New Trends in Bioremediation Technologies Toward Environment-Friendly Society: A Mini-Review
- 1Department of Human Physiology, Vidyasagar University, Medinipur, India
- 2Department of Chemoinformatics, Infochemistry Scientific Center, Saint Petersburg National Research University of Information Technologies, Mechanics and Optics (ITMO University), Saint-Petersburg, Russia
- 3Food Technology Department, Faculty of Agriculture, Benha University, Moshtohor, Egypt
Today's environmental balance has been compromised by the unreasonable and sometimes dangerous actions committed by humans to maintain their dominance over the Earth's natural resources. As a result, oceans are contaminated by the different types of plastic trash, crude oil coming from mismanagement of transporting ships spilling it in the water, and air pollution due to increasing production of greenhouse gases, such as CO2 and CH4 etc., into the atmosphere. The lands, agricultural fields, and groundwater are also contaminated by the infamous chemicals viz., polycyclic aromatic hydrocarbons, pyrethroids pesticides, bisphenol-A, and dioxanes. Therefore, bioremediation might function as a convenient alternative to restore a clean environment. However, at present, the majority of bioremediation reports are limited to the natural capabilities of microbial enzymes. Synthetic biology with uncompromised supervision of ethical standards could help to outsmart nature's engineering, such as the CETCH cycle for improved CO2 fixation. Additionally, a blend of synthetic biology with machine learning algorithms could expand the possibilities of bioengineering. This review summarized current state-of-the-art knowledge of the data-assisted enzyme redesigning to actively promote new research on important enzymes to ameliorate the environment.
Introduction
The present growth and development of modern human societies are sustained by the stability of the Holocene climate (Revell, 2020). However, the invariability of the stable Holocene climate had been overwhelmingly abused by unrestrained consumption without genuine attention to the environment. Moreover, due to such negligence, the total wilderness of the earth has been dramatically reduced to only 35% of what it once was (Revell, 2020). Global warming (Change, 2018), polar ice meltdown (Hansen et al., 2015), reduction of biodiversity (Underwood et al., 2009; Handa et al., 2014; Delgado-Baquerizo et al., 2020), and extinctions of important wild-life species (Thomas et al., 2004) are influencers in the global climate change. Our environment is not only ruined but also destroyed by human activities. Now, we must reverse the process (Revell, 2020). In addition, according to a recent report, corals are dying as a result of high ocean-water pH (Hoegh-Guldberg et al., 2017). However, corals are crucial for underwater biodiversity (Wagner et al., 2020). Moreover, ocean pollution is increasing due to plastic articles, crude oils (Price et al., 2003), etc., which are not coral-friendly (Monteiro et al., 2018; Forrest et al., 2019). However, plastic pollution has been identified (Xanthos and Walker, 2017), and alternative materials such as bioplastics (Peelman et al., 2013; Ashter, 2016; Brodin et al., 2017) and plant-based materials are replacing non-biodegradable plastics (Ashori, 2008; Mooney, 2009; Su et al., 2018). Besides, the termination of non-biodegradable plastic production is possible by strict government restrictions (Xanthos and Walker, 2017). Conversely, we are still very dependent on hydrocarbon oil (Holdren, 2006). Thus, disasters like oil spills in the middle of oceans have become common events (Magris and Giarrizzo, 2020). For example, the artic oil spill released about 21,000 tons of diesel into rivers and subsoil from a fuel tank near Norilsk, Russian Federation (Reuters, June 9, 2020).
Furthermore, the air we breathe is also not very healthy (Koenig, 2000; Carvalho, 2016; West et al., 2016). According to a recent report, the air quality index (AQI) in some cities are in critical condition (Chelani et al., 2002; Kumar and Goyal, 2011). Besides, the emission of high amounts of greenhouse gases such as CO2 and methane has also threatened the respiratory health of humans and animals (Marrero, 2010; Li S. et al., 2018). Additionally, soil finds itself to not be an exception to this list. Soil, as well as groundwater, is contaminated by notorious chemicals such as polycyclic aromatic hydrocarbons (PAH), pyrethroids pesticides (Holmes et al., 2008; Deng et al., 2020), bisphenol-A, and dioxanes etc., (Lee and Peart, 2000; Haritash and Kaushik, 2009). Bioremediation is one way to restore our environment from devastating damage (Vidali, 2001). Besides, bioremediation is an environment-friendly approach that uses the microbial enzyme to metabolize the pollutant as a nutrient for microbes (Vidali, 2001). For example, of bioremediation by enzyme engineering, which can improve the function of the microbial enzyme (Ali et al., 2020) by means of directed evolution (Kuchner and Arnold, 1997) and rational (Cedrone et al., 2000) and semi-rational approaches (Lutz, 2010). However, engineering enzymes in the data-assisted synthetic biology landscape could accelerate the hunt of the “super-enzyme” in environmental perspectives. However, as this is a new frontier to the scientific literature body, only a handful of the kindest efforts are available at present (Ajjolli Nagaraja et al., 2020; Lawson et al., 2020; Mou et al., 2020; Robinson et al., 2020; Siedhoff et al., 2020; Wittmann et al., 2020). Herein, we have summarized current state-of-the-art knowledge of the data-assisted enzyme redesigning (Figure 1) to promote new studies on enzyme redesigning from an environmental perspective.
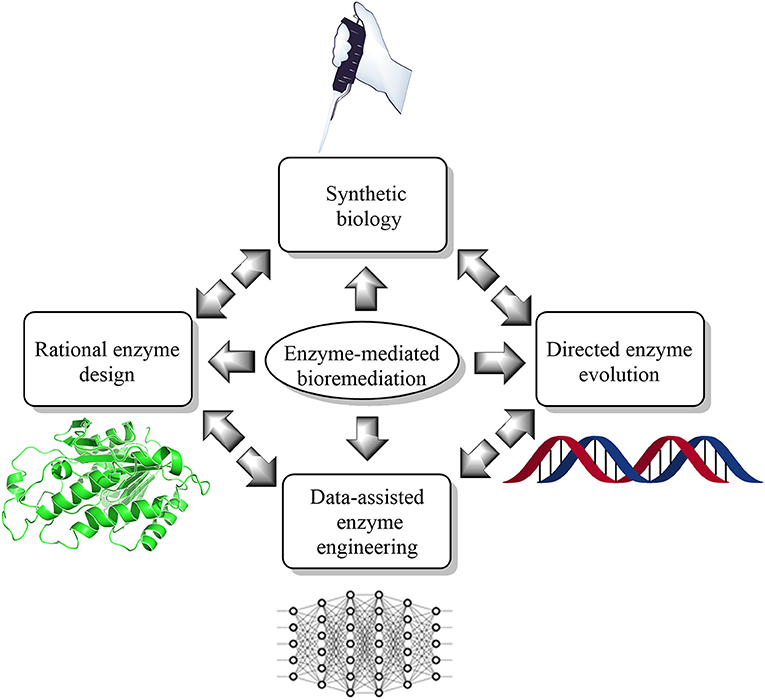
Figure 1. Current state-of-the-art trends and technologies for enzyme-mediated bioremediation, which include synthetic biology (enzyme reengineering and redesigning in vitro), rational enzyme design (molecular modeling and simulation), directed enzyme evolution (enhanced enzyme selection), and data-assisted enzyme engineering [artificial intelligence (AI) and machine learning (ML)].
