- 1Faculty of Medicine and Health Sciences, Orthopedic Service, Department of Surgery, Université de Sherbrooke, Sherbrooke, QC, Canada
- 2Research Centre on Aging, Sherbrooke, QC, Canada
- 3Département des Sciences de l’activité Physique, Université du Québec à Montréal, Montreal, QC, Canada
- 4Centre de Recherche Institut Universitaire de Gériatrie de Montréal, Montreal, QC, Canada
- 5Laboratoire Scribens, Département de génie Électrique, École Polytechnique de Montréal, Montréal, QC, Canada
Background: Turning is a challenging mobility task requiring coordination and postural stability. Optimal turning involves a cranio-caudal sequence (i.e., the head initiates the motion, followed by the trunk and the pelvis), which has been shown to be altered in patients with neurodegenerative diseases, such as Parkinson’s disease as well as in fallers and frails. Previous studies have suggested that the cranio-caudal sequence exhibits a specific signature corresponding to the adopted turn strategy. Currently, the assessment of cranio-caudal sequence is limited to biomechanical labs which use camera-based systems; however, there is a growing trend to assess human kinematics with wearable sensors, such as attitude and heading reference systems (AHRS), which enable recording of raw inertial signals (acceleration and angular velocity) from which the orientation of the platform is estimated. In order to enhance the comprehension of complex processes, such as turning, signal modeling can be performed.
Aim: The current study investigates the use of a kinematic-based model, the sigma-lognormal model, to characterize the turn cranio-caudal signature as assessed with AHRS.
Methods: Sixteen asymptomatic adults (mean age = 69.1 ± 7.5 years old) performed repeated 10-m Timed-Up-and-Go (TUG) with 180° turns, at varying speed. Head and trunk kinematics were assessed with AHRS positioned on each segments. Relative orientation of the head to the trunk was then computed for each trial and relative angular velocity profile was derived for the turn phase. Peak relative angle (variable) and relative velocity profiles modeled using a sigma-lognormal approach (variables: Neuromuscular command amplitudes and timing parameters) were used to extract and characterize the cranio-caudal signature of each individual during the turn phase.
Results: The methodology has shown good ability to reconstruct the cranio-caudal signature (signal-to-noise median of 17.7). All variables were robust to speed variations (p > 0.124). Peak relative angle and commanded amplitudes demonstrated moderate to strong reliability (ICC between 0.640 and 0.808).
Conclusion: The cranio-caudal signature assessed with the sigma-lognormal model appears to be a promising avenue to assess the efficiency of turns.
Introduction
Functional mobility is a key component of the quality of life in older adults. Basic daily activities involve the execution of mobility tasks, such as walking, turning, standing up and sitting down. Turning, defined as a change in walking direction, is a specifically challenging mobility task which requires inter-limb coordination and postural stability to adequately follow the central nervous system instructions (Mancini et al., 2015a; Mellone et al., 2016). Turning must also be planned in advance to efficiently and safely process and execute the information leading to the modified trajectory (Patla et al., 1999). Deficits in postural transitions, such as turning, have been identified in frails (Galán-Mercant and Cuesta-Vargas, 2014) and persons with neurological deficits (Salarian et al., 2009; Mancini et al., 2015a) and are associated with a higher risk of falling (Mancini et al., 2016). It has also been shown that objective turn metrics (e.g., number of steps while turning) are able to identify individuals with mobility impairments better than traditional gait speed and clinical measures of mobility (Carpinella et al., 2007; Salarian et al., 2009; Zampieri et al., 2010; King et al., 2012; Spain et al., 2012). Consequently, studies have suggested an increased vulnerability to impairments during the turn compared to straight-line walking due to the complexity of the task and the neural systems involved (Herman et al., 2011). Recently, Hulbert et al. (2015) have suggested categorizing turning deficits into axial and perpendicular deficits, where perpendicular deficits relates to suboptimal movement in the limbs while axial deficits refers to inadequate movement of the head to trunk rotational axis. Perpendicular deficits would, therefore, include: an increased number of steps, related to the use of a compensatory strategy; a reduced step length, to maintain postural stability; and a modified turn strategy. Alternatively, axial deficits would include segment rigidity and segment rotation which would require the adoption of compensatory strategies, and segment coordination and timing, leading to overall uncoordinated movements. On a global scheme, all of these deficits may be viewed inter-related since full body control and coordination is required to safely execute a turn. Thus, Hulbert suggests that axial deficits may lead to altered control in perpendicular segments. If so, axial deficits may appear first and early assessment of such deficits may lead to better prevention.
In healthy individuals, it has been shown that efficient turning involves a cranio-caudal sequence of movement where the head initiates the motion, followed by the trunk and then the pelvis to efficiently steer the body into the desired new direction (Fuller et al., 2007; Hong et al., 2009). This sequence was shown to be altered in people with neurodegenerative disease and those who are recurrent fallers, exhibiting increased coupling of the segments (Ferrarin et al., 2006; Crenna et al., 2007; Hong et al., 2009; Wright et al., 2012; Spildooren et al., 2013). However, all of these observations were made in motion capture laboratories using camera-based stereophotogrammetric systems. Although powerful, such systems are expensive, complex to use, require a large dedicated space and have a constrained volume of acquisition (Zhou and Hu, 2008). As such, these systems are not well-adapted to clinical settings. To efficiently be used in a clinical context, a system must preferably be portable, configurable, relatively low-cost, easy to use, and output information must be easily interpreted from a clinical perspective (Ginsburg, 2005; Anderson et al., 2012; Gaudreault et al., 2012). Advances in wearable technology offer new possibilities for researchers and clinicians to assess mobility. Inertial measurement systems are among promising wearable sensors which have gathered an increasing interest in the past decade because of their portability, autonomy, acquisition frequency, and general form factor (size, and configuration) (Zhou and Hu, 2008; Horak et al., 2015). Inertial measurement systems include attitude and heading reference systems (AHRS), also referred to in the literature as magnetic and inertial measurement unit (MIMU), magnetic angular rate and gravity sensor, or Inertial and Meagnetic Unit (MIMU). AHRS are comprised of 3-axes accelerometers, gyroscopes, and magnetometers from which information is fed into a fusion algorithm to estimate the orientation of the module in a global reference frame based on gravity and magnetic North. Therefore, using multiple AHRS affixed on contiguous segments makes it possible to assess a person’s joints kinematics in different contexts. The diversity of sensors included within AHRS makes them good representative of commonly named movement monitors. This measurement system allows not only the quantity of activity performed to be monitored but also the quality of that motion through spatiotemporal gait and turn characteristics analysis as well as joint kinematics (Horak et al., 2015; Lebel et al., 2016).
Although multiple studies have used AHRS to assess mobility, the focus has always been on the raw sensors’ information (i.e., acceleration and/or segment angular velocity). Consequently, turn duration and turn speed were identified as useful measures to characterize age-related changes (Sheehan et al., 2014; Vervoort et al., 2016), identify recurrent fallers from non-fallers (Greene et al., 2010; Zakaria et al., 2015; Mancini et al., 2016), differentiate between healthy controls and early Parkinson’s disease patients (Salarian et al., 2009, 2010; Zampieri et al., 2010; El-Gohary et al., 2013; Mancini et al., 2015a), and frails (Galán-Mercant and Cuesta-Vargas, 2014). Although segment and joint orientation information may provide information on a person’s functional capabilities that is more easily interpreted, it is far less exploited. Validity studies have proven that the accuracy of the orientation data is sufficient for coarse clinical kinematic assessment (Ferrari et al., 2010; Zhang et al., 2013; Lebel et al., 2017). However, literature also clearly highlights possible variations in accuracy with changing magnetic environment (Roetenberg et al., 2007; Palermo et al., 2014; Schiefer et al., 2014; Yadav and Bleakley, 2014) while accuracy has also been shown to vary across joints and tasks (Palermo et al., 2014; Lebel et al., 2016). Recently, Lebel et al. (2017) suggested that this variation may be partly linked to an optimal region of operation for segment angular velocity. These uncertainties regarding orientation data accuracy may explain the current underutilization of such data. However, these limitations are mainly present in extremity kinematics, where segment velocities are higher and magnetic perturbations are more common (Palermo et al., 2014; Lebel et al., 2017). During a turn, both the head and the trunk’s angular velocity are within the optimal region of operation and magnetic perturbations can be assumed as minimal. Hence, the kinematic variation of the head relative to the trunk during a turn appears to be a good candidate to investigate the added value of AHRS orientation data analysis to derive meaningful clinical outcomes.
