- 1Jiangsu Key Laboratory of Molecular and Functional Imaging, Department of Radiology, Zhongda Hospital, Medical School, Southeast University, Nanjing, China
- 2Department of Radiology, The First Affiliated Hospital of USTC, Division of Life Sciences and Medicine, University of Science and Technology of China, Hefei, China
Purpose: To evaluate the diagnostic value of the combination of whole-tumor dynamic contrast-enhanced magnetic resonance imaging (DCE-MRI) and whole-lesion texture features based on T2–weighted images for cervical cancer with parametrial invasion.
Materials and Methods: Sixty-two patients with cervical cancer (27 with parametrial invasion and 35 without invasion) preoperatively underwent routine MRI and DCE-MRI examinations. DCE-MRI parameters (Ktrans, Kep, and Ve) and texture features (mean, skewness, kurtosis, uniformity, energy, and entropy) based on T2-weighted images were acquired by two observers. All parameters of parametrial invasion and non-invasion were analyzed by one-way analysis of variance. The diagnostic efficiency of significant variables was assessed using receiver operating characteristic analysis.
Results: The invasion group of cervical cancer demonstrated significantly higher Ktrans (0.335 ± 0.050 vs. 0.269 ± 0.079; p < 0.001), lower energy values (0.503 ± 0.093 vs. 0.602 ± 0.087; p < 0.001), and higher entropy values (1.391 ± 0.193 vs. 1.24 ± 0.129; p < 0.001) than those in the non-invasion group. Optimal diagnostic performance [area under curve [AUC], 0.925; sensitivity, 0.935; specificity, 0.829] could be obtained by the combination of Ktrans, energy, and entropy values. The AUC values of Ktrans (0.788), energy (0.761), entropy (0.749), the combination of Ktrans and energy (0.814), the combination of Ktrans and entropy (0.727), and the combination of energy and entropy (0.619) were lower than those of the combination of Ktrans, energy, and entropy values.
Conclusion: The combination of DCE-MRI and texture analysis is a promising method for diagnosis cervical cancer with parametrial infiltration. Moreover, the combination of Ktrans, energy, and entropy is more valuable than any one alone, especially in improving diagnostic sensitivity.
Introduction
Cervical cancer is one of the most common malignant diseases of the female reproductive system, and it seriously threatens women's health and life. Accurate preoperative staging of cervical cancer plays an important role in clinical treatment decisions and prognosis. As a matter of principle, surgery is performed for cervical cancer without parametrial involvement, while tumors with parametrial invasion are treated with radio-chemotherapy. To the best of our knowledge, cervical cancer with parametrial invasion is closely related to recurrence and survival after treatment (Chung et al., 2010; Munagala et al., 2010; Noh et al., 2014; Kong et al., 2016; Xia et al., 2016; Dai et al., 2018). Therefore, accurate diagnosis of cervical cancer with parametrial invasion is of great clinical significance. Parametrial invasion is usually evaluated by conventional magnetic resonance (MR) imaging and gynecological examination. Several previous investigations showed that traditional imaging features, such as full-thickness disruption of the normal cervix stroma with nodular or spiculated lesions extending to the adjacent parametrium on T2-weighted images, were considered to be parametrial invasion (Freeman et al., 2012; Patel-Lippmann et al., 2017); however, image analysis is a subjective procedure with low interobserver agreement. An objective and quantitative method for evaluating parametrial infiltration in clinical practice is needed.
Currently, many new techniques have been applied at the molecular level, such as deep learning, proteomics, and protein interaction network (Wang et al., 2019, 2020; Deng et al., 2020; Hu et al., 2020). Besides, several imaging techniques (Park et al., 2014; Zhou et al., 2016) and radiomics (Mu et al., 2015; Meng et al., 2017) have been reported in the assessment of patients with parametrial invasion to determine the stage and treatment of cervical cancer. Chiappa et al. reported that 3D ultrasound volumes can be used to more precisely define the location and degree of cervical cancer invasion (Chiappa et al., 2015). Several studies show that the apparent diffusion coefficient (ADC) value of cervical cancer is significantly lower in cancer with parametrial invasion than in cancer without parametrial invasion (Park et al., 2014; Woo et al., 2018). Park et al. reported that merging high b-value diffusion-weighted MR imaging with background body signal suppression and T2-weighted high-spatial-resolution imaging could improve diagnostic efficiency of predicting cervical cancer with parametrial infiltration (Park et al., 2014). Recently, histogram analysis of ADC has shown potential to predict outcomes after concurrent chemo-radiotherapy in patients with cervical cancer (Meng et al., 2017). Moreover, the study showed that tracer uptake heterogeneity in tumors characterized by texture features based on fluorodeoxyglucose-positron emission tomography (18-FDG PET) is highly relevant to the stage of cervical cancer (Mu et al., 2015). To our knowledge, however, no reported studies demonstrated the role of dynamic contrast-enhanced magnetic resonance imaging (DCE-MRI) or texture analysis in evaluating cervical cancer with parametrial invasion.
