- 1Department of Integrative Biology and Physiology, University of California, Los Angeles, Los Angeles, CA, United States
- 2Molecular, Cellular, and Integrative Physiology Interdepartmental Program, University of California, Los Angeles, Los Angeles, CA, United States
- 3Bioinformatics Interdepartmental Program, University of California, Los Angeles, Los Angeles, CA, United States
- 4Institute for Quantitative and Computational Biosciences, University of California, Los Angeles, Los Angeles, CA, United States
- 5Molecular Biology Institute, University of California, Los Angeles, Los Angeles, CA, United States
The success of genome-wide association studies (GWAS) has significantly advanced our understanding of the etiology of coronary artery disease (CAD) and opens new opportunities to reinvigorate the stalling CAD drug development. However, there exists remarkable disconnection between the CAD GWAS findings and commercialized drugs. While this could implicate major untapped translational and therapeutic potentials in CAD GWAS, it also brings forward extensive technical challenges. In this review we summarize the motivation to leverage GWAS for drug discovery, outline the critical bottlenecks in the field, and highlight several promising strategies such as functional genomics and network-based approaches to enhance the translational value of CAD GWAS findings in driving novel therapeutics
Introduction
Coronary artery disease (CAD) is a leading cause of mortality worldwide (1). CAD is well recognized as a complex disease with both genetic and environmental contributions (2). The heritability of CAD is estimated to be 40–50% (3), and the genetics of CAD plays an indispensable role in unraveling the pathogenic processes and ultimately facilitating the discovery of novel therapeutics. In the past decade, our understanding of the genetic architecture and mechanistic underpinnings for CAD has been substantially accelerated and broadened, primarily attributable to the successful global collaborative efforts in large-scale human genome-wide association studies (GWAS). These efforts have helped reveal hundreds of novel genetic variants demonstrating significant associations with CAD.
In contrast to the gratifying successes of GWAS, the development of CAD drugs has stagnated over the past decades, especially when compared to other therapeutic areas (4). What is particularly concerning is the fact that the drug development effort has been primarily concentrated on correcting previously established CAD risk factors such as lipid levels, coagulation factors, and hypertension, instead of targeting novel pathways revealed from recent studies (Figure 1). This decoupling between mechanistic discovery studies and drug development is striking. Therefore, it is of critical importance to form strategies that leverage the recent genetic discoveries from GWAS and other relevant efforts such as multi-dimensional data integration and systems genetics to allow for efficient identification of novel and reliable CAD drug targets. In this review, we summarize the state of CAD GWAS discovery, delineate the significant challenges of translating GWAS to drug targets, discuss successful examples of GWAS driven CAD drug target discovery, and outline promising strategies to further catalyze the translation of CAD GWAS into novel therapeutic options.
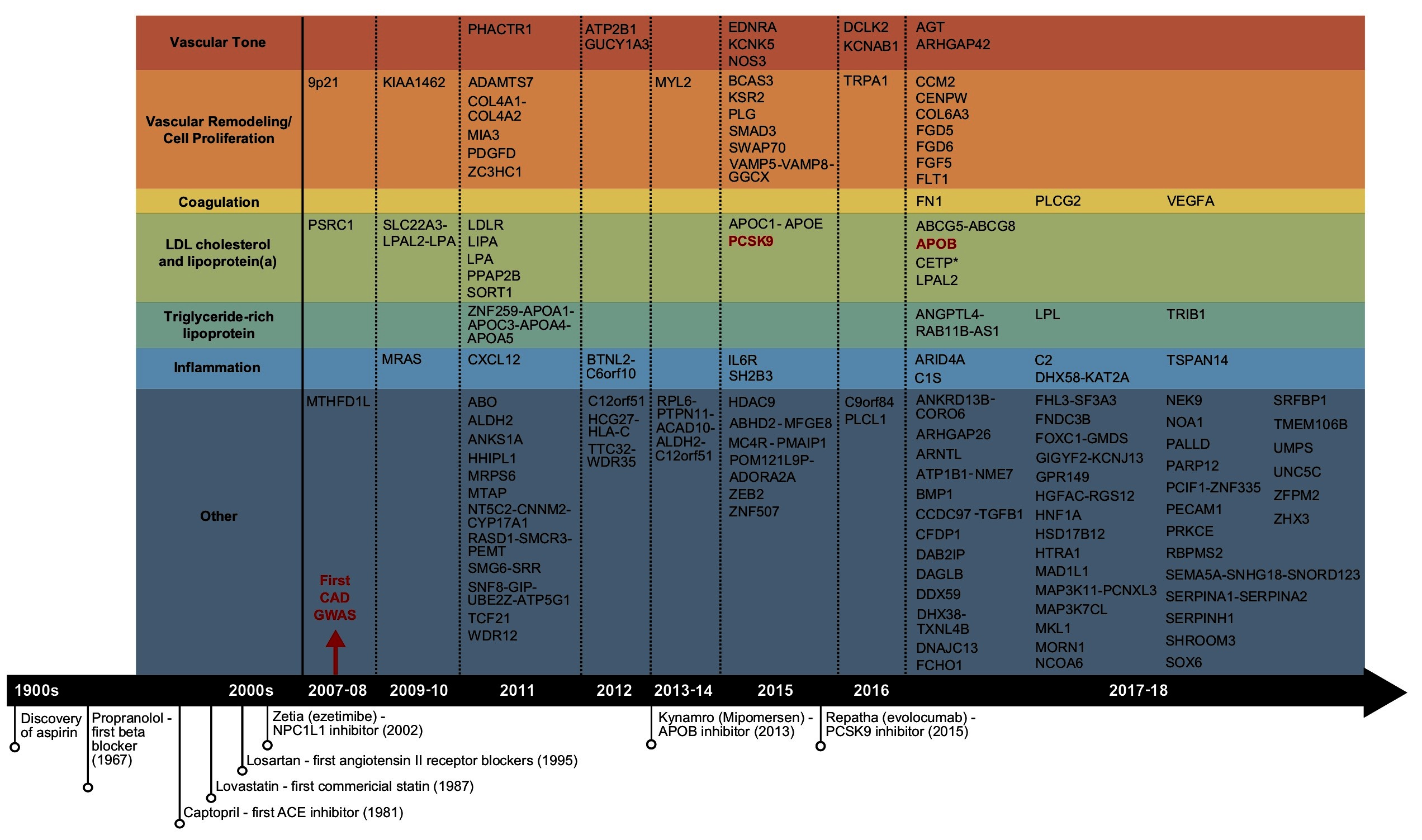
Figure 1. Summary of the reported CAD GWAS loci and important CAD drug discoveries. Candidate genes under GWAS loci identified to date were retrieved from the reported genes column in GWAS Catalog, organized by year. Only one candidate gene per locus was shown. GWAS loci that overlap with the targets of commercialized drugs were shown in red.
