- 1Department Climate and Environmental Consultancy, Deutscher Wetterdienst, Offenbach am Main, Germany
- 2Institute of Meteorology, Freie Universität Berlin, Berlin, Germany
- 3Water Management and Flood Protection, Wupperverband, Wuppertal, Germany
Water boards in Germany require decadal predictions to develop optimized management and adaptation strategies, especially within the claims of flood protection and water distribution management. Specifically, the Wupper catchment water board in western Germany is interested in decadal predictions of drought indices, which are correlated to dam water levels. For the management of small catchments, they need multi-year means and multi-year seasonal means of the hydrological seasons for forecast years 1–3 at high spatial resolution. Thus, the MPI-ESM-LR global decadal prediction system with 16 ensemble members at 200 km resolution was statistically downscaled with EPISODES to ~11 km in Germany. Simulated precipitation was recalibrated, correcting model errors and adjusting the ensemble spread. We tested different recalibration settings to optimize the skill. The 3-year mean and 3-year seasonal mean SPI (Standardized Precipitation Index), indicating excess or deficit of precipitation, was calculated. We evaluated the prediction skill with HYRAS observations, applying skill scores and correlation coefficients, and tested the significance of the skill at a 95% level via 1,000 bootstraps. We found that the high-resolution statistical downscaling is able to preserve the skill of the global decadal predictions and that the recalibration can clearly improve the precipitation skill in Germany. Multi-year annual and August–October mean SPI predictions are promising for several regions in Germany. Additionally, there is potential for skill improvement with increasing ensemble size for all temporal aggregations, except for November–January. A user-oriented product sheet was developed and published on the Copernicus Climate Change Service website (https://climate.copernicus.eu/decadal-predictions-infrastructure). It provides 3-year mean probabilistic SPI predictions for the Wupper catchment and north-western Germany. For 2021–2023, a high probability of negative SPI (dry conditions) is predicted in most of the area. The decadal prediction skill is higher than using the observed climatology as reference prediction in several parts of the area. This case study was developed in cooperation with the Wupper catchment water board and discussed with further German water managers: The skill of high-resolution decadal drought predictions is considered to be promising to fulfill their needs. The product sheet is understandable, well-structured and can be applied to their working routines.
Introduction
Water resources and food security are strongly impacted by large-scale droughts (Benson and Clay, 1998). In spring and summer 2018, Central and Northern Europe were hit by strong drought conditions, resulting in reductions of crop yields up to 50%, which are projected to be a common occurrence in the mid of the 21st century (Toreti et al., 2019). This long-lasting and strong summer drought seems to be more extreme than the drought of 2003, resulting in larger influence on forest ecosystems in Central Europe (Schuldt et al., 2020). The combined drought and heat affected river navigation, impacting the transportation and tourism sectors (Wieland and Martinis, 2020). On the other hand, Central Europe experienced extreme rainfall and flooding in summer 2021, damaging local critical infrastructure systems, such as bridges, schools and hospitals (Koks et al., 2021). The observed heavy rainfall amounts strongly exceeded historical records in several parts of the affected area and caused over 200 fatalities (Kreienkamp et al., 2021). This catastrophe discloses the needs to decrease vulnerabilities and improve adaptive capacities in the context of future challenges of climate variations (Bosseler et al., 2021).
Such events raise the question which climate information on extreme events is needed by the German water management sector to implement appropriate actions to adapt to future climate variability (Changnon, 2003). Answers are found in intensive discussions with climate data users on workshops and individual meetings at the Deutscher Wetterdienst (DWD) and Copernicus Climate Change Service (C3S). To optimize management and adaptation plans mainly for water distribution and flood protection in the face of climate variations in the upcoming years, water boards require decadal predictions of atmospheric variables for hydrological impact modeling in Germany—since planning processes often take time. Additionally, the skill and uncertainties of the predictions need to be communicated. For instance, the Wupper catchment water board (Wupperverband) controls water level and quality of 14 dams in a catchment area of 813 km2 in western Germany. It requires decadal predictions of the Standard Precipitation Index (SPI, McKee et al., 1993) or alternatively of the Standardized Precipitation Evapotranspiration Index (SPEI, Vicente-Serrano et al., 2010) for the coming 3 years, because drought indices are correlated to water levels of dams (Lorza-Villegas et al., 2021). To manage smaller dams and river catchments high spatial resolution is essential. Different temporal aggregations, such as multi-year means of the calendar year and the four hydrological seasons, are required to cover different water management and also natural processes, like the vegetation period.
These needed decadal climate predictions of the next 1–10 years lie between seasonal forecasts and climate projections and are of particular interest for mid-term water resource managers (Meehl et al., 2009). Multi-model decadal predictions were coordinated in the Coupled Model Intercomparison Project Phase 6 (CMIP6, Eyring et al., 2016), and the World Meteorological Organization (WMO) Lead Centre for Annual to Decadal Climate Predictions (ADCP) publishes a Global Annual to Decadal Climate Update (GADCU, Hermanson et al., 2022). Predictability arises from greenhouse gas and aerosol forcing (Van Oldenborgh et al., 2012) and the initialization of the ocean (Matei et al., 2012), land surface or sea ice (Bellucci et al., 2015) with observations. Thus, skill is found for ocean temperature in the North Atlantic subpolar gyre (Hermanson et al., 2014), surface temperature, precipitation and atmospheric circulation (Smith et al., 2019), such as the North Atlantic Oscillation (NAO) impacting western Europe (Athanasiadis et al., 2020; Smith et al., 2020). Statistical postprocessing procedures like recalibration (Pasternack et al., 2018, 2021) can further improve skill by adjusting model bias, drift and ensemble spread toward observed statistics.
