- 1Alaska Fisheries Science Center, National Marine Fisheries Service, National Oceanic and Atmospheric Administration, Seattle, WA, United States
- 2Joint Institute for the Study of the Atmosphere and Ocean, University of Washington, Seattle, WA, United States
- 3Pacific Marine Environmental Laboratory, Oceans and Atmospheric Research, National Oceanic and Atmospheric Administration, Seattle, WA, United States
- 4School of Aquatic and Fishery Science, College of the Environment, University of Washington, Seattle, WA, United States
- 5Centre for Marine Socioecology, Institute for Marine and Antarctic Studies, College of Sciences and Engineering, University of Tasmania, Hobart, TAS, Australia
The Alaska Climate Integrated Modeling (ACLIM) project represents a comprehensive, multi-year, interdisciplinary effort to characterize and project climate-driven changes to the eastern Bering Sea (EBS) ecosystem, from physics to fishing communities. Results from the ACLIM project are being used to understand how different regional fisheries management approaches can help promote adaptation to climate-driven changes to sustain fish and shellfish populations and to inform managers and fishery dependent communities of the risks associated with different future climate scenarios. The project relies on iterative communications and outreaches with managers and fishery-dependent communities that have informed the selection of fishing scenarios. This iterative approach ensures that the research team focuses on policy relevant scenarios that explore realistic adaptation options for managers and communities. Within each iterative cycle, the interdisciplinary research team continues to improve: methods for downscaling climate models, climate-enhanced biological models, socio-economic modeling, and management strategy evaluation (MSE) within a common analytical framework. The evolving nature of the ACLIM framework ensures improved understanding of system responses and feedbacks are considered within the projections and that the fishing scenarios continue to reflect the management objectives of the regional fisheries management bodies. The multi-model approach used for projection of biological responses, facilitates the quantification of the relative contributions of climate forcing scenario, fishing scenario, parameter, and structural uncertainty with and between models. Ensemble means and variance within and between models inform risk assessments under different future scenarios. The first phase of projections of climate conditions to the end of the 21st century is complete, including projections of catch for core species under baseline (status quo) fishing conditions and two alternative fishing scenarios are discussed. The ACLIM modeling framework serves as a guide for multidisciplinary integrated climate impact and adaptation decision making in other large marine ecosystems.
Introduction
Significant increases in sea surface temperature (SST) over the next century are projected for most ocean systems (IPCC, 2014, 2018). Global warming is expected to have strong impacts on ocean temperature and ocean productivity in high latitude systems (Arrigo and Van Dijken, 2015; Smith et al., 2019). However, the effect of warming climate conditions on marine ecosystems and species are expected to be system- and species-dependent, and the footprint of environmental change may exhibit considerable variation across space and time (Poloczanska et al., 2013; Cheung et al., 2016; Spencer et al., 2019). High latitude marine ecosystems such as the Bering Sea are expected to experience large deviations from historical ocean conditions (Hermann et al., 2019; Spencer et al., 2019). Indeed, increased ocean temperature has already impacted the Bering Sea marine ecosystem through shifts in trophic demand and overwinter survival (Heintz et al., 2013), species interactions (Spencer et al., 2016), shifting spatial distributions (Barbeaux and Hollowed, 2018; Stevenson and Lauth, 2019; Thorson, 2019), and overall system productivity (IPCC, 2014; Hermann et al., 2019). Bering Sea ecosystems are also threatened by the effects of ocean acidification on valuable crab stocks and important pelagic prey species (Comeau et al., 2010; Long et al., in press).
In anticipation of these changes, the US National Marine Fisheries Service (NMFS) established a Climate Science Strategy (NCSS) that called for scientists from each management region to conduct research to understand, prepare for, and respond to, climate impacts on the distribution and abundance of managed species and the ecosystems in which they reside (Buser et al., 2016). In response to this national call to action, an interdisciplinary team of researchers was formed in 2015 to develop the Alaska Climate Integrated Modeling (ACLIM) projec1. The goals of ACLIM were to:
• Identify key risks to eastern Bering Sea (EBS) fisheries, and the region’s marine social-ecological system associated with various future levels of climate-driven change.
• Evaluate climate-resilient adaptation pathways and identify and avoid maladaptive approaches (sensu Wise et al., 2014).
• Identify sources of uncertainty in risk and projected changes in order to inform future research and monitoring to improve projections and advice.
We addressed these goals by: (1) applying a multi-model approach (sensu Kaplan et al., 2019; Lotze et al., 2019) to project the distribution and abundance of commercially important fish and fisheries in the EBS under various climate change and fishing scenarios (Figure 1); (2) evaluating the economic and biological performance of the fishing scenarios for consideration by the North Pacific Fishery Management Council (NPFMC), the federal fisheries management body for the region (sensu Holsman et al., 2019); and (3) decomposing uncertainty in future climate projections according to structural, scenario, and parameter uncertainty sources (Cheung et al., 2016; Payne et al., 2016; Reum et al., in press).
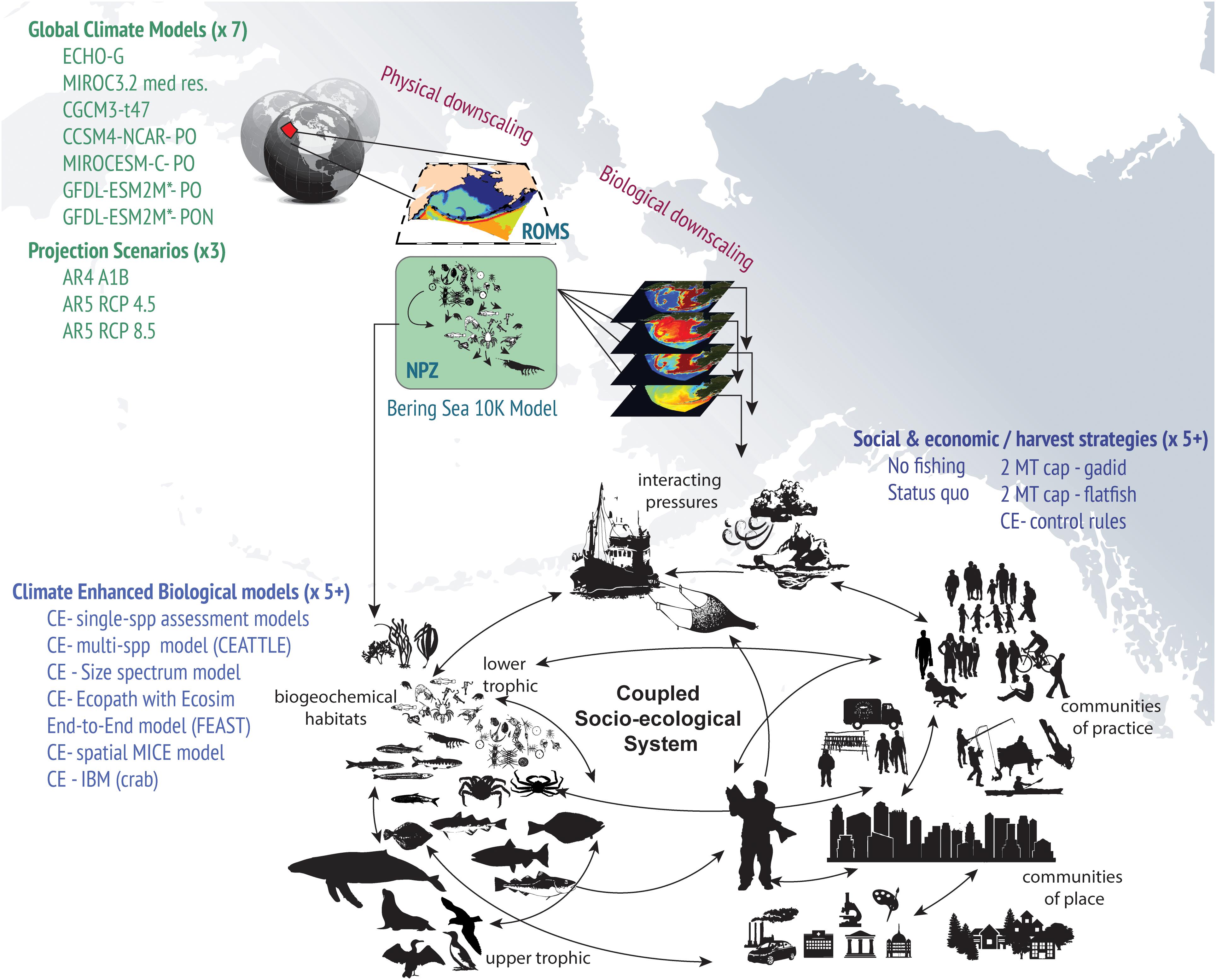
Figure 1. Illustration of the elements of ACLIM’s integrated modeling enterprise for evaluating climate change impacts on socio-ecological systems in Alaska.
This paper describes the ACLIM research framework, its approach to quantifying uncertainty and multi-model inference, and the program’s approach to interfacing science with regional fishery management councils. This paper is designed to provide an overview of the research framework. For in-depth details of each modeling approach, the reader is directed to relevant publications.
History
The ACLIM Team selected the EBS as a case study for the development and implementation of a regional climate impact, assessment, and management planning enterprise. The EBS supports abundant fish and shellfish resources that are of considerable economic and social value to the region, the United States, and the world. For example, the estimated 2017 first-wholesale value for commercial harvest of all species (crab, groundfish, clams, scallops, salmon, halibut) in the United States shelf and slope regions of the EBS was $2.68 billion (Fissel et al., 2019). In addition, the major physical and biogeochemical processes governing ecosystem production have been studied for at least 40 years, providing opportunities for formulation and parameterization of the responses of marine species to changes in atmospheric, oceanographic, and biogeochemical drivers (Sigler et al., 2016b; Stabeno et al., 2016). An Ecosystem Approach to Fisheries Management (EAFM, Dolan et al., 2015) is used in the region (Stram and Evans, 2009) and managers and stakeholders are actively seeking improved climate- and ecosystem-based information for decision making.
Key features of the EBS include: seasonal ice cover, distinct biophysical domains driven by surface forcing and tidal mixing, ice associated algal and phytoplankton blooms, and fall phytoplankton blooms (Hunt et al., 2011; Stabeno et al., 2012, 2017; Wang et al., 2012; Baker and Hollowed, 2014; Cheng et al., 2015; Hermann et al., 2019). The role of temperature and sea ice on the seasonal availability of high energy content planktonic prey (large zooplankton) has been shown to be associated with overwintering survival of walleye pollock (Gadus chalcogrammus) and Pacific cod (Gadus macrocephalus), two abundant and economically important species (Heintz et al., 2013; Duffy-Anderson et al., 2017). Laboratory studies have quantified key bioenergetic responses for commercially important groundfish and the impacts of ocean acidification on key developmental processes of commercially important crab stocks (Long et al., 2013, 2019).
The origins of the ACLIM project can be traced back to a long legacy of interdisciplinary research programs. Early versions of the current high spatial and temporal resolution oceanographic model [a Regional Ocean Modeling System (ROMS) model with 10 km horizontal resolution for the Bering Sea, Bering10K] were developed as part of the US GLOBEC Northeast Pacific program, a partnership between the National Science Foundation (NSF) and the National Oceanic and Atmospheric Administration (NOAA) (Curchitser et al., 2005). Early food web models were developed as part of the Southeast Bering Sea Carrying Capacity research program, a partnership between the Coastal Ocean Program, the Pacific Marine Environmental Laboratory, and the NMFS (Aydin et al., 2007). Development of climate-enhanced single-species projection models (CE-SSM, Ianelli et al., 2016) and fully coupled end-to-end ecosystem models (Hermann et al., 2013, 2016; Ortiz et al., 2016), and fisher’s choice models (Haynie and Pfeiffer, 2013) were all developed as part of the Bering Sea Project (BSP); a partnership between the NSF, the North Pacific Research Board (NPRB), and the NOAA (Wiese et al., 2012). The Climate-Enhanced Age-based model with Temperature-specific Trophic Linkages and Energetics (CEATTLE, Holsman et al., 2016) was funded directly by NMFS research initiatives focused on the development of integrated ecosystem assessments and stock assessment improvement. This legacy of collaborative research led to a mechanistic understanding of key biophysical linkages governing fish production (Sigler et al., 2016a) and completed model performance verification studies that served as the foundation for the ACLIM project. Briefly, the ACLIM framework generates dynamically coupled, regionally downscaled projections of the oceanography and biogeochemistry of the EBS ecosystem derived from earth system models (ESMs) driven under contrasting future emission scenarios [representative concentration pathways (RCPs)] (Table 1). Recognizing that there are strengths and weaknesses to every modeling approach (e.g., Hollowed et al., 2013), investigators proposed a multi-model inter-comparison approach (Stock et al., 2011; Stouffer et al., 2017). Projected ocean and biogeochemical conditions are directly or indirectly utilized to project the future of marine species and fisheries in the region (Figure 1 and Table 1) using a suite of population dynamics models with various levels of complexity (Figure 2 and Table 2).
