- 1Department of Computer Science and Technology, Tsinghua University, Beijing, China
- 2Institute of Precision Medicine, Tsinghua University, Beijing, China
- 3College of Engineering, China Agricultural University, Beijing, China
- 4Department of Biomedical Engineering, Tsinghua University, Beijing, China
- 5Musculoskeletal Department, Beijing Rehabilitation Hospital, Beijing, China
- 6Orthopaedics, Chinese PLA General Hospital, Beijing, China
Although intelligent technologies has facilitated the development of precise orthopaedic, simple internal fixation, ligament reconstruction or arthroplasty can only relieve pain of patients in short-term. To achieve the best recover of musculoskeletal injuries, three bottlenecks must be broken through, which includes scientific path planning, bioactive implants and personalized surgical channels building. As scientific surgical path can be planned and built by through AI technology, 4D printing technology can make more bioactive implants be manufactured, and variable structures can establish personalized channels precisely, it is possible to achieve satisfied and effective musculoskeletal injury recovery with the progress of multi-layer intelligent technologies (MLIT).
1 Introduction
In the past 30 years, intelligent technologies has facilitated the development of precise orthopaedic, an important direction in orthopedics/sports medicine, in the following three areas (Figure 1):
1) Digital orthopedics technologies have promoted the appreciation of digital anatomy (Pei and Yan, 2014) and biomechanics (Bhandarkar and Dhatrak, 2022). The three-dimensional models of bones reconstructed by digital orthopedics technologies, provide a means to establish the spatial relationship between anatomical structures. Shape statistical analysis allows that Elaborate anatomical analysis of bone can be performed. F. Chen Chen et al. (2018a) proposed a method to automatically identify three subtypes of femur cavities by k-means clustering analysis algorithm, on the basis of measuring the angle between the coronal plane and the radius of the femoral curvature (RFC) plane digitally. This method can provide a possible solution for the scientific design of Intramedullary (IM) nails, which will potentially facilitate IM nail implantation and reduce complications. Ghezlbash al. Ghezelbash et al. (2020) reviews the relevant findings of in vitro and finite element model studies on load-sharing in healthy, aged, degenerate and damaged human lumbar motion segments. They believed finite element model studies could improve understandings of functional biomechanics of human lumbar spine in normal and perturbed conditions.
2) 3D printing is an innovative technology for personalized treatment. Y. Liu et al. Liu et al. (2020) have prepared 3D printed polycaprolactone-hydroxyapatite (PCL-HA) porous scaffolds with loaded heparan sulfate (HS). This PCL-HA-HS scaffolds can accelerate the repairing of biological bone defects with sound compression resistance and good biocompatibility, which may be an effective biomaterial for bone defect repair. The study of B. Liu et al. Liu et al. (2021) showed that 3D printing technology can realize prosthesis stabilization and new bone regeneration in treating bone defects of limbs, to make patients achieve satisfactory limb function recovery. For total knee arthroplasty surgery, 3D printing navigation templates could predict prosthesis size accurately and provide an effective and precise guidance of osteotomy (Ding et al., 2017). 3D printing technology allowed accurate surgical simulation using life-size models, to valuate complex pelvic deformities precisely. This technology can improve anatomical appreciation and make personalized preoperative planning (Hughes et al., 2017), leading to reduce the risk of neurovascular injury.
3) Accurate surgical operations are carried out by robotics. In the past 5 years, the number of publications on orthopedic robotics has increased from 2500 to 6500 (Bernardo and Edoardo, 2021). Robot-assisted orthopedics surgeries can not only improve the accuracy of the operations (Jamwal et al., 2021), reduce operation time, radiation dose, and complications (Ye and Chen, 2009), but also optimize the learning curve (Nicolas et al., 2016; Kam et al., 2019; Tian et al., 2019).
However, simple internal fixation, ligament reconstruction or arthroplasty can only relieve pain of patients in short-term, because of the complexity of the musculoskeletal system (Neumann, 2010). The following problems limit the long-term therapeutic efficacy of precise orthopaedic:
1) Current surgical planning paths are the optimal geometric paths, but not the optimal bio-mechanical paths, which may not result in the optimal functional recovery of patients. The osseous tissue is not a uniform organization, which is mainly made up of two types of structural tissues, namely cancellous (trabecular) and cortical bone (Wubneh et al., 2018). The geometric center and the density center of the femoral head are different. Moreover, the density of different parts varies each other (Ahrend et al., 2021). Johns Hopkins University’s Vijayan R. Vijayan et al. (2019) reported an algorithm for automatic spinal pedicle screw planning using Active Shape Model (ASM) registration. But the manual path drawn by doctor is different with the automatic path calculated by the ASM algorithm. The fact that the clinical experiences, like predictions of bone mineral densities of different parts, may be considered into the manual preoperative planning by surgeons can result in the differences.
2) The fact that traditional bone grafts and bone substitute materials cannot provide signals for endogenous repair (Loebel and Burdick, 2018), can cause failure to induce bone formation or promote angiogenesis. Therefore, traditional orthopedics treatments may provide unsatisfied musculoskeletal rehabilitations. Bone grafts with shape memory effect, stimuli responsiveness, can maximize the new bones forming and the neovascularization, which are of great significance for improving the recovery of patients.
As shown in Figure 2, similarly to the construction process of a building, MLIT for musculoskeletal injuries consists of three stages: scheme design, scheme implementation, and enhancements (Table 1).
Therefore, the multi-layer intelligent technologies (MLIT), including smart materials, variable structures, and intelligent therapeutic planning, which are on basis of the musculoskeletal biomechanical characteristics and the rehabilitation model of stimulus implants, will be the main trend of precise orthopaedic. Specifically, three bottlenecks must be broken through to achieve satisfied recovery: scientific path should be planned and built by AI algorithms, bioactive implants should be manufactured with smart materials, and personalized channels should be established by variable structures. This article will discuss the feasibility of satisfied and effective musculoskeletal injury recovery in the following aspects:
1) Scientific surgical path can be planned and built by through AI technology
2) Responsive bioactive implants made through 3D printing technology can provide signals for endogenous repair
3) Personalized surgical channels can be established through intelligent robotics precisely
2 Status of multi-layer intelligent technologies
2.1 Scientific surgical path can be planned and built by through AI technology
Surgical placement and appropriate implant fixation had equal importance as the inherent implant characteristics in maintaining long-term implant stability (Li et al., 2019). Biomechanical surgical path must be planned on basis of the pathogenesis of musculoskeletal diseases, to reach the satisfied musculoskeletal injury recovery. This requires physical parameters to be predicted precisely, to establish build kinesiology models of musculoskeletal system. In the past decade, AI technologies has made dramatic advances on Orthopaedic in the following four aspects (Zhou et al., 2020a; Lopez et al., 2021a) (Figure 3): automatic reconstruction, physical parameters predicting, preoperative planning and intraoperative images registration.
2.1.1 Automatic reconstruction algorithm
In the early study, threshold segmentation or seed growth combined with manual repair are often used to segment medical images like CT, MRI etc. This semi-automatic method is very inefficient. AI technology, especially the U-net (Li et al., 2017; Falk et al., 2019), has promoted the rapid development of medical image segmentation. In general, variants of the U-net can be divided into four categories (Figure 4): ① Design encoder or decode structures. Cascade decoder conducted more effective decoding of hierarchically encoded features (Liang et al., 2019). The encoder of TernausNet removed the fully connected layers and replace them with a single convolutional layer of 512 channels that serves as a bottleneck central part of the network, and the decoder of TernausNet transposed convolutions layers that doubles thesize of a feature map (Iglovikov and Shvets, 2018). TernausNet was helpful to prevent over-fitting. ②Optimize connection modes of encoding module and decoding module. MNet proposed a skip connection method to balance the spatial representation inter axes via learning (Dong et al., 2022); UNet++ redesigned skip connections to exploit multiscale features in image segmentation (Zhou et al., 2020b).③ Set new loss function. Loss function is a method to measure the quality of model prediction, which is of course important to an AI model. Dice loss is a common loss function for medical image segmentation. However, it may cause oscillation during training when the prediction is close to ground truth (Chen et al., 2018b). Cos-Dice loss function was used in W-net to make the network more stable (Chen et al., 2018b). The adjustable penalty weights of the misclassified voxels were used in dice coefficient to adapt to unbalanced class frequency (Huang et al., 2018). Loss functions based on the Tversky index (Salehi et al., 2017; Abraham and Khan, 2018; Huang et al., 2018; Das and Zhang, 2020)were used to address the issue of data imbalance. ④Import attention mechanism. Swin Unet was a semantic segmentation of brain tumors in MRI Images using a swin transformer encoder which can extract features at five different resolutions by utilizing shifted windows for computing self-attention (Hatamizadeh et al., 2021). RA-UNet proposed a 3D hybrid residual attention-aware segmentation method to precisely extract the liver volume of interests (VOI) and segment tumors from the liver VOI (Jin et al., 2018). TransUNet was a variant of U-net using a transformer encodes which tokenized image patches from a convolution neural network (CNN) feature map as the input sequence for extracting global contexts (Chen et al., 2021). UNEt TRansformers (UNETR) utilized a transformer as the encoder to learn sequence representations of the input volume and effectively capture the global multi-scale information (Hatamizadeh et al., 2022). Particularly in 2021, the nn-Unet proposed by Isensee F. et al. Isensee et al. (2021) at Heidelberg University in Germany surpasses most existing approaches on 23 public datasets used in international biomedical segmentation competitions. Variants of the U-net or other deep learning algorithms are widely used in segmentations for pelvic, spine, femur, knee arthroscopy and other orthopedics fields (Zeng et al., 2017; Balagopal et al., 2018; Kolařík et al., 2019; Isensee et al., 2021).
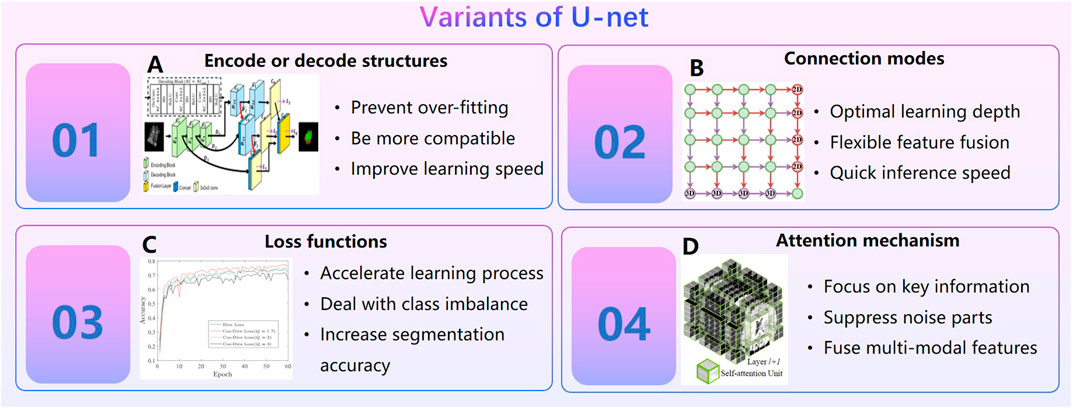
FIGURE 4. Variants of U-net. (A). Cascade decoder: A Universal Decoding Method for Biomedical Image Segmentation (Liang et al., 2019). (B). MNet: Rethinking 2D/3D Networks for Anisotropic Medical Image Segmentation (Dong et al., 2022). (C). A Novel Focal Tversky loss function with improved Attention U-Net for lesion segmentation (Abraham and Khan, 2018). (D). Swin UNETR: Swin Transformers for Semantic Segmentation of Brain Tumors in MRI Images.
2.1.2 Physical parameters predicting method
Extraordinarily important is that AI technology perform well in predicting physical parameters of organizations. In 2019, a review by Rogers M.A. Rogers and Aikawa (2019) of Harvard University in the United States showed that AI technology can analyze the cardiovascular calcification on quantitatively large data sets. In 2020, Black K.M. et al. Black et al. (2020) of the University of Michigan automatically detected kidney stones composition from digital photographs of stones by ResNet-10, and precisions for each stone type were above 75%. In 2021, the study of Lopez F. et al. Lopez et al. (2021b) showed that the DCCN approach is the best method with a precision of 98% for four kidney stones classification with in-vivo endoscopic images. In 2021, Molnar D. Molnar et al. (2021) et al. of Gothenburg in Sweden proposed a Crop-Net for fat prediction, with a precision of 99.4%.
In the field of orthopedics, AI technology can predict bone density accurately (Figure 5). Hsieh C.I. from Chang Gung Hospital in Taiwan (Hsieh et al., 2021) proposed the Dual-energy X-ray A bsorptiometry (DXA)to predict bone mineral density and fracture risk, and the accuracy of hip osteoporosis prediction reached 95%. In 2020, the BMDCNN is applied to predict the bone mineral density (BMD) of the lumbar spine by Yasaka K. Yasaka et al. (2020) from the University of Tokyo, and the internal and external AUC of osteoporosis AUC 0.965 and 0.970.