The drought indices required by water managers compare the available water amount with a long-term climatological value (Palmer, 1965). The SPI divides the anomaly of rainfall by its standard deviation (McKee et al., 1993) but works inappropriately in dry areas because rising temperature and evapotranspiration due to climate change are not regarded (Lloyd-Hughes and Saunders, 2002). The SPEI (Vicente-Serrano et al., 2010) standardizes the difference between precipitation and potential evapotranspiration (PET), i.e., the climate water balance. If the PET is parameterized following Thornthwaite (1948), the SPEI cannot be assessed for colder regions like Germany (Paxian et al., 2019). Several recent studies analyze the prediction skill of drought indices: Paxian et al. (2019) find high 4-year mean SPEI skill in the tropics, e.g., northern Africa, and smaller SPI skill hot spots at 5° resolution. Higher 2° resolution improves spatial structures mostly without reducing skill. Solaraju-Murali et al. (2021) investigate the SPEI skill for the months before the wheat harvest and find decadal prediction skill for several global regions. Decadal predictions are feasible for soil water storage in North America, given a correct soil initialization (Chikamoto et al., 2015), and skillful for Sahel summer rainfall (Sheen et al., 2017). In Europe, Solaraju-Murali et al. (2019) detect skill for the 5-year mean summer SPI in Scandinavia and neighboring areas and for the SPEI in Southern Europe. A C3S case study for the energy sector1 finds skillful predictions of the 10-year extended winter precipitation in Southern European river basins based on a multi-model NAO prediction.
To reach a higher resolution of climate predictions different downscaling approaches were analyzed in former studies: Dynamical downscaling has larger computational costs but reveals skill for annual and seasonal temperature means (Feldmann et al., 2019) and added values for temperature and precipitation in some European areas compared to the global model (Reyers et al., 2019). Regional prediction skill is stated for user-oriented quantities and extremes, such as frost, heat wave or growing degree days (Moemken et al., 2020). Cost-efficient statistical-dynamical downscaling reveals skill for annual mean wind speed and wind energy output, partly preserving and partly improving the global model skill (Moemken et al., 2016). For West African rainfall, dynamical downscaling of decadal predictions shows the ability to reduce bias (Paxian et al., 2016) and improve skill (Paeth et al., 2017). In the United States, statistical downscaling improves decadal rainfall predictions for impacts assessments at high resolution (Salvi et al., 2017). As to drought indices, downscaling of a multi-model seasonal SPEI prediction for 6 months in winter and spring for water management in South Korea improves skill (Sohn et al., 2013). Instead, global model skill is mostly preserved in statistical downscaling of seasonal predictions for hydropower production in Germany and Portugal (Ostermöller et al., 2021).
Given the skill of decadal predictions and their importance for society in terms of climate adaptation and resilience, first steps toward developing user-oriented decadal prediction products can be taken (Kushnir et al., 2019). The information should be tailored to user needs, paying attention to its format and its applicability in user working routines (Bruno Soares et al., 2018). The design of climate service prototypes based on seasonal forecasts in the EUPORIAS project highlights the importance of user interaction and involvement in the development of successful services (Buontempo et al., 2018). To address the needs of climate-sensitive sectors and inform decision-making in the context of climate risks the C3S offers climate datasets and sector-specific applications already (Buontempo et al., 2019). Its first step toward prototype climate services based on decadal predictions is the C3S_34c contract developing case studies for the insurance, agriculture, energy and infrastructure sectors (Dunstone et al., 2022). Both the user co-development and the exchange between scientific partners proved to be essential for developing sectoral applications for decadal predictions to be published on the C3S website2.
Thus, this study analyzes high-resolution decadal drought predictions needed by German water boards to set up water management and flood protection plans. The Wupper catchment is chosen as case study, and its water board co-develops the C3S_34c climate service on decadal predictions for the infrastructure sector3. To achieve the necessary high resolution, the German decadal prediction system MPI-ESM is statistically downscaled to a resolution of ~11 km in Germany. We chose the cost-efficient statistical downscaling due to the large decadal hindcast set. Simulated precipitation is statistically recalibrated to address model errors and standardized to calculate the SPI because it might be difficult to assess the PET for SPEI in colder regions such as Germany. The SPI prediction is estimated for 3-year means of the calendar year and four hydrological seasons, and skill is evaluated with observations. A user-oriented product sheet is developed and discussed with different German water managers. Thus, Section MATERIALS AND METHODS of the manuscript describes the model and observational data applied and the methods used: the statistical downscaling, the recalibration procedure, the calculation of the SPI, the skill assessment and the computation and display of the probabilistic prediction. Section RESULTS illustrates the impacts of downscaling and recalibration and shows the SPI skill and prediction for 2021–2023 for all temporal aggregations. In addition, the resulting product sheet is presented, and the user co-production and feedback is illuminated. Finally, Section DISCUSSION gives a summary of the major results, highlights relevant conclusions and draws a final outlook.
Materials and Methods
This section presents the global decadal prediction system, the statistical downscaling for Germany and the observational dataset used in this study. Furthermore, the post-processing including the statistical recalibration and the calculation of the SPI are described. Finally, the methods to calculate probabilistic predictions and to assess prediction skill scores are explained.
