- 1Department of Society and Conservation, W. A. Franke College of Forestry and Conservation, University of Montana, Missoula, MT, United States
- 2Montana Climate Office, W. A. Franke College of Forestry and Conservation, University of Montana, Missoula, MT, United States
- 3Department of Forest Management, W. A. Franke College of Forestry and Conservation, University of Montana, Missoula, MT, United States
Growing demand for water resources coupled with climate-driven water scarcity and variability present critical challenges to agriculture in the Western US. Despite extensive resources allocated to downscaling climate projections and advances in understanding past, current, and future climatic conditions, climate information is underutilized in decisions made by agricultural producers. Climate information providers need to understand why this information is underutilized and what would better meet the needs of producers. To better understand how agricultural producers perceive and utilize climate information, we conducted five focus groups with farmers and ranchers across Montana. Focus groups revealed that there are fundamental scalar issues (spatial and temporal) that make climate information challenging for producers to use. While climate information is typically produced at regional, national, or global spatial scales and at a seasonal and mid- to end-of-century temporal scales, producers indicated that decision-making takes place at multiple intermediate and small temporal and spatial scales. In addition, producers described other drivers of decision-making that have little to do with climate information itself, but rather aspects of source credibility, past experience, trust in information, and the politics of climate change. Through engaging directly with end-users, climate information providers can better understand the spatial and temporal scales that align with different types of agricultural producers and decisions, as well as the limitations of information provision given the complexity of the decision context. Increased engagement between climate information providers and end-users can also address the important tradeoffs that exist between scale and uncertainty.
Introduction
Growing demand for water resources coupled with climate-driven water scarcity and variability present critical challenges to agriculture and food production, with impacts to food security, rural livelihoods, and ecosystem services. In the context of climate change, many regions will likely experience changes in precipitation patterns and increasingly frequent and/or intense drought (Trenberth, 2011; Dai, 2012; Trenberth and Dai, 2014; Cook et al., 2015; Kuwayama et al., 2019). Agricultural producers are particularly vulnerable to drought and other changes to water resources, especially in the US West where drought is prevalent (Mount et al., 2016; Ziolkowska, 2016; Kuwayama et al., 2019) (we use the term agricultural producers to encompass both farmers and ranchers, who are hereafter referred to as “producers”). For instance, in fiscal year 2014, the federal government designated $873 million across 11 western states for drought-related crop insurance and programs that were a result of drought emergency declarations (Mount et al., 2016).
To address these challenges, many scientists have suggested that climate information can help producers make better decisions and reduce risk in the face of drought and climate variability (Hewitt et al., 2012; Tall et al., 2014; Vaughan and Dessai, 2014). Extensive resources have been allocated to develop and disseminate climate information, including downscaled climate projections and improved information on past, current, and future climatic conditions. Despite these efforts, numerous studies have found that climate information is often underutilized in decisions made by agricultural producers (Mase and Prokopy, 2014; Haigh et al., 2015; Davis et al., 2016). This is due, in part, to a well-documented disconnect between the specific climatic information being produced and the utility of that information for decision-makers (Dilling and Lemos, 2011; Lemos et al., 2012; Preston et al., 2013; Dunne et al., 2015). As McCrea et al. (2005) suggest, just because climate information appears to be useful, does not mean it will be used.
While climate information that is readily available to agricultural producers is extensive, information that is specifically designed to aid agricultural producers in decision-making is limited. Across numerous studies, the barriers to utilizing climate information most often cited by agricultural producers are perceptions of low accuracy and concerns that information is not provided at useful temporal or spatial scales (Mase and Prokopy, 2014). Climate information is, by in large, produced at a regional, national, or global spatial scales and at a seasonal and mid-end of century temporal scales. Yet, agricultural decisions take place at multiple intermediate and smaller scales, and involve a diversity of producers whose interests and values are as diverse as the landscapes they manage.
Addressing these scalar mismatches is not an easy task. There are many challenges associated with communicating the science of climate change and this is particularly evident in the agricultural sector (Rejesus et al., 2013; Wilke and Morton, 2015). Model skill varies widely across different spatial and temporal scales, which means the accuracy of forecasts changes as scale changes (e.g., Giorgi, 2002). Further, scalar mismatches interact with other reasons for underutilization, including issues related to salience, credibility, and legitimacy. Thus, climate information providers are not only challenged by limits to the accuracy and certainty of current science, but also the broader decision context within which climate information is considered. As Wilke and Morton (2015) state, science does not “speak for itself” and there is great opportunity for scientists and science communicators to better interpret and translate complex climate information.
Drawing on focus groups with farmers and ranchers in Montana, we examine a number of scalar issues related to the utility of climate information for agricultural producers. We also consider how climate information providers might address these challenges to make forecasts and projections more relevant to agriculture and to better meet the needs of end-users.
Challenges Related to Temporal Scale
Understanding and projecting climate trends at a range of temporal scales (5-year, 10-year, mid-century, and end-of-century) is critical to maintaining the vitality of Montana's agricultural sector. Changes in temperature and precipitation are key aspects of climate that affect snowpack, water availability, plant available water (e.g., soil moisture), soil health, crop yields, and other factors important to agricultural production and livelihoods (Whitlock et al., 2017; USGCRP, 2018; IPCC, 2019).
Despite the increasing availability of climate information, there is a well-established mismatch between the temporal scales in which it is produced and the temporal scales of agricultural decision-making (Mase and Prokopy, 2014; Dunne et al., 2015). In other words, the temporal resolution of climate information does not always align with that of specific decisions. While many important agricultural decisions are made several months in advance and can benefit from climate information such as 3-month seasonal climate forecasts, the time horizon for decisions changes throughout the year, and differs based on type of operation and type of decision (Diehl et al., 2015; Haigh et al., 2015). For example, decisions about irrigating may be made on a daily or weekly basis, decisions about what crop to plant might be made several months in advance, and decisions about irrigation infrastructure may be considered for many years. While producers make both short-term tactical decisions and longer-term strategic decisions (Hollinger, 1991), they tend to focus on shorter timeframes and the near future (Stokes and Howden, 2010; Heal and Millner, 2014; Dunne et al., 2015). Thus, longer-term climate information about, such as mid- or end of century projections, may not be at a temporal scale that is not relevant to agricultural producer decision-making.
Even 3-month seasonal climate forecasts (SCFs) are underutilized in decision-making (Lemos et al., 2012; Mase and Prokopy, 2014; Reeves et al., 2015; Davis et al., 2016; Soares and Dessai, 2016). Seasonal climate forecasts predict future conditions at the seasonal scale (e.g., 3 months in advance) and typically include probabilities to indicate level of certainty (Rickards et al., 2014; Davis et al., 2016). The assumption is that SCFs enable producers to improve short-term coping, minimize risk, take advantage of favorable conditions, and prepare for variability (Ash et al., 2007; Rickards et al., 2014). Several model-based studies have demonstrated that use of SCFs can increase profitability as compared with reliance on knowledge of past conditions and more conventional decision-making (see Meza et al., 2008 for a review of these studies) and may help producers move from short-term coping to adaptation to longer-term climate risks (Tubiello et al., 2007). Yet, despite efforts from the scientific community, SCFs have not been widely adopted by producers (McCrea et al., 2005; Lemos et al., 2012; Mase and Prokopy, 2014).
Not only is some climate information less suited to the shorter time-frames of agricultural decision-making, there is often a disconnect in the timing of climate information and when producers are making decisions (Ash et al., 2007; Furman et al., 2011). As Lemos et al. (2012) argue, climate information must be timely to be useful. For example, for decisions made 6–9 months in advance, such as whether to calve in late winter or late spring, 3-month seasonal climate forecasts have little utility. On the other hand, too much lead time may limit utilization (Nyamekye et al., 2020). Put simply, climate information cannot be utilized in decision-making if it is not available when producers are making key decisions. However, using decision calendars to identify the timing of various key decisions among farmers in the U.S. Corn Belt, Haigh et al. (2015) found that there are opportunities for providing additional climate information to producers at key times during the year.
The relationship between temporal scale and accuracy is also important. Perceptions of accuracy (or lack thereof) also lead producers to emphasize shorter-term weather forecasts over longer-term climate forecasts. For example, Crane et al. (2010) found that farmers in Georgia stressed the importance of weather information (such as for the next week), which they often check on a daily basis during the growing season, relative to seasonal climate forecasts, because the latter were perceived to have lower accuracy.
The lack of confidence that producers have in SCFs is not unfounded. As one example, the 2017 flash drought across Montana and the High Plains region was not anticipated by SCFs, which did not predict the well below-normal precipitation in May–July (Jencso et al., 2019; Hoell et al., 2020). A flash drought is “an unusually rapid onset drought event characterized by a multi-week period of accelerated intensification that culminates in impacts to one or more sectors,” such as agricultural or hydrological impacts (Pendergrass et al., 2020). Temperature and precipitation forecasts are typically most accurate up to lead times of 1 month and 2 weeks, respectively (Lavers et al., 2009; Yuan et al., 2011) and there is a need to improve accuracy of the 2 and 3-month precipitation and temperature forecasts (Hoell et al., 2020).
