- Department of Biosystems Science and Engineering (D-BSSE), ETH Zürich, Basel, Switzerland
Harnessing the potential of optogenetics in biology requires methodologies from different disciplines ranging from biology, to mechatronics engineering, to control engineering. Light stimulation of a synthetic optogenetic construct in a given biological species can only be achieved via a suitable light stimulation platform. Emerging optogenetic applications entail a consistent, reproducible, and regulated delivery of light adapted to the application requirement. In this review, we explore the evolution of light-induction hardware-software platforms from simple illumination set-ups to sophisticated microscopy, microtiter plate and bioreactor designs, and discuss their respective advantages and disadvantages. Here, we examine design approaches followed in performing optogenetic experiments spanning different cell types and culture volumes, with induction capabilities ranging from single cell stimulation to entire cell culture illumination. The development of automated measurement and stimulation schemes on these platforms has enabled researchers to implement various in silico feedback control strategies to achieve computer-controlled living systems—a theme we briefly discuss in the last part of this review.
1 Introduction
Recent progress in synthetic biology has established light as a leading tool for observation as well as stimulation of synthetic constructs in cellular contexts (MacDonald and Deans, 2016; Toettcher et al., 2011a; Deisseroth, 2011; Müller et al., 2015; Krueger et al., 2019; Baumschlager and Khammash, 2021; Gheorghiu et al., 2021; Forlani and Di Ventura, 2021; Pérez et al., 2021). Light can be precisely localized in space and instantaneously turned on and off. Achieving this degree of spatio-temporal control establishes light as an exceptionally attractive alternative to other existing stimulation techniques such as chemical induction (Fracassi et al., 2016). Inspired by photosensitive proteins found in nature, synthetic biologists have engineered phototosensors and photoreceptors into living cells to control cellular processes such as gene expression (Müller et al., 2015) (Figure 1) and protein-protein interaction (Toettcher et al., 2011b). This use of light to influence cellular processes is commonly referred to as optogenetics (Toettcher et al., 2011a; Deisseroth, 2011). Several optogenetic tools, pertaining to different cell types and spanning different wavelengths of light, have been developed and studied in the last decade (Tischer and Weiner, 2014; Müller et al., 2015; Repina et al., 2017; Kolar et al., 2018; Baumschlager and Khammash, 2021; Gheorghiu et al., 2021; Forlani and Di Ventura, 2021; Pérez et al., 2021). Applications of these optogenetic tools have been expanding rapidly in various disciplines, such as developmental biology (Johnson and Toettcher, 2018; Krueger et al., 2019).
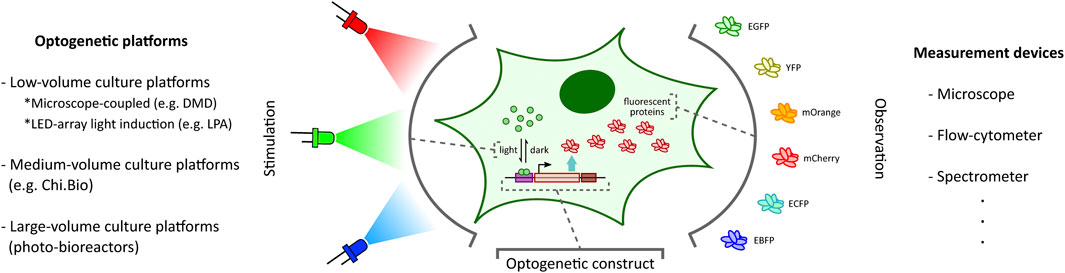
FIGURE 1. Optogenetic stimulation and target output observation in cellular context. Optogenetic studies involve placing a desired genetic construct under a light-activated tool (Kolar et al., 2018) (e.g. light-inducible transcription factors (Benzinger and Khammash, 2018) illustrated here) with an observable target output (e.g. fluorescent proteins (Rodriguez et al., 2017) illustrated here). Depending on the application requirements, one can choose from a plethora of optogenetic platforms (left: DMD-based platform (Rullan et al., 2018), LPA (Gerhardt et al., 2016), Chi.Bio (Steel et al., 2020)) and measurement devices (right) to stimulate and investigate the optogenetic constructs, respectively.
The ability to provide accurate, tunable, and controlled delivery of light is crucial for the effective application of optogenetics. In recent years, there has been a rapid acceleration in the development of new strategies and hardware-software platforms fulfilling this requirement (Figure 1). Custom-designed light stimulation devices (optogenetic platforms) targeted at different cell culture volumes are now available to address the specific needs of various applications. Certain specialized devices can further facilitate light induction capability at intracellular resolution and support high-throughput operation. Many of these platforms also include dedicated devices to measure cellular outputs such as fluorescent protein reporters (Rodriguez et al., 2017) (Figure 1) or cell growth, enabling real-time observation of target cell behaviour in response to light stimulation.
Optogenetic platforms equipped with a combination of light stimulation and cellular activity measurement devices have allowed for the implementation of in silico feedback control (Figure 2) of cellular processes ranging in application from simple gene expression regulation (Kumar et al., 2021) to multicellular morphogenesis studies (Hartmann et al., 2020). An in silico feedback control loop first involves the quantitative measurement of a desired cellular output (e.g. fluorescence) by a suitable measurement device. The output measurement data are then sent to a control computer which performs dedicated computations over the data using feedback controllers (Figure 2) such as integral and model predictive control strategies to determine a light intensity that the target cells should be stimulated with in order to achieve a desired output behaviour (e.g. set-point tracking). The computed light intensity is then applied to the target cells via the light stimulation device. This sequence of measurement, computation, and stimulation are executed iteratively at fixed time intervals to achieve closed-loop feedback control of the target process.
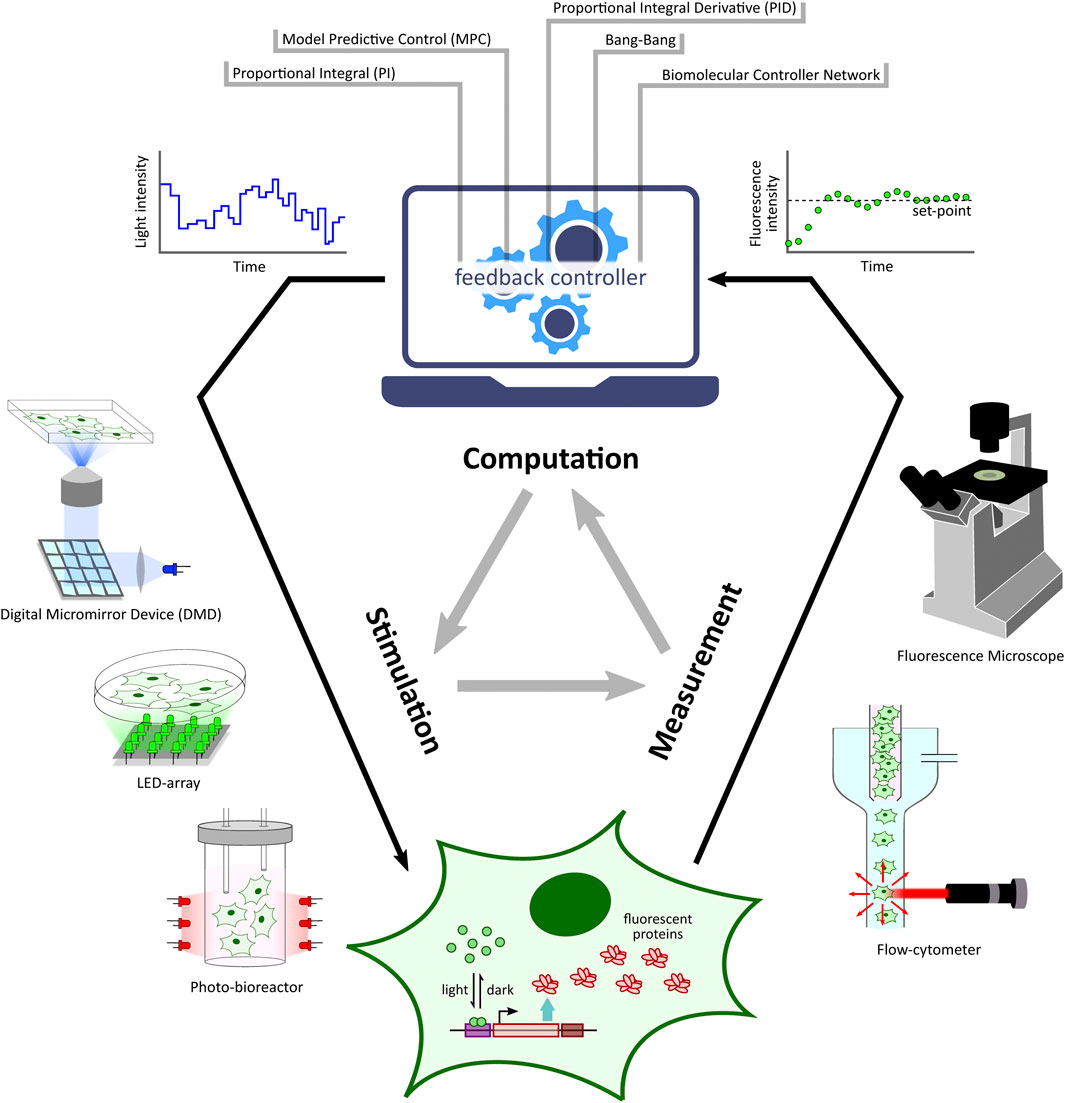
FIGURE 2. Optogenetic in silico feedback control framework. Given a target cell (engineered with desired optogenetic constructs and fluorescent reporter) culture, fluorescence measurements from target cells (measured via specialized devices, e.g. microscope, flow-cytometer, etc.) are sent to a computer where they are quantified. The quantified output is then used to run a controller (e.g. PI, MPC, etc.) simulation. The controller computes the required light input intensity for cells in order to achieve a desired output behaviour, such as tracking a desired set-point. This input light intensity is then applied to the target cells via a suitable light stimulation platform, thus closing the feedback loop. This measurement-computation-stimulation loop is performed at fixed intervals driving the output dynamics as desired.
This review aims to introduce and describe various optogenetic platforms and in silico control strategies developed for synthetic biology studies. In this article, we first categorize these platforms into three broad groups based on their intended target cell culture volume (
2 Low-Volume Culture Platforms
This category spans optogenetic platforms with target cell culture volumes ranging from a few microliters to a milliliter. These platforms are usually employed for short-term experiments to characterize and optimize optogenetic tools and downstream genetic circuits. They are also effective in studies such as pattern formation, requiring light-stimulation and observation at intra-/inter-cellular resolution. These platforms can be further divided into two groups: set-ups having a light stimulation device coupled with microscope systems, and set-ups having a dedicated LED-array light induction device adapted for micro-multiwell plates. The first group of platforms mostly facilitates higher spatial resolution and dynamic studies, whereas the second group is usually considered in high-throughput experiments pertaining to steady-state studies.