Scientific Background of Enzyme Redesigning
Directed Evolution (DE) of Enzymes
Enzyme redesigning by “directed evolution” was introduced in 1997 (Kuchner and Arnold, 1997). In that same year, 40x optimization of arsenic resistance property was reported (Crameri et al., 1997). After that, many research groups reported the DE of enzymes because it is a novel approach to redesign biocatalyst (Kuchner and Arnold, 1997), (MacBeath et al., 1998). For example, directed evolution is successful in improving different enzymes, viz., Staphylococcal nuclease (100-fold) (Pedersen et al., 1998), an efficient RNA polymerase (Xia et al., 2002), a Cre recombinase (Santoro and Schultz, 2002), a new enzyme function by synthetic transformation (Turner, 2009), or enzymes with industrial values (Zhao et al., 2002; Cherry and Fidantsef, 2003; Eijsink et al., 2005; Liang et al., 2014; Porter et al., 2016). The biosynthetic pathway is also completely manipulated by enzyme redesigning (Johannes and Zhao, 2006). Besides, directed evolution methods were progressively improved with time (Arnold and Georgiou, 2003; Lutz and Patrick, 2004; Leemhuis et al., 2009). Table 1 summarizes enzyme engineering approaches and new trends, that could improve enzyme-mediated bioremediation toward an environment-friendly society.
Rational Approach of Enzyme Redesigning
Rational and semi-rational approaches of the enzyme redesigning are supervised by high-end computation power for the mutant library preparation (Cedrone et al., 2000). Conversely, in directed evolution, a random mutation library is prepared without computation power (Kuchner and Arnold, 1997). Therefore, in DE, a random mutation library preparation is more tedious and time-consuming. However, active learning, machine learning, and deep learning-assisted enzyme redesigning are state-of-the-art methods for enzyme redesigning. Since the year 2013, machine learning has become popular in studying science and engineering (Zhang et al., 2020). Furthermore, in the late 20th century, computational technologies contribute to data-assisted enzyme engineering (Cedrone et al., 2000; Chen, 2001; Lutz, 2010; Otten et al., 2010; Steiner and Schwab, 2012). For example, successful examples of the rational and combinatorial approaches of enzyme redesigning are as follows: site-directed mutagenesis with a combinatorial approach (Cedrone et al., 2000), a structure-based improvement of the non-ribosomal peptide synthetase (Chen, 2001), active site redesigning (Toscano et al., 2007), enantioselectivity-based improvement (Otten et al., 2010), site-directed saturation mutation analysis (Schneider et al., 2010), de novo substrate-based enzyme engineering (Steiner and Schwab, 2012), a combinatorial approach for improving alcohol dehydrogenase (Zhang et al., 2015), the rational-designed dual active site of a protein scaffold (Shu et al., 2016), 100x optimization of a selenoenzyme (Wang et al., 2018), heavy enzyme redesigning (Scott et al., 2019), 40x catalytic and 39x stereoselectivity enhancement of a decarboxylase (Payer et al., 2018), and widening active site tunnel by backbone redesigning (Rigoldi et al., 2020), etc.
Data-Assisted Enzyme Engineering (DAEE)
Studies on the structure–function relationship of enzymes are possible with the help of the latest biophysical tools. Moreover, the protein database (PDB) (Sussman et al., 1998) and similar databases (El-Gebali et al., 2019) offer excellent opportunities for a data scientist to analyze and optimize particular enzyme structures from a large volume of data. On the other hand, machine learning (Mazurenko et al., 2019) and deep learning are two approaches where specific algorithms are needed (LeCun et al., 2015). So far, there are limited numbers of research studies available in this new field of enzyme engineering.
Synthetic Biology and Data-Assisted Enzyme Engineering
Synthetic biology offers the possibility to redesign the chemical composition of biological molecules. It can also engineer natural DNA polymerase to catalyze a new type of genetic material called Xeno nucleic acids (XNA) (Glasscock et al., 2016). On the other hand, machine learning offers excellent advantages to handle big data. Thus, analyzing big data with machine learning provides new insights to improve the enzyme (Mazurenko et al., 2019). Besides, the synthetic biology industry or syndustry is a growing area of the bioeconomy (Bueso and Tangney, 2017), and it includes a wide range of enzyme applications. In comparison to some standard techniques of CO2 fixation including cell-free synthetic biology and ultrahigh-throughput enzyme engineering approaches using omics-based big data, the CETCH cycle was designed to be 3x faster, providing more possibilities for mutant library generation and screening (Young and Alper, 2010 Schwander et al., 2016; Quaglia et al., 2017; Badenhorst and Bornscheuer, 2018; García-Granados et al., 2019; Jiang et al., 2020).
Data-Assisted Synthetic Biology and Bioremediation
In the literature, the majority of bioremediation reports are about the natural capacity of the microbial enzymes. However, the natural enzymatic efficiency is slow compared to any redesigned or “tailor-made” enzymes (Schwander et al., 2016). Also, a microorganism has to follow a very long route to reach the final TCA cycle (Dutta et al., 2018). Yet, bioremediation with the data-assisted synthetic biology is overlooked. Conversely, bioremediation properties/pathways of a natural enzyme could be optimized by data-assisted assisted enzyme engineering.
Air Pollution, CO2 Fixation, and Ribulose-1,5-Bisphosphate Carboxylase/Oxygenase (RUBisCO)
Air pollution due to greenhouse gases is a significant problem for public health (Costello et al., 2009; Bierwirth, 2018) as well as on the atmosphere and climate change (Costello et al., 2009; Ramanathan and Feng, 2009; El Zein and Chehayeb, 2015). Ribulose-1,5-bisphosphate carboxylase-oxygenase (RuBisCO) is a primary enzyme that catalyzes CO2 fixation in photosynthetic plants (Hatch and Slack, 1970). As plants hold great potential to reduce air pollution (Chung et al., 2011), redesigning RuBisCO could be an ideal target for ecology and environmental protection. The structure of RuBisCO varies with the plant species, and some RuBisCO variants are also available in the PDB database. Yet, studies on the identification of vital amino acids are still sparse. But, Ile-165 and Met-331 mutations of RuBisCO in Rhodospirillum rubrum might alter the enzyme function. Besides, the Ala-47 mutation at the C-terminus near the active site significantly improves the carboxylation efficiency of RuBisCO.
Furthermore, some “form-I and -III” mutations (Rhodospirillum rubum) in the C-terminus have resulted in the loss of the enzyme activity (Satagopan et al., 2014). Moreover, nitrosylation is crucial for RuBisCO activation in Galdieria sulphuraria, which has been overlooked for many years (Stec, 2012). Additionally, Mg2+ and few amino acids serve a vital role in the activation and carbamoylation process of RuBisCO (Okano et al., 2002). These results are encouraging to optimize RuBisCO by data-assisted enzyme engineering.