The purpose of our study was to investigate the diagnostic value of the combination of whole-tumor volumetric DCE-MRI and texture features based on T2-weighted images for predicting parametrial invasion. These quantitative parameters might improve the diagnostic accuracy of cervical cancer with parametrial infiltration prior to treatment.
Materials and Methods
Patients
This study was approved by our institutional review board and the patients provided written informed consent to participate. Seventy-five patients with histopathologically confirmed cervical cancer were admitted to our hospital from September 2017 to April 2019. Routine MRI sequences and DCE-MRI examinations were preoperatively performed. Thirteen patients were excluded according to the following criteria: (1) tumor diameter was <1 cm (n = 7); (2) image quality was not available for the next analysis (n = 4); (3) diameter of the necrotic lesions in the tumor was more than 5 mm (n = 2). Finally, 62 patients (25–56 years old, mean age 45 years) were eligible for the study (27 with parametrial invasion and 35 without invasion), including FIGO stage IA (n = 14), IB (n = 9), IIA (n = 12), IIB (n = 15), IIIA (n = 7), and IIIB (n = 5).
Imaging Acquisition
MRI was acquired by using a 3.0 T Signa HDxT MRI machine (GE Healthcare, USA) with an 8-channel phased array body coil. The scanning program for the pelvis was as follows: unenhanced axial T1-weighted imaging, axial and sagittal T2-weighted imaging, axial T2-weighted imaging with fat saturation, axial diffusion weighted imaging, axial DCE-MRI, and axial and sagittal contrast-enhanced T1-weighted imaging with fat saturation.
T2-weighted imaging was obtained using a fast spin echo sequence. The following protocol was used: repetition time (TR)/echo time (TE), 4600/30 ms; number of excitations, 2; section thickness, 6 mm; intersection gap, 2 mm; field of view (FOV), 240 × 240 mm; matrix size, 320 × 256; and total time, 2 min and 14 s.
DCE-MRI was obtained using T1-weighted fat-suppression images and a three dimensional (3D) liver acceleration volume acquisition (LAVA) sequence during the injection of 0.1 mmol/kg of gadodiamide (Omniscan, GE Healthcare, USA) at a rate of 2 ml/s and following a 20-mL saline flush at the same rate. The contrast medium was injected after the acquisition of three sets of pre-contrast T1 mapping using three flip angles: 5, 10, and 15 (total: 43 dynamics). The following protocol was used: TR/TE, 4.2/2.2 ms; 15° flip angle; FOV, 380 × 340 mm; matrix size, 320 × 224; section thickness, 4 mm; time resolution, 7.0 s; and total time, 4 min, 40 s.
Imaging Analysis and Parameter Acquisition
DCE-MRI data were processed by Omni-Kinetics (O.K.; GE Healthcare, China) software. Multi-flip angle T1 mapping transformed the signal intensity into contrast agent concentration. For the evaluation of the arterial input function (AIF), a region of interest (ROI) was manually placed on the iliac artery. Tumors were outlined on each slice from DCE-MRI images in order to show the volume of interest (VOI) of the whole tumor. The DCE-MRI parameters (Ktrans [the volume transfer constant], Kep [the flux rate constant], and Ve [fractional extravascular extracellular space volume]), were calculated using a modified Tofts model.
Texture features were acquired by axial T2-weighted images with ITK-SNAP software (version 3.6.0) and O.K. software. For each patient, the VOI was calculated using ITK-SNAP software by manually delineating the ROI along the edge of the tumor on each slice for the entire tumor by referencing the corresponding contrast-enhanced images. Texture features were extracted from the delineated VOI by the O.K. software.
DCE-MRI and texture features were independently measured by two radiologists using the O.K. software (XX.L. and TT.L. with 6 years and 12 years of clinical experience, respectively, in gynecologic oncology MR imaging). Reader 1 measured DCE-MRI and texture features twice in 1 week to estimate intraobserver reproducibility, and his first measurement was compared with the measurement obtained by reader 2 to assess interobserver agreement. The mean of the two measured data of reader 1 was statistically analyzed. The intraclass correlation coefficient (ICC) of more than 0.75 edindicated good agreement.