2.1 Microscope-Coupled Optogenetic Platforms
Light microscopes, by design, have suitable episcopic or diascopic light sources for transmitted-light, reflected-light, or fluorescence imaging. Without requiring any additional hardware modification, these imaging light sources can also be directly employed for optogenetic stimulation within the microscope field of view. In (Kennedy et al., 2010), the authors used fluorescence imaging blue (488-nm) light source, installed on their microscope, for stimulation purpose, and demonstrated light-induced activation of transcription and DNA recombination. A similar idea was adopted by Toettcher et al., 2013, where the authors used a 650-nm LED light source placed at the episcopic illumination port of the microscope for field of view stimulation, and ensured global 750-nm light illumination via the discopic side. This configuration allowed them to control both the activation (with 650-nm) and inactivation (with 750-nm) of PhyB (phytochrome B)-PIF (phytochrome-interacting transcription factor) interaction under a microscope. Further, in (Araki et al., 2014), the authors devised an automated fluorescence microscopy system in which they used fluorescence excitation light source for photoactivation and used a motorized field diaphragm in the fluorescence pathway to reduce the target area of illumination in the microscope field of view. Although having a simple design with precise control over illumination timing or duration, these direct episcopic/diascopic illumination microscope-coupled platforms have extremely limited utility for spatially-localized delivery of light. One way to achieve precise spatial control over light stimulation is by integrating sophisticated projection hardware with the microscope.
One of the initial microscope-coupled projector-based optogenetic platforms [inspired by (Wang et al., 2007)] was employed for the spatio-temporal control of cell signalling by using a light-switchable protein-protein interaction by Levskaya et al., 2009. In their work, the authors engineered a light-controlled membrane recruitment module based on phytochrome-PIF interaction in mammalian cells. Using this module, they demonstrated spatially localized activation of Rho-family GTPases (Rac1, Cdc42, and RhoA) inducing cell protrusion at a desired cell-membrane location via a spatially localized delivery of light. The light-delivery platform they proposed used a digital micromirror device (DMD) projection set-up integrated with a fluorescence microscope. A similar set-up was also used by Toettcher et al., 2011c demonstrating feedback control over light-gated protein-protein (phytochrome and PIF-tagged inputs) interaction. They used live-cell measurements under the microscope to update light-illumination intensities delivered via a DMD-based device. DMDs are extensively used as spatial light modulators in commercial projection systems (Dudley et al., 2003), and here in these platforms DMDs facilitated projecting user-defined mask images onto the microscope sample plane where the target cells are present (Figure 3A). In the last decade, several improved microscope-coupled DMD or LCD projector setups have been designed and demonstrated in cellular optogenetic control applications (Zhu et al., 2012; Chait et al., 2017; Rullan et al., 2018; Kumar et al., 2021; Fox et al., 2022; Singh et al., 2021). In most of these platforms, a DMD (Rullan et al., 2018) or LCD (Chait et al., 2017) projector, along with additional optical elements like lenses, filters, etc., is placed at the episcopic illumination port of the microscope. The optical elements are chosen and positioned in such a way that the projection image is focused onto the microscope sample plane through the microscope objective. An illustration of a generic DMD based set-up is shown in Figure 3A. The intensity of a pixel in the input projection image determines the light intensity received by the region (in the microscope sample plane) where that particular pixel is being projected. This allows a user to target separate regions of the sample plane with different light intensities, thus enabling spatial light-stimulation intensity variation at intra-/inter-cellular resolution (within the microscope field of view). Together with suitable software packages integrated with cell segmentation, tracking and quantification routines (Rullan et al., 2018; Fox et al., 2022; Pedone et al., 2021), these platforms uniquely facilitate independent stimulation and real-time observation of multiple single cells (present in the microscope field of view) in parallel. In (Fox et al., 2022), the authors also discuss the effect of erosion in light-stimulation regions as DMD systems can induce illumination bleed-through on the microscope sample plane. Suitably-defined light-erosion around illumination regions helps in reducing the bleed-through, and improves the precision of single-cell targeting in dense cell regions in the microscope field of view.
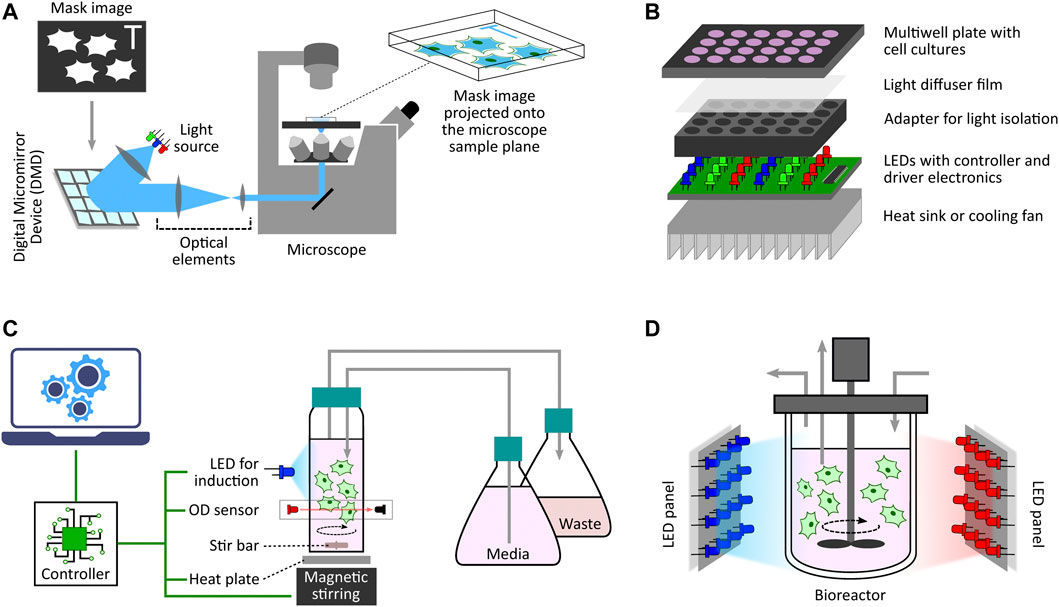
FIGURE 3. Diverse optogenetic platform architectures. (A) Microscope-coupled DMD-based platform illustration. The illumination light from LEDs is steered to fall onto a DMD at a particular angle. A DMD has micrometer-sized mirrors that can be individually tilted to ON and OFF positions. ON position allows the incident light to follow a defined path towards the microscope opening port whereas OFF position lets the incident light divert away after reflection. Once a given mask image is sent to the DMD controller, it sets the tilt-position of all DMD micromirrors accordingly with each micromirror representing an individual pixel of the image. These micromirrors oscillate between ON and OFF positions at a very high frequency, and the duty cycle of each micromirror is determined by the corresponding pixel intensity (gray value) in the mask image. When incident light is applied, the oscillating micromirrors allow the projection of the mask image towards the microscope opening port. The projection light then follows a series of optical elements (lens, filters, apertures, etc.) before entering the microscope port which then guides it towards the microscope objective lens. All optical elements are chosen and positioned in such a way that the projection image is focused onto the microscope sample plane allowing spatio-temporal and graded illumination of target cells placed under the microscope field of view. (B) Microtiter multiwell plate LED array illustration. These devices, in general, have a layered structure. The main layer is the electronic board fit with LEDs at regular matrix locations aligned with the target plate wells. This board can have multiple LEDs grouped together to provide multiple wavelengths per well. It also contains LED driver electronics and micro-controllers needed to set LED intensities and turn them ON/OFF as and when desired in a pre-programmed manner. To provide independent illumination to each well, an opaque adapter can be placed on top of the LED board isolating individual LEDs. The multiwell plate containing target cell culture is then placed on top of the adapter such that each well receives light from the LED underneath independent and isolated from other wells. One can also place a light diffuser film between the plate and the adapter to provide more homogeneous illumination within each well. Furthermore, if required, one can also add a heat sink or other cooling system under the LED board to dissipate heat generated by the electronics board which may adversely effect the target cell culture. (C) Milli-culture optogenetic platform illustration. The target cell culture is usually placed in a glass vial which can be externally illuminated with LEDs. Cell culture incubation temperature is maintained with a heat plate below the vial or a heated water bath. Aeration is achieved using devices such as magnetic stirrers. Some platforms also have an integrated system to measure and regulate growth conditions, such as maintaining a desired cell culture density during an experiment. They have dedicated OD sensors as well as media replenishing and culture removal mechanisms to provide turbidostat, chemostat, or morbidostat modalities. These platforms usually have separate micro-controllers/processors to execute these functionalities either in a pre-programmed manner or a live run by receiving updated commands from a computer during the course of an experiment. (D) Photobioreactor illustration. This is a generic photo-bioreactor set-up where the cells are usually cultured in a glass (transparent) off-the-shelf bioreactor. Two or more high-power LED panels are placed around the bioreactor to provide sufficient illumination of the target cell culture as and when required. Some designs also involve LED strips wrapped around the bioreactor for better illumination.
All microscope-coupled optogenetic platforms (with/without projector) can, in principle, facilitate multiple light-stimulation wavelength operations. The DMD projector used in (Rullan et al., 2018) has red, green and blue LEDs as light sources allowing stimulation with possibly three separate wavelengths. Therefore, one can use these platforms when there are multiple orthogonal optogenetic constructs in the target cellular system. These platforms also require suitable cell culture set-ups compatible with imaging and light stimulation under the microscope. Projector-based platforms focus a 2-D projection image onto the microscope sample plane which requires the target cells to be present in a monolayer fashion under the microscope. Adherent mammalian cell lines, such as HEK-293, can be cultured in simple petri dishes (Toettcher et al., 2011c) or imaging-compatible multiwell plates for experiments with these platforms. For non-adherent cell types such as yeast cells, one needs to use culture set-ups in which cells are constrained to grow in a monolayer. In (Kumar et al., 2021), the authors cultured yeast cells in a monolayer for up to 6 h by sandwiching them between a solid nutrient agarose pad and a glass coverslip. Microfluidic chips provide another way for achieving constrained cell proliferation under the microscope as shown in several studies for yeast (Rullan et al., 2018; Durán et al., 2020), bacteria (Chait et al., 2017; Lugagne et al., 2017), and mammalian cells (Woodruff and Maerkl, 2016). These chips are suitable for long-term experiments as they facilitate constant replenishment of nutrients and removal of dead cells via medium flow. In situ time-varying media exchange or mixing is also possible with these chips (interested readers can refer to (Gale et al., 2018) for an in-depth discussion on microfluidics technology). One unique approach is culturing adherent cells directly on high-density arrays of microscopic organic LEDs (OLEDs) (Steude et al., 2016). This OLED set-up facilitates light induction at a very high spatial resolution and can be placed onto the microscope sample plane for imaging. Although innovative, this set-up is extremely delicate in the sense that one needs to have a very thin (of the order of a few micrometers) encapsulation layer over the OLEDs in order to protect the electronics from aqueous cell culture medium. There are also some emerging microscope-based technologies allowing spatial stimulation control in 3-D space. They are briefly introduced in “Other emerging techniques” section, later in this review.
As previously described, microscope-coupled optogenetic platforms provide light stimulation capability with a high spatial resolution (at the level of intra-/inter-cellular resolution), and also facilitate feedback control operation (by allowing both stimulation and fluorescence measurement) over the target cells. However, they have certain limitations that need to be addressed. First, it is difficult to controllably regulate cell density under a microscope. Cells continuously proliferate under favourable conditions, with the inevitable outcome that the microscope field of view will become fully confluent over time (dependent on cell doubling time), even if the experiment is started with only a few cells. This makes tracking, stimulating and imaging a single cell challenging in long-term optogenetic control experiments. One can use a microfluidic “mother machine” device (Chait et al., 2017; Durán et al., 2020) under the microscope to ensure single cell tracking in long-term experiments, but these special cell culturing devices are only compatible with bacterial or yeast cells. Additionally, while designing synthetic constructs in cells, one is restricted to using fluorescent proteins as a reporter system because observation on these optogenetic platforms is mostly carried out via fluorescence imaging. Furthermore, these optogenetic platforms come with a hefty financial burden. Advanced microscopes, which can cost more than $100k, are one of the most expensive equipments in a biology lab, and the commercially available pattern projector set-ups (for example, Mosaic - Andor Oxford Instruments, Polygon - Mightex, DMD module - Nikon, etc.), capable of coupling with a microscope, are also expensive (∼ $25–60k).