Traditionally, cranio-caudal sequence is assessed in biomechanics laboratories using camera-based stereophotogrammetric systems and analyzed in the temporal domain. Differences in temporal sequences are interpreted to be linked to different turning strategies. Such interpretations suggest that the cranio-caudal sequence exhibits a specific signature according to the adopted turn strategy. The so-called movement signature concept corresponds to the specific way (timing, force, amplitude, velocity) the movement is performed. Through signal modeling, the complex system involved in human movement can be reduced to a simpler form in order to better understand it. In this specific case, signal modeling is believed to provide insights into the mobility deficits. Human movement can be modeled using different paradigms which include, but are not limited to: equilibrium point models, minimization-based models, kinematic-based models and neural networks (Plamondon et al., 2014). Based on the Kinematics Theory, human movement can be seen as the cumulative response of an important number of biological systems (Plamondon, 1995a,b, 1998; Plamondon et al., 2003). Each system will produce a velocity vector from which their cumulative sum will, in the end, result in the movement of a segment. The motion can, therefore, be seen as the spatiotemporal representation of the energy induced on a specific body segment. The different systems involved in the planning and the execution of a specific task is controlled by the central nervous system. Therefore, assessment of human motion produced during a specific task can provide insights into the fundamentals of the motor control system (Wolpert et al., 1995). Analysis of the human motion through linear system modeling and an impulse response approach, therefore, seems to be a promising avenue for better characterization and early identification of motor control system deficits. Among those kinematic-based models are the delta- and sigma-lognormal models (Plamondon, 1995a,b; Djioua, 2007). These models rely on mathematical grounds to demonstrate that the lognormal function properly models the impulse response of the neuromuscular network in the case of rapid movements and can be seen as the optimal representation of the movement’s kinematics (Djioua and Plamondon, 2009). Their applications ranges from human motor control phenomena explanations and the factors affecting it (Plamondon and Alimi, 1997; Plamondon et al., 2013a) to scripted signature verification (Djioua and Plamondon, 2009; Woch and Plamondon, 2010; Woch et al., 2011; Plamondon et al., 2013b; Diaz et al., 2016) and detection of fine motor control problems (O’Reilly and Plamondon, 2011; O’Reilly et al., 2014) as well as applications to monitor the evolution of fine motor control in kindertgarden children (Duval et al., 2015; Rémi et al., 2015). Indeed, directional rapid movements produce an asymmetrical bell-shaped velocity profile. This can be represented by lognormal functions with characteristic parameters and can be related to the system commands and its ability to respond (command impulse delay, command magnitude, execution delay, and response time). However, can such model be used to analyze axial control specifically? Preliminary studies within the angular domain have shown that the wrist flexion and extension in monkeys could be fit very well with a delta-lognormal model (Plamondon, 1995a), but no extensive study has further explore the interest of using the Kinematic Theory for the analysis of angular movement control.
This study investigates the possibility of characterizing the turn cranio-caudal signature via a sigma-lognormal model using the head relative to the trunk velocity profile derived from the orientation data assessed with AHRS. Specifically, this paper aims at (i) presenting and illustrating the methods required for head-trunk signature recognition based on AHRS recording of motion and (ii) evaluating the robustness and the reliability of the identified cranio-caudal signature parameters.
Materials and Methods
Protocol and Instrument
The present study experimental protocol is based on the execution of a 10-m Timed-Up-and-Go (TUG). The TUG is a clinically recognized test to assess mobility and balance which combines basic mobility tasks (sit-to-stand, walk and turn) (Rehabilitation Institute of Chicago, 2010). Upon signal, the participant stands-up, walks out to the 10-m mark, turns around, and walks back to his initial seated position (Figure 1A).
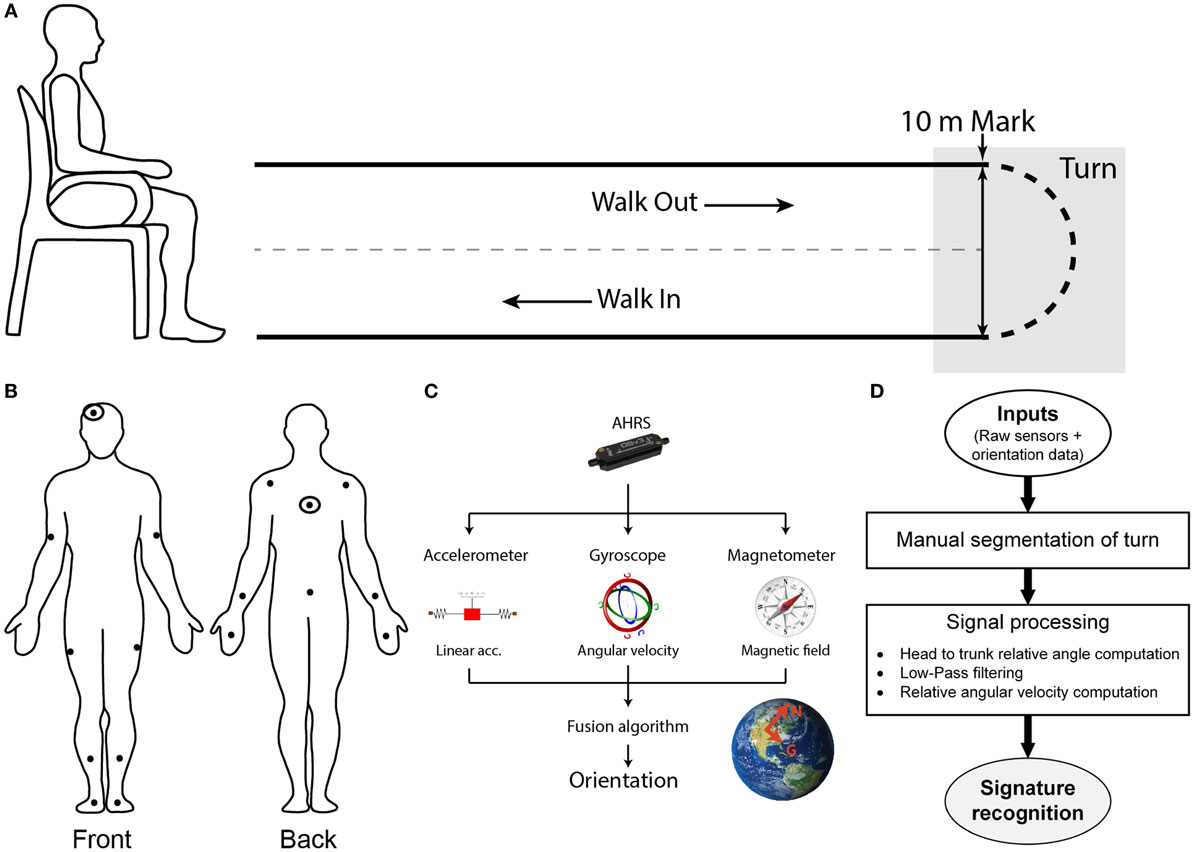
Figure 1. Setup, protocol, and methodology. (A) Spatial schematic of a 10-m Timed-Up-and-Go (TUG) task. Participants initiate the task sitting on a chair. Upon signal, the participant stands-up, walk out for 10 m, turn around when the 10-m mark is reached, walks back toward the chair and sits down. The turn portion of the TUG is targeted for the present study. (B) Participants are equipped with a suit comprised of sensors which position are illustrated this diagram. Signals from the double-marked sensors (head and trunk) were used for the signature recognition. (C) Sensors used are composed of 3-axis accelerometer, gyroscope, and magnetometer to measure linear acceleration, angular velocity, and magnetic field. All of the data are passed on to the fusion algorithm embedded in the sensor estimate the orientation of the module, expressed in an Inertial reference frame. (D) Global workflow of the algorithm to recognize the cranio-caudal signature of a turn.