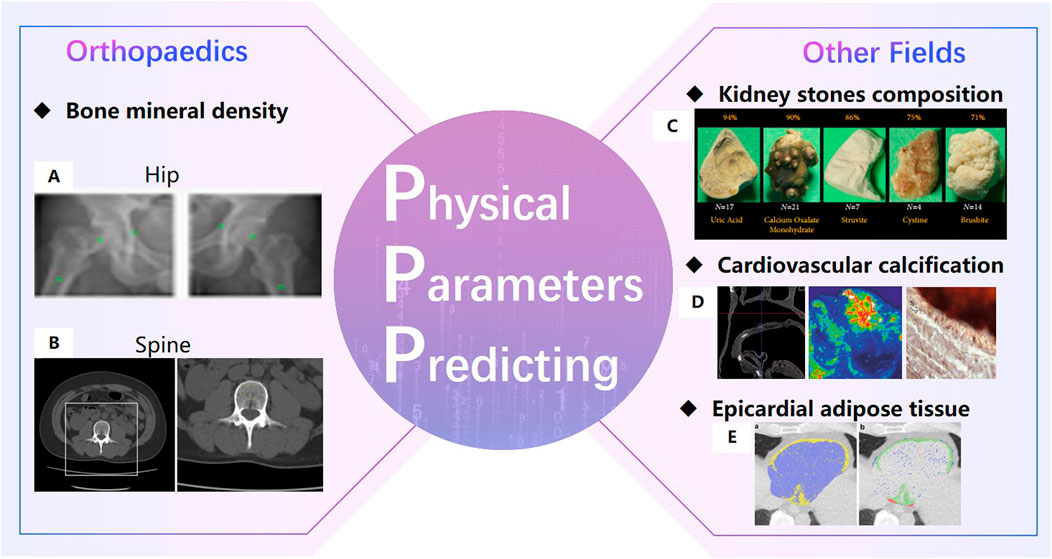
FIGURE 5. Physical Parameters Predicting (Hsieh et al., 2021). (A). Bone mineral density prediction of hip (Yasaka et al., 2020). (B). Bone mineral density prediction of lumbar spine. (C) automatically detect kidney stones composition (Black et al., 2020). (D). cardiovascular calcification (Dhivya et al., 2015).(E). Epicardial adipose tissue prediction (Molnar et al., 2021).
2.1.3 Preoperative planning
Preoperative planning is an essential part of Clinical Decision Support System(CDSS). Screw placement position (Cai et al., 2019), implants size (Dong et al., 2021; Polce et al., 2021), osteotomy morphology are three common preoperative planning needs in Orthopaedic surgery (Figure 6). Target reconstructions, landmark/anatomical components recognization (Cai et al., 2019; Siemionow et al., 2021), physical parameters (bone mineral density (Caprara et al., 2021), morphological parameters (Soodmand et al., 2019)), finite element analysis (Zheng et al., 2018a; Caprara, 2021), personal information and other risk constraints may be possible inputs for preoperative planning. AI technologies can play a role in the processing of planning inputs. AI applications in bone reconstructions and physical parameters predictions have been discussed in Section 2.1.1 and 2.1.2. It is well-attended to predict how bone adapts to different loads in surgical planning (Jordi et al., 2022). FE is commonly performed in biomechanics analysis. It is necessary to build statistical shape models (SSM) based on a set of landmark points (Zheng and Yu, 2017) for FE analysis. An SSM built by the training dataset is a set of annotated images (Zheng and Yu, 2017). AI technologies make the landmarks/anatomical components recognization automatically (Siemionow et al., 2021). Nathan proposed a deep learning method based on the PointNet++ architecture for biomechanical modeling of facial tissue deformation in orthognathic surgical planning (Lampen et al., 2022). Xiao Xiao et al. (2021) proposed an estimating Reference Bony Shape Models for Orthognathic surgical planning using 3D point-cloud deep learning. Due to the incompleteness of information and uncertainty of healing prediction, preoperative planning dose not have a rapid development like reconstruction technologies.
2.1.4 Intraoperative registration
The accuracy of robotic assisted surgery mainly depends on the space registration. The 2D-3D registration is commonly used in spinal surgery or pelvic surgery and the 3D-3D registration is commonly used in TKA (total knee arthroplasty) surgery. There are always tow workflows of 2D-3D registration (Figure 7): 1) Projection based 2D-3D registration. Digitally reconstructed radiographs (DRR) are generated from CT images, and then mutual information, normalized cross correlation, sum of square differences or other similarity measurements are used to calculate the relationship between X‐ray images and DRRs (Guo et al., 2019). 2) Reconstruction based 2D-3D registration. 3D point clouds are reconstructed from 2D x-ray or ultrasonic images. Transformation matrix are calculated through tow 3D point clouds (Wang et al., 2018). The intraoperative point clouds of knee are commonly collected by probes with markers in TKA surgery. The preoperative and intraoperative point clouds are matched through ICP or CPD algorithms.
Due to the sparsity inconsistency of point clouds between preoperative images and intraoperative images, deformation differences caused by different parameters of different imaging devices, the accuracy of surgical registration methods has been paid a great attention, but not yet been well solved. Registration methods based on deep-learning (Lu et al., 2021) have shown be capable of addressing the limitations of conventional registration methods (Figure 7), as they have a better performance in predicting deformations, learning 3D point clouds features, generating DRRs and optimizing registration strategy. Unsupervised learning (Chen et al., 2022) estimate voxel-to-voxel deformable transformation by maximizing image similarity (Fan et al., 2019). Weakly-supervised regularization loss is able to map thecomplex appearance to a common space (Blendowski et al., 2021). Self-supervised anatomical embedding (SAM) (Yan et al., 2022) is capable of computing dense anatomical/semantic correspondences between two images at the pixel level. Feature learning methods use the deep neural network to learn a robust feature correspondence search (Huang et al., 2021a), which includes a local PPF feature using the distribution of neighbour points (Deng et al., 2018), a rotation-invariant hand-craft feature (Gojcic et al., 2019), a global features (Qi et al., 2017) etc. There are three registration strategies using AI (Huang et al., 2021a): ICP-based variations, graph-based, GMM-based and semi-definite registration methods. Deep Closest Point(DCP) uses deep features to estimate correspondences to avoid spurious local optima of most ICP algorithms (Wang and Solomon, 2019). The surface registration are solved effectively by transforming the registration problem into a graph matching problem (Le-Huu and Paragios, 2017). For Gaussian mixture models (GMM), DeepGMR (Yuan et al., 2020) uses a neural network to learn pose-invariant point-to-distribution parameter correspondences. DRRs are simulated by algorithm which may differ from the real X-ray images. To improve the quality of DRRs, GAN-based training, material decomposition, and incremental learning are proposed. A GAN-based disentanglement learning framework (Han et al., 2021) can transfer the rib structural priors from DRRs. DeepDRR is a framework for DRRs embedding material decomposition and scatter estimation in 3D and 2D, combined with analytic forward projection and noise injection (Unberath et al., 2018). Incremental learning can improve continuously acquire new knowledge by continuously acquiring new knowledge (Jiang et al., 2021).
2.2 Responsive bioactive implants made through 3D printing technology can provide signals for endogenous repair
Autograft, allografts and metal-based material scaffolds are commonly used in the clinical treatment of bone defects. However, chronic inflammation, immune rejection or stress shield and inflammation have hindered their clinical application. New bioactive responsive implants made through 3D printing technology and adaptively expanded, which can provide signals for endogenous repair, is highly necessary to promote bone regeneration (Figure 8). Bioactive materials, especially bioactive polymers high osteoinduction, excellent angiogenesis, biocompatibility and unlimited size, can interact with proteins, cells or tissues in vivo and cause biological reactions.
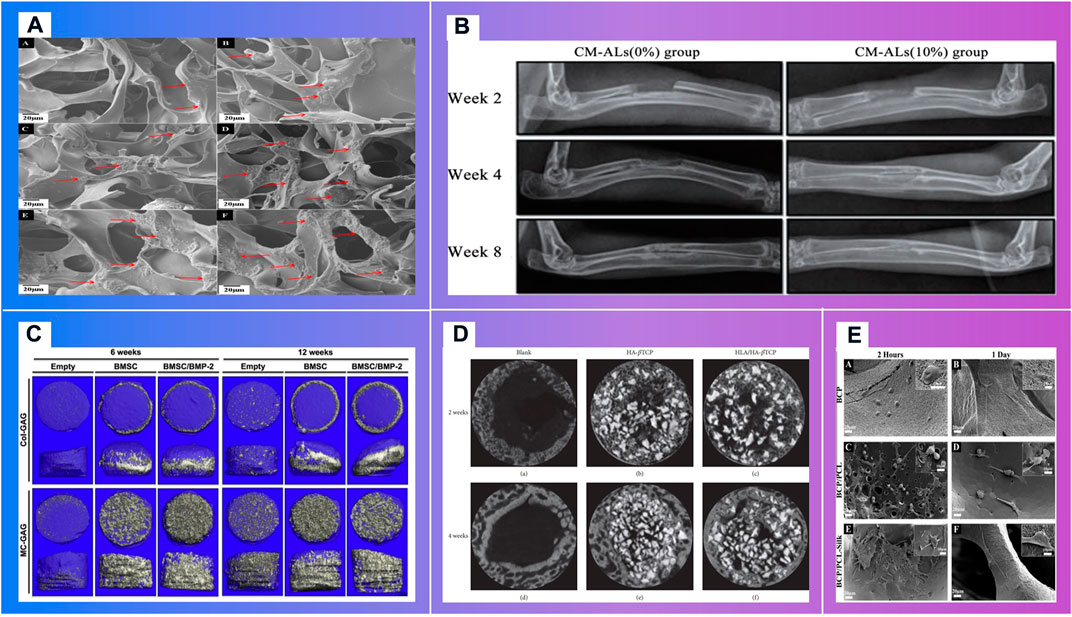
FIGURE 8. Bioactive materials demonstrating the osteoblast migrations. (A). In vitro mineralization on mCT scanning of BMSCs cultured on Col-GAG and MC-GAG scaffolds in the absence and presence of BMP-2 (Makvandi et al., 2020) (B). In vitro mineralization on mCT scanning of BMSCs cultured on Col-GAG and MC-GAG scaffolds in the absence and presence of BMP-2 (Ren et al., 2016) (C). X-ray analysis of new bone formation in CM-ALs (0%) and CM-ALs (10%) implanted groups (Wu et al., 2017). (D). Micro-CT images of the artificial defects at weeks 2 and 4. (Chang et al., 2016) (E). Morphology of cultured HOB on (A,B) BCP, (C,D) BCP/PCL and (E,F) BCP/PCL–silk scaffolds after 2 and 24 h (Roohani-Esfahani et al., 2012)
2.2.1 Responsive bioactive materials can promote the reconstruction of organizations
Bioactive materials can guide bone regeneration (Mistry et al., 2015) (Figure 9). Dhivya S.et al. Dhivya et al. (2015) explored a nanoparticulate mineralized collagen glycosaminoglycan scaffold that induces healing of critical-sized rabbit cranial defects. These inorganic nano materials delivery of bioactive agents. However, their widespread employment may be reduced due to the possible toxicity and the lack of biodegradability (Makvandi et al., 2020).
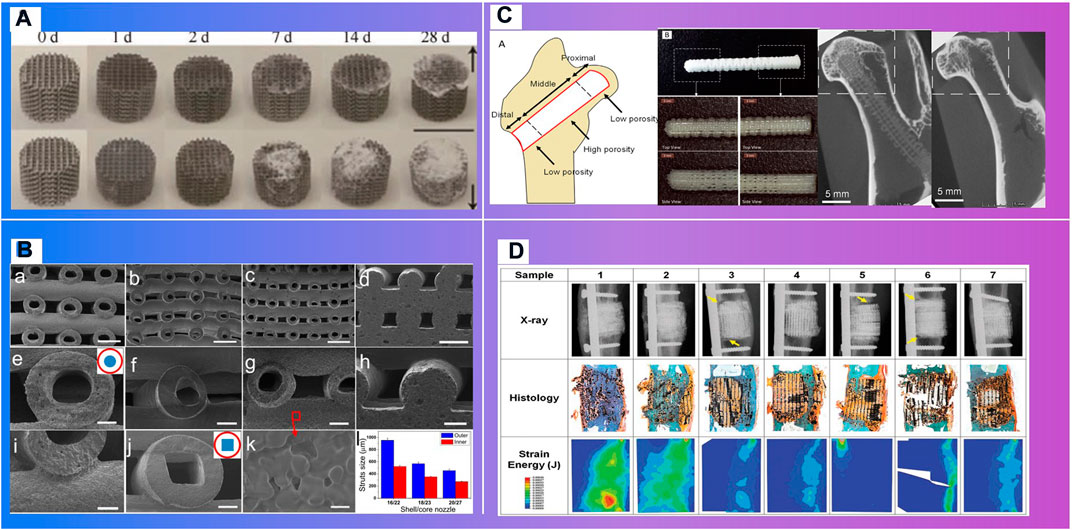
FIGURE 9. Materials with biodegradability. (A). Degradation process (Li et al., 2020). (B). SEM images of printed HSP bioceramic scaffolds via core/shell nozzle of 16/22 18/23 and 20/27 and printed SSP bioceramic scaffolds as the control (Luo et al., 2015). (C). A functionally graded scaffold (Kawai et al., 2018). (D). Mathematical modeling of total strain energy for defects implanted with the Sr-HT-Gahnite scaffold at 12 months postimplantation (Li et al., 2019).
