieve an effective resolution of 5 m, after trade-offs between resolution and computational demand have been factored. The 12.5 m DTM, enhanced to 5 m in critical areas, strikes a balance between detail and computational feasibility, ensuring reliable flood predictions while maintaining model efficiency. This refinement ensures a more precise representation of river morphology and flow patterns, particularly in areas with complex topography. The higher resolution is especially important for capturing small-scale features that influence flood dynamics, such as levees, embankments, and floodplains, which are critical for accurately simulating flood extents and depths. For instance, finer resolution helps in identifying subtle elevation changes that can divert floodwaters or create localized pooling. Land-cover and land-use data at 30 m resolution are obtained from the United States Geological Survey (USGS) Earth Explorer platform (https://earthexplorer.usgs.gov/). To ensure consistency between the DTM and land-use datasets, the land-use data are resampled to match the 12.5 m resolution of the DTM using a nearest-neighbor interpolation technique. This approach preserves the categorical nature of the land-use data while aligning it with the higher-resolution DTM. The resampled land-use data are then integrated with the DTM to account for the impact of land surface characteristics on hydrological processes, such as infiltration and surface runoff.
Hydrometric indicators, including flow rates, precipitation, and evaporation data from 2001 to 2020, are acquired from the Emergency Plan of Flood Control, Typhoon Prevention, and Drought Relief for Shouchang Town, Jiande City (Liang et al., 2022). The meteorological data include records of major storm events, such as those occurring on June 15, 2011; June 8, 2014; May 27, 2008; April 29, 2012; and June 17, 2017. These events were selected to represent a range of flood scenarios for model calibration and validation.
3.2 Processing of input datasets
The study utilizes the 2D version of HEC-RAS 6.5, a hydraulic modeling software designed by the U.S. Army Corps of Engineers, using the following key steps:
• Mapping the topographic data, both DTM and land use to the study domain:
In this study, the DTM and land use data are mapped to the study domain. The DTM is preprocessed to remove artifacts and outliers using a combination of manual inspection and automated filtering techniques. For instance, Python's pandas library is applied to handle and clean the data, removing any erroneous elevation values, including negative values and extreme outliers that could distort the terrain representation. Cross-sections are then defined and adjusted within the model to enhance terrain accuracy, achieving the higher resolution of 5 m. This involved interpolating missing data points using spatial interpolation techniques, ensuring a more precise representation of river morphology and flow patterns.
• Meteorological data processing:
At this step the rainfall data is preprocessed by calculating the inverse distance weights (IDW) for each gauge, since all the coordinates are available, based on proximity to the hydrological station. This is implemented using Python's scipy.spatial and numpy libraries to compute the weights and normalize them. The weighted rainfall values are then aggregated to compute a total weighted rainfall for each station. Discharge data is then merged with the weighted rainfall data for modeling. Missing or inconsistent discharge records are handled using linear interpolation and by applying a rolling mean to smooth the data, ensuring continuity and reliability in the input dataset. The evaporation data is not explicitly processed but is averaged and directly loaded into the model. Any gaps in the evaporation dataset are filled using a simple mean imputation method.
• River geometry and mesh generation:
HEC-RAS employs a finite-volume solution scheme to generate structured and unstructured meshes. The river geometry is extracted using RAS Mapper (U.S. Army Corps of Engineers, n.d.f), which involved delineating the main channel and bank-lines within the original DTM. Python's geopandas library is used to preprocess and validate the spatial data, ensuring accurate representation of the river system (U.S. Army Corps of Engineers, n.d.c; Ongdas et al., 2020). HEC-RAS Mapper was utilized to develop the RAS terrain, outline the geometric dataset, extract the terrain data, and visualize the outcomes in the form of tables and maps (U.S. Army Corps of Engineers, n.d.b). To further enhance accuracy, cross-sections were manually adjusted within the model to better align with observed river morphology. This step included filtering out noise in the DTM data and refining the mesh resolution to 5 m resolution.
• Visualization and output:
HEC-RAS Mapper was utilized to develop the RAS terrain, outline the geometric dataset, extract terrain data, and visualize the outcomes in the form of tables and maps. Python's matplotlib and seaborn libraries are used for additional visualization and analysis of the preprocessed data, ensuring that the final input datasets met the required quality standards.
3.3 Rainfall–runoff model: HEC-HMS
For this hydrodynamic modeling project, HEC-HMS and HEC-RAS 6.5 are employed as the primary tools to simulate and analyze flood behavior. These models utilize high-resolution ALOS POLAR satellite imagery and DEM (Digital Elevation Model) data to ensure accuracy. The integration of these technologies supports the development of effective flood management strategies and enhances the town's resilience against natural disasters. The overall workflow in this study is presented in Figure 2 ranging from data preparation, model development to the visualization of the outputs.
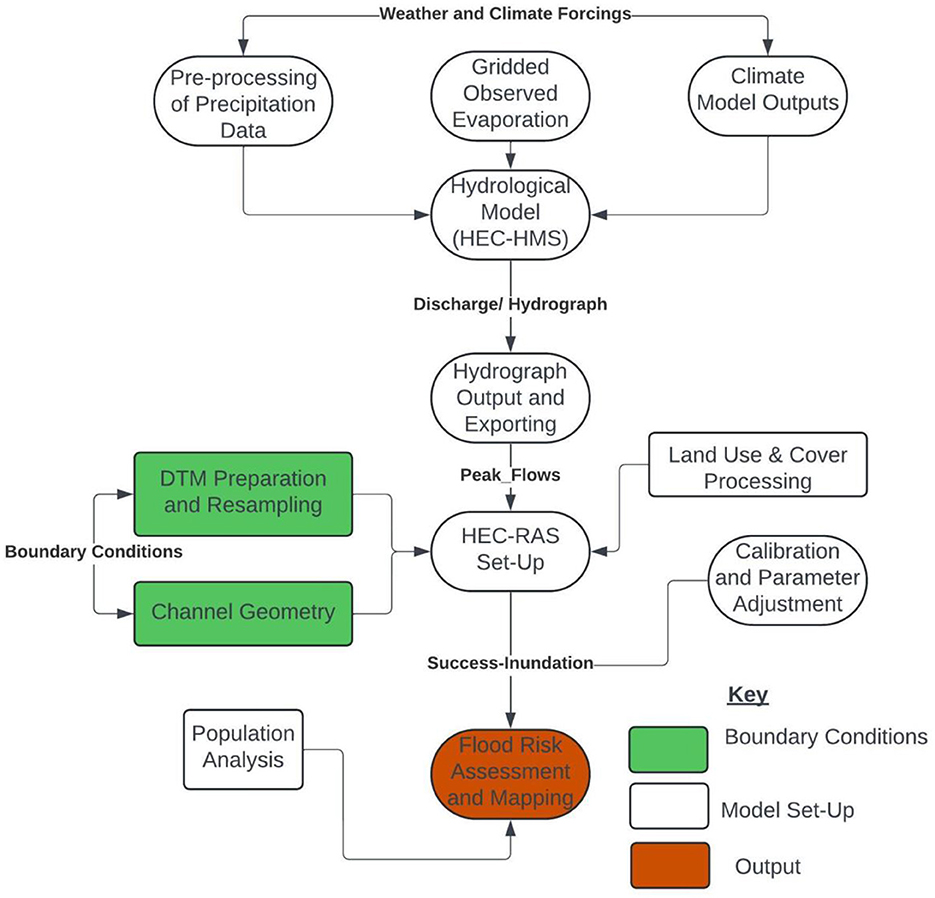
Figure 2. Schematic representation of the workflow involved in data preparation, model setup, and simulation of the case studies.
Flood model set-up is generated by integrating QGIS with the HEC-HMS (rainfall-runoff model) and hydraulic model HEC-RAS for the study area. A rainfall-runoff model is created in HEC-HMS to prepare the input data. The output data of the HEC-HMS model simulation is employed as input data to run the HEC-RAS 2D flow model and generate flood risk and flood inundation maps. HEC-RAS 6.5 is used to facilitate precise floodplain mapping and hydrodynamic simulations of how floodwaters flow through river reaches under various scenarios (U.S. Army Corps of Engineers, n.d.b, n.d.f), while HEC-HMS is used to simulate watershed runoff and rainfall's impact on flood peaks (U.S. Army Corps of Engineers, n.d.e). Major storm events (June 15 2011, June 8, 2014, May 27 2008, April 29 2012, and June 17 2017) that occurred in the past 20 years within the Shouchang River basins are selected to develop the flood events for current flood mapping and analysis. Based on simulation outcomes, flood risk and inundation maps are generated for the Shouchang River basin, with a specific focus on the May 27th 2008 event.
The application of 2D hydraulic modeling using HEC-RAS 6.5 is useful to calculate velocity, water surface elevation (WSE), and cross-sectional average at distinct cross-sections within the river (U.S. Army Corps of Engineers, n.d.d). This process employs the Saint-Venant (SV) equations for 2D flow simulation, considering key assumptions such as small channel slope and hydrostatic pressure distribution. Manning's roughness coefficients of 0.3 for the main stream channel and 0.035 for the floodplain sections are utilized in this model. Boundary conditions, including critical depth, normal depth, known water surface elevations, and rating curves, are established to define the upstream and downstream ends of the simulated river system.
To create the basin model for the study area, a hydrologic model, combined with GIS tools (HEC-GeoHMS), is employed (U.S. Army Corps of Engineers, n.d.d). HEC-HMS simulates the runoff response of the various sub-basins to a certain amount and precipitation distribution over a specified period (U.S. Army Corps of Engineers, n.d.f,n). In cases where high-resolution data were unavailable, land use and cover data were calibrated using the RAS Mapper tool within HEC-RAS. This involved overlaying the DEM with available imagery from open datasets such as Google Earth and Microsoft Bing Maps, identifying land use categories, and refining classifications based on elevation and terrain features for improved accuracy. Based on the land-use classification, impervious percentage, lag time and curve numbers are calculated for each sub-basin which are presented in the results section. Other physical features such as the distance along the stream path, the stream slope and length, the longest flow direction for each sub-basin, and, the location of the sub-basin elevation and its centroid are also derived from the model.
• Model parameters
The HEC-HMS model relies on several key parameters to simulate rainfall-runoff processes, including:
i. Curve number (CN)
This represents the runoff potential of the land surface based on soil type and land use. CN values are calculated for each of the five sub-basins using weighted averages based on land use and soil type distributions.
In subbasins with diverse land use or soil types, calculating a single CN requires weighting the values according to their respective areas. The weighted Curve Numbers here are determined by applying Equation 1.
Where CNi represents the Curve Number for land use/soil type and Areai is the corresponding area. For example, if a subbasin is 30% forest with a CN of 55, 50% agricultural land with a CN of 75, and 20% urban area with a CN of 85, the weighted CN would also have a direct impact on the final values of the lag times.
ii. Lag time (Tl):
This represents the time delay between peak rainfall and peak runoff. It is calculated using Equation 2:
Where Tl is Lag time (hours), L Hydraulic length of the sub-basin (ft or m), S is Potential maximum retention after runoff begins (inches) ~ , CN is Curve number (dimensionless, typically 0–100) and Y is the Average land slope of the watershed (percentage, in decimal form).
iii. Impervious percentage
This parameter is intended to reflect the fraction of the sub-basin area that is impervious, directly influencing runoff volume.
iv. Stream slope and length
Derived from the DEM, these parameters influence the flow velocity and timing of runoff.
v. Initial abstraction
This is incorporated to represent the amount of rainfall absorbed by the soil before runoff begins, typically estimated as 20% of the potential maximum retention (SS).
