- Department of Plant Science, University of Manitoba, Winnipeg, MB, Canada
Poor competitive ability and sensitivity to many herbicides create challenges for weed management in legume production. The resource-ratio (R*) hypothesis may provide insight into how to manipulate the competitive balance between nitrogen (N)-fixing legume crops and non-leguminous weed species. A field study was conducted to test whether the level of soil mineral N affected yield loss of an annual legume crop, soybean [Glycine max (L.) Merr.], in the presence of four different populations of an interfering non-leguminous weed, volunteer canola (Brassica napus L.), compared with a weed-free control. The experiment consisted of banding five rates of urea fertilizer (0–180 kg N ha-1) prior to seeding soybean and volunteer canola, and was repeated in three environments in Manitoba, Canada. Soybean yield remained unaffected by N rate in the absence of volunteer canola. Interference from the volunteer canola populations caused a linear decline in soybean yield by 2.6 kg ha-1 for every 1 kg ha-1 increase in soil mineral N. In the presence of volunteer canola, soybean yield decreased by 17% from the lowest to the highest soil mineral N. In the lowest-N conditions (30 kg residual-N ha-1), soybean yield was greatest (3,350 kg ha-1) and volunteer canola seed production and aboveground biomass were lowest (decline in canola seed production by 19%, 50%, and 74% of the maximum seed production in the 2015i, 2015ii, and 2016 environments, respectively). Therefore, growing legume crops like soybean on fields with lower soil mineral N may reduce interference from unmanaged non-leguminous weeds. As N fertilization intensifies interference of many weed species, tailoring weed management in legume crops around their capacity for N-fixation could provide the crop with a competitive advantage, thereby minimizing the impact of weed interference on legume crop yield.
1 Introduction
Legumes grown for seed production can benefit cropping systems and contribute to the diversity of crop rotations. Most notably, legumes can form symbioses with nitrogen (N)-fixing bacteria and access pools of atmospheric N (dinitrogen gas) unavailable to most other plant species (Walley et al., 2007). Legumes, however, are sensitive to many herbicides and generally have reduced competitive ability compared with other crops. These qualities make weed management particularly challenging in legume crops. Unmanaged weeds in legume crops result in yield losses and weed seedbank additions. The development of integrated weed management (IWM) strategies based on the unique characteristics of legume crops may facilitate their inclusion in crop rotations, thereby promoting the benefits of a diverse cropping system.
Nitrogen is the most limiting macronutrient for crop production in many farming systems worldwide (Fageria and Baligar, 2005). Nitrogen fertilizer application is frequently studied with focus on crop response only, while potential impacts on non-target organisms are often ignored (Di Tomaso, 1995). In fact, many weed species can respond to soil mineral N more than (or similar to) crop species (Blackshaw et al., 2003). Greater N assimilation by weeds results in increased weed biomass and seed production (Blackshaw et al., 2003; Tungate et al., 2006). In this context, fertilizer application may increase interference from unmanaged weeds with the present and potentially also future crops (Blackshaw et al., 2004; Song et al., 2021).
Timing, placement and source of N fertility all have been studied with respect to their potential impact as cultural weed management tools in field crop production (Di Tomaso, 1995; Blackshaw et al., 2004). For example, placing N fertilizer in bands beneath the soil surface rather than surface broadcasting reduced the competitive ability of wild oat (Avena fatua L.) (Kirkland and Beckie, 1998), jointed goatgrass (Aegilops cylindrica Host.) (Mesbah and Miller, 1999), foxtail barley (Hordeum jubatum L.) (Blackshaw et al., 2000) and downy brome (Bromus tectorum L.) (Rasmussen, 1995). Nitrogen use efficiency (Liebman and Robichaux, 1990) and the effect of spatial dynamics of crop species on nutrient utilization (Johri et al., 1992) could also influence the competitive balance among crop and weed species (Di Tomaso, 1995). Crop species with the capacity to fix atmospheric N (i.e., legumes) may have a competitive advantage over non-leguminous weeds in lower-N conditions.
Soybean [Glycine max (L.) Merr.] enter symbioses with Bradyrhizobium japonicum and typically acquire 50–85% of their total N requirements from N-fixation (Salvagiotti et al., 2008; Rao and Reddy, 2010). Biological N-fixation in soybean conforms to a negative exponential relationship with soil mineral N (Salvagiotti et al., 2008). This allows a soybean plant to adapt to lower-N environments and thrive under these conditions. Due to N-fixation, soybean yield commonly does not respond to N fertilization under weed-free conditions (Salvagiotti et al., 2008; Brar and Lawley, 2020); although some evidence supports a positive yield response at low N rates (Osborne and Riedell, 2006; Song et al., 2021). A negative association between residual soil N and soybean yield among ten sites under organic production, suggested that yield differences, in part, were due to increased weed interference at sites with higher soil inorganic N (Carkner and Entz, 2017). In a different study, a preceding roller-crimped cereal rye (Secale cereale L.) cover crop immobilized soil mineral N prior to termination in spring (Wells et al., 2013). Temporarily lower soil mineral N following cover crop termination then caused N-deprivation in redroot pigweed (Amaranthus retroflexus L.) and reduced weed interference in soybean. Altered nutrient availability was identified as a key component of weed suppression by roller-crimped cereal rye mulch in organic rotational no-till systems (Wells et al., 2013; Menalled et al., 2021).
The resource-ratio (R*) hypothesis provides a conceptual framework to shift the competitive balance away from non-leguminous weeds when legume crops are grown in lower-N environments. The most common postulate of the resource-ratio hypothesis predicts that when neighboring species compete for the same resource, the species that can survive at the lowest level of the resource will out-compete its neighboring species when the resource is limited (Tilman, 1982; Tilman, 1985; Miller et al., 2005). In this context, lower-N conditions may reduce the competitive ability of weed species that rely on soil mineral N for growth, development and reproduction. Consequently, N-fixation is expected to enhance the competitive ability of legume crops relative to non-leguminous weeds under low soil N conditions.
To test the utility of the resource-ratio hypothesis as a cultural tool for weed management in annual legume production, the current study examined an economically-damaging herbicide-resistant (HR) weed in western Canadian soybean production (Gregoire et al., 2021). Volunteer canola (Brassica napus L.) is primarily derived from large canola harvest losses (Cavalieri et al., 2016). Following seed dispersal, volunteer canola can enter secondary seed dormancy (Gulden et al., 2003) and persist throughout crop rotations (Beckie and Warwick, 2010). While the persistence of volunteer canola in the soil seedbank depends upon environmental and edaphic factors (Geddes, 2021), timely soil disturbance can contribute to a reduction of volunteer canola populations prior to soybean production (Geddes and Gulden, 2017). However, limitation of volunteer canola seed production in crops grown subsequent to canola is imperative to limiting persistence of this weed (Geddes and Gulden, 2018; Geddes and Gulden, 2021). Soybean and canola have similar herbicide resistance traits (Mierau et al., 2020). In western Canada, volunteer canola is the most abundant weed species present after post-emergence weed control in soybean (Leeson et al., 2017). Geddes and Gulden (2018) showed that even a low level of urea fertilizer (23 kg N ha-1) applied before soybean planting doubled volunteer canola seed production. Likewise, wheat (Triticum aestivum L.) and cereal rye inter-row living mulches decreased volunteer canola seed production but not soybean yield in the absence of fertilization (Geddes and Gulden, 2021). This reduction in weed seed production could have been due to uptake of soil mineral N by the living mulches, thereby leaving volunteer canola N-deprived. Based on these observations, it is likely that soil N dynamics influence volunteer canola interference in soybean. However, the extent to which N dynamics influence the competitive balance between soybean and volunteer canola remains unknown. Evaluation of the effect of soil mineral N on interference between these two species may help elucidate the utility of the resource-ratio hypothesis as a cultural tool for weed management in legume production.