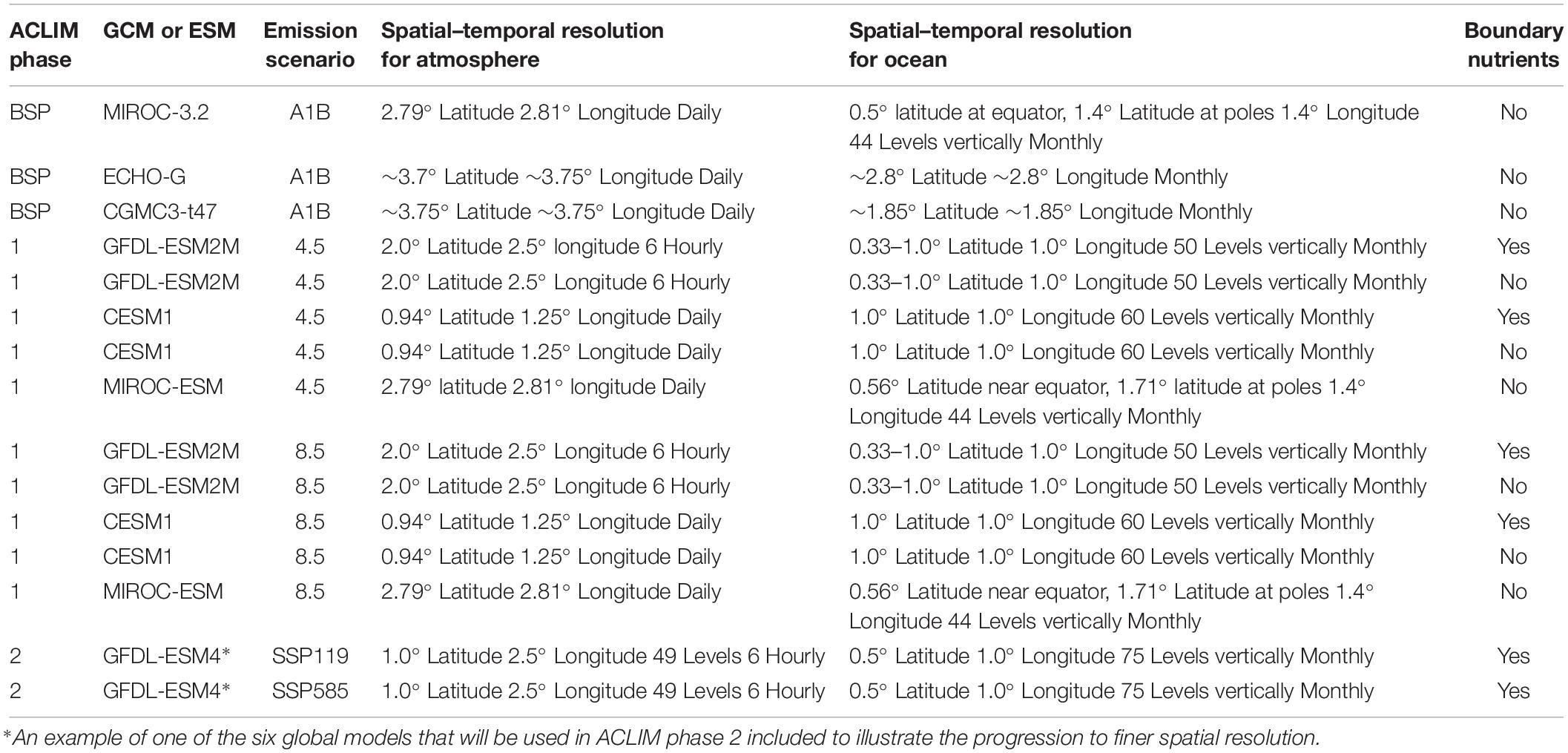
Table 1. Summary of global or earth system models and scenarios used in ACLIM and Bering Sea Project (BSP) experiments.
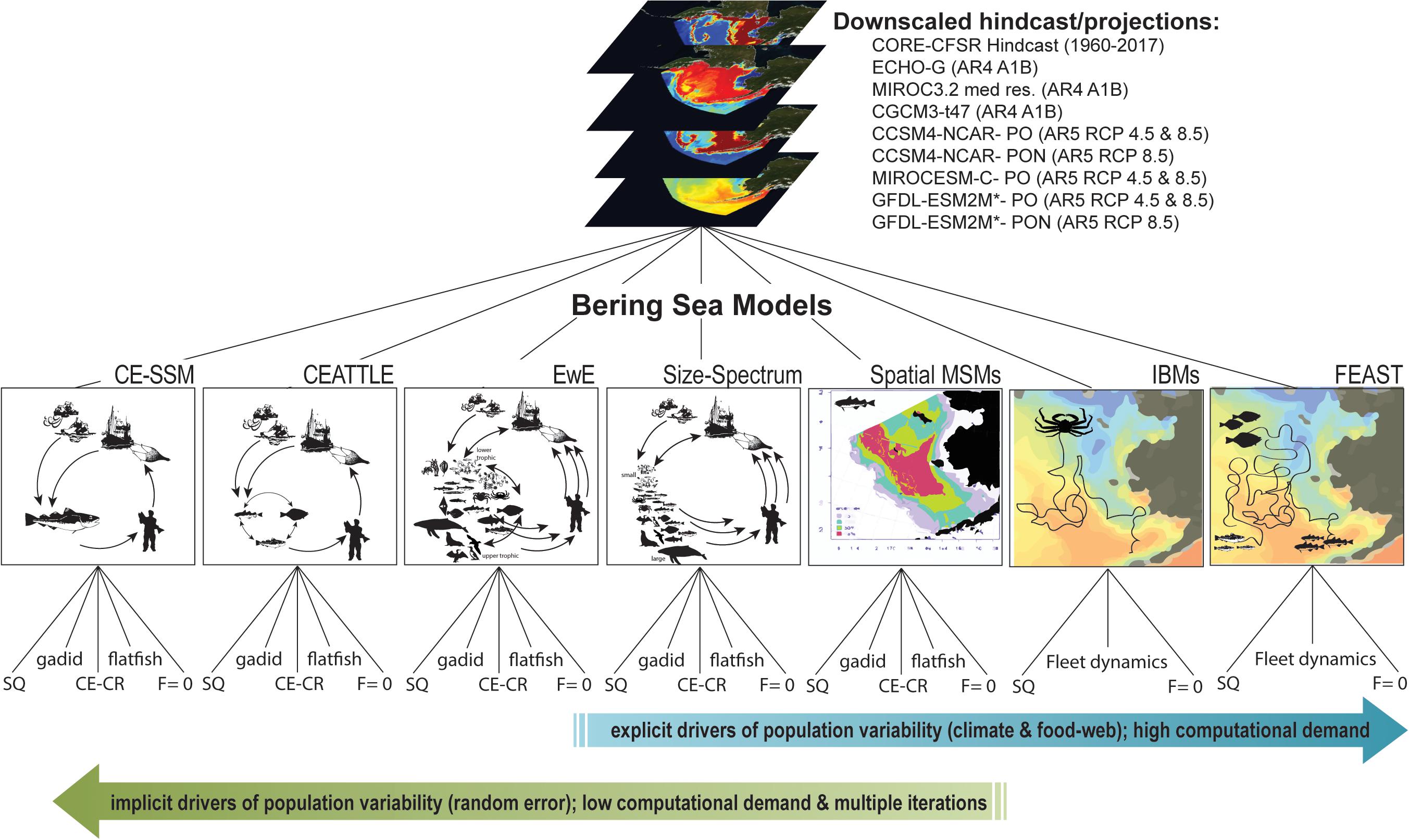
Figure 2. Illustration of the multiple models and climate and fishing scenarios used in the ACLIM project.
Using the multi-model approach, projections of fish and shellfish distribution and abundance are assessed for the current (2006–2020), mid-century (2030–2050), and end-of-century (2080–2100) time periods under suites of potentially viable fishing scenarios and management strategies that are vetted through the NPFMC. Projected stock conditions (e.g., size-at-age, abundance, reproductive potential, reproductive success, and distribution) for six core species (walleye pollock; Pacific cod; yellowfin sole, Limanda aspera; northern rock sole, Lepidopsetta polyxystra; arrowtooth flounder, Atheresthes stomias; and snow crab, Chionoecetes opilio) are compared to assess the performance of current and alternative fishing scenarios with respect to the ecosystem, social and economic goals of the NPFMC. Projections of key ecosystem status indicators (e.g., species diversity, mean trophic level of the catch) are derived from ecosystem models and are evaluated under current and alternative fishing scenarios. In this context, fishing scenarios include both the suite of constraints imposed by a given fisheries management strategy and external processes influencing fishing behavior [e.g., allocation of total allowable catch (TAC) between fishing sectors, fuel costs, world markets] (Groeneveld et al., 2018; Fulton et al., 2019). The implications of shifting spatial distributions of commercial species on the coupled social-ecological system are assessed using spatially explicit models (Table 2). Collectively, these projections provide the scientific information needed to identify thresholds for management action and viable adaptation strategies. For example, many regions monitor proxies for reproductive potential (i.e., spawning stock biomass) and establish biological reference points for reductions in fishing mortality or the development of rebuilding plans. Examination of the performance of current and alternative fishing scenarios and management strategies helps to identify climate-ready harvest control rules that are robust to changing climate, and to inform the public and management of trade-offs associated with different options for the management of marine resources under a changing climate.
The origins of the EAFM approach used in the region and the keen interest of managers and stakeholders in improved climate- and ecosystem-based information for decision making can be traced to the iterative communication between scientists, managers, and stakeholders. The NPFMC was one of the first Councils in the United States to adopt an ecosystem considerations report (Livingston et al., 2001). This report has evolved over the years and is now considered an integral part of the NPFMC’s annual assessment reviews (Zador et al., 2017) and in 2018, the NPFMC adopted a Fishery Ecosystem Plan for the Bering Sea2. The shared recognition of the scientific and management community of the potential risks of changing climate conditions on sustainable fishery management in the region underscored the need for a climate module within the FEP which would serve as a strategic planning tool for the NPFMC. The ACLIM modeling framework is designed to fill this strategic need.
Climate Model Structural Uncertainty and Emission Scenarios
Projections are completed in phases that are tied to the availability of updated ESM projections and funding. Phase 1 is nearing completion. Phase 1 utilized output from six ESMs developed for the IPCC fourth or fifth Assessment Reports (based on output from the third or fifth Coupled Model Intercomparison Project, “CMIP3” or “CMIP5”) (Table 1). In this phase, the ACLIM framework was developed and tested, providing multi-model projections of the impacts on fish, invertebrates, and fisheries under status quo and two fishing scenarios. In phase 2, the entire suite of biological models (Table 2) will be updated with ESM output developed for the IPCC Sixth Assessment Report (i.e., CMIP6) and evaluated under an expanded suite of fishing scenarios that will include alternative management strategies. Comparison of results from phase 1 and 2 will allow analysts to explore how improvements in ESMs affect projected impacts on fish and fisheries. This paper focuses on phase 1 of the project.