• Calibration process
The HEC-HMS model was calibrated using observed streamflow data from historical storm events. The calibration process involved the following steps:
In order to achieve parameter initialization, the initial values for CN, lag time, and impervious percentage were derived from land use and soil data. Sensitivity analysis, adjusted against key parameters such as CN and lag time is adjusted iteratively to minimize the difference between simulated and observed runoff hydrographs. Performance metrics: The Nash-Sutcliffe Efficiency (NSE) and Root Mean Square Error (RMSE) are then used to evaluate model performance. The model is considered well-calibrated when NSE values exceeded 0.7 and RMSE values are minimized. Finally validation is done on the calibrated model by use of independent storm events not included in the calibration process to ensure its reliability under different conditions.
3.4 Model for discharge predictions
The predictive model for discharge predictions involves several key steps, including data collection, normalization, model calibration, and validation. The normalization step, which was previously omitted, is crucial for adjusting the flow data to account for seasonal variations and extreme events.
A total of 35 storms are simulated in this study. This step involves applying a normalization technique to the historical flow data, ensuring that the data is scaled appropriately before being integrated into the model. The normalized data is then used to recalibrate the model parameters, improving the accuracy of the discharge predictions.
The model is calibrated and validated using observed streamflow data from historical storm events. The calibration process involved adjusting key parameters, that is, Manning's roughness coefficient, grid sizes, and computation intervals to minimize discrepancies between simulated and observed data. To evaluate the model's performance, the following error metrics were computed.
• Flood volume error
This metric assesses the accuracy of the model in predicting the total volume of floodwater. The absolute and relative errors for flood volume were calculated using Equations 3, 4:
Where; efl is the absolute error for flood volume (m3), Ofl is the relative error for flood volume (%), qt is the forecasted discharge at time t (m3/s), Ot is the observed discharge at time t (m3/s) and Δt is the time interval in hours.
• Peak discharge error
This metric evaluates the model's ability to predict the maximum discharge during a flood event. The absolute and relative errors of peak discharge were computed using Equations 5, 6:
Where epd is the absolute error of peak discharge (m3/s), θpd is the relative error of peak discharge, opeak is the observed peak discharge (m3/s) and qpeak is the forecasted peak discharge.
• Peak time error
This metric is intended to measure the temporal discrepancy between the forecasted and observed peak discharge times. The formula used is shown in Equation 7:
Where Tpeak is the forecasted time and is the flood observation time.
These error metrics provide a comprehensive assessment of the model's performance, highlighting its strengths and areas for improvement. The calibration process ensured that the model accurately captures terrain characteristics and hydrodynamic behaviors, as evidenced by the low error values for flood volume, peak discharge, and peak time.
3.5 Hydrologic model: HEC-RAS
The model is designed to provide key outputs, including velocity, water surface elevation (WSE), and flood inundation maps for 10-, 20-, and 100-year periods. These outputs facilitate the assessment of the impacts of increased flooding under different scenarios. The flood inundation is determined by simulating water flow across the terrain and identifying regions where water depth exceeds a threshold value, typically above ground level. This process involves the following steps:
• Terrain and hydraulic inputs
The terrain layer is constructed using a 12.5 m resolution DEM and 10 m resolution land cover data in vector format. Geometry for the terrain is defined, including boundary conditions, Manning's roughness coefficient, and unsteady flow data. A 2D flow area is outlined, generating a computational mesh with an initial 80 m cell size to model water movement efficiently.
• Setting of the boundary conditions
The upstream boundary (near Wutancun) receives hydrograph data from 2001 to 2020, while the downstream boundary (at the Xin'an River confluence) uses a 0.01 slope and normal depth conditions. These boundary conditions ensure that the model accurately represents the hydrological behavior of the study area (Figure 3).
• Flood inundation mapping
The model produces flood inundation maps by simulating water flow across the terrain and identifying areas where water depth exceeds a predefined threshold. These maps provide insights into the extent and depth of flooding, which are critical for assessing flood risk and planning evacuation routes. The inundation area is directly related to the evacuation analysis, as it identifies regions that are likely to be affected by flooding and require evacuation.
• Calibration and validation
The model is calibrated for the 2008, 2011, and 2014 flood events and validated using 2017 data. Calibration involved adjusting Manning's roughness coefficient, grid sizes, and computation intervals to ensure the model accurately captures terrain characteristics and hydrodynamic behaviors.
• Land use and land cover (LULC) analysis
Time series of yearly global LULC maps, generated from ESA Sentinel-2 imagery at 10-meter resolution, were processed in QGIS to estimate the influence of land use changes on runoff generation. The analysis revealed a systematic increase in impervious surfaces, which has likely amplified runoff and flood risk over time. These changes were incorporated into the model to improve its accuracy.
• Evacuation analysis
The flood inundation maps were used to identify areas at high risk of flooding and to plan evacuation routes. By correlating the inundation extent with population density and infrastructure data, the model helps prioritize evacuation efforts and allocate resources effectively. These classifications are produced using the Planetary Computer platform, which applies models to annual Sentinel-2 scene collections (Esri, n.d.; Karra et al., n.d.). The land-use categories in the study area include: Residential areas—high-density urban zones with impervious surfaces; Farmlands—Agricultural lands primarily used for citrus, bamboo, and other crops; Forests—dominated by coniferous species such as Cunninghamia lanceolata and Pinus massoniana; Water bodies—Reservoirs and rivers; and Infrastructure—Roads, dikes, and power facilities. These land-use types influence hydrological processes such as infiltration, surface runoff, and evapotranspiration, which are critical for flood modeling. Temporal changes in land use, particularly the expansion of developed areas and the reduction of forested regions, are analyzed using annual land-use maps from 2017 to 2023. This analysis revealed a systematic increase in impervious surfaces, which has likely amplified runoff and flood risk over time.
The project's spatial reference system (SRS) is set for the development of the RAS terrain layer using a projection file from https://spatialreference.org/. Shown in Figure 2 is the upstream boundary (near Wutancun) receives hydrograph data from 2001 to 2020, while the downstream boundary (at the Xin'an River confluence) uses a 0.01 slope and normal depth conditions. The model produces flood inundation maps, providing insights into water flow through the study area. The calibration of the flood model involves adjusting three key parameters: Manning's roughness coefficient, grid sizes, and the computation interval. These adjustments ensure the model performs accurately, capturing terrain characteristics and hydrodynamic behaviors effectively. There is a significant temporal land cover change over time. For instance, the available data from 2017 to 2023 shows evidence of systematic expansion of high intensity developed areas and a proportional shrinking of the forested areas, as illustrated in Figure 4.
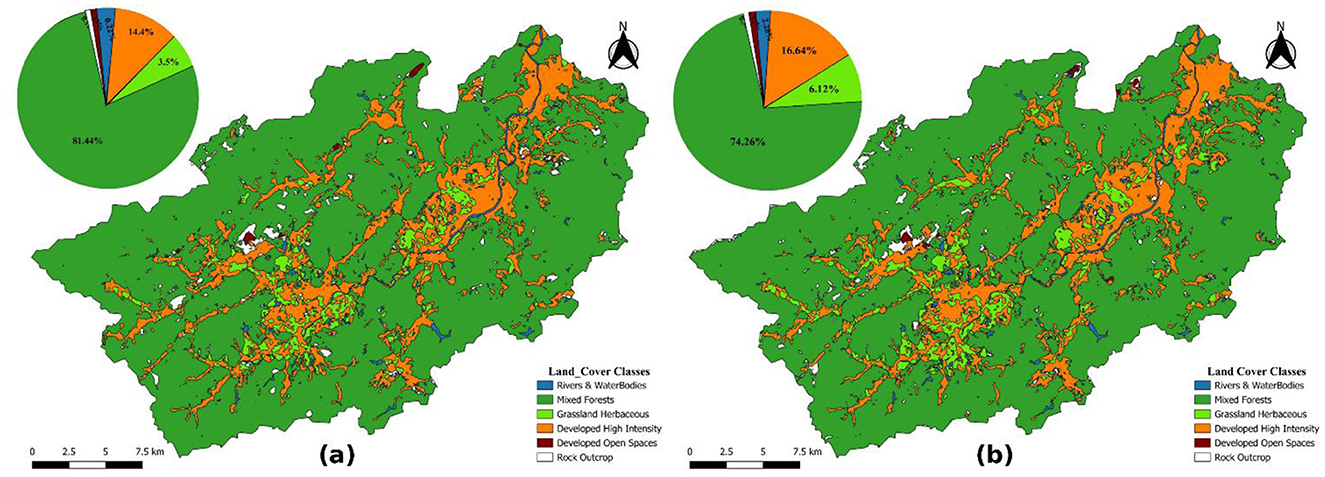
Figure 4. Mean land use and land cover states over the study area for the years 2017 (a) and 2023 (b) based on Sentinel 2 datasets. The spatial characteristics indicates major changes being an increase in the proportion of urban areas, and a decline in mixed forests.
Manning's roughness coefficients (n values) are derived for Cross-Sections and 2D Flow Areas directly within the RAS Mapper (U.S. Army Corps of Engineers, n.d.b, n.d.d). The final n values raster and sub-layers for calibration regions are generated using the Manning's n group layer (Krest Engineers, n.d.).
Base n values, along with overrides, are assigned and linked to the model's geometry. Depending on the most recent type of land use and cover the, the Manning's values applied are as shown in Table 1 (CivilGEO, n.d.; Soliman et al., 2022).
Calibration regions, defined through a polygon layer, are refined during the analysis in between the upper boundary and the lower boundary in order to improve the model's performance and accuracy. In HEC-RAS, visualization is managed through the RAS Mapper (U.S. Army Corps of Engineers, n.d.b). Initial conditions account for moisture, infiltration, and temperature gradients by running the model for 1 h, with a 0.1 wrap-up time (U.S. Army Corps of Engineers, n.d.a). The diffusion wave equation for unsteady flow remains at its default setting. Interval settings for computations, hydrographs, and mapping outputs are configured before starting the simulation to ensure accurate and reliable modeling.
4 Results and discussion
4.1 Peak flows and precipitation
This study involved the analysis of 35 storm events in the Shouchang River Basin, occurring between the year 2001 and 2020, and successfully identified nine major storms that generated peak runoff averaging 1,000 cubic meters per second (cms). Among these, three events—May 2008, July 2002, and July 2010—exhibited single peak flows, while the remaining six displayed multiple peaks (May 2016, June 2017, June 2014, June 2012, June 2011, and April 2012). This distinction in peak behavior maybe be explained by a combination of hydrological, meteorological, and geomorphological factors.
4.1.1 Single-peak events
As an attempt to explain the rainfall-discharge synchronization, the first three graphs in Figure 5, correlate precipitation at different stations (Dakengyuan, Datong, Qudou, Shouchang, Yuankou) with discharge response in different storms. The lag time between peak rainfall and peak discharge varies across storms, indicating hydrological response differences among sub-basins. On the other hand, the rainfall distribution across stations; some stations, like Dakengyuan and Qudou, received significantly higher precipitation amounts during certain storm events. In some cases, the spatial distribution of rainfall shows that higher precipitation at multiple stations leads to a sharper and more synchronized peak in discharge.