Ocean Water Oil Spill, Bioremediation, and Methane Monooxygenase (MMO)
Modern human society depends on petroleum hydrocarbons. However, human activities on the oil spill and hydrocarbon pollution occur in many parts of the world, especially in the middle-east (Elsayed and Ammar, 2020; Nwachukwu et al., 2020; Wang D. et al., 2020). Bacterial enzymes metabolize crude oil fractions containing hydrocarbons (Stauffer et al., 2008). For example, methane monooxygenase (MMO), commonly found in methanotrophic bacteria (Singh and Singh, 2017), could be a perfect target for data-assisted enzyme engineering to improve oil-bioremediation strategy. MMOs are of two types, i.e., soluble methane monooxygenase (sMMO) and particulate methane monooxygenase (pMMO) (Lipscomb, 1994). The structural features of sMMO are previously discussed in a more detailed manner (Banerjee et al., 2019). The sMMO active site is mainly composed of E144, H147, E209, E243, and H246 residues. Similarly, Culpepper et al. have characterized the molecular structure of pMMO (Culpepper and Rosenzweig, 2012), and Rigoldi et al. have shown improved catalytic efficiency of pMMO improved by widening the diameter of the active site (Rigoldi et al., 2020). A recent study on sMMO showed an essential role of O2 transport passage to the active site termed as W308-tunnel (Jones et al., 2020). Thus, reengineering sMMO might improve the enzymatic efficiency.
Soil and Groundwater Contamination By Pahs and Aromatic Ring Hydroxylating Dioxygenase (ARHD)
The polycyclic aromatic hydrocarbon is a harmful chemical comprising 16 variants (PAHs) added to the priority list by the US Environmental Protection Agency (Andersson and Achten, 2015; Zelinkova and Wenzl, 2015; Dutta et al., 2017, 2018). These PAHs' physiochemical and toxic properties raise a significant concern over their impact on soil and groundwater contamination (Wang et al., 2009). Moreover, several reports showed evidence of PAH contamination in different soil and groundwater sources (Sushkova et al., 2018; Haleyur et al., 2019; Lu et al., 2019; Liang et al., 2020; Pacwa-Płociniczak et al., 2020; Picariello et al., 2020; Wang Y. et al., 2020; Wolf et al., 2020; Ambade et al., 2021a,b; Qiao et al., 2021). Therefore, the PAH contamination problem requires more research in this direction using chemical cleavage. PAHs are composed of two or more fused aromatic rings (Haritash and Kaushik, 2009), which can cleave by the aromatic ring hydroxylating dioxygenase, estradiol ring cleavage dioxygenase, and estradiol ring cleavage dioxygenase (Arora et al., 2009). Aromatic ring hydroxylating dioxygenase (ARHD) is a promising enzyme for this purpose, composed of an iron–sulfur flavoprotein and an iron–sulfur ferredoxin subunit (Butler and Mason, 1996). The advantage of this enzyme is that it can catalyze biodegradation of more than one PAH species and initiates the degradation of 44 different aromatic compounds (Parales and Resnick, 2006). Therefore, this enzyme might be a promising tool to implement in environmental applications (Tan and Parales, 2016), and its further reengineering using a data-assisted enzyme engineering approach could be advantageous.
Conclusion
In this condensed review, we have identified different approaches of data-assisted enzyme engineering that could be applied on RuBisCO for air pollution, methene monooxygenase for crude-oil bioremediation, and aromatic ring hydroxylating dioxygenase for bioremediation of PAHs from soil and groundwater. Future directions can be referred to a design and development of the pipelines, algorithms, and protocols, integrating aforementioned state-of-the-art technologies for enzyme-mediated bioremediation, such as synthetic biology, rational enzyme design, directed enzyme evolution, and AI/ML-assisted enzyme engineering. Overall, this review might help to potentiate more research on this direction, which is an urgent need in this present environmental crisis. However, challenges remain active to apply data-assisted synthetic biology in improving bioremediation, but with computation power and up-gradation of the coding skills, these could be overcome.
Author Contributions
SS and KD conceptualized the topic. KD performed the scientific literature search and wrote the manuscript. SS proofread the manuscript. SS and IK wrote part of the manuscript. All co-authors read the manuscript. All authors contributed to the article and approved the submitted version.
Conflict of Interest
The authors declare that the research was conducted in the absence of any commercial or financial relationships that could be construed as a potential conflict of interest.
Publisher's Note
All claims expressed in this article are solely those of the authors and do not necessarily represent those of their affiliated organizations, or those of the publisher, the editors and the reviewers. Any product that may be evaluated in this article, or claim that may be made by its manufacturer, is not guaranteed or endorsed by the publisher.
Acknowledgments
Council of Scientific and Industrial Research (CSIR), Govt. of India, New Delhi, India is also sincerely acknowledged by KD for Senior Research Fellowship (SRF), sanction letter no. 09/599(0082)/2019-EMR-I. Special thanks are also extended to Mr. Todd Axel Johnsen from the Infochemistry Scientific Centre, ITMO University for his assistance in editing and proofreading the manuscript.
References
Ajjolli Nagaraja, A., Charton, P., Cadet, X. F., Fontaine, N., Delsaut, M., Wiltschi, B., et al. (2020). A machine learning approach for efficient selection of enzyme concentrations and its application for flux optimization. Catalysts 10:291. doi: 10.3390/catal10030291
Ali, M., Ishqi, H. M., and Husain, Q. (2020). Enzyme engineering: reshaping the biocatalytic functions. Biotechnol. Bioeng. 117, 1877–1894. doi: 10.1002/bit.27329
Ambade, B., Sethi, S. S., Kumar, A., Sankar, T. K., and Kurwadkar, S. (2021a). Health risk assessment, composition, and distribution of polycyclic aromatic hydrocarbons (PAHs) in drinking water of Southern Jharkhand, East India. Arch. Environ. Contam. Toxicol. 80, 120–133. doi: 10.1007/s00244-020-00779-y
Ambade, B., Sethi, S. S., Kurwadkar, S., Kumar, A., and Sankar, T. K. (2021b). Toxicity and health risk assessment of polycyclic aromatic hydrocarbons in surface water, sediments and groundwater vulnerability in Damodar River Basin. Groundwater Sustain. Dev. 13:100553. doi: 10.1016/j.gsd.2021.100553
Amidi, S., Amidi, A., Vlachakis, D., Paragios, N., and Zacharaki, E. I. (2017). Automatic single-and multi-label enzymatic function prediction by machine learning. PeerJ 5:e3095. doi: 10.7717/peerj.3095
Andersson, J. T., and Achten, C. (2015). Time to say goodbye to the 16 EPA PAHs? Toward an up-to-date use of PACs for environmental purposes. Polycycl. Aromat. Comp. 35, 330–354. doi: 10.1080/10406638.2014.991042
