- Department of Geography and Sustainable Development, University of Miami, Coral Gables, FL, United States
In recent years, there has been increasing research on the impact of climate change related processes on the occurrence of conflicts in different parts of the world. Analysis of conflicts over extended time periods indicate the possible role of climate change and climate variability on the occurrence of conflicts, particularly in the tropics. With the recent release of the UCDP georeferenced dataset on specific locations of conflicts between 1989 and 2014, we can now examine the temporal patterns of conflicts at a finer spatial resolution. In this study, the role of El Niño Southern Oscillation (ENSO) on the spatio-temporal patterns of conflicts has been analyzed by using a variety of geospatial analysis techniques. The results of the analysis reveal that there were a greater number of conflicts during El Niño years at both the global and continental scale (except North America). In addition, the spatial patterns of conflicts showed greater concentration of intensifying and consecutive hot spots in South and Southeast Asia, the Middle East, and Central and Eastern Africa during El Niño years vs. La Niña years. Specifically, intensifying hot spots of conflicts overlapped with the relatively arid and semi-arid areas of the Global South.
Introduction
The latest report of the Intergovernmental Panel on Climate Change (IPCC) highlights the potential role of climate change on the increasing rivalry between and within countries over shared resources. This can lead to an increase in the possibility of conflicts in already vulnerable areas often characterized by poor adaptation and mitigations strategies coupled with preexisting dysfunction in the broader political and social arena (IPCC, 2014). One of the main reasons attributed to the increase in conflicts due to climate change is droughts, which lead to a shortage of staple food crops, thereby resulting in the decline in primary sources of income along with widespread hunger. This further results in the propensity of rural to urban migration and ultimately civil unrest and in some cases violent conflicts (Burke et al., 2009; Zhang et al., 2011).
The indirect role of climate change, in the form of prolonged droughts on the Darfur conflict is widely considered to be the first human conflict triggered by climate change (Byers and Dragojlovic, 2004; Mazo, 2009; Akasha, 2012). However, several more recent detailed analyses have indicated that climate change may not be the sole reason for the Darfur conflict, but rather a complex set of factors including past legacy of violence, manipulation of ethnic divisions by elites, and a malfunctioning political system (Kevane and Gray, 2008; Sunga, 2011; Verhoeven, 2011). There is widespread evidence of the role of climate change-induced shortage in freshwater and arable land resulting in food insecurity. This food insecurity has led to armed and violent conflicts in several parts of the African continent (Burke et al., 2009; Benjaminsen et al., 2012; O'Loughlin et al., 2012, 2014; Carter and Veale, 2014).
For instance, several studies have cited a historically severe drought in Syria which resulted in the breakdown of agriculture in the rural areas creating widespread food shortages. This drought drove widespread rural-urban migration, particularly to the large cities of Syria (Gleick, 2014; Kelley et al., 2015). In fact, it has been estimated that 1.5 million people moved to the larger urban areas. This further caused an increase of already existing widespread unemployment in the urban areas, which ultimately resulted in the complete breakdown of social and political systems and the spread of civil unrest across the country.
The main objective of the present study is to examine the role of widely researched global teleconnection, ENSO on the spatial patterns of hotspots intensity of conflicts. ENSO occurs in the tropical Pacific spanning roughly a 2–7-year cycle and has had worldwide impacts on global weather. During the warm phase of ENSO, El Niño years, there are widespread droughts resulting from deficient rainfall in most parts of South and Southeast Asia, Australia, and Africa, while untimely excessive rainfall and flooding are reported along the west coasts of North and South America. During the cold phase of ENSO (La Niña years) normal to excessive rainfall is reported in Asia, Africa, and Australia. The aggravating role of ENSO on the historical occurrences of conflicts has been widely validated (Davis, 2002; Grove, 2007; Fagan, 2009) as well as in the modern era with conflicts in the warmer tropics doubling from 1950 to 2004 (Hsiang et al., 2011). However, there are limited studies examining the spatial patterns at the regional scale. Therefore, in this study various geostatistical analysis tools have been utilized to reveal the spatial patterns of conflicts and its relation to one of the most widely studied teleconnections, ENSO.
Data and Methods
In this study, conflicts data were accessed from the UCDP Georeferenced Event Dataset (UCDP GED), consisting of individual events of organized violence that occurred from 1989 to 2014 (Sundberg and Melander, 2013; Croicu and Sundberg, 2015). The different types of conflicts included state-based conflict, non-state conflict, and one-sided violence at the level of individual villages (Figures 1, 2). At the spatial scale, the location of each conflict was recorded in the form of x and y coordinates with the best spatial resolution available at the individual village or town level, country, and region. At the temporal scale, the finest resolution was at the daily level. The dataset includes records of only events linkable to a UCDP/PRIO Armed Conflict, a UCDP Non-State Conflict or a UCDP One-Sided Violence instance (Croicu and Sundberg, 2015). In addition to the year and location information, some of the other specific information available in the dataset include type of violence (state-based conflict, non-state conflict, one-sided conflict), name of the conflict, names of the actors engaged in the conflict (government, civilians), and number of sources available only for 2013 and 2014.