Global Decadal Climate Predictions
The global decadal predictions are taken from the Max Planck Institute for Meteorology Earth System Model Low Resolution Version 1.2 (MPI-ESM-LR) which consists of the coupled atmosphere-ocean model ECHAM6/MPIOM. The atmosphere reveals a horizontal resolution of ~200 km and 47 vertical levels, and the oceanic component features a GR15 (~1.5°) resolution and 40 levels (Jungclaus et al., 2013; Pohlmann et al., 2013; Stevens et al., 2013). The atmosphere is initialized nudging full-fields of ERA40 reanalyses (Uppala et al., 2005) before 1978 and ERA5 reanalyses (Hersbach et al., 2020) after. The ocean initialization is based on temperature and salinity anomalies from the EN4 observations (Good et al., 2013) assimilated in the ocean via an Ensemble Kalman filtering method (Brune et al., 2015). This MPI-ESM-LR assimilation run is the basis for the initialization of global decadal predictions on 1st November in every year from 1960 until 2020 for a 10-year simulation period. The prediction ensemble consists of 16 members which were started from different ocean states. The external forcing was taken from CMIP6, including observed states before 2015 and the SSP245 (Shared Socioeconomic Pathways, Fricko et al., 2017) scenario after. The global decadal prediction data of MPI-ESM-LR will soon in 2022 be available on the ESGF (Earth System Grid Federation) node at DWD4.
Statistical Downscaling
To fulfill the needs of the German water management sector for high-resolution predictions the empirical–statistical downscaling method EPISODES (Kreienkamp et al., 2018, 2020) was applied to downscale the MPI-ESM-LR global predictions to ~11 km in Germany. The other global decadal prediction systems of the C3S_34c partners, i.e., CMCC-CM2-SR5, EC-Earth3 and DePreSys4, could not provide the necessary input data for statistical downscaling. In this method, statistical relationships are searched between local HYRAS observations (Rauthe et al., 2013; Frick et al., 2014) in Germany and the large-scale atmospheric state in NCEP/NCAR reanalyses (Kalnay et al., 1996) in greater Central Europe. These relationships are then transferred to the simulated MPI-ESM-LR large-scale predictions (Kreienkamp et al., 2018):
The first step includes the detection of analog days, selecting those 35 days of the reanalysis that are most similar to a certain model day (“perfect prognosis” approach, e.g., Klein et al., 1959; San-Martín et al., 2017). This selection is based on temperature, relative humidity and geopotential height fields at different vertical levels (500, 700, 850, and 1,000 hPa) interpolated to a reduced grid of 100 km resolution. For the selected 35 analog days, linear regressions are derived between the large-scale quantities and the small-scale observations (e.g., of near surface temperature or precipitation), and then applied to the value of the respective large-scale predictor from the global prediction. This first interim prediction for each day is, however, inconsistent for the downscaled variables and grid points of the reduced 100 km grid. In the second step, the short-term precipitation and temperature variation of the interim prediction is compared to the short-term variation of all days in the observational archive, and the most similar day is selected consistently for all output variables and the entire output grid. The final synthetic time series at high resolution results from summing up this selected short-term variation and the daily climatology of observations of that day in year to be forecasted. Thus, this statistical downscaling approach provides multi-variable and multi-site consistent time series. The operationally downscaled decadal prediction dataset will be available on the ESGF node at DWD4 during 2022 or on request before.
Observations
The precipitation observations for evaluating the skill of high-resolution decadal predictions were taken from the HYRAS observations. They provide gridded daily precipitation data for Germany and corresponding river catchments in neighboring countries at 5 km spatial resolution. The gridded fields were derived from up to 6,200 precipitation stations applying the REGNIE procedure. This method combines inverse distance weighting and multiple linear regression including orographical conditions and thus, preserves the station values in their grid boxes (Rauthe et al., 2013). The time period was extended to 1951–2020 for precipitation. The dataset also includes daily grids for mean, minimum and maximum temperature and relative humidity (Razafimaharo et al., 2020). The HYRAS precipitation dataset is available via the open data section of the DWD Climate Data Center (CDC)5.
Hydrological Seasons and Wupper Catchment
The German water management sector is interested in decadal predictions of annual means and all four hydrological seasons for forecast years 1–3. Thus, the yearly averages of January–December, February–April (FMA), May–July (MJJ), August–October (ASO) and November–January (NDJ) were calculated for EPISODES and HYRAS precipitation data. Additionally, model and observational data were interpolated to a common regular 0.1° horizontal grid. The considered case study is located in the Wupper catchment in the German federal state North Rhine-Westphalia in western Germany. The location of this catchment in shown in Figure 1 and marked in each plot of the results chapter. In addition, all results are also shown for whole Germany (as a second focus) to fulfill similar needs of other German water managers gathered on a user workshop (see Section User Co-production and User Feedback on the Usability of the Product Sheet).
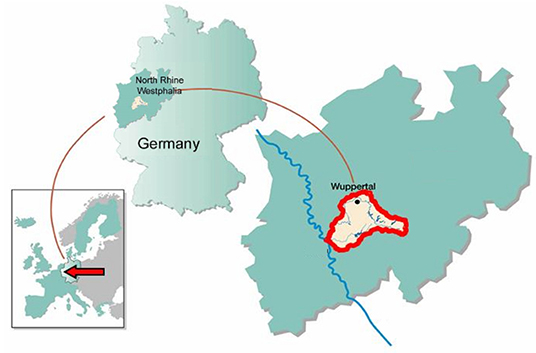
Figure 1. Location of the Wupper catchment in Europe, in western Germany and in the German federal state North Rhine-Westphalia (received from Wupper catchment water board and published as part of the product sheet on https://climate.copernicus.eu/decadal-predictions-infrastructure, modified).
Recalibration
The statistical downscaling EPISODES was developed for climate projections and aims at selecting the large-scale input variables with strongest relationships to the local target variables to provide high-resolution data consistent for different variables in space and revealing hardly any systematic bias. However, EPISODES does not consider to choose the large-scale variables with highest skill in reproducing the observed variability in the past. Thus, skill is preserved at high resolution but hardly enhanced (Ostermöller et al., 2021).