To enable assessment of kinematics, participants are instrumented with the IGS-180 suit (Synertial Ltd., UK) containing 17 AHRS (OS3D, Inertial Labs, USA) as shown in Figure 1B. Each AHRS measures raw inertial signals (segment linear acceleration, angular velocity and magnetic fields) and derives the orientation of the module, and hence the orientation of the segment it is attached to, in a global reference frame (Figure 1C). A validity study performed on this system revealed an acceptable accuracy and an excellent agreement for both the head and trunk sensors when compared with an optoelectronic gold standard during a turn (Lebel et al., 2017). The IGS-180 enables acquisition of data (raw inertial data and orientation data) over its 17 sensors at 60 Hz. Sensor to body alignment, required to express the sensor movement into anatomical planes of reference, is performed with the participant standing in a neutral position (standing up, looking straight-ahead with palms facing their thighs) at the beginning of each trial.
Signal Processing
Figure 1D gives an overview of the global workflow of the algorithm, including the signal processing. Trials are manually reviewed and segmented using the avatar in IGS-Bio, the application available with the IGS-180. Specifically, the procedure described below was followed to ensure systematic segmentation of the turns:
i. visual identification of the point in time at which a misalignment between the head–trunk–pelvis axis appears;
ii. establishment of the beginning of the previous gait cycle (i.e., heel strike preceeding initial misalignment) → Beginning of turn;
iii. identification of the point in time at which the head-trunk-pelvis axis is realigned; and
iv. localization of the beginning of the next gait cycle (i.e., heel strike following realignment) → End of turn;
All trials were segmented by the same evaluator in order to avoid bias. Further signal processing is performed in Matlab v2015a (MathWorks, USA). For each trial, the relative orientation of the head to the trunk is computed and expressed in anatomical planes of reference. The resulting relative angle signals are then filtered using a fourth order low-pass Butterworth filter with a cutoff frequency of 1.5 Hz. The cutoff frequency was determined from a residual-based analysis of the relative orientation signal, using an acceptable threshold of 2° and was performed over repeated trials (Carbonneau et al., 2013). The residual threshold was based on the reported accuracy of orientation data obtained with the present system (Lebel et al., 2013, 2017). For each trial, the cutoff frequency that yielded the acceptable residual threshold was calculated. The final cutoff frequency was calculated from the mean and SD values obtained over repeated trials analysis to cover 95% of the cases. The resulting filtered angle profile was then transferred back into its quaternion form and used to compute the relative angular velocity profile.
Let us define
Then, the quaternion may be expressed as:
The angular velocity of the head relative to the trunk (ω) can then be determined by Eq. 4 (Rico-Martinez and Gallardo-Alvarado, 2000).
The axial component of the angular velocity, corresponding to the axial velocity profile of the head relative to the trunk, is then available to be used for further signature analysis.
Conceptual Framework and Parameters of Turn Signature
The optimal turn cranio-caudal sequence generates a change in relative angular orientation of the head to the trunk which segments are realigned upon completion of the transition. The turn cranio-caudal signature conceptual framework, therefore, has two main components: the analysis of the relative head to trunk maximum angle reached during the turn and the investigation of the relative angular velocity profile derived from it via the sigma-lognormal model approach.
Relative Angular Velocity Profile Analysis
According to the Kinematics Theory, the impulse response of the neuromuscular system (NMS) can be identified by analyzing the characteristics of the movement itself. If it is assumed that the NMS encompasses the motor cortex down to the muscles, all neuronal activities processed prior to the NMS consequently translates into a delay in the impulse command sent to the system. The NMS itself is made of multiple motor units which can be modeled as non-linear sub-systems organized in such a way that allows them to work efficiently (Plamondon, 1995a; Djioua, 2007). The impulse response of such linearized system follows an asymmetric positive bell-shaped curve described by a lognormal function. If one considers the control strategy of a movement from an energy point of view, the velocity of the end effector becomes the basic unit of the motion and should, therefore, follow a lognormal profile. Thus, Plamondon and his team proposed and validated the use of the sigma-lognormal model on the velocity profile to analyze the human motion during scripted signature (Plamondon, 1995a; Plamondon et al., 2003; Djioua, 2007; Djioua and Plamondon, 2009; O’Reilly and Plamondon, 2009; Javier et al., 2013).
Here, we use the sigma-lognormal model to characterize the turn cranio-caudal signature. The two segments involved (head and trunk) can be seen as two NMSs, each one having its own lognormal impulse response. The output of each of these systems will, therefore, follow a lognormal profile for simple movements. In our study, we are interested in analyzing a more complex NMS, the head-trunk system, from which output can be seen as the vectorial summation of both basic systems outputs. Specifically, the cranio-caudal velocity profile can be decomposed into two phases corresponding to the moment the head initiates the turn, moving away from the trunk (phase 1) and the moment the trunk engages into the turn, closing the gap with the head (phase 2). We can, therefore, mathematically describe this complex system as the substraction of the two illustrated velocity profiles (Figure 2A; Eq. 5). The impulse response of the NMS is a lognormal (Plamondon et al., 2003), asymmetric bell-shaped curve (Figure 2B) from which the exact representation follows the equation in the insert and depends upon the magnitude of the commanded signal (D), the time occurrence of this command (t0), the execution delay (μ) and the response time (σ). The latter two were defined on a log scale. Indeed,
where t0i is the time of occurrence of the ith input command; μ is the log time delay of the NMS, the time delay on a logarithmic scale; σ is the log response time of the NMS, the response time on a logarithmic scale; and D is the amplitude of the command sent to the NMS.
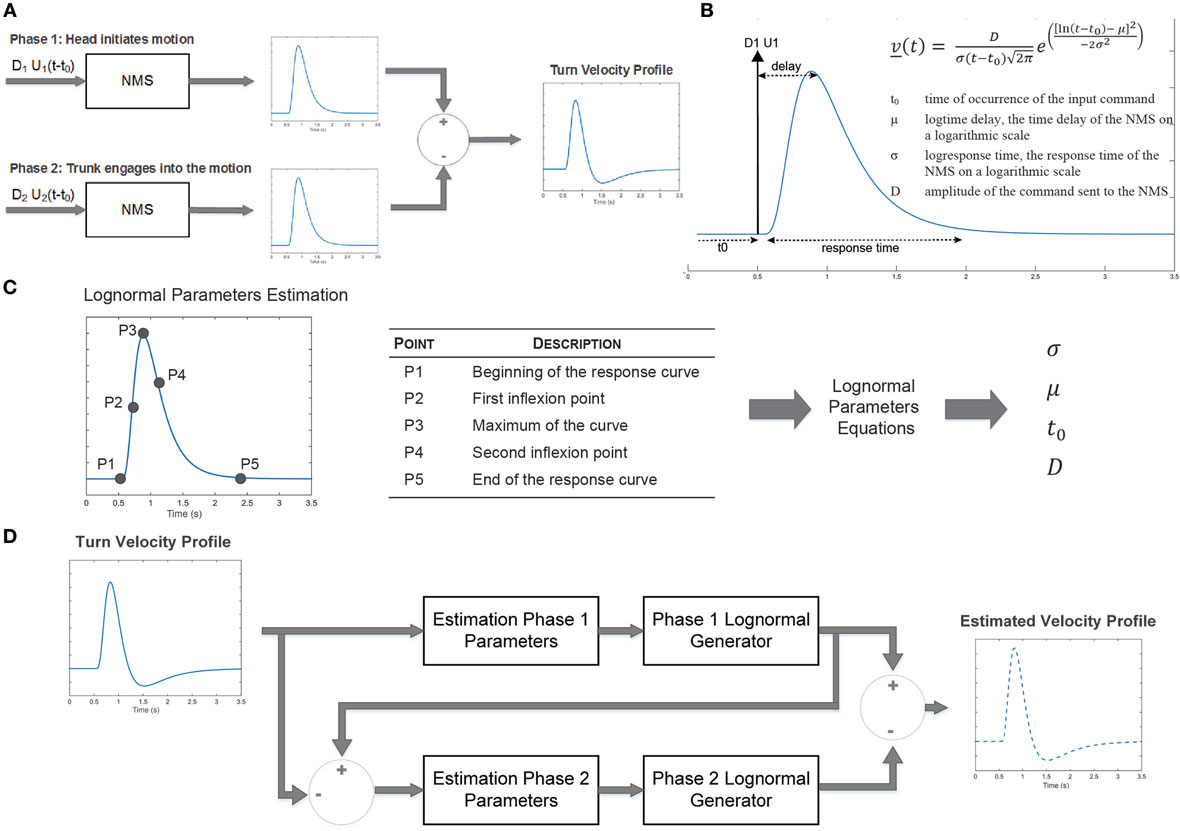
Figure 2. Sigma-log normal model conceptual framework. (A) Upon initiation of a turn, a first command is sent to the neuromuscular system (NMS) to initiate the head motion. A second command is sent to initiates the movement of the trunk. The difference of the NMS impulse responses generates the head to trunk velocity profile corresponding to the cranio-caudal signature. (B) The NMS impulse response is characterized by an asymmetric bell-shaped curved which can be characterized by the delay between command initiation and the median of the velocity as well as the response time. (C) Parameters of the sigma-lognormal profile can be estimated through the localization of specific points on the curve. (D) The sigma-lognormal model estimates the parameters of the two lognormal signal phases from which the velocity profile is estimated.