GWAS Discovery for CAD and Its Implications for Drug Target Discovery
The completion of the human genome project, the rapid declining cost of genome sequencing, the rising feasibility of global multi-group collaborations, and the increasing accessibility of shared data repositories have collectively fueled the explosion of genetic studies of CAD, particularly GWAS. GWAS were typically designed to profile common known variants, often defined as variants with allele frequency ≥ 0.5% (5), on pre-designed microarrays containing primarily single nucleotide polymorphisms (SNPs). Since the first CAD GWAS in 2007 (6), over 18 GWAS studies have been carried out in the past decade, with the most recent and largest study involving 34,541 cases and 261,984 controls (7). These studies revealed a total of 163 genetic loci linked to CAD (8) (Figure 1), explaining 30–40% of CAD heritability (7, 9, 10). The swift pace of GWAS has greatly facilitated the comprehensive construction of the CAD genetic landscape, and has led to rapid accumulation of potential causal variants and genes.
Overall, GWAS have played a key role in not only confirming classic CAD risk factors such as LDL cholesterol, hypertension, and coagulation, but also highlighting the causal roles of cellular proliferation and adhesion, extracellular matrix, and inflammation (Figure 1), which are processes related to the endothelial and smooth muscle cells in the vascular wall and the immune system (3, 11). Unfortunately, to date no novel CAD GWAS genes beyond a few involved in classic risk factors have been established as viable drug targets for CAD, a pattern that resonates for GWAS of most complex traits (Figure 1) (12). The disconnection between CAD GWAS findings and treatment targets is disappointing and has been criticized, but could also implicate major untapped opportunities (13). In particular, the causal variants and genes involved in the new causal pathways informed by GWAS have been encouraging early stage advances in uncovering novel therapeutic options targeting the vascular wall components, cell proliferation, and inflammation. For example, the ADAMTS7 loci, coding for a metalloproteinase with thrombospondin motifs 7, was implicated for atherosclerotic progression through smooth muscle cell migration, a mechanism independent of classic CAD risk factors (14). Upon confirmation of its causal role in affecting atherosclerosis occurrence in vivo (15), development of the ADAMTS7 pharmacophore has progressed towards establishing inhibitors via virtual screening (16). Tocilizumab, an anti-inflammatory agent blocking interleukin-6, was found to improve endothelial function (17). Antibodies targeting CD47, a key anti-phagocytic and tumorigenic molecule, were also shown to ameliorate atherosclerosis by stimulating efferocytosis (18).
Despite the potential promises, several factors could have complicated the extraction of therapeutic value from GWAS. First, the functional regulatory circuits from most variants to disease outcomes remain elusive. This is reflected by both the difficulty in pinpointing the causal variants and the corresponding target genes, especially for variants located in non-coding regions. In fact, the exact effector genes and functions for over 50% of the CAD GWAS loci are unclear. For example, the 9p21 locus was the strongest CAD locus but is located in a gene desert (6, 19, 20). Multiple follow-up studies have suggested several effectors for this locus, including the non-coding RNA ANRIL (21), CDKN2A/CDKN2B (22, 23), and interferon-gamma signaling (24). However, the detailed mechanism is still under debate after a decade of research (25). Moreover, even if a CAD variant is located within a gene-rich region, the most adjacent gene(s) may not be the functional candidate (26). Second, even if the candidate genes can be unequivocally determined, the functions of the genes are not necessarily well established, and extensive functional studies are required to derive a mechanistic understanding of how the candidate genes lead to CAD risks. Third, most common variants only confer weak to moderate CAD risk (<20% change in risk), most likely due to evolutionary pressure which selects against non-synonymous SNPs in disease genes involved in key physiological processes (12, 27–30). The prevalence of moderate/weak effect sizes of CAD risk variants makes prioritization of drug targets difficult. Lastly, it has been suspected that the top CAD risk variants identified so far predominantly inform on genes active in the early and slow phase of CAD development, whereas variants affecting late and rapid CAD phases tend to be missed by GWAS as these are likely more dependent on specific contexts such as particular environmental exposures or inflammatory states that are poorly controlled in most GWAS (31). Indeed, a recent study of Crohn’s disease that focuses on disease course or prognosis using a within-cases design revealed loci that are completely different from those derived from case-control studies (32). This is also likely the case for CAD. Therefore, drug targets derived from CAD GWAS findings may not carry the expected efficacy to counteract CAD progression.
Strategies to Fast-Forward the Translation of GWAS to Treatment Targets
To bypass the challenges facing the translation of GWAS findings to therapeutic targets as outlined above, a number of strategies have been designed and attempted. These efforts mainly focus on integrating GWAS hits with other data types that help inform on the functions of candidate genes, pathways, and networks, narrow down and prioritize the causal candidates, and leverage the matching patterns between disease mechanisms and molecular patterns of drugs (Figure 2).
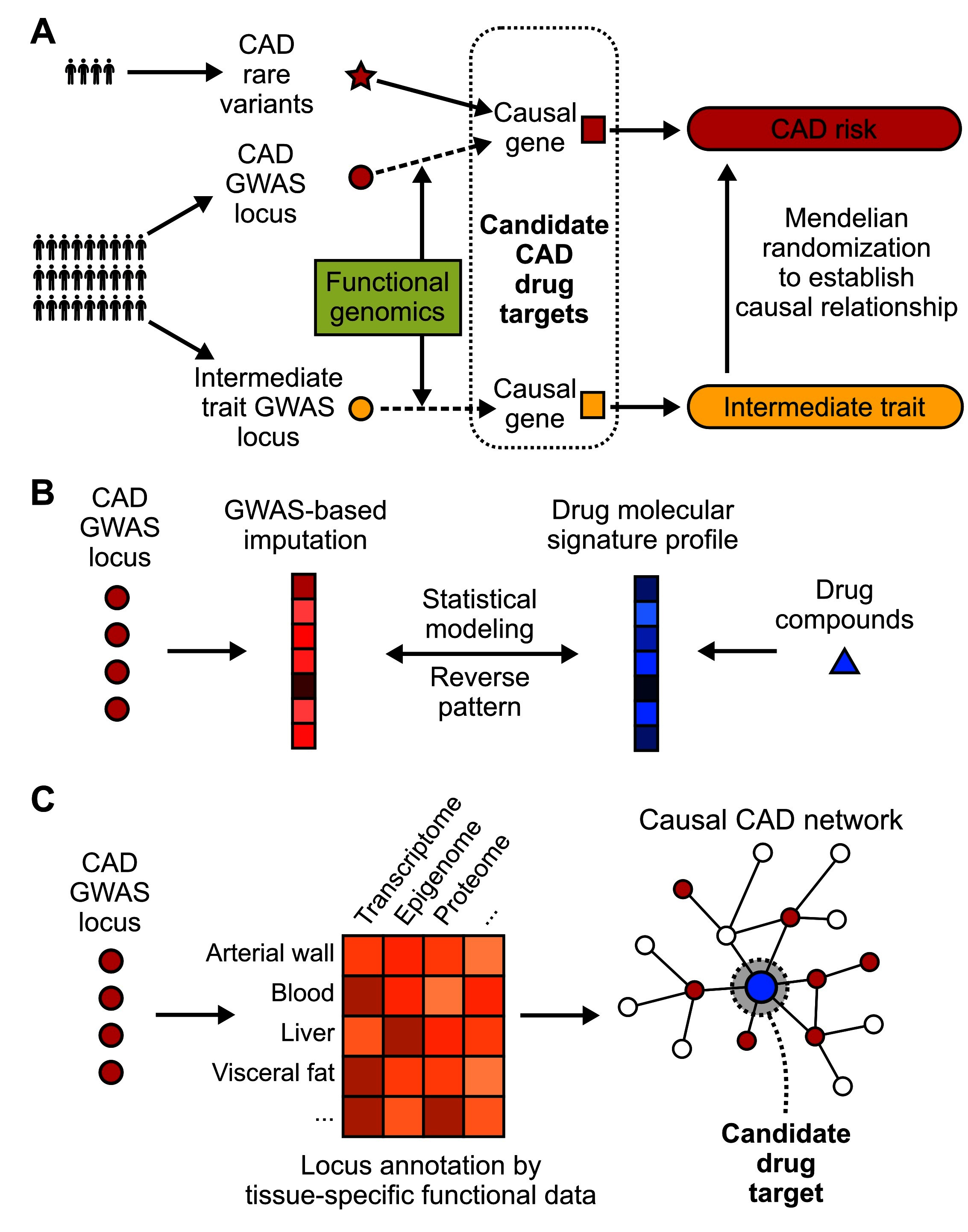
