- 1Department of Synthetic Chemistry and Biological Chemistry, Graduate School of Engineering, Kyoto University, Kyoto, Japan
- 2Graduate School of Science and Engineering, Yokohama National University, Yokohama, Japan
Owing to its applicability in sustainable engineering, flow electrochemical synthesis in a proton-exchange membrane (PEM) reactor has attracted considerable attention. Because the reactions in PEM reactors are performed under electro-organic and flow-synthetic conditions, a higher number of reaction parameters exist compared to ordinary reactions. Thus, the optimization of such reactions requires significant amounts of energy, time, chemical and human resources. Herein, we show that the optimization of alkyne semihydrogenation in PEM reactors can be facilitated by means of Bayesian optimization, an applied mathematics strategy. Applying the optimized conditions, we also demonstrate the generation of a deuterated Z-alkene.
Introduction
Electrochemical synthesis, which largely entails reactions driven by electricity, has attracted considerable attention in sustainable development (Johnson et al., 2017; Moeller, 2018; Sauermann et al., 2018; Ashikari et al., 2019; Shida et al., 2019; Yano et al., 2020; Zhou et al., 2020; Dong et al., 2021). An important characteristic of electrochemical reactions is the reduction of energy losses. Because these reactions can generally be performed under mild conditions, such as room temperature and ambient pressure, energy losses such as carbon dioxide emissions can be minimized. Another characteristic is pollution reduction. Because electrosynthesis takes advantage of electricity as an oxidant and/or reductant in the place of hazardous chemical reagents, it produces less waste than other conventional chemical reactions. Therefore, electrochemical synthesis is considered a green technology owing to its mild and clean characteristics (Fuchigami and Tajima, 2006; Ibanez et al., 2014), prompting renewed interest in its development.
The proton-exchange membrane (PEM) reactor is an innovative electrolysis unit, originally developed for fuel cell technology (Smitha et al., 2005; Wang et al., 2011). The PEM reactor features many characteristics designed to overcome the disadvantages of conventional electrosynthetic processes, such as the necessity of supporting electrolytes. Moreover, because the PEM reactor is a flow reactor (Masui et al., 2019; Ashikari et al., 2020; Colella et al., 2020; Harenberg et al., 2020; Ichinari et al., 2020; Otake et al., 2020; Saito and Kobayashi, 2020; Watanabe et al., 2020; Ahn et al., 2021; Prieschl et al., 2021; Sivo et al., 2021), it offers numerous advantages, including precise control of the reaction time, the applicability of successive reactions, and scalability, which are rarely applicable in batch electrosynthesis (Elsherbini and Wirth, 2019; Noël et al., 2019; Hu et al., 2020). Therefore, various applications of PEM reactor systems utilizing electrosynthetic processes, such as the reduction of toluenes (Takano et al., 2016; Fukazawa et al., 2018) and alkenes (Ogumi et al., 1981), and asymmetric hydrogenation of α,β-unsaturated acids (Fukazawa et al., 2020), have been reported (Raoult et al., 1984; Jőrissen, 1996; Kishi et al., 2020; Fukazawa et al., 2021). In particular, the electrocatalytic hydrogenation of various alkynes in the PEM reactor is noteworthy because it allows for excellent product selectivity (Fukazawa et al., 2019; Nogami et al., 2020). It is worth mentioning that the hydrogenation reaction in the PEM reactor utilizes water as a proton source, enabling the avoidance of explosive hydrogen gas. Various Z-alkenes have been selectively synthesized via the semihydrogenation of alkynes in PEM reactors.
Therefore, because of the importance of semihydrogenation as a sustainable process and that of Z-alkenes as versatile building blocks in organic synthesis for pharmaceuticals, perfumes, and natural products (Oger et al., 2013; Chinchilla and Nájera, 2014; Vilé et al., 2016), we were interested in determining the process parameters that influence the reaction. Herein, we report our investigation of the electrocatalytic semihydrogenation of alkynes using a PEM reactor, focusing on optimization of the parameters that control the selectivity and efficiency of the electro-chemical process. Notably, we designed and developed a novel PEM reactor, which allows for reaction temperature control, to reveal the effects of the reaction parameters, including the temperature, on the reaction efficiency and selectivity. Moreover, because organo-electro synthesis involves more parameters than classical organic synthesis, it appears to provide numerous reaction-condition patterns, necessitating a time-consuming reaction optimization process. To facilitate this optimization process, which must result in energy loss reduction, we attempted to take advantage of Bayesian optimization, an iterative response surface-based global optimization allowing to predict a further point to survey (Mockus, 2011). Thus, herein, we report the detailed results of Bayesian optimization, applied to facilitate the optimization of alkyne semihydrogenation in the PEM reactor, ultimately resulting in the production of highly valued deuterated Z-alkenes (Atzrodt et al., 2018).
Materials and Methods
Materials
Chemicals were purchased from FUJIFILM Wako Pure Chemical Corporation, or Tokyo Chemical Industry Co., Ltd., and were used without further purification unless otherwise noted.