The lognormal equation parameters may be calculated using specific points of the velocity profile (Figure 2C) following equations, Eqs. 6–9 (Djioua, 2007; Djioua and Plamondon, 2009; O’Reilly and Plamondon, 2009).
Indeed, from the velocity signal it is possible to identify the time at which the motion is initiated and terminated, the time at which the maximum velocity is reached as well as both inflection points. These points are first identified for phase 1 of the motion. The lognormal model parameters are then derived from these points and phase 1 response is estimated. A similar process is followed for phase 2, allowing a full reconstruction of the velocity signal (Figure 2D). From the estimated lognormal equation parameters, it is also possible to deduce further characteristics of the lognormal impulse response which could help interpret the NMS. The time delay , defined as the rapidity at which the system responds to the command, and the time response (s), corresponding to the time it takes the system to react and execute the movement, are defined by Eqs. 10 and 11, respectively (Plamondon et al., 2003).
Finally, the quality of the reconstructed signature is evaluated using a signal-to-noise ratio (SNR) approach described in equation Eq. 12, as proposed by O’Reilly and Plamondon (2009).
In Eq. 12, v corresponds to the measured velocity profile, while is the reconstructed or estimated profile.
Experimental Concept Overview
The complete set of metrics proposed for characterization of the turn cranio-caudal signature is summarized in Table 1. In order for these parameters to be of true interest, they must be robust to task velocity natural variation and be reliable.
Detailed Experimental Protocol and Participants
The robustness and reliability of the proposed approach was tested on a sample of older adults. The project was approved by the Centre de Recherche de l’Institut Universitaire de Gériatrie de Montreal ethics board and participants provided written informed consent. Sixteen asymptomatic adults aged between 55 and 83 years old (mean age = 69.1 years, 50% female, height = 1.61 ± 0.08 m, weight = 63.2 ± 10.1 kg; BMI = 24.3 ± 3.2 kg/m2) participated in the study. Participants performed repeated 10-m TUGs equipped with the IGS-180, as explained in Section “Protocol and Instrument.” TUGs were executed both at normal and fast paces, each condition being repeated twice.
Traditional Metrics
For comparison purposes, data were also analyzed using traditional metrics. As such, the accelerometer signal from the trunk AHRS was analyzed to determine the number of steps the participants took during the turn (Salarian et al., 2010). Analysis of the number of steps is based on a threshold on the acceleration measured by the trunk sensors. Validity of the method was assessed by visual comparison over five trials. Mean and max angular velocity during obtained during the turn was computed using the angular velocity data provided by the trunk AHRS’ gyroscope (Salarian et al., 2009, 2010; Mancini et al., 2015a).
Data Analysis
For each trial, the introduced cranio-caudal signature metrics were calculated along with the traditional turn parameters.
A quality control process ensured that only the trials with a SNR greater than 10 dB were kept. The selected threshold is slightly lower than the generally accepted rule for SNR in controlled experiments (usually 15 dB), but this threshold was shown to be satisfying in this specific context. Indeed, this slightly more permissive SNR takes into account the complexity of the experiment and the possible sources for uncertainties such as the manual segmentation of the turn from the TUG task. The effects of velocity on the different metrics as well as their reliability were then analyzed. The robustness of the cranio-caudal turn signature metrics to natural task-related velocity variations and their reliability over repeated trials are important properties that need to be established before their validity can be further explored. All statistical analyses were performed using SPSS (v23.0.0 from IBM) and considered a significance level of 0.05.
Velocity Effect and Reliability
Each participant performed four TUGs (two at a normal pace, two at a fast pace). The effect of velocity on the metrics was, therefore, evaluated by taking the mean of each metric per participant and velocity and comparing them using a Wilcoxon signed-rank test. Reliability was assessed using a two-way random, absolute, average-measures intra-class correlation coefficient (Weir, 2005) performed on the repeated measurement of each metric [i.e., ICC(2,4) for absolute agreement]. The following guidelines were used for interpretation (Koo and Li, 2016):
• 0.00 ≤ ICC < 0.50 Poor reliability
• 0.51 ≤ ICC < 0.75 Moderate reliability
• 0.75 ≤ ICC < 0.90 Good reliability
• 0.91 ≤ ICC ≤ 1.00 Excellent reliability
Results
The ability of the sigma-lognormal model to estimate the cranio-caudal signature is shown in Figure 3. The left panel of this figure illustrates the variation in relative head to trunk angle captured during the turn for a healthy individual. The right panel corresponds to the relative head to trunk angular velocity profile for the same turn (blue curve—measured; red dotted curve—reconstructed profile using the sigma-lognormal approach). Analysis of the SNR revealed a median of 17.7 [14.6, 26.6], confirming the ability of the model to fit the data.
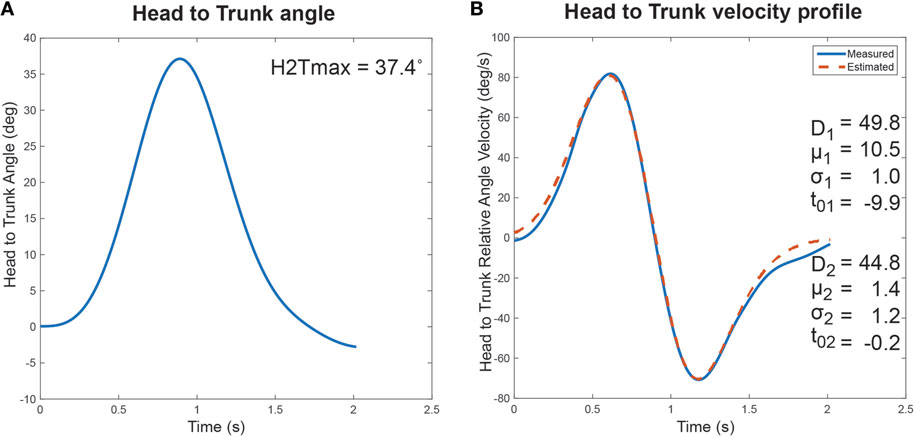
Figure 3. Cranio-Caudal Signature Determination. The proposed cranio-caudal signature approach is composed of both the analysis of the relative head to trunk angle achieved during the turn and the head to trunk relative angular velocity profile, modeled with the sigma-lognormal approach. (A) Change in head to trunk relative angle during a normal turn. The maximum angle reached is identified as a signature variable. (B) The blue curve illustrates the relative head to trunk angular velocity profile during the turn, as derived from the attitude and heading reference system measurement. The red dotted line illustrates the reconstructed profile, using the sigma-lognormal model. The parameters used to achieve the reconstruction are listed as inserts.
The robustness of the proposed parameters to velocity variations as well as their reliability shall now be verified.
Velocity Effect
Normal pace TUGs were significantly slower than fast TUG (Normal pace TUG duration: 20.3 ± 2.8 s; fast pace TUG duration: 17.0 ± 1.7 s; p = 0.001). Figure 4 illustrates the turn’s cranio-caudal signature captured for the same healthy individual performing a normal pace and a fast pace TUG.