Further, producers have indicated that information on climate trends for the next 10 years would be particularly useful (Meehl et al., 2009; Dunne et al., 2015). But there is a paucity of 10-year predictions (Meehl et al., 2009), partially due to the uncertainty inherent in climate projections at this timescale. However, while accuracy is a concern for both producers and climate information providers, some studies suggest that the timing and timeframe of forecasts may be just as important to adoption as improving accuracy (Haigh et al., 2015).
Challenges Related to Spatial Scale
Climate data is predominantly produced and communicated at regional, climate zone, state, national, and global spatial scales (e.g., Whitlock et al., 2017; USGCRP, 2018). However, agricultural producers typically make decisions at the scale of the pasture, field, farm, and/or ranch. Whitlock et al. (2017), for example, aggregates temperature and precipitation trends as well as mid-end of century projections into seven climate divisions, based on climatic, political, agricultural, and watershed boundaries. The average size of these climate divisions is 13 million acres, compared with the average size of a farm/ranch in the state which is 2,156 acres (USDA Ag Facts – Montana, 2018). Climate information at such large spatial scales often fails to capture the specific “on-the-ground” micro-climates of a particular farm or ranch, and thus information at this scale may be less useful for agricultural producers. For example, in highly heterogeneous landscapes like western rangelands, the predictive power of climate models remains to be demonstrated (Sayre et al., 2012; Sayre, 2017).
Further, the appropriate spatial scale varies with the type of decision and type of operation. For example, Reeves et al. (2015) found that while SCFs are at a spatial scale that is often not useful to ranchers, large-scale forecasts like SCFs can inform yearly decisions about stocking rates. Dunne et al. (2015) found that while SCFs needed to be at a smaller scale, like field or farm/ranch to be useful, 10-year projections can be useful at a larger spatial scale. Research with federal land managers and municipal managers also indicates that climate information is often produced at scales that are not relevant to decision-makers (Archie et al., 2014).
Finally, as with temporal scale, there are important trade-offs associated with downscaling global climate projections to finer spatial resolutions. In areas like Montana, where the landscape is complex, higher resolution, small-scale outputs are needed to capture changes in terrain and vegetation (Abatzoglou and Brown, 2012). However, uncertainty often increases when projections from global climate models (GCMs) are downscaled from regional to local scales (Gay and Estrada, 2010). The complex relationships between land, ocean, and atmosphere that are captured by global climate models (GCMs) operate at very large scales. But the processes that are relevant at smaller spatial scales, such as the way topography influences temperature and precipitation, are inherently more variable and heterogeneous and thus more difficult to capture in GCMs. Thus, more localized or small-scale processes are difficult to account for in GCMs and downscaled projections can be more uncertain at increasingly smaller scales if they don't adequately represent the relevant atmospheric and hydrologic processes.
How Other Reasons for Underutilization Interact With Scale
Beyond temporal and spatial mismatches, to be useful, climate information needs to be salient (relevant to the needs of decision-makers), credible (scientifically robust), and legitimate (unbiased and respectful of different views) (Cash et al., 2006). Simply “fixing” the scalar mismatches will not fully address these needs, because the process by which science influences action is complex (Wynne, 2006). Climate information enters into a dynamic decision context, influenced by myriad local and non-local forces, including on-farm finances and livelihood goals, the cost of inputs and commodity prices, institutional supports and policy constraints, local knowledge, social norms and networks, risk perceptions, climate beliefs, management practices, and biophysical conditions (Dessai et al., 2009; Crane et al., 2010; Furman et al., 2011; Yung et al., 2015). Thus, decisions about how to respond to and prepare for drought and climate variability, including if and how to use climate information, are embedded within complex interactions between the household, community, market, government, and environment (Meinke et al., 2009; Crane et al., 2010). Because different decisions require climate information at different temporal and spatial scales, the “fit” between scale and decision context is particularly important, but also quite complex.
In addition, features of the climate information can limit adoption, including perceptions of low forecast accuracy, forecasts presented out of context which reduced farmers' ability to apply them, and forecasts that are difficult to understand (McCrea et al., 2005; Mase and Prokopy, 2014). Perceptions of source credibility, the credibility of the information provider, can influence how and if climate information is translated into action (Dong et al., 2018). Perceptions of the accuracy of past forecasts and the trustworthiness of climate information providers both contribute to source credibility (Cash et al., 2006, Pannell and Vanclay, 2011).
Because there are trade-offs associated with model skill and scale, the temporal and spatial scale of information may influence the credibility and thus the use of climate information.
However, Dunne et al. (2015) found that perceptions of low accuracy did not prevent producers from using season climate forecasts in decision-making. Further, acknowledging uncertainty and effectively communicating that uncertainty can increase perceptions of credibility and legitimacy (Murphy et al., 2016). Thus, as Haigh et al. (2015) argue, fitting the forecast to the decision context may be just as important as improving accuracy.
Materials and Methods
This research is part of the Montana Drought and Climate project, which aims to improve the efficacy of climate information for Montana farmers and ranchers. As recommended by Gold et al. (2013), Montana Drought and Climate includes an interdisciplinary team from the natural and social sciences and employs an iterative process that directly engages with the end-users of climate information. As part of this project, we conducted focus groups with farmers and ranchers in Montana to understand their past experience with drought, their perceptions and use of climate information, and the decision context within which they operate. We use the term agricultural producer or “producer” throughout this paper to include both farmers and ranchers.
Study Sites
Research was conducted in Montana because the state has a diversity of agricultural operations and a range of hydrometeorological conditions. An increase of 1.5–4.5 degree Celsius is projected for Montana by the end of the century (RCP 4.5 scenario). Precipitation is projected to increase during winter and spring, and decrease during summer (analysis from MACAv2-METDATA-CMIP5 data; Abatzoglou and Brown, 2012). Decreases in summer precipitation, along with the loss of low-elevation snowpack from rising temperatures, will likely shift the timing of peak runoff and reduce base flows in many of the rivers around the state. In addition, changes from a snow-dominated system to a more rain-dominated system during late winter and early spring will further alter the historic hydrograph. This shift in timing will have significant impacts on reservoir management, irrigation demands, and overall water supply within the region1. These changes also impact soil moisture and can lead to late-summer landscape-scale aridification (Seneviratne et al., 2010). With increasing temperatures across the state, years with precipitation deficits will experience more intense and longer lasting droughts. Furthermore, the co-occurrence of warm and dry conditions have been projected to increase, making drought not only more intense but also more likely to occur (Diffenbaugh et al., 2015). Whitlock et al. (2017) predicts increases of 20% in the interannual variability of precipitation across the state by the end of the century. This suggests that wet years are getting wetter and/or dry years are getting drier, and that there will be larger year to year fluctuations with some years being very wet and some years very dry, more so than in the past, making management decisions for farmers and ranchers that much more challenging (Whitlock et al., 2017).
In Montana, there are ~28,000 farmers and ranchers who contribute $2.4 billion to the state's annual economy (Montana Department of Agriculture, 2016). Producers make up about 5% of the population and 17% of state's population is employed in agriculture-related jobs (Montana Department of Agriculture, 2016). The state has 17 million acres of irrigated and dryland cropland and 40 million acres dedicated to livestock grazing. Most ranches in the state are native rangeland beef cattle cow-calf operations (Montana Department of Agriculture, 2016). Montana's primary crops are wheat, hay, and barley, but farmers also grow alfalfa, apples, canola, cherries, corn, flax, garlic, oats, potatoes, pulses, safflower, and sugar beets (Montana State Water Plan, 2015; Montana Department of Agriculture, 2016). Like most of the Western U.S., agriculture is the largest consumptive user of water in Montana, using ~10.4 million acre feet of water, for a total of 96% of all surface and groundwater use (Montana State Water Plan, 2015). Competition for limited water resources continues to grow due to energy development, municipal growth, and rural residential development.
While reservoir storage and irrigation offer some buffer during drought years, even the state's larger reservoirs cannot compensate for prolonged, severe drought (Montana State Water Plan, 2015). Further, most surface water resources in the state are overallocated and basins are sometimes closed (Hibbs, 2008). Drought can reduce rangeland and cropland productivity (USDA Climate Hub), even for irrigated producers (Kundzewicz, 2007). Drought in Montana has caused economic hardships for producers, increased conflict over water use and allocation, and increased psychological, social, and financial strain at the individual, household, and community scales (Johnson and Smith, 2003; Yung et al., 2015). In listening sessions conducted with farmers and ranchers for the Montana Climate Assessment (2017), producers indicated a need for climate information that is localized and relevant to decision-making.
Focus Groups
We chose focus groups because they enabled us to interact with groups of producers through a structured dialogue that provided insights into their views and practices. Focus groups provide opportunities for participants to share both similar and dissimilar perspectives, enabling researchers to understand a range of views (Morgan, 1996). Focus groups also replicate the real-life social nature of knowledge production, in this case providing a window into how producers navigate different climatic conditions and climate information. Focus groups are often employed in marketing research to better understand how people respond to and interpret specific types of visual and textual information (Calder, 1977), which is analogous to the goals of this project.