Statistical Analysis
Quantitative metrics were indicated as mean ± standard error, and the normality test was assessed using the Kolmogorov-Smirnov method. The normal distribution of the DCE-MRI and texture feature parameters data was compared between the invasion group and the non-invasion group was using one-way analysis of variance; comparisons among the metrics of each group were processed using least significant difference (LSD) test. Non-normal distribution of data were compared using the Mann–Whitney test. Receiver operating characteristic (ROC) curve analysis was used to assess the diagnostic ability of DCE-MRI and texture feature parameters in diagnosing parametrial invasion. The AUC was compared using the Delong Clarke-Pearson method (DeLong et al., 1988). Cut-off values were obtained by maximizing Youden's index (sensitivity+specificity-1). Statistical analysis was performed using SPSS 23.0 (IBM Corp., Armonk, NY) and GraphPad Prism 8.0 (GraphPad software, San Diego, CA). P < 0.05 was considered as the threshold for statistical significance.
Results
Excellent intra- and interobserver agreements were found in the measurements of DCE-MRI and texture features metrics (Table 1). The intra- and interobserver ICCs of Ktrans, Kep, and Ve were 0.917 and 0.841, 0.934 and 0.893, and 0.842 and 0.927, respectively. Meanwhile, the intra- and interobserver ICCs of the mean value were 0.924 and 0.858, of skewness were 0.925 and 0.915, of kurtosis were 0.826 and 0.935, of uniformity were 0.904 and 0.912, of energy were 0.879 and 0.892, and of entropy were 0.924 and 0.921.
Metrics derived from DCE-MRI and texture features were compared between the invasion group and the non-invasion group and are summarized in Table 1 and Figures 1, 2. The invasion group indicated a significantly higher Ktrans (0.335 ± 0.050 vs. 0.269 ± 0.079; p < 0.001), lower energy values (0.503 ± 0.093 vs. 0.602 ± 0.087; p < 0.001), and higher entropy values (1.391 ± 0.193 vs. 1.24 ± 0.129; p < 0.001) than the non-invasion group, while there was no significant difference for Kep, Ve, mean, skewness, kurtosis, or uniformity.
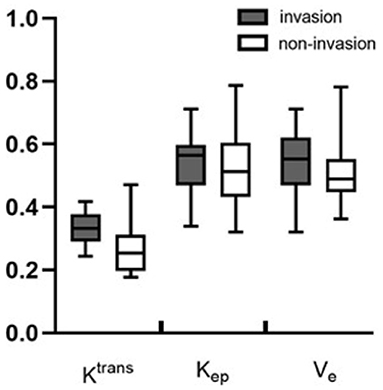
Figure 1. Boxplots of the DCE-MRI parameters of Ktrans, Kep, and Ve between the invasion and non-invasion groups.
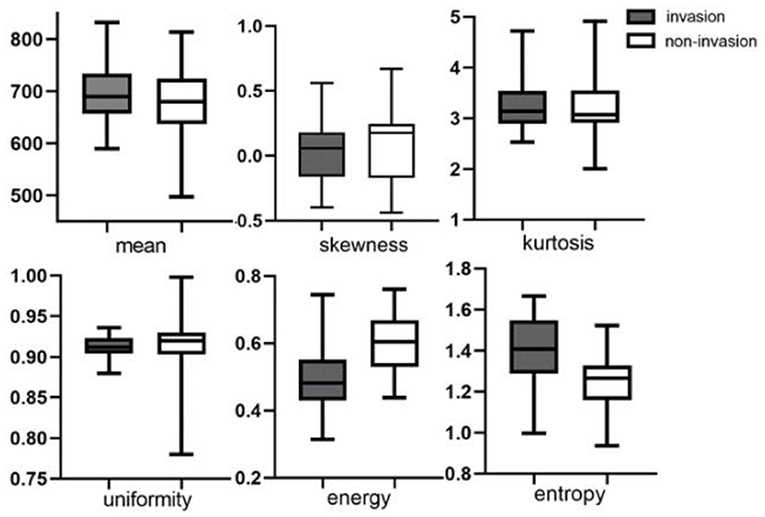
Figure 2. Boxplots of texture features of mean value, skewness, kurtosis, uniformity, energy, and entropy between invasion and non-invasion groups.