2.2 LED-Array Light Induction Device
For applications not requiring light stimulation at single-cell resolution, there are also custom-designed LED arrays or matrices adapted for population-level induction of cells grown in microwell plates. One simple design was used by Ye et al., 2011 where the authors demonstrated light-inducible transgene expression in mammalian cells. Their device comprises a matrix of 6 or 12 blue LEDs (placed above 6- or 12-well cell culture plates) connected with a regulated DC power supply controlled by Labview software from a computer. A detailed protocol for the construction of an improved programmable device (intended for 12-well format plates) has been provided by Tucker et al., 2014. This design provides independent programming of upto three LED outputs. Additionally, it includes LCD display interface and keypad for setting the duration, interval and intensity of LED pulses without needing any computer for configuration. Although innovative, these simple devices do not provide optical isolation between adjacent well LEDs, and hence do not offer independent induction control among multiple wells. Researchers have proposed a multitude of other evolved and refined optogenetic platforms for multiwell plates. A general architecture of these platforms is illustrated in Figure 3B.
In (Mertiri et al., 2011; Chen et al., 2012) inspired by (Johnson and Sheldon 2007), the authors presented an optical microplate system having an 8 × 12 matrix of 96 LEDs (128 brightness levels) suitable for light induction in a 96-well plate from below, and demonstrated its use in the high-throughput screening of algal photosynthesis. By using a standard black 96-well plate to encase the LED matrix, this design ensures very little spillover between adjacent LEDs, and hence each cell culture-containing well of a 96-well plate (black walls and transparent bottom), placed on top of the device receives light from separate LEDs underneath. These LEDs are individually controlled (time and intensity) via a serial microcontroller, thus facilitating parallel runs of high-throughput experiments. A graphical user interface (GUI) in Labview software is used to program different light patterns on the device. This optical microplate design was further augmented by Davidson et al., 2013 with multi-color LEDs providing two-color control in each well. Further, Hennemann et al., 2018 proposed a set-up where three fixed-color channel LEDs (470, 525, and 620 nm) are used in an 8 × 8 layout (for 64 separate wells) that can achieve 256 brightness levels. They also designed a python-based GUI for easy configuration of LEDs for light induction experiments. An improved and refined design in optoPlate-96 (Bugaj and Lim, 2019) facilitates one- to three-color illumination (4,096 brightness levels) for individual wells of a 96-well plate (or neighbouring groups of four wells in a 384-well plate). As per user requirements, different wavelength LEDs can be substituted on this modular platform. Additionally, a dedicated active heat management system comprising a heat sink and a cooling fan has been incorporated in this design to minimize heat generation from on-board electronics.
Similar optogenetic platforms have been proposed and demonstrated in applications for cell/tissue cultures in 24-well plates. In (Müller et al., 2014), the authors used a simple illumination box having LEDs mounted on the inner lid of an enclosed box in which the cell/tissue culture well plate can be placed for stimulation. Here, the wells are not optically separated, and thus cannot be independently illuminated. Platforms devised in (Hannanta-Anan and Chow, 2016; Lee et al., 2013; Gerhardt et al., 2016) have improved designs with adequate optical separation through black walls and independent control over individual well LEDs in the 24-well black-wall transparent-bottom plate. In the device proposed by Hannanta-Anan and Chow (2016), not individual LEDs but columns of four LEDs (with six columns in total) are independently controllable. The Light Plate Apparatus (LPA) proposed by Gerhardt et al., 2016 has two configurable and independent LEDs for each well of the 24-well plate, and each LED intensity can be adjusted with 4,096 brightness levels. This device uniquely uses solder-free sockets for LEDs which makes it much easier to change/replace LEDs without any wear and tear. Gerhardt et al., 2016 also designed a user-friendly web tool to program the device for static (constant light stimulation) as well as dynamic (periodically varying light stimulation) experiments.
An improved design (LAVA), intended for independent well stimulation of 24-well or 96-well culture plates, has been recently proposed by Repina et al., 2020. Here, the authors have shown a detailed characterization and quantification of illumination uniformity, device overheating, phototoxicity, and spatio-temporal light-patterning resolutions in their proposed design. Provisions for improved operation concerning these modalities have also been incorporated in the set-up. The authors also demonstrated spatial patterning (with ∼ 100 μm resolution) using a photomask on this platform. This optogenetic device can be configured for an experiment via an easy-to-use and intuitive GUI, which allows users to set time-varying and complex light intensity patterns for each independent well.
These multiwell plate optogenetic platforms usually consist of low-cost widely-available LEDs which always have brightness differences due to variation in the manufacturing process. This implies that two LEDs of the same type will always display slightly different light intensities, even when powered by the same electrical current. This intensity difference may lead to discrepancies when replicating light stimulation experiments. That is why the LEDs in these set-ups have to be characterized and power-corrected to reduce intensity variation between them (and in turn reduce the well to well variation). Mertiri et al., 2011 and Chen et al., 2012 followed a manual calibration procedure by manually measuring with a light meter the light intensity each well receives, and scaling the power setting in each well by a suitable calibration factor. In (Grødem et al., 2020), the authors propose an automated calibration method for optoPlate (Bugaj and Lim, 2019) LEDs. They use a programmable microscope stage and optical power meter to automatically measure all LEDs on the device and then compute the required calibration values needed to adjust the electrical current supplied to each LED in order to reduce the light intensity variation between LEDs. In (Gerhardt et al., 2016), the authors describe two calibration methods for their LPA device: image analysis (for calibrating LEDs of same wavelength) and probe spectrometer (for calibrating LEDs of different wavelength) methods. The first method involves capturing top-down images of the device with LEDs lit at a constant intensity, and then further analysing the pixel intensities in the image using a MATLAB script to measure differences in light intensities between wells. These pixel intensity values are then used to compute grayscale adjustment and dot correction parameters for each LED. The other probe spectrometer method involves measuring the photon flux of each LED using a probe spectrometer aligned via a 3D printed probe adapter, and then adjusting dot correction based on measured values. These calibration procedures have been shown to reduce LED brightness variability to
In contrast to microscope-coupled platforms, these LED-array devices are low-cost (under $1k), and can be designed in-house with readily-available components. Building and assembling these set-ups require only basic knowledge and experience with electronics and mechanical design tools. There are also some off-the-shelf commercially available options, such as Lumos from Axion Biosystems, but they are expensive (∼ $13–26k for Lumos) compared to previously discussed custom-designed devices. The small form-factor of these platforms makes them easy to transport; and they can also be placed inside incubators, thus ensuring favourable conditions for cell cultures during the experiment. Some of these set-ups also incorporate a thermal management system (heat sink, cooling fan, etc.), for example in (Bugaj and Lim, 2019; Repina et al., 2020), to negate the effect of heat generated by LEDs and electronic circuitry onto the target cells under stimulation. In most of these devices, the illuminating LEDs can be substituted with the wavelengths required for individual application, and some of the improved ones (mentioned previously) also facilitate up to three wavelength inductions in the same experiment. These set-ups can also be fit with diffuser films/sheets, such as in (Gerhardt et al., 2016), to ensure homogeneous illumination inside the well. They are also high throughput devices, and depending on the number of wells in the multiwell plate being used, one can perform that many parallel studies with cell cultures. As mentioned previously, these platforms (unlike microscope-coupled platforms) do not provide precise spatial control over light delivery. But, one can use simple photomasks to achieve a desired spatial-pattern light illumination onto lawns of cells cultured in well plates or petri dishes (Levskaya et al., 2005; Beyer et al., 2015; Romano et al., 2021; Morales et al., 2021). Using illumination through photomasks, the authors in (Levskaya et al., 2005; Romano et al., 2021) have also demonstrated creating a high-definition high-contrast chemical image (bacteriograph) of the projected light mask pattern similar to images captured on camera films.
One major disadvantage of these LED-array platforms is their inability to perform measurements during an experiment. One can only obtain a steady-state final measurement after light induction is finished; one cannot perform dynamic or periodic time-point measurements. Hence, these devices do not, in general, offer feedback control capability. However, Chen et al., 2020 use the optoPlate design (Bugaj and Lim, 2019) to achieve simultaneous light-stimulation of every cell culture in a 96-well matriplate while intermittently imaging under a widefield microscope. One can place these optoPlates on top of the 96-well sample plate to achieve illumination from above and imaging from below when placed under an inverted microscope. Recently, researchers in (Soffer et al., 2021) have proposed an innovative light delivery system (RT-OGENE) to allow both microwell plate illumination with a 2 × 2 RGB LED matrix (so only four wells can be illuminated simultaneously) and fluorescence/absorbance measurement with a simple camera without needing an expensive microscopy platform. The set-up involves a camera and a long-pass filter placed on top of the cell culture well-plate with optical filters and illumination LEDs placed underneath. This whole platform is placed inside a custom-designed incubation chamber fit with temperature control system. By having both stimulation and measurement devices available on this platform, they demonstrated closed-loop feedback control of a CcaR-CcaS optogenetic construct in Escherichia coli. In addition to multiwell plates, this device is also shown to be compatible with previously described microfluidic cell culture platforms. Another interesting approach of augmenting a multimode microplate reader with illumination sources has been explored in (Richter et al., 2015). Here, the authors attached a fiber-optics waveguide with the injection cylinder of Tecan Infinite M200 pro multimode microplate reader. By default, in this plate reader the injection cylinder can inject liquids into defined wells of the microwell sample plate; but with this proposed modification one can also illuminate those defined wells via a waveguide. A separate microcontroller was integrated with the plate reader control software to define illumination position and wavelength (the waveguide was connected to an input LED light source containing eight different LEDs) in the set-up. Although this platform has the potential to facilitate feedback control operation (stimulation via waveguide and measurement via plate reader) over multiwell sample plates, the wells can only be stimulated sequentially, thus limiting its throughput. At the same time, Tecan microplate readers are very expensive (∼ $40k), thus limiting its adoption.
3 Medium-Volume Culture Platforms
This section spans optogenetic platforms with intended target cell culture volumes of a few to hundreds of milliliters. These volumes are usually considered in studies where certain constraints on growth conditions or cell density are needed during long-term experiments. For example, in the optogenetic experiments in (Aditya et al., 2021), cells needed to be continuously maintained in exponential growth phase. Low-volume culture platforms are not suitable for those studies as it is difficult to regulate cell density at such low volumes. Most of the platforms described here are coupled with turbidostat, chemostat, or morbidostat set-ups in order to facilitate operation in those conditions. A generic architecture of these platforms is shown in Figure 3C.