However, decadal prediction skill can be improved by post-processing techniques addressing systematic model errors, model drifts, trends and ensemble spread, like the Decadal Forecast Recalibration Strategy (DeFoReSt, Pasternack et al., 2018). This procedure uses a parametric drift correction (Kruschke et al., 2015) which applies third order polynomial parameters to correct the model drift over forecast years (Gangstø et al., 2013). A linear trend along start years is used to consider non-stationary drifts (Kharin et al., 2012). The conditional bias and the ensemble spread are adjusted by a third order and second order polynomial approach over forecast years and a linear approach over start years. The training of the recalibration parameters of a certain decadal prediction of 10 years is performed in cross validation mode omitting those decadal predictions as training data which were initialized within this prediction period. The adjustment of bias, drift, conditional bias and ensemble spread was shown to improve the skill (Pasternack et al., 2018). A more flexible improvement of DeForReSt applies a systematic model selection via non-homogeneous boosting to asses model orders directly from the dataset without restricting them before as well as an additive term for the ensemble spread correction (Pasternack et al., 2021). This boosted recalibration procedure was used in this study in two different settings: the first version follows the maximum orders of DeFoReSt (denoted as “standard recalibration version”) and the second version applies a third order polynomial as maximum order along start years to correct the ensemble mean (denoted as “optimized recalibration version”) to consider a higher interannual variability of high-resolution precipitation. In both settings, the boosting selects the best model orders of the fits only restricted by the maximum orders defined.
The annual precipitation means of January-December and the four hydrological seasons of EPISODES were recalibrated separately. All hindcast years were adjusted, whereas the training period was set to 1961–2020 (1961–2019 for NDJ) following the availability of HYRAS observations serving for both recalibration and skill assessment. This “unfair” procedure (Risbey et al., 2021) uses future data for recalibration of hindcasts in the past, which are not available for operational predictions and might include artificial skill. Thus, we performed a test of a “fair” recalibration applying only the preceding 30 years for the correction of a decadal prediction of a certain start date. The evaluation period was shortened to 1991–2020 since 1991 was the first start date of a decadal prediction to be recalibrated by 30 years from the past. However, we found major skill patterns to be robust between both approaches (not shown). Since long time periods are needed to achieve robust results when skill and recalibration vary in time, we decided to apply the original recalibration approach with cross validation (as described above).
For annual means and three of four hydrological seasons, the first year 1961 showed extremely dry conditions after optimized recalibration, identifying a clear outlier compared to the residual time series. Thus, this single year has been omitted in further SPI processing steps. Since the recalibration might destroy the standardization of the SPI (Paxian et al., 2019) it is executed before the SPI is calculated in this study [see Section Standardized Precipitation Index (SPI)]. This study applies the recalibration software tool (Pasternack et al., 2021) of the “Free Evaluation System Framework for Earth System Modeling” (FREVA, Kadow et al., 2021). Information on code availability can be found in these cited articles.
Standardized Precipitation Index (SPI)
The drought index SPI divides the anomaly of precipitation by its standard deviation (McKee et al., 1993). The parameter estimation for standardizing precipitation uses the Gamma distribution function. The resulting SPI values can be interpreted as follows (Lloyd-Hughes and Saunders, 2002): normal water availability is defined for values between −1 and 1, lower values describe dry and higher values wet conditions. The SPI calculation of this study uses the caeli package6 of the WARSA working group at the Institute of Technology and Resources Management in the Tropics and Subtropics of the Cologne University of Applied Sciences in Germany because it is used by the Wupper catchment water board for their routine work. This assures that the resulting SPI values are comparable to their former results.
To provide proper standardized prediction products, the SPI needs to be calculated for each dataset (EPISODES ensemble members and HYRAS for different temporal aggregations) separately. The data needs to be aggregated, including temporal smoothing, spatial interpolation and ensemble averaging, before calculating the SPI. Thus, the yearly precipitation means of January-December and the four hydrological seasons of the recalibrated EPISODES hindcast set and the HYRAS observations were averaged for forecast years 1–3. The EPISODES ensemble mean was computed to calculate the SPI for EPISODES separately for the individual ensemble members and the ensemble mean. Thus, both probabilistic and ensemble mean SPI predictions can be analyzed. Since the SPI cannot be calculated for negative precipitation means, those time steps and grid boxes revealing negative means after statistical recalibration were set to zero.
The chosen time period for the standardization of HYRAS data per grid box is the evaluation period 1962–2020 (1962–2019 for NDJ), including all 3-year means within this period. The EPISODES model period is the same one but extended by the current 3-year mean forecast, i.e., 2021–2023. This is done because the chosen SPI algorithm of the WARSA working group does not allow to use a different time period for parameter estimation (needs to be equal for observations and model) and application of parameters for computing standardized time series (needs to include the current 3-year mean forecast). Thus, the period of standardization for EPISODES is only one 3-year mean longer (denoting the current 3-year mean forecast 2021–2023) than that for HYRAS, which is a marginal difference given the considered total period of almost 60 3-year means. This standardization can also be classified as “unfair” (Risbey et al., 2021) because it applies future data for standardizing past hindcasts which might produce artificial skill (see Section Recalibration). Since the caeli package does not allow computing the SPI based on a subset of the input data, we could not test the impact of this “unfair” approach on the skill. However, this algorithm is essential for this climate service being part of the routine working environment of the Wupper catchment water board. Thus, skill results need to be interpreted with caution.