Figure 2. Strategies to translate CAD GWAS into drug targets. (A) Identification of CAD causal genes as candidate drug targets by incorporating functional genomics, rare variants and Mendelian randomization. Loss-of-function rare variants can be linked to downstream genes. The connection between common variants and causal genes usually requires integration of functional genomics data. Mendelian randomization can further filter the drug target selection pool by incorporating causal intermediate traits. (B) A “target-less” approach to reposition existing drug compounds for CAD by evaluating the existence of opposite patterns between drug molecular profiles and GWAS imputed molecular profiles of disease. (C) Network-based approaches that model CAD GWAS data along with other omics data from CAD relevant tissues or cell types in the context of gene networks, which have the power to pinpoint key network regulators as candidate drug targets with more potent effects.
Use of Rare Variant Association Studies to Prioritize Targets
As discussed above, common variants uncovered from GWAS studies are numerous in number while carrying weak to subtle effect sizes, making it challenging to prioritize viable targets. Rare genetic variants (frequencies lower than 0.5%) that are associated with diseases, on the other hand, usually exhibit stronger perturbations in gene functions and are under stronger evolutionary pressure. Therefore, rare variants, especially those leading to loss-of-function, provide a natural setting mimicking human knockout cases to assess phenotypic and clinical consequences of variants, and their power in informing causal disease genes and drug targets has been long recognized (Figure 2A) (33, 34). Aggregation of rare mutations in 10 genes, including APOA5 (35), APOC3 (36), ASGR1 (37), ANGPTL3 (38), ANGPTL4 (39), LPA (40), LDLR (35), LPL (41), NPC1L1 (42), and PCSK9 (43), has been linked to CAD risk through whole exome or whole genome sequencing-based studies. Out of the 10 genes, 5 (ANGPTL4, APOC3, LPA, NPC1L1, PCSK9) have been explored as drug targets for CAD (3). To date, the main success of this approach lies in the approval of PCSK9 antibodies by the FDA. Carriers with inactivating mutations on PCSK9 were found to have markedly lower LDL cholesterol level and CAD risk, which led to the discovery of two FDA-approved monoclonal antibodies, Alirocumab and Evolucumab. However, the potential of drug discovery using CAD rare variants is also limited by both the small number of robust rare variants found so far, and their low cumulative contribution to CAD risk in the general population (9). Additionally, most of them are involved in the previously established pathways rather than novel mechanisms. Nevertheless, these rare variants provide compelling causal inference of the downstream genes and pathways in CAD pathogenesis, and they are more likely to be specific to a disease (broad effects could be detrimental in human knockouts) and have safer profiles, key components for success as drug targets. Future GWAS will likely evolve from SNP array design to whole genome sequencing to profile both common and rare variants (44), thus further expanding the pool of loss-of-function variants for drug target selection. Some of the novel rare variants may inform on novel causal mechanisms not captured by common variants, or converge on genes and pathways already informed by common variants thus serving to enhance the causal inference at a more functional level.
Functional Genomics to Identify and Prioritize Causal GWAS Genes
In contrast to rare coding mutations whose target genes and downstream mechanisms can be more readily uncovered through traditional functional studies, identifying the causal genes that are responsible for the observed link between GWAS risk variants and CAD is not an easy task. It is estimated that two thirds of the predicted target genes of GWAS locus are not the closest by proximity (45, 46), thus traditional proximity-based locus mapping could introduce false interpretations that bias drug target selection. This challenge can be substantially alleviated by functional genomics tools that explore potential mechanisms linking causal variants to biological phenotypes (Figure 2A) (44). Supported by next-generation sequencing, typical functional molecular traits that may be characterized include expression quantitative trait locus (eQTL), non-coding RNA, transcription factor binding sites, epigenetic modification and chromatin interaction (26). The advance of gene editing technologies such as CRISPR/Cas has also significantly improved the efficiency of validation experiments (47). Recent functional genomics studies have substantially refined the candidate causal genes for CAD loci such as SORT1 (48), TRIB1 (49), ADAMTS7 (15, 50), and TCF21 (51, 52). Noteworthy, there have also been integrative functional genomics studies that combined genomics, epigenomics and transcriptomics profiling to prioritize causal variants and affected genes (7, 46, 53). For example, Miller et al. integrated Assay for Transposase Accessible Chromatin (ATAC-seq) and chromatin immunoprecipitation-sequencing (ChIP-seq) to unravel the cis-regulatory mechanisms in human coronary artery smooth muscle cells, and prioritized 64 variants over 7 candidate CAD loci including 9p21.3, SMAD3, PDGFD, IL6R, BMP1, CCDC97/TGFB1 and LMOD1 (53). Haitjema et al. also leveraged circular chromosome conformation capture sequencing (4C-seq) with RNA-seq and eQTL to identify 294 novel candidate CAD genes (54). These studies greatly contribute to the accumulation of viable treatment targets for follow-up drug development efforts.
Encouragingly, functional studies following GWAS are being further catalyzed by large-scale community efforts in establishing multi-cell or multi-tissue mapping of regulatory annotations. The advent of publicly available depositories such as GTEx (55), ENCODE (56) and Epigenome Roadmap (57) is gradually removing the hurdle to acquire multi-dimensional data resources necessary for the investigation of complex traits like CAD.