One of the initial designs in this category was proposed by Milias-Argeitis et al., 2011 in their pioneering work of demonstrating in silico feedback control of gene expression in yeast. This set-up involved a custom-built light delivery system with a light pulser device (having two triplets, red and far-red wavelengths, of high power LEDs) and an electronics box containing LED driver electronics which can be connected with a computer via a multifunction DAQ (Data Acquisition) board. The custom-designed electronics provided both manual as well as computer-controlled (needed for feedback control experiments) operation of the device. This system can be used both with multiwell cell culture plates (without independent illumination of separate wells) by placing sample plates underneath the light pulser device (Milias-Argeitis et al., 2011), and with glass/transparent tube cultures (Ruess et al., 2015). The authors in (Ruess et al., 2015) used this system with a hot plate magnetic stirrer to maintain a desired cell culture temperature and provide sufficient aeration to the target culture. They also diluted the culture manually at regular intervals to keep the cell density within reasonable bounds during an experiment. Another simple design with a higher-throughput set-up (capable of running 15 tubes independently in parallel) was used by Baumschlager et al., 2017 (also in (Benzinger and Khammash, 2018)). This set-up comprises a water bath (to maintain a desired temperature) having custom-built 15-tube holders, which can hold 25-ml glass cell culture tubes, each fitted with a magnetic stirring device and a custom-made LED pad (with a white diffusion filter to ensure homogeneous illumination) located underneath each culture tube. This set-up allows for having a pre-programmed illumination sequence for each tube in an experiment, but does not allow for the real-time change of LED intensity during the experiment, and thus doesn’t have feedback control operation capability. A similar but improved Light Tube Array (LTA) platform was proposed by Olson et al., 2014 which is capable of providing an isolated optical environment for 64 standard 14-ml culture tubes. Each tube can be illuminated with four different LEDs independently with the given LED driver electronics that can be pre-programmed with light-intensity sequences. Although the set-up does not include any stirring or temperature control mechanism in itself, the whole tube rack can be placed in a suitably-sized shaking incubator.
Several other innovative and advanced platforms combining multiple functionalities and automation principles have been proposed as well as demonstrated in various applications, notably feedback control over cellular processes (owing to the availability of both light-stimulation and measurement devices on these platforms). In (Melendez et al., 2014) and improved in (Stewart and McClean, 2017), the authors exhibited controlling intracellular protein concentration with a CRY2-CIB1 optogenetic construct in yeast by using their automated culturing optogenetic platform. The set-up they proposed involves a 27-ml working volume transparent culture vessel where cells can either be grown in batch culture or maintained as continuous culture for several days in chemostat mode by using the automated media influx and culture efflux system. Provisions for temperature control, agitation and aeration to oxygenate cells in the vessel are available on this platform. A separate microscope-based measurement apparatus is also integrated with the set-up, allowing fluorescence imaging and quantification of single cells in the culture by running them through a single channel microfluidic flow cell mounted on the microscope. Light induction in the culture vessel is performed with a high-powered blue LED, all controlled by a computer via a custom-designed control board. Another automated optogenetic platform demonstrated by Milias-Argeitis et al., 2016 employs a custom-designed turbidostat to maintain cell culture at a desired optical density (OD), and an automated sampler to facilitate real-time measurement via flow cytometer. This platform also has integrated culture incubation, LED-based light induction, and a culture stirring system for proper aeration in the 25-ml glass centrifuge cell-culture tube. By using computer control over the whole apparatus, Milias-Argeitis et al., 2016 exhibited optogenetic cell growth-rate control of a culture of bacterial cells for over 24 h. Another similar platform was proposed by Harrigan et al., 2018 which comprises eight (in two incubator arrays of four each) or more (expandable) separate temperature-controlled and magnetically-stirred chambers for 50-ml conical cell culture tubes and LED stimulation. The authors also designed an automated integrated liquid handling module capable of moving cell culture from individual chambers to a flow cytometer for measurement or to a waste reservoir for dilution as well as replenishing the individual chambers with fresh media.
Recently, researchers have put forth various innovative and compact optogenetic platform designs pertaining to this medium-volume culture category. One particularly unique design, Chi.Bio, was presented by Steel et al., 2020 which establishes a very compact and low-cost approach of optogenetic stimulation and in situ fluorescence measurements. Their system is divided into reactors, pump boards and mini control computers. The reactor is a compact housing for 30-ml clear glass test tubes (open to the atmosphere), and it has integrated tunable light-induction LEDs, temperature control and stirring systems, an OD sensor, and a spectrometer for measuring multiple fluorescence proteins in situ. One pump board per reactor is needed to achieve media or culture flow to/from the reactor culture tube providing turbidostat/chemostat/morbidostat operation capability. Furthermore, the mini control computer can control eight such integrated reactor pump board set-ups independently in parallel. The software stack enables users to control and monitor any peripherals, and set-up experiments via a user-friendly web user interface. Any advanced procedures and computations in an automated experiment, for example feedback control algorithms, are easily implementable via their python framework. One downside of this platform is the low sensitivity of its low-cost integrated chip-based spectrometer compared to much more expensive flow-cytometers. This might be a limiting factor in some applications with synthetic genetic constructs requiring very sensitive fluorescence measurements. But one can also integrate separate liquid handling modules with this set-up to facilitate flow-cytometric or microscopic measurements in addition to the in situ fluorescence measurement. The ReacSight strategy proposed by Bertaux et al., 2021a offers such an enhancement. This is a generic and flexible strategy for adding different instruments in the automated measurement framework of different optogenetic platforms. Bertaux et al., 2021a also demonstrated this strategy with Chi.Bio reactors as an example, and this combined platform of ReacSight and Chi.Bio has been further used in some recent optogenetic control studies (Aditya et al., 2021, 2022).
Another interesting optogenetic platform with a custom-designed multicolor fluorescence monitoring device was proposed by Pen et al., 2021. Here, the cell culture chamber is a continuous cell culturing module (allowing turbidostat or chemostat mode operation) adapted for a 30-ml cylindrical glass vial with a gasket cap (for gas handling functions), and includes temperature control and magnetic stirring systems. Additionally, this set-up by Pen et al., 2021 also has a separate module for in situ fluorescence measurement. There is a provision of dipping a multifiber (optic bundle) probe into the cell culture through the lid which allows excitation light propagation from excitation LEDs onto the cell culture and subsequent collection of fluorescence emission signals which are then guided towards a microPMT (Photomultiplier tubes) for fluorescence measurements. These PMTs are expensive but have a higher sensitivity and higher dynamic range compared to the fluorescence sensor used in Chi.Bio. This platform can also be modified and used for light stimulation of the cell culture via the fluorescence excitation LEDs.
Similar to the LED-array light induction devices discussed in the previous section, these optogentic platforms are only intended for target stimulation and observation on a population level, meaning they cannot be employed for single-cell studies. Standalone optogenetic platforms without a measurement device can cost around $10k or less for 10–16 parallel throughput set-ups. However, to achieve feedback control, one would need to integrate them with other measurement devices like flow-cytometer or microscope which are very expensive. As mentioned previously, the Chi.Bio set-up (Steel et al., 2020) does offer a low-cost integrated spectrometer, but it is not suitable for measuring weak fluorescence signals. Unlike low-volume culture platforms, most of these devices have integrated turbidostat, chemostat, or morbidostat operation set-ups which can be used to maintain growth conditions and regulate cell densities in the target culture during an optogenetic experiment. Moreover, most of these platforms can only work with suspension cell cultures. In the non-optogenetic biology literature, there has been extensive development of turbidostat/chemostat/morbidostat set-ups; therefore, a wide variety of designs, intended for different applications, are now available (Toprak et al., 2013; Miller et al., 2013; Takahashi et al., 2015; Hoffmann et al., 2017; Wong et al., 2018; Bergenholm et al., 2019; McGeachy et al., 2019; Gopalakrishnan et al., 2020). With some basic electronics know-how, one can easily integrate light-stimulation LEDs or other light sources into these already existing devices to achieve optogenetic stimulation.
4 Large-Volume Culture Platforms
Here we consider optogenetic platforms aimed at suspension cell culture volumes beyond hundreds of milliliters. These large volumes are usually handled in large-scale bioreactors which are one of the staple devices in biochemical/bioprocess industries (Najafpour, 2015). Given the scope of this review article, we will restrict our discussion to bioreactor platforms employed in academic research.
There have been a number of incremental studies on metabolic engineering and protein production regulation in lab-scale bioreactors. One of the major goals of metabolic engineering research today is to maximize the yield of a given bioproduction process. To achieve this goal, there is an ever-growing interest in the synthetic biology community to build genetic constructs allowing for dynamic control of metabolism (Venayak et al., 2015; Lynch, 2016; Ni et al., 2021). The idea is to introduce externally inducible systems in metabolic pathways with different induction strategies (Lalwani et al., 2018; Bertaux et al., 2021b). Several induction strategies (e.g. chemical induction, temperature control etc.) have been explored in this context. A list of these strategies, pertaining to Escherichia coli and Saccharomyces cerevisiae cell-cultures, has been summarized in (Lalwani et al., 2018). With the recent development in optogenetics and related genetic tools, one can not help but foresee its application in metabolic engineering with its unique benefits (light induction is inexpensive and provides precise temporal control) (Carrasco-López et al., 2020; Pouzet et al., 2020). However, this strategy of using light induction for efficient bioproduction is still in its nascent phase of realization, and only a few studies with simple light delivery devices have been carried out in recent years.
In (Zhao et al., 2018), the authors demonstrated production of isobutanol and methyl-1-butanol via light stimulation of an engineered S. cerevisiae culture. In their study, they used 500-ml, 2-L and 5-L capacity bioreactors with custom-designed light delivery set-ups. Multiple (2 for 500-ml and three for 2-L) LED matrix panels were used to surround the first two (500-ml and 2-L) transparent glass bioreactors, while multiple blue LED strips were wrapped around the 5-L bioreactor to achieve light stimulation of respective cell-cultures. A similar set-up for a 2-L bioreactor was used by Lalwani et al., 2021 to demonstrate light-induced mevalonate and isobutanol production in engineered E. coli. In (Zhao et al., 2021), the authors presented a series of sensitive optogenetic circuits (OptoAMP) and demonstrated their ability to enhance the production of lactic acid, isobutanol, and naringenin on a photo-bioreactor platform. They used a commercial high power LED-matrix panel to illuminate the 5-L bioreactor in their experiments. These simple light delivery devices are inexpensive and can be coupled with other suitable (transparent) bioreactors which are usually expensive on their own. A representative design of these platforms is illustrated in Figure 3D.
One problem encountered when illuminating a large volume culture is insufficient penetration of light at high cell densities (Carrasco-López et al., 2020). This challenge can be overcome by either improving the sensitivity of optogenetic circuits (Zhao et al., 2021), risking a reduction in dynamic range and an increase in leaky dark activation, or by improving the light delivery system. One can design the inside of a bioreactor with provisions for additional light sources to enhance light penetration, for example, having multiple LED rods dipped in the target cell culture via suitably designed lids. Similar design strategies have been proposed by Carrasco-López et al., 2020 in their opinion article, but these designs have not been implemented yet. Within the realm of microalgae biotechnology, large photobioreactors are commonly used for microalgae cultivation. One can also employ their designs (Posten, 2009; Chen et al., 2011) for bioreactor illumination with little or no modifications. Furthermore, to provide feedback control, a liquid handling module similar to the design proposed by Harrigan et al., 2018 can be used to move samples from the bioreactor to a suitable measurement device such as flow-cytometer. To the best of our knowledge, no optogenetic feedback control as envisioned in (Carrasco-López et al., 2020; Pouzet et al., 2020) has been demonstrated yet with these bioreactor platforms.