Flow Electrochemical Synthesis
DC power source (P4K8-3-LDe, MATSUSADA Precision Inc.) was used for an electrolysis. The syringe pumps (Harvard Model PHD 2000 or PHD ULTRA) equipped with gastight syringes (purchased from SGE) were used for introduction of the reaction solutions into the PEM reactor via PTFE tubes. The assembly of the gold-plated stainless type PEM reactor is according to the previously reported procedure (Fukazawa et al., 2019). The newly designed PEM reactor consisting of a titanium metal was manufactured by DFC Co., Ltd. The PEM (Nafion® NR212) was purchased from Chemours Japan Co., Ltd. and cut for the reactions (1 × 4 cm2 active area). For the reaction in the new PEM reactor, the metal on carbon was manufactured by ISHIFUKU Metal Industry Co., Ltd., and was dispersed onto a carbon-based gas diffusion layer (SIGRACET GDL39BB, SGL Carbon SE) by Chemix Inc. to make the electrode. A PTFE spacer (thickness, 0.25 mm) was manufactured by DFC Co., Ltd. The concentration of products after an electrolysis were determined by GC analyses.
Results and Discussion
Initially, we used a PEM reactor previously developed by us (Fukazawa et al., 2019). To conduct the semihydrogenation of alkynes, a solution of the alkyne was introduced into the cathodic chamber of the PEM reactor, and water was introduced into the anodic chamber. In the anodic chamber, water oxidation yields a proton, which can penetrate the PEM to reach the cathode. The proton is then reduced to an adsorbed active hydrogen (Had) on the surface of the cathode catalyst, which comprises a platinum group metal. Finally, Had reduces the alkyne to a Z-alkene (Scheme 1).
We commenced our investigation by focusing on the effect of electrochemical parameters, including the electro-catalyst materials and current density. Using Pd or Pt electrocatalysts, we conducted the flow reduction of diphenylacetylene (1) as a model substrate. As shown in Table 1, we assessed the reaction conditions based on two criteria: total current efficiency and molar selectivity for semihydrogenation. First, we used a combination of Pt cathode and anode catalysts (entries 1–3) because Pt catalysts are commonly used in hydrogenation reactions (Sawama et al., 2018). We found that Pt electrocatalysts can provide high current efficiency, but the selectivity for the Z-alkene (cis-stilbene, 2) against the alkane (dibenzyl, 3), which can be generated by an over-reduction of 2, was moderate. We considered that this moderate selectivity was due to the high reactivity of the Had species on the Pt catalyst surface (Nogami et al., 2020). Thus, we switched to a Pd catalyst (entries 4–6) and as anticipated, higher selectivities were attained. Finally, we observed that the use of Pd for both the cathode and anode catalysts (entries 7–9) afforded nearly quantitative efficiencies and high selectivities (>90%).
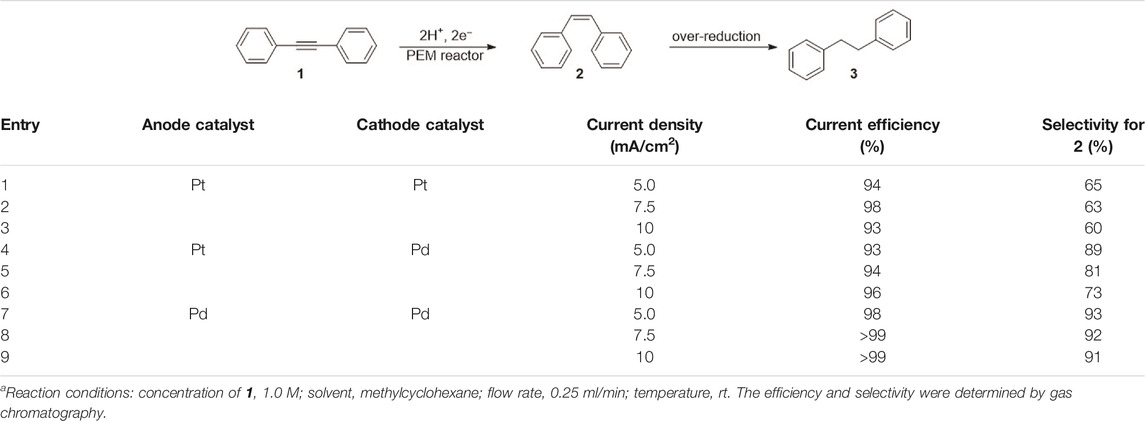
TABLE 1. Initial investigation of the semihydrogenation of 1 in the conventional PEM reactor, varying the anode, cathode, and current densitya.
The selectivity appeared to depend on the current density, and a higher current density led to a slightly lower selectivity. Moreover, in this current density range, it was nearly quantitative efficiencies. On the other hand, a higher current density appears to be beneficial for higher current efficiency. Thus, to obtain both a higher current efficiency and selectivity, we applied a current density of 7.5 mA/cm2 and Pd anode and cathode catalysts for further investigation, although the difference between the results in entries 7–9 is small.