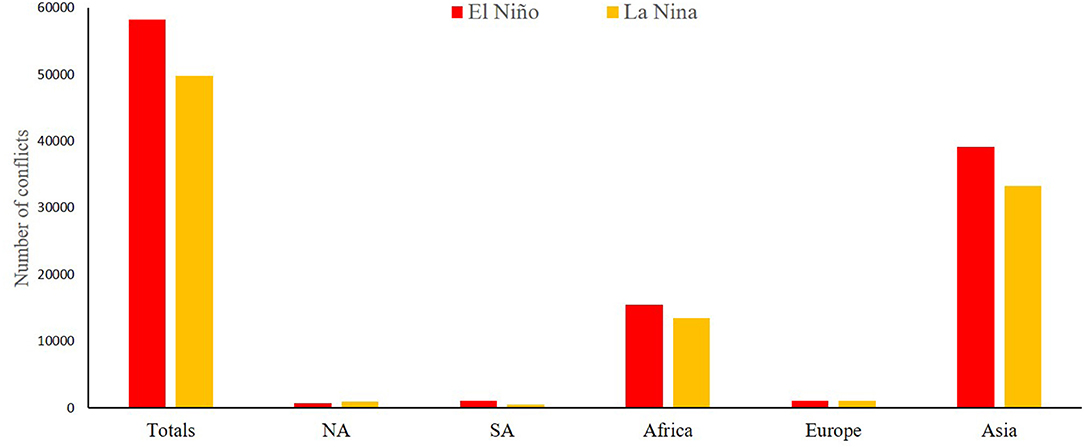
Figure 1. Conflicts at the global and continental scale during El Niño and La Niña years during 1989–2014.
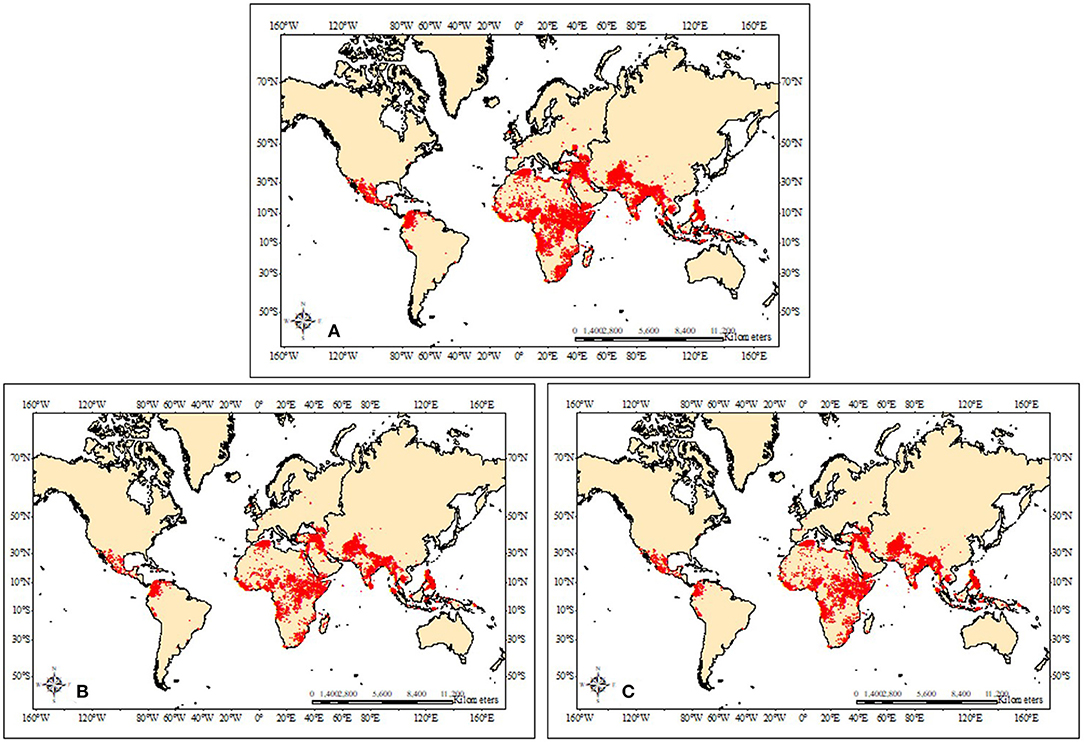
Figure 2. Distribution of conflicts from 1989 to 2014 (A) All years; (B) El Niño years; (C) La Niña years.
The impact of El Niño Southern Oscillation (ENSO) on regional climate patterns has been a major theme in climatology for many decades. It is considered as one of the most important climate phenomena on earth due to its ability to change the global atmospheric circulation, which in turn, influences temperature and precipitation across the globe. It consists of episodic fluctuations in temperature between the ocean and atmosphere in the tropical east-central Pacific Ocean (extending approximately between the International Date Line and 120 degrees west). It typically consists of three phases, which consists of the two opposite phases, “El Niño” and “La Niña,” and “Neutral” in the middle of the continuum between the two phases. Specifically, the El Niño phase is characterized by a warming or above-average sea surface temperatures (SST) in the tropical central and eastern Pacific Ocean surface. As a result, the rainfall over parts of South and Southeast Asia, and Australia tends to decline, while increased rainfall totals are observed over the tropical Pacific Ocean (Sen Roy, 2006). In addition, the low-level surface easterly winds, tend to weaken with some cases starting to blow as westerly winds (L'Heureux, 2014). It is also referred to as the warm phase of ENSO. This phenomenon was originally identified by fishermen off the coast of South America in the 1600s, with the appearance of abnormally warm water in the tropical Pacific Ocean. The name was chosen based on the time of year (around December) during which these warm waters events tended to occur (National Ocean Service, 2020). The opposite of El Niño is La Niña, which is characterized by a cooling of the tropical central and eastern Pacific Ocean surface, or below-average SST. During the cooler phase of ENSO, there is an overall increase in rainfall across South and Southeast Asia, and Australia. The normal easterly wind patterns along the equator become more established. It is also referred to as the cold phase of ENSO. Finally, the relatively less pronounced and explored mode of ENSO is the Neutral phase. The conditions during this phase are less distinct with closer to average SST in the tropical Pacific Ocean (L'Heureux, 2014).