We conducted focus groups with producers in five locations in Montana—Harlowton, St. Ignatius, Choteau, Fairfield, and Chester—which were selected because they represent a range of different types of agriculture, including dry land pulse and wheat farming; irrigated barley, wheat, and hay; and livestock production on native rangelands (see Figure 1 for map of study sites). We utilized a typical focus group sampling strategy and aimed to include a diversity of different types of producers through five study sites with different types of agriculture in different parts of the state, and through inviting different types of producers to participate. The sample is best described as a purposive, non-probability sample which attempts to represent the population of Montana agricultural producers through inclusion of a range of different types of producers in the focus groups, rather than through statistical representation. In each location, we contacted local extension agents, USDA staff, agriculture–focused NGOs, and other community members to obtain the names of potential participants and then called producers to invite them to participate. A total of 34 producers participated in the five focus groups. Participants were all owner-operators and were predominantly male. As intended, the sample included a diversity of producers, with 90% of participants raising livestock, 44% practicing at least some dryland farming, and 70% with at least some irrigated acres.
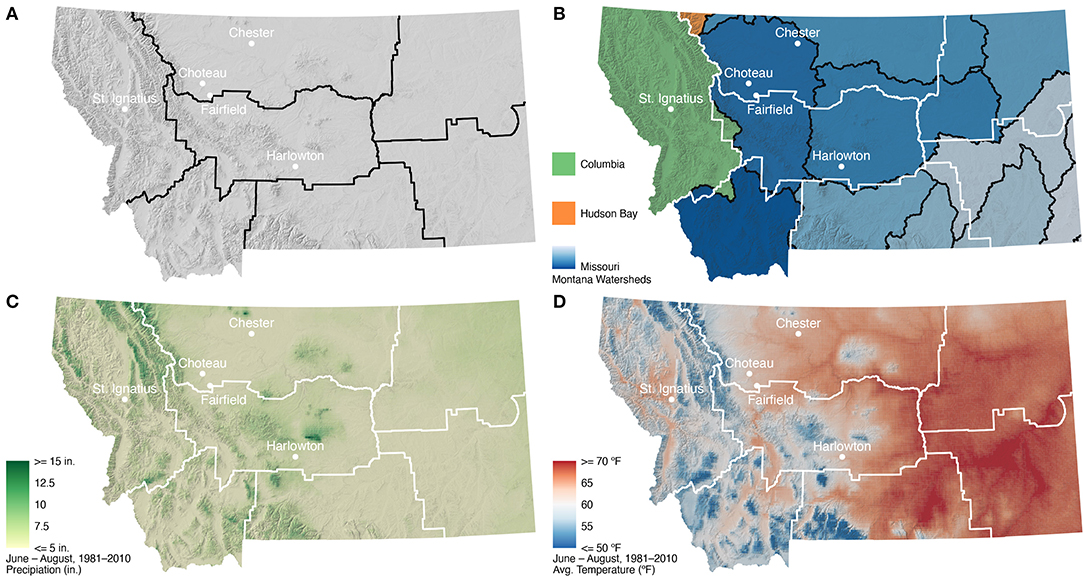
Figure 1. Map showing seven climate divisions as defined by NOAA's Climate Prediction Center (A), boundaries of Montana watersheds (B), and average precipitation (C) and average temperature (D) June-Aug 1981–2010. Map also indicates the location of the five study sites.
Focus groups were 2 h long and researchers utilized an interview guide to ensure consistency and comparability across the five groups (Hesse-Biber and Leavy, 2006). Producers were asked about their previous experience with and responses to drought; how climate information is (or is not) integrated into their decision-making; and their climate information needs, including needs relative to spatial and temporal scale. Participants also reviewed and reflected on hard-copy climate information that included descriptions of recent conditions, 3-month seasonal forecasts, and mid-century climate projections (see Figures 2–4 and https://climate.umt.edu/mtdrought/mtdrought-june-2018.pdf for climate information utilized in focus groups).
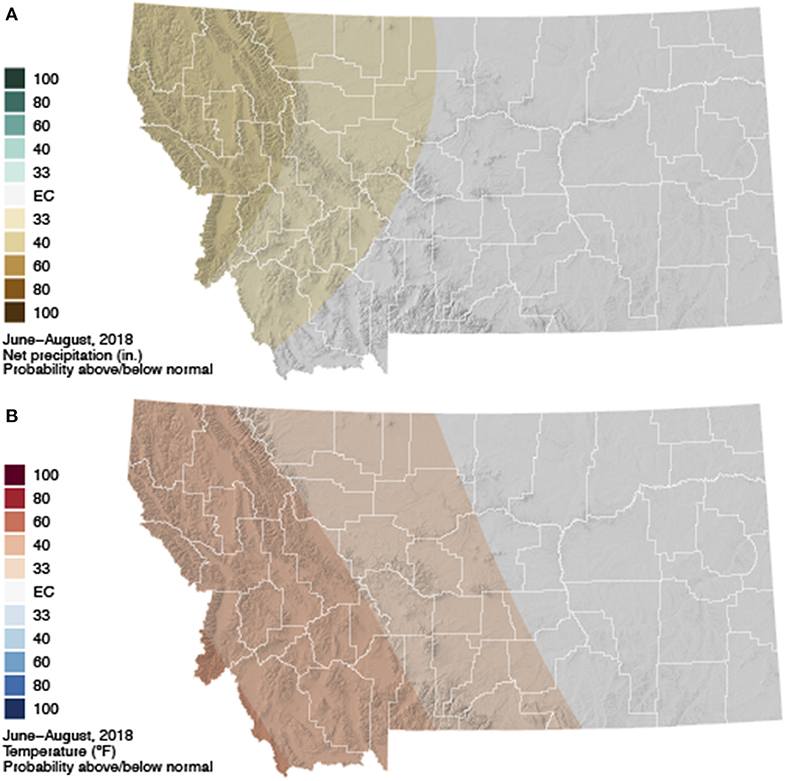
Figure 2. Three-month seasonal climate forecasts (SCFs) for precipitation (A) and temperature (B) for June–August 2018. The three-month forecasts are presented as probability above or below normal conditions, defined as average conditions for the years of 1981–2010. Data are from NOAA's Climate Prediction Center.
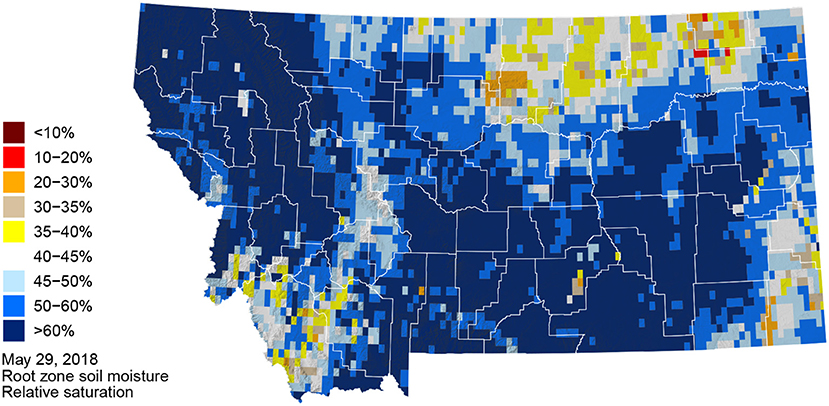
Figure 3. Satellite-derived root zone soil wetness on May 29, 2018. Root zone soil wetness is a measure of how much water has saturated the soil; it is the relative saturation between completely dry and completely saturated soil between 0 and 100 cm depth. Soil saturation maps were derived from NASA's Soil Moisture Active Passive (SMAP) satellite program “SPL4SMGP” data product. Soil moisture is mapped using a combination of radar and radiometer measurements from space and surface observations at an ~9-km spatial resolution.
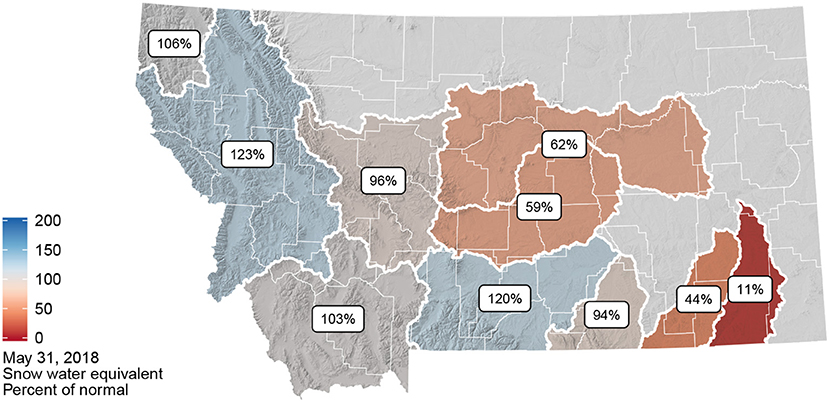
Figure 4. Percent of normal snow water equivalent (SWE) on May 31, 2018. SWE is the amount of water contained within the snowpack. SWE is measured across the West by the USDA Natural Resources Conservation Service's SNOTEL network of snow monitoring stations, aggregated spatially by hydrologic basins. The SWE percent of normal represents the current snow water equivalent found at selected SNOTEL sites in each hydrological basin compared to the normal value for those sites from 1981 to 2010.
All sessions were recorded, transcribed verbatim, and coded using NVivo 9. Analysis was an iterative process, whereby researchers compared emergent findings from the data with existing theory and literature (Layder, 1998). The data excerpts included below focus specifically on aspects of temporal and spatial scale, and how scale interacts with producers' decision context.