Structural differences among global climate models and uncertainty regarding emission scenarios were addressed in phase 1 of the ACLIM project by comparing outcomes based on multiple climate models under several emission scenarios (Van Vuuren et al., 2011; Van Vuuren and Carter, 2014; Table 1). In this phase, output from six global climate models (three from CMIP3 and three from CMIP5) were selected from the full suite of global climate models considered by IPCC (Table 1). The CMIP3 suite was selected during the BSP to span a broad range of potential sea ice dynamics (Hermann et al., 2016). Selected models from the CMIP5 suite included: the Geophysical Fluid Dynamics Laboratory (GFDL) – ESM 2M (ESM2M) (Dunne et al., 2012); the National Center for Atmospheric Research (NCAR) Community Earth Systems Model (CESM) (Kay et al., 2015); and the MIROC ESM (Watanabe et al., 2011) (Table 1). These three models were selected because they projected a broad range of global patterns for precipitation and SST, and provided contrasting views of future ocean conditions in the EBS. Output from these models under two RCPs (4.5 and 8.5; Van Vuuren et al., 2011; Van Vuuren and Carter, 2014) were used to drive the Bering10K regional model. RCP 8.5 and 4.5 represent a high-emission business-as-usual scenario and an intermediate scenario, respectively.
Climate Projection Downscaling
Previous analysis of the skill of global scale ESMs over the historical period revealed that these coarse resolution models are unable to adequately resolve the seasonal spatial patterns of sea ice extent and bottom water temperatures that are key structural features of the EBS shelf (Vancoppenolle et al., 2013). Additionally, model intercomparisons of 21 global biogeochemical models’ abilities to reproduce observed net primary productivity in the Arctic Ocean revealed several limitations that varied by region (Lee et al., 2016). Many of these deficiencies related to mixed layer depth and sea ice concentration in the simulations.
To address these potential deficiencies, the ACLIM framework deploys the Bering10K ROMS model (Hermann et al., 2016, 2019) to dynamically downscale CMIP5 projection simulations for the Bering Sea region. In this framework, the ROMS ocean model is forced at the surface by heat fluxes, freshwater fluxes, and wind stress values derived from prescribed atmospheric states based on the global model projections and modeled surface temperature (SST), and at the lateral boundaries by temperature, salinity, and current speeds from the ocean component of the global model projections. In two simulations, nitrate and ammonium values from the biogeochemical component of the ocean model in the global projections were also used in the lateral boundary condition variables; in these instances, simulations were run under projected nutrient boundary conditions and alternatively with World Ocean Atlas-derived climatological nutrient boundary conditions to contrast the relative impact of temporal trends in projected nutrients. This downscaling framework allows for approximately a 100-fold increase in the number of horizontal grid points compared to that of the global models (Figure 3). The Bering10K model also includes its own sea ice and biogeochemical models through which the climate model forcing data influence the local dynamics. When forced in hindcast mode with surface and lateral boundary conditions from the data-assimilating Climate Forecast System Reanalysis (CFSR), the Bering10K model has demonstrated significantly improved representation of features such as advection pathways, mixed layer depth, sea ice extent, and the strength and interannual variability of the Bering Sea cold pool compared to that seen in CFSR itself (Hermann et al., 2016; Kearney et al., in press).
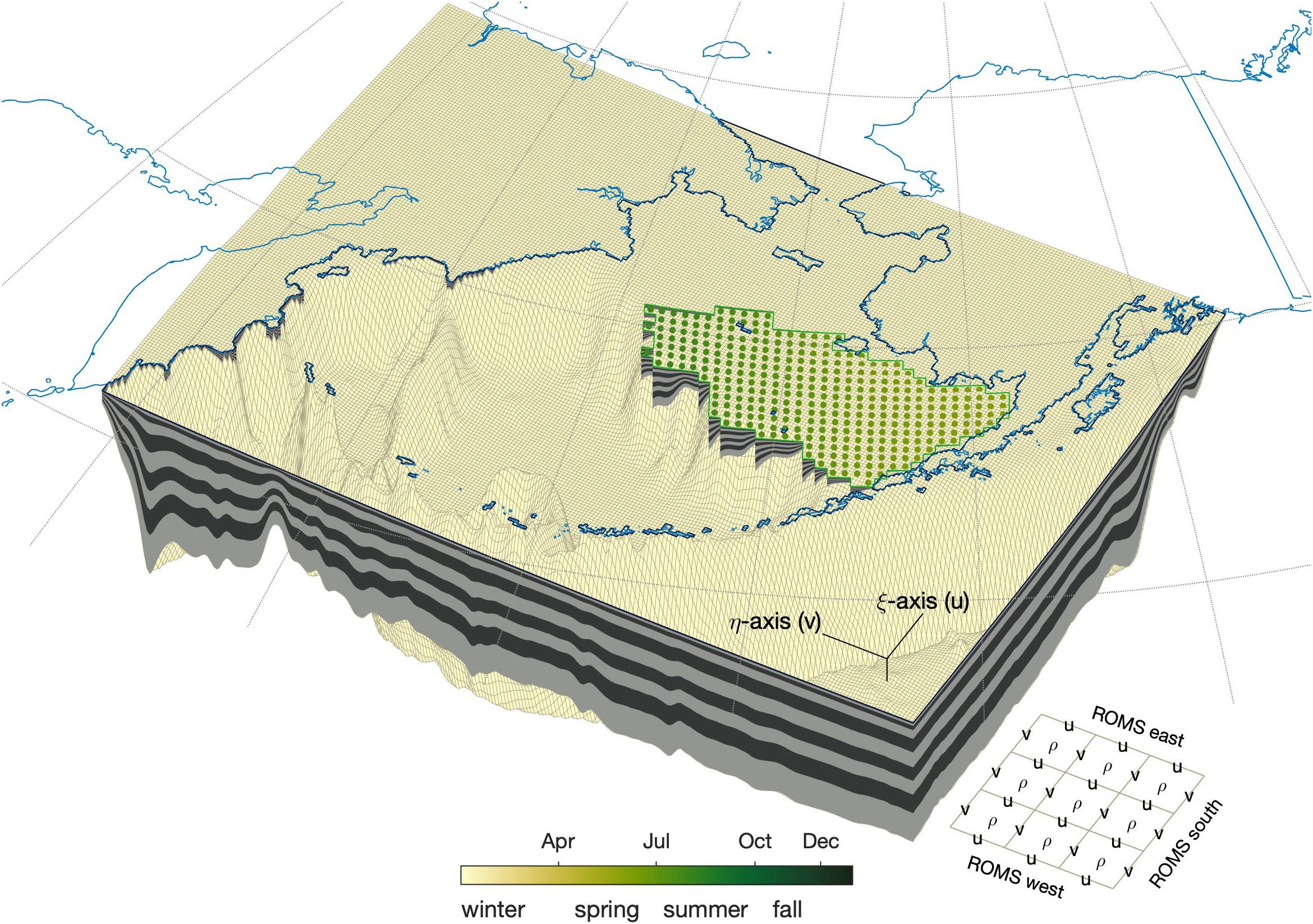
Figure 3. Geometry of the ROMS domain. The model domain grid is laid along the bathymetry with the native 10-km resolution of the ROMS grid. Real coastlines are in light blue with the ROMS land mask in a darker blue. The EBS sampling polygon is shown in green, along with the locations used for survey-replication sampling (colors indicate the day of year associated with each sampling location; the color-bar further shows the season subdivisions used for seasonal indices). The zoomed-in grid in the lower right shows the orientation of the grid as well as the location of rho-, u-, and v-points.
Since phytoplankton and zooplankton are responding to both physics and nutrients, the Bering10K ocean model is coupled to a biogeochemical model (BESTNPZ) that simulates lower trophic level dynamics for the Bering Sea (Gibson and Spitz, 2011; Kearney et al., in press). Within the ACLIM framework, the use of this single biogeochemical model to derive biological indices for all the downscaled climate simulations is the one place where neither structural nor parameter uncertainty is addressed via an ensemble approach. During development of the ACLIM modeling framework, the merits of including multi-model- or parameter-varying ensembles of biogeochemical models were discussed but ultimately not included due to the need to limit the permutations assessed in the project. Therefore, for phase 1, the only uncertainty related to lower trophic dynamics that is quantified is that relating to projected trends in nutrient boundary conditions.
Relevant biogeochemical and physical properties (Tables 3–5) were projected for the period 2006–2100 for scenarios based on CMIP5 models. Since CMIP3 did not cover the entire time period 2006–2100, we used CMIP3 for those periods to the extent possible (2003–2040). In addition, a hindcast simulation spanning the period of 1970–2018, forced by a combination of version 2 forcing for Coordinated Ocean-Ice Reference Experiment, i.e., COREII (Large and Yeager, 2009) (1970–1994), the CFSR (Saha et al., 2010) (1995–March 2011), and the Climate Forecast System Operational Analysis, i.e., CFSv2-OA (April 2011–Sep 2018) was performed for use in calibrating the various upper trophic level models for past decades. Comparison of these hindcasts using different ESM boundary conditions revealed potential temperature biases. Several methods have been used to address systematic biases in global model temperatures relative to current observed temperatures (Piani et al., 2010). In comparative studies, bias can be accounted for by evaluating relative changes in mean state between time periods (Hermann et al., 2019). However, when animals respond to environmental thresholds, relative environmental changes may not be adequate when downscaled variables are used to drive responses of secondary producers and higher trophic levels (Small et al., 2015; see section “Bias Corrections for Biological Responses”). To address this issue, projections with and without bias corrections are compared.
Explorations of the Potential Role of Biological Complexity
The ACLIM framework employs a multi-model approach for projection of biological responses to explore the trade-offs between computational speed and ecosystem realism inherent in the selection of higher trophic level models (Hollowed et al., 2013). When models of varying complexity are considered jointly (some with high spatial resolution and species interactions and others with well-defined distributions for key parameters), multi-model projections can provide a more complete suite of future projections for evaluating climate change impacts on ecosystems and resource-dependent human communities (Plagányi et al., 2011; Tittensor et al., 2018). Within the ACLIM framework the suite of models range from minimally realistic single-species climate-enhanced stock projection models (CE-SSM) that are capable of detailed treatment of process error and measurement error, to whole ecosystem models that track potential structural changes within the ecosystem that may emerge from complex ecosystem interactions (Figure 2 and Tables 2, 5; Plagányi et al., 2011; Stock et al., 2011). The diverse multi-model projection suite provides a reasonable range of representative futures (with sufficient contrast in climate scenarios) that can be used to evaluate short- and long-term implications of management actions under future climate change.
The ACLIM framework leveraged eight types of stock or ecosystem projection models (Table 2):
• Trait-based vulnerability analyses (Hare et al., 2016; Spencer et al., 2019). The VA model utilizes expert judgment to assess sensitivity, exposure and vulnerability to climate change and does not project the specific outputs shown in Table 5.
• Climate-enhanced single species stock projection models (Wilderbuer et al., 2013; Ianelli et al., 2016; Spencer et al., 2016);
• Climate-enhanced multi-species stock projection models (Holsman et al., 2016);
• Individual-based models (sensu Rose et al., 2015);
• Foodweb models using Ecopath with Ecosim (Aydin et al., 2007);
• Multi-species size-spectrum models based on the R package “MIZER” (Scott et al., 2014; Reum et al., 2019);
• End-to-end model [Forage Euphausiid Abundance in Space and Time (FEAST)] (Ortiz et al., 2016);
• VAST-MICE: Spatiotemporal models of intermediate complexity for ecosystems (MICE) using the vector autoregressive spatiotemporal (VAST) package (Thorson et al., 2019).