Candidate tools for weed management must meet two criteria to be considered effective. These criteria include (a) differential impact on the crop and weed species, and (b) consistency of efficacy (Li et al., 2016). This study was designed to test the influence of soil mineral N on weed interference in legume production. Volunteer canola and soybean were used as exemplar (non-leguminous) weed and (legume) crop species. The objectives were to test whether soil mineral N affects soybean and volunteer canola growth and seed production (a) differently and (b) consistently among weed populations and environments.
2 Materials and methods
2.1 Study environments
The impact of soil mineral N on volunteer canola interference in soybean was evaluated in three environments at the Ian N Morrison Research Station near Carman, MB, Canada. In 2015, two adjacent experiments were conducted (considered different environments), one seeded on May 12th (hereafter referred to as “2015i”) and one seeded on May 29th (hereafter referred to as “2015ii”) (49.495°N, 98.038°W; Sandy loam soil, Hisbin Series Orthic Black Chernozem). The experiment was repeated in 2016 (seeded on June 8th; hereafter referred to as “2016”) in a different field (49.496°N, 98.042°W; Sandy clay loam soil, Denham Series Orthic Black Chernozem). The preceding crop in each year was wheat (Triticum aestivum L.), and environments were selected based on lower levels of nitrate-N in the top 60 cm of the soil profile (Table 1).
2.2 Experimental design and treatment structure
In each environment, a split-block randomized complete block design was used to test the main and interaction effects of volunteer canola population and level of soil mineral N on canola interference in soybean. Main plots (2.3 by 13.5 m) consisted of four different glyphosate-resistant canola populations in soybean and one weed-free control. These populations included both the F1 (crop) and F2 (volunteer) generations of the hybrid ‘DKL 73-45 RR’ (Monsanto Canada Inc., Winnipeg, MB, CA) and two open-pollinated varieties ‘Café’ and ‘Rugby’ (SeCan, Kanata, ON, CA). Seed from the F1 73-45 population was pre-treated with Acceleron® (containing: difenoconazole, metalaxyl, fludioxonil and thiamethoxam; Monsanto Canada Inc., Winnipeg, MB, CA), while all other canola populations remained untreated. These varieties were chosen as the canola populations because previous research identified variation in competitive ability among hybrid and open-pollinated canola (Beckie et al., 2008), and between F1 and F2 generation hybrid canola (Seerey and Shirtliffe, 2010). Experimental blocks were split (13.5 by 2.3 m) by the rate of urea fertilizer applied (0, 22.5, 45, 90 and 180 kg N ha-1), creating 2.3 by 2.3 m subplots. Fertilizer was applied parallel to the soybean rows in bands spaced 19 cm apart at a depth of 8 cm prior to seeding using double-disc openers. Background soil macronutrients, organic matter and pH were evaluated by extracting two soil cores for each experimental replicate at random down to depths of 0–15 cm and 15–60 cm using a Dutch auger, and submitting the samples to a local soil testing laboratory (AGVISE Laboratories, Northwood, ND, USA). Soil mineral N was considered the amount of nitrate-N in the top 60 cm of the soil profile in each environment (Table 1) plus the amount of urea fertilizer N applied in each treatment (equating to soil mineral N ranging from 71–251 kg N ha-1 and 30–210 kg N ha-1 in the 2015 and 2016 environments, respectively). A 0.5 m wide strip of soybean only was left between adjacent canola populations (main plots) to ensure isolation and clear differentiation among the populations. A 0.5 m wide unfertilized strip was left between fertilizer treatments (split blocks) to prevent potential overlap among the soil mineral N levels. The experiment was replicated four times in each environment.
Following spring cultivation and immediately before seeding soybean, the canola populations were seeded at a density of 80 viable seeds m-2 at 1 cm depth and using a double-disk seeder with 19 cm row spacing (R-Tech Industries Ltd., Homewood, MB, CA). Glyphosate-resistant soybean ‘DKL 23-10 RY’ (treated with Acceleron® containing: fluxapyroxad, pyraclostrobin, metalaxyl, imidacloprid; Monsanto Canada Inc., Winnipeg, MB, CA) was seeded at 2 cm depth in 38 cm rows and at a target density of 400,000 plants ha-1 (500,000 viable seeds ha-1) over the entire experiment perpendicular to the direction of the canola rows. Cell-Tech™ granular soybean inoculant (Monsanto BioAg and Design™, Monsanto Company, St. Louis, MO, USA) was applied in the soybean row at a rate of 4.0 kg ha-1. A single application of glyphosate (Roundup WeatherMax®, 670 g ae ha-1, Monsanto Canada Inc., Winnipeg, MB, CA) was applied at soybean stage BBCH 13 (V1) to remove all weeds except for the volunteer canola. The herbicide was applied at 276 kPa using AIXR 110015 TeeJet® nozzles (TeeJet® Technologies, Wheaton, IL, USA) and with 100 L ha-1 water carrier.
2.3 Data collection
Soybean plant density was determined at stage BBCH 12–13 (~V1) by counting the number of soybean plants in two randomly selected 1 m lengths of two adjacent soybean rows in each subplot. Volunteer canola plant density was determined at the same time (canola stage BBCH 14–15; 4–5 leaf) by counting the number of canola plants in two randomly placed 1 by 1 m quadrats aligned with the edge of a soybean row in each subplot. At canola stage BBCH 82 (beginning of pod ripening) and soybean stage BBCH 77 (~R5), aboveground biomass was collected from a 0.5 by 0.5 m quadrat placed along the edge of a soybean row in each subplot. Soybean and canola plants were separated, dried at 60°C until constant weight, and dry weights were determined. The volunteer canola biomass samples were hand-threshed, cleaned using a hand sieve (2.7 mm round hole, Can-Seed Equipment Ltd., Winnipeg, MB, CA) and seed blower (Agriculex, Model CB-1, Guelph, ON, CA), and the weight of seed from each biomass sample was determined (g seed m-2). Individual seed weight (g thousand seeds-1) of canola was determined by weighing triplicate subsamples of 200 seeds from each subplot. The total weight of canola seeds and the individual seed weight in each sample were used to determine the number of canola seeds produced per unit area (thousand seeds m-2). At soybean maturity, 1 m of two randomly selected soybean rows per subplot was hand-harvested and threshed using a stationary thresher. Soybean yield samples were cleaned using a Clipper M2BC seed cleaner (Blount/Ferrell-Ross Bluffton, IN, USA), weighed, and adjusted to 13% moisture. Daily temperature and precipitation data were collected using the on-site Environment Canada weather station (Figure 1).