Results
The Spatial Scale of Climate Information Is Often Too Large
In assessing the utility of climate information, producers drew heavily on their understanding and experience with the local biophysical conditions and micro-climates, and suggested that every farm and ranch is different due to diversity across the landscape. They argued that these differences meant that large-scale climate information was not always useful because it often failed to capture this localized variability.
Many producers drew on their personal observations, concluding that “the country differs so much in the way a crow flies of 10 miles.” They pointed to a combination of biophysical conditions such as weather, climate, and soils, which they suggested create a diverse landscape. Farmers and ranchers explained how this diversity creates unique local conditions for each agricultural operation, as one rancher proclaimed “the biggest thing in agriculture, his place is different. [Their place] is different…Every operation is different.”
Nearly all producers suggested that useful climate information must be presented at a scale that captures the diversity that they observe on the landscape. When producers discussed seasonal climate forecasts, many producers adamantly called for site-specificity, suggesting that landscape diversity makes locally specific information more valuable than general information. One farmer stated, “for it to be useful for us, it has to be site-specific.” A producer explained that, “if you're planning on a trend that includes the whole state of Montana, well, you might just be way off base in the end because that trend isn't what's happening on your place.”
As an example, some producers indicated that there was too much landscape diversity within their county for aggregated county level data to be useful. One rancher explained that within their county, “living here, it's not the same…You've got almost three different worlds.” To this producer, these three different worlds represented stark geographical boundaries where weather and climate differ dramatically from one area to the next. Another producer stated, “you can actually almost scratch a line, most the time, on the way the showers come through.” In one case, a producer noted that the highway was a clear division between two distinct metrological areas during a recent drought, saying “everything west of the highway, they were extremely dry, on the eastern side of the county, so same county, but it was completely different.” Several producers contrasted “the bigger Montana picture,” with “three miles down the road,” and what is happening on “just my farm.”
A handful of producers pointed to specific ranges, mountains, hills, or ridges as influential on weather and climatic conditions across the landscape. They used these physical features to emphasize the importance of locally specific information. A rancher pointed to a specific example, saying “If there's a mountain—the [town A] sitting here vs. [town B], there's a hell of a lotta difference.” Meanwhile, a farmer mentioned “the [nearby hills]definitely affects our moisture here.” Another rancher also explained that “the change in elevation makes quite a difference. I'm 900 feet higher than [town C], and that temperature is cooler up there.”
Several producers also noted that small-scale variations in soil type meant that information from large-scale soil moisture maps was not always useful. A farmer drew on personal experience monitoring his own soils to conclude that “every field is different.” Meanwhile, other producers acknowledged that all “soils are different.” According to these producers, “a difference in soil type” can determine “how it holds the moisture. No matter how you manage it, there's a difference.” Therefore, a rancher noted that decisions “have to take the soil conditions into account whether it's got clay in it or sand” or any other type of soil. A producer with high concentrations of gravel in their soil pointed out how wind can deplete soil moisture “in a week from adequate to none.”
Ultimately, a rancher explained “what everyone's looking for in the end… you're focused on your bubble.” In the focus groups, nearly all producers repeatedly indicated that to be useful, climate information needed to capture local landscape diversity, which meant it needed to be at the scale of their farm or ranch, or even the scale of a specific field.
Timing and Temporal Scale Influences Utility
In focus groups, producers reviewed Montana Climate Assessment (MCA) (2017) findings in our Montana Drought & Climate newsletter which ranged in temporal scale from seasonal climate forecasts to mid-century projections.
Upon reviewing the projections, many producers suggested that climate information at longer temporal scales was not useful in their decision-making processes “on-the-ground.” Consistent with prior research (Breuer et al., 2008; Crane et al., 2010; Mase and Prokopy, 2014; Dunne et al., 2015; Chatrchyan et al., 2017), most producers in this study argued that short-term weather forecasts and seasonal climate forecasts are more useful in management decisions than mid-century climate projections. A few producers were quick to point to the utility of seasonal climate forecasts for intermediate planning to “see what the rest of the summer's gonna be…and [if] we're gonna be hot and dry and 90–100 degrees, you're probably gonna change some decisions.”
In contrast to the utility of SCFs, several producers stated that mid-century climate projections were difficult to link with the decisions they are making in the present. One producer expanded on this point, saying:
On a 20-year forecast, it gets back, to me, is it an actionable item? Yeah, we all know the climate's gonna change, but, really, fundamentally, what changes is an individual producer gonna make now? I mean we're all trying to improve our soil. We're all trying to improve our genetics, and turn the ground over to the next generation better than we had it, but, to me, it gets down to, what changes can I really make? It's more a policy type deal…
To me, this climate outlook for 30 years in advance—okay, that's good information, I guess. But nobody is gonna look at this and make a “management” decision based upon that. They might say, “Okay, that's interesting,” but they're not gonna probably make a management decision for the most part, based on that.
Other producers expressed similar views stating that “a mid-century forecast isn't gonna do a producer any good” and, similarly, that mid-century projections are “too far out.” One producer explained, “In agriculture, we're a little longer-term with 5 or 10 years, but going out to 2040, you and I don't give a damn.” Producers described mid-century climate projections as “disconnected” “mismatched,” saying that “finding a “fit”… remains a major challenge” between climate information provision and producers' needs. One producer attributed this disconnect to the shorter time horizon of agricultural decisions and the demands of making a living, saying: “you gotta survive this year and, at best, look into next year. After that…that's as far as we're gonna—that's as far as I'm gonna go.” Across all focus groups, many producers made it clear that mid-century climate projections did not align with the temporal scale at which they made decisions.
Several producers identified their “lifetime” as a yardstick for thinking long-term. When asked, “How far back do you go when you think about past experiences and events?” one producer responded with “your lifetime.” In reflecting on mid-century climate projections, many producers argued that the timeframe simply was not relevant to their decision-making. Some producers even expressed indifference to the implications of mid-century climate projections saying that “none of us will be here.” With regard to climate change, another producer stated, “In my lifetime, I'm not gonna worry about it. I am the average age farmer, and I'm about to quit.”
In addition to feedback on the time-frame of climate projections, nearly all producers indicated that the timing of when climate information is provided is important for the information to be useful. For example, when asked about dates for sending information such as the Montana Drought & Climate newsletter, one producer suggested that in order for it to serve as a “tool in the toolbox” the spring edition should be sent on March 1st rather than April 1st. He explained, “if I'm gonna look at this, the first of April is too late for what we do most of the time here” and that “the 1st of March gives you anywhere from 2 weeks to a month before the spring work really starts to happen.” Most producers also suggested that they needed climate information more frequently in the springtime (March through June) as opposed to evenly distributed throughout the year, which is often the case when climate information is produced quarterly. They recommended “March, April, May, June” and then “November,” saying that “By the time you get to July, you pretty much are decided. Our decisions are done.” Thus, the timing of climate information needs to align with the timing of key decisions in order to be useful.
How Scale Interacts With Accuracy, Credibility, and Trust
Perceptions of accuracy and source credibility also influenced many producers' willingness to utilize climate information, sometimes reducing trust in forecasts and projections. Concerns differed according to the temporal scale of the information, with skepticism increasing with longer temporal scales. Many producers used their own past experiences of weather and climate, and of climate information, as a touchstone or gage to judge the utility and trustworthiness of the specific climate information shared during focus groups.
In some of the focus groups, climate information for longer temporal scales, such as mid-century projections, reduced source credibility and trust. Some producers suggested that the focus on “climate change” in the mid-century projections felt political and indicated that climate information producers had “an agenda” and were making a “pitch.” Referring specifically to the Mid-Century Outlook, which described projections for temperature and precipitation change, one producer stated: “To me, your Outlook gives you an agenda, and I don't think you want an agenda.” One producer explicitly linked the mid-century projections to trust, saying “It comes back to…as we had mentioned earlier, not trusting the science” and referenced “the Al Gore thing.” Several producers shared that the presence of the mid-century projections and the references to climate change caused them to ask if the research team was “objective.”
When asked if their concerns about a political agenda related to the mid-century projections influenced their views of the 3-month seasonal forecasts and the information about current drought and soil moisture conditions, some producers indicated that “there's definitely bleed-over” and that it appeared that “this is what you're leading into.” For one producer, when climate information focused solely on the negative impacts of climate change he was “always suspect of it” and more likely to “discount it.” He went on to state:
But, if somebody can point out that this is gonna be the negatives and but these are gonna be some positives, then at least I'm more likely to trust that source that they're not just putting forth their political agenda. Because so much of the information is so lopsided. And, again, it might be accurate, and it might not be…when the temperature goes up, there's positives and negatives both. If you don't portray that, then most of us just, “Oh, yeah, they have a certain agenda. I'm not gonna listen to that.”
Another producer suggested that “global warming is what people have used to make money… there's people just making tons of money off of that terminology.”
While several producers suggested that using the phrase “changing climate” would be less political than “changes in the climate,” a few producers shared that they did not view the mid-century projections as political, indicating a diversity of perspectives on this. Those producers who indicated that they “believed” in climate change often drew on their own experience to substantiate that belief. According to one producer:
When I was growing up, as a little kid, I remember all the water holes that used to sit around Montana that have been dry for the last 35 years, 30 years. My dad even, the other day, said he believed in global warming. Then he said, “No, not so much global warming as climate change.”