The ACLIM framework enables analysts to evaluate the contributions of different sources uncertainty. The inclusion of MICE assessment (Plagányi et al., 2014) in the ACLIM framework provides opportunities to explore the contribution of process error and scenario uncertainty in single- and multi-species projections. Two MICE models in particular are included in ACLIM; the CEATTLE model (Holsman et al., 2016), and a CE-version of the spatiotemporal models of intermediate complexity for ecosystems (i.e., “MICE-in-space” model; Thorson et al., 2019). These models can be run relatively quickly, allowing sensitivity testing of the implications of uncertainty in climate linkages to: predator–prey overlap (and hence mortality rates); prey switching, prey availability, and metabolic rates (growth and maturation rates); and reproductive success (via the spawner–recruit relationships). Of these processes, the linkages between climate variability and future fish production are the most influential in terms of projected stock status and the most challenging to parameterize correctly (Szuwalski et al., 2015) because the processes governing climate impacts on fish and crabs are temporally varying and stage-specific (Bailey, 2000). The inclusion of food web, size spectrum, and end-to-end models provides an opportunity to evaluate the relative contributions of structural uncertainty, species interactions, fishing, and ecosystem changes to future states of nature.
Techniques for assessing the predictive skill of ecosystem models are emerging and they reveal a modest ability to reconstruct observed dynamics in stock status (Olsen et al., 2016). FEAST is a spatially explicit end-to-end ecosystem model that tracks core species in space and time (Table 2). Movements are determined from an evaluation of the relative quality of the current location with respect to foraging needs (demands on metabolic rate and prey availability) to adjacent cells within the ROMS Bering 10K grid (Figure 3). In long-term projections, small errors can accumulate in a spatial model of this complexity (Punt et al., 2016b). To address this issue, FEAST can be nudged by initiating the model using the projected environmental conditions at mid-century (2030–2050) and end of century (2080–2100) and seeding the starting abundance of core species using output from simpler single- or multi-species climate enhanced models (Table 1).
Bias Corrections for Biological Responses
A critical element of the ACLIM framework is the demonstration that the modeling suite used for projections is skillful in reconstructing observed population dynamics of core species and catch. To confirm this skill, it was necessary to demonstrate that when driven by hindcasts of observed oceanographic and biogeochemical conditions, the projected higher trophic level and fishing responses were consistent with observed historical interannual fluctuations. Functional forms and parameters used in the ACLIM were derived from a combination of retrospective studies external to the model (e.g., laboratory studies of metabolic rate or consumption, Holsman et al., 2016), retrospective data analysis based on observed data (e.g., climate envelope studies, Spencer et al., 2016), or retrospective analysis based on output from previous Bering10K hindcasts (e.g., spawner–recruitment relationships). In the case of CE-SSMs and the CE-MSM, environmentally linked age- or size- based statistical assessment models were used to derive functional forms and base parameters (see Holsman et al., 2016; Ianelli et al., 2016; Spencer et al., 2016 for examples). For all models, once parameterized, we drove our hindcast period (2006–2017) with reanalysis-based (e.g., Saha et al., 2010) and data-assimilating input products, which successfully tied our hindcast simulation time series to their real-world counterpart datasets. These hindcasts incorporate observed variations due to radiative forcing changes from natural and anthropogenic sources and internal natural variability.
When incorporating downscaled physical and biogeochemical indices (Tables 3–5) into hindcast-trained models, it was necessary to account for both systematic biases in each global model as well as mismatch at any given time due to internal variability of each model compared to the hindcast period. While we have identified methods to address the former, the latter is left unaddressed during phase 1 of the ACLIM simulations. The existing simulations include only a single decade of overlap (2006–2017) between the hindcast and projection simulations. This time period is not long enough to separate model bias from decadal variability due to internal oscillations such as ENSO or the PDO. For the phase 2 of ACLIM simulations, we will extend the downscaled climate model projections to include several decades from the historical period (i.e., 1970-present). This will allow for the diagnosis of model biases vs. the hindcast and observations, and allow for smoother forcing of the upper trophic level models as they cross the hindcast-to-projection threshold. Given the lack of a sufficient overlap period for phase 1 calculations, we assumed that the mean and variance during the reference overlap period are representative for both the hindcast and projections under present-day radiative conditions, and that conditions during the reference period are not anomalous.
Bias corrections must be considered carefully when projected environmental data are used to drive biological responses. Global climate model ensemble projections routinely apply additive bias correction (e.g., the “delta method”; Ho et al., 2012; Hawkins et al., 2013). The procedure adjusts projections based on mean differences between the hindcast and projection variable in a period of overlap. However, the procedure is not straightforward to apply to biological projections such as biomass densities that are bounded by zero because the resulting values can take negative values. Instead, a proportional correction can be applied. As with the additive correction method, the biomass density projection is re-centered based on the mean difference between the projection and hindcast overlap period, but the proportional change observed in the uncorrected projection (that is, variable level relative to the mean value from the overlap) is carried through to the re-centered projection (Buser et al., 2009; Haerter et al., 2011; Reum et al., in press).
Fishing Scenarios and Management Strategies
There are myriad pathways through which climate change can impact marine industries (Allison and Bassett, 2015). Bounding the range of possible management futures within the context of global shared socio-economic pathways is challenging and requires strong communication between management and modeling teams (O’neill et al., 2014; Groeneveld et al., 2018). In phase 1, a narrow suite of fishing scenarios was selected which represented two variations in TAC allocations across fishing sectors within the existing constraints of the NPFMC’s existing EAFM management strategy. These fishing scenarios reflected two alternatives to status quo that have a significant impact on stakeholders (Ono et al., 2017).
The NPFMC’s EAFM in the EBS employs a complex suite of management measures that are designed to sustain fisheries using science-based precautionary harvest control rules that are designed to sustain the reproductive potential of the stocks, preserve essential fish habitats, maintain a sustainable forage base for fish and other top trophic level consumers, and preserve ecosystem structure by limiting the overall removal of groundfish from the ecosystem (Stram and Evans, 2009; Hollowed et al., 2011). Under the US guidelines for the Magnuson Stevens Fishery Management Act, the TAC must be less than or equal to the Acceptable Biological Catch (ABC) and the combined TACs for federal groundfish fisheries in the Bering Sea Aleutian Island (BSAI) region cannot exceed the 2 million t system level cap. Groundfish fisheries are constrained by bycatch [Prohibited Species Caps (PSC)] that limit on non-groundfish species targeted by other commercial, recreational, and subsistence harvester (Pacific halibut, Hippoglossus stenolepis; Pacific herring, Clupea pallassi, salmon, and crab). The management system also includes catch share provisions and sector limitations designed to ensure that: a diverse suite of fishing sectors and communities have access; gear conflicts are avoided; and prey for protected species (such as marine mammals) is protected (Stram and Evans, 2009; Abbott and Haynie, 2012; Reimer and Haynie, 2018; Kroetz et al., 2019). In 2018, the NPFMC adopted a Fisheries Ecosystem Plan for the Bering Sea that specifically calls for the exploration of climate impacts on EBS fisheries (NPFMC, 2018). These features of the management system needed to be adequately represented in the suite of models employed by the ACLIM project.
In phase 1, all fishing scenarios employed the NPFMC’s EBFM Management Strategy with respect to estimation of biological reference points, prevention of overfishing, and prohibitions on fishing forage fish. The fishing scenarios explored four alternatives of the groundfish TAC across fishing sectors under the 2 million t cap: (a) no fishing; (b) the status quo; (c) a 2 million t cap which allows for the expansion of flatfish fisheries (10% increase in the total cap allocation to different flatfish species under the overall system level cap); and (d) a shift in the groundfish TAC allocation across species such that potential pollock and/or cod TAC at high stock sizes could expand despite its impact on fishing opportunities for non-pollock and cod groundfish fishers under the cap (10% increase in the allocation of gadids under the cap). This suite of alternative management strategies allows the NPFMC to explore trade-offs between harvesting more pollock and cod or more flatfish.
Stakeholder engagement is a critical element of successful management strategy evaluations (MSEs; Colenbrander and Sowman, 2015; Jones et al., 2016; Punt et al., 2016a). The selection of the initial suite of fishery scenarios that only modified allocations of groundfish TAC across species and fishing sectors had the benefit of being easily understood by managers and stakeholders. This provided an excellent opportunity to introduce the utility of the ACLIM framework for management planning in a public forum through multiple workshops. A benefit of the workshops was the two-way communication between stakeholders, managers, and the scientific community. The current scenarios are also valuable as they provide insight to managers of the trade-offs of sustained increases in allocation to one group of species. As of this submission, the first phase of projections of climate conditions to the end of the 21st century are complete for CE-SSM, CE-MSM, EwE, and MIZER models, including projections of catch for core species under no fishing, baseline (status quo) fishing conditions, and two alternative fishing scenarios. Projections based on FEAST and IBM models are in preparation. FEAST runs will not include the fisher response capability. Incorporating fisher’s responses within the spatial–temporal ecosystem model would require fleet/sector level data and predictions that was beyond what could be done in phase 1. In phase 2, scenarios generated from the multi-model framework will be expanded to explore fishing scenarios where the performance of alternative climate-informed fishery management strategies will be tested to identify and avoid maladaptive pathways (Wise et al., 2014) and explore climate resilient options (Holsman et al., 2019; Karp et al., 2019). This is challenging given the broad suite of potential fishery management strategies that could be considered by the modeling team (Fulton et al., 2019) and possible shifts in societal choices regarding marine resources (Groeneveld et al., 2018). An integrated approach of vetting strategies with the public based on results from climate-enhanced single- and multi-species models will help to narrow the suite of candidate fishery management approaches applied to the fully integrated spatial–temporal ecosystem model (FEAST). Broader suites of fishery management strategies and parameter settings can be explored across other biological models.
Fishery-Dependent Community Impacts Assessments
Several models have been developed to assess the economic impacts of changing groundfish distributions and abundance. Climate envelope assessments coupled with spatial impacts on vital rates have been used to assess climate impacts on regional (Le Bris et al., 2018) and global (Cheung et al., 2019) fisheries. Models have been used to assess climate change impacts on regional economies (Seung et al., 2015; Seung and Ianelli, 2016) and global supplies of fish on fishery dependent communities (Merino et al., 2012). Evaluation of community impacts and adaptation options examine multiple pathways through which changes in the quantity, location, and value of harvest translate into regional economic impacts on communities (Seung and Miller, 2018).
In phase 2, shifting species ranges can impact fisheries in multiple ways (Pinsky et al., 2018). Fisher responses can be directly included in FEAST. Alternatively, expected shifts in the spatial distribution of fish and shellfish can be predicted from spatiotemporal models informed by size-specific and non-local responses to climate projections (Thorson et al., 2017; Thorson, 2019) and implications for shifting distribution on core parameters in single- or multi-species models can be modeled as a function of projected environmental conditions (Spencer et al., 2016). For example, the CEATTLE multi-species model uses a climate-specific overlap index (Carroll et al., in revision) for predator and prey species to inform annually varying predation mortality. In phase 2 of ACLIM, the Spatial Economics Toolbox for Fisheries (FishSET) could be linked with the spatiotemporal model to project variables that influence fishers’ choices regarding where and when to fish (Haynie and Pfeiffer, 2012).
Data Synthesis and Inference
The outcomes of projections derived from the ACLIM framework can be synthesized using techniques commonly used in the stock assessment community. Multi-model inference can be used for several purposes, including evaluating the extent to which general conclusions regarding management actions are robust to structural assumptions (e.g., Murawski et al., 2010; Payne et al., 2016; Kaplan et al., 2019), using simple models (such as CE-SSM and CEATTLE, MICE-in-space, and perhaps even EwE) to identify the most consequential uncertainty which can then be used to prioritize sensitivity runs for more complex models such as FEAST, and improve stability in management advice (Stewart and Hicks, 2018). Structural uncertainty is a source of uncertainty that is usually hard to qualify but can be amongst the largest sources of uncertainty when providing management advice (Hill et al., 2007). Consequently, structural uncertainty has become a major focus for MSEs (Punt et al., 2016a).