Arnold, F. H., and Georgiou, G. (2003). Directed Enzyme Evolution: Screening and Selection Methods. New York, NY: Springer Science and Business Media. doi: 10.1385/1592593968
Arora, P. K., Kumar, M., Chauhan, A., Raghava, G. P., and Jain, R. K. (2009). OxDBase: a database of oxygenases involved in biodegradation. BMC Res. Notes 2, 1–8. doi: 10.1186/1756-0500-2-67
Ashori, A. (2008). Wood–plastic composites as promising green-composites for automotive industries! Bioresour. Technol. 99, 4661–4667. doi: 10.1016/j.biortech.2007.09.043
Ashter, S. A. (2016). Introduction to Bioplastics Engineering. Norwich, NY: William Andrew. doi: 10.1016/B978-0-323-39396-6.00001-4
Badenhorst, C. P., and Bornscheuer, U. T. (2018). Getting momentum: from biocatalysis to advanced synthetic biology. Trends Biochem. Sci. 43, 180–198. doi: 10.1016/j.tibs.2018.01.003
Baek, C., Lee, S.-W., Lee, B.-J., Kwak, D.-H., and Zhang, B.-T. (2019). Enzymatic weight update algorithm for DNA-based molecular learning. Molecules 24:1409. doi: 10.3390/molecules24071409
Banerjee, R., Jones, J. C., and Lipscomb, J. D. (2019). Soluble methane monooxygenase. Annu. Rev. Biochem. 88, 409–431. doi: 10.1146/annurev-biochem-013118-111529
Bierwirth, P. (2018). Carbon Dioxide Toxicity and Climate Change: A Major Unapprehended Risk for Human Health. Web Published: ResearchGate. doi: 10.13140/RG.2.2.16787.48168. Available online at: http://grapevine.com.au/~pbierwirth/co2toxicity.pdf
Bonk, B. M., Weis, J. W., and Tidor, B. (2019). Machine learning identifies chemical characteristics that promote enzyme catalysis. J. Am. Chem. Soc. 141, 4108–4118. doi: 10.1021/jacs.8b13879
Brodin, M., Vallejos, M., Opedal, M. T., Area, M. C., and Chinga-Carrasco, G. (2017). Lignocellulosics as sustainable resources for production of bioplastics–a review. J. Clean. Prod. 162, 646–664. doi: 10.1016/j.jclepro.2017.05.209
Bueso, Y. F., and Tangney, M. (2017). Synthetic biology in the driving seat of the bioeconomy. Trends Biotechnol. 35, 373–378. doi: 10.1016/j.tibtech.2017.02.002
Butler, C. S., and Mason, J. R. (1996). Structure-function analysis of the bacterial aromatic ring-hydroxylating dioxygenases. Adv. Microb. Physiol. 38, 47–84. doi: 10.1016/S0065-2911(08)60155-1
Cadet, F., Fontaine, N., Li, G., Sanchis, J., Chong, M. N. F., Pandjaitan, R., et al. (2018). A machine learning approach for reliable prediction of amino acid interactions and its application in the directed evolution of enantioselective enzymes. Sci. Rep. 8, 1–15. doi: 10.1038/s41598-018-35033-y
Cai, Y., Yang, H., Li, W., Liu, G., Lee, P. W., and Tang, Y. (2018). Multiclassification prediction of enzymatic reactions for oxidoreductases and hydrolases using reaction fingerprints and machine learning methods. J. Chem. Inf. Model. 58, 1169–1181. doi: 10.1021/acs.jcim.7b00656
Carbonell, P., Wong, J., Swainston, N., Takano, E., Turner, N. J., Scrutton, N. S., et al. (2018). Selenzyme: enzyme selection tool for pathway design. Bioinformatics 34, 2153–2154. doi: 10.1093/bioinformatics/bty065
Carvalho, H. (2016). The air we breathe: differentials in global air quality monitoring. Lancet Resp. Med. 4, 603–605. doi: 10.1016/S2213-2600(16)30180-1
Cedrone, F., Ménez, A., and Quéméneur, E. (2000). Tailoring new enzyme functions by rational redesign. Curr. Opin. Struct. Biol. 10, 405–410. doi: 10.1016/S0959-440X(00)00106-8
Change, P. C. (2018). Global Warming of 1.5° C. Geneva, Switzerland: World Meteorological Organization.
Chelani, A., Rao, C. C., Phadke, K., and Hasan, M. (2002). Formation of an air quality index in India. Int. J. Environ. Stud. 59, 331–342. doi: 10.1080/00207230211300
Chen, R. (2001). Enzyme engineering: rational redesign versus directed evolution. Trends Biotechnol. 19, 13–14. doi: 10.1016/S0167-7799(00)01522-5
Cherry, J. R., and Fidantsef, A. L. (2003). Directed evolution of industrial enzymes: an update. Curr. Opin. Biotechnol. 14, 438–443. doi: 10.1016/S0958-1669(03)00099-5
Chung, C.-Y., Chung, P.-L., and Liao, S.-W. (2011). Carbon fixation efficiency of plants influenced by sulfur dioxide. Environ. Monit. Assess. 173, 701–707. doi: 10.1007/s10661-010-1416-5
Costello, A., Abbas, M., Allen, A., Ball, S., Bell, S., Bellamy, R., et al. (2009). Managing the health effects of climate change: lancet and University College London Institute for Global Health Commission. Lancet 373, 1693–1733. doi: 10.1016/S0140-6736(09)60935-1
Crameri, A., Dawes, G., Rodriguez Jr, E., Silver, S., and Stemmer, W. P. (1997). Molecular evolution of an arsenate detoxification pathway by DNA shuffling. Nat. Biotechnol. 15, 436–438. doi: 10.1038/nbt0597-436
Culpepper, M. A., and Rosenzweig, A. C. (2012). Architecture and active site of particulate methane monooxygenase. Crit. Rev. Biochem. Mol. Biol. 47, 483–492. doi: 10.3109/10409238.2012.697865
Dale, J. M., Popescu, L., and Karp, P. D. (2010). Machine learning methods for metabolic pathway prediction. BMC Bioinformatics 11, 1–14. doi: 10.1186/1471-2105-11-15
De Ferrari, L., and Mitchell, J. B. (2014). From sequence to enzyme mechanism using multi-label machine learning. BMC Bioinformatics 15, 1–13. doi: 10.1186/1471-2105-15-150
de Oliveira Almeida, R., and Valente, G. T. (2020). Predicting metabolic pathways of plant enzymes without using sequence similarity: models from machine learning. Plant Genome 13:e20043. doi: 10.1002/tpg2.20043
Delgado-Baquerizo, M., Reich, P. B., Trivedi, C., Eldridge, D. J., Abades, S., Alfaro, F. D., et al. (2020). Multiple elements of soil biodiversity drive ecosystem functions across biomes. Nat. Ecol. Evol. 4, 210–220. doi: 10.1038/s41559-019-1084-y
Deng, F., Sun, J., Dou, R., Yu, X., Wei, Z., Yang, C., et al. (2020). Contamination of pyrethroids in agricultural soils from the Yangtze River Delta, China. Sci. Tot. Environ. 731:139181. doi: 10.1016/j.scitotenv.2020.139181
Dutta, K., Shityakov, S., Das, P. P., and Ghosh, C. (2017). Enhanced biodegradation of mixed PAHs by mutated naphthalene 1, 2-dioxygenase encoded by Pseudomonas putida strain KD6 isolated from petroleum refinery waste. 3 Biotech 7, 1–11. doi: 10.1007/s13205-017-0940-1
Dutta, K., Shityakov, S., Khalifa, I., Mal, A., Moulik, S. P., Panda, A. K., et al. (2018). Effects of secondary carbon supplement on biofilm-mediated biodegradation of naphthalene by mutated naphthalene 1, 2-dioxygenase encoded by Pseudomonas putida strain KD9. J. Hazard. Mater. 357, 187–197. doi: 10.1016/j.jhazmat.2018.05.024
Eijsink, V. G., Gåseidnes, S., Borchert, T. V., and Van Den Burg, B. (2005). Directed evolution of enzyme stability. Biomol. Eng. 22, 21–30. doi: 10.1016/j.bioeng.2004.12.003
El Zein, A. L., and Chehayeb, N. A. (2015). The effect of greenhouse gases on earth's temperature. Int. J. Environ. Monit. Anal. 3:74. doi: 10.11648/j.ijema.20150302.16