Interestingly, a couple of producers also questioned the motives of for-profit climate information providers, but these critiques were focused on short-term weather forecasts. Note that producers were not provided with any weather information nor were any of the focus group questions focus on weather; however, producers often used their experience of weather forecasts to explain their views on seasonal climate forecasts and other climate information. One producer described this problem, saying:
Some sources that, like the Weather Channel…they're really advertising this and that or whatever, for that to me is a turn off because, obviously, they're there to market something to you, suck you into this, or suck you into that. I mean, it's entertaining if you have nothing else to do. So that's a trust issue for me.
Another producer linked trust and accuracy, suggesting that Weather Underground was more trustworthy and accurate when it was “owned locally.” She explained:
My problem with Weather Underground, when they started it, that was created by a Montana boy, and it was owned by a Montana boy. Then they sold it to the Weather Channel, and I'm sorry, it turned to garbage…That was an app that I trusted. I don't trust it anymore, and I'm looking for one that I can trust because they forgot who they were working for…it's disheartening cuz when it was owned locally, or at least I felt it was owned locally, they were on the mark. They were accurate, no horsing around, and then Weather Channel got it, and it was just like, oh, crumb!
In response to contradictory weather forecasts, several producers discussed combining forecasts from multiple sources and assuming it would end up in the “middle.” According to one producer, “I don't think there is one agency or one tool that you can be very specific on trusting…You tend to look at three, four, five, six different things and almost make your own forecast a little bit.” But a couple of producers also wondered “If there's no other NOAAs out there collecting this raw data, why is there such a variety of forecasts?” For a more detailed description of this finding, please see Snitker (2020). While these statements focus on weather forecasts as opposed to longer-term climate forecasts and projections, they indicate the extent to which conflicting information and perceptions about financial motivations can reduce trust and source credibility.
Information that was difficult to understand also reduced trust. The data visualizations and maps were not accessible for some producers. Several producers noted that they found maps and graphs depicting current conditions and seasonal forecasts “confusing” and “hard to see,” saying that they “don't quite understand” them. Many producers found the shading on the maps difficult to interpret, wondering “which shading goes to which shading” and finding it difficult to “match the colors with the numbers.” One producer shared that “I'm color blind, so graphs without numbers, I can't seem to tell the difference.” Another producer suggested that when the information was not easy to understand they would “start to doubt” it, indicating that understandability also influenced trust and source credibility.
Many producers also expressed concern about the accuracy of the 3-month seasonal forecasts. One producer described their concerns saying “when it comes out to the farm, that kind of probability and confidence level I don't put a lot of faith in.” Another producer explicitly linked accuracy to temporal scale, saying:
Forgive me, the climatologists, I'm sorry, but…To me, it looks like it's anyone's guess. I mean, honestly, we have a 10-day forecast, and that's anyone's guess. The 4-day forecast I love. It's pretty, amazingly accurate. Beyond 4 days? I either get my hopes up…or it goes dash.
Again, temporal scale was related to perceptions of accuracy.
As described by Snitker (2020), many of these producers in these focus groups compared seasonal climate forecasts to their own experience of climatic conditions and judged the accuracy of the forecast accordingly. If the forecast did not match what they experienced that often reduced their willingness to “trust” or rely on future forecasts. In reviewing the climate information specifically produced by Montana Drought and Climate, many producers made it clear that skepticism about the accuracy or utility of this information was influenced by their past experiences with weather and climate information from other sources. This suggests views about the accuracy of climate information from one source influences trust in other sources.
Many producers suggested that they did not utilize mid-century climate projections because of a lack of trust in their accuracy. As one producer expressed, Personally, I have a hard time going past a month on weather. Two weeks is pushing it. A week is—I can believe a week. Other than that, it can change—especially up here, it just change. All the different winds come swirling around. It changes real fast. Another producer echoed a similar sentiment with regard to seasonal climate forecasts, adding that the timeframe contributed to that feeling of distrust, saying “I don't know, I don't trust the forecast.” Thissuggests that, despite the efforts of climate scientists to improve the accuracy of longer-term projections, many producers in Montana primarily use and trust shorter-term forecasts.
Many producers relied on their own observations to predict future weather and climate. They described “watching how nature itself is functioning,” saying “we watch everything,” including “the sky,” “the animals,” “the way the wind blows,” and “the mountains” as markers for what's to come. Another producer explained, “We get up in the morning, and for us, we look at the mountains. That pretty much tells us our day, whether or not we've got wind clouds…and they tell us what we're doing and how we're gonna do it.” While these statements focus on weather and not climate, some producers also relied on their own observations to anticipate months in advance. This producer described a phenomena that several others mentioned:
Be honest with you, as unscientific as this sounds, my grandfather and dad both believed really strongly in the old forecasts. I don't live on them, but I keep track of them…90 days from fog, there's something gonna come through. It may not give you anything, but you're gonna have a system coming through.
Other producers explained that they relied on official forecasts and on their own experiences, saying “My own experiences, some science thrown in there. I'll mix it up in a bowl, and a little bit of prayer, and away you go.”
Discussion
Previous research suggests that climate information is underutilized by agricultural producers (Hewitt et al., 2012; Mase and Prokopy, 2014; Tall et al., 2014; Vaughan and Dessai, 2014; Haigh et al., 2015; Davis et al., 2016). Our findings suggest that climate information is often produced at spatial scales that are too large and temporal scales that are too long to be useful for producers. With regard to spatial scale, producers emphasized that local conditions are highly variable due to landscape diversity and micro-climates, and that variability is not captured in climate information at the county, climate zone, or other aggregated scales. They suggested that climate information at the farm or ranch-scale (or even smaller scales) would be more useful. Similarly, past research with federal land managers and municipal managers found that lack of information at relevant spatial scales was a barrier to climate adaptation (Archie et al., 2014).
While the literature examining spatial scale and the utility of climate information is somewhat limited (existing studies include Dunne et al., 2015; Reeves et al., 2015), a closer look at climate information for Montana illustrates the challenges of representing variation at spatial scales relevant to producers. The Montana Climate Assessment presented mid-century and end of century climate projections for seven climate divisions as defined by NOAA's Climate Prediction Center (Figure 1A). These domains were selected to present a first look at broad trends in Montana's climate future. Spatially, these large areas attempt to capture regional variability in climate patterns, and bound that variability by political divisions (usually, county borders). However, this scale of climate data does not resolve the local climatological variation within each climate division due to differences in topography, vegetation, and land use. For example, the climate divisions intersect hydrological watersheds (Figure 1B), which are relevant for producers who are dependent on irrigation from snowpack and upstream sources. However, the availability of irrigation water in Choteau (where most hay production is irrigated) bears little relationship to the amount of precipitation available in Chester (where most farms are rainfed), even though they fall within the same climate division. While the current climate division model is imperfect, there are tradeoffs associated with downscaling to the farm/ranch or field scales that producers want. For example, Sayre (2017) concludes that scientific efforts to characterize variation across western rangelands through more intensive measurements only documented more variation, making it even more challenging to describe that variation.
When considering the temporal scale of climate information, our findings suggest that short-term weather forecasts and seasonal climate forecasts are more useful to producers than mid-century projections, in part because shorter timeframes were perceived to be more accurate. Further, producers did not find mid-century climate projections to be particularly useful for present-day decisions. This is consistent with research on climate adaptation outside of the agricultural arena, where multiple studies have found that individuals and organizations have a difficult time taking action now in preparation for future climate impacts, despite evidence that proactive, anticipatory adaptation is important (Biesbroek et al., 2011; Simonsson et al., 2011). Biesbroek et al. (2011) suggest that short-term nature of decision-making prevents end-users from envisioning the relevance of mid to end-of-century climate projections for more immediate decisions. Finally, in our study, producers also mistrusted mid-century projections because they linked them to a broader politics of climate change. Further, perceptions of accuracy and the politics of climate information influenced views on source credibility, suggesting an important interaction between temporal scale, accuracy, and trust.
Our findings are consistent with Dunne et al. (2015) in suggesting that shorter temporal scales might be more useful to producers. Previous research and our study suggest a large unmet need for usable climate forecast information that occurs from months to decades and at farm scales. This represents a “grand challenge” for climate scientists and organizations that aim to provide “usable” climate information (National Research Council, 2012). Currently, climate scientists must balance the need for high resolution climate information with the limitations of current model's ability to represent small-scale processes, increased computational expenses, and the inherent uncertainties of current climate modeling methodologies at higher spatial resolutions (see e.g., Flint and Flint, 2012). Said simply, climate scientists cannot downscale projections beyond a certain scale because these projections would convey false precision and process representation, thereby resulting in poor decisions. The contribution of local variability (e.g., topography, land use, vegetation) to uncertainty in modeled climate forecasts is expected to increase at smaller scales and as shorter decadal or multiannual time scales are considered (Giorgi, 2002). In addition, downscaled climate models cannot characterize the multitude of important processes that inform producers' decisions at the farm scale (e.g., distributed soil moisture, crop specific growth responses, or seasonal drought onset or cessation). This additional level of prediction requires the coupling of downscaled climate model outputs with high resolution earth systems models that are able to represent the dynamic interactions of the water and carbon cycles (e.g., Maneta and Silverman, 2013).