Given the multiple sources of potential uncertainty in climate impact assessments, multi-model ensembles within and across models of different complexity are of interest. Ensemble modeling approaches will be applied to synthesize the information to derive overall system level trends (Ianelli et al., 2016; Rosenberg et al., 2018; Lotze et al., 2019). Selection of models used to derive ensemble estimates may be informed by an analysis of among model correlations (Stewart and Hicks, 2018). Current decisions relevant to formulating the ensemble include selection criteria for model inclusion in the ensemble (e.g., Butterworth et al., 1996) and weighting criteria for the models included in the ensemble (Anderson et al., 2017) among others. In applications for stock assessments, the results from each model could be weighted by its fit to the available data using a Bayesian approach (e.g., Butterworth et al., 1996; Hill et al., 2007) to create probability distributions for model outputs. Model selection and weighting for ecological projections is more challenging due to the lack of observations for tuning. Our approach has been to tailor ensemble syntheses within each application and question, and different approaches are illustrated in recent publications (e.g., Hermann et al., 2019; Kearney et al., in press; Reum et al., in press). Another key outcome of this analysis will be an evaluation of which parameters and processes within the linked model most determine uncertainty; such parameters and processes could be the target of future research (see Reum et al., in press); such parameters and processes could be the target of future research.
The performance of each “climate scenario/biological model/fishing scenario” combination relative to the goals of EAFM can be evaluated using indicators of social, industry, and ecosystem status (Long et al., 2015; Levin et al., 2018). Evaluation of the performance of fishing scenarios from the multi-model suite in the ACLIM project will involve two approaches. Initially, output from each “climate scenario/biological model/fishing scenario” combination will be evaluated relative to an agreed upon suite of standard indicators previously selected by the NPFMC and its advisory bodies (Zador et al., 2017; Fissel et al., 2019; Table 6). Output from vulnerability assessments, whole ecosystem models, and FEAST can be used to calculate ecosystem and socio-economic indicators (Table 6). Subsequently, indicators derived from ensemble of “climate scenario/biological model/fishing scenario” combinations will be evaluated. This two-step process will enable analysts to contrast the synthesized projection relative to the range of possible outcomes from models of different complexity.
Initial Results
The results of the first phase of the ACLIM suite revealed substantial differences in projected spatially averaged air temperature in the Bering Sea based on the GFDL and the MIROC ESM with projected air temperatures differing by approximately 5°C at end of century (see Figure 2 in Hermann et al., 2019). This result illustrates the importance of considering the ensemble projections. Under RCP 8.5, Bering Sea shelf average mean bottom temperatures may warm by as much as 5°C by 2100, with associated loss of large zooplankton (Figure 4), whereas, under the lower emission scenario, bottom temperatures will warm by approximately 2.5°C (Figure 4).
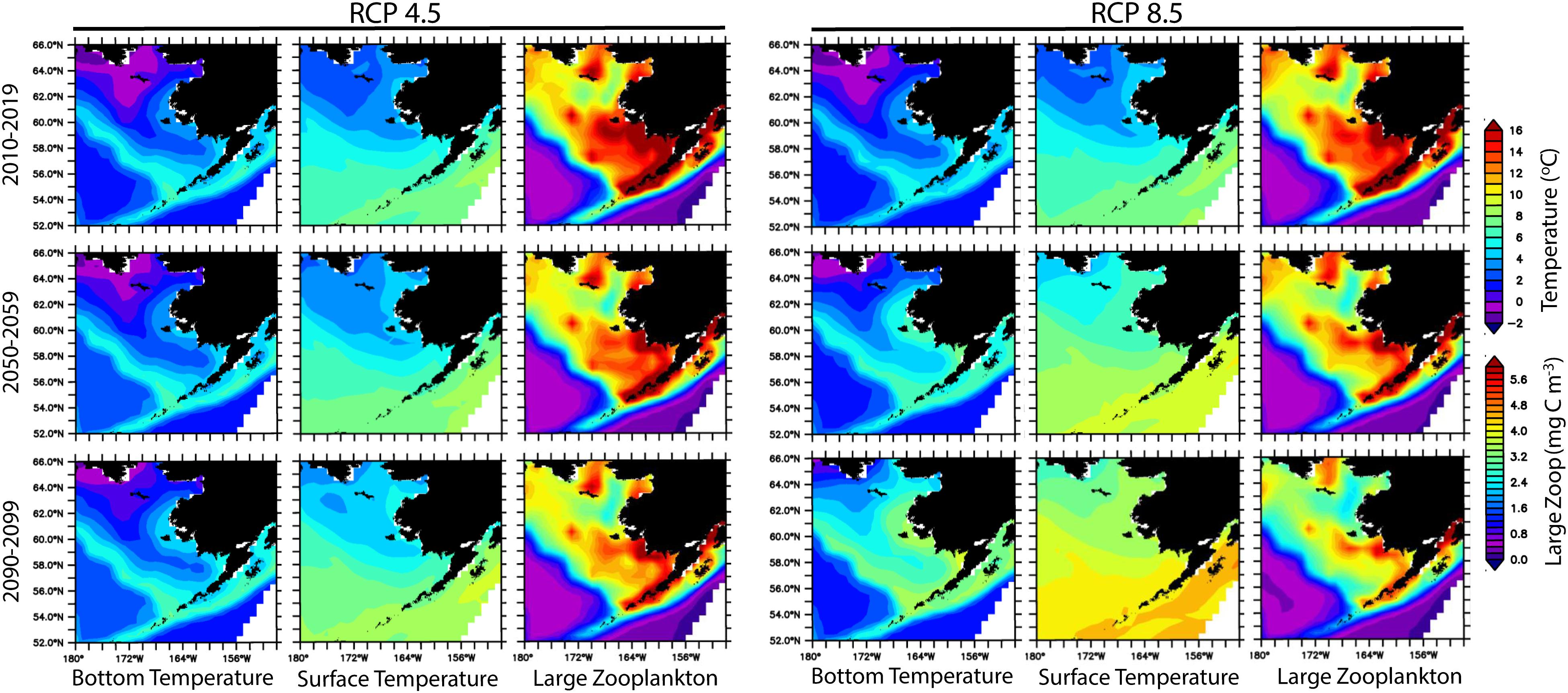
Figure 4. Ensemble projections of bottom temperature, surface temperature, and large zooplankton based on Representative Concentration Pathway 4.5 and 8.5 using the MIROC and GFDL models. Large Zooplankton = Large Copepods mean + Euphausiids mean (see Table 4).
Results from a sub-set of the full ACLIM multi-model suite illustrate the utility of applying the ACLIM framework. Comparison of projections of future status of walleye pollock and Pacific cod from three different modeling approaches under the status quo fishing scenario under RCP 8.5 using the size spectral model (Reum et al., in press), the CE-MSM projection model (CEATTLE), and the vulnerability assessment (Spencer et al., 2019) provide an interesting contrast and exemplify the importance of the multi-model approach employed by the ACLIM team. Projections from the size spectral model that incorporated bioenergetics and predator–prey interactions indicated that future status of walleye pollock will decline, while results were more modest and mixed for Pacific cod (see Figure 4 in Reum et al., in press). The CE-MSM model incorporated temperature effects on growth and recruitment of walleye pollock and Pacific cod. This model projected warm ocean conditions will negatively impact both stocks through impacts on survival to age-1. In contrast, Spencer et al. (2019) utilized a trait based approach and expert judgment of 34 experts to assess the vulnerability of walleye pollock and Pacific cod to changing climate. Their results indicate that walleye pollock and Pacific cod exhibit numerous traits that would allow these populations to adapt to a changing climate (e.g., broad spatial distribution, mixed prey, large populations, relatively high rate of production). This preliminary comparison illustrates the importance of contrasting outcomes using a multi-model approach.
Summary
Alaska Climate Integrated Modeling is a novel multidisciplinary modeling study designed to quantify the impacts of climate change on Bering Sea species and fisheries. The management strategies used to project future capture of marine species, processing, and distribution represent a realistic suite of future options. The evaluation of alternative management strategies allows analysts to assess the performance under a range of climate change scenarios. The ACLIM framework is designed to quantify the contributions of climate forcing scenario, model parameter, and management uncertainty in projected impact assessments.
The operationalized framework developed through ACLIM aligns global projections of climate impacts on the physical biogeochemical environment with assessments of the impacts of these changes on ecosystems and humans. Ideally, the ACLIM framework would be re-employed in parallel with new climate assessments to provide climate ready fisheries management advice that enables resiliency to a rapidly changing climate. During each climate assessment cycle, the generalized ACLIM approach will involve three steps for rapidly generating updated climate change assessments for the Bering Sea following: (1) release of new IPCC emission projections of global climate models will be downloaded and interpolated to generate boundary conditions for the high resolution regional ocean model (Bering 10K or its successor); (2) identify novel management challenges that require climate-specific MSEs. In each of these cases (or both combined) a new climate assessment will be initiated; and (3) with input from stakeholders and fisheries management councils, various harvest and management strategies will be used to iteratively develop and refine MSEs. This will enhance the global assessment of climate impacts on the world’s oceans as well as regional management actions to ensure climate resilience for the Bering Sea ecosystem and the fishing industry it supports.
Identifying harvest strategies that perform well under non-stationary environmental conditions is a challenging (Szuwalski and Hollowed, 2016). Recent studies indicate that ecosystem dynamics can substantially influence optimal harvest strategies in multi-species fisheries (Kasperski, 2015) and impact the cost of harvesting commercial species (Haynie and Pfeiffer, 2013), thus climate-driven changes to predation and production could alter future optimal harvest strategies. The proposed iterative ACLIM framework conducted on a ∼5 year cycle is modeled after the annual stock assessment cycle in the region; the approach should ensure that fisheries management decisions account for climate-driven changes to fish production and distribution and that climate-ready fisheries management in the region reflects the most recent global climate and carbon emission projections and best available ecosystem and socioeconomic science.
The ACLIM modeling framework is designed to inform the NPFMC of the performance of current and alternative management approaches in a changing climate. The scenarios will help to identify and test climate-resilient management options (Holsman et al., 2019). The Climate Action Module within the Bering Sea FEP provides a vehicle for communicating results to managers, stakeholders and the public. The scope of the framework serves to integrate the socio-ecological research community providing a forum for improving and adapting the framework. The near future projections (2030–2050) provide useful information regarding how changing climate will affect peoples’ livelihoods, longer term projections inform the public of what is at risk in the region.
Five key messages have emerged from first phase of ACLIM. Structural uncertainty in ESMs used as boundary conditions for the ROMs model is a key consideration in the assessment of climate impacts on marine resources. Comparison of projected change based on boundary conditions from different earth systems models differs strongly with differences being comparable in scale to differences stemming from different RCPs in a single model. Structural uncertainty in ecosystem complexity should be considered in regional impact assessments. Results from a subset of models from phase 1 of ACLIM revealed alternative response pathways for walleye pollock and Pacific cod. Ensuring the conservation measures currently in place in an existing EAFM system was critical to managers and stakeholders because these measures do preserve resources into the future. Aligning fishing scenarios with the evolving EAFM approach of the NPFMC requires strong community engagement.
Data Availability Statement
The datasets generated for this study are available on request to the corresponding author.
Author Contributions
ABH was the lead author and a principal investigator for the project. KH was a principal investigator for the project and was also responsible for the CE-MSMs. ACH was a principal investigator for the project and was also responsible for fishing scenarios and economic modeling. AJH was a principal investigator for the project and was also responsible for downscaling ESM models using ROMS. AP was the co-investigator responsible for CE-SSM, and CE-MSM models and management strategy evaluations. KA was the co-investigator responsible for FEAST model. JI was the co-investigator responsible for CE-SSM models of pollock. SK was the co-investigator responsible for community impact assessment and economic modeling. WC was the co-investigator responsible for ESM model selection and climate downscaling. AF was post-doc responsible for the development of function to estimate catch estimates under status quo and alternative fishing scenarios. KK was a research scientist responsible for nutrient phytoplankton zooplankton model. JR was post-doc responsible for size spectral model. PS was the co-investigator responsible for spatial CE-SSM for pollock that includes predator overlap impact. IS was the co-investigator responsible for CE-SSM models for flatfish. WS was the co-investigator responsible for IBM for snow crab. CS was the co-investigator responsible for spatial CE-SSM for snow crab. GW was a graduate student responsible for CE food-web model. TW was the co-investigator responsible for CE-SSM models for flatfish.