El-Gebali, S., Mistry, J., Bateman, A., Eddy, S. R., Luciani, A., Potter, S. C., et al. (2019). The Pfam protein families database in 2019. Nucleic Acids Res. 47, D427–D432. doi: 10.1093/nar/gky995
Elsayed, N., and Ammar, S. (2020). Sustainability governance and legitimization processes: gulf of Mexico oil spill. Sustain. Account. Manage. Pol. J. 11, 253–278. doi: 10.1108/SAMPJ-09-2018-0242
Forrest, A., Giacovazzi, L., Dunlop, S., Reisser, J., Tickler, D., Jamieson, A., et al. (2019). Eliminating plastic pollution: how a voluntary contribution from industry will drive the circular plastics economy. Front. Marine Sci. 6:627. doi: 10.3389/fmars.2019.00627
Fox, R. J., and Huisman, G. W. (2008). Enzyme optimization: moving from blind evolution to statistical exploration of sequence–function space. Trends Biotechnol. 26, 132–138. doi: 10.1016/j.tibtech.2007.12.001
Gado, J. E., Beckham, G. T., and Payne, C. M. (2020). Improving enzyme optimum temperature prediction with resampling strategies and ensemble learning. J Chem Inf Model. 60, 4098–4107. doi: 10.1101/2020.05.06.081737
García-Granados, R., Lerma-Escalera, J. A., and Morones-Ramírez, J. R. (2019). Metabolic engineering and synthetic biology: synergies, future, and challenges. Front. Bioeng. Biotechnol. 7:36. doi: 10.3389/fbioe.2019.00036
Glasscock, C. J., Lucks, J. B., and DeLisa, M. P. (2016). Engineered protein machines: emergent tools for synthetic biology. Cell Chem. Biol. 23, 45–56.
Haleyur, N., Shahsavari, E., Jain, S. S., Koshlaf, E., Ravindran, V. B., Morrison, P. D., et al. (2019). Influence of bioaugmentation and biostimulation on PAH degradation in aged contaminated soils: response and dynamics of the bacterial community. J. Environ. Manage. 238, 49–58. doi: 10.1016/j.jenvman.2019.02.115
Handa, I. T., Aerts, R., Berendse, F., Berg, M. P., Bruder, A., Butenschoen, O., et al. (2014). Consequences of biodiversity loss for litter decomposition across biomes. Nature 509, 218–221. doi: 10.1038/nature13247
Hansen, J., Sato, M., Hearty, P., Ruedy, R., Kelley, M., Masson-Delmotte, V., et al. (2015). Ice melt, sea level rise and superstorms: evidence from paleoclimate data, climate modeling, and modern observations that 2° C global warming is highly dangerous. Atmospheric Chem. Phys. Discus. 15, 3761–3812. doi: 10.5194/acpd-15-20059-2015
Haritash, A., and Kaushik, C. (2009). Biodegradation aspects of polycyclic aromatic hydrocarbons (PAHs): a review. J. Hazard. Mater. 169, 1–15. doi: 10.1016/j.jhazmat.2009.03.137
Hatch, M. D., and Slack, C. R. (1970). Photosynthetic CO2-fixation pathways. Annu. Rev. Plant Physiol. 21, 141–162. doi: 10.1146/annurev.pp.21.060170.001041
Heckmann, D., Lloyd, C. J., Mih, N., Ha, Y., Zielinski, D. C., Haiman, Z. B., et al. (2018). Machine learning applied to enzyme turnover numbers reveals protein structural correlates and improves metabolic models. Nat. Commun. 9, 1–10. doi: 10.1038/s41467-018-07652-6
Hoegh-Guldberg, O., Poloczanska, E. S., Skirving, W., and Dove, S. (2017). Coral reef ecosystems under climate change and ocean acidification. Front. Marine Sci. 4:158. doi: 10.3389/fmars.2017.00158
Holdren, J. P. (2006). The energy innovation imperative: addressing oil dependence, climate change, and other 21st century energy challenges. Innovations 1, 3–23. doi: 10.1162/itgg.2006.1.2.3
Holmes, R. W., Anderson, B. S., Phillips, B. M., Hunt, J. W., Crane, D. B., Mekebri, A., et al. (2008). Statewide investigation of the role of pyrethroid pesticides in sediment toxicity in California's urban waterways. Environ. Sci. Technol. 42, 7003–7009. doi: 10.1021/es801346g
Jiang, N., Ma, L., and Lu, Y. (2020). Cell-free synthetic biology in the new era of enzyme engineering. Chin. J. Chem. Eng. 28, 2810–2816. doi: 10.1016/j.cjche.2020.05.037
Johannes, T. W., and Zhao, H. (2006). Directed evolution of enzymes and biosynthetic pathways. Curr. Opin. Microbiol. 9, 261–267. doi: 10.1016/j.mib.2006.03.003
Jones, J. C., Banerjee, R., Shi, K., Aihara, H., and Lipscomb, J. D. (2020). Structural studies of the methylosinus trichosporium OB3b soluble methane monooxygenase hydroxylase and regulatory component complex reveal a transient substrate tunnel. Biochemistry 59, 2946–2961. doi: 10.1021/acs.biochem.0c00459
Koenig, J. Q. (2000). Health Effects of Ambient Air Pollution: How Safe is the Air We Breathe? Norwell, MA: Springer Science and Business Media. doi: 10.1007/978-1-4615-4569-9
Kuchner, O., and Arnold, F. H. (1997). Directed evolution of enzyme catalysts. Trends Biotechnol. 15, 523–530. doi: 10.1016/S0167-7799(97)01138-4
Kumar, A., and Goyal, P. (2011). Forecasting of daily air quality index in Delhi. Sci. Tot. Environ. 409, 5517–5523. doi: 10.1016/j.scitotenv.2011.08.069
Lawson, C., Mart,í, J. M., Radivojevic, T., Jonnalagadda, S. V. R., Gentz, R., Hillson, N. J., et al. (2020). Machine learning for metabolic engineering: a review. Metab. Eng. 63, 34–60. doi: 10.1016/j.ymben.2020.10.005
LeCun, Y., Bengio, Y., and Hinton, G. (2015). Deep learning. Nature 521, 436–444. doi: 10.1038/nature14539
Lee, H.-B., and Peart, T. E. (2000). Bisphenol A contamination in Canadian municipal and industrial wastewater and sludge samples. Water Qual. Res. J. 35, 283–298. doi: 10.2166/wqrj.2000.018
Leemhuis, H., Kelly, R. M., and Dijkhuizen, L. (2009). Directed evolution of enzymes: library screening strategies. IUBMB Life 61, 222–228. doi: 10.1002/iub.165
Li, G., Rabe, K. S., Nielsen, J., and Engqvist, M. K. (2019). Machine learning applied to predicting microorganism growth temperatures and enzyme catalytic optima. ACS Synth. Biol. 8, 1411–1420. doi: 10.1021/acssynbio.9b00099
Li, S., Bush, R. T., Santos, I. R., Zhang, Q., Song, K., Mao, R., et al. (2018). Large greenhouse gases emissions from China's lakes and reservoirs. Water Res. 147, 13–24. doi: 10.1016/j.watres.2018.09.053
Li, Y., Wang, S., Umarov, R., Xie, B., Fan, M., Li, L., et al. (2018). DEEPre: sequence-based enzyme EC number prediction by deep learning. Bioinformatics 34, 760–769. doi: 10.1093/bioinformatics/btx680
Li, Y. H., Xu, J. Y., Tao, L., Li, X. F., Li, S., Zeng, X., et al. (2016). SVM-Prot 2016: a web-server for machine learning prediction of protein functional families from sequence irrespective of similarity. PLoS ONE 11:e0155290. doi: 10.1371/journal.pone.0155290
Liang, M., Liang, H., Rao, Z., and Xu, D. (2020). Occurrence of polycyclic aromatic hydrocarbons in groundwater from rural areas in eastern China: spatial distribution, source apportionment and health cancer risk assessment. Chemosphere 259:127534. doi: 10.1016/j.chemosphere.2020.127534
Liang, Y., Ang, E. L., and Zhao, H. (2014). “Directed evolution of enzymes for industrial biocatalysis,” in Industrial Biocatalysis, ed P. Grunwald (Singapore: Pan Stanford Publishing Pte. Ltd.), 73–110.