ROC analysis showed that setting the Ktrans cut-off value to ≥0.286 min−1 produced the best diagnostic performance for diagnosing parametrial infiltration (AUC, 0.788; sensitivity, 0.839; specificity, 0,657). The best diagnostic ability could be obtained by setting the threshold value of energy at ≤0.488 (AUC, 0.761; sensitivity, 0.710; specificity, 0.714). Setting the critical value of entropy at ≥1.387 obtained the best diagnostic index (AUC, 0.749; sensitivity, 0.581; specificity, 0.943). The combination of Ktrans and energy had a significantly better diagnostic index than an independent diagnosis of Ktrans, energy, and entropy (p = 0.036, p = 0.029, p = 0.047). However, using a combination of Ktrans and entropy (AUC, 0.727; sensitivity, 0.806; specificity, 0.657) and a combination of energy and entropy (AUC, 0.619; sensitivity, 0.548; specificity, 0.771) as the diagnostic marker achieved a significantly worse performance than other single and combination parameters. The combination of Ktrans, energy, and entropy (AUC, 0.925; sensitivity, 0.935; specificity, 0.829) resulted in a significantly better diagnostic performance than Ktrans, energy, entropy, a combination of Ktrans and energy, a combination of Ktrans and entropy, or a combination of Ktrans and energy (p = 0.042, p = 0.037, p = 0.018, p = 0.048, p = 0.007, p = 0.029, respectively) (Figure 3). Table 2 shows the detailed diagnostic performances. The representative images of DCE-MRI and texture features of cervical cancer with and without invasion are summarized in Figures 4, 5.
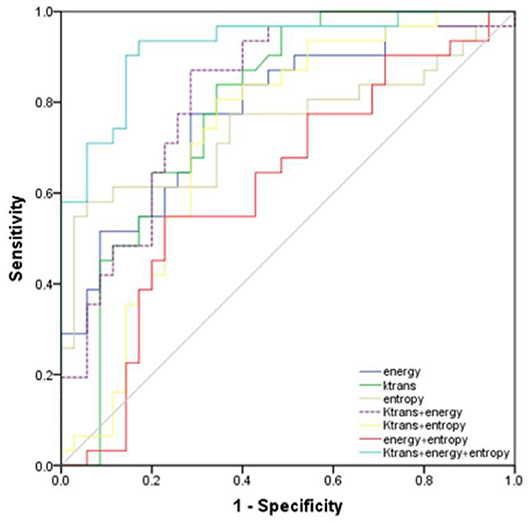
Figure 3. Receiver operating characteristic curves of the energy, Ktrans, entropy, combination of Ktrans and energy, combination of Ktrans and entropy, combination of energy and entropy, combination of Ktrans, energy, and entropy for diagnosis of invasion and non-invasion of cervical cancer.
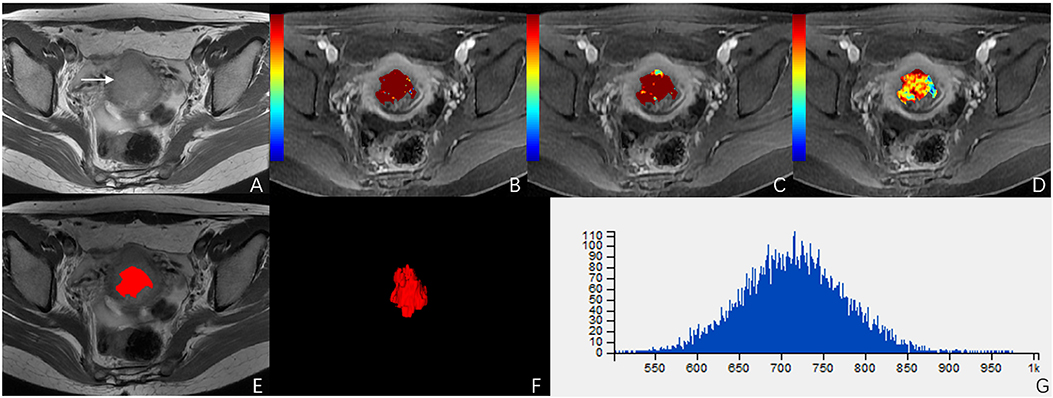
Figure 4. A 51-year-old woman with stage IIB cervical cancer. (A) T2-weighted image (T2WI) shows a slightly hyperintense cervical mass. (B–D) Ktrans, Kep, and Ve parametric maps are derived from DCE-MRI. The corresponding values are 0.577/min, 0.639/min, 0.694. (E,F) The volume of the tumor is drawn from T2WI. (G) Histogram map of the entire tumor.