Polymer materials consisting of inorganic nanomaterials and organic polymer materials, with high ductility, biocompatibility and biodegradability, are widely used in bone repair scaffolds (Figure 11). Their specific structure and surface properties can be specifically recognized and interact with target biomolecules. Makvandi P.et al. Makvandi et al. (2020) developed a graphene oxide (GO)–Chitosan (CS)–Hyaluronic acid (HA) based bioactive composite scaffold containing an osteogenesis-inducing drug simvastatin (SV). The elongated morphology of the cells after 48 h incubation has demonstrated the osteoblast migration using the SV loaded GO–CS–HA scaffolds. A collagen–hydroxyapatite(HA) scaffold (Unnithan et al., 2017) with a degree of interconnectivity of 99%, can not only deliver biological factors, promote stem cell differentiation and ossification (Thitiset et al., 2013; Villa et al., 2015), but also theoretically be applied in a load bearing application when combined with mechanical fixation (Zhang et al., 2018). Mouse BMSCs isolated from the femur and tibia of CD1 wild type animals, were seeded dropwise on to the top of either the Col–HA scaffold. Cells well were attached on the scaffolds after 12 h of seeding. In the study of in vivo bone formation in a mouse calvaria defect, scaffolds were implanted. After 3 weeks of implantation, calvaria containing critical size defects filled with BMSCs combined with either the Col–HA scaffold in X-ray images (Unnithan et al., 2017). The research of Ren X. et al. Ren et al. (2016) demonstrated that a nanoparticulate mineralized collagen glycosaminoglycan scaffold showed more efficient mineralization of MC-GAG scaffolds than non-mineralized Col-GAG scaffolds in either the histologic analyses or mCT scanning images. Wu H. et al. Wu et al. (2017) has fabricated a chitosan-based microsphere delivery system to controlled release of alendronate (AL), which can release AL for up to 30 days. CM-ALs (10%) scaffolds showed better performance in large-sized bone defects repairs than CM-ALs (0%). The new bone increasing ratio (NBIR) of HLA/HA-βTCP samples was 1.78 times higher than the blank group at week 2 (Chang et al., 2016). The study of Roohaniesfahani S.I. et al. Roohani-Esfahani et al. (2012) showed that, BCP/PCL scaffolds with silk layer is more favorable than BCP/PCL scaffolds with collagen layer in mechanical properties and biological properties.
More importantly, responsive bioactive materials, especially shape memory polymers (SMP) (Figure 10), can remember the temporary shape and return to the original shape under the condition of the external stimuli, like heat, pH, electricity, magnetic field, etc (Zhang et al., 2012; Rezwan et al., 2006; Zheng et al., 2018b). This specific shape memory could simplify complex transplant procedures, with excellent chemical stability, biocompatibility and biodegradability, which can stimulate specific cellular responses. Deng Z.et al. Deng et al. (2016) designed and synthesized a series of shape memory copolymers with electroactivity, super stretchability and tunable recovery temperature based on poly(e-caprolactone) (PCL) with different molecular weight and conductive amino capped aniline trimer. They proved that they can enhance myogenic differentiation from C2C12 myoblast cells. Xie R.et al. Xie et al. (2017) prepared a novel polyurethane or hydroxyapatite based SMP porous foam for the treatment of load-bearing bone defects by gas foaming. The foam can match the trabecular bone, possess the feasibility of minimally invasive delivery. And it can also overcome the disadvantages of traditional polymer foams in terms of insufficient mechanical properties, inadequate pore structures, low biocompatibility and inconvenience in operation. The rabbit femoral defect model (Xie et al., 2018) demonstrated that a SMP foam bone scaffold could play an important role in promoting neovascularization and bone remodeling. A shape memory PCL porous scaffold was made from photocrosslinking (ε-caprolactone) (PCL) polydiacrylate through SCPL method (Zhang et al., 2014), and it evidently promoted the adhesion and proliferation of osteoblasts. SMP scaffolds show strong self adaptability, as evidenced by the following facts. One of these is that smaller SMP scaffolds than bone defect size can match bone defect boundary after shape expansion (Zhang et al., 2012; Xie et al., 2017), the other is that larger SMP scaffolds can promote the inward growth of bone, due to the binding force with bone tissue (Zhang et al., 2014; Bao et al., 2016).
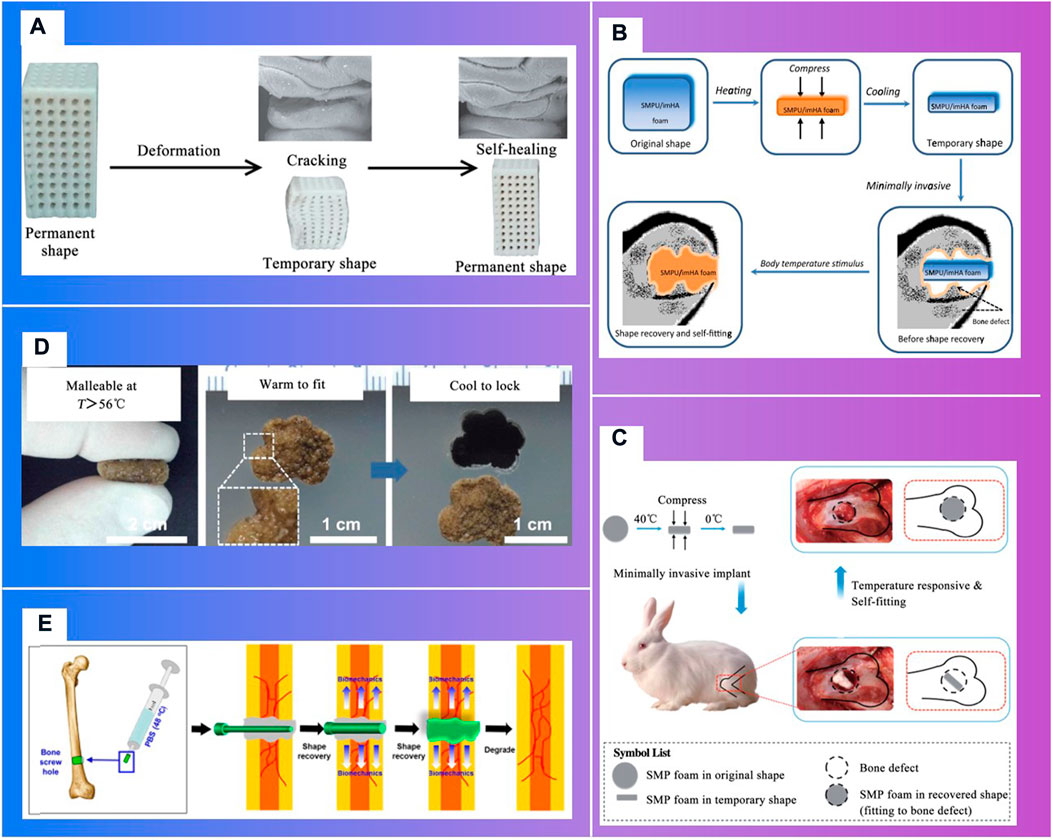
FIGURE 10. Shape memory polymers in bone tissue engineering. (A) t Fixing of a temporary shape of a 3D-printed PLA/HA scaffold through compression (Senatov et al., 2016) (Xie et al., 2017). (B). Schematic of body-temperature responsive SMPU/imHA foam for minimally invasive delivery in application of bone regeneration (Xie et al., 2017). (C). Schematic of self-adaptive SMP foam as a bone scaffold for bone regeneration (Xie et al., 2018). (D). Self-adaptive process of polydopamine-coated shape memory porous PCL scaffolds (Zhang et al., 2014). (E). Schematic illustration showing the application of shape-memory-capable scaffold of the Hap/PLMC naofibers (Xie et al., 2018).
2.2.2 3D bioprinting technology can personalize implants
Three-dimensional printing technologies that can fabricate the microstructure of materials precisely, have shown distinct advantages to personalize implants in bone tissue engineering (Luo et al., 2015). In clinical medicine, 3D printing technologies can be divided into two stages (Figure 11): one is conventional 3D printing objects without cellular information exchange, like surgical guiding paltes (Butscher et al., 2013), prosthesis (Xu et al., 2013; Turnbull et al., 2018); the other is bioactive 3D printing objects can provide signals for endogenous repair, which particularly is of great significance to bone regeneration and vascular reconstruction, such as degradable scaffolds (Xu et al., 2019a), living cell printing (Kolesky et al., 2014), and stimuli-responsive printing materials with programmable behavior (Gladman et al., 2016) (Qi et al., 2014).
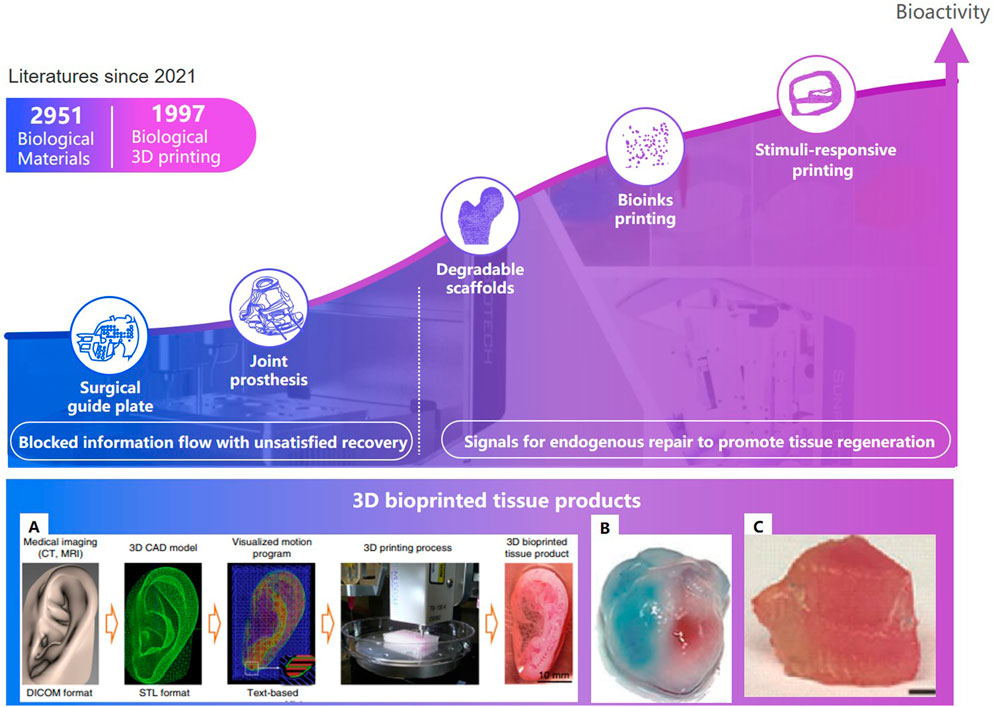
FIGURE 11. Development of tissue engineering and 3D bioprinted tissue product. (A). The ITOP system (Groll et al., 2018).(B). Ventricles (Zhang et al., 2016). (C). Thyroid cartilage (Van Belleghem et al., 2020).
Degradable 3D printing scaffolds can gradually release the occupied space with the recovery of the damaged part (Figure 7.C) (Li et al., 2020). Kawai T. et al. Kawai et al. (2018) of Stanford University designed and 3D printed a functionally graded scaffold (FGS) made of polycaprolactone (PCL) and b-tricalcium phosphate (b-TCP) to treat Osteonecrosis of the femoral head. The in vivo degradation rate and the bone ingrowth ratio of the scaffold is significantly higher than the empty-tunnel group. Li et al. Li et al. (2019) at University of Sydney developed a 3D-printed Sr-HT-Gahnite scaffolds implanted into critical-sized segmental defects in sheep tibia. Compared with bone autografts, the scaffolds possessing both osteoconductive and osteoinductive properties, can induce substantial bone formation and defect bridging. Spiral fractures were observed in the study. The fractures may have a negative effect on the recovery. Thus, implants should be fixed at appropriate position.