Next, we investigated the parameters related to flow chemistry: the flow rate. As shown in Figure 1A, the flow rate (residence time) has little effect on the current efficiency. Whereas, the selectivity of 2 against 3 slightly decreased with a longer residence time. This may because that the longer residence time makes a longer-stay of the chemicals on the catalyst surface, so that the frequency of the generated 2 meeting with the Had, which leads to the over-reduction, would be increased.
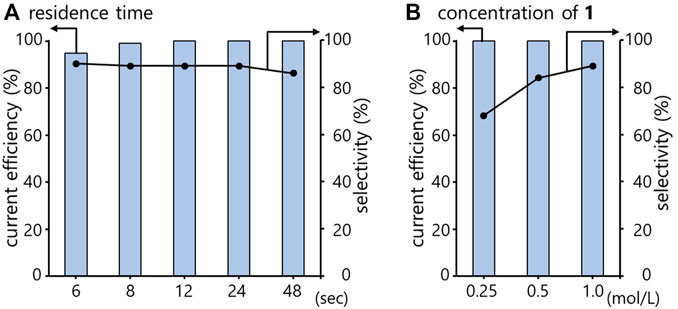
FIGURE 1. Semihydrogenation of 1 in a PEM reactor varying the residence time (A) and the concentration of 1 (B). Bar, current efficiency; dot, selectivity for 2. Conditions: solvent, methylcyclohexane; electrocatalyst, Pd; temperature, rt; current density, 7.5 mA/cm2; Concentration of 1 in (A), 1.0 M; residence time in (B), 24 s.
The effect of the substrate remaining on the catalyst surface was also confirmed by another investigation, as shown in Figure 1B. When the substrate concentration was changed, a higher concentration resulted in a higher selectivity. This may because the higher concentration of 1 lead to a plenty of the unreacted substrate, which prevents the unselective over-reduction.
During the present investigation, we found that the parameters can be classified as follows: those having a significant impact, such as the flow rate, electrocatalyst material, and concentration, and those having a minor impact, such as the current density. We hypothesized that another parameter that is likely to have an impact on the reaction would be the reaction temperature. Because the present PEM reactor was large, we expected that temperature control inside the reactor would be difficult. Thus, we designed a novel PEM reactor consisting of titanium metal (TP340) with a higher heat conductivity than the gold-plated stainless steel and carbon separator (Figure 2). The new PEM reactor was designed to be smaller than the previous, while having the same reaction space. When the reaction was performed in the new reactor, similar results to those obtained using the previous one were observed (see Supplementary Table S4 in Supporting Information). The smaller reactor shape enabled us to control the reaction temperature by attaching a heater to the reactor.
With the temperature-controllable PEM reactor in hand, we began optimizing the reaction conditions. As mentioned, we chose the parameters that had a significant impact on the reaction result (high current efficiency and selectivity), including the concentration, residence time (or flow rate), and temperature. Naturally, optimizing a reaction with multiple parameters requires considerable energy, time, and chemical and human resources. Thus, to minimize resource requirements, we decided to utilize Bayesian optimization for reaction optimization (Ahneman et al., 2018; Kondo et al., 2020; Sato et al., 2021; Shields et al., 2021; Sugisawa et al., 2021).
The optimization protocol is as follows: 1) from the initial results (data set), a candidate set of experimental conditions, including concentration, residence time, and temperature, which is expected to afford better results, is chosen by the algorithm; 2) the reaction is conducted according to the selected condition; 3) the result is added to the data set, and a new candidate set of conditions is again selected from the renewed database; 4) 2 and 3 are repeated until the chosen candidate is the same as the previous condition (Figure 3). The details of the Bayesian optimization are described in the Supporting Information.
Because both the current efficiency and product selectivity are important in semihydrogenation, we multiplied these two numbers to be an “integrated index” as an output of the experiment. This means that we aimed to maximize the integrated index using Bayesian optimization. In practice, we utilized a grid search, whereby the search space is separated as a grid (vide infra). In short, the optimized parameters were three concentrations (0.25, 0.5, and 1.0 M), three residence times (6, 12, and 24 s), and six temperatures (30, 40, 50, 60, 70, and 80°C). As an initial dataset, we had assumed using the existing results in a practical reason at first, but those reactions were done in room temperature, which seemed not suitable for the optimization. Thus, we conducted three reactions varying the concentration under the same temperature (30°C) and the same residence time (6 s), and set them as an initial dataset (shown in Supplementary Table S4 in supporting information).