There is no fixed frequency for the phase change of ENSO. Nonetheless, on an average El Niño and La Niña events occur every 2–7 years, with larger number of El Niño events than La Niña events. In addition, El Niño on average typically last for 9–12 months, while La Niña events occur for longer periods of 1–3 years (NOAA, 2020). However, there have been periods when El Niño events have lasted for 2–4 years, such as in 1990 to 1995 (Trenberth and Hoar, 1996). Both phases of ENSO tend to start developing during March to June, reaching their peak intensity during December to April, and finally weakening around May to July. Information about the classification of El Niño and La Niña Years were accessed from the Golden gate Weather Services (GGWS, 2016). The occurrence of conflicts was analyzed for all years together and separately for El Niño vs. La Niña years.
In order to reveal the differences in spatial extent of conflicts during the different phases of ENSO, Standard Deviational Ellipses (SDEs) were calculated for each year under the different phases separately (Figure 3). This is a widely used technique for measuring the trend in spatial spread and orientation for a set of locations. The SDE is calculated based on the deviation of X and Y location coordinates from the calculated mean center of coordinates. It is determined by taking into consideration the standard deviation of the x and y coordinates from the mean center to define the axes of the ellipse (Freedman and Sen Roy, 2012; Tullis-Joyce and Sen Roy, 2021). It is calculated using the following methodology:
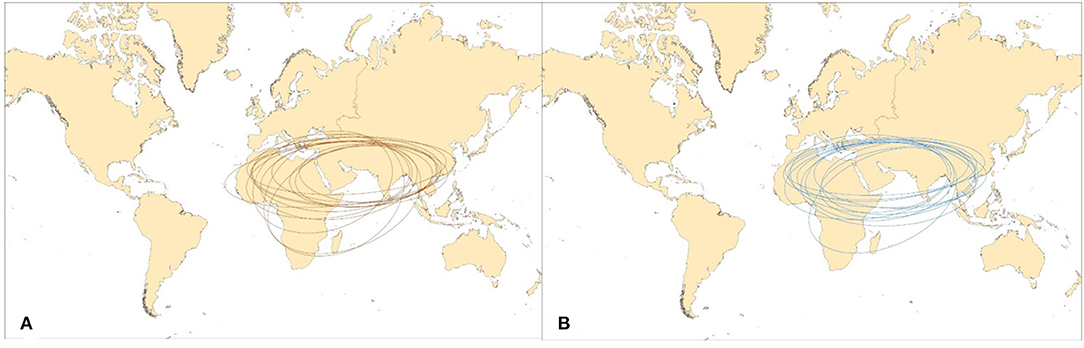
Figure 3. Annual Standard Deviational Ellipses (SDE) derived on the geographic location of conflicts each year from 1989 to 2014 (A) El Niño years; (B) La Niña years.
Where xi and yi are the coordinates for features i, {} represents the Mean Center for the features, and n is equal to the total number of features.
Where are the deviations of xy-coordinates from the Mean Center.
The standard deviations for the x-axis and y-axis are:
For further information about the mathematical background for the calculation of SDE readers are referred to Gong (2002) and ESRI (2021a).
In order to examine the spatial variation in not only the frequency but also the temporal variation in the concentration of hot spots over space, Emerging Hot Spots Analysis (EHSA) was used in this study. This technique calculates space time cubes through which the different categories of hotspots are identified, which include consecutive, intensifying, persistent, diminishing, sporadic, oscillating, and historical hotspots (Figure 4). A space–time cube is a netCDF file containing points in x and y coordinates, with a z-coordinate for time. This cube were used to calculate the hotspot trends among the cubes of data within the study area, consequently classifying them into cold- or hot-spot variations (Bunting et al., 2018; Wan and Sen Roy, 2021). It takes into consideration the neighborhood distance and time step parameter values to calculate the Getis-Ord Gi* statistic (Hot Spots Analysis) for each bin. Each bin is examined within the setting of neighboring bins to assess how intense clustering is for both high and low values. The results from this analysis are in the form of z-score, p-value, and binning category for each bin in the space-time cube. The time series of these z-scores at the locations analyzed is then evaluated using the Mann-Kendall statistic. Thus, the results from this analysis is a clustering trend z-score, p-value, and binning category for each location. Finally, the time series of the values at the locations analyzed is assessed using the Mann-Kendall statistic. The result from this analysis is useful in understanding where the presence tends to cluster in the frequency of occurrence over time (Carter et al., 2019; ESRI, 2021b). The definitions of various types of hotspots are briefly described below:
• A consecutive hot spot is a location with a single uninterrupted run of statistically significant (90% or higher) hot spot bins for all time-step intervals, with an increasing intensity over time (ESRI, 2021b).
• An intensifying hot spot is a location that has been a statistically significant (90% or higher) hot spot for 90% of the time-step intervals, including the final time step, with no distinct increasing or decreasing intensity (ESRI, 2021b).
• A persistent hot spot is a location that has been a statistically significant (90% or higher) hot spot for 90% of the time-step intervals with no discernible trend indicating an increase or decrease in the intensity of clustering over time, with a decreasing intensity of clustering over time (ESRI, 2021b).
• A diminishing hotspot is a location that has been a statistically significant (90% or higher) hot spot for 980% of the time-step intervals, including the final time step (ESRI, 2021b).
• A sporadic hot spot is a location, which are statistically significant (90% or higher) hot spots for <90% of the time, with none of the time steps as significant hot spots (ESRI, 2021b).
• An oscillating hot spot is defined as a location that is a hot spot for the final time step interval but has been a statistically significant (90% or higher) cold spot in previous time step intervals (ESRI, 2021b).
• Finally, a historical hot spot is a location which has been a hot spot for at least 90% of the time step intervals, even though it may not be a hot spot in the latest time step interval (ESRI, 2021b).