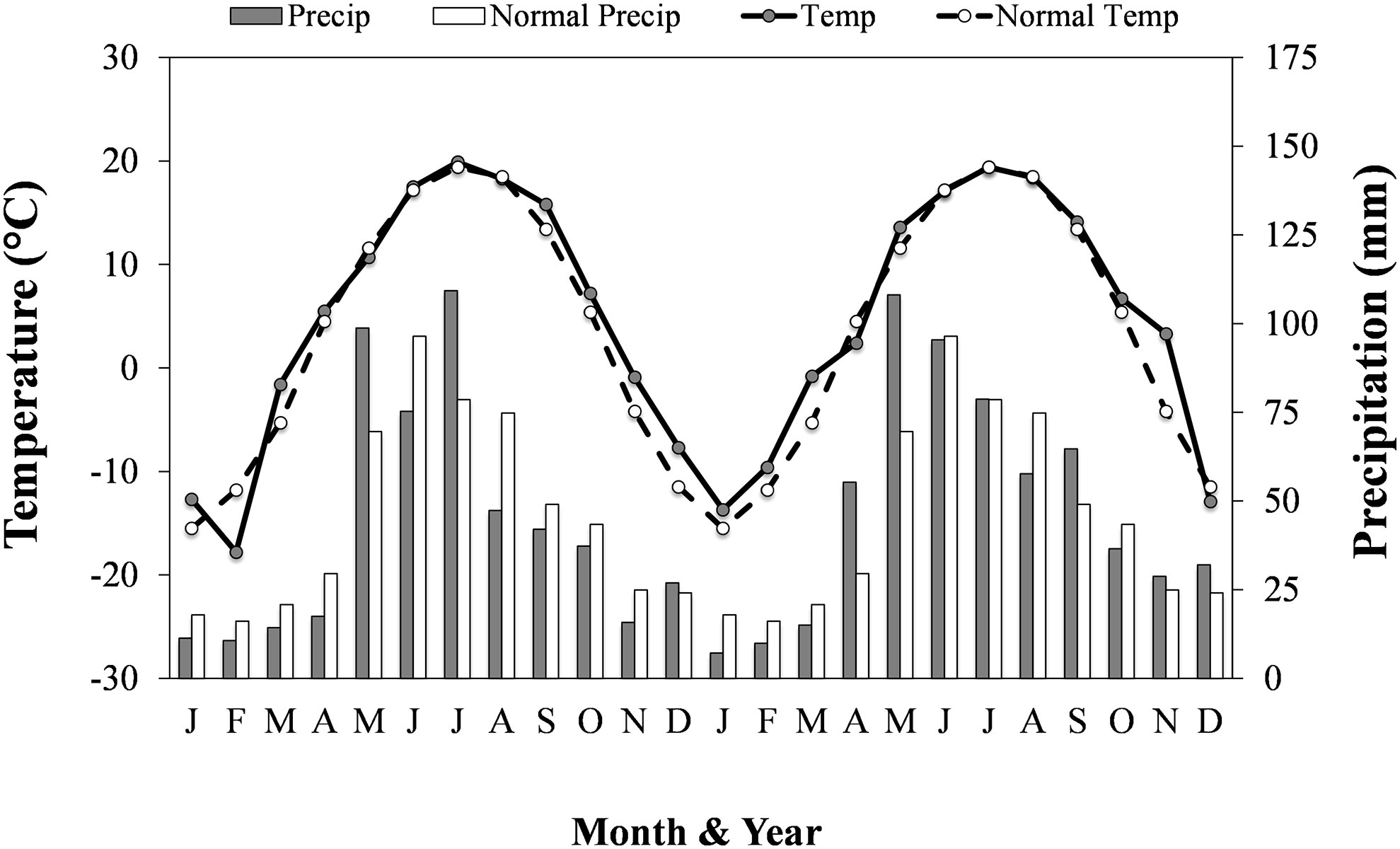
Figure 1 Mean daily air temperature (Temp; °C) and total precipitation (Precip; mm) for each month in each year of the study and the regional climatic normal (1980-2010) mean daily air temperature (Normal temp) and total precipitation (Normal precip) for each month. Adapted from: Environment Canada (2017).
2.4 Statistical analysis
The MIXED procedure (Littell et al., 2006) in SAS 9.4 (SAS Institute, Inc., Cary, NC, USA) was used for analysis of covariance (ANCOVA) of the level of soil mineral N (kg ha-1) with each response variable. The soybean response variables included plant density, biomass, and grain yield. The canola response variables included plant density, biomass, and seed production per unit area. Total aboveground biomass (soybean and canola biomass together) was also included as a response variable. The Shapiro–Wilk test was used to assess the assumption of normality and visual inspection of residual vs. predicted values was used to examine homogeneity of variance (Littell et al., 2006). Lund’s test (Lund, 1975) was used to remove extreme outliers.
In a preliminary analysis of variance, linear and quadratic single-degree-freedom contrasts for unequally spaced N fertilization treatments were used to explore the relationship of each response variable with N fertilization rate (Littell et al., 2006). Linear and quadratic models were explored because volunteer canola can lodge at very high N levels, potentially causing decline in seed production following a quadratic trajectory (Sheppard and Bates, 1980). In this analysis, N fertilization rate was considered a categorical variable. The main factors and interactions of environment (3 levels), volunteer canola population (4 levels) and N fertilization (5 levels) were considered fixed factors. Experimental block nested within environment and the interaction of volunteer canola population with experimental block nested within environment were considered random factors. Based on significance (α = 0.05) of the linear and quadratic contrasts in the preliminary categorical model, a respective continuous linear mixed regression model, saturated with all relevant main and interaction fixed factors [i.e., environment, volunteer canola population and linear and/or quadratic terms for soil mineral N (residual N + fertilizer N)] was developed. An ANCOVA was conducted using soil mineral N (kg N ha-1) as a continuous variable, while volunteer canola population and environment remained categorical (Littell et al., 2006). This analysis used the same random factors as described for the categorical model. After checking whether the slopes of each response variable regressed over soil mineral N were different from zero (α = 0.05) for at least one of the population by environment interactions, the model was adjusted to test whether a difference existed among slopes within each level of main and interaction factor of population and environment (Littell et al., 2006). An iterative hierarchical reductionist approach was used to converge the saturated model to achieve the most parsimonious model structure by sequentially removing insignificant higher-order factors (Luschei and Jackson, 2005). Model fit was assessed using the Akaike Information Criterion (AIC) and the likelihood ratio test. To further adjust for homoscedasticity, the covariance structure of residuals was adjusted by selecting the R-matrix repeated group effect based on minimization of the AIC (Onofri et al., 2010). This was done only when the increased number of model parameters was justified using the likelihood ratio test (Luschei and Jackson, 2005). Marginal (R2(M)) and conditional (R2(C)) coefficients of determination (i.e., variance explained by fixed factors or both fixed and random factors, respectively) were calculated for each linear mixed regression model following the methods of Nakagawa and Schielzeth (2013).
The same procedure described above was used to analyze the soybean grain yield data. In this analysis, an additional variable was used to create an augmented factorial treatment structure and include the control measurements of soybean yield in the absence of volunteer canola at each level of soil mineral N (Piepho et al., 2006). Each main and interaction fixed factor was nested within the new categorical variable (presence vs. absence of canola) to assess whether a difference in response of soybean yield to soil mineral N existed between the presence and absence of volunteer canola at each level of each fixed factor (Piepho et al., 2006). In the case where regression equations for soybean yield differed between the presence and absence of canola, single-degree-freedom contrasts were used to determine the level of soil mineral N at which a significant difference between the equations began (Littell et al., 2006).