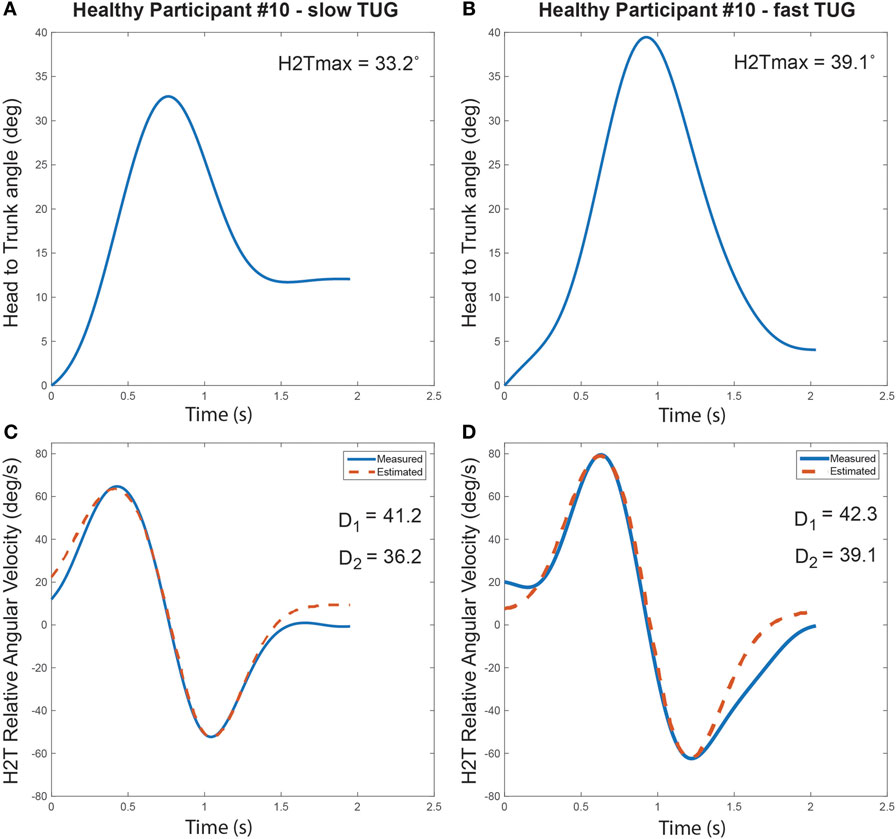
Figure 4. Turn cranio-caudal signature for a normal pace (A,C) and a fast pace Timed-Up-and-Go (TUG) (B,D), executed by the same healthy participant. (A,B) Relative head to trunk angle variation captured during the turns. (C,D) Measured and estimated relative head to trunk angular velocity profile captured during the turns along with the computed signature parameters.
The dispersion of the cranio-caudal signature metrics (H2Tmax and D1,2) across participants is shown in Figure 5. The averaged peak head to trunk angle reached during the turn varied from 25.6° ± 8.9° for normal pace TUG to 24.5° ± 8.4° for fast pace trials, a difference not statistically significant (p = 0.683). The difference between the commanded amplitudes computed for normal pace versus fast pace were not statistically different (D1 normal pace: 24.8 ± 12.3, D1 fast pace: 28.5 ± 11.0, p = 0.470; D2 normal pace: 29.2 ± 11.0, D2 fast pace: 24.1 ± 10.1, p = 0.124). Similarly, the pace of the trials also did not have any significant effect on the timing parameters (t01 normal pace: −4.40 ± 6.30 s, t01 fast pace: −4.37 ± 5.56 s, p = 0.836; t02 normal pace: −7.0 ± 6.3 s, t02 fast pace: −4.5 ± 3.4 s, p = 0.198; normal pace: 0.62 ± 0.15 s, fast pace: 0.57 ± 0.21 s, p = 0.363; normal pace: 1.34 ± 0.22 s, fast pace: 1.17 ± 0.32 s, p = 0.158; s1 normal pace: 0.28 ± 0.09 s, s1 fast pace: 0.26 ± 0.06 s, p = 0.638; s2 normal pace: 0.23 ± 0.05 s, s2 fast pace: 0.22 ± 0.06 s, p = 0.198). For comparison purposes, Figure 6 illustrates the dispersion observed across participants for the traditional turn metrics. Both the number of steps (NbSteps normal pace: 3.9 ± 0.8, NbSteps fast pace: 3.9 ± 0.7, p = 0.685) and the mean turn velocity (turnvelmean normal pace: 1.54 ± 0.25 rad/s, turnvelmean fast pace: 1.53 ± 0.15 rad/s, p = 0.925) were not significantly affected by velocity. However, the maximum velocity was significantly different (turnvelmax normal pace: 3.83 ± 0.40 rad/s, turnvelmax fast pace: 4.08 ± 0.42 rad/s, p = 0.009).
Reliability
Reliability was assessed for all repeated trials performed by the participants (i.e., normal and fast trials). Table 2 reports the ICC for each metric together with their 95% confidence intervals. Cranio-caudal signature metrics were shown to have a moderate to good reliability with ICCs, varying from 0.64 to 0.81. Furthermore, it was found that both traditional turn velocity metrics (mean and max turn velocity) had a moderate agreement while the number of steps revealed a poor reliability.
Discussion
The current study demonstrated for the first time that it is possible to successfully capture the cranio-caudal signature from the relative angular velocity profile deduced from the AHRS orientation data. In past studies, a cranio-caudal sequence was identified using camera-based stereophotogrammetric systems (Ferrarin et al., 2006; Crenna et al., 2007; Hong et al., 2009; Wright et al., 2012; Spildooren et al., 2013; Hulbert et al., 2015). These studies predominantly assessed the temporal sequence in which the segments (head, trunk and pelvis) are engaged in turning as well as the maximum angle reached by the head relative to the trunk and pelvis. In a study comparing recurrent fallers to non-fallers performing a 360° on-spot turning task, Wright et al. (2012) showed that all participants initiated the turn by rotating the head and that the extent of that head rotation is greater in non-fallers. Additionally, in a population with Parkinson’s disease, it was also shown that both the temporal cranio-caudal sequence as well as the maximum rotation of the head to the trunk are altered compared to controls, reflecting the so-called “en-bloc” strategy (Ferrarin et al., 2006; Crenna et al., 2007; Hong et al., 2009; Spildooren et al., 2013). Hence, it has been well demonstrated that the cranio-caudal sequence exhibited during the turn contains useful information. However, it is also documented that camera-based systems have restrictions (cost, required volume of operation, occlusions) which limit their use in a clinical settings (Zhou and Hu, 2008). Alternatively, inertial measurement systems have the portability required to be used outside laboratory settings, but the type of information provided by this system is different, and thus requires data to be analyzed differently. Orientation data, expressed in a global reference frame, allow us to measure the change in orientation of the head relative to the trunk. In this study, we investigated the possibility to capture and characterize the cranio-caudal signature from the orientation data provided by AHRS using a two-step process: First, the relative head to trunk angular profile is analyzed to assess the maximum angle reached. Then, the relative angular velocity profile of the head to the trunk is derived from that relative orientation information and investigated with the sigma-lognormal model. While orientation and inertial data (acceleration and angular velocity) can be used to directly characterize the turn, the choice to use a model is based on an assumption that this model will provide insights into the NMS which will help understand mobility deficits. The model has already been proven to be linked to the NMS in different situations, but had never been used on relative angular velocity. The combined analysis of the maximum relative head to trunk angle with a sigma-lognormal approach on the velocity profile of this joint, therefore, presents a promising avenue to enable cranio-caudal signature analysis with AHRS.