Skill Assessment
The quality of the EPISODES predictions is assessed in comparing those initialized in the past with HYRAS observations in the evaluation period 1962–2020 (1962–2019 for NDJ). The skill of the ensemble mean is evaluated by means of the Pearson (or anomaly) correlation coefficient, and the probabilistic prediction skill of the full ensemble is estimated by the Ranked Probability Skill Score (RPSS) compared to the observed climatology in the evaluation period (describing a distribution of equal weights for all categories) chosen as reference prediction.
The strength of the linear relationship between the ensemble mean prediction (X) for the selected forecast years initialized in the past and the corresponding observation (Y) along all hindcast start years (N) is assessed by the Pearson (or anomaly) correlation coefficient (rxy). Predictions and observations are considered as anomalies with respect to the corresponding long-term climatological mean (μ). A correlation coefficient of zero indicates no correlation between prediction and observation, whereas values of 1 and −1 define a high positive and negative correlation, respectively (see e.g., Ernste, 2011):
The RPSS compares the probabilistic skill of a decadal prediction in reproducing the past observed variability with the skill of a reference prediction which can be used alternatively, e.g., the observed climatology in the evaluation period (defining a distribution of equal weights for all categories). Anomalies of predictions initialized in the past and observations with respect to a long-term climatology are grouped in the three categories of equal frequency “below normal”, “normal,” and “above normal.” The limits are based on the 33rd and 66th tercile values of the predicted and observed climate characteristics of a reference period (see Section Calculation and Display of Probabilistic Predictions). Computing this separately for model and observations results in an inherent bias correction. The squared error between the cumulative probabilities of predictions Pj, k and observations Oj, k for n start years and K categories (here: three) is defined as ranked probability score (RPSP, O). The predicted probability of each category is assessed empirically (as described in Siegert, 2014), following the frequency of individual ensemble members per category. The observed probability of a category is zero if the observed value is located in a higher category than selected and one if not. The RPSS relates the RPSP, O between predictions and observations to the RPSR, O between the alternative reference prediction and observations (Ferro et al., 2008; Wilks, 2011; Kruschke et al., 2014):
If the decadal prediction is better than the reference prediction in reproducing past observations, the RPSS is larger than zero, if worse than smaller. If they perform equally well, it is zero. If the decadal prediction is in perfect agreement with the past observations, it is one.
However, the RPS is biased due to the finite prediction ensemble size. Thus, the ensemble-size adjusted FairRPS (Ferro, 2013; Richling et al., 2017) is estimated assuming the ensemble size grows to infinity to be able to compare predictions of different ensemble sizes. For the ensemble size M and the cumulative number of members of the prediction ensemble E corresponding to a certain category k, the FairRPSt of one forecast-event pair t can be defined as follows. The FairRPSS can be estimated based on the FairRPSP, O and the FairRPSR, O following the equation before:
For the (anomaly) correlation coefficient, RPSS and FairRPSS, the significance is tested to analyze if small sample sizes cause random variations influencing the skill assessment. Since the distribution of the RPSS is not known, the test applies non-parametric bootstrapping choosing randomly 1,000 samples of equal sizes from the given time period with replacement. A block bootstrapping allows for autocorrelation in decadal predictions. The random samples are analyzed using a significance level of 95%. If correlation coefficient, RPSS or FairRPSS are significantly different from zero, the skill analysis has not been impacted by random variations. Please note that such assessments of significance are subject to issues with multiple testing and should include control of the False Discovery Rate (Wilks, 2016). However, for more than 5,000 grid boxes in Germany and a significance level of 90% we would need ~50,000 non-parametric bootstraps for this approach which is not possible due to restrictions in computing time and sample size (~60 start years). Some tests revealed that 1,000 bootstraps clearly preserve the robust overall spatial structure of significance, but some small details might vary slightly (not shown). Thus, we highlight that one should act with caution to not over-interpret the significance of skill of single grid boxes. Only regional clusters of significant grid boxes are considered to be robust.
The correlation coefficients and (Fair)RPSS of this study were assessed based on the FREVA (Kadow et al., 2021) software tools MurCSS (Murphy-Epstein decomposition and Continuous Ranked Probability Skill Score, Illing et al., 2014) and ProblEMS (PROBabiListic Ensemble verification for MiKlip using SpecsVerification, Richling et al., 2017, based on routines from Siegert, 2014), respectively. Code is available as described in these cited articles or on request.
Calculation and Display of Probabilistic Predictions
Based on the distribution of the decadal prediction ensemble probabilistic SPI predictions are calculated. The 16 individual ensemble predictions are divided into the three categories of equal frequency “dry”, “normal,” and “wet,” defined by low, medium and high SPI values. The groups are split by the 33rd and 66th tercile thresholds of the predicted climate characteristics of the WMO reference period 1981–2010. Finally, the predicted probability of occurrence [%] of each category is based on the frequency of ensemble members per category. However, since the number of ensemble members (16) is still restricted the estimated probability is adjusted considering the uncertainty of small sample sizes (Dirichlet-Multinomial Model, Agresti and Hitchcock, 2005). An inherent bias correction is included when the probabilistic model prediction is shown in conjunction with the tercile thresholds from observations in the reference period. The probabilistic predictions were estimated based on FREVA (Kadow et al., 2021) software tools. Original code can be accessed as pointed out in this article, and code adapted for this case study can be provided on request.