3 Results and discussion
3.1 Grain yield and seed production
3.1.1 Soybean grain yield
In the presence of volunteer canola, soybean yield decreased linearly by 2.6 kg ha-1 for every 1 kg ha-1 increase in soil mineral N (P < 0.001) (Figure 2). In the absence of volunteer canola, soybean yield was unaffected by increasing soil mineral N. These findings corroborate the observation by Carkner and Entz (2017), who found a negative association between soil inorganic N and soybean yield under weedy conditions in organic agricultural production. In their study, soybean yield loss may have been caused by greater weed interference at sites with higher soil inorganic N. In Russia, N fertilization increased weed-free soybean yield by up to 44%, but yield loss due to multiple weed interference also increased from 48% to 89% when fertilizer was applied at 36 kg N ha-1 (Song et al., 2021). In contrast, application of up to 125 kg N ha-1 as broadcast sodium nitrate did not impact yield of organic rotational no-till soybean planted into roller-crimped cereal rye, although these results were not compared with those under weed-free conditions (Menalled et al., 2021). In the current study, a significant difference (P < 0.05) between the regression models for soybean yield in the presence and absence of volunteer canola was found above 15 kg N ha-1 (Figure 2). This indicated that volunteer canola interference would not impact soybean yield at very low levels of soil mineral N. It is important to note, however, that this observation was outside the range of N levels in the current study as the lowest level of soil mineral N tested was 30 kg N ha-1. Together, these results suggest that planting soybean in fields with lower soil mineral N or strategies that can reduce soil mineral N during soybean production – such as preceding cover crops (Wells et al., 2013; Menalled et al., 2021) or inter-seeded living mulches (Geddes and Gulden, 2021) – may reduce interference from non-leguminous weeds.
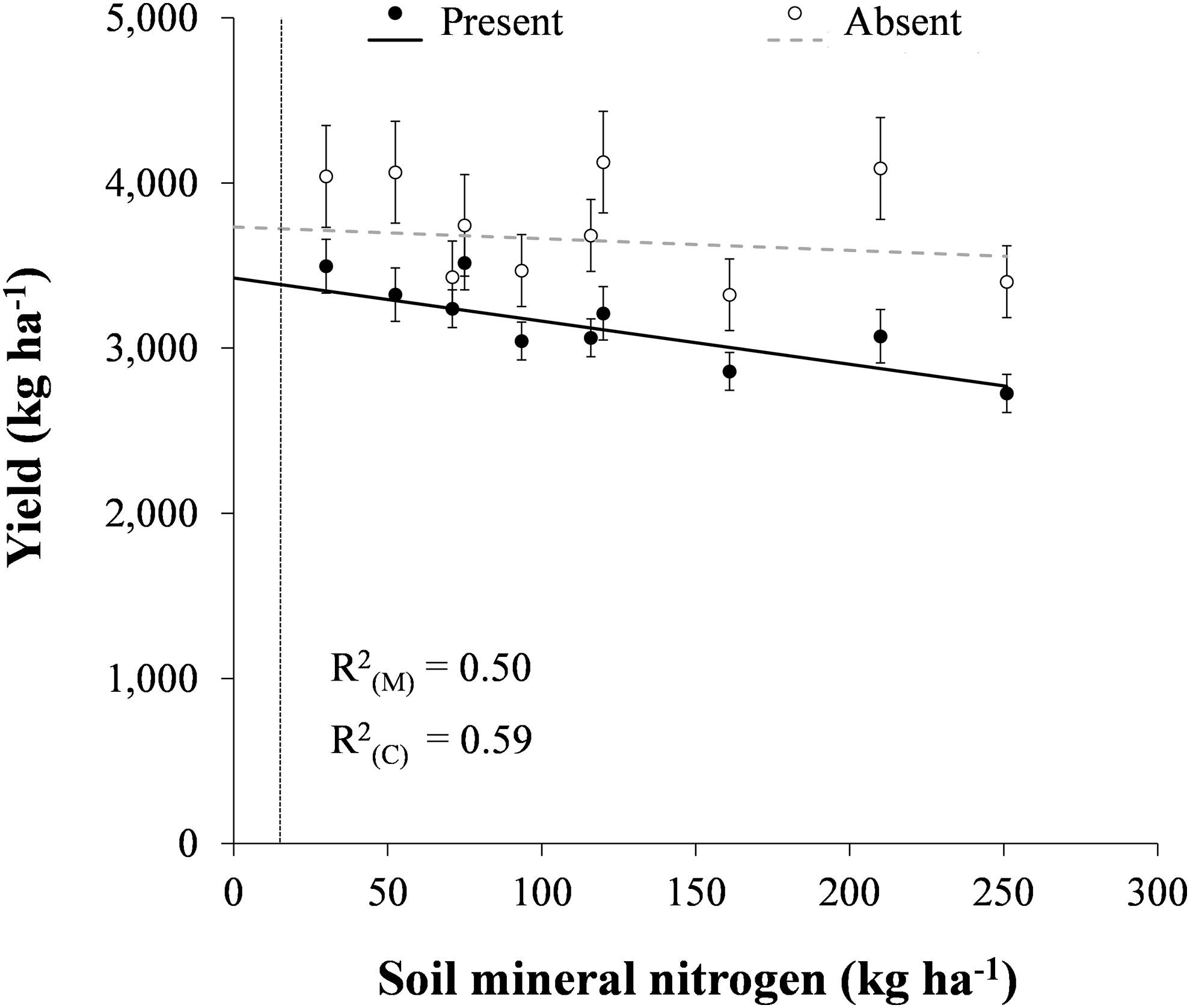
Figure 2 Soybean yield in response to soil mineral nitrogen in the presence and absence of volunteer canola. A significant difference (α = 0.05) in soybean yield between the presence and absence of volunteer canola interference was found at soil mineral N levels greater than the vertical dissecting line (15 kg N ha-1). Dots indicate means of soybean yield for each level of soil mineral nitrogen in a combined analysis of volunteer canola populations and environments. Error bars indicate ± SEM. The black line indicates a significant linear component, while the grey line indicates lack of significant difference of the linear component from 0 (α = 0.05). Marginal (R2(M)) and conditional (R2(C)) coefficients of determination are indicated for the entire linear mixed effects model. All model parameters are described in Supplementary Table S1.
3.1.2 Volunteer canola seed production
The number of volunteer canola seeds produced per unit area increased linearly with increasing soil mineral N to a level of 183 kg N ha-1 (Figure 3). Total volunteer canola seed production per unit area began to decline at increasing soil mineral N content above 183 kg N ha-1, perhaps due to lodging of canola plants. Volunteer canola seed production was influenced consistently by soil mineral N (P ≤ 0.001) even though the magnitude of seed production differed among earlier- and later-seeded environments (P = 0.001). The linear or quadratic relationships of volunteer canola seed production in response to soil mineral N were similar among canola populations and environments (Supplementary Tables S1, S2). These results correspond with past research where canola yield had a quadratic relationship that peaked at similar levels of N fertilization (Cutforth et al., 2009). Similarly, Geddes and Gulden (2018) also reported that 23 kg N ha-1 urea fertilizer applied at soybean planting about doubled volunteer canola seed production compared with soil residual N between 11–34 kg N ha-1. Nitrogen fertilization up to 36 kg N ha-1 increased the competitiveness of common ragweed (Ambrosia artemisiifolia L.), barnyard grass [Echinochloa crus-galli (L.) Beauv], and American slough grass [Beckmannia syzigachne (Steud.) Fernald] in soybean, resulting in significant yield losses (Song et al., 2021).