In order for the approach to be truly of interest, the signature metrics have to be reliable and robust to speed variations. Comparing the metrics computed during fast TUG to the ones computed for the TUG performed at normal pace has shown that velocity does not produce significant variations in the metrics. These results are in conjunction with Akram et al. (2010) who demonstrated, using a camera-based system, that the cranio-caudal timing sequence is robust to walking speed variations. Furthermore, the metrics have shown moderate to strong reliability over the four repeated trials. At this point, it is difficult to relate the results to other published work as this is, to our knowledge, the first time a similar approach has been used to characterize the cranio-caudal sequence. For comparison purposes, traditional metrics were also captured during each trial. These metrics (number of steps, mean turn velocity and max turn velocity) correspond to the current most popular metrics used in the literature to characterize the turn behavior using inertial measurement systems (Greene et al., 2010; Salarian et al., 2010; Zampieri et al., 2010; El-Gohary et al., 2013; Galán-Mercant and Cuesta-Vargas, 2014; Sheehan et al., 2014; Mancini et al., 2015b, 2016; Zakaria et al., 2015; Smith et al., 2016; Vervoort et al., 2016). Both the number of steps and the mean turn velocity were robust to a change in speed, but the maximum turn velocity was found to be significantly higher at fast pace TUG. According to Hulbert et al. (2015), the number of steps taken during a turn relates to the strategy adopted to perform that turn. The results from the current study illustrate that the turn strategy itself was not modified with TUG speed. In the literature, turn duration was identified as an objective biomarker of the ability of the neural control system to perform postural transitions (Horak and Mancini, 2013). Therefore, the observed increased maximum turn velocity with increasing TUG pace combined with the constant mean velocity can be interpreted as an adaptive strategy to maintain the same turn duration, denoting a good ability to change motor program among the participants. However, from these results, we must be cautious when interpreting a difference in maximum velocity to differentiate populations, as the extent of the difference may also be due to speed difference. If the instruction is not standardized (e.g., “perform the test as fast but safely as possible”), results of the maximum velocity may be biased. With respect to reliability, those traditional metrics performed poorer than the signature metrics as a result. The number of steps even showed poor reliability as assessed with an ICC. Previously, Salarian et al. (2010) had reported a strong agreement for that same metrics. The difference may be explained by the small variation between individuals within our sample. Indeed, the number of steps required to perform a 180° lacks variability in the current study as participants were all healthy elderly. Salarian et al. (2010) used both healthy controls and Parkinson’s disease patients to test for reliability, increasing the variability between individuals. In the near future, a test-retest reliability of cranio-caudal signature parameters could be re-evaluated using a similar approach to enable better comparison with the literature. The lack of variability between healthy individuals is a good thing when trying to differentiate two groups with clearly different behavior (e.g., Parkinson’s disease patients versus healthy controls). However, it raises concerns regarding the sensitivity to the change of such metric. The better reliability of the cranio-caudal signature metrics observed between individuals suggests a better resolution of the metrics, offering the potential to a better sensitivity to change. If true, such metrics could be useful to monitor changes in motor control with age or disease progression within individuals. One limit to this study is the fact that the proposed cranio-caudal signature methodology was directly validated using an inertial system which is known to have a certain inaccuracy. In a recent study, it was demonstrated that the segment of interest here had a mean root-mean-squared difference between 3.1° and 4.4° during a turn with peak values around 6°(Lebel et al., 2017). However, peak error will occur around maximum velocity which, in the case of the sigma-lognormal model, is defined by Eq. 13 below. The impact of this inaccuracy on timing parameters is minor as the reported agreement is good. As a result, inaccuracy in Vmax measurement could result in inaccuracy in the estimation of parameter D. However, recalling that the effect of the pace of the trial on D was shown to be not statistically significant across individuals, it can be assumed that the model is robust to the measurement inaccuracies:
Now that we have established the required methodology to derive the cranio-caudal signature based on AHRS data and verified the reliability of the metrics, there is a possibility of applying it to different populations to verify the sensitivity of the metrics.
The proposed algorithm allows for the characterization of the quality of a turn using AHRS in an innovative manner. It also demonstrates the power of orientation data assessed with AHRS. The full potential of such an approach will only be reached when combined with automatic recognition and segmentation of activities (Nguyen et al., 2015; Ayachi et al., 2016a,b). Additionally, this work also shows that the sigma-lognormal model can be used to fit the cranio-caudal signature. Although this model has been proven well-suited for rapid (Plamondon et al., 2014) and slow movements (Duval et al., 2015) in different situations, the movement of the head to the trunk during the turn is somewhat different and it was previously unclear if such a model could be applied here. The present results confirm this hypothesis. However, further validation of the model in this specific context of use would be beneficial in order to provide a deeper understanding of the parameters values in this particular framework.
Conclusion
The present study has shown that cranio-caudal signature during the turn can be captured using AHRS and a sigma-lognormal model. Metrics deduced from the signature profile were shown to be robust to speed variations and reliable. Comparison with traditional turn metrics leads us to believe that the proposed approach is a promising avenue to enhance early deficits identification.
Ethics Statement
Participants gave their informed consent following the procedure approved by the Centre de Recherche de l’Institut Universitaire de Gériatrie de Montreal ethics board.
Author Contributions
KL developed the algorithm, designed the analysis, and drafted the manuscript. HN provided significant feedback on the analysis of the study and the manuscript. RP provided substantial feedback on the use of the Sigma-Lognormal model and its interpretation and reviewed the manuscript. CD conceived the experiment, helped in data interpretation, and reviewed the paper. PB helped in the conception of the algorithm, the interpretation of the data, and reviewed the analysis and the manuscript.
Conflict of Interest Statement
The authors declare that the research was conducted in the absence of any commercial or financial relationships that could be construed as a potential conflict of interest.
Funding
This work was conducted as part of the Ecological Mobility in Aging and Parkinson (EMAP) research group supported by a Canadian Institute of Health Research (CIHR) team in Mobility in Aging grant: Quantifying, characterizing, and modeling the whole-body mobility of individuals in their natural environment; from normal aging to Parkinson’s disease. KL was financially supported for this study by the Fonds de recherche du Québec—Santé (FRQS) and the Research Centre on Aging.
References
Akram, S. B., Frank, J. S., and Fraser, J. (2010). Effect of walking velocity on segment coordination during pre-planned turns in healthy older adults. Gait Posture 32, 211–214. doi: 10.1016/j.gaitpost.2010.04.017
Anderson, J., Wagner, J., Bessesen, M., and Williams, L. C. (2012). Usability testing in the hospital. Hum. Fac. Ergon. Manuf. Serv. Indus. 22, 52–63. doi:10.1002/hfm.20277
Ayachi, F., Nguyen, H., Goubault, E., Boissy, P., and Duval, C. (2016a). The use of empirical mode decomposition-based algorithm and inertial measurement units to auto-detect daily living activities of healthy adults. IEEE Trans. Neural Syst. Rehabil. Eng. 24, 1060–1070. doi:10.1109/TNSRE.2016.2519413
Ayachi, F. S., Nguyen, H. P., Lavigne-Pelletier, C., Goubault, E., Boissy, P., and Duval, C. (2016b). Wavelet-based algorithm for auto-detection of daily living activities of older adults captured by multiple inertial measurement units (IMUs). Physiol. Meas. 37, 442. doi:10.1088/0967-3334/37/3/442
Carbonneau, E., Fontaine, R., and Smeesters, C. (2013). “A practical approach to determine appropriate cutoff frequencies for motion analysis data,” in 37th Annual Meeting of the American Society of Biomechanics, Omaha, NE.
Carpinella, I., Crenna, P., Calabrese, E., Rabuffetti, M., Mazzoleni, P., Nemni, R., et al. (2007). Locomotor function in the early stage of Parkinson’s disease. IEEE Trans. Neural Syst. Rehabil. Eng. 15, 543–551. doi:10.1109/TNSRE.2007.908933
Crenna, P., Carpinella, I., Rabuffetti, M., Calabrese, E., Mazzoleni, P., Nemni, R., et al. (2007). SIAMOC Best Clinical Paper Award 2006: the association between impaired turning and normal straight walking in Parkinson’s disease. Gait Posture 26, 172–178. doi:10.1016/j.gaitpost.2007.04.010
Diaz, M., Fischer, A., Ferrer, M. A., and Plamondon, R. (2016). Dynamic signature verification system based on one real signature. IEEE Trans. Cybern. 1–12. doi:10.1109/TCYB.2016.2630419
Djioua, M. (2007). Contributions à la compréhension, à la généralisation et à l’utilisation de la théorie cinématique dans l’analyse et la synthèse du mouvement humain. PhD, École Polytechnique de Montréal.