2.2.3 Bio implants through bio-inks can migrate in planned direction
Bioinks is a formulation of cells suitable for processing by an automated biofabrication technology that may also contain biologically active components and biomaterials (Groll et al., 2018). Biomaterials may consist of cells, collagen, and other bioactive ingredients; Auxiliary biomaterials, such as gelatin, alginate hydrogel, carbomer glue, etc, can be used to improve mechanical strength of implants, maintain the shape of the printed object, and ensure the adhesion and survival rate of cells. In 2015, Kang H.W. et al. Kang et al. (2016) of the Wake Forest Institute for Regenerative Medicine developed a system that deposits cell-laden hydrogels together with synthetic biodegradable polymers that impart mechanical strength. The printed mandible, skull, cartilage and skeletal muscle can incorporate multiple cell types at precise locations to recapitulate native structure and function. In 2016, Zhang Y. S. Zhang et al. (2016) of Harvard Medical School proposed a novel hybrid strategy based on 3D bioprinting to fabricate endothelializedmyocardium. Endothelial cells controlled anisotropy, can gradually migrate towards the peripheries of the microfibers.
With the continuous upgrading of material technology, biological 3D printing strategy is constantly updated. In 2019, Van Belleghem S.et al. Van Belleghem et al. (2020), from the University of Maryland College Park, proposed a 3D printing strategy for dual bioinks. The graft consisting of both degradable and nondegradable parts, providing long term mechanical integrity and shape retention. A pre-programmed responsive bioactive materials can be used in various application where the human intervention is not possible. This technology may lead to satisfied repair. The materials which responds to external stimuli is called 4D printing (Tibbits, 2014). A porous PLA/HAP scaffolds can stand up to three compression-heating-compression cycles without delamination. The scaffolds can narrow the cracks during heating, which may resulted in ‘self-healing’. The significant changes of post implantation have higher requirements to ensure the stability during deformation process of the implants, and that the deformation not cause additional damage to the surrounding tissues.
2.3 Personalized surgical channels can be established through intelligent robotics precisely
In clinical, there are linear channels established, due to the limitation of the structure of instruments (Nicolas et al., 2016; Zhang et al., 2019). Fracture or scoliosis treatments are great different between children and the elderly, because children have better self-healing in growth. Linear channels may be not meet the biomechanics needs, especially for the elderly. As smart materials and variable structures developed, such as memory alloys, concentric tube structures, etc., curved-trajectory, with less trauma and better therapeutic effect, attracts the researchers’ great attention (Figure 12). Moreover, intelligent sensing technology and intelligent operation technology also make the surgery more efficiency.
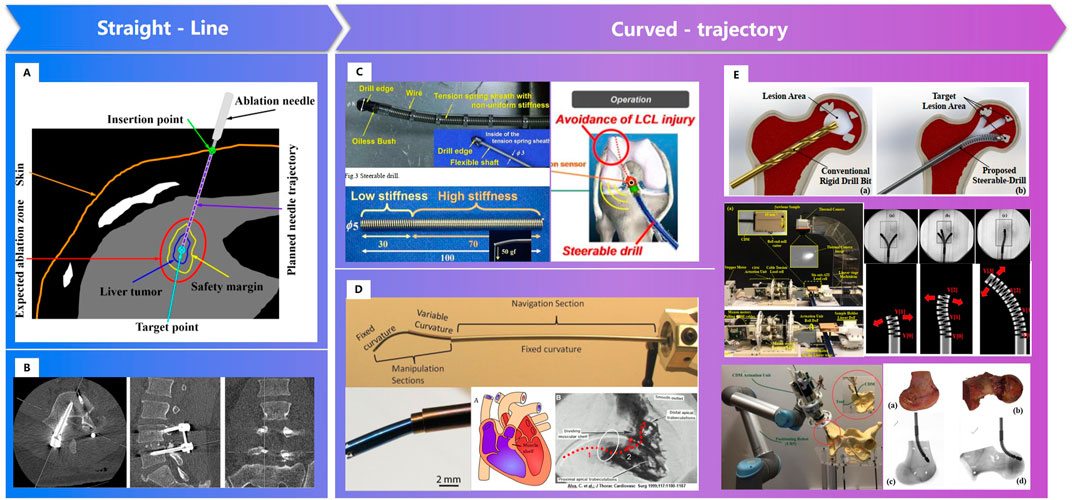
FIGURE 12. Surgical channels constructed by robot. (A). Straight needle trajectory planning for radiofrequency ablation and microwave ablation of liver tumors (Zhang et al., 2019). (B). Linear surgical channel for spinal screw internal fixation (Nicolas et al., 2016). (C). A “Steerable Drill” for ACL Reconstruction to Create the Arbitrary Trajectory of a Bone Tunnel (DePhillipo et al., 2018). (D). Endoscopic Add-on Stiffness Probe (Gilbert et al., 2014). (E). A less-invasive surgical workstation to treat osteolytic lesions behind a well-fixed acetabular implant (Bakhtiarinejad et al., 2020)- (Alambeigi et al., 2014).
2.3.1 Personalized surgical channels can be established through variable structures
Personalized surgical channels are linear channels, curved- channels or linear- curved mixed channels based on the status of patients. In 2011, Watanabe H. Watanabe et al. (2011) et al. developed a steerable drill for ACL reconstruction to construct an arbitrary trajectory of a bone tunnel. Gilbert H. Gilbert et al. (2014) adopted a needle-sized tentacle-like robot that require access through constrained paths in transnasal skull base surgery. In 2012, Gosline, A. H. et al. Gosline et al. (2012) manufactured a steerable curved concentric tube robot that can enter the heart through the vasculature, using a unique metal MEMS process.
Since 2014, Alambeigi F. of Johns Hopkins University et al. Alambeigi et al. (2014), Alambeigi et al. (2016a), Alambeigi et al. (2016b); Wilkening et al., 2017; Bakhtiarinejad et al., 2020) has researched the novel steerable drill using a continuum dexterous manipulator (CDM), and carried out experiments on both simulated and human cadaveric bones. Bakhtiarinejad M. Bakhtiarinejad et al. (2020) studied the use of curved drilling technique for treatment of osteonecrosis of femoral head. The biomechanical study demonstrated that a novel robot-assisted curved core decompression (CCD) technique is introduced to provide surgeons with direct access to the lesions causing minimal damage to the healthy bone. The progress of bistable structure, shape memory polymer and intelligent variable structure promoted the adaptability of surgical robot, which enable the biomechanical channels for orthopaedics.
2.3.2 Intelligent sensing technology can lead to precise trajectory in complex environment
Unlike industrial scene, the intraoperative environments are always complex. The robot cannot perceive, explain and understand the surrounding environment as well as surgeons is one of the key factors that the robotics ca not perform surgery automatically. Deformation predicting and tracking, and haptic feedback are tow common intelligent sensing technologies in surgical robotics (Figure 13). Shademan Shademan et al. (2016) demonstrated in vivo supervised autonomous soft tissue surgery which can suture a wound automatically. Deep reinforcement learning policies were used in Pattern Cutting task to induce tension in the material as cutting proceeds (Thananjeyan et al., 2017). Force sensors were developed to estimate interaction forces in robotic surgery (Kesner and Howe, 2011; Marban et al., 2018). And a Semi-Supervised Deep Neural Network Model was applied to understand the operation pattern (Marban et al., 2018).
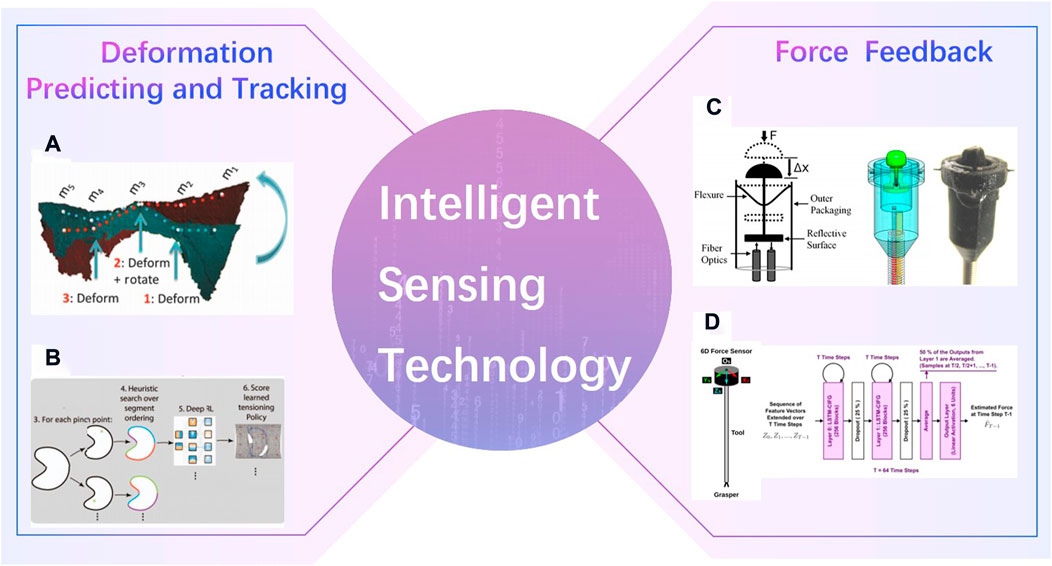
FIGURE 13. Intelligent Sensing Technology. (A) in vivo supervised autonomous soft tissue surgery (Shademan et al., 2016) (B). Deep reinforcement learning policies were used in Pattern Cutting task (Thananjeyan et al., 2017). (C). Force sensors in robotic surgery (Kesner and Howe, 2011) (D). Semi-Supervised Deep Neural Network Model (Marban et al., 2018).
2.3.3 Robotic skill learning can improve the automation level of surgical robotics
The surgical robotics in clinical are commonly semi-automatic. They can perform intraoperative actions based on the double closed-loop control of force position mixing in puncture (Wells et al., 2016), cochlear implant (Wang et al., 2020), fracture reduction (Lei et al., 2019) or other surgeries. However, it is impossible to dynamically adjust the task strategy according to the actual situation (Graham et al., 2008; Zhang, 2018). Skill learning (Silver et al., 2016; Mahler et al., 2019) make robots execute task which difficult by traditional control methods (Levine et al., 2016; Sun et al., 2017; Mandlekar et al., 2018), like pouring water and screwing screws, automatically.
3 Future research directions for satisfied repair of musculoskeletal injuries
The multi-layer intelligent technologies (MLIT) offer possibilities to achieve satisfied repair of musculoskeletal injuries. However, there are still no wide range of clinical applications, because of the following reasons: 1) the pathogenesis like the femoral head necrosis or the spinal tumor, the healing process like the ligament or the bone repair, and the biomechanical or kinematics models are not clarified in detail. 2) the real environment is hard to simulate. As there are differences between the simulation environment and the real environment, further verification of some research results should be implemented. 3) some theoretical designed surgical plan cannot be realized as expected. Further research will be needed for satisfied repair of musculoskeletal injuries:
3.1 The research on the pathogenesis or the healing process can be more effective through new AI technologies
3.1.1 Biomechanical models based on multi-physical parameter prediction will be built
Current biomechanical models are always based on 3D reconstructed models. However, morphology and parameters of the ligament, meniscus (Antico et al., 2020) and other tissue are always artificially devised (Viris et al., 2016), and the same density of different bone tissues like cortical bone, cancellous bone and trabecular bone structures are commonly set in biomechanical analysis. It is said that kinematics simulations are greatly different from real scene (Bell et al., 1996). To build real biomechanical models, physical parameter such as the bone density, ligament elastic modulus, must be predicted precisely.
Although some physical parameters predicting methods are proposed, which are discussed in Section2.1.2, lots of parameters still cannot be predicted for the lack of data with high-quality labels. Standard labeling procedure, and cross check of data labels are necessary.
3.1.2 Causal relationship between symptoms and physiological factors will be discovered by causal discovery
R Ganz (Siebenrock et al., 2010) proposed a surgical plan which can retain the vascular supply of the femoral head, to guarantee the oxygen and nutritional supply to the cells (Kramer et al., 2009). However, excessive vascular supply may be not conducive to recovery. Further research on the pathogenesis or the healing process for femoral head necrosis, especially the causality, but not only the correlation between the symptoms and physiological factors should be deserved. Now causal discovery, which can promote the research of disease mechanism, has been applied in hippocampal function analysis (Mannoor et al., 2013; Sanchez-Romero et al., 2018). Causal discovery can be a useful theory for the research on the pathogenesis or the healing process.
3.2 Intelligent technologies will make add the functionality of implants and make the implants manufacture process more efficiently
3.2.1 Implant with intelligent sense will provide real recovery status
Implants bioprinting combined with intelligent sense is also a research hotspot (Figure 14). In 2013, Mannoor M.S.et al. Mannoor et al. (2013) generated a bionic ear via additive manufacturing of biological cells with structural and nanoparticle derived electronic elements. In 2019, Jodat, Y.A. et al. Jodat et al. (2020) designed an odor-perceptive nose-like hybrid, with 3D cartilage-like tissue constructs. It is composed of a mechanically robust cartilage-like construct and a biocompatible biosensing platform. Patients can not recover only by surgery without rehabilitation training as the human body systems has changed after operations. Doctors can obtain the patient’s status through regular follow-up. These data were often temporary and fragmented, and could not truly reflect the patient’s postoperative status. The smart prosthesis persona IQ of Zimmer Biomet has passed FDA certification (Zimmerbiomet, 2021). Persona IQ can not only implant in patients like the traditional prosthesis, but also record the range, speed and other index of gait, for assessing postoperative recovery progress. Implant with intelligent sense will provide real recovery status for changing rehabilitation plans.