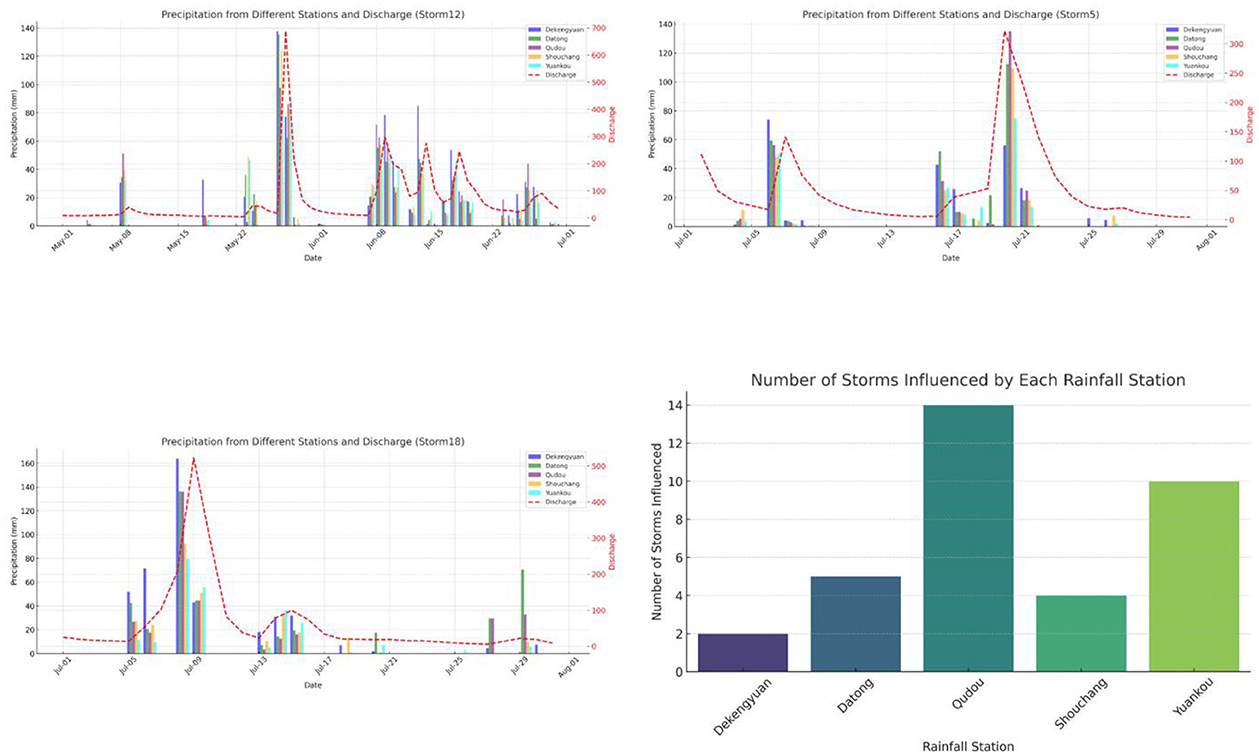
Figure 5. Graphical illustration of the relationship between precipitation at different stations and the resulting discharge across multiple storms. The bar chart indicates the number of storms influenced by each rainfall station.
The bar graph (d) shows that Qudou had the greatest influence on the number of storms, followed by Yuankou. As part of storm-specific responses, Storm 18 indicates a strong peak in discharge closely following the highest precipitation event (July 9), suggesting a quick runoff response. Storm 5 [Graph (b) exhibits a double peak], indicating prolonged and secondary rainfall pulses from multiple sources. Storm 12 (third precipitation-discharge graph) has a high correlation between multiple rainfall pulses and discharge peaks, reinforcing the idea of multiple peak storm events due to staggered rainfall patterns. The analysis on the number of all storms influenced by each gauging station suggests Qudou is the most influential station, affecting the highest number of storms (14), while Dakengyuan has the least impact.
These single peak events are typically associated with short-duration, high-intensity rainfall concentrated over a brief period. Such storms generate rapid surface runoff, particularly in sub-basins with steep slopes, low infiltration capacity, and high imperviousness. The following factors were considered as influential to the single peak storms:
• Concentrated rainfall over short duration
Single peak storms are often driven by intense convective precipitation, where heavy rainfall occurs in a short span, leading to a single, sharp peak in discharge. For example, the May 2008 flood event, which resulted in a single sharp peak exceeding 800 cms, was likely driven by a tropical system or monsoonal burst, causing rapid saturation and overland flow.
• Limited influence from sub-basin lag effects
When precipitation is uniform across the basin without major secondary rainfall pulses, runoff converges quickly to the main river, forming a single dominant flood peak. This contrasts with storms characterized by extended rainfall duration or intermittent bursts, which tend to produce multiple peaks.
• Soil moisture and initial abstraction
Antecedent soil moisture conditions play a critical role in shaping runoff responses. If the soil is already near saturation before the event, runoff generation is immediate, leading to a single dominant peak. This was evident in the July 2002 and July 2010 events, where pre-saturated soils likely amplified the runoff response.
• Hydrological model response
The Peak Rate Factor (PRF) in models like the Clark Unit Hydrograph significantly influences the shape of the hydrograph. Higher PRF values produce sharper hydrographs, indicating a single concentrated discharge peak rather than extended, spread-out flows. In this study, the PRF value of 200 was found to be suitable for the basin, producing a flatter hydrograph that aligns with the observed single peak behavior during intense storms.
• Management implications
Early warning systems should be prioritized in regions where Qudou and Yuankou dominate runoff response. Real-time precipitation monitoring at these key stations would improve flood prediction accuracy. Understanding the cumulative impact of rainfall from multiple stations can aid in reservoir operation adjustments and emergency flood response strategies
4.1.2 Multiple-peak events
In contrast, six events—May 2016, June 2017, June 2014, June 2012, June 2011, and April 2012—exhibited multiple peaks, also averaging around 1,000 cms. These events are characteristic of prolonged or intermittent rainfall, where precipitation occurs over an extended period or in multiple bursts. The outcome is illustrated in Figure 6.
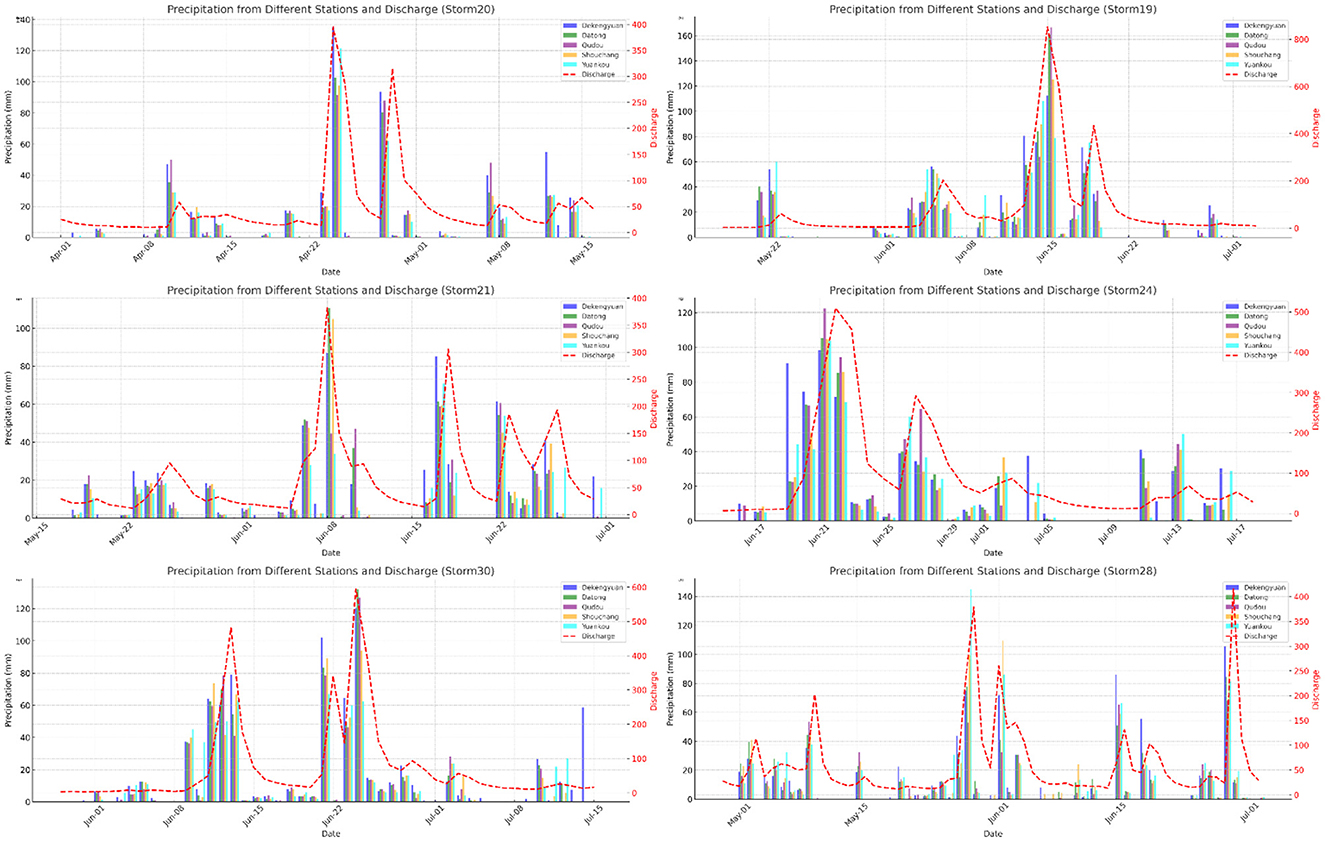
Figure 6. Six multiple-peak events indicating prolonged and intermittent rainfall patterns with distinct hydrological triggers—(May 2016, June 2017, June 2014, June 2012, June 2011, and April 2012).
The following factors were found to be key in influencing the occurrence of the multiple peaked storms:
• Multiple rainfall pulses and synchronicity of sub-basin runoff
Unlike single-peak storms, multiple-peak events are characterized by successive rainfall pulses over an extended period. For example, the June 2017 and June 2014 events show several peaks, reflecting intermittent rainfall bursts rather than a single deluge. The lag times between sub-basins (ranging from 100 to 150 min) mean that flow accumulation does not occur simultaneously, leading to staggered contributions and multiple peaks.
• Variable sub-basin contributions
Sub-basins with shorter lag times (e.g., Subbasin 5) contribute runoff first, while delayed runoff from other sub-basins creates additional peaks. This pattern is evident in the June 2012 and April 2012 hydrographs, which show sequential flow surges, suggesting staggered contributions from different areas.
• Complex terrain and flow routing
Multiple peaks may also result from valley storage effects, where floodwaters temporarily accumulate in low-lying areas before being released gradually. This phenomenon is particularly evident in the June 2011 event, where multiple peaks likely resulted from a combination of direct runoff and delayed storage release from floodplains or reservoirs.
• Variable soil saturation and runoff response
Early rainfall can prime the system, allowing for more efficient runoff generation during subsequent rainfall bursts. For example, in the June 2017 event, the first burst of rain likely saturated the soil, enabling higher runoff volumes during later rainfall, which reinforced the multiple peaks.
• Human and structural influences
Urbanization and artificial storage structures (e.g., reservoir releases or flood control operations) may modulate peak flows, leading to secondary peaks. The hydrographs for June 2014 and June 2012 show signs of flow regulation, where peaks might have been influenced by controlled discharges upstream.
• Hydrological implications
The distinction between single and multiple peak events has significant implications for flood risk management. Single peak events, driven by short-duration, high-intensity storms, require rapid response mechanisms to mitigate flash flood risks, particularly in steep and impervious sub-basins. In contrast, multiple peak events, associated with prolonged rainfall, necessitate strategies to manage cumulative runoff and reduce the risk of sustained flooding in downstream areas.
The observed patterns also highlight the importance of antecedent soil moisture conditions in shaping hydrological responses. Wetter soils, resulting from prior rainfall, reduce infiltration capacity, and increase runoff generation during subsequent storms, leading to higher and more frequent peaks. This is evident in the June 2014 and June 2017 events, where sustained rainfall led to multiple peaks despite moderate individual rainfall intensities.