Mendelian Randomization (MR) to Facilitate Drug Target Selection
Previous successes in drug development for CAD have testified to the effectiveness of modulating intermediate causal risk factors such as circulating cholesterol levels and blood pressure in lowering CAD risk. Therefore, knowing the causal relationship between an intermediate phenotype that correlates with CAD status is of monumental importance as it can help prioritize biomarkers as intervention targets for CAD therapeutics (58, 59) (Figure 2A). The investigation of causal intermediate traits for CAD can be facilitated by MR, which utilizes genetic variants as instrumental variables to assess the causal relationship between exposure (e.g., LDL cholesterol, HDL cholesterol, weight-hip ratio) and outcome (CAD occurrence) (60). We are seeing both successful and ongoing efforts in developing drugs modulating LDL cholesterol, triglyceride-rich lipoproteins and lipoprotein (a) (3), whose causal relationships with CAD have been robustly verified in MR studies (61–63). On the contrary, MR studies revealed inconsistent relationship between HDL cholesterol and CAD (64–66). In concordance with this lack of robust support for the causality of HDL in CAD, substantial obstacles have been met during the development of inhibitors for CETP (cholesteryl ester transfer protein), a gene harboring several loci associated with HDL cholesterol level (67). Three commercial CETP inhibitors, Dalcetrapib, Obicetrapib and Anacetrapib, all failed to achieve clinical efficacy during phase III clinical trials and were discontinued (68).
In addition to the well explored causal pathways such as cholesterol and blood pressure regulation, MR studies have informed several additional causal intermediate phenotypes, such as inflammation (69), uric acid (70), and iron status (71), that could serve as targets for future CAD drug development. By utilizing both summary-level GWAS statistics and UK Biobank data, a recent MR study demonstrated the causal association of waist-to-hip ratio adjusted for body mass index with coronary heart disease, thus providing new opportunities of intervening CAD risk by reducing abdominal obesity (72).
GWAS-Based “Target-Free” Drug Repositioning
Drug repurposing approaches could leverage known drugs used for other diseases that target the newly uncovered CAD causal genes and pathways to counteract CAD. For example, better understanding of CAD pathways involved in inflammation and cell cycle has promoted the repurposing of drugs targeting diseases such as rheumatoid arthritis (17) and cancer (18). On the other hand, given the challenging nature of identifying both the causal genes from GWAS and matching it with the target of drug compounds, “target-free” approaches have been developed which require no prior knowledge of targets for either drugs or GWAS variants and can simultaneously take many genetic loci into consideration (73) (Figure 2B). The fundamental concept behind these approaches is to impute gene expression profiles from GWAS summary statistics, compare the expression patterns against gene expression profiles of drugs, then prioritize top drug candidates whose profiles show reverse patterns compared with GWAS-imputed signatures. This approach is especially useful for repositioning existing drugs whose chemical properties and molecular responses have been well characterized and made accessible from public data repositories such as CMap (74) and its successor, the L1000 platform (75), as well as other chemoinformatic resources (76, 77).
To facilitate such efforts, the work by Gamazon et al. represents one of the first transcriptome imputation pipelines where disease relevant gene expression is estimated from a tissue-dependent model trained with personal genotype data and reference transcriptome (78). Gusev et al. and So et al. further developed summary GWAS statistics based transcriptome imputation methods, which relieved the requirement for individual genotype data (73, 79). In addition, inferring gene expression changes from GWAS enables researchers to assess transcriptome-wide associations with CAD that could yield novel candidate genes for functional and therapeutic investigation (45, 79). Although direct application of the “target-free” approaches for CAD is still under-explored, a computational framework has been developed to reposition existing drugs for psychiatry (73). The framework, built on a GWAS-based transcriptome imputation pipeline named MetaXcan (80), first imputed the gene expression profiles of 10 brain regions for 7 psychiatric disorders based on GWAS and reference transcriptome data from GTEx (55). This disease transcriptome information was then used to match with drug-induced gene expression profiles from the CMap database (74) to prioritize drugs that showed opposite gene expression patterns compared to the disease patterns. These platforms are potentially translatable to CAD.
Network-Based Drug Discovery Approaches
The success of GWAS-driven drug target identification heavily relies on the fundamental assumption of how genetic risk variants eventually contribute to disease phenotype. An “omnigenic” model for the genetics of complex traits has been recently proposed (30). This provocative model objects the common belief that risk variants drive disease etiology through functional clustering in biological pathways, and emphasizes that all genes in disease-relevant cells could affect core disease processes through the coordination of gene regulatory networks.
Motivated by the gene network hypothesis, the CAD field has been actively investing on the development and application of systems genetics frameworks that integrate genetics and other data dimensions in the context of network topology to help prioritize candidate CAD genes (Figure 2C) (27–29, 81–84). The implementation of network-based target identification strategies poses several unique advantages over other methods. First, gene networks have the potential to comprehensively map the regulatory circuits under physiological or pathological conditions, thus improving the biological relevance of predicted targets. Second, gene networks serve as a natural platform for data integration, where GWAS and information from other omics space can be collectively leveraged to pinpoint network hotspots where key perturbation events likely happen. Third, gene networks enable the identification of essential disease genes, which is unlikely to tolerate high frequency loss-of-function variation at the population level and to be discovered by GWAS (85). Several methods have been developed to find network essential genes, or key drivers, by considering both network topology and external disease signatures (27, 81, 86). The validity of the predicted key drivers in driving CAD relevant traits has been well supported (27, 29, 82), and the key drivers have the potential to serve as novel drug targets with strong therapeutic effects due to their central importance in regulating the disease networks. For instance, Zhao et al. recently prioritized CAD key drivers and proposed plausible targets using network approaches (28). Extensive in vitro and in vivo gene perturbation experiments are required to evaluate the feasibility of using key driver genes as drug targets. If proven valid, network-based discoveries could provide exciting opportunities to formulate more focused and data-informed hypotheses for downstream therapeutic investigation. Nevertheless, it is important to caution that modulation of network key drivers may result in a lack of specificity and increase the risks for side effects due to their broad impact on numerous network genes.
One critical challenge for network-based CAD drug discovery is the availability of high-quality gene networks from CAD relevant cells, tissues, and subjects. Many existing networks are literature-based and lack tissue/cell specificity. Even for data-driven networks, data collection bias exists. For example, human network construction usually requires large numbers of clinical samples that are difficult to acquire, especially for samples from internal tissues. A major breakthrough is the establishment of the STARNET networks involving tissue-specific data from ~600 CAD patients (87). This resource, in combination with other networks generated from mouse models or non-disease human subjects, is invaluable for future CAD network studies. Coordinated efforts by the research community are needed to enhance the coverage of data-driven networks from CAD relevant tissues and cell types.
Conclusions and Future Directions
GWAS has been highly successful in elucidating the genetic architecture of CAD and driving the discovery of novel biology. While confirming the genes and pathways targeted by classic CAD treatments, GWAS opens doors to a vast number of under-recognized candidates where future CAD drugs could originate. The field of GWAS-driven drug discovery is still at its infancy, and significant challenges remain. However, it is encouraging that numerous methodological advances have been made to address the bottlenecks, and application of these approaches is expected to facilitate future translational research in CAD.