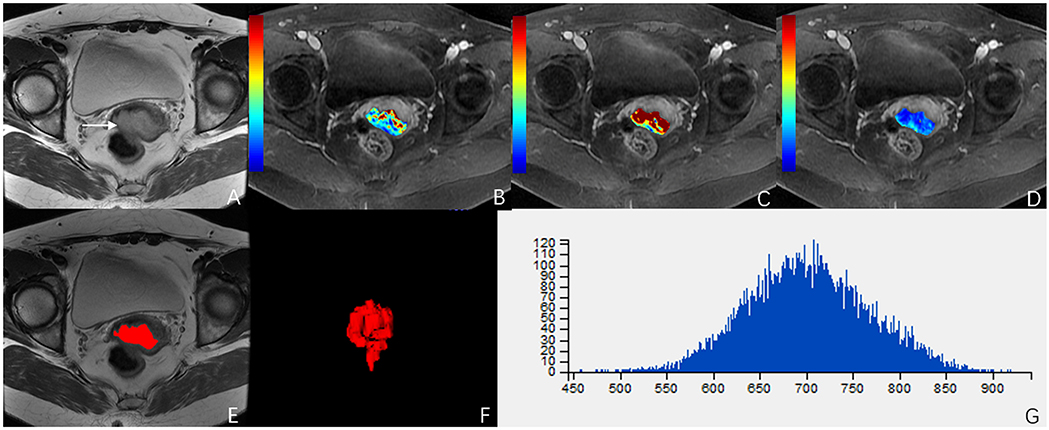
Figure 5. A 49-year-old woman with stage IIA cervical cancer. (A) T2-weighted image (T2WI) shows a slightly hyperintense cervical mass. (B–D) Ktrans, Kep, and Ve parametric maps are derived from DCE-MRI. The corresponding values are 0.196/min, 0.430/min, 0.396. (E,F) The volume of the tumor is drawn from T2WI. (G) Histogram map of the entire tumor.
Discussion
The signal intensity kinetics acquired by DCE-MRI suggest the underlying microvessel density, perfusion, permeability, and the extracellular-extravascular space composition of tumors (Zahra et al., 2007; Bonekamp et al., 2016). DCE-MRI can predict the response to and outcomes of radiotherapy in patients with cervical cancer (Tao et al., 2019). Tao et al. reported (2019) that the Ktrans of high-grade ductal carcinoma in situ of the breast is higher than that of low-grade ductal carcinoma. Li et al. (2015) reported that the Ktrans of high-grade glioma is higher than that of low-grade glioma. stoLikewise, the present study infound that the Ktrans value of the invasive group was higher than that of the non-invasive group. The parameter Ktrans reflected tumor angiogenesis, which is proportional to the density of the tumor vessels. This indicated that the angiogenesis of the invasive cervical cancer group was greater than that of the non-invasive group, and the malignant degree of the invasive group was higher than that of the non-invasive group. The more malignant the tumors are, the more angiogenesis they have. Early cervical cancer consists mainly of neovascularization, but the blood vessels are few in number and have low permeability. The growth rate of advanced cervical cancer is faster than that of early cervical cancer, and the tumor's demand for blood oxygen is increased, so that a large number of new blood vessels are formed. The angiogenesis and the permeability of the blood vessels is increased. The tortuous course of the blood vessels increases their permeable area. Moreover, the endothelial cells of blood vessels are irregular. Therefore, the contrast agent permeates through the gaps in the blood vessels more easily than it does in early cervical cancer. We can conclude that the parameters of Ktrans in the infiltration group were higher than those in the non-infiltration group.