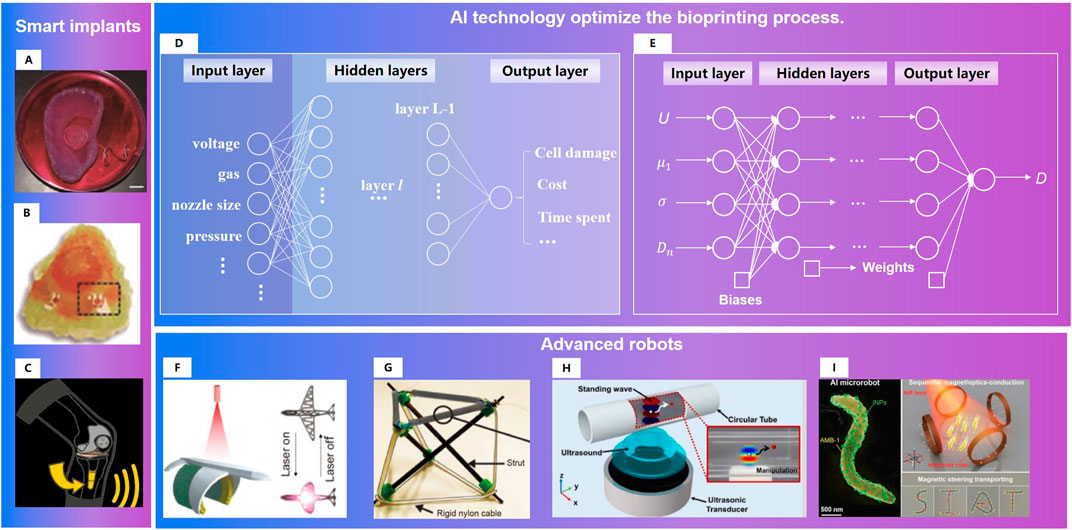
FIGURE 14. Research directions for satisfied repair of musculoskeletal injuries: 1) Smart implants. (A)A bionic ear (Mannoor et al., 2013). (B). An odor-perceptive nose-like hybrid (Jodat et al., 2020). (C). Smart prosthesis (Zimmerbiomet, 2021) 2)AI technology optimize the bioprinting process. (D). Example neural network for process optimization in three-dimensional bioprinting (Ruberu et al., 2021). (E). FCNNs for multi-objective optimization of drop-on-demand (DOD) bioprinting (Shi et al., 2019). 3)Advanced robots. (F). Tissue engineering robot with light activated deformable wing (Xu et al., 2019b). (G). Variable-stiffness tensegrity structures (Zappetti et al., 2020). (H). An ultrasound-controlled actuator for targeted drug delivery (Lee et al., 2020). (I). Acoustic powering and magnetic steering for actuating and navigating microrobots (Aghakhani et al., 2020).
3.2.2 Intelligent models 3D/4D printing process will optimize the manufacturing process
The performance of 3d/4d printed matter is not only related to its own physical, mechanical and biological properties, but also affected by the process parameters of the printing process (Naghieh et al., 2020). Study of Zheng W. et al. Zheng et al. (2021) showed the following facts: ① Higher pressures caused instability of extruded biomaterial, and subsequently, poor printability; ②Fell under or above the range of nozzle speeds caused poor strand printability; ③ The more viscous the biomaterial, the more appropriate printability could be achieved. Due to the complex conditions, it is hard to build an accurate mathematical printing model. Data driven AI technology (Shi et al., 2019; Yu and Jiang, 2020; Ruberu et al., 2021) has obvious advantages in the multi-objective optimization of biological printing process (Figure 14).
3.3 Advanced materials and variable structures will improve the treatment performance of robotics
As the physiological state of patients are different and changing (Ionov, 2018), such as temperature, pH (Ca2+ concentration), to achieve satisfied repair in long-term therapy, personalized surgical channels should be conducted (Xu et al., 2019b; Zappetti et al., 2020; Wang et al., 2022) or the endogenous repairs should be guided by the micro nano robots (Aghakhani et al., 2020; Lee et al., 2020). Combining the improvement of intraoperative operation skills and the providing signals for postoperative repair, the treatment effect can be improved through robots.
4 Conclusion
Satisfied recovery, a key goal of precise orthopaedics, has became possible, as AI technology in orthopaedics has progressed in the following apsects: physical parameters can be predicted precisely through AI technology, bioactive implants made through 3D printing technology can provide signals for endogenous repair, and personalized surgical channels can be established through intelligent robotic technology. However, the multi-layer intelligent technologies (MLIT) has not been used widely. With further study on biomechanical models for multi-physical parameter prediction, stimulus response mechanism of bioactive implants, smart implants, intelligent modeling of 3D/4D printing process and variable structure in long-term therapy (Senatov et al., 2016; Lin et al., 2019; Su et al., 2019), satisfied repair may be achieved by the multi-layer intelligent technologies (MLIT).
Author contributions
NG: Conceptualization, investigation, writing–review, and editing. JT: Investigation, review. LW: Investigation, writing and editing. KS: Investigation. LM: Methodology, Supervision. HM: Investigation and supervision. ZZ: Methodology, and supervision. FS: Supervision.
Funding
The study was funded by the National Natural Science Foundation of China Youth Fund (Grant No.62103224), and Special support from China Postdoctoral Foundation (Grant No.2020TQ0168).
Conflict of interest
The authors declare that the research was conducted in the absence of any commercial or financial relationships that could be construed as a potential conflict of interest.
Publisher’s note
All claims expressed in this article are solely those of the authors and do not necessarily represent those of their affiliated organizations, or those of the publisher, the editors and the reviewers. Any product that may be evaluated in this article, or claim that may be made by its manufacturer, is not guaranteed or endorsed by the publisher.
References
Abraham, N., and Khan, N. M. A Novel Focal Tversky loss function with improved Attention U-Net for lesion segmentation. Preprint, 2018.
Aghakhani, A., Yasa, O., Wrede, P., and Sitti, M. (2020). Acoustically powered surface-slipping mobile microrobots. Proc. Natl. Acad. Sci. U. S. A. 117 (7), 3469–3477. doi:10.1073/pnas.1920099117
Ahrend, M. D., Teunis, T., Noser, H., Schmidutz, F., Richards, G., Gueorguiev, B., et al. (2021). 3D computational anatomy of the scaphoid and its waist for use in fracture treatment. J. Orthop. Surg. Res. 16 (1), 216. doi:10.1186/s13018-021-02330-8
Alambeigi, F., Murphy, R. J., Basafa, E., Taylor, R. H., and Armand, M. (2014). Control of the coupled motion of a 6 DoF robotic arm and a continuum manipulator for the treatment of pelvis osteolysis. Annu. Int. Conf. IEEE Eng. Med. Biol. Soc. 36, 6521–6525. doi:10.1109/EMBC.2014.6945122
Alambeigi, F., Wang, Y., Murphy, R. J., Iordachita, I., and Armand, M. (2016). Toward robot-assisted hard osteolytic lesion treatment using a continuum manipulator. Annu. Int. Conf. IEEE Eng. Med. Biol. Soc. 2016, 5103–5106. doi:10.1109/EMBC.2016.7591875
Alambeigi, F., Sefati, S., Murphy, R. J., Iordachita, I., and Armand, M. Design and characterization of a debriding tool in robot-assisted treatment of osteolysis. Proceedings of the IEEE International Conference on Robotics and Automation (ICRA), Stockholm, Sweden, 2016, pp. 5664–5669. doi:10.1109/ICRA.2016.7487787
Antico, M., Sasazawa, F., Dunnhofer, M., Camps, S., Jaiprakash, A., Pandey, A., et al. (2020). Deep learning-based femoral cartilage automatic segmentation in ultrasound imaging for guidance in robotic knee arthroscopy. Ultrasound Med. Biol. 46 (2), 422–435. doi:10.1016/j.ultrasmedbio.2019.10.015
Bakhtiarinejad, M., Alambeigi, F., Chamani, A., Unberath, M., Khanuja, H., and Armand, M. “A biomechanical study on the use of curved drilling technique for treatment of osteonecrosis of femoral head,” in Proceedings of the Medical Image Computing and Computer-Assisted Intervention, 2020, Cham (Springer).
Balagopal, A., Kazemifar, S., Dan, N., Lin, M. H., Hannan, R., Owrangi, A., et al. (2018). Fully automated organ segmentation in male pelvic CT images. Phys. Med. Biol. 63 (24), 245015. doi:10.1088/1361-6560/aaf11c
Bao, M., Wang, X., Yuan, H., Lou, X., Zhao, Q., and Zhang, Y. (2016). HAp incorporated ultrafine polymeric fibers with shape memory effect for potential use in bone screw hole healing. J. Mat. Chem. B 4 (31), 5308–5320. doi:10.1039/C6TB01305h
Bell, K. L., Garrahan, N., Kneissel, M., Loveridge, N., Grau, E., Stanton, M., et al. (1996). Cortical and cancellous bone in the human femoral neck: Evaluation of an interactive image analysis system. Bone 19 (5), 541–548. doi:10.1016/S8756-3282(96)00245-1
Bernardo, I., and Edoardo, B. (2021). Robotics in orthopaedic surgery: Why, what and how? Archives Orthop. Trauma Surg. 141 (12), 2035–2042.
Bhandarkar, S., and Dhatrak, P. (2022). Optimization of a knee implant with different biomaterials using finite element analysis. Mater. Today Proc. 59 (1), 459–467. doi:10.1016/j.matpr.2021.11.466
Black, K. M., Law, H., Aldoukhi, A., Deng, J., and Ghani, K. R. (2020). Deep learning computer vision algorithm for detecting kidney stone composition. BJU Int. 125 (6), 920–924. doi:10.1111/bju.15035
Blendowski, M., Hansen, L., and Heinrich, M. P . (2021). Weakly-supervised learning of multi-modal features for regularised iterative descent in 3D image registration. Med. Image Anal. 67, 101822. doi:10.1016/j.media.2020.101822
Butscher, A., Bohner, M., Doebelin, N., Hofmann, S., and Müller, R. (2013). New depowdering-friendly designs for three-dimensional printing of calcium phosphate bone substitutes. Acta biomater. 9 (11), 9149–9158. doi:10.1016/j.actbio.2013.07.019
Cai, D., Wang, Z., and Liu, Y., Automatic path planning for navigated pedicle screw surgery based on deep neural network, Proceedings of the 2019 WRC Symposium on Advanced Robotics and Automation (WRC SARA). 2019.
Caprara, S., Fasser, M. R., and Spirig, J. M., (2021). Bone density optimized pedicle screw instrumentation improves screw pull-out force in lumbar vertebrae. Comput. Methods Biomechanics Biomed. Eng. 203, 1–11. doi:10.1080/10255842.2021.1959558
Caprara, S. (2021). Towards the integration of computational methods in spinal surgical planning: A combination of deep learning, statistical, and finite element methods. Switzerland: ETH Zurich Research Collection. doi:10.3929/ethz-b-000475269
Chang, Y. L., Lo, Y. J., Feng, S. W., Huang, Y. C., Tsai, H. Y., Lin, C. T., et al. (2016). Bone healing improvements using hyaluronic acid and hydroxyapatite/beta-tricalcium phosphate in combination: An animal study. Biomed Res. Int. 2016, 1–8. doi:10.1155/2016/8301624
Chen, F., Zhao, Z., Gao, C., Liu, J., Su, X., Zhao, J., et al. (2018). Clustering of morphological features for identifying femur cavity subtypes with difficulties of intramedullary nail implantation. IEEE J. Biomed. Health Inf. 22 (4), 1209–1217. doi:10.1109/JBHI.2017.2761980
Chen, W., Zhang, Y., He, J., Qiao, Y., Chen, Y., Shi, H., et al. W-net: Bridged U-net for 2D medical image segmentation. 2018, preprint.
Chen, J., Lu, Y., and Yu, Q., (2021). TransUNet: Transformers make strong encoders for medical image segmentation. Comput. Vis. Pattern Recognit. doi:10.48550/arXiv.2102.04306
Chen, Z., Wei, J., and Li, R., (2022). “Unsupervised multi-modal medical image registration via discriminator-free image-to-image translation,” in Proceedings of the International Joint Conference on Artificial Intelligence, Vienna, Austria.
Das, K., and Zhang, Q. . Convolutional recurrent residual U-net embedded with attention mechanism and focal Tversky loss function for cancerous nuclei detection. Preprint, 2020.
Deng, Z., Guo, Y., Zhao, X., Li, L., Dong, R., Guo, B., et al. (2016). Stretchable degradable and electroactive shape memory copolymers with tunable recovery temperature enhance myogenic differentiation. Acta Biomater. 46, 234–244. doi:10.1016/j.actbio.2016.09.019
Deng, H., Birdal, T., and Ilic, S. (2018). “Ppfnet: Global context aware local features for robust 3d point matching,” in Proceedings of the IEEE Conference on Computer Vision and Pattern Recognition, 18-23 June 2018, Salt Lake City, UT, USA, 195–205.