Here we anticipate the following future directions that will help further advance the field. First, there is a need for broader collaboration to conduct large-scale functional genomics studies in human tissues and cell types that implement cutting-edge high-throughput profiling technologies over multiple omics to map the tissue- and cell-type specific regulatory circuit of GWAS loci. In particular, application of cell-type specific analyses at multi-omics levels will help address the functional heterogeneity in CAD relevant tissues, which will lead to refined understanding of disease etiology and lay a solid foundation for more accurate prediction of drugs that can counteract the specific pathogenic processes in the right cell types and tissues (88). The recent launch of the Human Cell Atlas project represents one of the first stepping stones towards this direction (89). Second, more efficient platforms are needed to facilitate sharing of summary-level GWAS data as well as databases and data repositories that curate multi-omics functional information. For example, in the neurological disorder field, there are emerging efforts like CommonMind (http://commonmind.org), PsychENCODE (90) and BrainSeq (91). Similar coordinated efforts by the CAD community will accelerate identification of CAD drug targets. Third, the translational value of GWAS data can be better exploited by the development of novel analytical pipelines that integrate multi-dimensional data from animals, humans, and chemoinformatic databases. Some of the recently developed analytical pipelines can integrate GWAS and functional genomics data for target prediction, and are directly applicable to CAD (45, 79, 81, 92). Additional pipelines that couple disease datasets with drug footprints in a gene network framework will facilitate the identification of network regulators and pathways that can be accurately targeted. Lastly, gradual transition from initial target screening to GWAS-guided experimental validation of the predicted targets using a combination of in vitro, in vivo, and in silico methods will further the translational path.
Author Contributions
LS, MB and XY drafted and edited the manuscript.
Funding
LS is funded by the Burroughs Wellcome Fund Inter-school Training Program in Chronic Diseases Scholarship and UCLA Dissertation Year Fellowship. XY is funded by the Leducq Foundation Transatlantic Network of Excellence, NIH/NIDDK DK104363, and NIH/NINDS NS103088.
Conflict of Interest Statement
The authors declare that the research was conducted in the absence of any commercial or financial relationships that could be construed as a potential conflict of interest.
Acknowledgments
The authors thank the reviewers for the constructive comments.
References
1. Abubakar I, Tillmann T, Global BA. regional, and national age-sex specific all-cause and cause-specific mortality for 240 causes of death, 1990-2013: a systematic analysis for the Global Burden of Disease Study 2013. Lancet (2015) 385(9963):117–71.
2. Khera AV, Emdin CA, Drake I, Natarajan P, Bick AG, Cook NR, et al. Genetic risk, adherence to a healthy lifestyle, and coronary disease. N Engl J Med Overseas Ed (2016) 375(24):2349–58. doi: 10.1056/NEJMoa1605086
3. Khera AV, Kathiresan S. Genetics of coronary artery disease: discovery, biology and clinical translation. Nat Rev Genet (2017) 18(6):331–44. doi: 10.1038/nrg.2016.160
4. Fordyce CB, Roe MT, Ahmad T, Libby P, Borer JS, Hiatt WR, et al. Cardiovascular drug development: is it dead or just hibernating? J Am Coll Cardiol (2015) 65(15):1567–82.
5. Zuk O, Schaffner SF, Samocha K, do R, Hechter E, Kathiresan S, et al. Searching for missing heritability: Designing rare variant association studies. Proc Natl Acad Sci U S A (2014) 111(4):E455–64. doi: 10.1073/pnas.1322563111
6. Wellcome Trust Case Control Consortium. Genome-wide association study of 14,000 cases of seven common diseases and 3,000 shared controls. Nature (2007) 447(7145):661–78. doi: 10.1038/nature05911
7. Van der Harst P, Verweij N. Identification of 64 novel genetic loci provides an expanded view on the genetic architecture of coronary artery disease novelty and significance. Circ Res (2018) 122(3):433–43. doi: 10.1161/CIRCRESAHA.117.312086
8. Erdmann J, Kessler T, Munoz Venegas L, Schunkert H. A decade of genome-wide association studies for coronary artery disease: the challenges ahead. Cardiovasc Res (2018) 385. doi: 10.1093/cvr/cvy084
9. Nikpay M, Goel A, Won HH, Hall LM, Willenborg C, Kanoni S, et al. A comprehensive 1000 Genomes-based genome-wide association meta-analysis of coronary artery disease. Nat Genet (2015) 47(10):1121–30. doi: 10.1038/ng.3396
10. Klarin D, Zhu QM, Emdin CA, Chaffin M, Horner S, Mcmillan BJ, et al. Genetic analysis in UK Biobank links insulin resistance and transendothelial migration pathways to coronary artery disease. Nat Genet (2017) 49(9):1392–7. doi: 10.1038/ng.3914
11. Kessler T, Vilne B, Schunkert H. The impact of genome‐wide association studies on the pathophysiology and therapy of cardiovascular disease. EMBO Mol Med (2016) 8(7):688–701. doi: 10.15252/emmm.201506174
12. Cao C, Moult J. GWAS and drug targets. BMC Genomics (2014) 15(Suppl 4):S5. doi: 10.1186/1471-2164-15-S4-S5
13. Schooling CM, Huang JV, Zhao JV, Kwok MK, Au Yeung SL, Lin SL. Disconnect between genes associated with ischemic heart disease and targets of ischemic heart disease treatments. EBioMedicine (2018) 28:311–5. doi: 10.1016/j.ebiom.2018.01.015
14. Reilly MP, Li M, He J, Ferguson JF, Stylianou IM, Mehta NN, et al. Identification of ADAMTS7 as a novel locus for coronary atherosclerosis and association of ABO with myocardial infarction in the presence of coronary atherosclerosis: two genome-wide association studies. The Lancet (2011) 377(9763):383–92. doi: 10.1016/S0140-6736(10)61996-4