Significant research is currently underway to develop improved statistical and physically based seasonal forecasts with longer lead times as well as decadal climate forecasts. However, there has been limited progress in terms of improving existing lead times and reducing uncertainty at finer spatial resolution (Weisheimer and Palmer, 2014; Murphy et al., 2016). Current approaches that show promise include combining climate models with observations of surface and ocean meteorology and satellite derived observations to better constrain the forecast models. As one example, distributed earth systems models that incorporate climate data associated with sea surface temperature oscillations (e.g., ENSO, the Pacific-Decadal Oscillation) and projections of anthropogenic forcing have shown promise with lead times from 10- to 45- months (Chikamoto et al., 2017). We encourage the forecasting and model development communities to continue the upward trajectory of precipitation and temperature forecast prediction skill. Continued improvements in these forecasts may provide producers with the early warnings that are necessary to implement proactive adaptation strategies.
While producers appear to prefer smaller spatial scales, previous studies indicate that different types of producers and different decisions require climate information at different temporal or spatial scales. For example, large-scale seasonal climate forecasts may be useful for rancher decisions about livestock stocking rates (Reeves et al., 2015). However, a dryland wheat farmer may need weather and soil conditions at a very local scale (e.g., a particular field or operation). And an irrigated hay producer may need information about water availability for irrigation as indicated by local stream gauges, regional reservoir capacities, and watershed-scale snowpack information. Thus, the appropriate spatial and temporal scale may differ depending on the specific decision context, including the type of end-user and the decision being made. This is consistent with research on federal land managers and municipal managers, which found that the former preferred climate information at much larger spatial scales as compared with the latter (Archie et al., 2014). Additional research on the scale required for different types of agricultural decisions is critical to improving the utility of climate information for agricultural producers.
Additionally, climate information providers should consider which scales are useful to other types of agricultural end-users. For example, agricultural advisors (crop advisors, extension agents, and private consultants) play an important role as knowledge brokers and boundary spanners, and are largely understudied (Prokopy et al., 2015). In a study of crop advisors, Lemos et al. (2012) found that advisors who were more concerned about long-term climate risks were more likely to provide climate information to producers, suggesting again that the decision-context, in this case the perceived temporal scale of risk, influences the relevance of climate information. Programs that engage advisors in learning about the utility of climate information could position them to more effectively communicate the relevance of that information to agricultural producers (Prokopy et al., 2015). As boundary spanners, advisors can also communicate the needs of producers, including needs related to temporal and spatial scale, to climate information providers.
Policy-makers and government agencies focused on agriculture might also be able to utilize climate information at longer temporal scales and larger spatial scales. Policies to support agricultural adaptation to climate change, as recommended by the National Sustainable Agriculture Coalition (2019), will need to address short-term and long-term needs, and support programs that help producers proactively adapt to changing conditions. Government agencies such as the USDA Climate Hubs and the Natural Resources Conservation Service can help producers connect climate information at longer and larger scales to more immediate decision-making.
Given the different needs of specific end-users, engagement with and feedback from producers and other agricultural end-users is important to make climate information more useful and relevant. Collaboration between producers of climate information and end-users not only increases the use of that information in decision-making (Jones et al., 2015; Soares and Dessai, 2016), collaborative approaches have also been found to increase understanding of climate information (Cliffe et al., 2016) and trust in the scientists producing that information (Pannell and Vanclay, 2011). Meaningful engagement between climate information providers and intended end-users can help address issues of temporal and spatial scale, and the tradeoffs between scale and uncertainty. More broadly, this kind of engagement can help climate information providers understand what type of information is useful in which contexts, since utility will depend in part on the type of agricultural operation and the specific region of the world.
Conclusion
As recommended above, climate information providers can work with end-users to better align the temporal and spatial scale of climate information with the needs of decision making, with attention to the ways that different types of decisions and decision-makers, from producers to crop advisors, require different scales. Our findings suggest that, for producers, climate information that focuses on shorter time scales (i.e., short term weather and seasonal climate forecasts) at time intervals that align with key decisions for producers will be more useful in decision-making. Producers also indicated that climate information that captures local biophysical variability is more valuable than large scale (e.g., regional, climate zone) information. However, climate information providers need to be attentive to the model limitations outlined above, as some of the information that producers need is currently difficult to produce with adequate precision. Further, more or better information alone is unlikely to address the widespread underutilization of climate information. Adaptation decisions are embedded in a complex decision context of interacting social, cultural, political, and environmental process, and climate information interacts with this complex decision context. Thus, we need a better understanding of how climate information interacts with a range of enabling and constraining factors, from local social norms, to access to financial resources, risk perceptions, and local knowledge. Perhaps paradoxically, we need to go beyond climate information to better understand how this information can be better integrated into adaptation decisions.
Data Availability Statement
The datasets presented in this article are not readily available because we want to ensure the confidentiality of our study participants. Requests to access the datasets should be directed to ada.smith@umontana.edu.
Ethics Statement
The studies involving human participants were reviewed and approved by University of Montana Institutional Review Board for the Protection of Human Subjects in Research. The participants provided their written informed consent to participate in this study.
Author Contributions
LY, EM, ASn, ASm, RB, and KJ designed the study. LY, EM, ASn, and RB collected the data. ASn and ASm analyzed the data. ASm led manuscript development. LY, ASn, EM, KJ and RB contributed to the manuscript. All authors contributed to the article and approved the submitted version.
Funding
This research was supported by the Montana Climate Office, the UM BRIDGES program, the United States Department of Agriculture National Institutes on Food and Agriculture (2017-67027-26313), the National Science Foundation Division of Graduate Education (1633831), and the National Oceanic and Atmospheric Administration National Integrated Drought Information System (University Corporation for Atmospheric Research subaward SUBAWD000858).
Conflict of Interest
The authors declare that the research was conducted in the absence of any commercial or financial relationships that could be construed as a potential conflict of interest.
Acknowledgments
We also wish to thank the all of the Montana farmers and ranchers who participated in focus groups in summer 2018. We greatly appreciate their time and insight.
Footnotes
1. ^U.S. DOI BOR. Basin Report: Missouri River. Available online at: http://www.usbr.gov/climate/secure/docs/2016secure/factsheet/MissouriRiverBasinFactSheet.pdf.
References
Abatzoglou, J. T., and Brown, T. J. (2012). A comparison of statistical downscaling methods suited for wildfire applications. Int. J. Climatol. 32, 772–780. doi: 10.1002/joc.2312
Archie, K. M., Dilling, L., Milford, J. B., and Pampel, F. C. (2014). Unpacking the “information barrier”: Comparing perspectives on information as a barrier to climate change adaptation in the interior mountain West. J. Environ. Manage. 133, 397–410. doi: 10.1016/j.jenvman.2013.12.015
Ash, A., McIntosh, P., Cullen, B., Carberry, P., and Smith, M. S. (2007). Constraints and opportunities in applying seasonal climate forecasts in agriculture. Austr. J. Agr. Res. 58, 952–965. doi: 10.1071/AR06188
Biesbroek, R., Klostermann, J., Termeer, C., and Kabat, P. (2011). Barriers to climate change adaptation in the Netherlands. Clim. Law 2, 181–199. doi: 10.1163/CL-2011-033
Breuer, N. E., Cabrera, V. E., Ingram, K. T., Broad, K., and Hildlebrand, P. E. (2008). AgClimate: a case study in participatory decision support system development. Clim Change 87, 385–403. doi: 10.1007/s10584-007-9323-7
Calder, B. J. (1977). Focus groups and the nature of qualitative marketing research. J. Market. Res. 14, 353–364. doi: 10.1177/002224377701400311
Cash, D. W., Borck, J. C., and Patt, A. G. (2006). Countering the loading-dock approach to linking science and decision making: comparative analysis of El Niño/Southern Oscillation (ENSO) forecasting systems. Sci. Technol. Human Values 31, 465–494. doi: 10.1177/0162243906287547
Chatrchyan, A. M., Erlebacher, R. C., Chaopricha, N. T., Chan, J., Tobin, D., and Allred, S. B. (2017). United States agricultural stakeholder views and decisions on climate change. WIREs Clim. Change 8, 1–21. doi: 10.1002/wcc.469
Chikamoto, Y., Timmermann, A., Widlansky, M. J., Balmaseda, M. A., and Stott, L. (2017). Multi-year predictability of climate, drought, and wildfire in southwestern NorthAmerica. Sci. Rep. 7, 1–12. doi: 10.1038/s41598-017-06869-7
Cliffe, N., Stone, R., Coutts, J., Reardon-Smith, K., and Mushtaq, S. (2016). Developing the capacity of farmers to understand and apply seasonal climate forecasts through collaborative learning processes. J. Agric. Educ. Exten. 10, 1–15. doi: 10.1080/1389224X.2016.1154473
Cook, B. I., Ault, T. R., and Smerdon, J. E. (2015). Unprecedented 21st century drought risk in the American Southwest and Central Plains. Sci. Adv. 1:e1400082. doi: 10.1126/sciadv.1400082
Crane, T. A., Roncoli, C., Paz, J., Breuer, N., Broad, K., Ingram, K. T., et al. (2010). Forecast skill and farmers' skills: seasonal climate forecasts and agricultural risk management in the southeastern United States. Wea. Climate Soc. 2, 44–59. doi: 10.1175/2009WCAS1006.1
Dai, A. (2012). Increasing drought under global warming in observations and models. Nat. Clim. Change 2, 1–7. doi: 10.1038/nclimate1633
Davis, M., Lowe, R., Steffen, S., Doblas-Reyes, F., and Rodó, X. (2016). “Barriers to using climate information: challenges in communicating probabilistic forecasts to decision-makers,” in Communicating Climate-Change and Natural Hazard Risk and Cultivating Resilience. Advances in Natural and Technological Hazards Research, Vol. 45, eds J. Drake, Y. Kontar, J. Eichelberger, T. Rupp, and K. Taylor (Cham: Springer). doi: 10.1007/978-3-319-20161-0_7
Dessai, S., Hulme, M., Lempert, R., and Pielke, R. A. (2009). Do we need better predictions to adapt to a changing climate? Earth Obser. 90, 111–112. doi: 10.1029/2009EO130003
Diehl, D. C., Galindo-Gonzalez, S., Dourte, D. R., Fraisse, C. W., Sloan, N. L., Bartels, W. L., et al. (2015). Toward engagement in climate training: findings from interviews with agricultural extension professionals. J. Rural Soc. Sci. 30, 25–50. Available online at: https://search-proquest-com.weblib.lib.umt.edu:2443/scholarly-journals/toward-engagement-climate-training-findings/docview/1695036866/se-2?accountid=14593 (Retrieved March 30, 2021).