DePhillipo, N. N., Moatshe, G., Brady, A., Chahla, J., Aman, Z. S., Dornan, G. J., et al. (2018). Effect of meniscocapsular and meniscotibial lesions in ACL-deficient and ACL-reconstructed knees: A biomechanical study. Am. J. Sports Med. 46 (10), 2422–2431. doi:10.1177/0363546518774315
Dhivya, S., Ajita, J., and Selvamurugan, N. (2015). Metallic nanomaterials for bone tissue engineering. J. Biomed. Nanotechnol. 11 (10), 1675–1700. doi:10.1166/jbn.2015.2115
Ding, H., Miao, Q., Liu, H., Wang, H., Tu, Q., Chen, J., et al. (2017). Preoperative design and clinical application of TKA assisted by CAD combined with 3D printing navigation templates. Chin. Orthop. J. Clin. Basic Res. 9 (5), 261–268. doi:10.3969/j.issn.1674-666X.2017.05.001
Dong, Q., Huang, Z., and Sun, Y., Prediction of accuracy and screw size by pedicle anatomic parameters and screws in idiopathic scoliosis with freehand screw placement based on machine learning. Preprint, 2021.
Dong, Z., He, Y., and Qi, X., “MNet: Rethinking 2D/3D networks for anisotropic medical image segmentation,” in Proceedings of the International Joint Conference on Artificial Intelligence, Vienna, Austria, 10 May 2022.
Falk, T., Mai, D., Bensch, R., Çiçek, Ö., Abdulkadir, A., and Marrakchi, Y. (2019). U-net: Deep learning for cell counting, detection, and morphometry. Nat. Methods 16 (1), 67–70. doi:10.1038/s41592-018-0261-2
Fan, J., Cao, X., Wang, Q., Yap, P., and Shen, D. (2019). Adversarial learning for mono- or multi-modal registration. Med. image Anal. 58, 101545. doi:10.1016/j.media.2019.101545
Ghezelbash, F., Schmidt, H., Shirazi-Adl, A., and El-Rich, M. (2020). Internal load-sharing in the human passive lumbar spine: Review of in vitro and finite element model studies. J. Biomech. 102, 109441. doi:10.1016/j.jbiomech.2019.109441
Gilbert, H., Hendrick, R., Remirez, A., and Webster, R. (2014). A robot for transnasal surgery featuring needle-sized tentacle-like arms. Expert Rev. Med. devices 11 (1), 5–7. doi:10.1586/17434440.2013.854702
Gladman, A. S., Matsumoto, E. A., Nuzzo, R. G., Mahadevan, L., and Lewis, J. A. (2016). Biomimetic 4D printing. Nat. Mat. 15 (4), 413–418. doi:10.1038/nmat4544
Gojcic, Z., Zhou, C., Wegner, J. D., and Wieser, A. (2019). “The perfect match: 3d point cloud matching with smoothed densities,” in Proceedings of the IEEE Conference on Computer Vision and Pattern Recognition, Long Beach, CA, June 15–20, 2019, 5545–5554.
Gosline, A. H., Vasilyev, N. V., Veeramani, A., Wu, M., Schmitz, G., Chen, R., et al. (2012). Metal MEMS tools for beating-heart tissue removal. IEEE Int. Conf. Robot. Autom, Saint Paul, MN, May 14–18, 2012, 1921–1926. doi:10.1109/ICRA.2012.6225210
Graham, A. E., Xie, S. Q., Aw, K. C., Mukherjee, A. S., and Xu, W. L. (2008). Bone–muscle interaction of the fractured femur. J. Orthop. Res. 26 (8), 1159–1165. doi:10.1002/jor.20611
Groll, J., Burdick, J. A., Cho, D. W., Derby, B., Gelinsky, M., Heilshorn, S. C., et al. (2018). A definition of bioinks and their distinction from biomaterial inks. Biofabrication 11 (1), 013001. doi:10.1088/1758-5090/aaec52
Guo, N., Yang, B., Ji, X., Wang, Y., Hu, L., and Wang, T. (2019). Intensity-based 2D-3D registration for an ACL reconstruction navigation system. Int. J. Med. Robot. 15, e2008. doi:10.1002/rcs.2008
Han, L., Lyu, Y., and Peng, C., GAN-based disentanglement learning for chest X-ray rib suppression. Preprint, 2021.
Hatamizadeh, A., Nath, V., Tang, Y., Yang, D., Roth, H. R., and Xu, D. (2021). Swin UNETR: Swin transformers for semantic segmentation of brain tumors in MRI images. Brainlesion Glioma, Multiple Scler. Stroke Trauma. Brain Inj. BrainLes 2021, 272–284. doi:10.1007/978-3-031-08999-2_22
Hatamizadeh, A., Yang, D., and Roth, H., (2022). “Unetr: Transformers for 3D medical image segmentation,” in 2022 IEEE/CVF Winter Conference on Applications of Computer Vision (WACV), Waikoloa, HI, 15 February 2022. doi:10.1109/WACV51458.2022.00181
Hsieh, C. I., Zheng, K., Lin, C., Mei, L., Lu, L., Li, W., et al. (2021). Automated bone mineral density prediction and fracture risk assessment using plain radiographs via deep learning. Nat. Commun. 12, 5472. doi:10.1038/s41467-021-25779-x
Huang, Q., Sun, J., Hui, D., Wang, X., and Wang, G. (2018). Robust liver vessel extraction using 3D U-Net with variant dice loss function. Comput. Biol. Med. 101, 153–162. doi:10.1016/j.compbiomed.2018.08.018
Huang, X., Mei, G., and Zhang, J., A comprehensive survey on point cloud registration. Preprint, 2021.
Huang, B., Cao, B., Zhang, J., Feng, Y., Wang, L., Chen, X., et al. (2021). Induction of ferroptosis in human nasopharyngeal cancer cells by cucurbitacin B: Molecular mechanism and therapeutic potential. Cell Death Dis. 1, 237–267. doi:10.1038/s41419-021-03516-y
Hughes, A. J., DeBuitleir, C., Soden, P., O’Donnchadha, B., Tansey, A., Abdulkarim, A., et al. (2017). 3D printing aids acetabular reconstruction in complex revision hip arthroplasty. Adv. Orthop. 2017, 1–7. doi:10.1155/2017/8925050
Iglovikov, V., and Shvets, A. TernausNet: U-net with VGG11 encoder pre-trained on ImageNet for image segmentation, Preprint, 2018.
Ionov, L. (2018). 4D biofabrication: Materials, methods, and applications. Adv. Healthc. Mat. 7 (17), e1800412. doi:10.1002/adhm.201800412
Isensee, F., Jaeger, P. F., Kohl, S. A. A., Petersen, J., and Maier-Hein, K. (2021). nnU-Net: a self-configuring method for deep learning-based biomedical image segmentation. Nat. Methods 18 (2), 203–211. doi:10.1038/s41592-020-01008-z
Jamwal, P. K., Hussain, S., and Ghayesh, M. H. (2021). Intrinsically compliant parallel robot for fractured femur reduction: Mechanism optimization and control. Robotics Aut. Syst. 141, 103787. doi:10.1016/j.robot.2021.103787
Jiang, J., Cetin, E., and Celiktutan, O. (2021). “IB-DRR: Incremental learning with information-back discrete representation replay,” in Proceedings of the 2021 IEEE/CVF Conference on Computer Vision and Pattern Recognition Workshops (CVPRW), Nashville, TN, USA, 19-25 June 2021.
Jin, Q., Meng, Z., Sun, C., and Leyi, W. (2018). Ran SRA-UNet: A hybrid deep attention-aware network to extract liver and tumor in CT scans. J. latex Cl. files octber. doi:10.3389/fbioe.2020.605132
Jodat, Y. A., Kiaee, K., Jarquin, D. V., Hernández, R., Wang, T., Joshi, S., et al. (2020). A 3D-printed hybrid nasal cartilage with functional electronic olfaction. Adv. Sci. (Weinh). 7 (5), 1901878. doi:10.1002/advs.201901878
Jordi, M., Anne, E., Maureen, v. E., Ruben, P., Tymour, F., Kees, J. B., et al. (2022). A review on the application of deep learning for CT reconstruction, bone segmentation and surgical planning in oral and maxillofacial surgery. Dentomaxillofacial Radiol. 51, 20210437. doi:10.1259/dmfr.20210437
Kam, J., Gan, C., Dimou, S., Awad, M., Kavar, B., Nair, G., et al. (2019). Learning curve for robot-assisted percutaneous pedicle screw placement in thoracolumbar surgery. Asian Spine J. 13 (6), 920–927. doi:10.31616/asj.2019.0033
Kang, H. W., Lee, S. J., Ko, I. K., Kengla, C., Yoo, J. J., and Atala, A. (2016). A 3D bioprinting system to produce human-scale tissue constructs with structural integrity. Nat. Biotechnol. 34 (3), 312–319. doi:10.1038/nbt.3413
Kawai, T., Shanjani, Y., Fazeli, S., Behn, A. W., Okuzu, Y., Goodman, S. B., et al. (2018). Customized, degradable, functionally graded scaffold for potential treatment of early stage osteonecrosis of the femoral head. J. Orthop. Res. 36 (3), 1002–1011. doi:10.1002/jor.23673
Kesner, S. B., and Howe, R. D. (2011). “Force control of flexible catheter robots for beating heart surgery,” IEEE Int Conf Robot Autom., 2011, 1589–1594. doi:10.1109/ICRA.2011.5979690
Kolařík, M., Burget, R., Uher, V., Říha, K., and Dutta, M. K. (2019). Optimized high resolution 3D dense-U-net network for brain and spine segmentation. Appl. Sci. (Basel). 9, 404. doi:10.3390/app9030404
Kolesky, D. B., Truby, R. L., Gladman, A. S., Busbee, T. A., Homan, K. A., and Lewis, J. A. (2014). 3D bioprinting of vascularized, heterogeneous cell-laden tissue constructs. Adv. Mat. 26 (19), 3124–3130. doi:10.1002/adma.201305506
Kramer, J., Scheurecker, G., Scheurecker, A., et al. (2009). Femoral head necrosis. Dordrecht, Netherlands: Springer Netherlands.
Lampen, N., Kim, D., Fang, X., Xu, X., Kuang, T., Deng, H. H., et al. (2022). Deep learning for biomechanical modeling of facial tissue deformation in orthognathic surgical planning. Int. J. Comput. Assist. Radiol. Surg. 17, 945–952. doi:10.1007/s11548-022-02596-1
Lee, H. S., Go, G., Choi, E., Kang, B., Park, J. O., and Kim, C. S. (2020). Medical microrobot - wireless manipulation of a drug delivery carrier through an external ultrasonic actuation: Preliminary results. Int. J. Control Autom. Syst. 18 (1), 175–185. doi:10.1007/s12555-019-0239-6
Le-Huu, D. K., and Paragios, N. (2017). “Alternating direction graph matching,” in Proceedings of the 2017 IEEE Conference on Computer Vision and Pattern Recognition (CVPR), Hawaii, United States, July 2017, (IEEE), 4914–4922. doi:10.1109/CVPR.2017.522
Lei, J., Zheng, G., and Hu, L., (2019). “Force/position control simulation of robot-assisted fracture reduction,” in Proceedings of the 2019 WRC Symposium on Advanced Robotics and Automation (WRC SARA) (IEEE), 21-22 August 2019, Beiging, China.