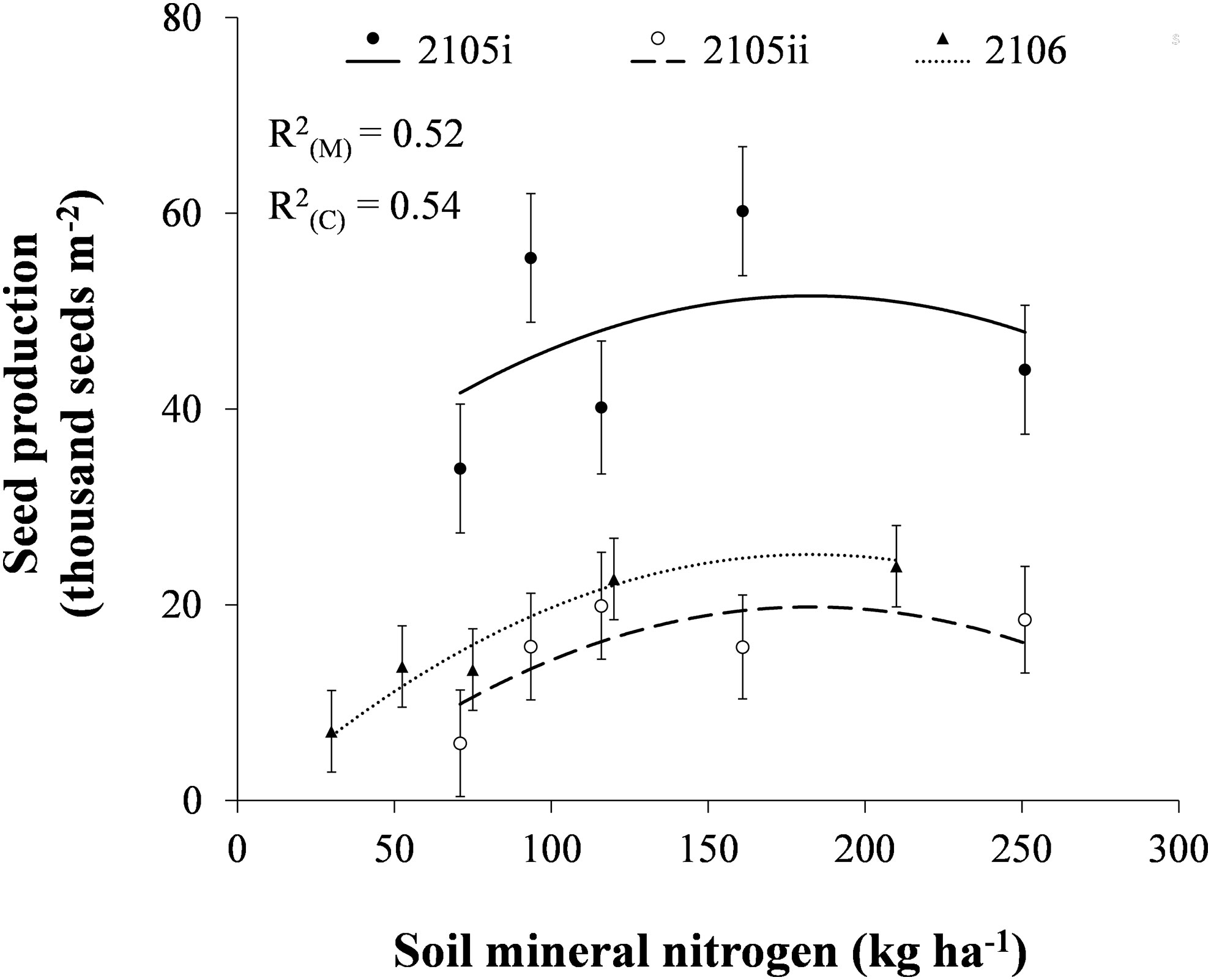
Figure 3 The number of volunteer canola seeds produced per unit area in response to soil mineral nitrogen in soybean in the 2015i, 2015ii, and 2016 environments. Dots indicate means of volunteer canola seed production for each level of soil mineral nitrogen in each environment in a combined analysis among volunteer canola populations. Error bars indicate ± SEM. All linear and quadratic components were significantly different from 0 (α = 0.05). Marginal (R2(M)) and conditional (R2(C)) coefficients of determination are indicated for the entire linear mixed effects model. All model parameters are described in Supplementary Table S1.
Volunteer canola seed production reached a maximum at about 183 kg N ha-1 which ranged from 19,800 to 51,600 seeds m-2 among environments (Figure 3). Mean density of volunteer canola ranged among environments from 16 to 27 plants m-2 (Supplementary Figure S1), where the environment with the lowest overall density produced the greatest amount of seed per unit area (Figure 3). About two-thirds of the seeds produced by volunteer canola in soybean contribute to seedbank additions, while the other third is removed with the harvested soybean seed (Geddes and Gulden, 2018; Geddes and Gulden, 2021). Together, these data indicate that higher-N conditions (183 kg N ha-1) in soybean likely resulted in seedbank inputs of about 13,200 to 34,400 seeds m-2 at canola densities ranging from 13 to 46 plants m-2 (Supplementary Figure S1). Thus, higher-N conditions resulted in volunteer canola seedbank inputs that ranged from about 3 to 8 times the regional average on-farm harvest seed losses in canola crops (Cavalieri et al., 2016). Since a single cohort of volunteer canola can persist more than three years in the soil seedbank in western Canada (Gulden et al., 2003), volunteer canola seedbank inputs in a soybean crop could have a large impact on densities of this weed in crops grown after soybean. These results, however, show that lower-N conditions in soybean can reduce volunteer canola seed production to as low as one-quarter that of higher-N conditions (30 vs. 183 kg N ha-1 in 2016; Figure 3).
3.2 Aboveground biomass
Soybean and volunteer canola biomass showed opposing quadratic relationships where peak interference from canola was observed around 159–174 kg N ha-1 (Figure 4). Total aboveground biomass (of soybean and volunteer canola together) responded positively to soil mineral N (P = 0.003). In the presence of volunteer canola, soybean biomass decreased as soil mineral N increased to 159 kg N ha-1. At levels greater than 159 kg N ha-1, soybean biomass increased with increasing soil mineral N. The quadratic relationship of soybean biomass in response to soil mineral N was consistent among volunteer canola populations but differed in magnitude (y-intercept) between the 2015 and 2016 environments (P < 0.001) (Figure 4; Supplementary Table S2). Insufficient mineral N supply for maximum soybean biomass and reduced N-fixation inhibited by the assimilation of mineral N (Salvagiotti et al., 2008) may have contributed to lower soybean biomass at intermediate N levels. Tissue N concentration may assist with confirming this hypothesis, however, these data were not collected in the current study. Alternatively, the level of soil mineral N that resulted in the lowest soybean biomass (159 kg N ha-1) was similar to that which resulted in the greatest volunteer canola biomass (174 kg N ha-1) (Figure 4). Like seed production (Figure 3), biomass of all volunteer canola populations responded similarly to soil mineral N and differed among earlier- and later-seeded environments in magnitude only (P = 0.008) (Figure 4). These biomass data showed that interference from volunteer canola in soybean peaked consistently at soil mineral N between 159 and 174 kg N ha-1. An association among soybean and volunteer canola plant densities (Supplementary Figures S1, S2) and their biomass in response to soil mineral N (Figure 4) was not apparent (Pearson r = -0.004 and -0.198, respectively). Together, these results support the hypothesis that increased soil mineral N in soybean causes greater interference from volunteer canola. These results also build upon our previous work (Geddes and Gulden, 2018) which suggested that planting soybean in lower-N environments could limit interference from volunteer canola.