Liao, J., Warmuth, M. K., Govindarajan, S., Ness, J. E., Wang, R. P., Gustafsson, C., et al. (2007). Engineering proteinase K using machine learning and synthetic genes. BMC Biotechnol. 7:16. doi: 10.1186/1472-6750-7-16
Lipscomb, J. D. (1994). Biochemistry of the soluble methane monooxygenase. Annu. Rev. Microbiol. 48, 371–399. doi: 10.1146/annurev.mi.48.100194.002103
Lu, C., Hong, Y., Liu, J., Gao, Y., Ma, Z., Yang, B., et al. (2019). A PAH-degrading bacterial community enriched with contaminated agricultural soil and its utility for microbial bioremediation. Environ. Pollut. 251, 773–782. doi: 10.1016/j.envpol.2019.05.044
Lutz, S. (2010). Beyond directed evolution—semi-rational protein engineering and design. Curr. Opin. Biotechnol. 21, 734–743. doi: 10.1016/j.copbio.2010.08.011
Lutz, S., and Patrick, W. M. (2004). Novel methods for directed evolution of enzymes: quality, not quantity. Curr. Opin. Biotechnol. 15, 291–297. doi: 10.1016/j.copbio.2004.05.004
MacBeath, G., Kast, P., and Hilvert, D. (1998). Redesigning enzyme topology by directed evolution. Science 279, 1958–1961. doi: 10.1126/science.279.5358.1958
Magris, R. A., and Giarrizzo, T. (2020). Mysterious oil spill in the Atlantic Ocean threatens marine biodiversity and local people in Brazil. Mar. Pollut. Bull. 153:110961. doi: 10.1016/j.marpolbul.2020.110961
Marrero, G. A. (2010). Greenhouse gases emissions, growth and the energy mix in Europe. Energy Econ. 32, 1356–1363. doi: 10.1016/j.eneco.2010.09.007
Mazurenko, S., Prokop, Z., and Damborsky, J. (2019). Machine learning in enzyme engineering. ACS Catal. 10, 1210–1223. doi: 10.1021/acscatal.9b04321
Mellor, J., Grigoras, I., Carbonell, P., and Faulon, J.-L. (2016). Semisupervised Gaussian process for automated enzyme search. ACS Synth. Biol. 5, 518–528. doi: 10.1021/acssynbio.5b00294
Monteiro, R. C., do Sul, J. A. I., and Costa, M. F. (2018). Plastic pollution in islands of the Atlantic Ocean. Environ. Pollut. 238, 103–110. doi: 10.1016/j.envpol.2018.01.096
Mooney, B. P. (2009). The second green revolution? Production of plant-based biodegradable plastics. Biochem. J. 418, 219–232. doi: 10.1042/BJ20081769
Mou, Z., Eakes, J., Cooper, C. J., Foster, C. M., Standaert, R. F., Podar, M., et al. (2020). Machine learning-based prediction of enzyme substrate scope: application to bacterial nitrilases. Proteins. 89, 336–347. doi: 10.22541/au.158888180.03951231
Ng, W. (2020). Machine learning models for predicting enzymatic activity are specific only for the class of enzyme. Wenfa Ng.
Nwachukwu, A., Okoro, B., Osuagwu, J., Nwakwasi, N., and Onyechere, I. (2020). Index and compaction properties of oil contaminated clay soils in Niger-Delta Region of Nigeria. doi: 10.36348/sjet.2020.v05i02.007
Okano, Y., Mizohata, E., Xie, Y., Matsumura, H., Sugawara, H., Inoue, T., et al. (2002). X-ray structure of Galdieria Rubisco complexed with one sulfate ion per active site. FEBS Lett. 527, 33–36. doi: 10.1016/S0014-5793(02)03148-4
Otten, L. G., Hollmann, F., and Arends, I. W. (2010). Enzyme engineering for enantioselectivity: from trial-and-error to rational design? Trends Biotechnol. 28, 46–54. doi: 10.1016/j.tibtech.2009.10.001
Pacwa-Płociniczak, M., Biniecka, P., Bondarczuk, K., and Piotrowska-Seget, Z. (2020). Metagenomic functional profiling reveals differences in bacterial composition and function during bioaugmentation of aged petroleum-contaminated soil. Front. Microbiol. 11:2106. doi: 10.3389/fmicb.2020.02106
Parales, R. E., and Resnick, S. M. (2006). “Aromatic ring hydroxylating dioxygenases,” in Pseudomonas, eds J. L. Ramos and R. C. Levesque (Boston, MA: Springer), 287–340. doi: 10.1007/0-387-28881-3_9
Payer, S. E., Pollak, H., Glueck, S. M., and Faber, K. (2018). A rational active-site redesign converts a decarboxylase into a C-C hydratase: “Tethered Acetate” supports enantioselective hydration of 4-hydroxystyrenes. ACS Catal. 8, 2438–2442. doi: 10.1021/acscatal.7b04293
Pedersen, H., Hölder, S., Sutherlin, D. P., Schwitter, U., King, D. S., and Schultz, P. G. (1998). A method for directed evolution and functional cloning of enzymes. Proc. Natl. Acad. Sci. U. S. A. 95, 10523–10528. doi: 10.1073/pnas.95.18.10523
Peelman, N., Ragaert, P., De Meulenaer, B., Adons, D., Peeters, R., Cardon, L., et al. (2013). Application of bioplastics for food packaging. Trends Food Sci. Technol. 32, 128–141. doi: 10.1016/j.tifs.2013.06.003
Pertusi, D. A., Moura, M. E., Jeffryes, J. G., Prabhu, S., Biggs, B. W., and Tyo, K. E. (2017). Predicting novel substrates for enzymes with minimal experimental effort with active learning. Metab. Eng. 44, 171–181. doi: 10.1016/j.ymben.2017.09.016
Picariello, E., Baldantoni, D., and De Nicola, F. (2020). Acute effects of PAH contamination on microbial community of different forest soils. Environ. Pollut. 262:114378. doi: 10.1016/j.envpol.2020.114378
Porter, J. L., Rusli, R. A., and Ollis, D. L. (2016). Directed evolution of enzymes for industrial biocatalysis. Chembiochem 17, 197–203. doi: 10.1002/cbic.201500280
Price, J. M., Johnson, W. R., Marshall, C. F., Ji, Z.-G., and Rainey, G. B. (2003). Overview of the oil spill risk analysis (OSRA) model for environmental impact assessment. Spill Sci. Technol. Bull. 8, 529–533. doi: 10.1016/S1353-2561(03)00003-3
Qiao, X., Zheng, B., Li, X., Zhao, X., Dionysiou, D. D., and Liu, Y. (2021). Influencing factors and health risk assessment of polycyclic aromatic hydrocarbons in groundwater in China. J. Hazard. Mater. 402:123419. doi: 10.1016/j.jhazmat.2020.123419