4.2 Modeling and predictive insights
The hydrological modeling results further support these observations. The AutoReg(2) model, while demonstrating strong predictive capability, shows some systematic errors in predicting peak flows, particularly during multiple peak events. This may be attributed to variations in soil moisture and rainfall characteristics, which influence the timing and magnitude of runoff. For instance, the model accurately predicts the timing of single peak events but may underestimate the cumulative effects of prolonged rainfall, as seen in the June 2014 and June 2017 events in Figure 7. These findings emphasize the need for improved model calibration to account for antecedent conditions and rainfall duration, particularly in basins prone to both flash and cumulative flooding.
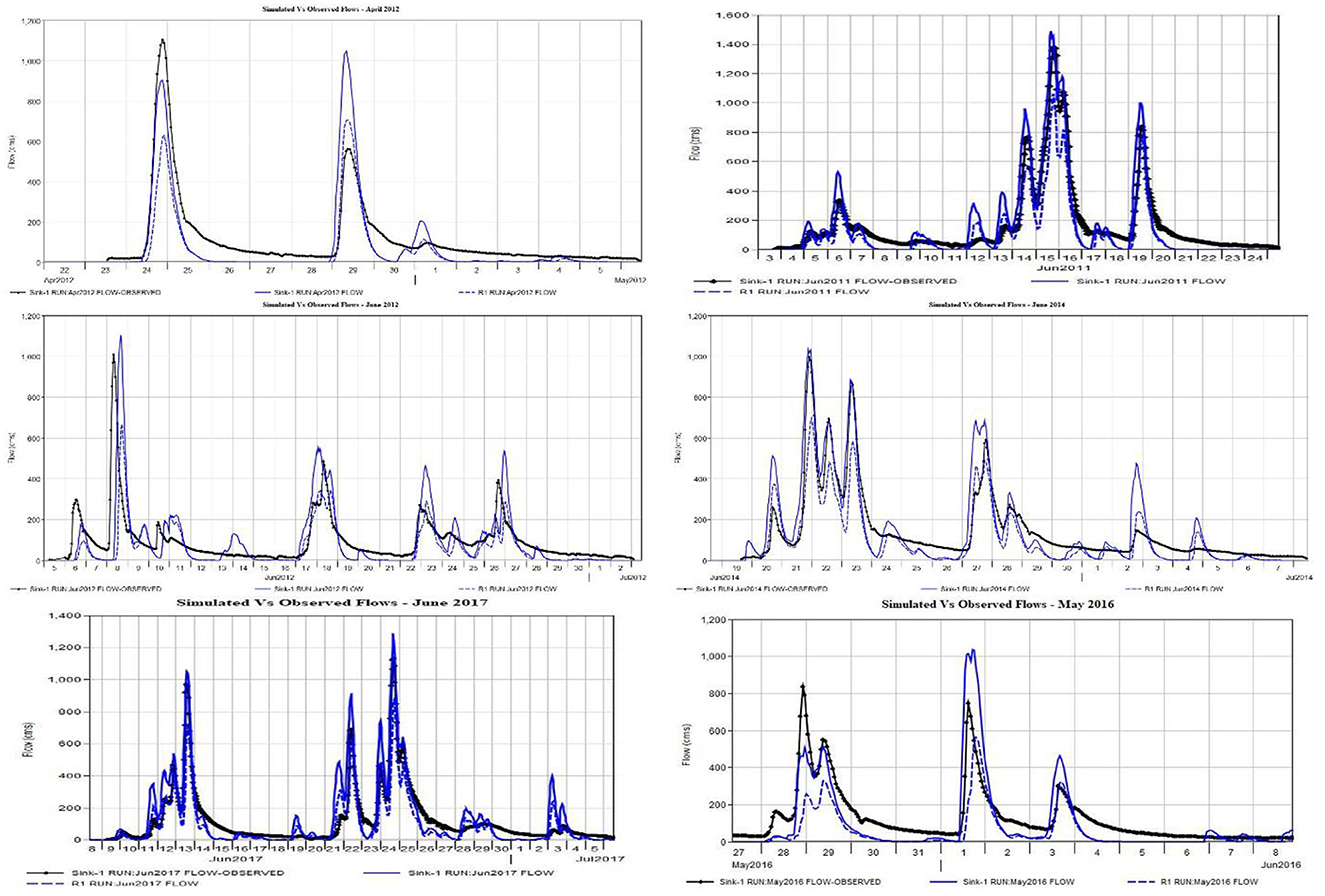
Figure 7. Observed vs. predicted discharges for multiple-peak events indicating prolonged or intermittent rainfall patterns with distinct hydrological triggers: (May 2016, June 2017, June 2014, June 2012, June 2011, April 2012).
The results show that the simulated flows (both Sink1 and R1) often underestimated the observed flows, especially during peak events. This is consistent with the finding that the mean observed flow is generally higher than the simulated flow from both models. This observation reinforces the need for further model calibration, particularly to improve the accuracy of mean flow predictions.
The simulation shows significant discrepancies during peak flow events, with Sink1 sometimes overestimating and R1 consistently underestimating the observed peaks. For example, in the June 2001 storm, the observed peak was 331 m3/s, while Sink1 simulated 223.88 m3/s and R1 simulated 78.82 m3/s.
The finding that Sink1 tends to overestimate peak flows in some cases while R1 underestimates them is consistent in highlighting the importance of improving peak flow simulations, especially for flood forecasting and management.
Figures 7, 8 indicate that both models generally followed the observed flow trends, but with varying degrees of accuracy. R1, despite underestimating peaks, often followed the overall trend more closely. The correlation analysis (e.g., 0.973 for Sink1 and 0.976 for R1 in May 2004) supports the idea that while R1 may underestimate peaks, it tracks the overall flow pattern better. This is useful for understanding model performance and identifying areas for improvement. For example, the June 2001 storm was a single-peaked event, while the June 2003 storm had multiple peaks. The observation that multiple-peaked storms show greater variations in simulated vs. observed peaks suggests that models may struggle more with complex hydrographs, which is critical for flood forecasting in regions prone to multi-peak storms. The lag time analysis across five sub-basins in the Shouchang River Basin reveals variations in hydrological responses to rainfall, with lag times ranging from 100 to 150 min. Subbasin 1, with the longest lag time, 150 min, indicates slower runoff due to gentler slopes or permeable soils, while Subbasin 5, with the shortest lag time, 100 min, suggests steeper slopes and quicker runoff, increasing the risk of flash floods. Synchronization of peak flows from multiple sub-basins poses a critical risk for downstream flooding in events heavy rainfall across the sub-basins.
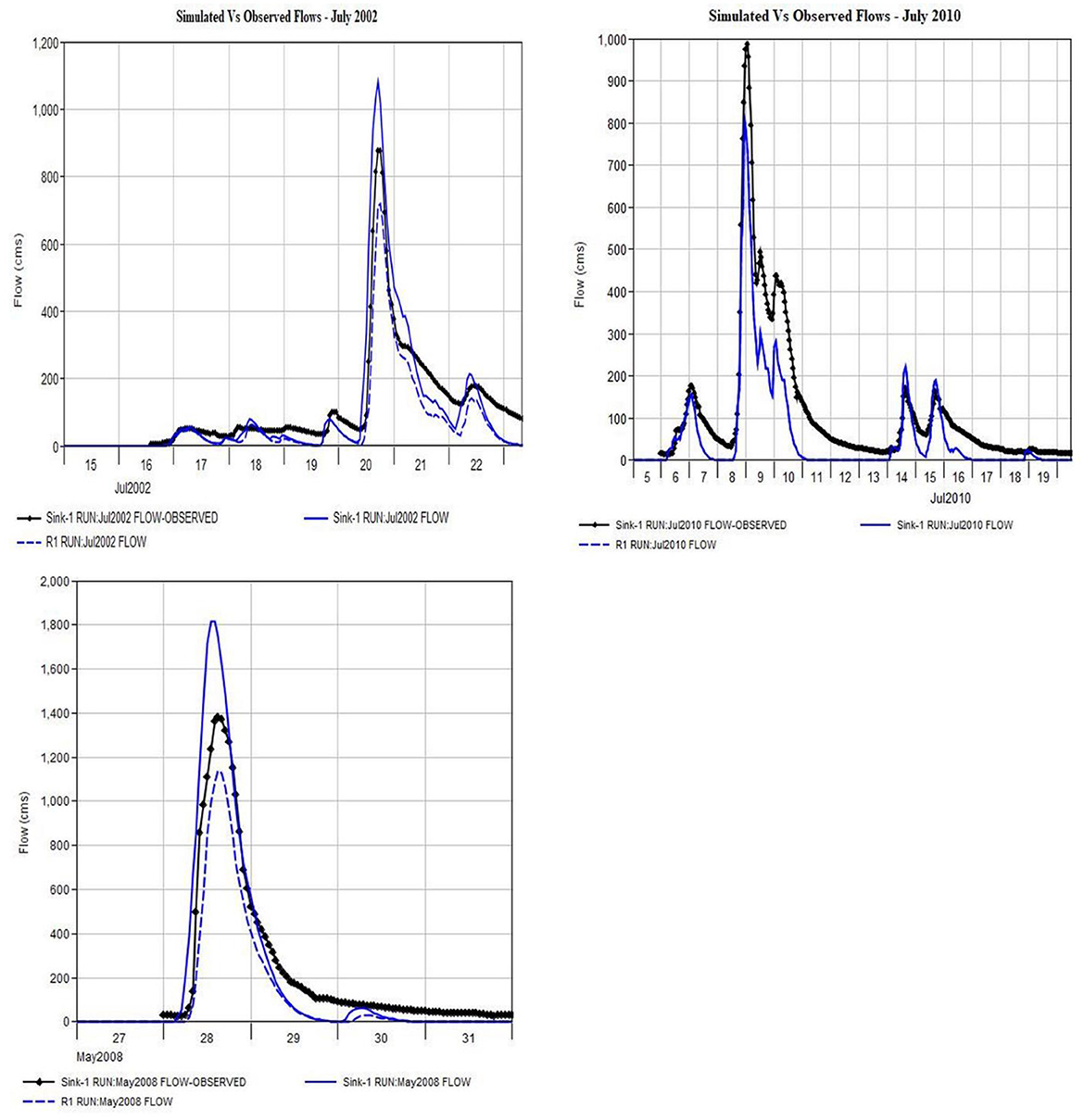
Figure 8. Observed vs. predicted discharges for single peak storms indicating short-duration, high-intensity rainfall events over a single dominant runoff generation zone.
In this study, peak rate factor (PRF) is applied in the Clark Unit Hydrograph and SCS (Soil Conservation Service) Unit Hydrograph methods to simulate runoff behavior. The PRF significantly influences runoff generation in HEC-HMS by affecting the shape and magnitude of the unit hydrograph, which directly impacts the timing and intensity of the runoff. A higher PRF reduces the time to peak and increases the peak discharge, resulting in more concentrated runoff in a shorter period. Based on Equation 3, a PRF value of 200 creates a relatively flatter hydrograph, which spreads runoff over a longer duration, resulting in lower peak discharge and thus making it most suitable for our catchment area (Equation 8).
Where Qp is peak runoff rate (cfs or m3/s), A is Drainage area (mi2 or km2), Tp is time to peak (hours), and PRF as Peak rate factor (unitless, typically 484 for standard SCS).