Because the Bayesian algorithm generally leads to a different result based on the random number used for the algorithm, we applied the optimization protocol twice using a different random number. The results of the optimization process are summarized in Table 2. In the first trial, the algorithm suggested the same condition as second and third candidates after two experiments (or iterations). Thus, we concluded that the optimization of the first trial was completed at the third iteration, giving an integrated yield of 92% (current efficiency, 99%; selectivity, 93%). Similarly, the second trial was completed after ten iterations, affording improved results (integrated index of 96%). To confirm that this Bayesian protocol did in fact supply the optimized condition, we applied all the other conditions in the search range. The results of the 54 grid conditions are summarized in Figure 4 (details are shown in the Supporting Information). In fact, the best integrated index among these conditions was 96%, which was indicated by the second optimization trial (cycles 3 and 6), meaning that this optimization process had determined the best conditions to afford the highest integrated index.
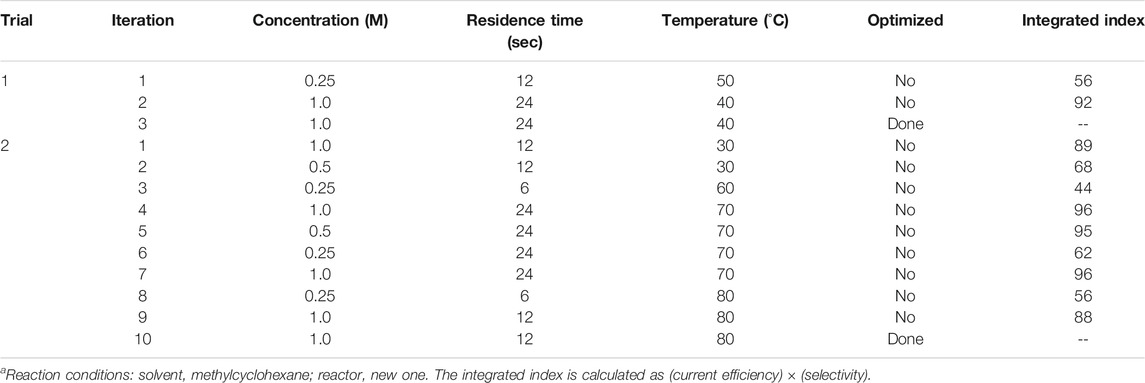
TABLE 2. Results for Bayesian optimization processa.
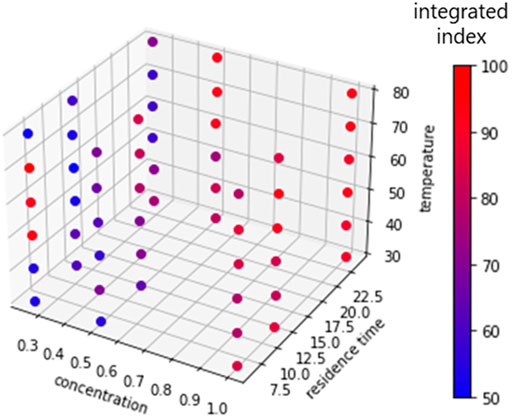
FIGURE 4. The results of the grid condition search for the semihydrogenation reaction. The integrated indexes are represented on a color scale: blue = low yield, red = high yield. See Supporting information for details.
Although we successfully optimized the reaction conditions using Bayesian, we realized that this protocol does not always yield the best result, as demonstrated in the first trial. This is due to the difference in the random number applied to the algorithm. Therefore, to assess the efficiency of the Bayesian process, we performed a statistical analysis. Because the results from all of the conditions were in hand, we simulated this optimization protocol 1,000 times using 1,000 random numbers. The result (see Supporting Information for details) indicated a 33% possibility of finding the conditions that resulted in an integrated index of >95 within five iterations. This means that the best condition (>95 integrated index) should be found during three trials of this Bayesian optimization protocol, whereby iteration is stopped within five times even though the optimization is completed. In other words, among the 51 conditions (the initial three conditions shown in Supplementary Table S4 are excluded), the expectancy of this process providing the best condition is 15 experiments.
With the optimized conditions (1.0 M, 12 s (linear velocity: 2.0 cm/min), and 70°C) in hand, our interest shifted to the robustness of this reaction. Thus, we attempted a long-time operation: electro-chemical semihydrogenation for 100 min. A current efficiency of >99% and selectivity of 98% were achieved, indicating that the PEM reactor can tolerate such a lengthy semihydrogenation under the optimized condition.
Finally, using the optimized condition, we utilized this semihydrogenation reaction to synthesize deuterated Z-alkenes (Wu et al., 2020). Among the various deuteration reactions (Atzrodt et al., 2007), the use of D2O as a deuterium source instead of D2 gas is one of the most safe and low-cost processes. After 20 min of operation using D2O, we obtained deuterated cis-stilbene 3-D, containing 93% deuterium at the alkenyl carbon (Scheme 2).