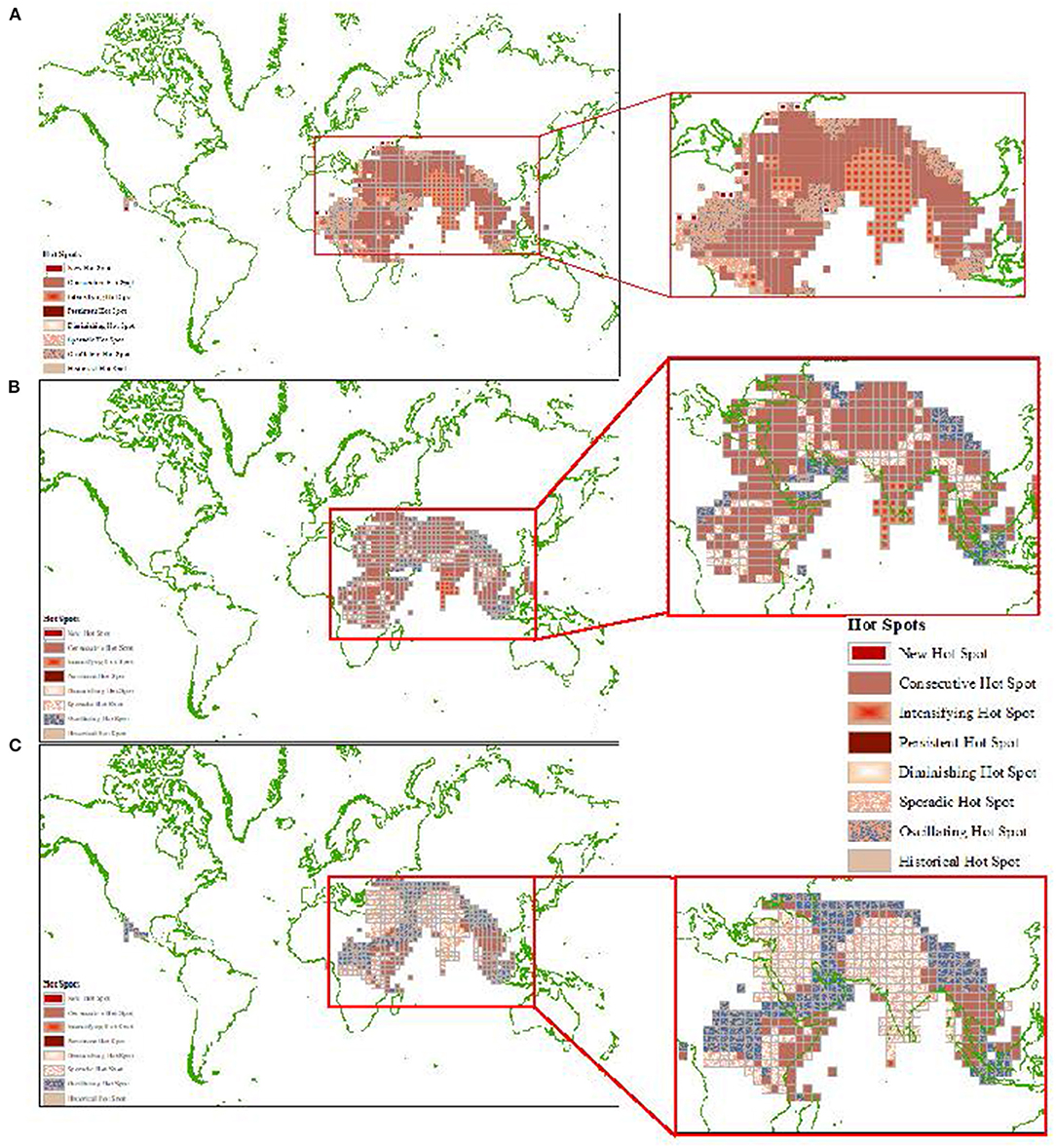
Figure 4. Results of emerging hot spots analysis of the spatial patterns of conflicts during 1989–2014 (A) All years; (B) El Niño years; (C) La Niña years.
In order to identify the intensity of the hotspots over time, this technique utilizes the Getis Ord Gi* statistic for each bin in the space time cube in relation to the values of the surrounding bins. For further information about EHSA technique, readers may refer to ESRI (2021b). The analysis was conducted for conflicts that occurred during the entire time period of 1989 to 2014, along with a separate analysis for El Niño and La Niña years.
Results and Discussion
The difference in total number of conflicts at the global and regional scales is presented in Figure 1. At the continental scale, except for North America, the number of conflicts was higher during El Niño years vs. La Niña years. The differences were largest in Asia (15,474 in El Niño years vs. 12,469 during La Niña years) and Africa (39,112 in El Niño years vs. 33,252 in La Niña years). The differences in the number of conflicts were <250 for both Europe (1,116 in El Niño years vs. 1,055 in La Niña years) and North America (727 in El Niño years vs. 952 in La Niña years).