Djioua, M., and Plamondon, R. (2009). A new algorithm and system for the characterization of handwriting strokes with delta-lognormal parameters. IEEE Trans. Pattern Anal. Mach. Intell. 31, 2060–2072. doi:10.1109/TPAMI.2008.264
Duval, T., Rémi, C., Plamondon, R., Vaillant, J., and O’reilly, C. (2015). Combining sigma-lognormal modeling and classical features for analyzing graphomotor performances in kindergarten children. Hum. Mov. Sci. 43, 183–200. doi:10.1016/j.humov.2015.04.005
El-Gohary, M., Pearson, S., Mcnames, J., Mancini, M., Horak, F., Mellone, S., et al. (2013). Continuous monitoring of turning in patients with movement disability. Sensors (Basel) 14, 356–369. doi:10.3390/s140100356
Ferrari, A., Cutti, A. G., Garofalo, P., Raggi, M., Heijboer, M., Cappello, A., et al. (2010). First in vivo assessment of “outwalk”: a novel protocol for clinical gait analysis based on inertial and magnetic sensors. Med. Biol. Eng. Comput. 48, 1–15. doi:10.1007/s11517-009-0544-y
Ferrarin, M., Carpinella, I., Rabuffetti, M., Calabrese, E., Mazzoleni, P., and Nemni, R. (2006). Locomotor disorders in patients at early stages of Parkinson’s disease: a quantitative analysis. Conf. Proc. IEEE Eng. Med. Biol. Soc. 1, 1224–1227. doi:10.1109/iembs.2006.260677
Fuller, J. R., Adkin, A. L., and Vallis, L. A. (2007). Strategies used by older adults to change travel direction. Gait Posture 25, 393–400. doi:10.1016/j.gaitpost.2006.05.013
Galán-Mercant, A., and Cuesta-Vargas, A. I. (2014). Differences in trunk accelerometry between frail and non-frail elderly persons in functional tasks. BMC Res. Notes 7:100. doi:10.1186/1756-0500-7-100
Gaudreault, N. D., Durand, M.-J., Bernier, M., and Côté, A.-M. (2012). Barriers and facilitators to using knee gait analysis report findings in physiotherapy practice. Novel Physiother. 2, 112. doi:10.4172/2165-7025.1000112
Ginsburg, G. (2005). Human factors engineering: a tool for medical device evaluation in hospital procurement decision-making. J. Biomed. Inform. 38, 213–219. doi:10.1016/j.jbi.2004.11.008
Greene, B., Mcgrath, D., O’neill, R., O’donovan, K., Burns, A., and Caulfield, B. (2010). An adaptive gyroscope-based algorithm for temporal gait analysis. Med. Biol. Eng. Comput. 48, 1251–1260. doi:10.1007/s11517-010-0692-0
Herman, T., Giladi, N., and Hausdorff, J. M. (2011). Properties of the ‘Timed Up and Go’ test: more than meets the eye. Gerontology 57, 203. doi:10.1159/000314963
Hong, M., Perlmutter, J. S., and Earhart, G. M. (2009). A kinematic and electromyographic analysis of turning in people with Parkinson disease. Neurorehabil. Neural Repair 23, 166–176. doi:10.1177/1545968308320639
Horak, F., King, L., and Mancini, M. (2015). Role of body-worn movement monitor technology for balance and gait rehabilitation. Phys. Ther. 95, 461–470. doi:10.2522/ptj.20140253
Horak, F. B., and Mancini, M. (2013). Objective biomarkers of balance and gait for Parkinson’s disease using body-worn sensors. Mov. Disord. 28, 1544–1551. doi:10.1002/mds.25684
Hulbert, S., Ashburn, A., Robert, L., and Verheyden, G. (2015). A narrative review of turning deficits in people with Parkinson’s disease. Disabil. Rehabil. 37, 1382–1389. doi:10.3109/09638288.2014.961661
Javier, G., Julian, F., Marcos, M.-D., and Réjean, P. (2013). 2011 International Conference on Document Analysis and Recognition Quality Analysis of Dynamic Signature Based on the Sigma-Lognormal Model. North America.
King, L. A., Mancini, M., Priest, K., Salarian, A., Rodrigues-De-Paula, F., and Horak, F. (2012). Do clinical scales of balance reflect turning abnormalities in people with Parkinson’s disease? J. Neurol. Phys. Ther. 36, 25–31. doi:10.1097/NPT.0b013e31824620d1
Koo, T. K., and Li, M. Y. (2016). A guideline of selecting and reporting intraclass correlation coefficients for reliability research. J. Chiropr. Med. 15, 155–163. doi:10.1016/j.jcm.2016.02.012
Lebel, K., Boissy, P., Hamel, M., and Duval, C. (2013). Inertial measures of motion for clinical biomechanics: comparative assessment of accuracy under controlled conditions – effect of velocity. PLoS ONE 8:e79945. doi:10.1371/journal.pone.0079945
Lebel, K., Boissy, P., Nguyen, H., and Duval, C. (2016). Autonomous quality control of joint orientation measured with inertial sensors. Sensors (Basel) 16, 1037. doi:10.3390/s16071037
Lebel, K., Boissy, P., Nguyen, H., and Duval, C. (2017). Inertial measurement systems for segments and joints kinematics assessment: towards an understanding of the variations in sensors accuracy. Biomed. Eng. Online 16, 56. doi:10.1186/s12938-017-0347-6
Mancini, M., El-Gohary, M., Pearson, S., Mcnames, J., Schlueter, H., Nutt, J. G., et al. (2015a). Continuous monitoring of turning in Parkinson’s disease: rehabilitation potential. NeuroRehabilitation 37, 3–10. doi:10.3233/NRE-151236
Mancini, M., El-Gohary, M., Pearson, S., Mcnames, J., Schlueter, H., Nutta, J. G., et al. (2015b). Continuous monitoring of turning in Parkinson’s disease: rehabilitation potential. NeuroRehabilitation 37, 3–10. doi:10.3233/NRE-151236
Mancini, M., Schlueter, H., El-Gohary, M., Mattek, N., Duncan, C., Kaye, J., et al. (2016). Continuous monitoring of turning mobility and its association to falls and cognitive function: a Pilot Study. J. Gerontol. A Biol. Sci. Med. Sci. 71, 1102–1108. doi:10.1093/gerona/glw019
Mellone, S., Mancini, M., King, L. A., Horak, F. B., and Chiari, L. (2016). The quality of turning in Parkinson’s disease: a compensatory strategy to prevent postural instability? J. Neuroeng. Rehabil. 13, 1–9. doi:10.1186/s12984-016-0147-4
Nguyen, H. P., Ayachi, F., Lavigne–Pelletier, C., Blamoutier, M., Rahimi, F., Boissy, P., et al. (2015). Auto detection and segmentation of physical activities during a Timed-Up-and-Go (TUG) task in healthy older adults using multiple inertial sensors. J. Neurol. Eng. Rehabil. 12, 36. doi:10.1186/s12984-015-0026-4
O’Reilly, C., and Plamondon, R. (2009). Development of a Sigma–Lognormal representation for on-line signatures. Pattern Recognit. 42, 3324–3337. doi:10.1016/j.patcog.2008.10.017
O’Reilly, C., and Plamondon, R. (2011). Impact of the principal stroke risk factors on human movements. Hum. Mov. Sci. 30, 792–806. doi:10.1016/j.humov.2010.07.010
O’Reilly, C., Plamondon, R., and Lebrun, L. H. (2014). Linking brain stroke risk factors to human movement features for the development of preventive tools. Front. Aging Neurosci. 6:150. doi:10.3389/fnagi.2014.00150
Palermo, E., Rossi, S., Patanè, F., and Cappa, P. (2014). Experimental evaluation of indoor magnetic distortion effects on gait analysis performed with wearable inertial sensors. Physiol. Meas. 35, 399. doi:10.1088/0967-3334/35/3/399
Patla, A. E., Adkin, A., and Ballard, T. (1999). Online steering: coordination and control of body center of mass, head and body reorientation. Exp. Brain Res. 129, 629–634. doi:10.1007/s002210050932
Plamondon, R. (1995a). A kinematic theory of rapid human movements – part I. Movement representation and generation. Biol. Cybern. 72, 295–307. doi:10.1007/BF00202785
Plamondon, R. (1995b). A kinematic theory of rapid human movements – part II. Movement time and control. Biol. Cybern. 72, 309–320. doi:10.1007/BF00202786
Plamondon, R. (1998). A kinematic theory of rapid human movements: part III. Kinetic outcomes. Biol. Cybern. 78, 133–145. doi:10.1007/s004220050420
Plamondon, R., and Alimi, A. M. (1997). Speed/accuracy trade-offs in target-directed movements. Behav. Brain Sci. 20, 279–303. doi:10.1017/S0140525X97001441
Plamondon, R., Djioua, M., and Mathieu, P. A. (2013a). Time-dependence between upper arm muscles activity during rapid movements: observation of the proportional effects predicted by the kinematic theory. Hum. Mov. Sci. 32, 1026–1039. doi:10.1016/j.humov.2012.07.006
Plamondon, R., O’reilly, C., Remi, C., and Duval, T. (2013b). The lognormal handwriter: learning, performing, and declining. Front. Psychol. 4:945. doi:10.3389/fpsyg.2013.00945
Plamondon, R., Feng, C., and Woch, A. (2003). A kinematic theory of rapid human movement. Part IV: a formal mathematical proof and new insights. Biol. Cybern. 89, 126–138. doi:10.1007/s00422-003-0407-9
Plamondon, R., O’reilly, C., Galbally, J., Almaksour, A., and Anquetil, É (2014). Recent developments in the study of rapid human movements with the kinematic theory: applications to handwriting and signature synthesis. Pattern Recogn. Lett. 35, 225–235. doi:10.1016/j.patrec.2012.06.004
Rehabilitation Institute of Chicago. (2010). Rehabilitation Measures Database: Timed Up and Go [Online]. Available at: http://www.rehabmeasures.org/Lists/RehabMeasures/DispForm.aspx?ID=903
Rémi, C., Vaillant, J., Plamondon, R., Prevost, L., and Duval, T. (2015). “Exploring the kinematic dimensions of kindergarten children’s scribbles,” in 17th Biennial Conference of the International Graphonomics Society, 79–82.