Funding
Multiple NOAA National Marine Fisheries programs provided support for ACLIM including Fisheries and the Environment (FATE), Stock Assessment Analytical Methods (SAAM) Science and Technology North Pacific Climate Regimes and Ecosystem Productivity, the Integrated Ecosystem Assessment Program (IEA), the Economics and Human Dimensions Program, NOAA’s Research Transition Acceleration Program (RTAP), the Alaska Fisheries Science Center (ASFC), the Office of Oceanic and Atmospheric Research (OAR), and the National Marine Fisheries Service (NMFS). Additionally, the International Council for the Exploration of the Sea (ICES) and the North Pacific Marine Science Organization (PICES) provided support for Strategic Initiative for the Study of Climate Impacts on Marine Ecosystems (SI-CCME) and the Strategic Initiative on the Human Dimension (SIHD) workshops, which facilitated development of the ideas presented in this manuscript. This publication is partially funded by the Joint Institute for the Study of the Atmosphere and Ocean (JISAO) under NOAA Cooperative Agreement NA15OAR4320063, Contribution No. 2019–1043. This is IEA publication number 2019_9.
Conflict of Interest
The authors declare that the research was conducted in the absence of any commercial or financial relationships that could be construed as a potential conflict of interest.
The handling Editor declared a past co-authorship with one of the authors KH.
Acknowledgments
We thank James Thorson and Martin Dorn for their helpful comments and suggestions that improved the manuscript. We also thank the journal reviewers for their helpful comments and suggestions. We thank Christine Stawitz for her contributions to the snow crab IBM. The scientific views, opinions, and conclusions expressed herein are solely those of the authors and do not represent the views, opinions, or conclusions of NOAA, the Department of Commerce, ICES, or PICES.
Footnotes
- ^ https://www.fisheries.noaa.gov/alaska/ecosystems/alaska-climate-integrated-modeling-project
- ^ https://www.npfmc.org/bsfep/
References
Abbott, J. K., and Haynie, A. C. (2012). What are we protecting? Fisher behavior and the unintended consequences of spatial closures as a fishery management tool. Ecol. Appl. 22, 762–777. doi: 10.1890/11-1319.1
Allison, E. H., and Bassett, H. R. (2015). Climate change in the oceans: human impacts and responses. Science 350, 778–782. doi: 10.1126/science.aac8721
Anderson, S. C., Cooper, A. B., Jensen, O. P., Minto, C., Thorson, J. T., Walsh, J. C., et al. (2017). Improving estimates of population status and trend with superensemble models. Fish Fish. 18, 732–741. doi: 10.1111/faf.12200
Arrigo, K. R., and Van Dijken, G. L. (2015). Continued increases in Arctic Ocean primary production. Prog. Oceanogr. 136, 60–70. doi: 10.1016/j.pocean.2015.05.002
Aydin, K. Y., Gaichas, S., Ortiz, I., Kinzey, D., and Friday, N. (2007). A Comparison of the Bering Sea, Gulf of Alaska, and Aleutian Islands Large Marine Ecosystems through Food Web Modeling. Washington, DC: U.S. Department of Commerce.
Bailey, K. M. (2000). Shifting control of recruitment of walleye pollock Theragra chalcogramma after a major climatic and ecosystem change. Mar. Ecol. Prog. Ser. 198, 215–224. doi: 10.3354/meps198215
Baker, M. R., and Hollowed, A. B. (2014). Delineating ecological regions in marine systems: integrating physical structure and community composition to inform spatial management in the eastern Bering Sea. Deep Sea Res. Part II Top. Stud. Oceanogr. 109, 215–240. doi: 10.1016/j.dsr2.2014.03.001
Barbeaux, S. J., and Hollowed, A. B. (2018). Ontogeny matters: climate variability and effects on fish distribution in the eastern Bering Sea. Fish. Oceanogr. 27, 1–15. doi: 10.1111/fog.12229
Busch, D. S., Griffis, R., Link, J., Abrams, K., Baker, J., Brainard, R., et al. (2016). Climate science strategy for the U.S. National Marine Fisheries Service. Mar. Policy 74:58–67. doi: 10.1016/j.marpol.2016.09.001
Buser, C. M., Künsch, H. R., Lüthi, D., Wild, M., and Schär, C. (2009). Bayesian multi-model projection of climate: bias assumptions and interannual variability. Clim. Dyn. 33, 849–868. doi: 10.1007/s00382-009-0588-6
Butterworth, D. S., Punt, A. E., and Smith, A. D. M. (1996). On plausible hypotheses and their weighting, with implications for selection between variants of the revised management procedure. Rep. Int. Whaling Comm. 46, 637–640.
Cheng, W., Curchitser, E., Stock, C., Hermann, A., Cokelet, E., Mordy, C., et al. (2015). What processes contribute to the spring and fall bloom co-variability on the Eastern Bering Sea shelf? Deep Sea Res. II Top. Stud. Oceanogr. 134, 128–140. doi: 10.1016/j.dsr2.2015.07.009
Cheung, W. W. L., Bruggeman, J., and Butenschön, M. (2019). “Projected changes in global and national potential marine fisheries catch under climate change scenarios in the twenty-first century,” in Impacts of Climate Change on Fisheries and Aquaculture: Synthesis of Current Knowledge, Adaptation and Mitigation Options, eds M. Barange, T. Bahri, M. C. M. Beveridge, K. Cochrane, S. Funge-Smith, and F. Poulain, (Rome: Food and Agriculture Organization of the United Nations), 63–85.
Cheung, W. W. L., Frölicher, T. L., Asch, R. G., Jones, M. C., Pinsky, M. L., Reygondeau, G., et al. (2016). Building confidence in projections of the responses of living marine resources to climate change. ICES J. Mar. Sci. 73, 1283–1296. doi: 10.1093/icesjms/fsv250
Colenbrander, D. R., and Sowman, M. R. (2015). Merging socioeconomic imperatives with geospatial data: a non-negotiable for coastal risk management in South Africa. Coast. Manage. 43, 270–300. doi: 10.1080/08920753.2015.1030321
Comeau, S., Jeffree, R., Teyssie, J.-L., and Gattuso, J.-P. (2010). Response of the Arctic pteropod Limacina helicina to projected future environmental conditions. PLoS One 5:e11362. doi: 10.1371/journal.pone.0011362
Curchitser, E. N., Haidvogel, D. B., Hermann, A. J., Dobbins, E. L., Powell, T. M., and Kaplan, A. (2005). Multi-scale modeling of the North Pacific Ocean: assessment and analysis of simulated basin-scale variability (1996–2003). J. Geophys. Res. Oceans 110:C11021.
Dolan, T. E., Patrick, W. S., and Link, J. S. (2015). Delineating the continuum of marine ecosystem-based management: a US fisheries reference point perspective. ICES J. Mar. Sci. 73, 1042–1050. doi: 10.1093/icesjms/fsv242
Duffy-Anderson, J. T., Stabeno, P. J., Siddon, E. C., Andrews, A. G., Cooper, D. W., Eisner, L. B., et al. (2017). Return of warm conditions in the southeastern Bering Sea: phytoplankton - Fish. PLoS One 12:e0178955. doi: 10.1371/journal.pone.0178955
Dunne, J. P., John, J. G., Shevliakova, E., Stouffer, R. J., Krasting, J. P., Malyshev, S. L., et al. (2012). GFDL’s ESM2 global coupled climate–carbon earth system models. Part II: carbon system formulation and baseline simulation characteristics. J. Clim. 26, 2247–2267. doi: 10.1175/jcli-d-12-00150.1
Fissel, B., Dalton, M., Garber-Yonts, B., Haynie, A., Kasperski, S., Lee, J., et al. (2019). Stock Assessment and Fishery Evaluation Report for the Groundfish Fisheries of the Gulf of Alaska and Bering Sea/Aleutian Islands Area: Economic Status of the Groundfish Fisheries off Alaska, 2017. Seattle, WA: Alaska Fisheries Science Center.
Fulton, E. A., Punt, A. E., Dichmont, C. M., Harvey, C. J., and Gorton, R. (2019). Ecosystems say good management pays off. Fish Fish. 20, 66–96. doi: 10.1111/faf.12324
Gibson, G. A., and Spitz, Y. H. (2011). Impacts of biological parameterization, initial conditions, and environmental forcing on parameter sensitivity and uncertainty in a marine ecosystem model for the Bering Sea. J. Mar. Syst. 88, 214–231. doi: 10.1016/j.jmarsys.2011.04.008
Groeneveld, R. A., Bosello, F., Butenschön, M., Elliott, M., Peck, M. A., and Pinnegar, J. K. (2018). Defining scenarios of future vectors of change in marine life and associated economic sectors. Estuar. Coast. Shelf Sci. 201, 164–171. doi: 10.1016/j.ecss.2015.10.020
Hare, J. A., Morrison, W. E., Nelson, M. W., Stachura, M. M., Teeters, E. J., Griffis, R. B., et al. (2016). A vulnerability assessment of fish and invertebrates to climate change on the Northeast U.S. continental shelf. PLoS One 11:e0146756. doi: 10.1371/journal.pone.0146756
Haerter, J. O., Hagemann, S., Moseley, C., and Piani, C. (2011). Climate model bias correction and the role of timescales. Hydrol. Earth Syst. Sci. 15, 1065–1079. doi: 10.5194/hess-15-1065-2011
Hawkins, E., Osporne, T. M., Ho, C. K., and Challinor, A. J. (2013). Calibration and bias correction of climate projections for crop modelling: an idealised case study over Europe. Agric. For. Meteorol. 170, 19–31. doi: 10.1016/j.agrformet.2012.04.007
Haynie, A. C., and Pfeiffer, L. (2012). Why economics matters for understanding the effects of climate change on fisheries. ICES J. Mar. Sci. 69, 1160–1167. doi: 10.1093/icesjms/fss021
Haynie, A. C., and Pfeiffer, L. (2013). Climatic and economic drivers of the Bering Sea pollock (Theragra chalcogramma) fishery: implications for the future. Can. J. Aquat. Fish. Sci. 70, 841–853. doi: 10.1139/cjfas-2012-0265
Heintz, R. A., Siddon, E. C., Farley, E. V., and Napp, J. M. (2013). Correlation between recruitment and fall condition of age-0 pollock (Theragra chalcogramma) from the eastern Bering Sea under varying climate conditions. Deep Sea Res. Part II Top. Stud. Oceanogr. 94, 150–156. doi: 10.1016/j.dsr2.2013.04.006
Hermann, A. J., Gibson, G. A., Bond, N. A., Curchitser, E. N., Hedstrom, K., Cheng, W., et al. (2013). A multivariate analysis of observed and modeled biophysical variability on the Bering Sea shelf: multidecadal hindcasts (1970-2009) and forecasts (2010-2040). Deep Sea Res. Part II Top. Stud. Oceanogr. 94, 121–139. doi: 10.1016/j.dsr2.2013.04.007
Hermann, A. J., Gibson, G. A., Bond, N. A., Curchitser, E. N., Hedstrom, K., Cheng, W., et al. (2016). Projected future biophysical states of the Bering Sea. Deep Sea Res. Part II Top. Stud. Oceanogr. 134, 30–47. doi: 10.1016/j.dsr2.2015.11.001
Hermann, A. J., Gibson, G. A., Cheng, W., Ortiz, I., Aydin, K., Wang, M., et al. (2019). Projected biophysical conditions of the Bering Sea to 2100 under multiple emission scenarios. ICES J. Mar. Sci. 76, 1280–1304.
Hill, S. L., Watters, G. M., Punt, A. E., McAllister, M. K., LeQuere, C., and Turner, J. (2007). Model uncertainty in the ecosystem approach to fisheries. Fish Fish. 8, 315–336. doi: 10.1111/j.1467-2979.2007.00257.x
Ho, C. K., Stephenson, D. B., Collins, M., Ferro, C. A. T., and Brown, S. J. (2012). Calibration strategies: a source of additional uncertainty in climate change projections. Bull. Am. Meteorol. Soc. 93, 21–26. doi: 10.1175/2011bams3110.1
Hollowed, A. B., Aydin, K. Y., Essington, T. E., Ianelli, J. N., Megrey, B. A., Punt, A. E., et al. (2011). Experience with quantitative ecosystem assessment tools in the northeast Pacific. Fish Fish. 12, 189–208. doi: 10.1111/j.1467-2979.2011.00413.x
Hollowed, A. B., Curchitser, E. N., Stock, C. A., and Zhang, C. I. (2013). Trade-offs associated with different modeling approaches for assessment of fish and shellfish responses to climate change. Clim. Change 119, 111–129. doi: 10.1007/s10584-012-0641-z
Holsman, K., Hazen, E., Haynie, A., Gourguet, S., Hollowed, A., Borgrad, S., et al. (2019). Toward climate-resiliency in fisheries management. ICES J. Mar. Sci. 76, 1368–1378.