The overall spread of conflicts was concentrated in the tropics, with sporadic occurrences in the higher latitudes of Europe and Northern and Southern America, with no presence in Australia (Figure 2). The regions of high concentration of conflicts also overlapped with the less developed regions in the tropics, widely referred to as the Global South. The Global South, consisting of Africa, Southeast Asia, the Middle East, Mexico, Central and South America, also overlaps with the less developed and lower income regions of the world. It consists of regions at different levels of development along with the highest population density and lowest per capita levels of income (Sen Roy, 2018a). Some of these regions in the Global South are also behind in technology, with massive gaps in basic infrastructure for their rapidly increasing populations. The economies of most of the countries in the Global South are less advanced and heavily dependent on the agricultural sector, with majority of their populations engaged in agricultural and pastoral activities. Moreover, there is substantial instability in the economic, political, and social spheres, which will increase the imminent impacts of climate change, such as severe weather events (including droughts, hurricanes, hailstorms, floods, landslide, and heat waves). This pervasive insecurity will lead to dislocation of populations, ethnic and local conflicts, starvation, and infectious diseases. As evident from Figure 2, the majority of the Global South is concentrated in the low-latitude regions near the tropics and subtropics, on both sides of the equator. It is noteworthy that 8 of the 10 most populous countries in the world are located in the Global South, which is projected to increase to 9 out of 10 countries by 2050 (Population Reference Bureau, 2015). Additionally, the top 10 countries with the highest fertility rates are all located in the Global South, specifically in Africa, accompanied with some of the lowest per capita GDP. Therefore, the effect of El Niño is not only limited to the agricultural sector, but also felt on the overall annual GDP in these regions.
However, this region also offers many opportunities in the form of emerging technologies, natural resources, and innovative approaches to dealing with the impacts of already occurring and impending impacts of climate change. For instance, one of the most menacing effects of global warming is considered to be sea level rise. As a result of sea level rise some of the smaller islands in the Caribbean and Pacific risk disappearing entirely. It is noteworthy that this is already happening in the densely populated Sundarban region located in the Bay of Bengal, where there is evidence of disappearance of some of the low-lying islands. This would affect a major proportion of the population in the Global South, due to the high density of population concentrated in the low lying coastal areas in the larger continents. Sea level rise would also have massive detrimental impacts on the small island nations, whose economies greatly depend on tourism. It is estimated that 25% of the population across the African continent will be impacted by sea level rise (Juma, 2010). On the other hand, desertification is another major impact of climate change that will affect major areas of the Global South, including the Middle East, Southern Africa, Central and East Asia. There is evidence that the recent conflicts in Darfur were aggravated by desertification (Juma, 2010). On the contrary, extensive flooding, in parts of northwestern Pakistan, Bangladesh, the Philippines and other parts of Asia, has been triggered by abnormally extreme heavy precipitation events and hurricanes. This has resulted in cutting off large populations' access to food and relief for days and weeks. There is also evidence of significant increasing trends in the occurrence of extreme temperatures and rainfall, which has widespread impacts on the vulnerable population such as that observed in India (Sen Roy and Balling, 2004; Sen Roy, 2009, 2019). Additionally, the adverse impacts of climate change are exaggerated on vulnerable populations including low-income communities, women, and children. Besides, the access to limited essential resources, including clean drinking water and livelihood opportunities, is compromised to a greater extent for the people living in the Global South.
In this context, it has been highlighted that in view of the uncertainties related with future climate conditions and projected changes in the frequency of extreme weather events, there is medium evidence and high agreement about the heightened displacement of people (IPCC, 2014). Most of this displacement will be concentrated in the low income densely-populated low lying regions of the Global South. People who have to involuntarily move out of their homes as a result of stress resulting from climate change and global warming are collectively called climate refugees. They are a subset of environmental refugees (Sen Roy, 2018b). Some of the direct impacts of climate change that lead to this forced movement of population, include droughts leading to food insecurity, sea level rise leading to loss of livelihood and homes, and intense competition for the limited resources. In addition, indirect impacts also include conflicts because of food insecurity such as observed in parts of Africa. In this context, one of the main aspects of climate change is global warming experiences in the form of rising temperatures. The effect of these steadily increasing temperatures is noticeable in the swift rate of melting of ice caps in the high altitude and latitude areas, which results in sea level rise and ultimate flooding of densely-populated low lying areas, particularly in the tropics. Therefore, it is critical to examine the impacts of climate change on conflicts in the Global South.
The general spatial extent of the total number of conflicts between El Niño and La Niña years, and all years does not show significant differences, other than a wider spread in northern part of South America, Southeastern Africa, and the adjacent island of Madagascar during El Niño years (Figures 2A–C). However, more rigorous geostatistical analysis revealed substantial differences in the spatial patterns. The annual SDEs mapped for each year separately reveal a wider spatial spread particularly over the African continent during El Niño years. Thus, there were not only greater number of conflicts during El Niño years, but also a greater variation in the spatial spread of conflicts with a more northeast-south southwest orientation in certain years (Figures 3A,B). It is noteworthy that El Niño is not the only cause for conflicts, but rather an additive factor in vulnerable situations caused by other processes such as border issues and religions.
In order to understand the trends in the spatial patterns of conflicts over the study period, the results of EHSA have been mapped (Figure 4). This technique is particularly useful for mapping local level trends in the spatial patterns of phenomena. The results of the spatial patterns analysis for conflicts for all years cumulatively reveal most of the hotspots concentrated over Central Africa, Middle East, South and Southeast Asia (Figure 4A). The intensifying hotspots are located mostly over South Asia, in the Indian subcontinent, including Sri Lanka and parts of Myanmar (Figure 4A). The core region of intensifying hot spots is surrounded by a concentric region of consecutive hotspots, which include Central Africa, Middle East, Afghanistan, Pakistan, Bangladesh, parts of Southern China, and Southeast Asia. Beyond the region of consecutive hot spots, there are regions of oscillating hotspots.