Following user needs collected on user workshops and individual user meetings prediction products should be displayed combing the prediction and its skill. Thus, the map of the final prediction product includes one dot per EPISODES grid box whose color describes the probabilistic prediction and whose size indicates the prediction skill, i.e., the RPSS compared to the reference prediction “observed climatology.” Thus, a decadal prediction with a better, similar or worse skill than applying the observed climatology as prediction is represented by a dot of large, medium or small size. Many users are interested in predictions of all three levels of skill to compare the whole prediction map with real observations and understand the concept of skill. Impact modelers need the whole dataset to drive e.g., hydrological models. Both prediction and skill of the hydrological output can be compared to those of the atmospheric input to understand the connection between different variables.
Results
In this chapter the impacts of statistical downscaling and recalibration on decadal prediction skill are analyzed, presenting (anomaly) correlation coefficients and the RPSS to investigate the skill of ensemble mean and probabilistic decadal predictions, respectively. Furthermore, we show the SPI prediction skill of annual means and all four hydrological seasons for forecast years 1–3 and the probabilistic prediction for 2021–2023. All results are presented for whole Germany first and then, the focus is set to the Wupper catchment area in western Germany marked in each plot. Please note that single grid boxes with significant skill should not be over-interpreted. Only regional clusters of significant grid boxes are considered to be robust (see Section Skill assessment). Finally, the product sheet of the case study published on the C3S website is presented, the user-cooperation is highlighted and the user feedback on its usability is evaluated.
Impacts of Statistical Downscaling and Recalibration
The decadal prediction skill for 3-year means of annual precipitation from MPI-ESM-LR is presented at ~200 km horizontal resolution in Germany. Some significantly positive correlation coefficients of 0.2–0.4 can be found in northern and eastern parts and negative correlation in south-western parts (Figure 2A). The statistical downscaling EPISODES succeeds in preserving the prevailing skill of the global decadal prediction system at higher horizontal resolution of ~11 km (Figure 2B). More local details can be seen and significantly positive correlation coefficients in several northern and eastern areas. The RPSS reveals rather similar patterns to the correlation. For MPI-ESM-LR, it is slightly negative in most regions, achieving statistical significance in the far south-western parts, except for a few positive values in some northern areas (Figure 2C). EPISODES again preserves the skill at higher resolution (Figure 2D), but more significantly negative RPSS values are stated in the southern parts. For the Wupper catchment, correlation and RPSS show slightly negative values for MPI-ESM-LR and EPISODES.
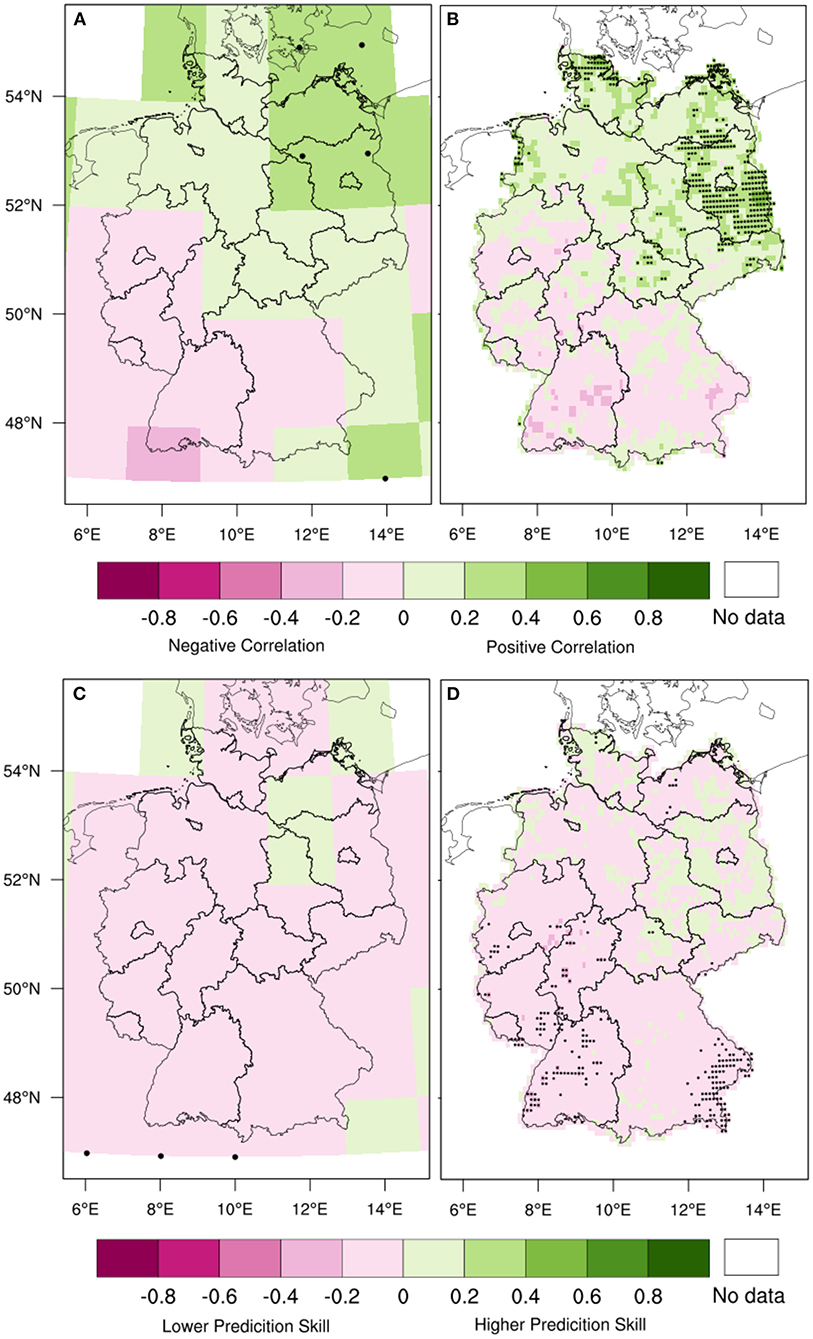
Figure 2. Decadal prediction skill for 3-year means of annual precipitation for forecast years 1–3 in the evaluation period 1961–2020: (Anomaly) correlation coefficient between MPI-ESM-LR at ~200 km (A) or EPISODES at ~11 km (B) and HYRAS observations and RPSS of MPI-ESM-LR (C) or EPISODES (D) compared to the observed HYRAS climatology as reference prediction. Dots indicate significant skill (significance level of 95%).