5 Other Emerging Techniques
Apart from the devices and systems covered in the previously discussed three categories (low-, medium-, and high-volume culture platforms), there are some other innovative and unique designs which were developed for specific applications. We would like to mention a few of these platforms which may have potential utility in emerging optogenetic applications.
A compact light delivery device (LED Thermo Flow) that can be directly connected to flow-cytometer injection ports was described by Brenker et al., 2016. In this design, a glass FACS tube containing target cell culture is inserted into a custom-built cylindrical sleeve. The sleeve contains multiple wavelength LEDs with manually-controllable intensities surrounded by water which is constantly circulated via a heated water pump to maintain the sample temperature at a desired value. The FACS tube, together with the sleeve, is then connected to a flow-cytometer injection port for direct measurement. This allows one to measure real-time effects of optogenetic stimulation and deduce kinetics of optogenetic tools.
In all the optogenetic platforms described previously, light illumination was targeted for cells growing in any cell culture lab-platform but not for cells proliferating within a live animal. In (Ye et al., 2011), the authors devised a system to provide light-stimulation to mammalian cells (engineered with a desired optogenetic construct) implanted intraperitoneally in mice. In their set-up, light from a high-brightness LED is delivered to the cells in hollow-fibre implants via a multimode silica glass optical fibre. The LED is powered by a regulated DC power supply whose output voltage (in turn, the LED light intensity) can be modulated via Labview software running on a computer.
All the platforms discussed so far in this review facilitated either precise spatial 2-D illumination (microscope-coupled platforms) or full 3-D homogeneous illumination with no spatial control. One of the emerging light induction techniques involves stimulating samples in 3-D with spatial precision. Holography-inspired multiphoton photo-excitation (3D-SHOT) (Pégard et al., 2017), DMD-based patterned illumination in lightsheet microscopy (Jiao et al., 2018), and three-dimensional multi-site random access photostimulation (3D-MAP) (Xue et al., 2022) are some of the recently proposed designs in this direction. These platforms have the potential to aid artificial tissue engineering or organoid development via optogenetic induction. To the best of our knowledge, these new techniques have only been applied in neuroscience research such as whole-brain neuronal activity studies in larval zebrafish (Jiao et al., 2018). Their application with non-neuronal cells or tissues is yet to be explored.
6 In Silico Feedback Control Strategies
Feedback control is a key concept in the control theory literature. It has a wide range of applications in our day to day life, from a thermostat controlling room temperature to the autopilot maintaining airplane attitude. Feedback control over a process requires a mechanism to tweak the process as well as a pathway to measure the process behaviour. Here, we will briefly discuss different feedback control methodologies pertaining to cellular biology contexts where the tweaking/stimulation of a target process (such as gene expression) is enabled by optogenetics. A brief introduction and description of optogenetic in silico feedback control procedure has been laid out previously in the introduction section, and a related illustration is shown in Figure 2.
Depending on the application as well as the capability of the optogenetic platform used, one can implement in silico feedback control over a target cell culture in two different settings: population control (Milias-Argeitis et al., 2011; Melendez et al., 2014; Milias-Argeitis et al., 2016; Harrigan et al., 2018) and single-cell control (Rullan et al., 2018; Kumar et al., 2021; Fox et al., 2022), as illustrated in Figure 4. Population control involves an aggregate measurement of the target cell culture and stimulation with a common input light intensity for all the cells in every measurement-computation-stimulation cycle. This can be achieved with those optogenetic platforms which are integrated with a measurement device (assuming that the required controller computation over measurements to compute the input light intensity can be performed on a computer). Meanwhile, exhibiting a reduced cell-to-cell output variability compared to population control (Rullan et al., 2018), single-cell control involves measuring single-cell outputs in a culture, running controller computations for each cell based on its quantified output, and then applying the computed light intensities to the respective target cells independently. This setting can be employed with microscope-coupled projector-based optogenetic platforms discussed previously.
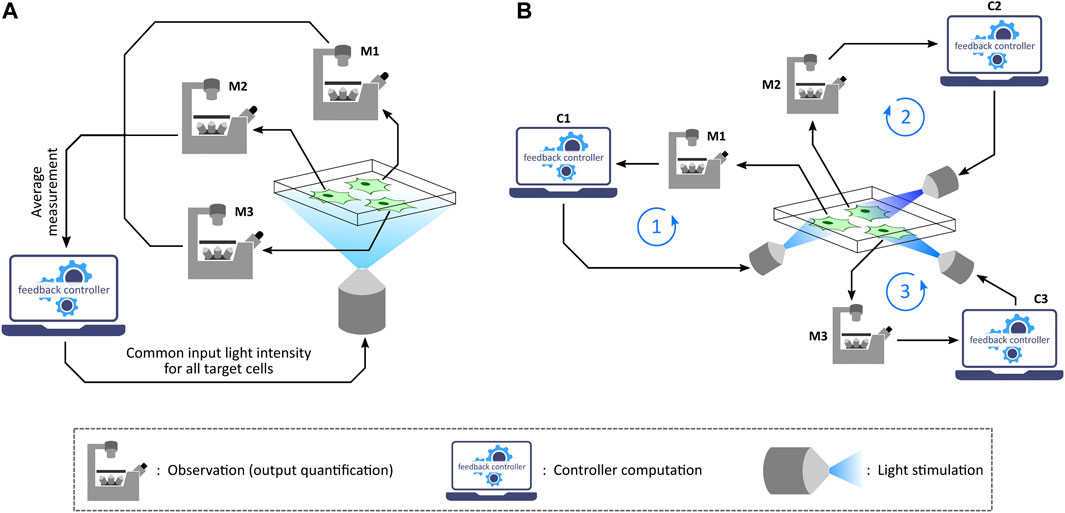
FIGURE 4. Population control and single-cell control. (A) In the population feedback control strategy, a statistically average measurement from all the target cells in a culture is sent to a computer. The feedback controller is then simulated over the average output value and then a common input light intensity is computed. This intensity is applied to all the target cells in the culture via a suitable illumination set-up. Note: M1, M2, and M3 represent the same microscope. (B) In the single-cell feedback control strategy, each cell undergoes independent and parallel treatment having its own feedback loop in action. Once a fluorescence image is captured under the microscope, the image is segmented to quantify output measurement from single cells. These quantified outputs are then sent to separate feedback controllers corresponding to individual cells. Input light intensities for each cell are computed and also applied to the corresponding cells separately via a projector-based set-up integrated with the microscope. Note: M1, M2, and M3 represent the same microscope; C1, C2, and C3 represent the same control computer.
Another critical aspect of in silico feedback control application is the type of controller algorithms employed in the computation. This controller algorithm has the task of computing an input light-intensity (which the target cell culture should be stimulated with) based on output measurements obtained from the target cell culture (in every measurement-computation-stimulation cycle), in order to achieve a desired output behaviour (Figure 2), for example, driving nascent RNA count to a desired set-point value (Kumar et al., 2021). One of the initial demonstrations of optogenetic in silico feedback control was realized by Milias-Argeitis et al., 2011. With their custom-designed optogenetic platform, the authors demonstrated robust regulation (desired set-point tracking) of gene expression fold change in genetically engineered S. cerevisiae by using an in silico feedback controller implementing Model Predictive Control (MPC) strategy. Further, Milias-Argeitis et al., 2016 carried out optogenetic in silico feedback regulation of gene expression as well as cell growth in suitably engineered E. coli cultures. They implemented two different control algorithms: Proportional-Integral (PI) and MPC. PI controllers have a very simple implementation offering a control input (light-intensity) value which is proportional to the sum of 1) the error between a desired set-point and the current measured output, and 2) the time-integral of this error. These PI controllers guarantee zero error at steady-state when the set-point is constant over time, but they are not suitable for tracking time-varying set-points. On the other hand, MPCs are capable of tracking dynamic set-points but they require a model of the target process in their implementation. Further technical details and a comparison between these two controllers are described in (Milias-Argeitis et al., 2016). Another approach using a Proportional-Integral-Derivative (PID) controller has been proposed by Soffer et al., 2021 where the authors employed a model-based design for tuning controller parameters. Other in silico controller implementations such as PI control (Toettcher et al., 2011c), bang-bang control (Melendez et al., 2014), MPC (Harrigan et al., 2018), and integral feedback (Rullan et al., 2018) have been demonstrated with their respective optogenetic platforms and target processes. Interested readers can refer to (Grosenick et al., 2015) for a list and brief descriptions of different controller types which can be realized in in silico control architecture for controlling any suitable target cellular process. We would like to note here that this list is formulated in the context of neuroscience applications, but similar conclusions can be extended to non-neuronal cellular applications as well. A similar list of feedback controllers (including their advantages and disadvantages) along with some examples in non-neuronal studies can be found in (Carrasco-López et al., 2020).
Optogenetic in silico feedback control has numerous emerging applications in biotechnology, biomedicine, bioproduction and various synthetic biology studies (Chen et al., 2013; Liu et al., 2018; Lugagne and Dunlop, 2019; Hartmann et al., 2020; Banderas et al., 2020; Carrasco-López et al., 2020; Pouzet et al., 2020; Perrino et al., 2021; Ruolo et al., 2021). Formation of emerging patterns from lateral inhibition via an optogenetic in silico feedback approach to emulate cell-to-cell communication (Figure 5A) was demonstrated by Perkins et al., 2020 using the optogenetic platform described in Rullan et al., 2018. Furthermore, Kumar et al., 2021 implemented several in silico biomolecular controller motifs in stochastic setting and exhibited transcription regulation in suitably engineered S. cerevisiae via single-cell optogenetics control. With this in vivo (transcription in yeast) - in silico (stochastic controller) hybrid closed-loop set-up, called Cyberloop (Figure 5B), they showed characterization and rapid-prototyping of autocatalytic integral (Briat et al., 2016a), antithetic integral (Briat et al., 2016b), antithetic integral rein (Gupta and Khammash, 2019), and biomolecular PID (Filo et al., 2022) controller motifs. These characterization results provide useful insights for optimal controller performance, which can further guide in vivo implementations of these biomolecular controller motifs.
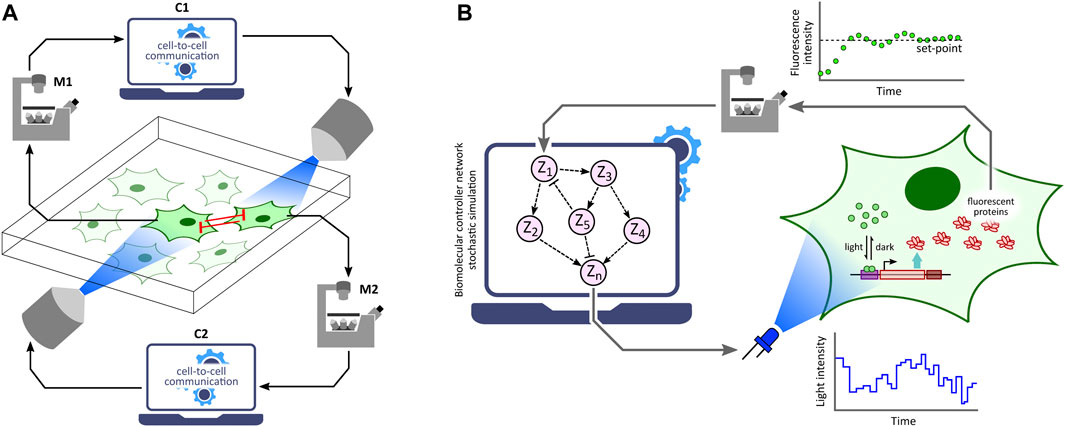
FIGURE 5. Applications of optogenetic in silico feedback control. (A) Cell-to-cell communication studies. Cellular outputs from neighboring cells are used to determine the light stimulation intensity of a target cell. Using this framework, lateral inhibition between neighboring cells has been shown to produce emerging checkerboard patterns in a 2-D grid of cells (Perkins et al., 2020). Note: M1 and M2 represent the same microscope; C1 and C2 represent the same control computer. (B) Rapid prototyping of biomolecular controller motifs. Stochastic simulation of biomolecular controller networks is run in the in silico feedback control framework with an in vivo target network. This allows rapid benchmarking and characterization of these controller motifs without requiring their in vivo implementations (Kumar et al., 2021).