However, the spatial patterns are substantially different when the years are split into El Niño and La Niña years. During the El Niño years, the spatial extent of consecutive hotspots is much wider over Central and Northeastern Africa and most of the Middle East, except the Arabian Desert. In Asia, the region of consecutive hotspots was concentrated in Afghanistan, Central India, Bangladesh, and the Indo-China peninsula, including Myanmar and the Mekong River basin region in Southeast Asia (Figure 4B). It is noteworthy, that there is widespread evidence of negative relationship between El Niño and precipitation (Sen Roy and Sen Roy, 2011). On the contrary, during La Niña years there are very limited areas of consecutive hotspots mainly concentrated in parts of Eastern Africa, the horn of Africa near Ethiopia and Somalia, and the Mekong River basin (Figure 4C). Most of Central Africa was replaced by oscillating and some sporadic hotspots, and predominantly sporadic hotspots across the Indian subcontinent. Thus, it is clearly evident that the consecutive and intensifying hotspots of conflicts have a much wider spread during El Niño years.
Furthermore, the areas experiencing consecutive hotspots during El Niño years, including South (Krishnamurthy and Goswami, 2000) and Southeast Asia (Xu et al., 2004), and Central and Eastern Africa (Nicholson and Kim, 1997), are associated with deficient precipitation and in some cases severe droughts. There is substantial literature highlighting the occurrence of below normal rainfall during El Niño years in these regions. As evidenced in the recent conflicts of Syria and Sudan, the disruption in basic food supplies can often lead to an imbalance in already fragile situations. It also leads to large scale rural-urban migration in search of employment opportunities, which in turn exerts unsustainable pressure on thinly spread and severely stressed urban infrastructure (Sen Roy, 2018b).
Specifically, conflicts during El Niño years tend to concentrate in arid and semiarid areas of Asia and Africa. These areas include North and South Sudan, and the Eastern highlands of Africa in Ethiopia, which experience semi-arid to arid weather conditions and associated with lower than normal rainfall during El Niño years (Seleshi and Demaree, 1995). Similarly, Central India, Pakistan, and Afghanistan, which are comprised mostly of semi-arid to arid areas experience deficient rainfall during El Niño years (Sen Roy et al., 2003; Mahmood et al., 2004). On the other hand, in the relatively wetter regions of Southern Africa, where El Niño has a stronger modulating role on the local rainfall, the spatial patterns of occurrence of conflicts do not show significant intensification. Similarly, the wetter regions of South Asia, including the Gangetic plains in the Indian subcontinent, and South East Asia, though significantly affected by El Niño in the form of deficient rainfall, do not show significant intensification of conflicts. On the contrary, during the La Niña years there is substantial de-intensification of the hot spots in South and Southeast Asia and Central America, matching with relative more wet years.
Conclusions
In the recent few decades, research on climate change has expanded significantly with greater consensus about questions regarding the buildup of greenhouse gases in the atmosphere and the magnified role of anthropogenic activities. Some of the main findings concerning long-term trends in climate change from the latest Fifth Assessment Report of the IPCC (Stocker et al., 2013) are briefly summarized below:
• It has been widely confirmed that the entire globe has experienced warming, although with considerable decadal and inter-annual variability. The average global linear trend for combined land and ocean surface temperatures indicate a warming of 0.85 (0.65 to 1.06) °C between 1880 and 2012. For the most recent decade, 2003–2012, this trend is 0.78 (0.72 to 0.85) °C.
• There is significant evidence demonstrating the very likely decrease in the number of cold days and nights, as well as an increase in the number of warm days and nights on a global scale. These findings are further intensified by the increased frequency of heat waves in parts of Europe, Asia, and Australia.
• Furthermore, increases in number of heavy precipitation events have been observed in many regions of the world.
In general, the maximum negative impact of climate change will be on the less developed regions of the world, concentrated mainly in the Global South. However, there is substantial differences in terms of regional variations in the impacts of climate change. Insufficient long-term data records, as well as scarce coverage in several countries lead to some of these variations. In addition, the severe social and economic impacts of climate change are further aggravated by pervasive poverty and absence of adequate public infrastructure. Therefore, a better understanding of the impacts of global environmental change will require a unified international effort to assemble, synthesize, and examine the relevant data in order to decide on the most critical actions (Stern, 2007). More importantly, there is widespread ambiguity about how local populations and their environments will be impacted by and adapt to these variable conditions at different spatial scales. This is particularly true in the more vulnerable regions of the Global South. The results of this study can help in the prevention or better management of conflicts in the future particularly in the Global South. Additionally, the results of this study may also help in the future prevention of conflicts through better management of resources, particularly in the arid and semiarid regions of the Global South.
Data Availability Statement
The original contributions presented in the study are included in the article/supplementary material, further inquiries can be directed to the corresponding author/s.
Author Contributions
The author confirms being the sole contributor of this work and has approved it for publication.
Conflict of Interest
The author declares that the research was conducted in the absence of any commercial or financial relationships that could be construed as a potential conflict of interest.
Publisher's Note
All claims expressed in this article are solely those of the authors and do not necessarily represent those of their affiliated organizations, or those of the publisher, the editors and the reviewers. Any product that may be evaluated in this article, or claim that may be made by its manufacturer, is not guaranteed or endorsed by the publisher.
References
Akasha, M. O. (2012). Climate Change as a Cause of Intra-State Conflicts: Darfur Case Study. Available online at: http://ssrn.com/abstract=2219947 (accessed September 15, 2012).
Benjaminsen, T. A., Alinon, K., Buhaug, H., and Buseth, J. T. (2012). Does climate change drive land-use conflicts in the Sahel? J. Peace Res. 49, 97–111. doi: 10.1177/0022343311427343
Bunting, R., Chang, O. Y., Cowen, C., Hankins, R., Langston, S., Warner, A., et al. (2018). Spatial patterns of larceny and aggravated assault in Miami-Dade county, 2007-2015. Professional Geographer. 70, 34–46. doi: 10.1080/00330124.2017.1310622
Burke, M. B., Miguel, E., Satyanath, S., Dykema, J. A, and Lobell, D. B. (2009). Warming increases the risk of civil war in Africa. Proc. Natl. Acad. Sci. U. S. A. 106, 20670–20674. doi: 10.1073/pnas.0907998106
Byers, M., and Dragojlovic, N. N. (2004). Darfur: a climate change-induced humanitarian crisis? Hum. Security Bull. 16–18.