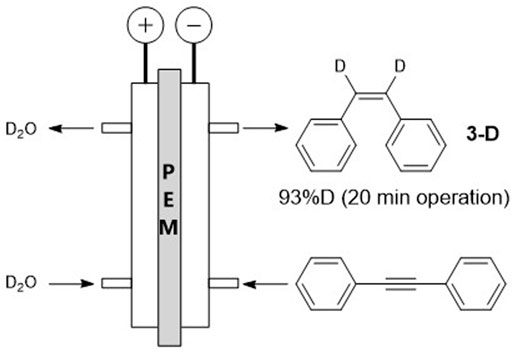
SCHEME 2. Schematic of alkyne semihydrogenation using D2O as a D source. Solvent, methylcyclohexane; concentration, 1.0 M; electrocatalyst, Pd; temperature, 70°C; current density, 7.5 mA/cm2; residence time, 12 s.
Conclusion
Using a PEM reactor, we revealed the parameters that have a significant impact on the semihydrogenation of alkynes, and showcased the efficient optimization of this reaction utilizing Bayesian optimization. Using our Bayesian protocol, the reaction was optimized within 15 experiments among 51 potential conditions. The optimized conditions allowed for long-term semihydrogenation. Moreover, the optimized conditions were applicable to reactions using deuterated water as a deuterium source, resulting in the production of deuterated Z-alkenes.
Because of its contribution to sustainability (reducing the consumption of time, chemical, and human resources, etc.), electrochemical and flow synthesis is expected to play a crucial role in the future. Despite the necessity for optimization, this process is time-consuming for electro-chemical flow reactions due to the high number of operating parameters. Our present report demonstrates the feasibility of quick optimization, which enables reduced resource requirements and promotes the sustainability of electrochemical flow reactions.
Data Availability Statement
The original contributions presented in the study are included in the article/Supplementary Material, further inquiries can be directed to the corresponding author.
Author Contributions
YA, MA and AN contributed to conception and design of the study. TT contributed to formal analysis. YA, TT, YT, and YY operated the investigation. YA wrote the first draft of the manuscript. MA and AN supervised the project. All authors revised the manuscript, approved the manuscript to be published, and agree to be accountable for all aspects of the work in ensuring that questions related to the accuracy or integrity of any part of the work are appropriately investigated and resolved.
Funding
This work was supported by the Core Research for Evolutional Science and Technology (CREST, Grant No. JPMJCR18R1). This work was also supported by JSPS KAKENHI Grant Numbers, JP20K15276 (Grant-in-Aid for Early-Career Scientists), JP20KK0121 (Fostering Joint International Research (B)), JP21H01936 (Grant-in-Aid for Scientific Research (B)), JP21H01706 (Grant-in-Aid for Scientific Research (B)), JP19K22186 (Grant-in-Aid for Challenging Exploratory Research), JP21H05080 (Grant-in-Aid for Transformative Research Areas (B)) and JP17K06910 (Grant-in-Aid for Scientific Research (C)). This work was also partially supported by AMED (JP20ak0101090 and JP21ak0101156), the Japan Science and Technology Agency’s (JST) A-step program (18067420), New Energy and Industrial Technology Development Organization (NEDO), the Japan Keirin Autorace Foundation, the Ogasawara Foundation for the Promotion of Science and Engineering, KYOTO Industrial Support Organization 21, and the Kyoto Technoscience Center.
Conflict of Interest
The authors declare that the research was conducted in the absence of any commercial or financial relationships that could be construed as a potential conflict of interest.
Publisher’s Note
All claims expressed in this article are solely those of the authors and do not necessarily represent those of their affiliated organizations, or those of the publisher, the editors, and the reviewers. Any product that may be evaluated in this article, or claim that may be made by its manufacturer, is not guaranteed or endorsed by the publisher.
Acknowledgments
The authors are grateful to Taiyo Nippon Sanso Co. for providing deuterated water and deuterium chloride. The authors are also grateful to ISHIFUKU Metal Industry Co. Ltd. for providing the palladium-carbon.