In applying the standard recalibration version with a linear trend along start years, the correlation coefficients of EPISODES clearly improve in some southern, south-western and far north-western areas (Figure 3A) compared to the unrecalibrated output (Figure 2B). However, some negative correlation remains in north-western parts and some significantly negative values in south-eastern parts. The usage of the optimized recalibration version applying a third order polynomial along start years is much more successful in adjusting the statistical properties of the high-resolution precipitation output to observations. It results in significantly positive correlation all over Germany, except for some small eastern areas (Figure 3B). In some regions correlations higher than 0.6 are calculated. Concerning the RPSS, the standard recalibration reveals some more significantly positive scores in the far northern parts but several more significantly negative scores in the western and south-eastern areas (Figure 3C) than the unrecalibrated model (Figure 2D). In contrast, the optimized recalibration version strongly improves RPSS values in north-western, western and southern Germany, achieving significantly positive scores in several regions (Figure 3D). In the Wupper catchment area, the standard recalibration degrades both scores but the optimized version results in strong improvements.
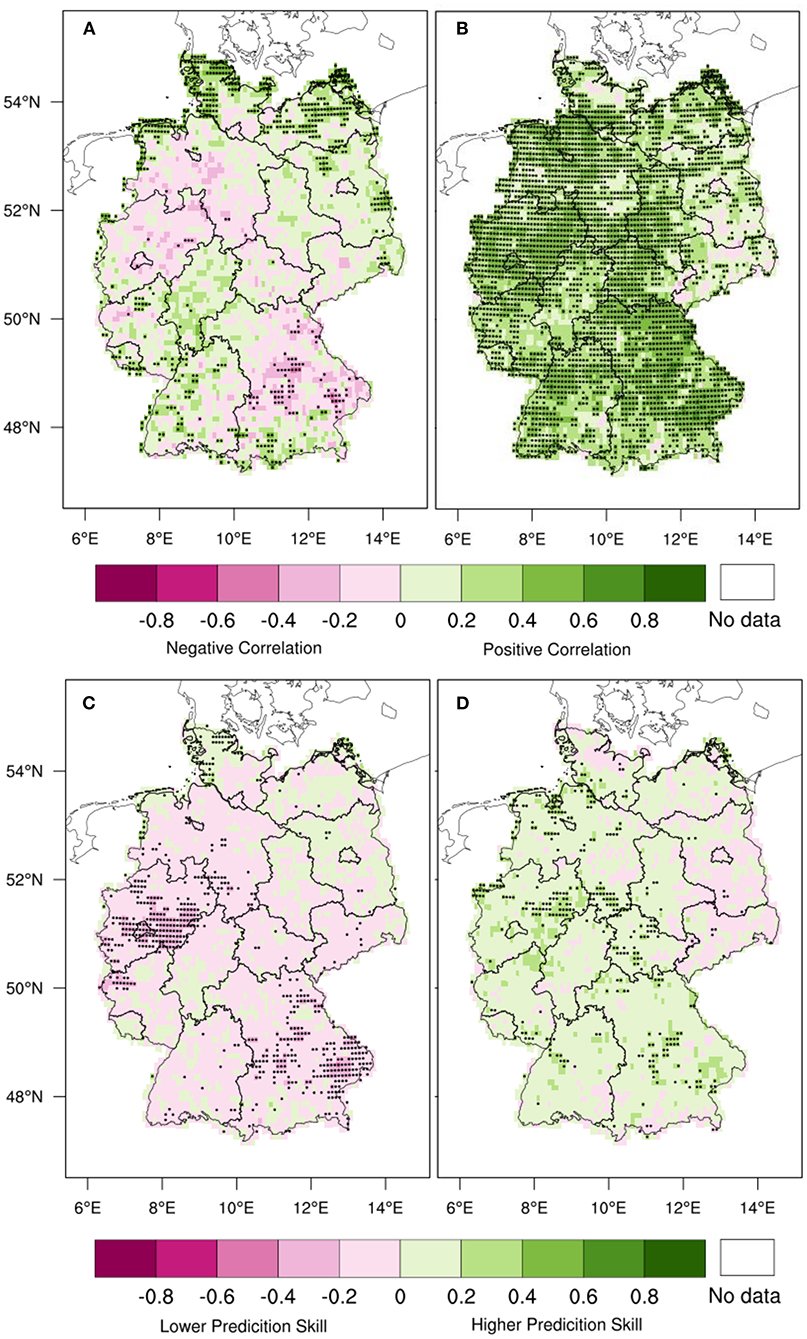
Figure 3. Decadal prediction skill for 3-year means of annual precipitation for forecast years 1–3 in the evaluation period 1961–2020: (Anomaly) correlation coefficient between EPISODES at standard (A) or optimized recalibration (B) and HYRAS observations and RPSS of EPISODES at standard (C) or optimized recalibration (D) compared to the observed HYRAS climatology as reference prediction. Dots indicate significant skill (significance level of 95%).