7 Conclusion
With the advent and ongoing development of various synthetic optogenetic tools that can be implemented in various cell types, a number of hardware-software platform designs have been co-developed. As discussed in this review, these optogenetic platforms have varying features and design methodologies depending on the application, target cell type, and target cell culture volumes. Their complexities range from simple LED strips to full-fledged microscope-coupled 3D hologram generators. Various simple set-ups, mentioned in this review, comprise readily available low-cost components, and can be easily built/assembled in basic research labs equipped with elementary electronic tools and minimal mechanical design expertise. They have the potential to reduce barriers for carrying out advanced optogenetics research with minimal resources. When integrated with an appropriate measurement device, some of these platforms also provide optogenetic in silico feedback control (Cybergenetics) capability, with which different feedback control strategies can be implemented and investigated. This capability has a wide scope of applications in biotechnology and synthetic biology studies.
Author Contributions
SK and MK conceived the review focus; SK wrote the first draft of the review; MK and SK contributed to the final version.
Funding
Open access funding provided by ETH Zurich.
Conflict of Interest
The authors declare that the research was conducted in the absence of any commercial or financial relationships that could be construed as a potential conflict of interest.
Publisher’s Note
All claims expressed in this article are solely those of the authors and do not necessarily represent those of their affiliated organizations, or those of the publisher, the editors and the reviewers. Any product that may be evaluated in this article, or claim that may be made by its manufacturer, is not guaranteed or endorsed by the publisher.
Acknowledgments
The authors would like to thank Dr. Stephanie Aoki, Dr. Armin Baumschlager and Dr. Craig Hamilton for their valuable feedback on the manuscript. The authors sincerely apologize to all colleagues whose work was not cited due to space limitations.
References
Aditya, C., Bertaux, F., Batt, G., and Ruess, J. (2021). A Light Tunable Differentiation System for the Creation and Control of Consortia in Yeast. Nat. Commun. 12 (1), 5829. doi:10.1038/s41467-021-26129-7
Aditya, C., Bertaux, F., Batt, G., and Ruess, J. (2022). Using Single-Cell Models to Predict the Functionality of Synthetic Circuits at the Population Scale. Proc. Natl. Acad. Sci. 119 (11), e2114438119. doi:10.1073/pnas.2114438119
Araki, N., Ikeda, Y., Kato, T., Kawai, K., Egami, Y., Miyake, K., et al. (2014). Development of an Automated Fluorescence Microscopy System for Photomanipulation of Genetically Encoded Photoactivatable Proteins (Optogenetics) in Live Cells. Microscopy 63 (3), 255–260. doi:10.1093/jmicro/dfu003
Banderas, A., Le Bec, M., Cordier, C., and Hersen, P. (2020). Autonomous and Assisted Control for Synthetic Microbiology. Ijms 21 (23), 9223. doi:10.3390/ijms21239223
Baumschlager, A., and Khammash, M. (2021). Synthetic Biological Approaches for Optogenetics and Tools for Transcriptional Light-Control in Bacteria. Adv. Biol. 5, 2000256. doi:10.1002/adbi.202000256
Baumschlager, A., Aoki, S. K., and Khammash, M. (2017). Dynamic Blue Light-Inducible T7 Rna Polymerases (Opto-t7rnaps) for Precise Spatiotemporal Gene Expression Control. ACS Synth. Biol. 6 (11), 2157–2167. doi:10.1021/acssynbio.7b00169
Benzinger, D., and Khammash, M. (2018). Pulsatile Inputs Achieve Tunable Attenuation of Gene Expression Variability and Graded Multi-Gene Regulation. Nat. Commun. 9 (1), 3521. doi:10.1038/s41467-018-05882-2
Bergenholm, D., Liu, G., Hansson, D., and Nielsen, J. (2019). Construction of Mini‐chemostats for High‐throughput Strain Characterization. Biotechnol. Bioeng. 116 (5), 1029–1038. doi:10.1002/bit.26931
Bertaux, F., Ruess, J., and Batt, G. (2021b). External Control of Microbial Populations for Bioproduction: A Modeling and Optimization Viewpoint. Curr. Opin. Syst. Biol. 28, 100394. doi:10.1016/j.coisb.2021.100394
Bertaux, F., Sosa-Carrillo, S., Fraisse, A., Aditya, C., Furstenheim, M., and Batt, G. (2021a). Enhancing Bioreactor Arrays for Automated Measurements and Reactive Control with Reacsight. bioRxiv. doi:10.1101/2020.12.27.424467
Beyer, H. M., Juillot, S., Herbst, K., Samodelov, S. L., Müller, K., Schamel, W. W., et al. (2015). Red Light-Regulated Reversible Nuclear Localization of Proteins in Mammalian Cells and Zebrafish. ACS Synth. Biol. 4 (9), 951–958. doi:10.1021/acssynbio.5b00004
Brenker, K., Osthof, K., Yang, J., and Reth, M. (2016). LED Thermo Flow - Combining Optogenetics with Flow Cytometry. J. Vis. Exp. (118), e54707. doi:10.3791/54707
Briat, C., Gupta, A., and Khammash, M. (2016b). Antithetic Integral Feedback Ensures Robust Perfect Adaptation in Noisy Biomolecular Networks. Cell. Syst. 2 (1), 15–26. doi:10.1016/j.cels.2016.01.004
Briat, C., Zechner, C., and Khammash, M. (2016a). Design of a Synthetic Integral Feedback Circuit: Dynamic Analysis and Dna Implementation. ACS Synth. Biol. 5 (10), 1108–1116. doi:10.1021/acssynbio.6b00014
Bugaj, L. J., and Lim, W. A. (2019). High-throughput Multicolor Optogenetics in Microwell Plates. Nat. Protoc. 14 (7), 2205–2228. doi:10.1038/s41596-019-0178-y
Carrasco-López, C., García-Echauri, S. A., Kichuk, T., and Avalos, J. L. (2020). Optogenetics and Biosensors Set the Stage for Metabolic Cybergenetics. Curr. Opin. Biotechnol. 65, 296–309. doi:10.1016/j.copbio.2020.07.012
Chait, R., Ruess, J., Bergmiller, T., Tkačik, G., and Guet, C. C. (2017). Shaping Bacterial Population Behavior through Computer-Interfaced Control of Individual Cells. Nat. Commun. 8 (1), 1535. doi:10.1038/s41467-017-01683-1
Chen, C.-Y., Yeh, K.-L., Aisyah, R., Lee, D.-J., and Chang, J.-S. (2011). Cultivation, Photobioreactor Design and Harvesting of Microalgae for Biodiesel Production: a Critical Review. Bioresour. Technol. 102 (1), 71–81. doi:10.1016/j.biortech.2010.06.159
Chen, M., Mertiri, T., Holland, T., and Basu, A. S. (2012). Optical Microplates for High-Throughput Screening of Photosynthesis in Lipid-Producing Algae. Lab. Chip 12 (20), 3870–3874. doi:10.1039/c2lc40478h
Chen, S., Harrigan, P., Heineike, B., Stewart-Ornstein, J., and El-Samad, H. (2013). Building Robust Functionality in Synthetic Circuits Using Engineered Feedback Regulation. Curr. Opin. Biotechnol. 24 (4), 790–796. doi:10.1016/j.copbio.2013.02.025
Chen, S. Y., Osimiri, L. C., Chevalier, M., Bugaj, L. J., Nguyen, T. H., Greenstein, R. A., et al. (2020). Optogenetic Control Reveals Differential Promoter Interpretation of Transcription Factor Nuclear Translocation Dynamics. Cell. Syst. 11 (4), 336–e24. doi:10.1016/j.cels.2020.08.009
Davidson, E. A., Basu, A. S., and Bayer, T. S. (2013). Programming Microbes Using Pulse Width Modulation of Optical Signals. J. Mol. Biol. 425 (22), 4161–4166. doi:10.1016/j.jmb.2013.07.036
Dudley, D., Duncan, W. M., and Slaughter, J. (2003). “Emerging Digital Micromirror Device (Dmd) Applications,” in MOEMS Display and Imaging Systems (International Society for Optics and Photonics), Vol. 4985, 14–25. doi:10.1117/12.480761
Durán, D. C., Hernández, C. A., Suesca, E., Acevedo, R., Acosta, I. M., Forero, D. A., et al. (2020). Slipstreaming Mother Machine: A Microfluidic Device for Single-Cell Dynamic Imaging of Yeast. Micromachines 12 (1), 4. doi:10.3390/mi12010004
Filo, M., Kumar, S., and Khammash, M. (2022). A Hierarchy of Biomolecular Proportional-Integral-Derivative Feedback Controllers for Robust Perfect Adaptation and Dynamic Performance. Nat. Commun. 13 (1), 2119. doi:10.1038/s41467-022-29640-7
Forlani, G., and Di Ventura, B. (2021). Light Express. Curr. Opin. Syst. Biol. 28, 100356. doi:10.1016/j.coisb.2021.100356
Fox, Z. R., Fletcher, S., Fraisse, A., Aditya, C., Sosa-Carrillo, S., Gilles, S., et al. (2022). Enabling Reactive Microscopy with MicroMator. Nat. Commun, 13 (1), 1–8.