Quaglia, D., Ebert, M. C., Mugford, P. F., and Pelletier, J. N. (2017). Enzyme engineering: a synthetic biology approach for more effective library generation and automated high-throughput screening. PLoS ONE 12:e0171741. doi: 10.1371/journal.pone.0171741
Ramanathan, V., and Feng, Y. (2009). Air pollution, greenhouse gases and climate change: global and regional perspectives. Atmos. Environ. 43, 37–50. doi: 10.1016/j.atmosenv.2008.09.063
Rigoldi, F., Donini, S., Torretta, A., Carbone, A., Redaelli, A., Bandiera, T., et al. (2020). Rational backbone redesign of a fructosyl peptide oxidase to widen its active site access tunnel. Biotechnol. Bioeng. 117, 3688–3698. doi: 10.1002/bit.27535
Robinson, S. L., Smith, M. D., Richman, J. E., Aukema, K. G., and Wackett, L. P. (2020). Machine learning-based prediction of activity and substrate specificity for OleA enzymes in the thiolase superfamily. Synth. Biol. 5:ysaa004. doi: 10.1093/synbio/ysaa004
Ryu, J. Y., Kim, H. U., and Lee, S. Y. (2019). Deep learning enables high-quality and high-throughput prediction of enzyme commission numbers. Proc. Natl. Acad. Sci. U. S. A. 116, 13996–14001. doi: 10.1073/pnas.1821905116
S Heck, G. O, Pintro, V. R, Pereira, R. M. B, Levin, N. F, and de Azevedo, W (2017). Supervised machine learning methods applied to predict ligand-binding affinity. Curr. Med. Chem. 24, 2459–2470. doi: 10.2174/0929867324666170623092503
Santoro, S. W., and Schultz, P. G. (2002). Directed evolution of the site specificity of Cre recombinase. Proc. Natl. Acad. Sci. U. S. A. 99, 4185–4190. doi: 10.1073/pnas.022039799
Satagopan, S., Chan, S., Perry, L. J., and Tabita, F. R. (2014). Structure-function studies with the unique hexameric form II ribulose-1, 5-bisphosphate carboxylase/oxygenase (Rubisco) from Rhodopseudomonas palustris. J. Biol. Chem. 289, 21433–21450. doi: 10.1074/jbc.M114.578625
Schneider, S., Gutiérrez, M., Sandalova, T., Schneider, G., Clapés, P., Sprenger, G. A., et al. (2010). Redesigning the active site of transaldolase TalB from Escherichia coli: new variants with improved affinity towards nonphosphorylated substrates. Chembiochem 11, 681–690. doi: 10.1002/cbic.200900720
Schwander, T., von Borzyskowski, L. S., Burgener, S., Cortina, N. S., and Erb, T. J. (2016). A synthetic pathway for the fixation of carbon dioxide in vitro. Science 354, 900–904. doi: 10.1126/science.aah5237
Scott, A. F., Luk, L. Y. P., Tuñón, I., Moliner, V., and Allemann, R. K. (2019). Heavy enzymes and the rational redesign of protein catalysts. Chembiochem 20:2807. doi: 10.1002/cbic.201900134
Shu, X. G., Su, J. H., Du, K. J., You, Y., Gao, S. Q., Wen, G. B., et al. (2016). Rational design of dual active sites in a single protein scaffold: a case study of heme protein in myoglobin. ChemistryOpen 5:192. doi: 10.1002/open.201500224
Siedhoff, N. E., Schwaneberg, U., and Davari, M. D. (2020). Machine learning-assisted enzyme engineering. Meth. Enzymol. 643, 281–315. doi: 10.1016/bs.mie.2020.05.005
Singh, J. S., and Singh, D. (2017). “Methanotrophs: an emerging bioremediation tool with unique broad spectrum methane monooxygenase (MMO) enzyme,” in Agro-Environmental Sustainability, eds J. Singh and G. Seneviratne (Cham: Springer), 1–18. doi: 10.1007/978-3-319-49727-3_1
Stauffer, E., Dolan, J., and Newman, R. (2008). “Chapter 7—Flammable and combustible liquids,” in Fire Debris Analysis, eds E. Stauffer, J. A. Dolan, and R. Newman (Burlington: Academic Press), 199–233. doi: 10.1016/B978-012663971-1.50011-7
Stec, B. (2012). Structural mechanism of RuBisCO activation by carbamylation of the active site lysine. Proc. Natl. Acad. Sci. U. S. A. 109, 18785–18790. doi: 10.1073/pnas.1210754109
Steiner, K., and Schwab, H. (2012). Recent advances in rational approaches for enzyme engineering. Comput. Struct. Biotechnol. J. 2:e201209010. doi: 10.5936/csbj.201209010
Su, Y., Yang, B., Liu, J., Sun, B., Cao, C., Zou, X., et al. (2018). Prospects for replacement of some plastics in packaging with lignocellulose materials: brief review. BioResources 13, 4550–4576. doi: 10.15376/biores.13.2.Su
Sushkova, S. N., Minkina, T., Deryabkina, I., Mandzhieva, S., Zamulina, I., Bauer, T., et al. (2018). Influence of PAH contamination on soil ecological status. J. Soils Sediments 18, 2368–2378. doi: 10.1007/s11368-017-1755-8
Sussman, J. L., Lin, D., Jiang, J., Manning, N. O., Prilusky, J., Ritter, O., et al. (1998). Protein Data Bank (PDB): database of three-dimensional structural information of biological macromolecules. Acta Crystallogr. D 54, 1078–1084. doi: 10.1107/S0907444998009378
Tan, W. A., and Parales, R. E. (2016). “Application of aromatic hydrocarbon dioxygenases,” in Green Biocatalysis, ed R. N. Patel (Hoboken, NJ: Wiley), 457–471. doi: 10.1002/9781118828083.ch17
Thomas, C. D., Cameron, A., Green, R. E., Bakkenes, M., Beaumont, L. J., Collingham, Y. C., et al. (2004). Extinction risk from climate change. Nature 427, 145–148. doi: 10.1038/nature02121
Toscano, M. D., Woycechowsky, K. J., and Hilvert, D. (2007). Minimalist active-site redesign: teaching old enzymes new tricks. Angew. Chemie Int. Ed. 46, 3212–3236. doi: 10.1002/anie.200604205
Turner, N. J. (2009). Directed evolution drives the next generation of biocatalysts. Nat. Chem. Biol. 5, 567–573. doi: 10.1038/nchembio.203
Underwood, E. C., Viers, J. H., Klausmeyer, K. R., Cox, R. L., and Shaw, M. R. (2009). Threats and biodiversity in the mediterranean biome. Divers. Distribut. 15, 188–197. doi: 10.1111/j.1472-4642.2008.00518.x