Texture analysis is a new image post-processing computer technology that quantitatively analyzes the distribution rules and characteristics of image pixels and reflects lesion heterogeneity and the fine differences of tumors. Energy reflects uniformity and texture the coarseness of the images. The better distributed the gray of the image is, the greater its value. In this study, the energy value of the non-invasive group was larger than that of the invasive group, which indicated that the images of the invasive group were less uniform than those of the non-invasive group. This may be owing to the cystoid degeneration and necrosis in the invasive cervical cancer group. Entropy, reflecting the basic degree of chaos in the gray levels, is a measure of the image information. It is mainly used to evaluate the uniformity of image texture. The entropy value of the parametrial infiltration group was higher than that of the non-infiltration group, which indicated that the distribution of image pixels in the infiltration group was more discrete and disordered than that in the non-infiltration group. The reason for this difference may be related to the degree of malignancy. Several studies showed that tumors with a high degree of malignancy have high heterogeneity (Ng et al., 2013; Zhang et al., 2017a,b), and a high entropy value represents high tumor heterogeneity (Guan et al., 2017), and this is in accordance with our study showing that advanced cervical cancer representing high heterogeneity has high entropy. Guan et al. (2017) showed that cervical cancers with higher (IIB-IVA) rather than lower (IB-IIA) FIGO stages had lower energy and higher entropy of texture features based on ADC images. This result is consistent with our study showing that advanced cervical cancer has lower energy and higher entropy than early stage cervical cancer. The mean value, skewness, kurtosis, and homogeneity had no statistical significance in diagnosing cervical cancer with parametrial infiltration. These parameters may have significance if we performed multiple sequences of MRI for texture analysis.
In the present study, Ktrans, energy, entropy, and combinations of them had the optimal diagnostic performance for diagnosing cervical cancer with parametrial infiltration; particularly, the combination of Ktrans, energy and entropy had the highest AUC (0.925) and sensitivity (93.5%). This indicated that the combination of Ktrans, energy, and entropy was more significant than the other parameters for the diagnosis of parametrial infiltration. In other words, DCE-MRI, representing quantitative perfusion information at the molecular level, and texture features, representing a mathematical model of the gray distribution of quantitative image pixels, are the most valuable for the diagnosis of cervical cancer with parametrial infiltration. Thus, we can use more accurate quantitative parameters at the microscopic level instead of making a subjective diagnosis with a larger margin of error to evaluate parametrial infiltration. Quantitative parameters can be used as an important ancillary diagnostic tool for routine MR examination and can provide a reference for the establishment of an artificial intelligence prediction model of cervical cancer with parametrial infiltration.
Several limitations of the present study are as follows. First, the sample size of this study was relatively small. Second, the ROIs of the tumors were manually performed, which might increase the variability of the data measurement. Third, our study did not distinguish the pathological types of cervical cancer, such as squamous cell carcinoma, adenocarcinoma, and small cell carcinoma.
In conclusion, this study showed that the invasion group of cervical cancer demonstrated significantly higher Ktrans, lower energy values, and higher entropy values than those in the non-invasion group. Both DCE-MRI and texture analysis were valuable in the diagnosis. A combination of DCE-MRI and texture analysis may be a promising method to improve accuracy in diagnosing cervical cancer with parametrial infiltration prior to treatment and has great significance in the medical field.
Data Availability Statement
All datasets generated for this study are included in the article/supplementary material.
Ethics Statement
The studies involving human participants were reviewed and approved by Medical research ethics board of the First Affiliated Hospital of USTC, Division of Life Sciences and Medicine, University of Science and Technology of China. The patients/participants provided their written informed consent to participate in this study. Written informed consent was obtained from the individual(s) for the publication of any potentially identifiable images or data included in this article.
Author Contributions
WW, TL, and BL contributed conception and design of the study. XL organized the database, performed the statistical analysis, and wrote the first draft of the manuscript. WW, XL, TL, and BL wrote sections of the manuscript. All authors contributed to manuscript revision, read and approve the submitted version.
Funding
This work was supported by the National Natural Science Youth Science Fund Project (No. 81501468), by the Fundamental Research Funds for the Central Universities (No. WK9110000002).
Conflict of Interest
The authors declare that the research was conducted in the absence of any commercial or financial relationships that could be construed as a potential conflict of interest.
References
Bonekamp, D., Wolf, M. B., Edler, C., Katayama, S., Schlemmer, H. P., Herfarth, K., et al. (2016). Dynamic contrast enhanced MRI monitoring of primary proton and carbon ion irradiation of prostate cancer using a novel hypofractionated raster scan technique. Radiother. Oncol. 120, 313–319. doi: 10.1016/j.radonc.2016.05.012
Chiappa, V., Di Legge, A., Valentini, A. L., Gui, B., Micco, M., Ludovisi, M., et al. (2015). Agreement of two-dimensional and three-dimensional transvaginal ultrasound with magnetic resonance imaging in assessment of parametrial infiltration in cervical cancer. Ultrasound Obstet. Gynecol. 45, 459–469. doi: 10.1002/uog.14637
Chung, H. H., Nam, B. H., Kim, J. W., Kang, K. W., Park, N. H., Song, Y. S., et al. (2010). Preoperative [18F]FDG PET/CT maximum standardized uptake value predicts recurrence of uterine cervical cancer. Eur. J. Nucl. Med. Mol. Imaging. 37, 1467–1473. doi: 10.1007/s00259-010-1413-5
Dai, Y. F., Xu, M., Zhong, L. Y., Xie, X. Y., Liu, Z. D., Yan, M. X., et al. (2018). Prognostic significance of solitary lymph node metastasis in patients with stages IA2 to IIA cervical carcinoma. J. Int. Med. Res. 46, 4082–4091. doi: 10.1177/0300060518785827
DeLong, E. R., DeLong, D. M., and Clarke-Pearson, D. L. (1988). Comparing the areas under two or more correlated receiver operating characteristic curves: a nonparametric approach. Biometrics 44, 837–845.