Holsman, K. K., Ianelli, J., Aydin, K., Punt, A. E., and Moffitt, E. A. (2016). A comparison of fisheries biological reference points estimated from temperature-specific multi-species and single-species climate-enhanced stock assessment models. Deep Sea Res. Part II Top. Stud. Oceanogr. 134, 360–378. doi: 10.1016/j.dsr2.2015.08.001
Hunt, G. L. J., Coyle, K. O., Eisner, L. B., Farley, E. V., Heintz, R. A., Mueter, F., et al. (2011). Climate impacts on eastern Bering Sea foodwebs: a synthesis of new data and an assessment of the oscillating control hypothesis. ICES J. Mar. Sci. 68, 1230–1243. doi: 10.1093/icesjms/fsr036
Ianelli, J., Holsman, K. K., Punt, A. E., and Aydin, K. (2016). Multi-model inference for incorporating trophic and climate uncertainty into stock assessments. Deep Sea Res. Part II Top. Stud. Oceanogr. 134, 379–389. doi: 10.1016/j.dsr2.2015.04.002
IPCC, (2014). “Summary for policymakers,” in Climate Change 2014: Impacts, Adaptation, and Vulnerability. Part A: Global and Sectoral Aspects. Contribution of Working Group II to the Fifth Assessment Report of the Intergovernmental Panel on Climate Change, eds C. B. Field, V. R. Barros, D. J. Dokken, K. J. Mach, M. D. Mastrandrea, T. E. Bilir, et al. (Cambridge: Cambridge University Press), 32.
IPCC, (2018). “Summary for policymakers,” in Report on the Impacts of Global Warming of 1.5°C Above Pre-Industrial Levels and Related Global Greenhouse Gas Emission Pathways, in the Context of Strengthening the Global Response to the Threat of Climate Change, Sustainable Development, and Efforts to Eradicate Poverty, eds V. P. Masson-Delmotte, H.-O. Pörtner, D. Roberts, J. Skea, P. R. Shukla, Y. Chen, et al. (Geneva: World Meteorological Organization), 32.
Jones, M. L., Catalano, M. J., Peterson, L. K., and Berger, A. M. (2016). “Stakeholder-centered development of harvest control rule for Lake Erie walleye,” in Management Science in Fisheries: An Introduction to Simulation-Based Methods, eds T. Charles, T. Edwards, and D. J. Dankel, (New York, NY: Routledge), 163–183.
Kaplan, I. C., Francis, T. B., Punt, A. E., Koehn, L. E., Curchitser, E., Hurtado-Ferro, F., et al. (2019). A multi-model approach to understanding the role of Pacific sardine in the California current food web. Mar. Ecol. Prog. Ser. 617–618, 307–321. doi: 10.3354/meps12504
Karp, M., Peterson, J., Lynch, P., Griffis, R., Adams, C., Arnold, W., et al. (2019). Accounting for shifting distributions and changing productivity in the development of scientific advice for fishery management. ICES J. Mar. Sci. 76, 1305–1315.
Kasperski, S. (2015). Optimal multi-species harvesting in ecologically and economically interdependent fisheries. Environ. Resour. Econ. 61, 517–557. doi: 10.1007/s10640-014-9805-9
Kay, J. E., Deser, C., Phillips, A., Mai, A., Hannay, C., Strand, G., et al. (2015). The community earth system model (CESM) large ensemble project: a community resource for studying climate change in the presence of internal climate variability. Bull. Am. Meteorol. Soc. 96, 1333–1349. doi: 10.1175/bams-d-13-00255.1
Kearney, K., Hermann, A., Cheng, W., Ortiz, I., and Aydin, K. (in press). A coupled pelagic-benthic-sympagic biogeochemical model for the Bering Sea: documentation and validation of the BESTNPZ (v2019.08.23) model within a high-resolution regional ocean model. Geosci. Model Dev. doi: 10.5194/gmd-2019-239
Kroetz, K., Reimer, M. N., Sanchirico, J. N., Lew, D. K., and Huetteman, J. (2019). Defining the economic scope for ecosystem-based fishery management. Proc. Natl. Acad. Sci. U.S.A. 116, 4188–4193. doi: 10.1073/pnas.1816545116
Large, W. G., and Yeager, S. G. (2009). The global climatology of an interannually varying air–sea flux data set. Clim. Dyn. 33, 341–364. doi: 10.1007/s00382-008-0441-3
Le Bris, A., Mills, K. E., Wahle, R. A., Chen, Y., Alexander, M. A., Allyn, A. J., et al. (2018). Climate vulnerability and resilience in the most valuable North American fishery. Proc. Natl. Acad. Sci. U.S.A. 115, 1831–1836. doi: 10.1073/pnas.1711122115
Lee, Y. J., Matrai, P. A., Friedrichs, M. A. M., Saba, V. S., Aumont, O., Babin, M., et al. (2016). Net primary productivity estimates and environmental variables in the Arctic Ocean: an assessment of coupled physical-biogeochemical models. J. Geophys. Res. Oceans 121, 8635–8669. doi: 10.1002/2016jc011993
Levin, P. S., Essington, T. E., Marshall, K. N., Koehn, L. E., Anderson, L. G., Bundy, A., et al. (2018). Building effective fishery ecosystem plans. Mar. Policy 92, 48–57. doi: 10.1016/j.marpol.2018.01.019
Livingston, P. A., Aydin, K., Boldt, J. L., Hollowed, A. B., and Napp, J. M. (2001). “Alaska marine fisheries management: advances and linkages to ecosystem research,” in Ecosystem-Based Management for Marine Fisheries: An Evolving Perspective, eds A. Belgrano, and C. W. Fowler, (Cambridge: Cambridge University Press), 113–152. doi: 10.1017/cbo9780511973956.006
Long, R. D., Charles, A., and Stephenson, R. L. (2015). Key principles of marine ecosystem-based management. Mar. Policy 57, 53–60. doi: 10.1016/j.marpol.2015.01.013
Long, W., Pruisner, P., Swiney, K. M., and Foy, R. J. (2019). Effects of ocean acidification on the respiration and feeding of juvenile red and blue king crabs (Paralithodes camtschaticus and P. platypus). ICES J. Mar. Sci. 76, 1335–1343.
Long, W. C., Swiney, K. M., Harris, C., Page, H. N., and Foy, R. J. (2013). Effects of ocean acidification on juvenile red king crab (Paralithodes camtschaticus) and Tanner crab (Chionoecetes bairdi) growth, condition, calcification and survival. PLoS One 8:e60959. doi: 10.1371/journal.pone.0060959
Lotze, H. K., Tittensor, D. P., Bryndum-Buchholz, A., Eddy, T. D., Cheung, W. W. L., Galbraith, E. D., et al. (2019). Global ensemble projections reveal trophic amplification of ocean biomass declines with climate change. Proc. Natl. Acad. Sci. U.S.A. 116, 12907–12912. doi: 10.1073/pnas.1900194116
Merino, G., Barange, M., Blanchard, J. L., Harle, J., Holmes, R., Allen, I., et al. (2012). Can marine fisheries and aquaculture meet fish demand from a growing human population in a changing climate? Glob. Environ. Change 22, 795–806. doi: 10.1016/j.gloenvcha.2012.03.003
Murawski, S. A., Steele, J. H., Taylor, P., Fogarty, M. J., Sissenwine, M. P., Ford, M., et al. (2010). Why compare marine ecosystems? ICES J. Mar. Sci. 67, 1–9. doi: 10.1093/icesjms/fsp221
NPFMC (2018). Bering Sea Fishery Ecosystem Plan. Anchorage, AK: North Pacific Fishery Management Council.
Olsen, E., Fay, G., Gaichas, S., Gamble, R., Lucey, S., and Link, J. S. (2016). Ecosystem model skill assessment. Yes we can! PLoS One 11:e0146467. doi: 10.1371/journal.pone.0146467
O’neill, B. C., Kriegler, E., Riahi, K., Ebi, K. L., Hallegatte, S., Carter, T. R., et al. (2014). A new scenario framework for climate change research: the concept of shared socioeconomic pathways. Clim. Change 122, 387–400. doi: 10.1007/s10584-013-0905-2
Ono, K., Haynie, A. C., Hollowed, A. B., Ianelli, J. N., McGilliard, C. R., and Punt, A. E. (2017). Management strategy analysis for multispecies fisheries, including technical interactions and human behavior in modelling management decisions and fishing. Can. J. Fish. Aquat. Sci. 75, 1185–1202. doi: 10.1139/cjfas-2017-0135
Ortiz, I., Aydin, K., Hermann, A. J., Gibson, G. A., Punt, A. E., Wiese, F. K., et al. (2016). Climate to fish: synthesizing field work, data and models in a 39-year retrospective analysis of seasonal processes on the eastern Bering Sea shelf and slope. Deep Sea Res. Part II Top. Stud. Oceanogr. 134, 390–412. doi: 10.1016/j.dsr2.2016.07.009
Payne, M. R., Barange, M., Cheung, W. W. L., Mackenzie, B. R., Batchelder, H. P., Cormon, X., et al. (2016). Uncertainties in projecting climate-change impacts in marine ecosystems. ICES J. Mar. Sci. 73, 1272–1282.
Piani, C., Haerter, J. O., and Coppola, E. (2010). Statistical bias correction for daily precipitation in regional climate models over Europe. Theor. Appl. Climatol. 99, 187–192. doi: 10.1007/s00704-009-0134-9
Pinsky, M. L., Reygondeau, G., Caddell, R., Palacios-Abrantes, J., Spijkers, J., and Cheung, W. W. L. (2018). Preparing ocean governance for species on the move. Science 360, 1189–1191. doi: 10.1126/science.aat2360
Plagányi, É., Bell, J. D., Bustamante, R. H., Dambacher, J. M., Dennis, D. M., Dichmont, C. M., et al. (2011). Modeling climate-change effects on Australian and Pacific aquatic ecosystems: a review of analytical tools and management implications. Mar. Freshw. Res. 62:1132–1147. doi: 10.1071/Mf10279
Plagányi, É. E., Punt, A. E., Hillary, R., Morello, E. B., Thébaud, O., Hutton, T., et al. (2014). Multispecies fisheries management and conservation: tactical applications using models of intermediate complexity. Fish Fish. 15, 1–22. doi: 10.1111/j.1467-2979.2012.00488.x
Poloczanska, E., Brown, C. J., Sydeman, W. J., Kiessling, W., Schoeman, D. S., Moore, P. J., et al. (2013). Global imprint of climate change on marine life. Nat. Clim. Change 3, 919–925.
Punt, A. E., Butterworth, D. S., Moor, C. L., De Oliveira, J. A. A. D., and Haddon, M. (2016a). Management strategy evaluation: best practices. Fish Fish. 17, 303–334.
Punt, A. E., Ortiz, I., Aydin, K. Y., Hunt, G. L. Jr., and Wiese, F. K. (2016b). End-to-end modeling as part of an integrated research program in the Bering Sea. Deep Sea Res. Part II Top. Stud. Oceanogr. 134, 413–423. doi: 10.1016/j.dsr2.2015.04.018
Reimer, M. N., and Haynie, A. C. (2018). Mechanisms matter for evaluating the economic impacts of marine reserves. J. Environ. Econ. Manage. 88, 427–446. doi: 10.1016/j.jeem.2018.01.009
Reum, J., Blanchard, J. L., Holsman, K. K., Aydin, K., and Punt, A. E. (2019). Species-specific ontogenetic diet shifts attenuate trophic cascades and lengthen food chains in exploited ecosystems. Oikos 128, 1051–1064. doi: 10.1111/oik.05630
Reum, J., Blanchard, J. L., Holsman, K. K., Aydin, K., Hollowed, A., and Hermann, A., et al. (in press). Ensemble projections of future climate change impacts on the Eastern Bering Sea food web using a multispecies size spectrum model. Front. Mar. Sci.