Rico-Martinez, J. M., and Gallardo-Alvarado, J. (2000). Simple method for the determination of angular velocity and acceleration of a spherical motion through quaternions. Meccanica 35, 111–118. doi:10.1023/A:1004853828657
Roetenberg, D., Baten, C. T. M., and Veltink, P. H. (2007). Estimating body segment orientation by applying inertial and magnetic sensing near ferromagnetic materials. IEEE Trans. Neural. Syst. Rehabil. Eng. 15, 469–471. doi:10.1109/TNSRE.2007.903946
Salarian, A., Horak, F. B., Zampieri, C., Carlson-Kuhta, P., Nutt, J. G., and Aminian, K. (2010). iTUG, a sensitive and reliable measure of mobility. IEEE Trans. Neural. Syst. Rehabil. Eng. 18, 303–310. doi:10.1109/TNSRE.2010.2047606
Salarian, A., Zampieri, C., Horak, F. B., Carlson-Kuhta, P., Nutt, J. G., and Aminian, K. (2009). Analyzing 180 degrees turns using an inertial system reveals early signs of progression of Parkinson’s disease. Conf. Proc. IEEE Eng. Med. Biol. Soc. 2009, 224–227. doi:10.1109/IEMBS.2009.5333970
Schiefer, C., Ellegast, R. P., Hermanns, I., Kraus, T., Ochsmann, E., Larue, C., et al. (2014). Optimization of inertial sensor-based motion capturing for magnetically distorted field applications. J. Biomech. Eng. 136, 121008–121008. doi:10.1115/1.4028822
Sheehan, K. J., Greene, B. R., Cunningham, C., Crosby, L., and Kenny, R. A. (2014). Early identification of declining balance in higher functioning older adults, an inertial sensor based method. Gait Posture 39, 1034–1039. doi:10.1016/j.gaitpost.2014.01.003
Smith, E., Walsh, L., Doyle, J., Greene, B., and Blake, C. (2016). The reliability of the quantitative timed up and go test (QTUG) measured over five consecutive days under single and dual-task conditions in community dwelling older adults. Gait Posture 43, 239–244. doi:10.1016/j.gaitpost.2015.10.004
Spain, R. I., St George, R. J., Salarian, A., Mancini, M., Wagner, J. M., Horak, F. B., et al. (2012). Body-worn motion sensors detect balance and gait deficits in people with multiple sclerosis who have normal walking speed. Gait Posture 35, 573–578. doi:10.1016/j.gaitpost.2011.11.026
Spildooren, J., Vercruysse, S., Heremans, E., Galna, B., Vandenbossche, J., Desloovere, K., et al. (2013). Head-pelvis coupling is increased during turning in patients with Parkinson’s disease and freezing of gait. Mov. Disord. 28, 619–625. doi:10.1002/mds.25285
Vervoort, D., Vuillerme, N., Kosse, N., Hortobágyi, T., and Lamoth, C. J. C. (2016). Multivariate analyses and classification of inertial sensor data to identify aging effects on the timed-up-and-go test. PLoS ONE 11:e0155984. doi:10.1371/journal.pone.0155984
Weir, J. P. (2005). Quantifying test-retest reliability using the intraclass correlation coefficient and the SEM. J. Strength Cond. Res. 19, 231–240. doi:10.1519/00124278-200502000-00038
Woch, A., and Plamondon, R. (2010). Characterization of bi-directional movement primitives and their agonist-antagonist synergy with the delta-lognormal model. Motor Control 14, 1–25. doi:10.1123/mcj.14.1.1
Woch, A., Plamondon, R., and O’reilly, C. (2011). Kinematic characteristics of bidirectional delta-lognormal primitives in young and older subjects. Hum. Mov. Sci. 30, 1–17. doi:10.1016/j.humov.2009.10.006
Wolpert, D. M., Ghahramani, Z., and Jordan, M. I. (1995). Are arm trajectories planned in kinematic or dynamic coordinates? An adaptation study. Exp. Brain Res. 103, 460–470. doi:10.1007/BF00241505
Wright, R. L., Peters, D. M., Robinson, P. D., Sitch, A. J., Watt, T. N., and Hollands, M. A. (2012). Differences in axial segment reorientation during standing turns predict multiple falls in older adults. Gait Posture 36, 541–545. doi:10.1016/j.gaitpost.2012.05.013
Yadav, N., and Bleakley, C. (2014). Accurate orientation estimation using AHRS under conditions of magnetic distortion. Sensors (Basel) 14, 20008–20024. doi:10.3390/s141120008
Zakaria, N. A., Kuwae, Y., Tamura, T., Minato, K., and Kanaya, S. (2015). Quantitative analysis of fall risk using TUG test. Comput. Methods Biomech. Biomed. Engin. 18, 426–437. doi:10.1080/10255842.2013.805211
Zampieri, C., Salarian, A., Carlson-Kuhta, P., Aminian, K., Nutt, J. G., and Horak, F. B. (2010). The instrumented timed up and go test: potential outcome measure for disease modifying therapies in Parkinson’s disease. J. Neurol. Neurosurg. Psychiatr. 81, 171–176. doi:10.1136/jnnp.2009.173740
Zhang, J. T., Novak, A. C., Brouwer, B., and Li, Q. (2013). Concurrent validation of Xsens MVN measurement of lower limb joint angular kinematics. Physiol. Meas. 34, N63–N69. doi:10.1088/0967-3334/34/8/N63
Keywords: turn, deficit, signature, inertial motion capture, IMU, attitude and heading reference system
Citation: Lebel K, Nguyen H, Duval C, Plamondon R and Boissy P (2017) Capturing the Cranio-Caudal Signature of a Turn with Inertial Measurement Systems: Methods, Parameters Robustness and Reliability. Front. Bioeng. Biotechnol. 5:51. doi: 10.3389/fbioe.2017.00051
Received: 08 May 2017; Accepted: 04 August 2017;
Published: 23 August 2017
Edited by:
Mariano Vázquez, Barcelona Supercomputing Center, SpainReviewed by:
Peter C. Fino, Oregon Health & Science University, United StatesGuanghao Sun, University of Electro-Communications, Japan
Copyright: © 2017 Lebel, Nguyen, Duval, Plamondon and Boissy. This is an open-access article distributed under the terms of the Creative Commons Attribution License (CC BY). The use, distribution or reproduction in other forums is permitted, provided the original author(s) or licensor are credited and that the original publication in this journal is cited, in accordance with accepted academic practice. No use, distribution or reproduction is permitted which does not comply with these terms.
*Correspondence: Patrick Boissy, patrick.boissy@usherbrooke.ca