Deng, A., Zhang, H., Wang, W., Zhang, J., Fan, D., Chen, P., et al. (2020). Developing Computational model to predict protein-protein interaction sites based on the XGBoost algorithm. Int. J. Mol. Sci. 21:2274. doi: 10.3390/ijms21072274
Freeman, S. J., Aly, A. M., Kataoka, M. Y., Addley, H. C., Reinhold, C., and Sala, E. (2012). The revised FIGO staging system for uterine malignancies: implications for MR imaging. Radiographics 32, 1805–1827. doi: 10.1148/rg.326125519
Guan, Y., Li, W., Jiang, Z., Zhang, B., Chen, Y., Huang, X., et al. (2017). Value of whole-lesion apparent diffusion coefficient (ADC) first-order statistics and texture features in clinical staging of cervical cancers. Clin. Radiol. 72, 951–958. doi: 10.1016/j.crad.2017.06.115
Hu, S., Chen, P., Gu, P., and Wang, B. (2020). A deep learning-based chemical system for QSAR prediction. IEEE J. Biomed. Health Inform. doi: 10.1109/JBHI.2020.2977009. [Epub ahead of print].
Kong, T.-W., Chang, S.-J., Piao, X., Paek, J., Lee, Y., Lee, E. J., et al. (2016). Patterns of recurrence and survival after abdominal versus laparoscopic/robotic radical hysterectomy in patients with early cervical cancer. J. Obstetr. Gynaecol. Res. 42, 77–86. doi: 10.1111/jog.12840
Li, X., Zhu, Y., Kang, H., Zhang, Y., Liang, H., Wang, S., et al. (2015). Glioma grading by microvascular permeability parameters derived from dynamic contrast-enhanced MRI and intratumoral susceptibility signal on susceptibility weighted imaging. Cancer Imag. 15:4. doi: 10.1186/s40644-015-0039-z
Meng, J., Zhu, L., Zhu, L., Ge, Y., He, J., Zhou, Z., et al. (2017). Histogram analysis of apparent diffusion coefficient for monitoring early response in patients with advanced cervical cancers undergoing concurrent chemo-radiotherapy. Acta Radiol. 58, 1400–1408. doi: 10.1177/0284185117694509
Mu, W., Chen, Z., Liang, Y., Shen, W., Yang, F., Dai, R., et al. (2015). Staging of cervical cancer based on tumor heterogeneity characterized by texture features on (18)F-FDG PET images. Phys. Med. Biol. 60, 5123–5139. doi: 10.1088/0031-9155/60/13/5123
Munagala, R., Rai, S. N., Ganesharajah, S., Bala, N., and Gupta, R. C. (2010). Clinicopathological, but not socio-demographic factors affect the prognosis in cervical carcinoma. Oncol. Rep. 24, 511–520. doi: 10.3892/or_00000887
Ng, F., Ganeshan, B., Kozarski, R., Miles, K. A., and Goh, V. (2013). Assessment of primary colorectal cancer heterogeneity by using whole-tumor texture analysis: contrast-enhanced CT texture as a biomarker of 5-year survival. Radiology 266, 177–184. doi: 10.1148/radiol.12120254
Noh, J. M., Park, W., Kim, Y. S., Kim, J. Y., Kim, H. J., Kim, J., et al. (2014). Comparison of clinical outcomes of adenocarcinoma and adenosquamous carcinoma in uterine cervical cancer patients receiving surgical resection followed by radiotherapy: a multicenter retrospective study (KROG 13-10). Gynecol. Oncol. 132, 618–623. doi: 10.1016/j.ygyno.2014.01.043
Park, J. J., Kim, C. K., Park, S. Y., Park, B. K., and Kim, B. (2014). Value of diffusion-weighted imaging in predicting parametrial invasion in stage IA2-IIA cervical cancer. Eur. Radiol. 24, 1081–1088. doi: 10.1007/s00330-014-3109-x
Patel-Lippmann, K., Robbins, J. B., Barroilhet, L., Anderson, B., Sadowski, E. A., and Boyum, J. (2017). MR imaging of cervical Cancer. Magn. Reson. Imaging Clin. N. Am. 25, 635–649. doi: 10.1016/j.mric.2017.03.007
Tao, W. J., Zhang, H. X., Zhang, L. M., Gao, F., Huang, W., Liu, Y., et al. (2019). Combined application of pharamcokinetic DCE-MRI and IVIM-DWI could improve detection efficiency in early diagnosis of ductal carcinoma in situ. J. Appl. Clin. Med. Phys. 20, 142–150. doi: 10.1002/acm2.12624
Wang, B., Wang, L., Zheng, C. H., and Xiong, Y. (2019). Imbalance data processing strategy for protein interaction sites prediction. IEEE/ACM Trans. Comput. Biol. Bioinform. doi: 10.1109/tcbb.2019.2953908. [Epub ahead of print].