The hydrological plots reveal a strong correlation between high precipitation events and rapid flow peaks, indicating limited infiltration and fast surface runoff. Notable flood events, such as the May 2008 flood, demonstrate peak flows exceeding 800 cms at the confluence of Reach 1 and Reach 2, likely driven by steep terrain and intense rainfall or saturated soils within the upstream sub-basins. Similarly, June 2012 and June 2017 events show multiple peaks, suggesting consecutive rainstorms or repeated rainfall, increasing the likelihood of flash floods or back-to-back flooding events. This pattern highlights the subbasin's susceptibility to rapid onset flooding disasters, requiring timely early warning and effective pre-disaster actions.
Subbasin 5, situated at the lowest boundary of the watershed, acts as a flow accumulation point for upstream subbasins, particularly during heavy rainfall. High runoff in this area results from contributions from the rest of the sub-basins, 1–4. Key junction points or outlets converge within Subbasin 5, making it essential for flood management, especially to protect downstream communities like Shouchang Town, which is vulnerable to flooding, as illustrated in Figure 9. Rainfall events in April and June 2012 show sharp peaks followed by rapid recession, indicating a flashy hydrological response likely due to impervious surfaces or steep slopes. In contrast, prolonged events like those in June 2014 and June 2017 exhibit smaller but multiple peaks. While individual peaks are moderate, the cumulative effect of sustained rainfall can saturate soils, increasing the potential for flooding.
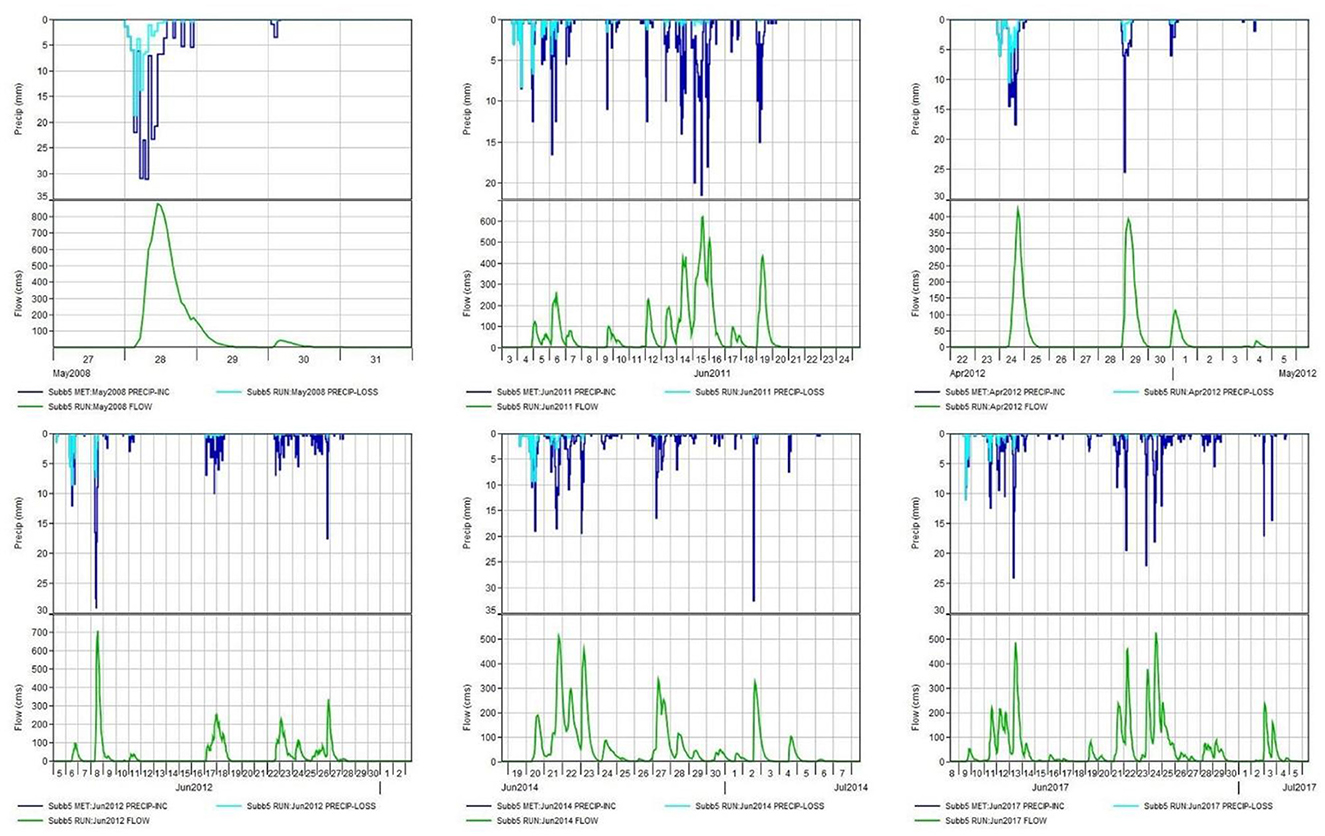
Figure 9. Response relationship between flow peaks and precipitation events for simulations of June 2012, June 2014, and June 2017.
The AutoReg(2) model demonstrates strong predictive capability with significant coefficients (p < 0.001), but moderate fit issues: an AIC of 4430.14 and RMSE of 79.54. A negative correlation coefficient (−0.586) and a bias of 76.51 indicate some systematic errors in predictions. It is noted that with the current setup, the model accurately predicts the timing and magnitude of runoff flows. However, variations in peak discharge across years reflect changes in soil moisture, with wetter soils producing higher runoff. Rainfall characteristics also influence flow patterns, where short, intense storms generate sharp peaks, while sustained rainfall leads to moderate but prolonged runoff. The rainfall data recorded at Gauge 5 is essential for feeding flow inputs into the HEC-RAS model, which is used for flood inundation mapping.
4.3 Principal component analysis (PCA) of storm-discharge relationships
The PCA Analysis and Station Influence on Storms data provide additional insights into the hydrological behavior of the Shouchang River Basin, particularly regarding the relationships between storm events, sub-basin characteristics, and discharge patterns. This analysis reveals that Principal Component 1 (PC1) accounts for 92.08% of the variance, while Principal Component 2 (PC2) explains 4.36% of the variance, as illustrated in Figure 10. This indicates that the majority of the variability in storm-discharge relationships is driven by a number of factors associated with PC1, which likely represents dominant hydrological processes such as basin characteristics etc.
• Dominance of PC1
The high variance explained by PC1 suggests that the primary drivers of peak discharge in the Shouchang River Basin are consistent across most storm events. These drivers may include: Rainfall intensity and duration: Short-duration, high-intensity storms (single peak events) and prolonged rainfall (multiple peak events) are likely key contributors to PC1; Sub-basin characteristics: Factors such as slope, soil permeability, and land use (e.g., impervious surfaces) significantly influence runoff generation and are likely captured by PC1; Antecedent soil moisture: Pre-storm soil conditions play a critical role in shaping runoff responses, particularly during multiple peak events.
• Secondary influence of PC2
The smaller variance explained by PC2 suggests secondary factors that modulate storm-discharge relationships. These may include: Spatial variability in rainfall distribution: Differences in rainfall patterns across sub-basins can lead to variations in runoff timing and magnitude; Flow routing and storage effects: Valley storage, floodplain interactions, and reservoir operations may be introducing additional complexity, particularly during multiple peak events.
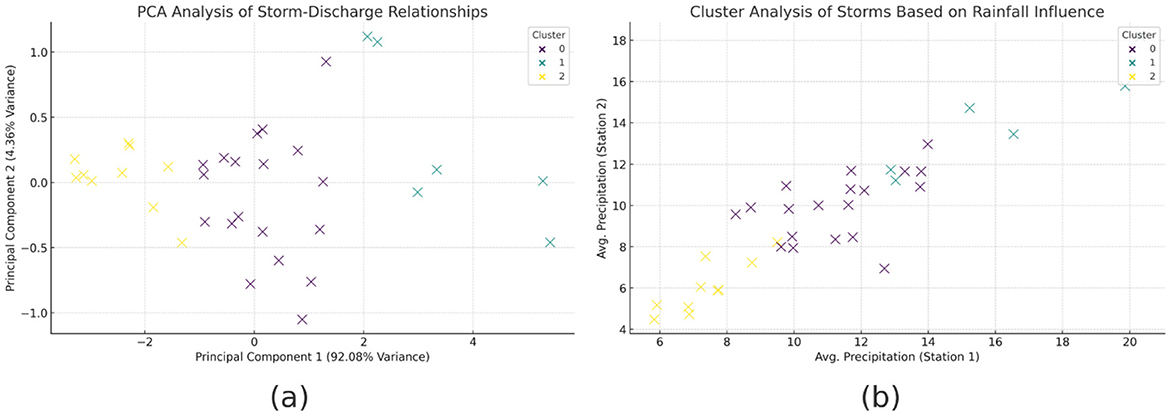
Figure 10. The principal component analysis (PCA) plot (a) and cluster analysis scatter plot (b) illustrating significant insights on the storm-discharge relationships and rainfall influence across different storm events.
4.4 Station influence on storms
The Station Influence on Storms data highlights the role of specific gauging stations in influencing storm events at Shouchang Town, providing further insights into the spatial variability of hydrological responses across the basin.
Qudou station: This station influenced the highest number of storms (14 out of 35), indicating its central role in the basin's hydrological dynamics. The storms influenced by Qudou are majorly associated with prolonged or intermittent rainfall events, given the station's location in a sub-basin with moderate lag times and variable runoff contributions.
Yuankou station: Influencing 10 storms, Yuankou is another critical station, particularly for events characterized by multiple peaks. The station's influence may reflect its position in a sub-basin with complex terrain and flow routing, where valley storage effects and delayed runoff contribute to multiple peaks.
Datong station: Influencing five storms, Datong is associated with both single and multiple peak events. The station's influence may be linked to its sub-basin's steep slopes and rapid runoff response, particularly during high-intensity storms.
Shouchang and Dekengyuan stations: These stations influenced very few storms, suggesting their sub-basins play a more localized role in the basin's hydrological behavior. Shouchang, located at the basin's outlet, is critical for downstream flood management, particularly during events with synchronized peak flows from upstream sub-basins.
• Hydrologic model calibration
Upon calibration for 2008, 2011, and 2014 floods the model is verified with the event data of 2017, demonstrating excellent predictive power with an overall volume accounting percent error of 0.4399% between simulated and observed discharges. Simulations for May 28–31, 2008, are run using various computation intervals.
4.5 Flood inundation area and depth analysis
The catchment area spans 690 km2, with flooding affecting ~28 villages in Shouchang towns, situated immediately downstream of the confluence of the Shouchang and Xin'an rivers. In the entire area, 54.4% of the affected area consists of urban land, with minimal agricultural use. Simulations reveal that Ximen and Datangbian villages are the worst-hit area, exacerbated by backwater effects. Floodwaters gradually breach levees and walls, advancing through floodplains and low-lying areas, as illustrated in the Figure 11.