Carter, J., Louderback, E. R., Vildosola, D., and Sen Roy, S. (2019). Crime in an Affluent City: spatial patterns of property crime in coral gables, FL. Eur. J. Crim. Policy Res. 26, 547–570. doi: 10.1007/s10610-019-09415-5
Carter, T. A., and Veale, D. J. (2014). The timing of conflict violence: hydraulic behavior in the Ugandan civil war. Conflict Manag. Peace 32, 370–394. doi: 10.1177/0738894214559674
Croicu, M., and Sundberg, R. (2015). UCDP GED Codebook Version 2, 0. Uppsala University, Uppsala. Available online at: https://ucdp.uu.se/downloads/ged/ucdp-ged-40-codebook.pdf
Davis, M. (2002). Late Victorian Holocausts: El Niño Famines and the Making of the Third World. Verso Books.
ESRI (2021a). How Directional Distribution (Standard Deviational Ellipse) Works. Avaialble online at: https://desktop.arcgis.com/en/arcmap/10.3/tools/spatial-statistics-toolbox/h-how-directional-distribution-standard-deviationa.htm (accessed December 6, 2021).
ESRI (2021b). How Emerging Hot Spot Analysis Works. Available online at: https://pro.arcgis.com/en/pro-app/latest/tool-reference/space-time-pattern-mining/learnmoreemerging.htm#GUID-09587AFC-F5EC-4AEB-BE8F-0E0A26AB9230 (accessed August 1, 2021).
Freedman, R., and Sen Roy, S. (2012). Spatial patterning of Manta Biostris in United States east coast offshore habitat. Appl. Geogr. 32, 652–659. doi: 10.1016/j.apgeog.2011.07.015
GGWS (2016). Climate Prediction Center: Cold & Warm Episodes by Season. Golden Gate Weather Services. Available online at: https://ggweather.com/enso/oni.htm
Gleick, P. H. (2014). Water, drought, climate change, and conflict in Syria. Weather Clim. Soc. 6, 331–340. doi: 10.1175/WCAS-D-13-00059.1
Gong, J. (2002). Clarifying the standard deviational ellipse. Geogr. Anal. 34, 155–167. doi: 10.1111/j.1538-4632.2002.tb01082.x
Grove, R. H. (2007). The great El Niño of 1789–93 and its global consequences: Reconstructing an extreme climate event in world environmental history. Medieval Hist. J. 10, 75–98. doi: 10.1177/097194580701000203
Hsiang, S. M., Meng, K. C., and Cane, M. A. (2011). Civil conflicts are associated with the global climate. Nature 476, 438–441. doi: 10.1038/nature10311
IPCC (2014). Climate Change 2014: Impacts, Adaptation, and Vulnerability. Part A: Global and Sectoral Aspects. Contribution of Working Group II to the Fifth Assessment Report of the Intergovernmental Panel on Climate Change, eds C.B Field, V. R. Barros, D. J. Dokken, K. J. Mach, M. D. Mastrandrea, T. E. Bilir, M. Chatterjee, K. L. Ebi, Y. O. Estrada, R. C. Genova, B. Girma, E. S. Kissel, A. N. Levy, S. MacCracken, P. R. Mastrandrea, and L. L.White (Cambridge; New York, NY: Cambridge University Press). p. 1132.
Juma, M. (2010). Security and Regional Cooperation in Africa: How Can We Make Africa's Security Architecture Fit for the New Challenges. Climate Change Resources Migration: Securing Africa in an Uncertain Climate. Cape Town: Heinrich Böll Foundation Southern Africa.
Kelley, C. P., Mohtadi, S., Cane, M. A., Seager, R., and Kushnir, Y. (2015). Climate change in the Fertile Crescent and implications of the recent Syrian drought. Proc. Natl. Acad. Sci. U. S. A.112, 3241–3246. doi: 10.1073/pnas.1421533112
Kevane, M., and Gray, L. L. (2008). Darfur: rainfall and conflict. Environ. Res. Lett. 3, 034006. doi: 10.1088/1748-9326/3/3/034006
Krishnamurthy, V., and Goswami, B. N. (2000). Indian monsoon–ENSO relationship on interdecadal timescale. J. Clim. 13, 579–595. doi: 10.1175/1520-0442(2000)013<0579:IMEROI>2.0.CO;2
L'Heureux, M. (2014). What is the El Niño–Southern Oscillation (ENSO) in a nutshell? Available online at: https://www.climate.gov/news-features/blogs/enso/what-el-ni%C3%B1o%E2%80%93southern-oscillation-enso-nutshell (accessed October 21, 2020).
Mahmood, A., Khan, T. M., and Faisal, N. (2004). Correlation between multivariate Enso Index (MEI) and Pakistan's summer rainfall. Pak. J. Meteorol. 1, 53–64.
Mazo, J. (2009). Climate change and security. Adelphi Papers 49, 73–86. doi: 10.1080/19445571003755538
National Ocean Service (2020). What are El Niño and La Niña? Available online at: https://oceanservice.noaa.gov/facts/ninonina.html (accessed October 21, 2020).
Nicholson, S. E., and Kim, J. (1997). The relationship of the El Niño–Southern Oscillation to African rainfall. Int. J. Climatol. 17, 117–135.
NOAA (2020). ENSO 101. Available online at: https://psl.noaa.gov/enso/ (accessed October 21, 2020).