Wang, W., Zhou, Y., Cheng, M. T., Wang, Y., Zheng, C. H., Xiong, Y., et al. (2020). Potential pathogenic genes prioritization based on protein domain interaction network analysis. IEEE/ACM Trans. Comput. Biol. Bioinform. doi: 10.1109/tcbb.2020.2983894. [Epub ahead of print].
Woo, S., Kim, S. Y., Cho, J. Y., and Kim, S. H. (2018). Apparent diffusion coefficient for prediction of parametrial invasion in cervical cancer: a critical evaluation based on stratification to a Likert scale using T2-weighted imaging. Radiol. Med. 123, 209–216. doi: 10.1007/s11547-017-0823-x
Xia, X., Xu, H., Wang, Z., Liu, R., Hu, T., and Li, S. (2016). Analysis of prognostic factors affecting the outcome of stage IB-IIB cervical cancer treated by radical hysterectomy and pelvic lymphadenectomy. Am. J. Clin. Oncol. 39, 604–608. doi: 10.1097/COC.0000000000000100
Zahra, M. A., Hollingsworth, K. G., Sala, E., Lomas, D. J., and Tan, L. T. (2007). Dynamic contrast-enhanced MRI as a predictor of tumour response to radiotherapy. Lancet Oncol. 8, 63–74. doi: 10.1016/s1470-2045(06)71012-9
Zhang, G. M., Shi, B., Sun, H., Jin, Z. Y., and Xue, H. D. (2017a). Differentiating pheochromocytoma from lipid-poor adrenocortical adenoma by CT texture analysis: feasibility study. Abdom. Radiol. (NY) 42, 2305–2313. doi: 10.1007/s00261-017-1118-3
Zhang, G. M., Sun, H., Shi, B., Jin, Z. Y., and Xue, H. D. (2017b). Quantitative CT texture analysis for evaluating histologic grade of urothelial carcinoma. Abdom. Radiol. 42, 561–568. doi: 10.1007/s00261-016-0897-2
Keywords: cervical cancer, parametrial invasion, DCE-MRI, texture analysis, T2-weighted imaging
Citation: Li X, Lin T, Liu B and Wei W (2020) Diagnosis of Cervical Cancer With Parametrial Invasion on Whole-Tumor Dynamic Contrast-Enhanced Magnetic Resonance Imaging Combined With Whole-Lesion Texture Analysis Based on T2- Weighted Images. Front. Bioeng. Biotechnol. 8:590. doi: 10.3389/fbioe.2020.00590
Received: 26 February 2020; Accepted: 14 May 2020;
Published: 11 June 2020.
Edited by:
Bing Wang, Anhui University of Technology, ChinaReviewed by:
Teng Li, Anhui University, ChinaZaiyi Liu, Guangdong Provincial People's Hospital, China
Jihong Sun, Sir Run Run Shaw Hospital, China
Copyright © 2020 Li, Lin, Liu and Wei. This is an open-access article distributed under the terms of the Creative Commons Attribution License (CC BY). The use, distribution or reproduction in other forums is permitted, provided the original author(s) and the copyright owner(s) are credited and that the original publication in this journal is cited, in accordance with accepted academic practice. No use, distribution or reproduction is permitted which does not comply with these terms.
*Correspondence: Wei Wei, weiweill@126.com