15. Bauer RC, Tohyama J, Cui J, Cheng L, Yang J, Zhang X, et al. Knockout of Adamts7, a novel coronary artery disease locus in humans, reduces atherosclerosis in mice. Circulation (2015) 131(13):1202–13. doi: 10.1161/CIRCULATIONAHA.114.012669
16. Müller M, Kessler T, Schunkert H, Erdmann J, Tennstedt S. Classification of ADAMTS binding sites: The first step toward selective ADAMTS7 inhibitors. Biochem Biophys Res Commun (2016) 471(3):380–5. doi: 10.1016/j.bbrc.2016.02.025
17. Bacchiega BC, Bacchiega AB, Usnayo MJG, Bedirian R, Singh G, Pinheiro Gdarc. Interleukin 6 Inhibition and coronary artery disease in a high‐risk population: A prospective community‐based clinical study. J Am Heart Assoc (2017) 6(3):e005038. doi: 10.1161/JAHA.116.005038
18. Kojima Y, Volkmer J-P, Mckenna K, Civelek M, Lusis AJ, Miller CL, et al. CD47-blocking antibodies restore phagocytosis and prevent atherosclerosis. Nature (2016) 536(7614):86–90. doi: 10.1038/nature18935
19. Helgadottir A, Thorleifsson G, Manolescu A, Gretarsdottir S, Blondal T, Jonasdottir A, et al. A common variant on chromosome 9p21 affects the risk of myocardial infarction. Science (2007) 316(5830):1491–3. doi: 10.1126/science.1142842
20. Mcpherson R, Pertsemlidis A, Kavaslar N, Stewart A, Roberts R, Cox DR, et al. A common allele on chromosome 9 associated with coronary heart disease. Science (2007) 316(5830):1488–91. doi: 10.1126/science.1142447
21. Holdt LM, Stahringer A, Sass K, Pichler G, Kulak NA, Wilfert W, et al. Circular non-coding RNA ANRIL modulates ribosomal RNA maturation and atherosclerosis in humans. Nat Commun (2016) 7:12429. doi: 10.1038/ncomms12429
22. Kojima Y, Downing K, Kundu R, Miller C, Dewey F, Lancero H, et al. Cyclin-dependent kinase inhibitor 2B regulates efferocytosis and atherosclerosis. J Clin Invest (2014) 124(3):1083–97. doi: 10.1172/JCI70391
23. Kim JB, Deluna A, Mungrue IN, Vu C, Pouldar D, Civelek M, et al. Effect of 9p21.3 coronary artery disease locus neighboring genes on atherosclerosis in mice. Circulation (2012) 126(15):1896–906. doi: 10.1161/CIRCULATIONAHA.111.064881
24. Harismendy O, Notani D, Song X, Rahim NG, Tanasa B, Heintzman N, et al. 9p21 DNA variants associated with coronary artery disease impair interferon-γ signalling response. Nature (2011) 470(7333):264–8. doi: 10.1038/nature09753
25. Pjanic M, Miller CL, Wirka R, Kim JB, Direnzo DM, Quertermous T. Genetics and genomics of coronary artery disease. Curr Cardiol Rep (2016) 18(10):102. doi: 10.1007/s11886-016-0777-y
26. Nurnberg ST, Zhang H, Hand NJ, Bauer RC, Saleheen D, Reilly MP, et al. From loci to biology: functional genomics of genome-wide association for coronary disease. Circ Res (2016) 118(4):586–606.
27. Mäkinen V-P, Civelek M, Meng Q, Zhang B, Zhu J, Levian C, et al. Integrative genomics reveals novel molecular pathways and gene networks for coronary artery disease. PLoS Genet (2014) 10(7):e1004502. doi: 10.1371/journal.pgen.1004502
28. Zhao Y, Chen J, Freudenberg JM, Meng Q, Rajpal DK, Yang X. Network-based identification and prioritization of key regulators of coronary artery disease loci. Arterioscler Thromb Vasc Biol (2016) 36(5):928–41. doi: 10.1161/ATVBAHA.115.306725
29. Shu L, Chan KHK, Zhang G, Huan T, Kurt Z, Zhao Y, et al. Shared genetic regulatory networks for cardiovascular disease and type 2 diabetes in multiple populations of diverse ethnicities in the United States. PLoS Genet (2017) 13(9):e1007040. doi: 10.1371/journal.pgen.1007040
30. Boyle EA, Li YI, Pritchard JK. An expanded view of complex traits: From polygenic to omnigenic. Cell (2017) 169(7):1177–86. doi: 10.1016/j.cell.2017.05.038
31. Björkegren JL, Kovacic JC, Dudley JT, Schadt EE. Genome-wide significant loci: how important are they?: systems genetics to understand heritability of coronary artery disease and other common complex disorders. J Am Coll Cardiol (2015) 65(8):830–45.
32. Lee JC, Biasci D, Roberts R, Gearry RB, Mansfield JC, Ahmad T, et al. Genome-wide association study identifies distinct genetic contributions to prognosis and susceptibility in Crohn's disease. Nat Genet (2017) 49(2):262–8. doi: 10.1038/ng.3755
33. Macarthur DG, Balasubramanian S, Frankish A, Huang N, Morris J, Walter K, et al. A systematic survey of loss-of-function variants in human protein-coding genes. Science (2012) 335(6070):823–8. doi: 10.1126/science.1215040
34. Bomba L, Walter K, Soranzo N. The impact of rare and low-frequency genetic variants in common disease. Genome Biol (2017) 18(1):77. doi: 10.1186/s13059-017-1212-4
35. do R, Stitziel NO, Won H-H, Jørgensen AB, Duga S, Angelica Merlini P, et al. Exome sequencing identifies rare LDLR and APOA5 alleles conferring risk for myocardial infarction. Nature (2015) 518(7537):102–6. doi: 10.1038/nature13917
36. Jørgensen AB, Frikke-Schmidt R, Nordestgaard BG, Tybjærg-Hansen A. Loss-of-function mutations in APOC3 and risk of ischemic vascular disease. N Engl J Med Overseas Ed (2014) 371(1):32–41. doi: 10.1056/NEJMoa1308027
37. Nioi P, Sigurdsson A, Thorleifsson G, Helgason H, Agustsdottir AB, Norddahl GL, et al. Variant ASGR1 associated with a reduced risk of coronary artery disease. N Engl J Med Overseas Ed (2016) 374(22):2131–41. doi: 10.1056/NEJMoa1508419
38. Stitziel NO, Khera AV, Wang X, Bierhals AJ, Vourakis AC, Sperry AE, et al. ANGPTL3 deficiency and protection against coronary artery disease. J Am Coll Cardiol (2017) 69(16):2054–63. doi: 10.1016/j.jacc.2017.02.030
39. Myocardial Infarction Genetics, C. ARDIoGRAM Exome Consortia Investigators, Stitziel NO, Stirrups KE, Masca NG, Erdmann J, et al. Coding Variation in ANGPTL4, LPL, and SVEP1 and the Risk of Coronary Disease. N Engl J Med (2016) 374(12):1134–44.