O'Loughlin, J., Linke, A. M., and Witmer, F. D. W. (2014). Effects of temperature and precipitation variability on the risk of violence in sub-Saharan Africa, 1980–2012. Proc. Natl. Acad. Sci U. S. A. 111, 16712–16717. doi: 10.1073/pnas.1411899111
O'Loughlin, J., Witmer, F. D. W., Linke, A. M., Laing, A., Gettelman, A., and Dudhia, J. (2012). Climate variability and conflict risk in East Africa, 1990-2009. Proc. Natl. Acad. Sci U. S. A. 109, 16344–18349. doi: 10.1073/pnas.1205130109
Population Reference Bureau. (2015). World Population Data Sheet 2014. Available online at: http://www.prb.org/pdf14/2014-world-population-data-sheet_eng.pdf (accessed June 24, 2015).
Seleshi, Y., and Demaree, G. (1995). Rainfall variability in the Ethiopian and Eritrean highlands and its links with the Southern Oscillation Index. J. Biogeogr. 22, 945–952. doi: 10.2307/2845995
Sen Roy, S. (2006). The impacts of ENSO, PDO, and local SSTs on winter precipitation in India. Phys. Geogr. 27, 464–474. doi: 10.2747/0272-3646.27.5.464
Sen Roy, S. (2009). A spatial analysis of extreme hourly precipitation patterns in India. Int. J. Climatol. 29, 345–355. doi: 10.1002/joc.1763
Sen Roy, S. (2018a). “Climate change in the Global South: trends and spatial patterns,” in Linking Gender to Climate Change Impacts in the Global South (Cham: Springer), 1–25. doi: 10.1007/978-3-319-75777-3
Sen Roy, S. (2018b). “Climate refugees,” in Linking Gender to Climate Change Impacts in the Global South (Cham: Springer), 1–25.
Sen Roy, S. (2019). Spatial patterns of trends in seasonal extreme temperatures in India during 1980–2010. Weather Climate Extremes 24, 100203. doi: 10.1016/j.wace.2019.100203
Sen Roy, S., and Balling, R. C. Jr. (2004). Trends in extreme daily precipitation indices in India. Int. J. Climatol. 24, 457–466. doi: 10.1002/joc.995
Sen Roy, S., Goodrich, G., and Balling, R. C. Jr. (2003). Influence of El Niño/Southern Oscillation, Pacific Decadal Oscillation, and local sea surface temperature anomalies on peak season monsoon precipitation in India. Clim. Res. 25, 171–178. doi: 10.3354/cr025171
Sen Roy, S., and Sen Roy, N. (2011). Influence of Pacific Decadal Oscillation and El Niño- Southern Oscillation on the summer monsoon precipitation in Myanmar. Int. J. Climatol. 31, 14–21. doi: 10.1002/joc.2065
Stern, N. (2007). The Economics of Climate Change: The Stern Review. Cambridge: Cambridge University Press.
Stocker, T. F., Qin, D., Plattner, G. K., Alexander, L. V., Allen, S. K., Bindoff, N. L., et al. (2013). “Technical summary,” in Climate change 2013: the physical science basis. Contribution of Working Group I to the Fifth Assessment Report of the Intergovernmental Panel on Climate Change. Cambridge: Cambridge University Press, 33–115.
Sundberg, R., and Melander, E. (2013). Introducing the UCDP georeferenced event dataset. J. Peace Res. 50, 523–532. doi: 10.1177/0022343313484347
Sunga, L. S. (2011). Does climate change kill people in Darfur? J. Hum. Rights Environ. 2, 64–85. doi: 10.4337/jhre.2011.01.04
Trenberth, K. E., and Hoar, T. J. (1996). The 1990–1995 El Niño-Southern oscillation event: longest on record. Geophys. Res. Lett. 23, 57–60. doi: 10.1029/95GL03602
Tullis-Joyce, P., and Sen Roy, S. (2021). Occurrence of Karenia brevis near Southwest Florida coast 1971 to 2017: a geospatial analysis. J. Coastal Conserv. 25, doi: 10.1007/s11852-021-00844-1
Verhoeven, H. (2011). Climate change, conflict and development in Sudan: global Neo-Malthusian narratives and local power struggles. Dev. Change 42, 679–707. doi: 10.1111/j.1467-7660.2011.01707.x
Wan, C., and Sen Roy, S. (2021). A geospatial characteristics of fire occurrences in southern hemispheric Africa and Madagascar during 2001-2020. J. For. Res. (In Press)
Xu, Z. X., Takeuchi, K., and Ishidaira, H. (2004). Correlation between El Niño–Southern Oscillation (ENSO) and precipitation in South-east Asia and the Pacific region. Hydrol. Process 18, 107–123. doi: 10.1002/hyp.1315
Keywords: conflicts, Global South, climate change, El Niño, La Niña, ENSO (El Nino Southern Oscillation), emerging hot spot analysis
Citation: Roy SS (2022) Role of ENSO on Conflicts in the Global South. Front. Clim. 4:752355. doi: 10.3389/fclim.2022.752355
Received: 02 August 2021; Accepted: 17 January 2022;
Published: 10 February 2022.
Edited by:
Huade Guan, Flinders University, AustraliaReviewed by:
Mark Doubell, South Australian Research and Development Institute, AustraliaHailong Wang, Sun Yat-sen University, China
Copyright © 2022 Roy. This is an open-access article distributed under the terms of the Creative Commons Attribution License (CC BY). The use, distribution or reproduction in other forums is permitted, provided the original author(s) and the copyright owner(s) are credited and that the original publication in this journal is cited, in accordance with accepted academic practice. No use, distribution or reproduction is permitted which does not comply with these terms.
*Correspondence: Shouraseni Sen Roy, ssr@miami.edu