Supplementary Material
The Supplementary Material for this article can be found online at: https://www.frontiersin.org/articles/10.3389/fceng.2021.819752/full#supplementary-material
References
Ahn, G.-N., Sharma, B. M., Lahore, S., Yim, S.-J., Vidyacharan, S., and Kim, D.-P. (2021). Flow Parallel Synthesizer for Multiplex Synthesis of Aryl Diazonium Libraries via Efficient Parameter Screening. Commun. Chem. 4, 53. doi:10.1038/s42004-021-00490-6
Ahneman, D. T., Estrada, J. G., Lin, S., Dreher, S. D., and Doyle, A. G. (2018). Predicting Reaction Performance in C-N Cross-Coupling Using Machine Learning. Science 360, 186–190. doi:10.1126/science.aar5169
Ashikari, Y., Kawaguchi, T., Mandai, K., Aizawa, Y., and Nagaki, A. (2020). A Synthetic Approach to Dimetalated Arenes Using Flow Microreactors and the Switchable Application to Chemoselective Cross-Coupling Reactions. J. Am. Chem. Soc. 142, 17039–17047. doi:10.1021/jacs.0c06370
Ashikari, Y., Saito, K., Nokami, T., Yoshida, J. i., and Nagaki, A. (2019). Oxo‐Thiolation of Cationically Polymerizable Alkenes Using Flow Microreactors. Chem. Eur. J. 25, 15239–15243. doi:10.1002/chem.201903426
Atzrodt, J., Derdau, V., Fey, T., and Zimmermann, J. (2007). The Renaissance of H/D Exchange. Angew. Chem. Int. Ed. 46, 7744–7765. doi:10.1002/anie.200700039
Atzrodt, J., Derdau, V., Kerr, W. J., and Reid, M. (2018). Deuterium- and Tritium-Labelled Compounds: Applications in the Life Sciences. Angew. Chem. Int. Ed. 57, 1758–1784. doi:10.1002/anie.201704146
Chinchilla, R., and Nájera, C. (2014). Chemicals from Alkynes with Palladium Catalysts. Chem. Rev. 114, 1783–1826. doi:10.1021/cr400133p
Colella, M., Tota, A., Takahashi, Y., Higuma, R., Ishikawa, S., Degennaro, L., et al. (2020). Fluoro‐Substituted Methyllithium Chemistry: External Quenching Method Using Flow Microreactors. Angew. Chem. Int. Ed. 59, 10924–10928. doi:10.1002/anie.202003831
Dong, X., Roeckl, J. L., Waldvogel, S. R., and Morandi, B. (2021). Merging Shuttle Reactions and Paired Electrolysis for Reversible Vicinal Dihalogenations. Science 371, 507–514. doi:10.1126/science.abf2974
Elsherbini, M., and Wirth, T. (2019). Electroorganic Synthesis under Flow Conditions. Acc. Chem. Res. 52, 3287–3296. doi:10.1021/acs.accounts.9b00497
Fuchigami, T., and Tajima, T. (2006). Development of New Methodologies toward Green Sustainable Organic Electrode Processes. Electrochemistry 74, 585–589. doi:10.5796/electrochemistry.74.585
Fukazawa, A., Minoshima, J., Tanaka, K., Hashimoto, Y., Kobori, Y., Sato, Y., et al. (2019). A New Approach to Stereoselective Electrocatalytic Semihydrogenation of Alkynes to Z-Alkenes Using a Proton-Exchange Membrane Reactor. ACS Sustain. Chem. Eng. 7, 11050–11055. doi:10.1021/acssuschemeng.9b01882
Fukazawa, A., Shimizu, Y., Shida, N., and Atobe, M. (2021). Electrocatalytic Hydrogenation of Benzoic Acids in a Proton-Exchange Membrane Reactor. Org. Biomol. Chem. 19, 7367. doi:10.1039/d1ob01197a
Fukazawa, A., Takano, K., Matsumura, Y., Nagasawa, K., Mitsushima, S., and Atobe, M. (2018). Electrocatalytic Hydrogenation of Toluene Using a Proton Exchange Membrane Reactor: Influence of Catalyst Materials on Product Selectivity. Bcsj 91, 897–899. doi:10.1246/bcsj.20180021
Fukazawa, A., Tanaka, K., Hashimoto, Y., Sato, Y., and Atobe, M. (2020). Electrocatalytic Asymmetric Hydrogenation of α,β-unsaturated Acids in a PEM Reactor with Cinchona-Modified Palladium Catalysts. Electrochemistry Commun. 115, 106734. doi:10.1016/j.elecom.2020.106734
Harenberg, J. H., Weidmann, N., Karaghiosoff, K., and Knochel, P. (2020). Continuous Flow Sodiation of Substituted Acrylonitriles, Alkenyl Sulfides and Acrylates. Angew. Chem. Int. Ed. 60, 731–735. doi:10.1002/anie.202012085
Hu, P., Peters, B. K., Malapit, C. A., Vantourout, J. C., Wang, P., Li, J., et al. (2020). Electroreductive Olefin-Ketone Coupling. J. Am. Chem. Soc. 142, 20979–20986. doi:10.1021/jacs.0c11214
Ibanez, J. G., Fitch, A., Frontana-Uribe, B. A., and Vasquez-Medrano, R. (2014). “Green Electrochemistry,” in Encyclopedia of Applied Electrochemistry. Editors G. Kreysa, K. Ora, and R.F. Savinell (New York, NY: Springer), 964–971. doi:10.1007/978-1-4419-6996-5_132