Fracassi, C., Postiglione, L., Fiore, G., and Di Bernardo, D. (2016). Automatic Control of Gene Expression in Mammalian Cells. ACS Synth. Biol. 5 (4), 296–302. doi:10.1021/acssynbio.5b00141
Gale, B., Jafek, A., Lambert, C., Goenner, B., Moghimifam, H., Nze, U., et al. (2018). A Review of Current Methods in Microfluidic Device Fabrication and Future Commercialization Prospects. Inventions 3 (3), 60. doi:10.3390/inventions3030060
Gerhardt, K. P., Olson, E. J., Castillo-Hair, S. M., Hartsough, L. A., Landry, B. P., Ekness, F., et al. (2016). An Open-Hardware Platform for Optogenetics and Photobiology. Sci. Rep. 6 (1), 35363. doi:10.1038/srep35363
Gheorghiu, M., Polonschii, C., Popescu, O., and Gheorghiu, E. (2021). Advanced Optogenetic-Based Biosensing and Related Biomaterials. Materials 14 (15), 4151. doi:10.3390/ma14154151
Gopalakrishnan, V., Krishnan, N. P., McClure, E., Pelesko, J., Crozier, D., Card, K. J., et al. (2020). A Low-Cost, Open Source, Self-Contained Bacterial Evolutionary Bioreactor (Eve). bioRxiv, 729434. doi:10.1101/729434
Grødem, E. O. S., Sweeney, K., and McClean, M. N. (2020). Automated Calibration of Optoplate Leds to Reduce Light Dose Variation in Optogenetic Experiments. Biotechniques 69 (4), 313–316. doi:10.2144/btn-2020-0077
Grosenick, L., Marshel, J. H., and Deisseroth, K. (2015). Closed-loop and Activity-Guided Optogenetic Control. Neuron 86 (1), 106–139. doi:10.1016/j.neuron.2015.03.034
Gupta, A., and Khammash, M. (2019). “An Antithetic Integral Rein Controller for Bio-Molecular Networks,” in 2019 IEEE 58th Conference on Decision and Control (CDC) (IEEE), 2808–2813. doi:10.1109/cdc40024.2019.9029864
Hannanta-Anan, P., and Chow, B. Y. (2016). Optogenetic Control of Calcium Oscillation Waveform Defines Nfat as an Integrator of Calcium Load. Cell. Syst. 2 (4), 283–288. doi:10.1016/j.cels.2016.03.010
Harrigan, P., Madhani, H. D., and El-Samad, H. (2018). Real-time Genetic Compensation Defines the Dynamic Demands of Feedback Control. Cell. 175 (3), 877–886. doi:10.1016/j.cell.2018.09.044
Hartmann, J., Krueger, D., and De Renzis, S. (2020). Using Optogenetics to Tackle Systems-Level Questions of Multicellular Morphogenesis. Curr. Opin. Cell. Biol. 66, 19–27. doi:10.1016/j.ceb.2020.04.004
Hennemann, J., Iwasaki, R. S., Grund, T. N., Diensthuber, R. P., Richter, F., and Möglich, A. (2018). Optogenetic Control by Pulsed Illumination. ChemBioChem 19 (12), 1296–1304. doi:10.1002/cbic.201800030
Hoffmann, S. A., Wohltat, C., Müller, K. M., and Arndt, K. M. (2017). A User-Friendly, Low-Cost Turbidostat with Versatile Growth Rate Estimation Based on an Extended Kalman Filter. PloS one 12 (7), e0181923. doi:10.1371/journal.pone.0181923
Jiao, Z.-F., Shang, C.-F., Wang, Y.-F., Yang, Z., Yang, C., Li, F.-N., et al. (2018). All-optical Imaging and Manipulation of Whole-Brain Neuronal Activities in Behaving Larval Zebrafish. Biomed. Opt. Express 9 (12), 6154–6169. doi:10.1364/boe.9.006154
Johnson, H. E., and Toettcher, J. E. (2018). Illuminating Developmental Biology with Cellular Optogenetics. Curr. Opin. Biotechnol. 52, 42–48. doi:10.1016/j.copbio.2018.02.003
Johnson, Z. I., and Sheldon, T. L. (2007). A High-Throughput Method to Measure Photosynthesis-Irradiance Curves of Phytoplankton. Limnol. Oceanogr. Methods 5 (11), 417–424. doi:10.4319/lom.2007.5.417
Kennedy, M. J., Hughes, R. M., Peteya, L. A., Schwartz, J. W., Ehlers, M. D., and Tucker, C. L. (2010). Rapid Blue-Light-Mediated Induction of Protein Interactions in Living Cells. Nat. Methods 7 (12), 973–975. doi:10.1038/nmeth.1524
Kim, C. K., Adhikari, A., and Deisseroth, K. (2017). Integration of Optogenetics with Complementary Methodologies in Systems Neuroscience. Nat. Rev. Neurosci. 18 (4), 222–235. doi:10.1038/nrn.2017.15
Kolar, K., Knobloch, C., Stork, H., Žnidarič, M., and Weber, W. (2018). Optobase: a Web Platform for Molecular Optogenetics. ACS Synth. Biol. 7 (7), 1825–1828. doi:10.1021/acssynbio.8b00120
Krueger, D., Izquierdo, E., Viswanathan, R., Hartmann, J., Pallares Cartes, C., and De Renzis, S. (2019). Principles and Applications of Optogenetics in Developmental Biology. Development 146 (20), dev175067. doi:10.1242/dev.175067
Kumar, S., Rullan, M., and Khammash, M. (2021). Rapid Prototyping and Design of Cybergenetic Single-Cell Controllers. Nat. Commun. 12 (1), 5651. doi:10.1038/s41467-021-25754-6
Lalwani, M. A., Ip, S. S., Carrasco-López, C., Day, C., Zhao, E. M., Kawabe, H., et al. (2021). Optogenetic Control of the Lac Operon for Bacterial Chemical and Protein Production. Nat. Chem. Biol. 17 (1), 71–79. doi:10.1038/s41589-020-0639-1
Lalwani, M. A., Zhao, E. M., and Avalos, J. L. (2018). Current and Future Modalities of Dynamic Control in Metabolic Engineering. Curr. Opin. Biotechnol. 52, 56–65. doi:10.1016/j.copbio.2018.02.007
Lee, J. M., Lee, J., Kim, T., and Lee, S. K. (2013). Switchable Gene Expression in escherichia Coli Using a Miniaturized Photobioreactor. PloS one 8 (1), e52382. doi:10.1371/journal.pone.0052382
Levskaya, A., Chevalier, A. A., Tabor, J. J., Simpson, Z. B., Lavery, L. A., Levy, M., et al. (2005). Engineering escherichia Coli to See Light. Nature 438 (7067), 441–442. doi:10.1038/nature04405
Levskaya, A., Weiner, O. D., Lim, W. A., and Voigt, C. A. (2009). Spatiotemporal Control of Cell Signalling Using a Light-Switchable Protein Interaction. Nature 461 (7266), 997–1001. doi:10.1038/nature08446
Liu, Z., Zhang, J., Jin, J., Geng, Z., Qi, Q., and Liang, Q. (2018). Programming Bacteria with Light-Sensors and Applications in Synthetic Biology. Front. Microbiol. 9, 2692. doi:10.3389/fmicb.2018.02692
Lugagne, J. B., and Dunlop, M. J. (2019). Cell-machine Interfaces for Characterizing Gene Regulatory Network Dynamics. Curr. Opin. Syst. Biol. 14 (1–8), 1–8. doi:10.1016/j.coisb.2019.01.001
Lugagne, J. B., Sosa Carrillo, S., Kirch, M., Köhler, A., Batt, G., and Hersen, P. (2017). Balancing a Genetic Toggle Switch by Real-Time Feedback Control and Periodic Forcing. Nat. Commun. 8 (1), 1671–1678. doi:10.1038/s41467-017-01498-0
Lynch, M. D. (2016). Into New Territory: Improved Microbial Synthesis through Engineering of the Essential Metabolic Network. Curr. Opin. Biotechnol. 38, 106–111. doi:10.1016/j.copbio.2016.01.009
MacDonald, I. C., and Deans, T. L. (2016). Tools and Applications in Synthetic Biology. Adv. drug Deliv. Rev. 105, 20–34. doi:10.1016/j.addr.2016.08.008
McGeachy, A. M., Meacham, Z. A., and Ingolia, N. T. (2019). An Accessible Continuous-Culture Turbidostat for Pooled Analysis of Complex Libraries. ACS Synth. Biol. 8 (4), 844–856. doi:10.1021/acssynbio.8b00529
Melendez, J., Patel, M., Oakes, B. L., Xu, P., Morton, P., and McClean, M. N. (2014). Real-time Optogenetic Control of Intracellular Protein Concentration in Microbial Cell Cultures. Integr. Biol. 6 (3), 366–372. doi:10.1039/c3ib40102b
Mertiri, Taulant., Chen, Meng., Holland, Thomas., and S Basu, Amar. (2011). “Optical Microplates for Photonic High Throughput Screening of Algal Photosynthesis and Biofuel Production,” in 2011 Annual International Conference of the IEEE Engineering in Medicine and Biology Society (IEEE), 482–485. doi:10.1109/iembs.2011.6090070
Milias-Argeitis, A., Rullan, M., Aoki, S. K., Buchmann, P., and Khammash, M. (2016). Automated Optogenetic Feedback Control for Precise and Robust Regulation of Gene Expression and Cell Growth. Nat. Commun. 7 (1), 12546. doi:10.1038/ncomms12546
Milias-Argeitis, A., Summers, S., Stewart-Ornstein, J., Zuleta, I., Pincus, D., El-Samad, H., et al. (2011). In Silico feedback for In Vivo Regulation of a Gene Expression Circuit. Nat. Biotechnol. 29 (12), 1114–1116. doi:10.1038/nbt.2018
Miller, A. W., Befort, C., Kerr, E. O., and Dunham, M. J. (2013). Design and Use of Multiplexed Chemostat Arrays. J. Vis. Exp. (72), e50262. doi:10.3791/50262
Morales, N. M., Patel, M. T., Stewart, C. J., Sweeney, K., and McClean, M. N. (2021). Optogenetic Tools for Control of Public Goods in saccharomyces Cerevisiae. Msphere 6 (4), e00581–21. doi:10.1128/msphere.00581-21
Müller, K., Naumann, S., Weber, W., and Zurbriggen, M. D. (2015). Optogenetics for Gene Expression in Mammalian Cells. Biol. Chem. 396 (2), 145–152. doi:10.1515/hsz-2014-0199
Müller, K., Zurbriggen, M. D., and Weber, W. (2014). Control of Gene Expression Using a Red- and Far-Red Light-Responsive Bi-stable Toggle Switch. Nat. Protoc. 9 (3), 622–632. doi:10.1038/nprot.2014.038
Ni, C., Dinh, C. V., and Prather, K. L. J. (2021). Dynamic Control of Metabolism. Annu. Rev. Chem. Biomol. Eng. 12, 519–541. doi:10.1146/annurev-chembioeng-091720-125738
Ochoa-Fernandez, R., Abel, N. B., Wieland, F.-G., Schlegel, J., Koch, L.-A., Miller, J. B., et al. (2020). Optogenetic Control of Gene Expression in Plants in the Presence of Ambient White Light. Nat. Methods 17 (7), 717–725. doi:10.1038/s41592-020-0868-y
Olson, E. J., Hartsough, L. A., Landry, B. P., Shroff, R., and Tabor, J. J. (2014). Characterizing Bacterial Gene Circuit Dynamics with Optically Programmed Gene Expression Signals. Nat. Methods 11 (4), 449–455. doi:10.1038/nmeth.2884
Pedone, E., De Cesare, I., Zamora-Chimal, C. G., Haener, D., Postiglione, L., La Regina, A., et al. (2021). Cheetah: a Computational Toolkit for Cybergenetic Control. ACS Synth. Biol. 10 (5), 979–989. doi:10.1021/acssynbio.0c00463
Pégard, N. C., Mardinly, A. R., Oldenburg, I. A., Sridharan, S., Waller, L., and Adesnik, H. (2017). Three-dimensional Scanless Holographic Optogenetics with Temporal Focusing (3d-Shot). Nat. Commun. 8 (1), 1228. doi:10.1038/s41467-017-01031-3