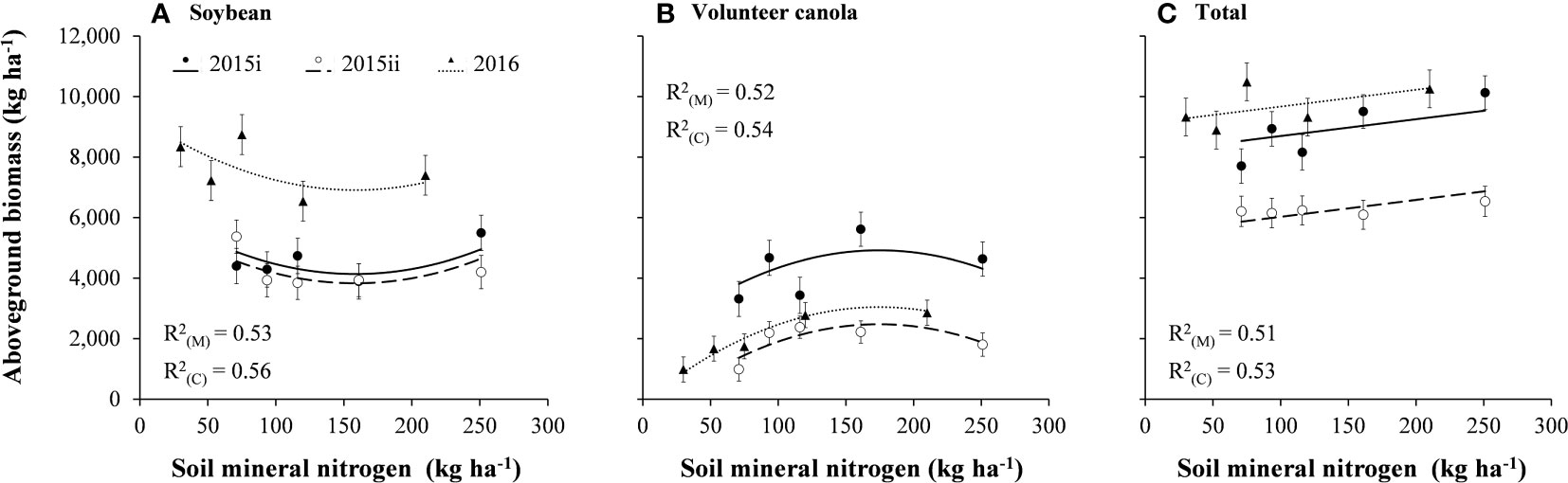
Figure 4 Aboveground biomass of (A) soybean, (B) volunteer canola, and (C) soybean plus volunteer canola (total) in response to soil mineral nitrogen in the 2015i, 2015ii, and 2016 environments. Dots indicate means of aboveground biomass at each level of soil mineral nitrogen in each environment in a combined analysis of volunteer canola populations. Error bars indicate ± SEM. All linear and/or quadratic components were significantly different from 0 (α = 0.05). Marginal (R2(M)) and conditional (R2(C)) coefficients of determination are indicated for the entire linear mixed effects model. All model parameters are described in Supplementary Table S1.
3.3 Environment
The number of volunteer canola seeds produced per unit area in the 2015i environment was consistently greater (by about 26,400 to 31,800 seeds m-2) than the 2015ii and 2016 environments (P = 0.001) (Figure 3). Likewise, volunteer canola biomass was greater by 1,880 to 2,440 kg ha-1 in the 2015i environment compared with the 2015ii and 2016 environments (P = 0.008) (Figure 4). The difference in volunteer canola biomass and seed production between these environments did not correspond with differences in soybean biomass (Figures 3, 4). Instead, soybean biomass was about 2,770 to 3,080 kg ha-1 greater in the 2016 environment compared with the 2015i and 2015ii environments (P < 0.001) (Figure 4). Differences in soybean biomass among these environments (Figure 4), however, did not manifest as differences in soybean yield (Supplementary Table S2).
Soil residual nitrate-N in each environment was in the lower range of soil mineral N that is still representative of both conventional and organic cropping systems in this region (Entz et al., 2001; AGVISE Laboratories, 2016). Soil residual N was 41 kg N ha-1 lower in the 2016 environment (30 kg N ha-1) than the 2015 environments (71 kg N ha-1) (Table 1). The other macronutrients were considered non-limiting and did not warrant additional fertilizer application. In each year, growing-season air temperatures closely followed that of the climatic normal (Figure 1). The amount of spring precipitation received near seeding (in May) was greater than normal in both years and reached 99 mm in 2015 and 108 mm in 2016 compared with the 30-year normal of 70 mm. The total amount of precipitation received between seeding and harvest was 340 mm, 260 mm and 292 mm in the 2015i, 2015ii and 2016 environments, respectively. Hence, greater growing-season precipitation in the 2015i environment may have contributed, in part, to greater volunteer canola biomass (Figure 4) and seed production (Figure 3) compared with the 2015ii and 2016 environments.
3.4 Ecological and management implications
Plant interference is comprised of both resource-dependent (direct) and resource-independent (indirect) competition (Harper, 1977). Resource-dependent competition describes rapid uptake of resources such as nutrients or water, while resource-independent competition describes morphological changes induced by the plant sensing environmental cues prior to the onset of direct competition (e.g., shade avoidance response). Plant interference, however, also can include other plant relationships not necessarily resulting in a negative outcome for both species (e.g., allelopathy). The resource-ratio hypothesis predicts the outcome of direct competition (Tilman, 1982), but ignores the broader implications of plant interference that also include indirect competition. The current study measured the interference of volunteer canola in soybean as a whole, but did not target direct competition alone because it is challenging to separate direct from indirect plant interference in field studies (Duke, 2015). Nevertheless, the current study showed clearly that the resource-ratio hypothesis predicted the outcome of interference between soybean and volunteer canola, indicating that direct competition likely played a pivotal role during interference between these two species. However, further research is warranted to reveal the mechanistic basis underpinning the relationship of soil mineral N and volunteer canola interference in soybean.
Investigation of other crop/weed species combinations is warranted to fully understand the utility of exploiting the resource-ratio hypothesis as a cultural weed management tool in legume production. The optimal level of N fertility differs among soybean and other legume species that meet less of their total N demand via N-fixation. Nevertheless, the current study agrees with other reports of reduced dry bean (Phaseolus vulgaris L.) competitiveness with weeds following N fertilization (Ugen et al., 2002; Saberali et al., 2012) which indicates that the utility of the resource-ratio hypothesis as a weed management tool likely extends beyond soybean and volunteer canola interference.