40. Emdin CA, Khera AV, Natarajan P, Klarin D, Won H-H, Peloso GM, et al. Phenotypic characterization of genetically lowered human lipoprotein(a) levels. J Am Coll Cardiol (2016) 68(25):2761–72. doi: 10.1016/j.jacc.2016.10.033
41. Khera AV, Won H-H, Peloso GM, O’Dushlaine C, Liu D, Stitziel NO, et al. Association of rare and common variation in the lipoprotein lipase gene with coronary artery disease. JAMA (2017) 317(9):937–46. doi: 10.1001/jama.2017.0972
42. Myocardial Infarction Genetics Consortium Investigators, Stitziel NO, Won HH, Morrison AC, Peloso GM, Do R, et al. Inactivating mutations in NPC1L1 and protection from coronary heart disease. N Engl J Med (2014) 371(22):2072–82. doi: 10.1056/NEJMoa1405386
43. Cohen JC, Boerwinkle E, Mosley TH, Hobbs HH. Sequence variations in PCSK9, low LDL, and protection against coronary heart disease. N Engl J Med Overseas Ed (2006) 354(12):1264–72. doi: 10.1056/NEJMoa054013
44. Visscher PM, Wray NR, Zhang Q, Sklar P, Mccarthy MI, Brown MA, et al. 10 Years of GWAS Discovery: Biology, Function, and Translation. Am J Hum Genet (2017) 101(1):5–22. doi: 10.1016/j.ajhg.2017.06.005
45. Zhu Z, Zhang F, Hu H, Bakshi A, Robinson MR, Powell JE, et al. Integration of summary data from GWAS and eQTL studies predicts complex trait gene targets. Nat Genet (2016) 48(5):481–7. doi: 10.1038/ng.3538
46. Brænne I, Civelek M, Vilne B, Di Narzo A, Johnson AD, Zhao Y, et al. Prediction of causal candidate genes in coronary artery disease loci. Arterioscler Thromb Vasc Biol (2015) 35(10):2207–17. doi: 10.1161/ATVBAHA.115.306108
47. Cong L, Ran FA, Cox D, Lin S, Barretto R, Habib N, et al. Multiplex genome engineering using CRISPR/Cas systems. Science (2013) 339(6121):819–23. doi: 10.1126/science.1231143
48. Musunuru K, Strong A, Frank-Kamenetsky M, Lee NE, Ahfeldt T, Sachs KV, et al. From noncoding variant to phenotype via SORT1 at the 1p13 cholesterol locus. Nature (2010) 466(7307):714–9. doi: 10.1038/nature09266
49. Douvris A, Soubeyrand S, Naing T, Martinuk A, Nikpay M, Williams A, et al. Functional analysis of the TRIB1 associated locus linked to plasma triglycerides and coronary artery disease. J Am Heart Assoc (2014) 3(3):e000884. doi: 10.1161/JAHA.114.000884
50. Pu X, Xiao Q, Kiechl S, Chan K, Ng FL, Gor S, et al. ADAMTS7 cleavage and vascular smooth muscle cell migration is affected by a coronary-artery-disease-associated variant. The American Journal of Human Genetics (2013) 92(3):366–74. doi: 10.1016/j.ajhg.2013.01.012
51. Miller CL, Haas U, Diaz R, Leeper NJ, Kundu RK, Patlolla B, et al. Coronary heart disease-associated variation in TCF21 disrupts a miR-224 binding site and miRNA-mediated regulation. PLoS Genet (2014) 10(3):e1004263. doi: 10.1371/journal.pgen.1004263
52. Nurnberg ST, Cheng K, Raiesdana A, Kundu R, Miller CL, Kim JB, et al. Coronary artery disease associated transcription factor TCF21 regulates smooth muscle precursor cells that contribute to the fibrous cap. PLoS Genet (2015) 11(5):e1005155. doi: 10.1371/journal.pgen.1005155
53. Miller CL, Pjanic M, Wang T, Nguyen T, Cohain A, Lee JD, et al. Integrative functional genomics identifies regulatory mechanisms at coronary artery disease loci. Nat Commun (2016) 7:12092. doi: 10.1038/ncomms12092
54. Haitjema S, Meddens CA, van der Laan SW, Kofink D, Harakalova M, Tragante V, et al. Additional candidate genes for human atherosclerotic disease identified through annotation based on chromatin organization. Circ Cardiovasc Genet (2017) 10(2):e001664. doi: 10.1161/CIRCGENETICS.116.001664
55. GTEx Consortium. The Genotype-Tissue Expression (GTEx) project. Nat Genet (2013) 45(6):580–5. doi: 10.1038/ng.2653
56. Encode Project Consortium. An integrated encyclopedia of DNA elements in the human genome. Nature (2012) 489(7414):57–74. doi: 10.1038/nature11247
57. Kundaje A, Meuleman W, Ernst J, Bilenky M, Yen A, Heravi-Moussavi A, et al. Integrative analysis of 111 reference human epigenomes. Nature (2015) 518(7539):317–30. doi: 10.1038/nature14248
58. Paternoster L, Tilling K, Davey Smith G. Genetic epidemiology and Mendelian randomization for informing disease therapeutics: Conceptual and methodological challenges. PLoS Genet (2017) 13(10):e1006944. doi: 10.1371/journal.pgen.1006944
59. Jansen H, Samani NJ, Schunkert H. Mendelian randomization studies in coronary artery disease. Eur Heart J (2014) 35(29):1917–24. doi: 10.1093/eurheartj/ehu208
60. Lawlor DA, Harbord RM, Sterne JAC, Timpson N, Davey Smith G. Mendelian randomization: Using genes as instruments for making causal inferences in epidemiology. Stat Med (2008) 27(8):1133–63. doi: 10.1002/sim.3034
61. Varbo A, Benn M, Smith GD, Timpson NJ, Tybjaerg-Hansen A, Nordestgaard BG. Remnant cholesterol, low-density lipoprotein cholesterol, and blood pressure as mediators from obesity to ischemic heart disease. Circ Res (2014).
62. Do R, Willer CJ, Schmidt EM, Sengupta S, Gao C, Peloso GM, et al. Common variants associated with plasma triglycerides and risk for coronary artery disease. Nat Genet (2013) 45(11):1345–52. doi: 10.1038/ng.2795
63. Clarke R, Peden JF, Hopewell JC, Kyriakou T, Goel A, Heath SC, et al. Genetic variants associated with Lp(a) lipoprotein level and coronary disease. N Engl J Med Overseas Ed (2009) 361(26):2518–28. doi: 10.1056/NEJMoa0902604
64. Voight BF, Peloso GM, Orho-Melander M, Frikke-Schmidt R, Barbalic M, Jensen MK, et al. Plasma HDL cholesterol and risk of myocardial infarction: a mendelian randomisation study. Lancet (2012) 380(9841):572–80. doi: 10.1016/S0140-6736(12)60312-2
65. Holmes MV, Asselbergs FW, Palmer TM, Drenos F, Lanktree MB, Nelson CP, et al. Mendelian randomization of blood lipids for coronary heart disease. Eur Heart J (2015) 36(9):539–50. doi: 10.1093/eurheartj/eht571
66. Helgadottir A, Gretarsdottir S, Thorleifsson G, Hjartarson E, Sigurdsson A, Magnusdottir A, et al. Variants with large effects on blood lipids and the role of cholesterol and triglycerides in coronary disease. Nat Genet (2016) 48(6):634–9. doi: 10.1038/ng.3561
67. Kingwell BA, Chapman MJ, Kontush A, Miller NE. HDL-targeted therapies: progress, failures and future. Nat Rev Drug Discov (2014) 13(6):445–64. doi: 10.1038/nrd4279
68. Tall AR, Rader DJ. Trials and Tribulations of CETP Inhibitors. Circ Res (2018) 122(1):106–12. doi: 10.1161/CIRCRESAHA.117.311978
69. Rosenson RS, Koenig W. Mendelian randomization analyses for selection of therapeutic targets for cardiovascular disease prevention: a note of circumspection. Cardiovasc Drugs Ther (2016) 30(1):65–74. doi: 10.1007/s10557-016-6642-9
70. Kleber ME, Delgado G, Grammer TB, Silbernagel G, Huang J, Kramer BK, et al. Uric acid and cardiovascular events: a mendelian randomization study. Clin J Am Soc Nephrol (2015) 26(11):2831–8. doi: 10.1681/ASN.2014070660
71. Gill D, del Greco MF, Walker AP, Srai SKS, Laffan MA, Minelli C. The effect of iron status on risk of coronary artery disease: a mendelian randomization study-brief report. Arterioscler Thromb Vasc Biol (2017) 37(9):1788–92.