Pen, U.-Y., Nunn, C. J., and Goyal, S. (2021). An Automated Tabletop Continuous Culturing System with Multicolor Fluorescence Monitoring for Microbial Gene Expression and Long-Term Population Dynamics. ACS Synth. Biol. 10 (4), 766–777. doi:10.1021/acssynbio.0c00574
Pérez, A. L. A., Piva, L. C., Fulber, J. P. C., de Moraes, L. M. P., De Marco, J. L., Vieira, H. L. A., et al. (2021). Optogenetic Strategies for the Control of Gene Expression in Yeasts. Biotechnol. Adv. 54, 107839. doi:10.1016/j.biotechadv.2021.107839
Perkins, M. L., Benzinger, D., Arcak, M., and Khammash, M. (2020). Cell-in-the-loop Pattern Formation with Optogenetically Emulated Cell-To-Cell Signaling. Nat. Commun. 11 (1), 1355. doi:10.1038/s41467-020-15166-3
Perrino, G., Hadjimitsis, A., Ledesma-Amaro, R., and Stan, G.-B. (2021). Control Engineering and Synthetic Biology: Working in Synergy for the Analysis and Control of Microbial Systems. Curr. Opin. Microbiol. 62, 68–75. doi:10.1016/j.mib.2021.05.004
Posten, Clemens. (2009). Design Principles of Photo-Bioreactors for Cultivation of Microalgae. Eng. Life Sci. 9 (3), 165–177. doi:10.1002/elsc.200900003
Pouzet, S., Banderas, A., Le Bec, M., Lautier, T., Truan, G., and Hersen, P. (2020). The Promise of Optogenetics for Bioproduction: Dynamic Control Strategies and Scale-Up Instruments. Bioengineering 7 (4), 151. doi:10.3390/bioengineering7040151
Repina, N. A., McClave, T., Johnson, H. J., Bao, X., Kane, R. S., and Schaffer, D. V. (2020). Engineered Illumination Devices for Optogenetic Control of Cellular Signaling Dynamics. Cell. Rep. 31 (10), 107737. doi:10.1016/j.celrep.2020.107737
Repina, N. A., Rosenbloom, A., Mukherjee, A., Schaffer, D. V., and Kane, R. S. (2017). At Light Speed: Advances in Optogenetic Systems for Regulating Cell Signaling and Behavior. Annu. Rev. Chem. Biomol. Eng. 8, 13–39. doi:10.1146/annurev-chembioeng-060816-101254
Richter, F., Scheib, U. S., Mehlhorn, J., Schubert, R., Wietek, J., Gernetzki, O., et al. (2015). Upgrading a Microplate Reader for Photobiology and All-Optical Experiments. Photochem. Photobiol. Sci. 14 (2), 270–279. doi:10.1039/c4pp00361f
Rodriguez, E. A., Campbell, R. E., Lin, J. Y., Lin, M. Z., Miyawaki, A., Palmer, A. E., et al. (2017). The Growing and Glowing Toolbox of Fluorescent and Photoactive Proteins. Trends Biochem. Sci. 42 (2), 111–129. doi:10.1016/j.tibs.2016.09.010
Romano, E., Baumschlager, A., Akmeriç, E. B., Palanisamy, N., Houmani, M., Schmidt, G., et al. (2021). Engineering Arac to Make it Responsive to Light Instead of Arabinose. Nat. Chem. Biol. 17 (7), 817–827. doi:10.1038/s41589-021-00787-6
Ruess, J., Parise, F., Milias-Argeitis, A., Khammash, M., and Lygeros, J. (2015). Iterative Experiment Design Guides the Characterization of a Light-Inducible Gene Expression Circuit. Proc. Natl. Acad. Sci. U.S.A. 112 (26), 8148–8153. doi:10.1073/pnas.1423947112
Rullan, M., Benzinger, D., Schmidt, G. W., Milias-Argeitis, A., and Khammash, M. (2018). An Optogenetic Platform for Real-Time, Single-Cell Interrogation of Stochastic Transcriptional Regulation. Mol. Cell. 70 (4), 745–756. doi:10.1016/j.molcel.2018.04.012
Ruolo, I., Napolitano, S., Salzano, D., di Bernardo, M., and di Bernardo, D. (2021). Control Engineering Meets Synthetic Biology: Foundations and Applications. Curr. Opin. Syst. Biol. 28, 100397. doi:10.1016/j.coisb.2021.100397
Singh, A. P., Wu, P., Ryabichko, S., Raimundo, J., Swan, M., Wieschaus, E., et al. (2022). Optogenetic Control of the Bicoid Morphogen Reveals Fast and Slow Modes of Gap Gene Regulation. Cell. Rep. 38 (12), 110543. bioRxiv. doi:10.1016/j.celrep.2022.110543
Soffer, G., Perry, J. M., and Shih, S. C. C. (2021). Real-time Optogenetics System for Controlling Gene Expression Using a Model-Based Design. Anal. Chem. 93 (6), 3181–3188. doi:10.1021/acs.analchem.0c04594
Steel, H., Habgood, R., Kelly, C. L., and Papachristodoulou, A. (2020). In Situ characterisation and Manipulation of Biological Systems with Chi.Bio. PLoS Biol. 18 (7), e3000794. doi:10.1371/journal.pbio.3000794
Steude, A., Witts, E. C., Miles, G. B., and Gather, M. C. (2016). Arrays of Microscopic Organic Leds for High-Resolution Optogenetics. Sci. Adv. 2 (5), e1600061. doi:10.1126/sciadv.1600061
Stewart, C. J., and McClean, M. N. (2017). Design and Implementation of an Automated Illuminating, Culturing, and Sampling System for Microbial Optogenetic Applications. J. Vis. Exp. (120), e54894. doi:10.3791/54894
Takahashi, C. N., Miller, A. W., Ekness, F., Dunham, M. J., and Klavins, E. (2015). A Low Cost, Customizable Turbidostat for Use in Synthetic Circuit Characterization. ACS Synth. Biol. 4 (1), 32–38. doi:10.1021/sb500165g
Tischer, D., and Weiner, O. D. (2014). Illuminating Cell Signalling with Optogenetic Tools. Nat. Rev. Mol. Cell. Biol. 15 (8), 551–558. doi:10.1038/nrm3837
Toettcher, J. E., Gong, D., Lim, W. A., and Weiner, O. D. (2011b). “Light Control of Plasma Membrane Recruitment Using the Phy-PIF System,” in Methods in Enzymology (Elsevier), Vol. 497, 409–423. doi:10.1016/b978-0-12-385075-1.00017-2
Toettcher, J. E., Gong, D., Lim, W. A., and Weiner, O. D. (2011c). Light-based Feedback for Controlling Intracellular Signaling Dynamics. Nat. Methods 8 (10), 837–839. doi:10.1038/nmeth.1700
Toettcher, J. E., Voigt, C. A., Weiner, O. D., and Lim, W. A. (2011a). The Promise of Optogenetics in Cell Biology: Interrogating Molecular Circuits in Space and Time. Nat. Methods 8 (1), 35–38. doi:10.1038/nmeth.f.326
Toettcher, J. E., Weiner, O. D., and Lim, W. A. (2013). Using Optogenetics to Interrogate the Dynamic Control of Signal Transmission by the Ras/erk Module. Cell. 155 (6), 1422–1434. doi:10.1016/j.cell.2013.11.004
Toprak, E., Veres, A., Yildiz, S., Pedraza, J. M., Chait, R., Paulsson, J., et al. (2013). Building a Morbidostat: an Automated Continuous-Culture Device for Studying Bacterial Drug Resistance under Dynamically Sustained Drug Inhibition. Nat. Protoc. 8 (3), 555–567. doi:10.1038/nprot.2013.021
Tucker, C. L., Vrana, J. D., and Kennedy, M. J. (2014). Tools for Controlling Protein Interactions Using Light. Curr. Protoc. Cell. Biol. 64 (1), 17–20. doi:10.1002/0471143030.cb1716s64
Vázquez-Guardado, A., Yang, Y., Bandodkar, A. J., and Rogers, J. A. (2020). Recent Advances in Neurotechnologies with Broad Potential for Neuroscience Research. Nat. Neurosci. 23 (12), 1522–1536. doi:10.1038/s41593-020-00739-8
Venayak, N., Anesiadis, N., Cluett, W. R., and Mahadevan, R. (2015). Engineering Metabolism through Dynamic Control. Curr. Opin. Biotechnol. 34, 142–152. doi:10.1016/j.copbio.2014.12.022
Wang, S., Szobota, S., Wang, Y., Volgraf, M., Liu, Z., Sun, C., et al. (2007). All Optical Interface for Parallel, Remote, and Spatiotemporal Control of Neuronal Activity. Nano Lett. 7 (12), 3859–3863. doi:10.1021/nl072783t
Warden, M. R., Cardin, J. A., and Deisseroth, K. (2014). Optical Neural Interfaces. Annu. Rev. Biomed. Eng. 16, 103–129. doi:10.1146/annurev-bioeng-071813-104733
Wong, B. G., Mancuso, C. P., Kiriakov, S., Bashor, C. J., and Khalil, A. S. (2018). Precise, Automated Control of Conditions for High-Throughput Growth of Yeast and Bacteria with Evolver. Nat. Biotechnol. 36 (7), 614–623. doi:10.1038/nbt.4151
Woodruff, K., and Maerkl, S. J. (2016). A High-Throughput Microfluidic Platform for Mammalian Cell Transfection and Culturing. Sci. Rep. 6 (1), 1–12. doi:10.1038/srep23937
Xue, Y., Waller, L., Adesnik, H., and Pégard, N. (2022). Three-dimensional Multi-Site Random Access Photostimulation (3d-Map). Elife 11, e73266. doi:10.7554/eLife.73266
Ye, H., Baba, M. D.-E., Peng, R.-W., and Fussenegger, M. (2011). A Synthetic Optogenetic Transcription Device Enhances Blood-Glucose Homeostasis in Mice. Science 332 (6037), 1565–1568. doi:10.1126/science.1203535
Zhao, E. M., Lalwani, M. A., Chen, J.-M., Orillac, P., Toettcher, J. E., and Avalos, J. L. (2021). Optogenetic Amplification Circuits for Light-Induced Metabolic Control. ACS Synth. Biol. 10 (5), 1143–1154. doi:10.1021/acssynbio.0c00642
Zhao, E. M., Zhang, Y., Mehl, J., Park, H., Lalwani, M. A., Toettcher, J. E., et al. (2018). Optogenetic Regulation of Engineered Cellular Metabolism for Microbial Chemical Production. Nature 555 (7698), 683–687. doi:10.1038/nature26141
Keywords: optogenetics, optogenetic platform, light stimulation device, light-control, feedback control, in silico control, cybergenetics
Citation: Kumar S and Khammash M (2022) Platforms for Optogenetic Stimulation and Feedback Control. Front. Bioeng. Biotechnol. 10:918917. doi: 10.3389/fbioe.2022.918917
Received: 12 April 2022; Accepted: 06 May 2022;
Published: 08 June 2022.
Edited by:
Pasquale Stano, University of Salento, ItalyReviewed by:
Megan McClean, University of Wisconsin-Madison, United StatesToshihiro Kushibiki, National Defense Medical College, Japan
Copyright © 2022 Kumar and Khammash. This is an open-access article distributed under the terms of the Creative Commons Attribution License (CC BY). The use, distribution or reproduction in other forums is permitted, provided the original author(s) and the copyright owner(s) are credited and that the original publication in this journal is cited, in accordance with accepted academic practice. No use, distribution or reproduction is permitted which does not comply with these terms.
*Correspondence: Sant Kumar, c2FudC5rdW1hckBic3NlLmV0aHouY2g=; Mustafa Khammash, bXVzdGFmYS5raGFtbWFzaEBic3NlLmV0aHouY2g=