Diffenbaugh, N. S., Swain, D. L., and Touma, D. (2015). Anthropogenic warming has increased drought risk in California. Proc. Natl. Acad. Sci. U.S.A. 112, 3931–3936. doi: 10.1073/pnas.1422385112
Dilling, L., and Lemos, M. C. (2011). Creating usable science: opportunities and constraints for climate knowledge use and their implications for science policy. Glob. Environ. Change 21, 680–689. doi: 10.1016/j.gloenvcha.2010.11.006
Dong, Y., Hu, S., and Zhu, J. (2018). From source credibility to risk perception: how and when climate information matters to action. Res. Conserv. Recycl. 136, 410–417. doi: 10.1016/j.resconrec.2018.05.012
Dunne, M. R., Lindesay, J. A., and Howden, M. (2015). Spatial and temporal scales of future climate information for climate change adaptation in viticulture: a case study of user needs in the Australian winegrape sector. Aust. J. Grape Wine Res. 21, 226–239. doi: 10.1111/ajgw.12138
Flint, L. E., and Flint, A. L. (2012). Downscaling future climate scenarios to fine scales for hydrologic and ecological modeling and analysis. Ecol. Process 1:2. doi: 10.1186/2192-1709-1-2
Furman, C., Roncoli, C., Crane, T., and Hoogenboom, G. (2011). Beyond the “fit”: introducing climate forecasts among organic farmers in Georgia (United States). Clim. Change 109, 791–799. doi: 10.1007/s10584-011-0238-y
Gay, C., and Estrada, F. (2010). Objective probabilities about future climate are a matter of opinion. Clim. Change 99, 27–46. doi: 10.1007/s10584-009-9681-4
Giorgi, F. (2002). Dependence of the surface climate interannual variability on spatial scale. Geophys. Res. Lett. 29:2101. doi: 10.1029/2002GL016175
Gold, A. J., Parker, D., Waskom, R. M., Dobrowolski, J., O'Neill, M., Groffman, P. M., et al. (2013). Advancing water resource management in agricultural, rural, and urbanizing watersheds: why land-grant universities matter. J. Soil Water Conserv. 68, 337–348. doi: 10.2489/jswc.68.4.337
Haigh, T., Tackle, E., Andresen, J., Widhalm, M., Carlton, J. S., and Angel, J. (2015). Mapping the decision points and climate information use of agricultural producers across the U.S. Corn Belt. Clim. Risk Manage. 7, 20–30. doi: 10.1016/j.crm.2015.01.004
Heal, G., and Millner, A. (2014). Reflections uncertainty and decision making in climate change economics. Rev. Environ. Econ. Policy 8, 120–137. doi: 10.1093/reep/ret023
Hesse-Biber, S. N., and Leavy, P. (2006). The Practice of Qualitative Research. Thousand Oaks, CA: Sage.
Hewitt, C., Mason, S., and Walland, D. (2012). The global framework for climate services. Nat. Clim. Change 2, 831–832. doi: 10.1038/nclimate1745
Hibbs, M. (2008). Interpreting the basin closure law in Montana: the permissibility of “prestream capture” – Montana Trout Unlimited v. Montana Department of Natural Resources and Conservation. Public Land Res. Law Rev. 29, 195–200. Available online at: https://scholarship.law.umt.edu/cgi/viewcontent.cgi?article=1262&context=plrlr (Retrieved March 30, 2021).
Hoell, A., Parker, B.-A., Downey, M., Umphlett, N., Jencso, K., Akyuz, F. A., et al. (2020). Lessons learned from the 2017 flash drought across the U.S. Northern great plains and Canadian prairies. Bull. Am. Meteorol. Soc. 101, E2171–E2185. doi: 10.1175/BAMS-D-19-0272.1
Hollinger, S. E. (1991). Incorporating Weather and Climate data into Integrated Crop Management Systems. Midwestern Climate Center Publication Number 91-01. Available online at: https://www.ideals.illinois.edu/bitstream/handle/2142/48934/ISWSMP-126.pdf?sequence=2&isAllowed=y#page=5 (Retrieved March 30, 2021).
IPCC (2019). Climate Change and Land: An IPCC Special Report on Climate Change, Desertification, Land Degradation, Sustainable Land Management, Food Security, and Greenhouse Gas Fluxes Interrestrial Ecosystems. doi: 10.25561/76618
Jencso, K., Parker, B., Downey, M., Hadwen, T., Hoell, A., Rattling Leaf, J., et al (2019). Flash Drought: Lessons Learned from the 2017 Drought Across the U.S. Northern Plains and Canadian Prairies. NOAA National Integrated Drought Information System. Available online at: https://www.drought.gov/sites/default/files/2020-09/NIDIS_LL_FlashDrought_2017_Final_6.6.2019.pdf (accessed March 30, 2021).
Johnson, J., and Smith, V. (2003). Adverse impacts of drought on crops and crop producers in the West. J. West. Agric. Econ. Assoc. 2, 9–13. Available online at: https://ageconsearch.umn.edu/record/27974/
Jones, L., Champalle, C., Chesterman, S., Cramer, L., and Crane, T. A. (2015). “Identifying constraining and enabling factors to the uptake of medium- and long-term climate information in decision making,” CCAFS Working Paper no. 113. CGIAR Research Program on Climate Change, Agriculture and Food Security(CCAFS). Copenhagen.
Kundzewicz, Z. W. (2007). Global freshwater resources for sustainable development. Ecohydrol. Hydrobiol. 7, 125–134. doi: 10.1016/S1642-3593(07)70178-7
Kuwayama, Y., Thompson, A., Bernknopf, R., Zaitchik, B., and Vail, P. (2019). Estimating the impact of drought on agriculture using the U.S. Drought monitor. Am. J. Agric. Econ. 101, 193–210. doi: 10.1093/ajae/aay037
Lavers, D., Luo, L., and Wood, E. F. (2009). A multiple model assessment of seasonal climate forecast skill for applications. Geophys. Res. Lett. 36:L23711. doi: 10.1029/2009GL041365
Layder, D. (1998). Sociological Practice: Linking Theory and Social Research. London: Sage. doi: 10.4135/9781849209946
Lemos, M. C., Kirchhoff, C. J., and Ramprasad, V. (2012). Narrowing the climate information usability gap. Nat. Clim. Change 2:789. doi: 10.1038/nclimate1614
Maneta, M. P., and Silverman, N. L. (2013). A spatially distributed model to simulate water, energy, and vegetation dynamics using information from regional climate models. Earth Interact. 17, 1–44. doi: 10.1175/2012EI000472.1
Mase, A. S., and Prokopy, L. S. (2014). Unrealized potential: a review of perceptions and use of weather and climate information in agricultural decision making. Weather Clim. Soc. 6, 47–61. doi: 10.1175/WCAS-D-12-00062.1
McCrea, R., Dalgleish, L., and Coventry, W. (2005). Encouraging use of seasonal climate forecasts by farmers. Int. J. Climatol. 25, 1127–1137. doi: 10.1002/joc.1164
Meehl, G. A., Goddard, L., Murphy, J., Stouffer, R. J., Boer, G., Danabasoglu, G., et al. (2009). Decadal prediction: can it be skillful? Bull. Am. Meteorol. Soc. 90, 1467–1485. doi: 10.1175/2009BAMS2778.1
Meinke, H., Howden, S. M., Struik, P. C., Nelson, R., Rodriguez, D., and Chapman, S. C. (2009). Adaptation science for agriculture and natural resource management: urgency and theoretical basis. Curr. Opin. Environ. Sustainabil. 1, 69–76. doi: 10.1016/j.cosust.2009.07.007
Meza, F. J., Hansen, J. W., and Osgood, D. (2008). Economic value of seasonal climate forecasts for agriculture: review of ex-ante assessments and recommendations for future research. J. Appl. Meteorol. Climatol. 47, 1269–1286. doi: 10.1175/2007JAMC1540.1
Montana Department of Agriculture (2016). Available online at: http://agr.mt.gov/ (accessed February 1, 2021).