The current study showed the successful exploitation of the resource-ratio hypothesis as a cultural tool to shift the competitive balance away from volunteer canola and in favor of soybean. The resource-ratio hypothesis met both prerequisite criteria used to test novel tools for weed management, including (a) differential impact on the crop and weed species, and (b) consistency of efficacy. Soil mineral N affected soybean and volunteer canola differently under lower- and higher-N conditions. Under lower-N conditions, volunteer canola aboveground biomass and seed production were suppressed to as low as one-quarter those of higher-N conditions (30 vs. 183 kg N ha-1 in 2016). Soybean grain yield under volunteer canola interference was the greatest in these same conditions (17% greater than higher-N conditions). Very low levels of soil mineral N (≤ 15 kg N ha-1), however, were required to completely alleviate soybean yield losses caused by volunteer canola interference. These effects of soil mineral N on yield loss caused by weed interference were consistent among volunteer canola populations and environments. Crop and weed biomass and weed seed production had similar relationships in response to soil mineral N in all of the weed populations and differed among environments in magnitude only. Therefore, in the presence of non-leguminous weed species, legume crops have the potential to benefit from (a) being grown in fields depleted of soil mineral N (Geddes and Gulden, 2018), (b) avoiding or limiting N fertilization (Song et al., 2021), and (c) the use of cover crops (Wells et al., 2013; Menalled et al., 2021) or inter-seeded living mulches (Geddes and Gulden, 2021) to assimilate and immobilize soil mineral N thereby limiting its availability to weeds. These results also suggest that N fertilization in legume crops may result in increased severity of yield losses when weeds are not managed adequately. The current study indicates that the resource-ratio hypothesis can be an effective tool for weed management and should be considered as one component of a comprehensive IWM program in legume production.
Data availability statement
The raw data supporting the conclusions of this article will be made available by the authors, without undue reservation.
Author contributions
CG and RG conceptualized, designed the study, analysed the data, interpreted the results, and edited the draft manuscript. CG conducted the experiments, collected the data, and wrote the first draft. RG secured funding and supervised the research. All authors contributed to the article and approved the submitted version.
Funding
This research was funded by the Canada and Manitoba governments through Growing Forward 2, a federal–provincial–territorial initiative, and the Manitoba Pulse and Soybean Growers. CG was supported by a Natural Sciences and Engineering Research Council of Canada Industrial Postgraduate Scholarship and a University of Manitoba Graduate Fellowship.
Acknowledgments
The authors thank Rebecca Dueck for providing technical assistance.
Conflict of interest
The authors declare that the research was conducted in the absence of any commercial or financial relationships that could be constructed as a potential conflict of interest.
Monsanto Canada Inc. provided soybean seed for the research, but did not contribute to study design, collection, analyses, interpretation of data, the writing of this article or the decision to submit for publication.
Publisher’s note
All claims expressed in this article are solely those of the authors and do not necessarily represent those of their affiliated organizations, or those of the publisher, the editors and the reviewers. Any product that may be evaluated in this article, or claim that may be made by its manufacturer, is not guaranteed or endorsed by the publisher.
Supplementary material
The Supplementary Material for this article can be found online at: https://www.frontiersin.org/articles/10.3389/fagro.2022.1040241/full#supplementary-material
References
AGVISE Laboratories (2016) Nutrient summary Canada 2016. Available at: https://www.agvise.com/soil-test-summaries (Accessed August 02, 2017).
Beckie H. J., Johnson E. N., Blackshaw R. E., Gan Y. (2008). Weed suppression by canola and mustard cultivars. Weed Technol. 22, 182–185. doi: 10.1614/WT-07-126.1
Beckie H. J., Warwick S. I. (2010). Persistence of an oilseed rape transgene in the environment. Crop Prot. 29, 509–512. doi: 10.1016/j.cropro.2009.11.013
Blackshaw R. E., Brandt R. N., Janzen H. H., Entz T., Grant C. A., Derksen D. A. (2003). Differential response of weed species to added nitrogen. Weed Sci. 51, 532–539. doi: 10.1614/0043-1745(2003)051[0532:DROWST]2.0.CO;2
Blackshaw R. E., Molnar L. J., Janzen H. H. (2004). Nitrogen fertilizer timing and application method affect weed growth and competition with spring wheat. Weed Sci. 52, 514–622. doi: 10.1614/WS-03-104R
Blackshaw R. E., Semach G., Li X., O’Donovan J. T., Harker K. N. (2000). Tillage, fertilizer and glyphosate timing effects on foxtail barley (Hordeum jubatum) management in wheat. Can. J. Plant Sci. 80, 655–660. doi: 10.4141/P99-132
Brar N., Lawley Y. (2020). Short-season soybean yield and protein unresponsive to starter nitrogen fertilizer. Agron. J. 112, 5012–5023. doi: 10.1002/agj2.20378
Carkner M. K., Entz M. H. (2017). Growing environment contributes more to soybean yield than cultivar under organic management. Field Crops Res. 207, 42–51. doi: 10.1016/j.fcr.2017.02.019
Cavalieri A., Harker K. N., Hall L. M., Gulden R. H. (2016). Evaluation of the causes of on-farm harvest losses in canola in the northern great plains. Crop Sci. 56, 2005–2015. doi: 10.2135/cropsci2016.01.0014
Cutforth H., McConkey B., Brandt S., Gan Y., Lafond G., Angadi S., et al. (2009). Fertilizer n response and canola yield in the semiarid Canadian prairies. Can. J. Plant Sci. 89, 501–503. doi: 10.4141/CJPS08128
Di Tomaso F. M. (1995). Approaches for improving crop competitiveness through the manipulation of fertilization strategies. Weed Sci. 43, 491–497. doi: 10.1017/S0043174500081522
Duke S. O. (2015). Proving allelopathy in crop-weed interactions. Weed Sci. 63 (sp1), 121–132. doi: 10.1614/WS-D-13-00130.1
Entz M. H., Guilford R., Gulden R. (2001). Crop yield and soil nutrient status on 14 organic farms in the eastern portion of the northern great plains. Can. J. Plant Sci. 81, 351–354. doi: 10.4141/P00-089
Environment Canada (2017) Past weather and climate: Historical data. Available at: www.climate.weather.gc.ca (Accessed February 26, 2017).
Fageria N. K., Baligar V. C. (2005). Enhancing nitrogen use efficiency in crop plants. Adv. Agron. 88, 97–185. doi: 10.1016/S0065-2113(05)88004-6
Geddes C. M. (2021). Burial environment drives seed mortality of kochia (Bassia scoparia), wild oat (Avena fatua), and volunteer canola (Brassica napus) irrespective of crop species. Plants 10, 1961. doi: 10.3390/plants10091961
Geddes C. M., Gulden R. H. (2017). Early autumn soil disturbance decreases persistence of volunteer summer-annual oilseed rape (Brassica napus). Weed Res. 57, 182–192. doi: 10.1111/wre.12248
Geddes C. M., Gulden R. H. (2018). Candidate tools for integrated weed management in soybean at the northern frontier of production. Weed Sci. 66, 662–672. doi: 10.1017/wsc.2018.32
Geddes C. M., Gulden R. H. (2021). Wheat and cereal rye inter-row living mulches interfere with early season weeds in soybean. Plants 10, 2276. doi: 10.3390/plants10112276
Gregoire P., Rosset J. D., Gulden R. H. (2021). Volunteer Brassica napus (L.) interference with soybean [Glycine max (L.) merr.]: Management thresholds, plant growth, and seed return. Can. J. Plant Sci. 101, 556–567. doi: 10.1139/cjps-2020-0258
Gulden R. H., Shirtliffe S. J., Thomas A. G. (2003). Secondary seed dormancy prolongs persistence of volunteer canola in western Canada. Weed Sci. 51, 904–913. doi: 10.1614/P2002-170
Johri A. K., Singh G., Sharma D. (1992). Nutrient uptake by wheat and associated weeds as influenced by management practices. Trop. Agric. 69, 391–393.
Kirkland K. J., Beckie H. J. (1998). Contribution of nitrogen fertilizer placement to weed management in spring wheat (Triticum aestivum). Weed Technol. 12, 507–514. doi: 10.1017/S0890037X00044225
Leeson J. Y., Gaultier J., Grenkow L. (2017). “Manitoba Weed survey of annual crops in 2016,” in Weed survey series publ. 17-2 (Saskatoon, SK: Agriculture and Agri-Food Canada), 203 p.