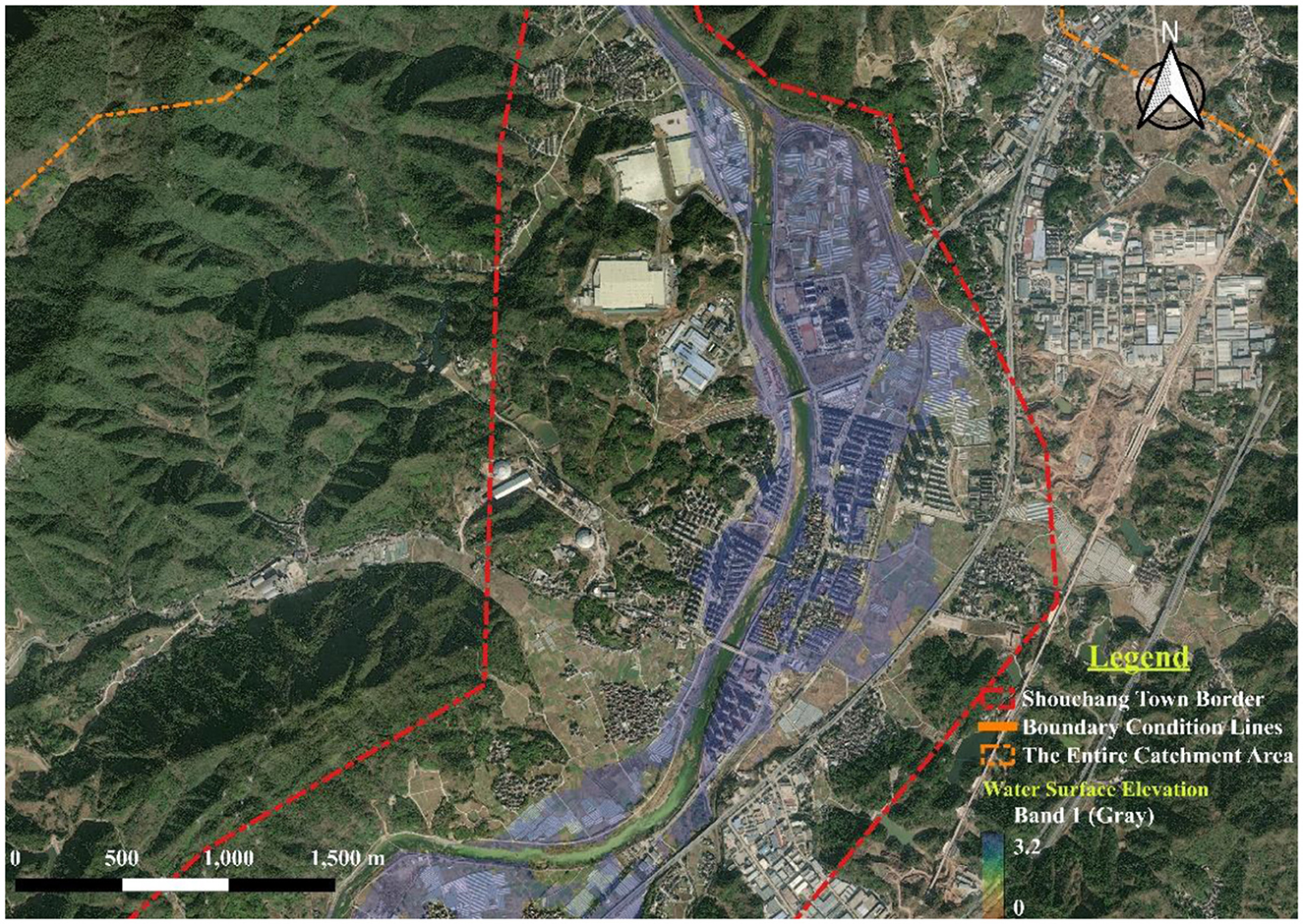
Figure 11. Water surface elevations showing the inundated zones at Shouchang area along the Shouchang River for the May 2008 flood event.
Using HEC-RAS 2D, depth maps are generated with the inundation levels categorized into high (>2 m), medium (1–2 m), and low (0–1 m) risk areas, as well as discharge variation as illustrated in Table 2. The classification is necessary to identify flood-prone regions, affected communication route—roads and rails and the affected rescue centers in the study area. The maps, displayed in RAS Mapper, align with reported flood data, with the maximum inundation depth occurring on May 28, the day after the peak discharge as shown in Figure 12. The RAS Mapper enhances 2D flow analysis by animating water surface elevation, velocity, and depth providing a clear visualization of flood dynamics. Typical flow rates within the channel were about 2 m/s, with some regions of remarkable reduction in channel width reaching 5 m/s (Figure 13).
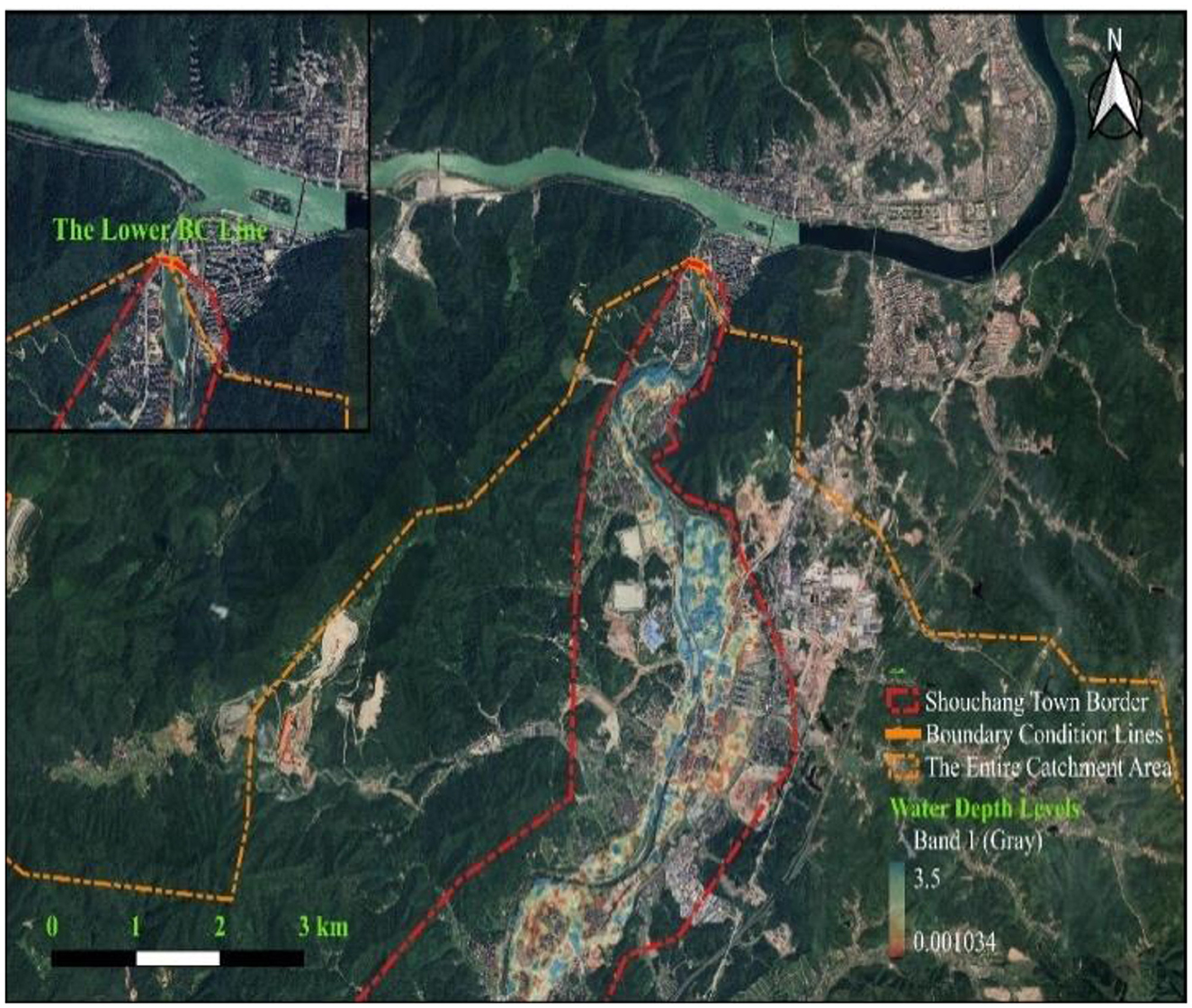
Figure 12. Flood depth extent around Shouchang Town in May 2008 relative to the lower boundary line.
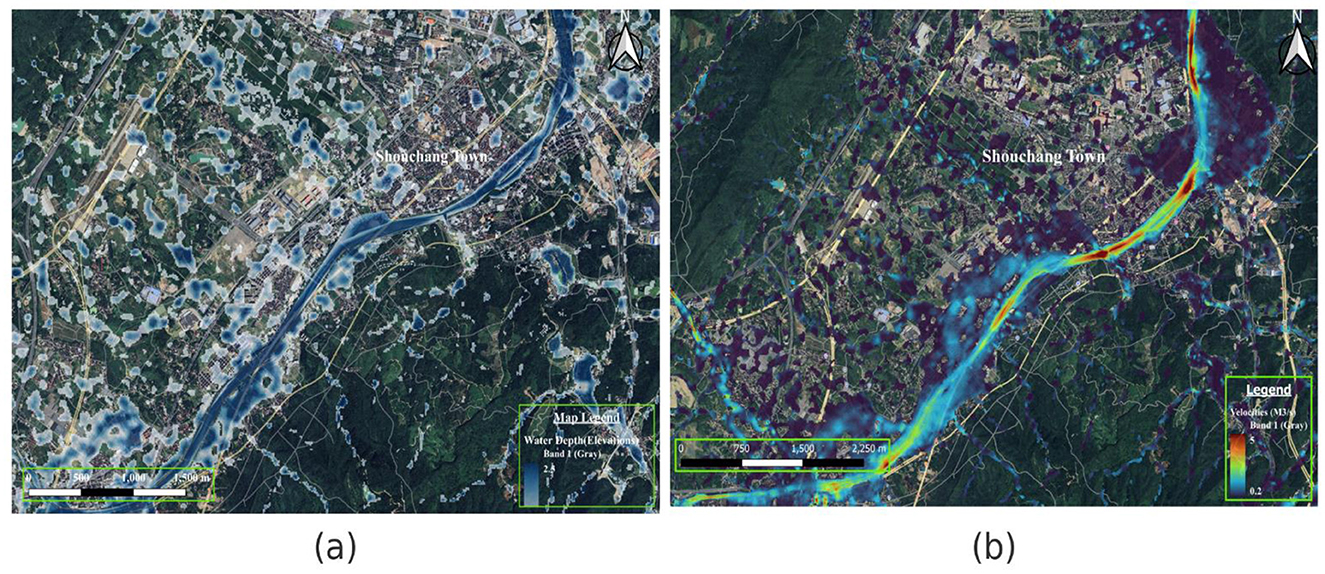
Figure 13. Flood extent showing the inundated areas as at 28th May 2008 over Shouchang Town (a), together with the simulated flow velocities (b) along the Shouchang River.
Calibration results show that flow levels change with varying discharge values, with maximum velocity and depth aligning with peak flooding. Simulation performance is also influenced by the compute interval and warmup time settings in HEC-RAS. It's ensured that initial conditions approximate steady-state or near-steady-state behavior to reduce warmup need to 8 h. This visualization capability improves understanding of the model performance flood behavior over time.
On examining how variations in upstream boundary conditions and initial channel conditions affect computational mesh performance, simulations using three different mesh sizes reveal no major variations due to the inclusion of break-lines and refinement regions along the channel. However, changing the initial conditions influences the time required to breach break-lines, reflecting differences in cell sizes across regions. This suggests that localized mesh adjustments impact simulation outcomes, even when overall discharge values remain constant.
4.6 Population evacuation transfer analysis
A good flood risk mapping project should integrate population distribution, road characteristics, terrain, and existing public infrastructure to optimize evacuation routes and resettlement sites. Based on local plans for Shouchang Town, a total of 28 villages are situated within exposure areas of sub-basin 5. Out of these 28 villages only 22 rescue centers were found to be unaffected and thus effective for sheltering flood victims. Four rescue centers would need relocation to higher grounds, including adding new resettlement sites and modifying transfer plans. These include Yongjiaqiao, Henanli, Ximen, and Datanbian Centers where current arrangements prove susceptible to flood events of similar threshold.