Vidali, M. (2001). Bioremediation. An overview. Pure Appl. Chem. 73, 1163–1172. doi: 10.1351/pac200173071163
Wagner, D., Friedlander, A. M., Pyle, R. L., Brooks, C. M., Gjerde, K. M., and Wilhelm, T. A. (2020). Coral reefs of the high seas: hidden biodiversity hotspots in need of protection. Front. Marine Sci. 7:776. doi: 10.3389/fmars.2020.567428
Wang, D., Guo, W., Kong, S., and Xu, T. (2020). Estimating offshore exposure to oil spill impacts based on a statistical forecast model. Mar. Pollut. Bull. 156:111213. doi: 10.1016/j.marpolbul.2020.111213
Wang, T., Li, J., Xu, J., Fan, X., Zhao, L., Qiao, S., et al. (2018). Rational redesign of the active site of selenosubtilisin with strongly enhanced glutathione peroxidase activity. J. Catal. 359, 27–35. doi: 10.1016/j.jcat.2017.12.006
Wang, Y., Li, B., Ma, Y., Yang, L., and Song, X. (2020). Characterizing the variation of dissolvable PAHs in receiving water in a reclaimed water irrigation region. Water 12:2766. doi: 10.3390/w12102766
Wang, Z., Ma, X., Na, G., Lin, Z., Ding, Q., and Yao, Z. (2009). Correlations between physicochemical properties of PAHs and their distribution in soil, moss and reindeer dung at Ny-Ålesund of the Arctic. Environ. Pollut. 157, 3132–3136. doi: 10.1016/j.envpol.2009.05.014
Watanabe, N., Murata, M., Ogawa, T., Vavricka, C. J., Kondo, A., Ogino, C., et al. (2020). Exploration and evaluation of machine learning-based models for predicting enzymatic reactions. J. Chem. Inf. Model. 60, 1833–1843. doi: 10.1021/acs.jcim.9b00877
West, J. J., Cohen, A., Dentener, F., Brunekreef, B., Zhu, T., Armstrong, B., et al. (2016). What we breathe impacts our health: improving understanding of the link between air pollution and health. Environ. Sci. Technol. 50, 4895–4904. doi: 10.1021/acs.est.5b03827
Wittmann, B. J., Yue, Y., and Arnold, F. H. (2020). Machine learning-assisted directed evolution navigates a combinatorial epistatic fitness landscape with minimal screening burden. bioRxiv [Preprint]. doi: 10.1101/2020.12.04.408955
Wolf, D., Cryder, Z., Khoury, R., Carlan, C., and Gan, J. (2020). Bioremediation of PAH-contaminated shooting range soil using integrated approaches. Sci. Tot. Environ. 726:138440. doi: 10.1016/j.scitotenv.2020.138440
Xanthos, D., and Walker, T. R. (2017). International policies to reduce plastic marine pollution from single-use plastics (plastic bags and microbeads): a review. Mar. Pollut. Bull. 118, 17–26. doi: 10.1016/j.marpolbul.2017.02.048
Xia, G., Chen, L., Sera, T., Fa, M., Schultz, P. G., and Romesberg, F. E. (2002). Directed evolution of novel polymerase activities: mutation of a DNA polymerase into an efficient RNA polymerase. Proc. Natl. Acad. Sci. U. S. A. 99, 6597–6602. doi: 10.1073/pnas.102577799
Yadav, S. K., and Tiwari, A. K. (2015). Classification of enzymes using machine learning based approaches: a review. Mach. Learn. Appl. 2, 30–49. doi: 10.5121/mlaij.2015.2404
Yang, K. K., Wu, Z., and Arnold, F. H. (2019). Machine-learning-guided directed evolution for protein engineering. Nat. Methods 16, 687–694. doi: 10.1038/s41592-019-0496-6
Young, E., and Alper, H. (2010). Synthetic biology: tools to design, build, and optimize cellular processes. J. Biomed. Biotechnol. 2010:130781. doi: 10.1155/2010/130781
Zelinkova, Z., and Wenzl, T. (2015). The occurrence of 16 EPA PAHs in food–a review. Polycycl. Aromat. Compd. 35, 248–284. doi: 10.1080/10406638.2014.918550
Zhang, J. M., Harman, M., Ma, L., and Liu, Y. (2020). Machine learning testing: survey, landscapes and horizons. IEEE Transac. Softw. Eng. 1. doi: 10.1109/TSE.2019.2962027
Zhang, R., Xu, Y., and Xiao, R. (2015). Redesigning alcohol dehydrogenases/reductases for more efficient biosynthesis of enantiopure isomers. Biotechnol. Adv. 33, 1671–1684. doi: 10.1016/j.biotechadv.2015.08.002
Zhao, H., Chockalingam, K., and Chen, Z. (2002). Directed evolution of enzymes and pathways for industrial biocatalysis. Curr. Opin. Biotechnol. 13, 104–110. doi: 10.1016/S0958-1669(02)00291-4
Zhou, K., Ng, W., Cortés-Peña, Y., and Wang, X. (2020). Increasing metabolic pathway flux by using machine learning models. Curr. Opin. Biotechnol. 66, 179–185. doi: 10.1016/j.copbio.2020.08.004
Keywords: environment, crude oils, greenhouse gases, polycyclic aromatic hydrocarbons, enzymes, machine learning, metabolic engineering
Citation: Dutta K, Shityakov S and Khalifa I (2021) New Trends in Bioremediation Technologies Toward Environment-Friendly Society: A Mini-Review. Front. Bioeng. Biotechnol. 9:666858. doi: 10.3389/fbioe.2021.666858
Received: 11 February 2021; Accepted: 26 May 2021;
Published: 02 August 2021.
Edited by:
Alicia Ponte-Sucre, Central University of Venezuela, VenezuelaReviewed by:
Gustavo Caetano-Anollés, University of Illinois at Urbana-Champaign, United StatesVeronica Mora, National University of Río Cuarto, Argentina
Copyright © 2021 Dutta, Shityakov and Khalifa. This is an open-access article distributed under the terms of the Creative Commons Attribution License (CC BY). The use, distribution or reproduction in other forums is permitted, provided the original author(s) and the copyright owner(s) are credited and that the original publication in this journal is cited, in accordance with accepted academic practice. No use, distribution or reproduction is permitted which does not comply with these terms.
*Correspondence: Sergey Shityakov, shityakoff@hotmail.com; Kunal Dutta, kunal_fb@hotmail.com; Ibrahim Khalifa, ibrahiem.khalifa@fagr.bu.edu.eg