Levine, S., Finn, C., Darrell, T., and Abbeel, P. (2016). End-to-End training of deep visuomotor policies. J. Mach. Learn. Res. 17 (1), 1334–1373. doi:10.1016/j.dam.2015.09.011
Li, X., Hao, C., Qi, X., Qi, D., Fu, C. W., and HengH-DenseUNet, P. A. (2017). H-DenseUNet: Hybrid densely connected UNet for liver and tumor segmentation from CT volumes. IEEE Trans. Med. Imaging 37 (12), 2663–2674. doi:10.1109/TMI.2018.2845918
Li, J., Dunstan, C. R., Entezari, A., Li, Q., Steck, R., Saifzadeh, S., et al. (2019). A novel bone substitute with high bioactivity, strength, and porosity for repairing large and load-bearing bone defects. Adv. Healthc. Mat. 8 (8), 1801298. doi:10.1002/adhm.201801298
Li, Y., Pavanram, P., Zhou, J., Lietaert, K., Taheri, P., Li, W., et al. (2020). Additively manufactured biodegradable porous zinc. Acta Biomater. 101, 609–623. doi:10.1016/j.actbio.2019.10.034
Liang, P., Chen, J., and Zheng, H., (2019). “Cascade decoder: A universal decoding method for biomedical image segmentation,” in Proceedings of the 2019 IEEE 16th International Symposium on Biomedical Imaging (ISBI 2019), Venice, Italy, April 8–11, 2019 (IEEE), pp. 339–342. doi:10.1109/ISBI.2019.8759430
Lin, C., Lv, J., Li, Y., Zhang, F., and Liu, Y., (2019). 4D-Printed biodegradable and remotely controllable shape memory occlusion devices. Adv. Funct. Mat. 29 (51), 1906569. doi:10.1002/adfm.201906569
Liu, Y., Wang, R., Chen, S., Xu, Z., Wang, Q., Yuan, P., et al. (2020). Heparan sulfate loaded polycaprolactone-hydroxyapatite scaffolds with 3D printing for bone defect repair. Int. J. Biol. Macromol. 148, 153–162. doi:10.1016/j.ijbiomac.2020.01.109
Liu, B., Sun, C., Hou, G., Yang, Z., Liu, Z., Tian, G., et al. (2021). Clinical application of 3D printing technology: Bone defect reconstruction of limbs based on ‘precision orthopaedics’. Chin. J. Minim. Invasive Surg. 21 (4), 303–308. doi:10.3969/j.issn.1009-6604.2021.04.004
Loebel, C., and Burdick, J. A. (2018). Engineering stem and stromal cell therapies for musculoskeletal tissue repair. Cell Stem Cell 22 (3), 325–339. doi:10.1016/j.stem.2018.01.014
Lopez, C., Gazgalis, A., Boddapati, V., Shah, R. P., Cooper, H. J., and Geller, J. A. (2021). Artificial learning and machine learning decision guidance applications in total hip and knee arthroplasty: A systematic review. Arthroplast. Today 11, 103–112. doi:10.1016/j.artd.2021.07.012
Lopez, F., Varela, A., Hinojosa, O., Mendez, M., Trinh, D. H., ElBeze, Y., et al. Assessing deep learning methods for the identification of kidney stones in endoscopic images. Proceedings of the 2021 43rd Annual International Conference of the IEEE Engineering in Medicine & Biology Society (EMBC), Mexico, 2021: 2778–2781. doi:10.1109/EMBC46164.2021.9630211
Lu, J., Ofverstedt, J., Lindblad, J., and Sladoje, N. Is image-to-image translation the panacea for multimodal image registration? A comparative study. 2021, ArXiv, 2021 abs/2103.16262.
Luo, Y., Zhai, D., Huan, Z., Zhu, H., Xia, L., Chang, J., et al. (2015). Three-dimensional printing of hollow-struts-packed bioceramic scaffolds for bone regeneration. ACS Appl. Mat. Interfaces 7 (43), 24377–24383. doi:10.1021/acsami.5b08911
Mahler, J., Matl, M., Satish, V., Danielczuk, M., DeRose, B., McKinley, S., et al. (2019). Learning ambidextrous robot grasping policies. Sci. Robot. 4 (26), eaau4984. doi:10.1126/scirobotics.aau4984
Makvandi, P., Wang, C., Zare, E. N., Borzacchiello, A., Niu, L., and Tay, F. R. (2020). Metal-based nanomaterials in biomedical applications: Antimicrobial activity and cytotoxicity aspects. Adv. Funct. Mat. 30 (22), 1910021. doi:10.1002/adfm.201910021
Mandlekar, A., Zhu, Y., and Garg, A., Robo Turk: A crowdsourcing platform for robotic skill learning through imitation. Proceedings of the International Conference on Robot Learning, Zürich, Switzerland, 2018:1–15.
Mannoor, M. S., Jiang, Z., James, T., Kong, Y., Malatesta, K. A., Soboyejo, W. O., et al. (2013). 3D printed bionic ears. Nano Lett. 13 (6), 2634–2639. doi:10.1021/nl4007744
Marban, A., Srinivasan, V., and Samek, W., (2018). “Estimation of interaction forces in robotic surgery using a semi-supervised deep neural network model,” in Proceedings of the 2018 IEEE/RSJ International Conference on Intelligent Robots and Systems (IROS) (IEEE), 01-05 Oct. 2018, Madrid, Spain.
Mistry, S., Kundu, D., Datta, S., and Basu, D. (2015). Comparison of bioactive glass coated and hydroxyapatite coated titanium dental implants in the human jaw bone. Aust. Dent. J. 56 (1), 68–75. doi:10.1111/j.1834-7819.2010.01305.x
Molnar, D., Enqvist, O., Ulén, J., Larsson, M., Brandberg, J., Johnsson, A. A., et al. (2021). Artificial intelligence based automatic quantification of epicardial adipose tissue suitable for large scale population studies. Sci. Rep. 11 (1), 23905. doi:10.1038/s41598-021-03150-w
Naghieh, S., Sarker, M. D., Sharma, N. K., Barhoumi, Z., and Chen, X. (2020). Printability of 3D printed hydrogel scaffolds: Influence of hydrogel composition and printing parameters. Appl. Sci. (Basel). 10 (1), 292. doi:10.3390/app10010292
Neumann, D. A. (2010). Kinesiology of the musculoskeletal system. Maryland Heights, Missouri, United States: Mosby, Elsevier.
Nicolas, N., Emilie, C. S., Vincent, C., Benoit, B., Matthieu, V., and Julien, B. (2016). Robot-assisted spine surgery: Feasibility study through a prospective case-matched analysis. Eur. Spine J. 25 (3), 947–955. doi:10.1007/s00586-015-3758-8
Pei, G. X., and Yan, Y. B. (2014). Current status and progress of digital orthopaedics in China. J. Orthop. Transl. 2 (3), 107–117. doi:10.1016/j.jot.2014.05.001
Polce, E. M., Kunze, K. N., and Paul, K. M. (2021). Machine learning predicts femoral and tibial implant size mismatch for total knee arthroplasty. Arthroplasty Today 8 (11), 268–277. doi:10.1016/j.artd.2021.01.006
Qi, G., Dunn, C. K., Qi, H. J., and Dunn, M. L. (2014). Active origami by 4D printing. Smart Mater. Struct. 23 (9), 094007. doi:10.1088/0964-1726/23/9/094007
Qi, C. R., Su, H., Mo, K., and Guibas, L. J. (2017). “Pointnet: Deep learning on point sets for 3d classification and segmentation,” in Proceedings of the IEEE Conference on Computer Vision and Pattern Recognition, Hawaii, United States, July 2017, pp. 77–85. doi:10.1109/CVPR.2017.16
Raviv, D., Zhao, W., Mcknelly, C., Papadopoulou, A., Kadambi, A., Shi, B., et al. (2014). Active printed materials for complex self-evolving DeformationsActive printed materials for complex self-evolving deformations. Sci. Rep. 4, 7422. doi:10.1038/srep07422
Ren, X., Tu, V., Bischoff, D., Weisgerber, D. W., Lewis, M. S., Yamaguchi, D. T., et al. (2016). Nanoparticulate mineralized collagen scaffolds induce in vivo bone regeneration independent of progenitor cell loading or exogenous growth factor stimulation. Biomaterials 89, 67–78. doi:10.1016/j.biomaterials.2016.02.020
Rezwan, K., Chen, Q. Z., Blaker, J. J., and Boccaccini, A. R. (2006). Biodegradable and bioactive porous polymer/inorganic composite scaffolds for bone tissue engineering. Biomaterials 27 (18), 3413–3431. doi:10.1016/j.biomaterials.2006.01.039
Rogers, M. A., and Aikawa, E. (2019). Cardiovascular calcification: Artificial intelligence and big data accelerate mechanistic discovery. Nat. Rev. Cardiol. 16 (5), 261–274. doi:10.1038/s41569-018-0123-8
Roohani-Esfahani, S. I., Lu, Z. F., Li, J. J., Ellis-Behnke, R., Kaplan, D. L., and Zreiqat, H. (2012). Effect of self-assembled nanofibrous silk/polycaprolactone layer on the osteoconductivity and mechanical properties of biphasic calcium phosphate scaffolds. Acta biomater. 8 (1), 302–312. doi:10.1016/j.actbio.2011.10.009
Ruberu, K., Senadeera, M., Rana, S., Gupta, S., Chung, J., Yue, Z., et al. (2021). Coupling machine learning with 3D bioprinting to fast track optimisation of extrusion printing. Appl. Mater. Today 22, 100914. doi:10.1016/j.apmt.2020.100914
Salehi, S. S. M., Erdogmus, D., and Gholipour, A. “Tversky loss function for image segmentation using 3D fully convolutional deep networks,” in Proceedings of the Machine Learning in Medical Imaging, Quebec City, QC, September 2017 (Springer). doi:10.1007/978-3-319-67389-9_44
Sanchez-Romero, R., Ramsey, J. D., and Zhang, K., Causal discovery of feedback networks with functional magnetic resonance imaging, Preprint, 2018.
Senatov, F. S., Niaza, K. V., Zadorozhnyy, M. Y., Maksimkin, A. V., Kaloshkin, S. D., and Estrin, Y. Z., (2016). Mechanical properties and shape memory effect of 3D-printed PLA-based porous scaffolds. J. Mech. Behav. Biomed. Mater. 57, 139–148. doi:10.1016/j.jmbbm.2015.11.036
Shademan, A., Decker, R. S., Opfermann, J. D., Leonard, S., Krieger, A., and Kim, P. C. W. (2016). Supervised autonomous robotic soft tissue surgery. Sci. Transl. Med. 8 (337), 337ra64. 337ra64-337ra64. doi:10.1126/scitranslmed.aad9398
Shi, J., Song, J., Song, B., and Lu, W. (2019). Multi-objective optimization design through machine learning for drop-on-demand bioprinting. Engineering 5 (3), 586–593. doi:10.1016/j.eng.2018.12.009
Siebenrock, K. A., Powell, J. N., and Ganz, R. (2010). Osteochondritis dissecans of the femoral head. HIP Int. 20 (4), 489–496. doi:10.1177/112070001002000412
Siemionow, K. B., Forsthoefel, C. W., Foy, M. P., Gawel, D., and Luciano, C. J. (2021). Autonomous lumbar spine pedicle screw planning using machine learning: A validation study. J. Craniovertebral Junction Spine 12 (3), 223–227.
Silver, D., Huang, A., Maddison, C. J., Guez, A., Sifre, L., van den Driessche, G., et al. (2016). Mastering the game of Go with deep neural networks and tree search. Nature 529 (7587), 484–489. doi:10.1038/nature16961
Soodmand, E., Zheng, G., Steens, W., Bader, R., Nolte, L., and Kluess, D. (2019). Surgically relevant morphological parameters of proximal human femur: A statistical analysis based on 3D reconstruction of CT data. Orthop. Surg. 11, 135–142. doi:10.1111/os.12416
Su, J. W., Gao, W., Trinh, K., Kendere, S. M., Pulatsu, E. T., Zhang, C., et al. (2019). 4D printing of polyurethane paint-based composites. Int. J. Smart Nano Mater. (29), 1–12. doi:10.1080/19475411.2019.1618409
Sun, F., Liu, C., and Huang, W., (2017). Object classification and grasp planning using visual and tactile sensing. IEEE Trans. Syst. Man Cybern. Syst. 46 (7), 969–979. doi:10.1109/TSMC.2016.2524059
Thananjeyan, B., Garg, A., and Krishnan, S., (2017). “Multilateral surgical pattern cutting in 2D orthotropic gauze with deep reinforcement learning policies for tensioning,” in Proceedings of the 2017 IEEE International Conference on Robotics and Automation (ICRA) (IEEE). 29 May 2017-03 June 2017, Singapore.
Thitiset, T., Damrongsakkul, S., Bunaprasert, T., Leeanansaksiri, W., and Honsawek, S. (2013). Development of collagen/demineralized bone powder scaffolds and periosteum-derived cells for bone tissue engineering application. Int. J. Mol. Sci. 14 (1), 2056–2071. doi:10.3390/ijms14012056
Tian, W., Liu, Y., Liu, B., He, D., Wu, J., Han, X., et al. (2019). Guideline for thoracolumbar pedicle screw placement assisted by orthopaedic surgical robot. Orthop. Surg. 11 (2), 153–159. doi:10.1111/os.12453
Tibbits, S. (2014). 4D printing: Multi-material shape change. Archit. Des. 84 (1), 116–121. doi:10.1002/ad.1710
Turnbull, G., Clarke, J., Picard, F., Riches, P., Jia, L., Han, F., et al. (2018). 3D bioactive composite scaffolds for bone tissue engineering. Bioact. Mater. 3 (3), 278–314. doi:10.1016/j.bioactmat.2017.10.001
Unberath, M., Zaech, A.-N., and Lee, S. C., (2018). “DeepDRR – a catalyst for machine learning in fluoroscopy-guided procedures,” in Medical image computing and computer assisted intervention – MICCAI 2018. MICCAI 2018. Lecture notes in computer science. Editors A. Frangi, J. Schnabel, C. Davatzikos, C. Alberola-López, and G. Fichtinger (Cham: Springer), 11073. doi:10.1007/978-3-030-00937-3_12
Unnithan, A. R., Sasikala, A. R. K., Park, C. H., and Kim, C. S. (2017). A unique scaffold for bone tissue engineering: An osteogenic combination of graphene oxide–hyaluronic acid–chitosan with simvastatin. J. Industrial Eng. Chem. 46, 182–191. doi:10.1016/j.jiec.2016.10.029
Van Belleghem, S., Torres, L., Santoro, M., Mahadik, B., Wolfand, A., Kofinas, P., et al. (2020). Hybrid 3D printing of synthetic and cell‐laden bioinks for shape retaining soft tissue grafts. Adv. Funct. Mat. 30 (3), 1907145. doi:10.1002/adfm.201907145
Vijayan, R., Silva, T. D., Han, R., Zhang, X., Uneri, A., Doerr, S., et al. (2019). Automatic pedicle screw planning using atlas-based registration of anatomy and reference trajectories. Phys. Med. Biol. 64 (16), 165020. doi:10.1088/1361-6560/ab2d66
Villa, M. M., Wang, L., Huang, J., Rowe, D. W., and Wei, M. (2015). Bone tissue engineering with a collagen-hydroxyapatite scaffold and culture expanded bone marrow stromal cells. J. Biomed. Mat. Res. 103 (2), 243–253. doi:10.1002/jbm.b.33225
Viris, A., Stefanoudakis, G., Petousis, M., Vidakis, N., Tsainis, A. M., and Kandyla, B. (2016). Evaluation of an intact, an ACL-deficient, and a reconstructed human knee joint finite element model. Comput. Methods Biomech. Biomed. Engin. 19 (3), 263–270. doi:10.1080/10255842.2015.1015526
Wang, Y., and Solomon, J. M. . Deep closest point: Learning representations for point cloud registration. Preprint, 2019.