4.7 Analysis of evacuation scope and target population
The downstream community neighborhoods of the Shouchang brook includes the villages shown in Figure 14 alongside the indicated communities rescue centers. An approximate population of 48,000 residents, with the Dongchang, Xihu, and Wangjiang communities constituting the highest population and making up the core of the local administrative structure of Shouchang (Stevens et al., 2015; Nieves et al., 2020), is exposed to floods caused by this river.
In the event of a heavy down pour equivalent to that of May 2008, under design flood conditions, the flash floods would result in a peak flow rate of ~1,400 m3/s. The floodwaters' powerful force would pose significant threats to infrastructure damage, loss of life and property and disruption of activities near the inundated areas. As a result, evacuation measures must be implemented for the affected administrative villages of Shouchang and its outskirts, under the unified coordination of the Hangzhou Municipal Government Flood Control and Drought Relief Command.
By overlaying risk maps with layers representing residential settlements and administrative villages, the evacuation scope and targets are identified. Figure 15, illustrating the maximum inundation area, shows that the inundation boundaries intersect with majority of the administrative villages and easily shows low risk areas with the potential of relocation as rescue centers. Thus, the evacuation scope includes all residents within the inundation zones of these administrative villages. In the case of the Pujiapeng community rescue center, the proximity from flood pathway is safe, however, the access routes to this center are not adequate. This would pose a significant challenge to a rescue operation since the terrain is also hilly.
4.8 Analysis of existing resettlement points and their transfer routes
Based on the progression of extreme flood events, inundation areas, water depth, and the distribution of evacuees, resettlement points, and transfer routes, evacuation routes and timing are determined. The evacuation routes are developed by analyzing transfer targets, resettlement points, and field investigations to identify potential danger points along the routes that may threaten the safety of evacuees. While most of these routes maybe assumed as safe, a number of them may pose danger to rescue operations. For instance, the Shili Shouchngjiang Ecology Leisure Greenway, illustrated in Figure 15, would pose challenges to rescuers since it would be substantively submerged near the Bujiapeng South Street. Alternative routes connecting to 320 National Road, such as Xifeng and Dong Gui Roads would be safer to use.
An emergency transfer plan is established by considering the current traffic conditions, community distribution, and resettlement points in the affected areas. For highly urbanized areas with flat terrain, where most residents live in multi-story buildings, on-site flood sheltering is feasible. In rural flood-protected areas, as in the case of Bujianpeng, Nanpucun, and Wushicun, where buildings are primarily low-rise, evacuation to higher ground away from floodwaters is recommended.
According to the current evacuation plans for Shouchang Town, most of the designated resettlement points are located within or on the edge of the Shouchang Brook (as shown in Figure 16). The existing resettlement points as compared to the number of affected residents for each administrative region are not adequate. Therefore, the current transfer and resettlement plans cannot be adequately implemented without minor adjustments.
The statistical analysis indicates that in the event of a record storm flood in the Shouchang river, a total of 12,524 people would require evacuation into Hengshan, Wangjiang, Dongmen, Chengbei, Henanli, and Shibaqiao Centers. However, these existing resettlement points provided by the Office of Shouchang Flood Control, Taiwan Prevention and Drought Relief Headquarters would only comfortably accommodate 4,350 people, leaving approximately over 8,174 people without resettlement options. This highlights that the capacity of the current resettlement points is significantly insufficient, necessitating the planning of additional resettlement points and the development of an additional emergency response plan.
4.9 Limitations and considerations for evacuation scope
While the evacuation scope was determined based on flood inundation maps, population distribution, and proximity to rescue centers, certain vulnerability factors such as age, health status, and mobility constraints were not explicitly incorporated into the analysis due to the unavailability of detailed demographic and health data. These factors are critical for identifying populations that may require additional assistance during evacuations, such as the elderly, disabled, or those with chronic illnesses. In the absence of such data, the current analysis assumes a uniform vulnerability across the population. However, it is strongly recommended that future studies and evacuation plans incorporate detailed demographic and health data to better address the needs of vulnerable groups. This would enable the development of more targeted evacuation strategies, such as prioritizing the evacuation of high-risk individuals, providing specialized transportation for those with mobility challenges, and ensuring that rescue centers are equipped to handle medical emergencies.
5 Summary and conclusions
The PCA and station influence data provide valuable insights for flood risk management in the Shouchang River. Stations like Qudou and Yuankou, which influence a large number of storms, should be prioritized for real-time monitoring and early warning systems. Understanding the spatial variability of rainfall and runoff contributions can help improve flood forecasting accuracy. The dominance of PC1 in the PCA analysis suggests that hydrological models should focus on accurately representing rainfall intensity, sub-basin characteristics, and antecedent soil moisture. The secondary influence of PC2 highlights the need to incorporate spatial variability and flow routing effects into model calibration, particularly for multiple peak events.
For flood mitigation strategies, sub-basins with steep slopes and rapid runoff responses (e.g., Datong) require measures to mitigate flash flood risks, such as improved land-use planning and floodplain management. Sub-basins with complex terrain and delayed runoff (e.g., Yuankou) may benefit from strategies to manage cumulative flooding, such as reservoir operations and valley storage enhancements.
The Curve Number (CN) analysis highlights variability in runoff potential, with CN values ranging from 72 to 77. Subbasins with higher CN values, such as Subbasin 5, exhibit greater runoff potential and faster peak flow responses. In contrast, Subbasin 1, with a lower CN, shows higher infiltration capacity and slower runoff. The impervious area percentages further influence runoff, with Subbasin 4 exhibiting the highest impervious cover (19.2%), increasing flood risks. The initial abstraction value of 20 mm, consistent across all sub-basins, indicates uniform rainfall absorption before runoff occurs, with higher CN sub-basins generating more runoff during intense rain events. Effective coordination of flow management is essential to mitigate peak flow synchronization and reduce flood risks as well as for computation of the lag times as shown in Table 3.
The integration of HEC-RAS and HEC-HMS generates precise flood forecasts, offering insights into flood extent, depths, velocities, and durations. It applies hydrodynamic modeling to assess flood risks within the Shouchang River Basin, combining hydraulic data with satellite imagery for accurate predictions. While existing levees near the Xin'an River confluence are generally sufficient, further improvements are recommended.
The research provides several important insights into flood inundation modeling and flood risk management. The choice of appropriate computational parameters to balance simulation accuracy and processing time and parameters influencing the HEC-RAS simulations such as grid spacing, computation intervals, and the use of break-lines and refinement regions around levees, is therefore very important for localized flood modeling.
Based on the flood risk maps and inundation analysis, four rescue centers (Yongjiaqiao, Henanli, Ximen, and Datangbian) should be relocated to higher grounds. This can be achieved by identifying safe zones outside the flood-prone areas, such as the point locations marked in yellow in Figure 17, and constructing new facilities or retrofitting existing structures to serve as evacuation centers. Relocating these centers will reduce the risk of flood damage to evacuation infrastructure, ensure safer and more accessible routes for residents during emergencies, and improve the overall efficiency of rescue operations. This will ultimately save lives and reduce property damage during future flood events.
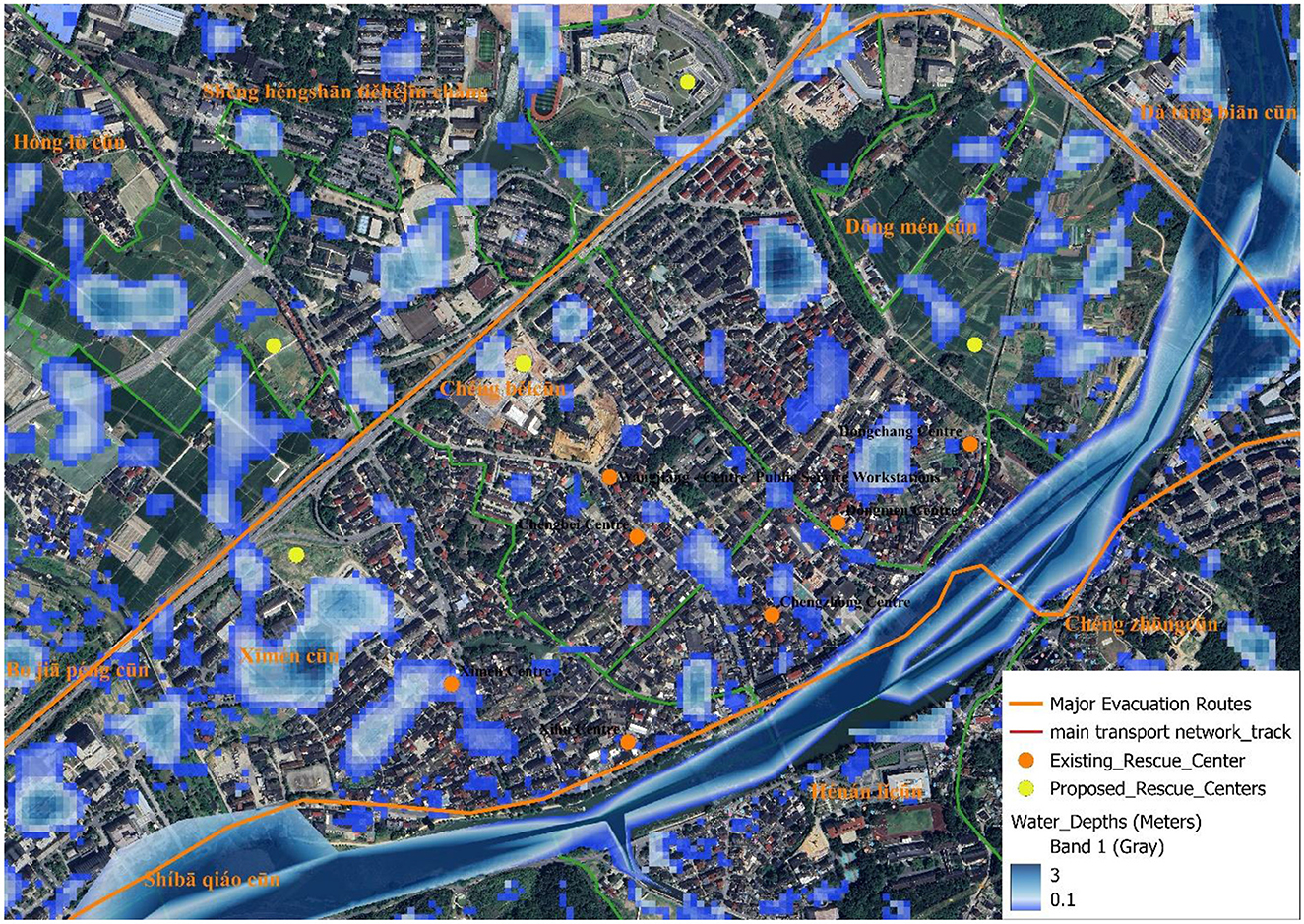
Figure 17. Map of Shouchang Town showing existing rescue centers (orange) and already proposed safer locations (yellow) for safe evacuation and transfer of flood victims.
It is also important that local authorities develop alternative evacuation routes, such as Xifeng and Dong Gui Roads, which are less likely to be submerged during floods. Enhance these routes with better signage, lighting, and emergency communication systems to facilitate smoother evacuations. Such improvement on evacuation routes will ensure faster and safer movement of residents to resettlement points, reducing the risk of casualties and minimizing disruptions to rescue operations.