72. Emdin CA, Khera AV, Natarajan P, Klarin D, Zekavat SM, Hsiao AJ, et al. Genetic association of waist-to-hip ratio with cardiometabolic traits, type 2 diabetes, and coronary heart disease. JAMA (2017) 317(6):626–34. doi: 10.1001/jama.2016.21042
73. So HC, Chau CK, Chiu WT, Ho KS, Lo CP, Yim SH, et al. Analysis of genome-wide association data highlights candidates for drug repositioning in psychiatry. Nat Neurosci (2017) 20(10):1342–9. doi: 10.1038/nn.4618
74. Lamb J, Crawford ED, Peck D, Modell JW, Blat IC, Wrobel MJ, et al. The connectivity map: using gene-expression signatures to connect small molecules, genes, and disease. Science (2006) 313(5795):1929–35. doi: 10.1126/science.1132939
75. Subramanian A, Narayan R, Corsello SM, Peck DD, Natoli TE, Lu X, et al. A next generation connectivity map: L1000 platform and the first 1,000,000 profiles. Cell (2017) 171(6):e17:1437–52. doi: 10.1016/j.cell.2017.10.049
76. Breen G, Li Q, Roth BL, O'Donnell P, Didriksen M, Dolmetsch R, et al. Translating genome-wide association findings into new therapeutics for psychiatry. Nat Neurosci (2016) 19(11):1392–6. doi: 10.1038/nn.4411
77. Pritchard J-LE, O’Mara TA, Glubb DM. Enhancing the promise of drug repositioning through genetics. Front Pharmacol (2017) 8:896. doi: 10.3389/fphar.2017.00896
78. Gamazon ER, Wheeler HE, Shah KP, Mozaffari SV, Aquino-Michaels K, Carroll RJ, et al. A gene-based association method for mapping traits using reference transcriptome data. Nat Genet (2015) 47(9):1091–8. doi: 10.1038/ng.3367
79. Gusev A, Ko A, Shi H, Bhatia G, Chung W, Penninx BWJH, et al. Integrative approaches for large-scale transcriptome-wide association studies. Nat Genet (2016) 48(3):245–52. doi: 10.1038/ng.3506
80. Barbeira A, Dickinson S, Torres J, Bonazzola R, Zheng J, Torstenson E. Exploring the phenotypic consequences of tissue specific gene expression variation inferred from GWAS summary statistics (2017). Available from: https://www.biorxiv.org/content/early/2017/10/03/045260.
81. Shu L, Zhao Y, Kurt Z, Byars SG, Tukiainen T, Kettunen J, et al. Mergeomics: multidimensional data integration to identify pathogenic perturbations to biological systems. BMC Genomics (2016) 17(1):874. doi: 10.1186/s12864-016-3198-9
82. Talukdar HA, ForoughiAsl H, Jain RK, Ermel R, Ruusalepp A, Franzén O, et al. Cross-Tissue Regulatory Gene Networks in Coronary Artery Disease. Cell Syst (2016) 2(3):196–208. doi: 10.1016/j.cels.2016.02.002
83. Lempiäinen H, Brænne I, Michoel T, Tragante V, Vilne B, Webb TR, et al. Network analysis of coronary artery disease risk genes elucidates disease mechanisms and druggable targets. Sci Rep (2018) 8(1):3434. doi: 10.1038/s41598-018-20721-6
84. Ghosh S, Vivar J, Nelson CP, Willenborg C, Segrè AV, Mäkinen V-P, et al. Systems genetics analysis of genome-wide association study reveals novel associations between key biological processes and coronary artery disease. Arterioscler Thromb Vasc Biol (2015) 35(7):1712–22. doi: 10.1161/ATVBAHA.115.305513
85. Bartha I, di Iulio J, Venter JC, Telenti A. Human gene essentiality. Nat Rev Genet (2018) 19(1):51–62. doi: 10.1038/nrg.2017.75
86. Zhang B, Zhu J, editors. “Identification of key causal regulators in gene networks,” in Proceedings of the World Congress on Engineering. (2013).
87. Franzen O, Ermel R, Cohain A, Akers NK, di Narzo A, Talukdar HA, et al. Cardiometabolic risk loci share downstream cis- and trans-gene regulation across tissues and diseases. Science (2016) 353(6301):827–30. doi: 10.1126/science.aad6970
88. Heath JR, Ribas A, Mischel PS. Single-cell analysis tools for drug discovery and development. Nat Rev Drug Discov (2016) 15(3):204–16. doi: 10.1038/nrd.2015.16
89. Regev A, Teichmann SA, Lander ES, Amit I, Benoist C, Birney E, et al. The Human Cell Atlas. eLife (2017) 6. doi: 10.7554/eLife.27041
90. Akbarian S, Liu C, Knowles JA, Vaccarino FM, Farnham PJ, Crawford GE, et al. The PsychENCODE project. Nat Neurosci (2015) 18(12):1707–12. doi: 10.1038/nn.4156
91. BrainSeq AHBGC. BrainSeq: neurogenomics to drive novel target discovery for neuropsychiatric disorders. Neuron (2015) 88(6):1078–83. doi: 10.1016/j.neuron.2015.10.047
Keywords: genome-wide association study, coronary artery disease, drug targets, multi-omics, functional genomics, networks
Citation: Shu L, Blencowe M and Yang X (2018). Translating GWAS Findings to Novel Therapeutic Targets for Coronary Artery Disease. Front. Cardiovasc. Med. 5:56. doi: 10.3389/fcvm.2018.00056
Received: 20 March 2018; Accepted: 11 May 2018;
Published: 30 May 2018
Reviewed by:
Sander W. van der Laan, University Medical Center Utrecht, NetherlandsBaiba Vilne, Technische Universität München, Germany
Copyright © 2018 Shu, Blencowe and Yang. This is an open-access article distributed under the terms of the Creative Commons Attribution License (CC BY). The use, distribution or reproduction in other forums is permitted, provided the original author(s) and the copyright owner are credited and that the original publication in this journal is cited, in accordance with accepted academic practice. No use, distribution or reproduction is permitted which does not comply with these terms.
*Correspondence: Xia Yang, Ph.D. eHlhbmcxMjNAdWNsYS5lZHU=