Morgan, D. L. (1996). Focus groups. Ann. Rev. Sociol. 22, 129–152. doi: 10.1146/annurev.soc.22.1.129
Mount, J., Hanak, E., Chappelle, C., Colby, B., Frank, R., Gartrell, G., et al. (2016). Improving the Federal Response to Western Drought. San Francisco, CA: Public Policy Institute of California. doi: 10.5070/P2CJPP8331897
Murphy, D., Wyborn, C., Yung, L., Williams, D. R., Cleveland, C., Eby, L., et al. (2016). Engaging communities and climate change futures with Multi-Scale, Iterative Scenario Building (MISB) in the Western United States. Human Organ. 75, 33–46. doi: 10.17730/0018-7259-75.1.33
National Research Council (2012). Challenges and Opportunities in the Hydrologic Sciences. Washington, DC: National Academy Press.
National Sustainable Agriculture Coalition (2019). Agriculture and Climate Change: Policy Imperatives and Opportunities to Help Producers Meet the Challenge. Washington, DC: National Sustainable Agriculture Coalition. Available online at: https://sustainableagriculture.net/wp-content/uploads/2019/11/NSAC-Climate-Change-Policy-Position_paper-112019_WEB.pdf (accessed March 31, 2021).
Nyamekye, A. B., Nyadzi, E., Dewulf, A., Werners, S., Van Slobbe, E., Biesbroek, R. G., et al. (2020). Forecast probability, lead time and farmer decision-making in rice farming systems in Northern Ghana. Clim. Risk Manage. 31:100258. doi: 10.1016/j.crm.2020.100258
Pannell, D., and Vanclay, F. (2011). Changing Land Management: Adoption of New Practices by Rural Landholders. Melbourne, VIC: CSIRO.
Pendergrass, A. G., Meehl, G. A., Pulwarty, R., Hobbins, M., Hoell, A., AghaKouchak, A., et al. (2020). Flash droughts present a new challenge for subseasonal-to-seasonal prediction. Nat. Clim. Chang. 10, 191–199. doi: 10.1038/s41558-020-0709-0
Preston, B. L., Dow, K., and Berkhout, F. (2013). The climate adaptation frontier. Sustainability 5, 1011–1035. doi: 10.3390/su5031011
Prokopy, L. S., Morton, L. W., Arbuckle, J. G., Mase, A. S., and Wilke, A. K. (2015). Agricultural stakeholder views on climate change: implications for conducting research and outreach. Bull. Am. Meteorol. Soc. 96, 181–190. doi: 10.1175/BAMS-D-13-00172.1
Reeves, J. L., Derner, J. D., Sanderson, M. A., Kronberg, S. L., Hendrickson, J. R., Vermeire, L. T., et al. (2015). Seasonal weather-related decision making for cattle production in the Northern Great Plains. Rangelands 37, 119–124. doi: 10.1016/j.rala.2015.03.003
Rejesus, R. M., Mutuc-Hensley, M., Mitchell, P. D., Coble, C. H., and Knight, T. O. (2013). U.S. Agricultural producer perceptions of climate change. J. Agric. Appl. Econ. 45, 701–718. doi: 10.1017/S1074070800005216
Rickards, L., Howden, M., and Crimp, S. (2014). “Channelling the future? The use of seasonal climate forecasts in climate adaptation,” in Climate Change Impact and Adaptation in Agricultural Systems. eds J. Fuhrer and P. Gregory (Oxfordshire: CABI), 233–252. doi: 10.1079/9781780642895.0233
Sayre, N. F. (2017). The Politics of Scale: A History of Rangeland Science. Chicago and London: The University of Chicago Press.
Sayre, N. F., Debuys, W., Bestelmeyer, B. T., and Havstad, K. M. (2012). “The range problem” after a century of rangeland science: new research themes for altered landscapes. Rangeland Ecol. Manage. 65, 545–552. doi: 10.2111/REM-D-11-00113.1
Seneviratne, S. I., Corti, T., Davin, E. L., Hirschi, M., Jaeger, E. B., Lehner, I., et al. (2010). Investigating soil moisture-climate interactions in a changing climate: a review. Earth Sci. Rev. 99, 125–161. doi: 10.1016/j.earscirev.2010.02.004
Simonsson, L., Swartling, A. G., Andre, K., Wallgren, O., and Klein, R. J. T. (2011). Perceptions of risk and limits to climate change adaptation: case studies of two Swedish Urban regions. Clim. Change Adapt. Devel. Nations 42, 449–459. doi: 10.1007/978-94-007-0567-8_23
Snitker, A. (2020). Local knowledge and climate information: the role of trust and risk in agricultural decisions about drought (Thesis), University of Montana, Missoula, MT.
Soares, M. B., and Dessai, S. (2016). Barriers and enablers to the use of seasonal climate forecasts amongst organisations in Europe. Clim. Change 137, 89–103. doi: 10.1007/s10584-016-1671-8
Stokes, C., and Howden, M. (2010). Adapting Agriculture to Climate Change: Preparing Australian Agriculture, Forestry and Fisheries for the Future. Melbourne, VIC: CSIRO Publishing. doi: 10.1071/9780643098084
Tall, A., Davis, A., and Agrawal, S. (2014). “Does climate information matter? Evaluating climate services for farmers: a proposed monitoring and evaluation framework for participatory assessment of the impact of climate services for male and female farmers,” CCAFS Working Paper no. 69. CGIAR Research Program on Climate Change, Agriculture and Food Security (CCAFS) (Copenhagen).
Trenberth, K. (2011). Changes in precipitation with climate change. Clim. Res. 47, 123–138. doi: 10.3354/cr00953
Trenberth, K., and Dai, A. (2014). Global warming and changes in drought. Nat. Clim. Change 4, 17–22. doi: 10.1038/nclimate2067
Tubiello, F. N., Soussana, J. F., and Howden, S. M. (2007). Crop and pasture response to climate change. Proc. Natl. Acad. Sci. U.S.A. 104, 19686–19690. doi: 10.1073/pnas.0701728104
USDA Ag Facts – Montana (2018). Available online at: https://agr.mt.gov/AgFacts (accessed February 1, 2021).
USGCRP (2018). “Impacts, risks, and adaptation in the United States,” in Fourth National Climate Assessment. eds D. R. Reidmiller, C.W. Avery, D. R. Easterling, K. E. Kunkel, K. L. M. Lewis, T. K. Maycock (Washington, DC: U.S. Global Change Research Program), 1515.
Vaughan, C., and Dessai, S. (2014). Climate services for society: Origins, institutional arrangements, and design elements for an evaluation framework. WIREs Clim. Change 5, 587–603. doi: 10.1002/wcc.290
Weisheimer, A., and Palmer, T. N. (2014). On the reliability of seasonal climate forecasts. J. R. Soc. Interface 11:20131162. doi: 10.1098/rsif.2013.1162
Whitlock, C., Cross, W., Maxwell, B., Silverman, N., and Wade, A. A. (2017). 2017 Montana Climate Assessment. Bozeman and Missoula MT: Montana State University and University of Montana, Montana Institute on Ecosystems. doi: 10.15788/M2WW8W
Wilke, A. K., and Morton, L. W. (2015). Climatologists' communication of climate science to the agricultural sector. Sci. Commun. 37, 371–395. doi: 10.1177/1075547015581927
Wynne, B. (2006). Public engagement as a means of restoring public trust in science: hitting the notes but missing the music. Commun. Gene. 9, 211–220. doi: 10.1159/000092659
Yuan, X., Wood, E. F., Luo, L., and Pan, M. (2011). A first look at climate forecast system version 2 (CFSv2) for hydrological seasonal prediction. Geophys. Res. Lett. 38:L13402. doi: 10.1029/2011GL047792
Yung, L., Phear, N., DuPont, A., Montag, J., and Murphy, D. (2015). Drought adaptation and climate change beliefs amongst working ranchers in Montana. Weather Clim. Soc. 7, 281–293. doi: 10.1175/WCAS-D-14-00039.1
Keywords: climate change, agricultural decision-making, climate decision-making, climate information, climate communication, adaptation, climate services
Citation: Smith AP, Yung L, Snitker AJ, Bocinsky RK, Metcalf EC and Jencso K (2021) Scalar Mismatches and Underlying Factors for Underutilization of Climate Information: Perspectives From Farmers and Ranchers. Front. Clim. 3:663071. doi: 10.3389/fclim.2021.663071
Received: 02 February 2021; Accepted: 19 March 2021;
Published: 21 April 2021.
Edited by:
Zhao Ma, Purdue University, United StatesReviewed by:
Daniel Andres Rodriguez, Federal University of Rio de Janeiro, BrazilAna Paula M. A. Cunha, Centro Nacional de Monitoramento e Alertas de Desastres Naturais (CEMADEN), Brazil
Copyright © 2021 Smith, Yung, Snitker, Bocinsky, Metcalf and Jencso. This is an open-access article distributed under the terms of the Creative Commons Attribution License (CC BY). The use, distribution or reproduction in other forums is permitted, provided the original author(s) and the copyright owner(s) are credited and that the original publication in this journal is cited, in accordance with accepted academic practice. No use, distribution or reproduction is permitted which does not comply with these terms.
*Correspondence: Ada P. Smith, ada.smith@umontana.edu