Finally, this study illustrates that although levees can play a crucial role in shaping flood inundation patterns and determining the areas affected by flooding, they may not be adequate. This observation amplifies the importance of in cooperating such infrastructure with early warnings in future modeling efforts to better simulate real-world flood behavior. This is particularly critical for flood vulnerable communities near Shouchang and the town outskirts. The south-east zone, including the neighborhoods around Henanli, Shibaqio and Datangbian, is shown to be especially prone to inundation during major flood events. These areas require special attention, and priority should be given to flood rescue operations and infrastructure improvements to prevent loss of life and reduce property damage during future floods.
Data availability statement
The raw data supporting the conclusions of this article will be made available by the authors, without undue reservation.
Author contributions
WM: Conceptualization, Data curation, Formal analysis, Methodology, Software, Writing – original draft. WJ: Resources, Supervision, Writing – review & editing. AM: Investigation, Methodology, Writing – review & editing. RK: Formal analysis, Visualization, Writing – original draft.
Funding
The author(s) declare that no financial support was received for the research and/or publication of this article.
Conflict of interest
The authors declare that the research was conducted in the absence of any commercial or financial relationships that could be construed as a potential conflict of interest.
Generative AI statement
The author(s) declare that no Generative AI was used in the creation of this manuscript.
Publisher's note
All claims expressed in this article are solely those of the authors and do not necessarily represent those of their affiliated organizations, or those of the publisher, the editors and the reviewers. Any product that may be evaluated in this article, or claim that may be made by its manufacturer, is not guaranteed or endorsed by the publisher.
References
Awah, L. S., Nyam, Y. S., Belle, J. A., and Orimoloye, I. R. (2024). Understanding drivers of changing flood dynamics for enhancing coastal community resilience: a participatory approach. Reg. Environ. Change 24:141. doi: 10.1007/s10113-024-02276-7
Boudreau, D., McDaniel, M., Sprout, E., and Turgeon, A. (n.d.) Flood. National Geographic Society. Available online at: https://education.nationalgeographic.org/ (accessed December 26, 2024).
CivilGEO (n.d.). Create and assign land cover data as Manning's roughness. Available online at: https://knowledge.civilgeo.com/create-and-assign-land-cover-data-as-mannings-roughness/ (accessed March 27, 2025).
Devitt, L., Neal, J., Coxon, G., Savage, J., and Wagener, T. (2023). Flood hazard potential reveals global floodplain settlement patterns. Nat. Commun. 14:2801. doi: 10.1038/s41467-023-38297-9
Dharmarathne, G., Waduge, A. O., Bogahawaththa, M., Rathnayake, U., and Meddage, D. P. P. (2024). Adapting cities to the surge: a comprehensive review of climate-induced urban flooding. Res. Eng. 22:102123. doi: 10.1016/j.rineng.2024.102123
Ding, W., Wu, J., Tang, R., Chen, X., and Xu, Y. (2022). A review of flood risk in China during 1950–2019: urbanization, socioeconomic impact trends and flood risk management. Water 14:3246. doi: 10.3390/w14203246
Du, J., Cheng, L., Zhang, Q., Yang, Y., and Xu, W. (2019). Different flooding behaviors due to varied urbanization levels within river basin: a case study from the Xiang River Basin, China. Int. J. Dis. Risk Sci. 10, 89–102. doi: 10.1007/s13753-018-0195-4
Esri (n.d.) Impact Observatory | Sentinel-2 10m Land Cover Time Series of the World from 2017-2021. Available online at: https://www.esri.com/en-us/home (accessed December 18 2024).
Islam, Md. Z., and Wang, C. (2024). Cost of high-level flooding as a consequence of climate change driver?: A case study of China's flood-prone regions. Ecol. Indic. 160:111944. doi: 10.1016/j.ecolind.2024.111944
Jiang, Y., Zevenbergen, C., and Ma, Y. (2018). Urban pluvial flooding and stormwater management: a contemporary review of China's challenges and ‘sponge cities' strategy. Environ. Sci. Policy 80, 132–143. doi: 10.1016/j.envsci.2017.11.016
Jonkman, S. N., Curran, A., and Bouwer, L. M. (2024). Floods have become less deadly: an analysis of global flood fatalities 1975–2022. Nat. Hazards 120, 6327–6342. doi: 10.1007/s11069-024-06444-0
Karra, K., Kontgis, C., Statman-Weil, Z., Mazzariello, J.C., Mathis, M., and Brumby, S.P. (2021). Global Land Use/Land Cover with Sentinel 2 and Deep Learning. Manhattan, NY: IEEE, 4704–4707.
Kioko, S., and Ouya, D. (n.d.). The importance of hydrological services and forecasting for decision-making in Eastern Africa. Nairobi: ICPAC. Available online at: https://www.icpac.net/news/the-importance-of-hydrological-services-and-forecasting-for-decision-making/ (accessed October 22, 2024).
Krest Engineers. (n.d.). Hydrology and hydraulics (H&H). Available online at: https://krestengineers.com/services/hydrology-and-hydraulics/ (accessed June 7, 2024).
Kumar, V., Sharma, K., Caloiero, T., Mehta, D., and Singh, K. (2023). Comprehensive overview of flood modeling approaches: a review of recent advances. Hydrology 10:141. doi: 10.3390/hydrology10070141
Liang, C., Lu, W., and Chen, Z. (2022). Flooding emergency plan: Yangtze River Delta, China. Department of Flood and Drought Disaster Prevention, GPH-GU 5210 – Global Health Disaster Preparedness and Response. New York, NY: NYU College of Global Public Health.
Ma, Y., Cui, Y., Tan, H., and Wang, H. (2022). Case study: diagnosing China's prevailing urban flooding—causes, challenges, and solutions. J. Flood Risk Manag. 15:e12822. doi: 10.1111/jfr3.12822
Mai, T., Mushtaq, S., Reardon-Smith, K., Webb, P., Stone, R., Kath, J., et al. (2020). Defining flood risk management strategies: a systems approach. Int. J. Dis. Risk Reduct. 47:101550. doi: 10.1016/j.ijdrr.2020.101550
Merz, B., Kuhlicke, C., Kunz, M., Pittore, M., Babeyko, A., Bresch, D. N., et al. (2020). Impact forecasting to support emergency management of natural hazards. Rev. Geophys. 58:e2020RG000704. doi: 10.1029/2020RG000704
Miller, J. D., and Hutchins, M. (2017). The impacts of urbanisation and climate change on urban flooding and urban water quality: a review of the evidence concerning the United Kingdom. J. Hydrol. Reg. Stud. 12, 345–362. doi: 10.1016/j.ejrh.2017.06.006
Nieves, J. J., Sorichetta, A., Linard, C., Bondarenko, M., Steele, J. E., Stevens, F. R., et al. (2020). Annually modelling built-settlements between remotely-sensed observations using relative changes in subnational populations and lights at night. Comput. Environ. Urban Syst. 80:101444. doi: 10.1016/j.compenvurbsys.2019.101444
Ongdas, N., Akiyanova, F., Karakulov, Y., Muratbayeva, A., and Zinabdin, N. (2020). Application of HEC-RAS (2D) for flood hazard maps generation for Yesil (Ishim) River in Kazakhstan. Water 12:2672. doi: 10.3390/w12102672
Rentschler, J., Salhab, M., and Jafino, B. A. (n.d.) Flood Risk Already Affects 1.81 Billion People. Climate Change and Unplanned Urbanization Could Worsen Exposure. World Bank Blogs. Available online at: https://blogs.worldbank.org/en/climatechange/flood-risk-already-affects-181-billion-people-climate-change-and-unplanned (accessed April 3, 2025).
Ritchie, H., Rosado, P., and Roser, M. (n.d.). Natural disasters. Our World in Data. Oxford: Global Change Data Lab. Available online at: https://ourworldindata.org/natural-disasters (accessed October 20, 2024).
Sohn, W., Kim, J.-H., Li, M.-H., Brown, R. D., and Jaber, F. H. (2020). How does increasing impervious surfaces affect urban flooding in response to climate variability?. Ecol. Indic. 118:106774. doi: 10.1016/j.ecolind.2020.106774
Soliman, M., Morsy, M. M., and Radwan, H. G. (2022). Assessment of implementing land use/land cover LULC 2020-ESRI global maps in 2d flood modeling application. Water 14:3963. doi: 10.3390/w14233963
Stevens, F. R., Gaughan, A. E., Linard, C., and Tatem, A. J. (2015). Disaggregating census data for population mapping using random forests with remotely-sensed and ancillary data. PLoS ONE 10:e0107042. doi: 10.1371/journal.pone.0107042
Toronto and Region Conservation Authority (2008). Performance Evaluation of Permeable Pavement and a Bioretention SWALE: Seneca College, King City, Ontario. Toronto, ON: Sustainable Technologies Evaluation Program.
U.S. Army Corps of Engineers (n.d.a) HEC-RAS River Analysis System 2D Modeling User's Manual. Washington, DC.
U.S. Army Corps of Engineers (n.d.b) HEC-RAS River Analysis System HEC-RAS Mapper User's Manual HEC-RAS Mapper User's Manual-2. Washington, DC.
U.S. Army Corps of Engineers (n.d.c) Development of the 2D Computational Mesh. Available online at: https://www.hec.usace.army.mil/ (accessed October 24 2024).
U.S. Army Corps of Engineers (n.d.d) HEC-RAS Mapper User's Manual HEC-RAS Mapper User's Manual. Washington DC.
U.S. Army Corps of Engineers (n.d.e) How Should HEC-HMS Be Used? Available online at: www.hec.usace.army.mil (accessed October 24, 2024).
U.S. Army Corps of Engineers (n.d.f) Introduction to HEC-RAS. Available online at: Army.mil (accessed October 23, 2024).
United States Environmental Protection Agency (US EPA) (n.d.). Water as a climate change solution. Available online at: https://www.epa.gov/climate-change-water-sector (accessed March 27, 2025).
Xinhua News Agency (n.d.). China intensifies disaster control efforts as torrential rain leads to more floods. Beijing. Available online at: https://english.news.cn/20240625/b17d9866c64247afa9a8daab369cf34b/ (accessed January 7, 2025).
Yu, Q., Wang, Y., and Li, N. (2022). Extreme flood disasters: comprehensive impact and assessment. Water 14:1211. doi: 10.3390/w14081211
Zhang, W., Villarini, G., Vecchi, G. A., and Smith, J. A. (2018). Urbanization exacerbated the rainfall and flooding caused by hurricane Harvey in Houston. Nature 563, 384–388. doi: 10.1038/s41586-018-0676-z
Keywords: flood-mapping, flood-forecasting, HEC-HMS-HEC-RAS modeling, victims evacuation and transfer, Shouchang River
Citation: Musyoka WW, Jun W, Mwanthi AM and Kiarii RW (2025) Flood early warning for early action—evacuation and transfer: case of Shouchang Town, Zhejiang Province, China. Front. Water 7:1553146. doi: 10.3389/frwa.2025.1553146
Received: 30 December 2024; Accepted: 17 March 2025;
Published: 08 April 2025.
Edited by:
Manish Pandey, Indian Institute of Technology, Kharagpur, IndiaReviewed by:
Anoop Kumar Shukla, Manipal Academy of Higher Education, IndiaAyat-Allah Bouramdane, International University of Rabat, Morocco
Copyright © 2025 Musyoka, Jun, Mwanthi and Kiarii. This is an open-access article distributed under the terms of the Creative Commons Attribution License (CC BY). The use, distribution or reproduction in other forums is permitted, provided the original author(s) and the copyright owner(s) are credited and that the original publication in this journal is cited, in accordance with accepted academic practice. No use, distribution or reproduction is permitted which does not comply with these terms.
*Correspondence: Wellington W. Musyoka, bXVzeW9rYXdlbGxpbmd0b25AZ21haWwuY29t