Ichinari, D., Ashikari, Y., Mandai, K., Aizawa, Y., Yoshida, J. i., and Nagaki, A. (2020). A Novel Approach to Functionalization of Aryl Azides through the Generation and Reaction of Organolithium Species Bearing Masked Azides in Flow Microreactors. Angew. Chem. Int. Ed. 59, 1567–1571. doi:10.1002/anie.201912419
Johnson, B. M., Francke, R., Little, R. D., and Berben, L. A. (2017). High Turnover in Electro-Oxidation of Alcohols and Ethers with a Glassy Carbon-Supported Phenanthroimidazole Mediator. Chem. Sci. 8, 6493–6498. doi:10.1039/c7sc02482g
Jőrissen, J. (1996). Ion Exchange Membranes as Solid Polymer Electrolytes (SPE) in Electro-Organic Syntheses without Supporting Electrolyte. Electrochim. Acta 41, 553-562. doi:10.1016/0013-4686(95)00342-8
Kishi, R., Ogihara, H., Yoshida-Hirahara, M., Shibanuma, K., Yamanaka, I., and Kurokawa, H. (2020). Green Synthesis of Methyl Formate via Electrolysis of Pure Methanol. ACS Sustain. Chem. Eng. 8, 11532–11540. doi:10.1021/acssuschemeng.0c02281
Kondo, M., Wathsala, H. D. P., Sako, M., Hanatani, Y., Ishikawa, K., Hara, S., et al. (2020). Exploration of Flow Reaction Conditions Using Machine-Learning for Enantioselective Organocatalyzed Rauhut-Currier and [3+2] Annulation Sequence. Chem. Commun. 56, 1259–1262. doi:10.1039/c9cc08526b
Masui, S., Manabe, Y., Hirao, K., Shimoyama, A., Fukuyama, T., Ryu, I., et al. (2019). Kinetically Controlled Fischer Glycosidation under Flow Conditions: A New Method for Preparing Furanosides. Synlett 30, 397–400. doi:10.1055/s-0037-1611643
Moeller, K. D. (2018). Using Physical Organic Chemistry to Shape the Course of Electrochemical Reactions. Chem. Rev. 118, 4817–4833. doi:10.1021/acs.chemrev.7b00656
Noël, T., Cao, Y., and Laudadio, G. (2019). The Fundamentals behind the Use of Flow Reactors in Electrochemistry. Acc. Chem. Res. 52, 2858–2869. doi:10.1021/acs.accounts.9b00412
Nogami, S., Nagasawa, K., Fukazawa, A., Tanaka, K., Mitsushima, S., and Atobe, M. (2020). Highly Selective and Efficient Electrocatalytic Semihydrogenation of Diphenylacetylene in a PEM Reactor with Pt-Pd Alloy Cathode Catalysts. J. Electrochem. Soc. 167, 155506. doi:10.1149/1945-7111/abaae7
Oger, C., Balas, L., Durand, T., and Galano, J.-M. (2013). Are Alkyne Reductions Chemo-, Regio-, and Stereoselective Enough to Provide Pure (Z)-Olefins in Polyfunctionalized Bioactive Molecules? Chem. Rev. 113, 1313–1350. doi:10.1021/cr3001753
Ogumi, Z., Nishio, K., and Yoshizawa, S. (1981). Application of the Spe Method to Organic Electrochemistry-II. Electrochemical Hydrogenation of Olefinic Double Bonds. Electrochimica Acta 26, 1779–1782. doi:10.1016/0013-4686(81)85163-8
Otake, Y., Shibata, Y., Hayashi, Y., Kawauchi, S., Nakamura, H., and Fuse, S. (2020). N‐Methylated Peptide Synthesis via Generation of an Acyl N‐Methylimidazolium Cation Accelerated by a Brønsted Acid. Angew. Chem. Int. Ed. 59, 12925–12930. doi:10.1002/anie.202002106
Prieschl, M., Cantillo, D., and Kappe, C. O. (2021). A Continuous Flow Bromodimethylsulfonium Bromide Generator: Application to the Synthesis of 2-Arylaziridines from Styrenes. J. Flow Chem. 11, 117–125. doi:10.1007/s41981-020-00125-2
Raoult, E., Sarrazin, J., and Tallec, A. (1984). Use of Ion Exchange Membranes in Preparative Organic Electrochemistry. L Anodic Methoxylation of Some Olefins. J. Appl. Electrochem. 14, 639–643. doi:10.1007/bf00626308
Saito, Y., and Kobayashi, S. (2020). Development of Robust Heterogeneous Chiral Rhodium Catalysts Utilizing Acid-Base and Electrostatic Interactions for Efficient Continuous-Flow Asymmetric Hydrogenations. J. Am. Chem. Soc. 142, 16546–16551. doi:10.1021/jacs.0c08109
Sato, E., Fujii, M., Tanaka, H., Mitsudo, K., Kondo, M., Takizawa, S., et al. (2021). Application of an Electrochemical Microflow Reactor for Cyanosilylation: Machine Learning-Assisted Exploration of Suitable Reaction Conditions for Semi-large-scale Synthesis. J. Org. Chem. 86, 16035–16044. doi:10.1021/acs.joc.1c01242