Rose, K. A., Curchister, E. N., Fiechter, J., Hedstrom, K., Bernal, M., Creekmore, S., et al. (2015). Demonstration of a fully-coupled end-to-end models for small pelagic fish using sardine and anchovy in the California current. Prog. Oceanogr. 138(Part B), 348–380. doi: 10.1016/j.pocean.2015.01.012
Rosenberg, A. A., Kleisner, K. M., Afflerbach, J., Anderson, S. C., Dickey-Collas, M., Cooper, A. B., et al. (2018). Applying a new ensemble approach to estimating stock status of marine fisheries around the world. Conserv. Lett. 11:e12363. doi: 10.1111/conl.12363
Saha, S., Moorthi, S., Pan, H. L., Wu, X., Wang, J., Nadiga, S., et al. (2010). The NCEP climate forecast system reanalysis. Bull. Am. Meteorol. Soc. 91, 1015–1057.
Scott, F., Blanchard, J. L., and Andersen, K. H. (2014). mizer: an R package for multispecies, trait-based and community size spectrum ecological modelling. Methods Ecol. Evol. 5, 1121–1125. doi: 10.1111/2041-210x.12256
Seung, C., and Ianelli, J. (2016). Regional economic impacts of climate change: a computable equilibrium analysis for an Alaska fishery. Nat. Resour. Model. 29, 289–333. doi: 10.1111/nrm.12092
Seung, C. K., Dalton, M. G., Punt, A. E., Poljak, D., and Foy, R. (2015). Economic impacts of changes in an Alaska crab fishery from ocean acidification. Clim. Chang. Econ. 6:1550017. doi: 10.1142/s2010007815500177
Seung, C. K., and Miller, S. (2018). Regional Economic analysis for North Pacific Fisheries. Washington, DC: United States Department of Commerce, 86.
Sigler, M. F., Hollowed, A. B., Holsman, K., Zador, S., Haynie, A., Himes-Cornell, A., et al. (2016a). Alaska Regional Action Plan for the Southeastern Bering Sea National Marine Fisheries Service. Silver Spring, MD: National Oceanic and Atmospheric Administration.
Sigler, M. F., Napp, J. M., Stabeno, P. J., Heintz, R. A., Lomas, M. W., and Hunt, G. L. (2016b). Variation in annual production of copepods, euphausiids, and juvenile walleye pollock in the southeastern Bering Sea. Deep Sea Res. Part II Top. Stud. Oceanogr. 134, 223–234. doi: 10.1016/j.dsr2.2016.01.003
Small, D. P., Calosi, P., Boothroyd, D., Widdicombe, S., and Spicer, J. I. (2015). Stage-Specific changes in physiological and life-history responses to elevated temperature and PCO2 during the larval development of the European lobster Homarus gammarus (L.). Physiol. Biochem. Zool. 88, 494–507. doi: 10.1086/682238
Smith, D. M., Screen, J. A., Deser, C., Cohen, J., Fyfe, J. C., García-Serrano, J., et al. (2019). The Polar Amplification Model Intercomparison Project (PAMIP) contribution to CMIP6: investigating the causes and consequences of polar amplification. Geosci. Model Dev. 12, 1139–1164. doi: 10.5194/gmd-12-1139-2019
Spencer, P. D., Hollowed, A. B., Sigler, M., Herrman, A., and Nelson, M. (2019). Trait-based climate vulnerability assessments in data-rich systems: an application to eastern Bering Sea fish and invertebrate stocks. Glob. Chang. Biol. 25, 3954–3971. doi: 10.1111/gcb.14763
Spencer, P. D., Holsman, K. K., Zador, S., Bond, N. A., Mueter, F. J., Hollowed, A. B., et al. (2016). Modelling spatially dependent predation mortality of eastern Bering Sea walleye pollock, and its implications for stock dynamics under future climate scenarios. ICES J. Mar. Sci. 73, 1330–1342. doi: 10.1093/icesjms/fsw040
Stabeno, P. J., Danielson, S. L., Kachel, D. G., Kachel, N. B., and Mordy, C. W. (2016). Currents and transport on the Eastern Bering Sea shelf: an integration of over 20 years of data. Deep Sea Res. Part II Top. Stud. Oceanogr. 134, 13–29. doi: 10.1016/j.dsr2.2016.05.010
Stabeno, P. J., Duffy-Anderson, J. T., Eisner, L. B., Farley, E. V., Heintz, R. A., and Mordy, C. W. (2017). Return of warm conditions in the southeastern Bering Sea: physics to fluorescence. PLoS One 12:e0185464. doi: 10.1371/journal.pone.0185464
Stabeno, P. J., Kachel, N. B., Moore, S. E., Napp, J. M., Sigler, M., Yamaguchi, A., et al. (2012). Comparisons of warm and cold years on the southeastern Bering Sea shelf and some implications for the ecosystem. Deep Sea Res. II Top. Stud. Oceanogr. 65–70, 31–45. doi: 10.1016/j.dsr2.2012.02.020
Stevenson, D. E., and Lauth, R. R. (2019). Bottom trawl surveys in the northern Bering Sea indicate recent shifts in the distribution of marine species. Polar Biol. 42, 407–421. doi: 10.1007/s00300-018-2431-1
Stewart, I. J., and Hicks, A. C. (2018). Interannual stability from ensemble modelling. Can. J. Fish. Aquat. Sci. 75, 2109–2113. doi: 10.1139/cjfas-2018-0238
Stock, C. A., Alexander, M. A., Bond, N. A., Brander, K. M., Cheung, W. W. L., Curchitser, E. N., et al. (2011). On the use of IPCC-class models to assess the impact of climate on living marine resources. Prog. Oceanogr. 88, 1–27. doi: 10.1016/j.pocean.2010.09.001
Stouffer, R. J., Eyring, V., Meehl, G. A., Bony, S., Senior, C., Stevens, B., et al. (2017). CMIP5 scientific gaps and recommendations for CMIP6. Bull. Am. Meteorol. Soc. 98, 95–105. doi: 10.1175/bams-d-15-00013.1
Stram, D. L., and Evans, D. C. K. (2009). Fishery management responses to climate change in the North Pacific. ICES J. Mar. Sci. 66, 1633–1639. doi: 10.1111/gcb.13564
Szuwalski, C., and Hollowed, A. (2016). Setting biological reference points under climate change. ICES J. Mar. Sci. 73, 1297–1305.
Szuwalski, C. S., Vert-Pre, K. A., Punt, A. E., Branch, T. A., and Hilborn, R. (2015). Examining common assumptions about recruitment: a meta-analysis of recruitment dynamics for worldwide marine fisheries. Fish Fish. 16, 633–648. doi: 10.1111/faf.12083
Thorson, J. T. (2019). Measuring the impact of oceanographic indices on species distribution shifts: the spatially varying effect of cold-pool extent in the eastern Bering Sea. Limnol. Oceanogr. 64, 2632–2645. doi: 10.1002/lno.11238
Thorson, J. T., Adams, G., and Holsman, K. (2019). Spatio-temporal models of intermediate complexity for ecosystem assessments: a new tool for spatial fisheries management. Fish Fish. 20, 1083–1099. doi: 10.1111/faf.12398
Thorson, J. T., Ianelli, J. N., and Kotwicki, S. (2017). The relative influence of temperature and size-structure on fish distribution shifts: a case-study on walleye pollock in the Bering Sea. Fish Fish. 18, 1073–1084. doi: 10.1111/faf.12225
Tittensor, D. P., Eddy, T. D., Lotze, H. K., Galbraith, E. D., Cheung, W., Barange, M., et al. (2018). A protocol for the intercomparison of marine fishery and ecosystem models: fish-MIP v1.0. Geosci. Model Dev. 11, 1421–1442.
Van Vuuren, D. P., and Carter, T. R. (2014). Climate and socio-economic scenarios for climate change research and assessment: reconciling the new with the old. Clim. Change 122, 415–429. doi: 10.1007/s10584-013-0974-2
Van Vuuren, D. P., Edmonds, J., Kainuma, M., Riahi, K., Thomson, A., Hibbard, K., et al. (2011). The representative concentration pathways: an overview. Clim. Change 109, 5–31. doi: 10.1007/s10584-011-0148-z
Vancoppenolle, M., Bopp, L., Madec, G., Dunne, J., Ilyina, T., Halloran, P. R., et al. (2013). Future Arctic Ocean primary productivity from CMIP5 simulations: uncertain outcome, but consistent mechanisms. Glob. Biogeochem. Cycles 27, 605–619. doi: 10.1002/gbc.20055
Wang, M., Overland, J. E., and Stabeno, P. (2012). Future climate of the Bering and Chukchi Seas projected by global climate models. Deep Sea Res. Part II Top. Stud. Oceanogr. 65–70, 46–57. doi: 10.1016/j.dsr2.2012.02.022
Watanabe, S., Hajima, T., Sudo, K., Nagashima, T., Takemura, T., Okajima, H., et al. (2011). MIROC-ESM 2010: model description and basic results of CMIP5-20c3m experiments. Geosci. Model Dev. 4, 845–872. doi: 10.5194/gmd-4-845-2011
Wiese, F. K., Wiseman, W. J. Jr., and Van Pelt, T. I. (2012). Bering Sea linkages. Deep Sea Res. Part II Top. Stud. Oceanogr. 65–70, 2–5. doi: 10.1016/j.dsr2.2012.03.001
Wilderbuer, T., Stockhausen, W., and Bond, N. (2013). Updated analysis of flatfish recruitment response to climate variability and ocean conditions in the Eastern Bering Sea. Deep Sea Res. Part II Top. Stud. Oceanogr. 94, 157–164. doi: 10.1016/j.dsr2.2013.03.021
Wise, R. M., Fazey, I., Stafford Smith, M., Park, S. E., Eakin, H. C., Archer Van Garderen, E. R. M., et al. (2014). Reconceptualising adaptation to climate change as part of pathways of change and response. Glob. Environ. Change 28, 325–336. doi: 10.1016/j.gloenvcha.2013.12.002
Keywords: climate change, fishery management strategy, Bering Sea, walleye pollock, Pacific cod, climate projections
Citation: Hollowed AB, Holsman KK, Haynie AC, Hermann AJ, Punt AE, Aydin K, Ianelli JN, Kasperski S, Cheng W, Faig A, Kearney KA, Reum JCP, Spencer P, Spies I, Stockhausen W, Szuwalski CS, Whitehouse GA and Wilderbuer TK (2020) Integrated Modeling to Evaluate Climate Change Impacts on Coupled Social-Ecological Systems in Alaska. Front. Mar. Sci. 6:775. doi: 10.3389/fmars.2019.00775
Received: 20 August 2019; Accepted: 02 December 2019;
Published: 14 January 2020.
Edited by:
Jamie C. Tam, Bedford Institute of Oceanography (BIO), CanadaReviewed by:
Nancy Shackell, Bedford Institute of Oceanography (BIO), CanadaDaniel Howell, Norwegian Institute of Marine Research (IMR), Norway
Copyright © 2020 Hollowed, Holsman, Haynie, Hermann, Punt, Aydin, Ianelli, Kasperski, Cheng, Faig, Kearney, Reum, Spencer, Spies, Stockhausen, Szuwalski, Whitehouse and Wilderbuer. This is an open-access article distributed under the terms of the Creative Commons Attribution License (CC BY). The use, distribution or reproduction in other forums is permitted, provided the original author(s) and the copyright owner(s) are credited and that the original publication in this journal is cited, in accordance with accepted academic practice. No use, distribution or reproduction is permitted which does not comply with these terms.
*Correspondence: Anne Babcock Hollowed, QW5uZS5Ib2xsb3dlZEBub2FhLmdvdg==