Liebman M., Robichaux R. H. (1990). Competition by barley and pea against mustard: Effects on resource acquisition, photosynthesis and yield. Agric. Ecosyst. Environ. 31, 155–172. doi: 10.1016/0167-8809(90)90217-2
Li M., Jordan N. R., Koide R. T., Yannarell A. C., Davis A. S. (2016). Meta-analysis of crop and weed growth responses to arbuscular mycorrhizal fungi: Implications for integrated weed management. Weed Sci. 64, 642–652. doi: 10.1614/WS-D-16-00050.1
Littell R. C., Milken G. A., Stroup W. W., Wolfinger R. D., Schabenberger O. (2006). SAS for mixed models. 2nd edn (Cary, NC: SAS Institute), 834 p.
Lund R. E. (1975). Tables for an approximate test for outliers in linear models. Technometrics 17, 473–476. doi: 10.1080/00401706.1975.10489374
Luschei E. C., Jackson R. D. (2005). Research methodologies and statistical approaches for multitactic systems. Weed Sci. 53, 292–403. doi: 10.1614/WS-04-123R
Menalled U. D., Pethybridge S. J., Pelzer C. J., Smith R. G., DiTommaso A., Ryan M. R. (2021). High seeding rates and low soil nitrogen environments optimize weed suppression and profitability in organic no-till planted soybean. Front. Agron. 3, 578567. doi: 10.3389/fagro.2021.678567
Mesbah A. O., Miller S. D. (1999). Fertilizer placement affects jointed goatgrass (Aegilops cylindrical) competition in winter wheat (Triticum aestivum). Weed Technol. 13, 374–377. doi: 10.1017/S0890037X00041889
Mierau A., Kurtenbach M. E., Johnson E. N., Gulden R. H., Weber J. D., May W. E., et al. (2020). Herbicide programs for control of glyphosate-resistant canola (Brassica napus) in glyphosate-resistant soybean. Weed Technol. 34, 540–546. doi: 10.1017/wet.2020.2
Miller T. E., Burns J. H., Munguia P., Walters E. L., Kneitel J. M., Richards P. M., et al. (2005). A critical review of twenty years’ use of the resource-ratio theory. Am. Nat. 165, 439–448. doi: 10.1086/428681
Nakagawa S., Schielzeth H. (2013). A general and simple method for obtaining R2 from generalized linear mixed-effects models. Methods Ecol. Evol. 4, 133–142. doi: 10.1111/j.2041-210x.2012.00261.x
Onofri A., Carbonell E. A., Piepho H. P., Mortimer A. M., Cousens R. D. (2010). Current statistical issues in weed research. Weed Res. 50, 5–24. doi: 10.1111/j.1365-3180.2009.00758.x
Osborne S. L., Riedell W. E. (2006). Starter nitrogen fertilizer impact on soybean yield and quality in the northern great plains. Agron. J. 98, 1569–1574. doi: 10.2134/agronj2006.0089
Piepho H. P., Williams E. R., Fleck M. (2006). A note on the analysis of designed experiments with complex treatment structure. HortScience 41, 446–452. doi: 10.21273/HORTSCI.41.2.446
Rao A. S., Reddy K. S. (2010). “Nutrient management in soybean,” in The soybean: Botany, production, and usesEd. Singh G. (London, UK: CAB International), 161–190
Rasmussen P. E. (1995). Effects of fertilizer and stubble burning on downy brome competition in winter wheat. Commun. Soil Sci. Plant Anal. 36, 951–960. doi: 10.1080/00103629509369347
Saberali S. F., Modarres-Sanavy S. A. M., Bannayan M., Baghestani M. A., Mashhadi H. R., Hoogenboom G. (2012). Dry bean competitiveness with redroot pigweed as affected by growth habit and nitrogen rate. Field Crops Res. 135, 38–45. doi: 10.1016/j.fcr.2012.06.016
Salvagiotti F., Cassman K. G., Specht J. E., Walters D. T., Weiss A., Dobermann A. (2008). Nitrogen uptake, fixation and response to fertilizer n in soybeans: A review. Field Crops Res. 108, 1–13. doi: 10.1016/j.fcr.2008.03.001
Seerey N. J., Shirtliffe S. J. (2010). Commercial generations of Brassica napus cause greater yield loss in Triticum aestivum, than volunteer B. napus generations. Can. J. Plant Sci. 90, 777–783. doi: 10.4141/CJPS09187
Sheppard S. C., Bates T. E. (1980). Yield and chemical composition of rape in response to nitrogen, phosphorus and potassium. Can. J. Soil Sci. 60, 153–162. doi: 10.4141/cjss80-019
Song J.-S., Im J.-H., Kim J.-W., Kim D.-G., Lim Y., Yook M.-J., et al. (2021). Modeling the effects of nitrogen fertilizer and multiple weed interference on soybean yield. Agronomy 11, 515. doi: 10.3390/agronomy11030515
Tilman D. (1982). Resource competition and community structure. Princeton monographs in population biology Vol. 17 (Princeton, NJ: University Press), 296 p.
Tilman D. (1985). The resource-ratio hypothesis of plant succession. Am. Nat. 125, 827–852. doi: 10.1086/284382
Tungate K. D., Burton M. G., Susko D. J., Sermons S. M., Rufty T. W. (2006). Altered weed reproduction and maternal effects under low-nitrogen fertility. Weed Sci. 54, 847–853. doi: 10.1614/WS-05-145R.1
Ugen M. A., Wien H. C., Wortmann C. S. (2002). Dry bean competitiveness with annual weeds as affected by soil nutrient availability. Weed Sci. 50, 530–535. doi: 10.1614/0043-1745(2002)050[0530:DBCWAW]2.0.CO;2
Walley F., Clayton G. W., Miller P. R., Carr P. M., Lafond G. P. (2007). Nitrogen economy of pulse crop production in the northern great plains. Agron. J. 99, 1710–1718. doi: 10.2134/agronj2006.0314s
Keywords: competition, integrated weed management, fertilization, legume, nitrogen, plant interference, soil fertility, yield loss
Citation: Geddes CM and Gulden RH (2022) Exploiting the resource-ratio (R*) hypothesis for weed management in legume crops: An example of volunteer Brassica napus in soybean. Front. Agron. 4:1040241. doi: 10.3389/fagro.2022.1040241
Received: 09 September 2022; Accepted: 17 October 2022;
Published: 27 October 2022.
Edited by:
Michael Walsh, The University of Sydney, AustraliaReviewed by:
Maxwel Coura Oliveira, McGill University, CanadaMd Asaduzzaman, New South Wales Department of Primary Industries, Australia
Copyright © 2022 Geddes and Gulden. This is an open-access article distributed under the terms of the Creative Commons Attribution License (CC BY). The use, distribution or reproduction in other forums is permitted, provided the original author(s) and the copyright owner(s) are credited and that the original publication in this journal is cited, in accordance with accepted academic practice. No use, distribution or reproduction is permitted which does not comply with these terms.
*Correspondence: Robert H. Gulden, Um9iLkd1bGRlbkB1bWFuaXRvYmEuY2E=
†Present address: Charles M. Geddes, Agriculture and Agri-Food Canada, Lethbridge Research and Development Centre, Lethbridge, AB, Canada