Sauermann, N., Meyer, T. H., Qiu, Y., and Ackermann, L. (2018). Electrocatalytic C-H Activation. ACS Catal. 8, 7086–7103. doi:10.1021/acscatal.8b01682
Sawama, Y., Park, K., Yamada, T., and Sajiki, H. (2018). New Gateways to the Platinum Group Metal-Catalyzed Direct Deuterium-Labeling Method Utilizing Hydrogen as a Catalyst Activator. Chem. Pharm. Bull. 66, 21–28. doi:10.1248/cpb.c17-00222
Shida, N., Imada, Y., Nagahara, S., Okada, Y., and Chiba, K. (2019). Interplay of Arene Radical Cations with Anions and Fluorinated Alcohols in Hole Catalysis. Commun. Chem. 2, 24. doi:10.1038/s42004-019-0125-4
Shields, B. J., Stevens, J., Li, J., Parasram, M., Damani, F., Alvarado, J. I. M., et al. (2021). Bayesian Reaction Optimization as a Tool for Chemical Synthesis. Nature 590, 89–96. doi:10.1038/s41586-021-03213-y
Sivo, A., Galaverna, R. d. S., Gomes, G. R., Pastre, J. C., and Vilé, G. (2021). From Circular Synthesis to Material Manufacturing: Advances, Challenges, and Future Steps for Using Flow Chemistry in Novel Application Area. React. Chem. Eng. 6, 756–786. doi:10.1039/d0re00411a
Smitha, B., Sridhar, S., and Khan, A. A. (2005). Solid Polymer Electrolyte Membranes for Fuel Cell Applications-A Review. J. Membr. Sci. 259, 10–26. doi:10.1016/j.memsci.2005.01.035
Sugisawa, N., Sugisawa, H., Otake, Y., Krems, R. V., NakamuraFuse, H. S., and Fuse, S. (2021). Rapid and Mild One‐Flow Synthetic Approach to Unsymmetrical Sulfamides Guided by Bayesian Optimization. Chem. Methods 1, 484–490. doi:10.1002/cmtd.202100053
Takano, K., Tateno, H., Matsumura, Y., Fukazawa, A., Kashiwagi, T., Nakabayashi, K., et al. (2016). Electrocatalytic Hydrogenation of O-Xylene in a PEM Reactor as a Study of a Model Reaction for Hydrogen Storage. Chem. Lett. 45, 1437–1439. doi:10.1246/cl.160766
Vilé, G., Albani, D., Almora-Barrios, N., López, N., and Pérez-Ramírez, J. (2016). Advances in the Design of Nanostructured Catalysts for Selective Hydrogenation. ChemCatChem 8, 21–33. doi:10.1002/cctc.201501269
Wang, Y., Chen, K. S., Mishler, J., Cho, S. C., and Adroher, X. C. (2011). A Review of Polymer Electrolyte Membrane Fuel Cells: Technology, Applications, and Needs on Fundamental Research. Appl. Energ. 88, 981–1007. doi:10.1016/j.apenergy.2010.09.030
Watanabe, E., Chen, Y., May, O., and Ley, S. V. (2020). A Practical Method for Continuous Production of sp3‐Rich Compounds from (Hetero)Aryl Halides and Redox‐Active Esters. Chem. Eur. J. 26, 186–191. doi:10.1002/chem.201905048
Wu, Y., Liu, C., Wang, C., Lu, S., and Zhang, B. (2020). Selective Transfer Semihydrogenation of Alkynes with H 2 O (D 2 O) as the H (D) Source over a Pd‐P Cathode. Angew. Chem. Int. Ed. 59, 21170–21175. doi:10.1002/anie.202009757
Yano, K., Itoh, T., and Nokami, T. (2020). Total Synthesis of Myc-Iv(c16:0, S) via Automated Electrochemical Assembly. Carbohydr. Res. 492, 108018. doi:10.1016/j.carres.2020.108018
Keywords: electrosynthesis, proton-exchange membrane reactor, electrocatalyst, semihydrogenation, Bayesian optimization
Citation: Ashikari Y, Tamaki T, Takahashi Y, Yao Y, Atobe M and Nagaki A (2022) Investigation of Parameter Control for Electrocatalytic Semihydrogenation in a Proton-Exchange Membrane Reactor Utilizing Bayesian Optimization. Front. Chem. Eng. 3:819752. doi: 10.3389/fceng.2021.819752
Received: 22 November 2021; Accepted: 29 December 2021;
Published: 26 January 2022.
Edited by:
Zhansheng Wu, Xi’an Polytechnic University, ChinaReviewed by:
Luis Fernando Arenas, Clausthal University of Technology, GermanyPeng Sun, Lanzhou Institute of Chemical Physics (CAS), China
Copyright © 2022 Ashikari, Tamaki, Takahashi, Yao, Atobe and Nagaki. This is an open-access article distributed under the terms of the Creative Commons Attribution License (CC BY). The use, distribution or reproduction in other forums is permitted, provided the original author(s) and the copyright owner(s) are credited and that the original publication in this journal is cited, in accordance with accepted academic practice. No use, distribution or reproduction is permitted which does not comply with these terms.
*Correspondence: Aiichiro Nagaki, YW5hZ2FraUBzYmNoZW0ua3lvdG8tdS5hYy5qcA==