Wang, T., Wang, L., and Tang, P., (2018). Automatic bone segmentation and ultrasound—CT registration for robotic assisted femoral shaft fracture reduction. J. Med. Imaging Health Inf. 8 (1), 151–156(6). doi:10.1166/jmihi.2018.2249
Wang, J., Liu, H., Ke, J., Hu, L., Zhang, S., Yang, B., et al. (2020). Image-guided cochlear access by non-invasive registration: A cadaveric feasibility study. Sci. Rep. 10, 18318. doi:10.1038/s41598-020-75530-7
Wang, S., Xie, Z., Yuan, F., Li, L., Liu, Y., Wang, T., et al. (2022). Bio-inspired physical intelligence for soft robotics. Chin. Sci. Bull. 67 (10), 959–975. doi:10.1360/TB-2021-1217
Watanabe, H., Kanou, K., Kobayashi, Y., and Fujie, M. G. (2011). “Development of a “steerable drill” for ACL reconstruction to create the arbitrary trajectory of a bone tunnel[C],” in IEEE/RSJ International Conference on Intelligent Robots and Systems, IROS 2011, San Francisco, CA, September 25–30, 2011 (IEEE).
Wells, T. S., Yang, S., Maclachlan, R. A., Lobes, L. A., Martel, J. N., and Riviere, C. N. (2016). Hybrid position/force control of an active handheld micromanipulator for membrane peeling. Int. J. Med. Robot. Comput. Assist. Surg. 12 (1), 85–95. doi:10.1002/rcs.1659
Wilkening, P., Alambeigi, F., Murphy, R. J., Taylor, R. H., and Armand, M. (2017). Development and experimental evaluation of concurrent control of a robotic arm and continuum manipulator for osteolytic lesion treatment. IEEE Robot. Autom. Lett. 2 (3), 1625–1631. doi:10.1109/LRA.2017.2678543
Wu, H., Lei, P., Liu, G., Zhang, Y. S., Yang, J., Zhang, L., et al. (2017). Reconstruction of large-scale defects with a novel hybrid scaffold made from poly(L-lactic acid)/Nanohydroxyapatite/Alendronate-loaded chitosan microsphere: In vitro and in vivo studies. Sci. Rep. 7 (1), 359. doi:10.1038/s41598-017-00506-z
Wubneh, A., Tsekoura, E. K., Ayranci, C., and Uludağ, H. (2018). Current state of fabrication technologies and materials for bone tissue engineering. Acta Biomater. 80, 1–30. doi:10.1016/j.actbio.2018.09.031
Xiao, D., Lian, C., Deng, H., Kuang, T., Liu, Q., Ma, L., et al. (2021). Estimating reference Bony shape models for orthognathic surgical planning using 3D point-cloud deep learning. IEEE J. Biomed. Health Inf. 25 (8), 2958–2966. Epub 2021 Aug 5. PMID: 33497345; PMCID: PMC8310896. doi:10.1109/JBHI.2021.3054494
Xie, R., Hu, J., Ng, F., Tan, L., Qin, T., Zhang, M., et al. (2017). High performance shape memory foams with isocyanate-modified hydroxyapatite nanoparticles for minimally invasive bone regeneration. Ceram. Int. 43 (6), 4794–4802. doi:10.1016/j.ceramint.2016.11.216
Xie, R., Hu, J., Hoffmann, O., Zhang, Y., Ng, F., Qin, T., et al. (2018). Self-fitting shape memory polymer foam inducing bone regeneration: A rabbit femoral defect study. Biochimica Biophysica Acta - General Subj. 1862 (4), 936–945. doi:10.1016/j.bbagen.2018.01.013
Xu, T., Binder, K. W., Albanna, M. Z., Dice, D., Zhao, W., Yoo, J. J., et al. (2013). Hybrid printing of mechanically and biologically improved constructs for cartilage tissue engineering applications. Biofabrication 5 (1), 015001. doi:10.1088/1758-5082/5/1/015001
Xu, X., Tao, J., Wang, S., Yang, L., Zhang, J., Zhang, J., et al. (2019). 3D printing of nerve conduits with nanoparticle-encapsulated RGFP966. Appl. Mater. Today 16, 247–256. doi:10.1016/j.apmt.2019.05.014
Xu, B., Han, X., Hu, Y., Lou, Y., Chen, C., Chen, Z., et al. (2019). A remotely controlled transformable soft robot based on engineered cardiac tissue construct. Small 15 (18), e1900006. doi:10.1002/smll.201900006
Yan, K., Cai, J., Jin, D., Miao, S., Guo, D., Harrison, A. P., et al. (2022). Sam: Self-Supervised learning of pixel-wise anatomical embeddings in radiological images. IEEE Trans. Med. Imaging, 1. doi:10.1109/TMI.2022.3169003
Yasaka, K., Akai, H., Kunimatsu, A., Kiryu, S., and Abe, O. (2020). Prediction of bone mineral density from computed tomography: Application of deep learning with a convolutional neural network. Eur. Radiol. 30 (6), 3549–3557. PMID: 32060712. doi:10.1007/s00330-020-06677-0
Ye, R., and Chen, Y. (2009). “Path planning for robot assisted femur shaft fracture reduction: A preliminary investigation,” in Proceedings of the 2009 IEEE International Conference on Virtual Environments, Human-Computer Interfaces and Measurements Systems (IEEE), 11-13 May 2009, Hong Kong, China, 113–117. doi:10.1109/VECIMS.2009.5068876
Yu, C., and Jiang, J. (2020). A perspective on using machine learning in 3D bioprinting. Int. J. Bioprint. 6 (1), 253. doi:10.18063/ijb.v6i1.253
Yuan, W., Eckart, B., Kim, K., Jampani, V., Fox, D., and Kautz, J. (2020). “DeepGMR: Learning Latent Gaussian Mixture Models for Registration,” in Computer Vision – ECCV 2020. ECCV 2020. Lecture Notes in Computer Science, October 29, 2020. Editors A. Vedaldi, H. Bischof, T. Brox, and J. M. Frahm (Springer, Cham) vol 12350. doi:10.1007/978-3-030-58558-7_43
Zappetti, D., Jeong, S. H., Shintake, J., and Floreano, D. (2020). Phase changing materials-based variable-stiffness tensegrity structures. Soft Robot. 7 (3), 362–369. doi:10.1089/soro.2019.0091
Zeng, G., Yang, X., and Li, J., (2017). “3D U-net with multi-level deep supervision: Fully automatic segmentation of proximal femur in 3D MR images,” in Proceedings of the International Workshop on Machine Learning in Medical Imaging, Cham (Springer).
Zhang, D., Burkes, W. L., Schoener, C. A., and Grunlan, M. A. (2012). Porous inorganic–organic shape memory polymers. Polymer 53 (14), 2935–2941. doi:10.1016/j.polymer.2012.04.053
Zhang, D., George, O. J., Petersen, K. M., Jimenez-Vergara, A. C., Hahn, M. S., and Grunlan, M. A. (2014). A bioactive “self-fitting” shape memory polymer scaffold with potential to treat cranio-maxillo facial bone defects. Acta biomater. 10 (11), 4597–4605. doi:10.1016/j.actbio.2014.07.020
Zhang, Y. S., Andrea, A., Simone, B., Su-Ryon, S., Kai, Z., Zahra, G. M., et al. (2016). Bioprinting 3D microfibrous scaffolds for engineering endothelialized myocardium and heart-on-a-chip. Biomaterials 110, 45–59. doi:10.1016/j.biomaterials.2016.09.003
Zhang, Z., Ma, Z., Zhang, Y., Chen, F., Zhou, Y., and An, Q. (2018). Dehydrothermally crosslinked collagen/hydroxyapatite composite for enhanced in vivo bone repair. Colloids Surfaces B Biointerfaces 163, 394–401. doi:10.1016/j.colsurfb.2018.01.011
Zhang, R., Wu, S., Wu, W., Gao, H., and Zhou, Z. (2019). Computer-assisted needle trajectory planning and mathematical modeling for liver tumor thermal ablation: A review. Math. Biosci. Eng. 16 (5), 4846–4872. PMID: 31499693. doi:10.3934/mbe.2019244
Zhang, Y. (2018). Springer, Singapore. Jointly published with People’s Medical Publishing House, Beijing, China (eBook). doi:10.1007/978-981-10-6044-1
Zheng, G., and Yu, W. (2017). Statistical shape and deformation models based 2D–3D reconstruction. Stat. Shape Deformation Analysis, 329–349. doi:10.1016/b978-0-12-810493-4.00015-8
Zheng, G., Tian, W., and Zhuang, X. (2018). “Biomechanical optimization-based planning of periacetabular osteotomy: Artificial intelligence and smart image-guided technology for orthopaedics,” in Intelligent orthopaedics 1093, 157–168. doi:10.1007/978-981-13-1396-7_13
Zheng, W., Wang, Y., Zhang, F., Li, C., Liu, Y., and Leng, J. (2018). Development of shape memory polymers micro/nanofiber membranes in biomedical applications. Sci. Sin. -Tech. 48 (8), 811–826. doi:10.1360/n092018-00126
Zheng, W., Dong, X., He, Y., Liu, Y., and Leng, J. (2021). Development of bioactive polymers and their composite materials in bone tissue engineering. J. Harbin Inst. Technol. 53 (8), 1. doi:10.11918/202007079
Zhou, X. Y., Guo, Y., Shen, M., and Yang, G. Z. (2020). Application of artificial intelligence in surgery. Front. Med. 14 (4), 417–430. doi:10.1007/s11684-020-0770-0
Zhou, Z., Siddiquee, M., Tajbakhsh, N., and Liang, J. (2020). UNet++: Redesigning skip connections to exploit multiscale features in image segmentation. IEEE Trans. Med. Imaging 39 (6), 1856–1867. doi:10.1109/tmi.2019.2959609
Zimmerbiomet The Smart Knee® by combining the power of Persona® the Personalized Knee® with CANARY caturio te with CHIRP system, Persona IQ is smart, connected and simple. Available at: https://www.zimmerbiomet.com/en/products-and-solutions/specialties/knee/persona-iq.html [Accessed August 20,2021]
Keywords: smart materials, variable structures, intelligent therapeutic planning, best recover of musculoskeletal, multi-layer intelligent technologies
Citation: Guo N, Tian J, Wang L, Sun K, Mi L, Ming H, Zhe Z and Sun F (2022) Discussion on the possibility of multi-layer intelligent technologies to achieve the best recover of musculoskeletal injuries: Smart materials, variable structures, and intelligent therapeutic planning. Front. Bioeng. Biotechnol. 10:1016598. doi: 10.3389/fbioe.2022.1016598
Received: 11 August 2022; Accepted: 14 September 2022;
Published: 30 September 2022.
Edited by:
Yansong Qi, Inner Mongolia People’s Hospital, ChinaReviewed by:
Qichang Mei, Ningbo University, ChinaLong Cui, Chinese Academy of Sciences (CAS), China
Liyuan Tao, Peking University Third Hospital, China
Copyright © 2022 Guo, Tian, Wang, Sun, Mi, Ming, Zhe and Sun. This is an open-access article distributed under the terms of the Creative Commons Attribution License (CC BY). The use, distribution or reproduction in other forums is permitted, provided the original author(s) and the copyright owner(s) are credited and that the original publication in this journal is cited, in accordance with accepted academic practice. No use, distribution or reproduction is permitted which does not comply with these terms.
*Correspondence: Fuchun Sun, ZmNzdW5AbWFpbC50c2luZ2h1YS5lZHUuY24=