Some additional analyses showed that precipitation skill enhances from 1- to 3-up to 5-year means because small-scale unpredictable noise is reduced. Furthermore, skill remains rather constant from the beginning to the middle and then clearly drops until the end of the simulation period of the decadal prediction, denoting a clear lead-time dependency. The skill against the reference prediction “uninitialized climate projection,” i.e., the same model system but without initialization, reveals that the impact of the initialization clearly remains until the mid of the predicted decade (not shown).
This analysis of precipitation is done in the full evaluation period 1961–2020 to be consistent for MPI-ESM-LR, EPISODES and both recalibration versions. Thus, the improvement of the optimized recalibration is found even when the outlier (year 1961) is included. However, in all following SPI analyses this outlier is omitted because the calculation of SPI is not possible.
SPI Prediction Skill
After applying the optimized recalibration version to annual precipitation means and all four hydrological seasons the corresponding SPI values and prediction skills for forecast years 1–3 are computed. The correlation coefficients of 3-year SPI of annual means (Figure 4A) are rather similar to those of precipitation (Figure 3B) in southern Germany. However, in western, central and north-western Germany the correlation is clearly improved, showing widespread areas of values between 0.4 and 0.6 and several regions exceeding a correlation of 0.6. In eastern Germany, the area of negative values is also reduced. For RPSS, similar results can be stated. Enhanced positive scores in western Germany and less negative scores in eastern Germany are shown for SPI (Figure 5A) compared to precipitation (Figure 3D). This also holds for the Wupper catchment area.
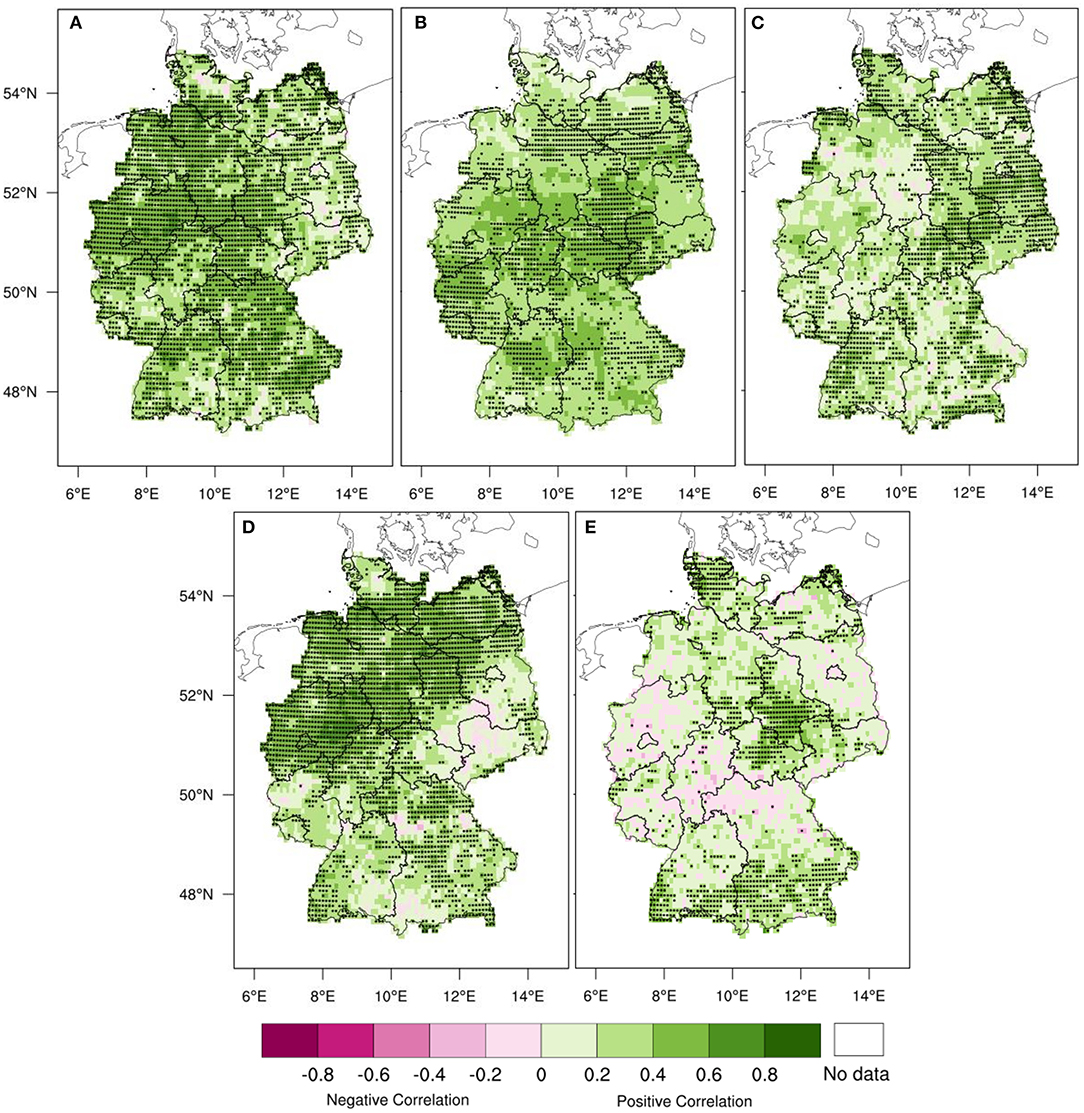
Figure 4. Decadal prediction skill for 3-year SPI of January–December (A), February–April (B), May–July (C), August–October (D) and November–January means (E) for forecast years 1–3 in the evaluation period 1962–2020 (1962–2019 for November–January): (Anomaly) correlation coefficient between EPISODES at optimized recalibration and HYRAS observations. Dots indicate significant skill (significance level of 95%).
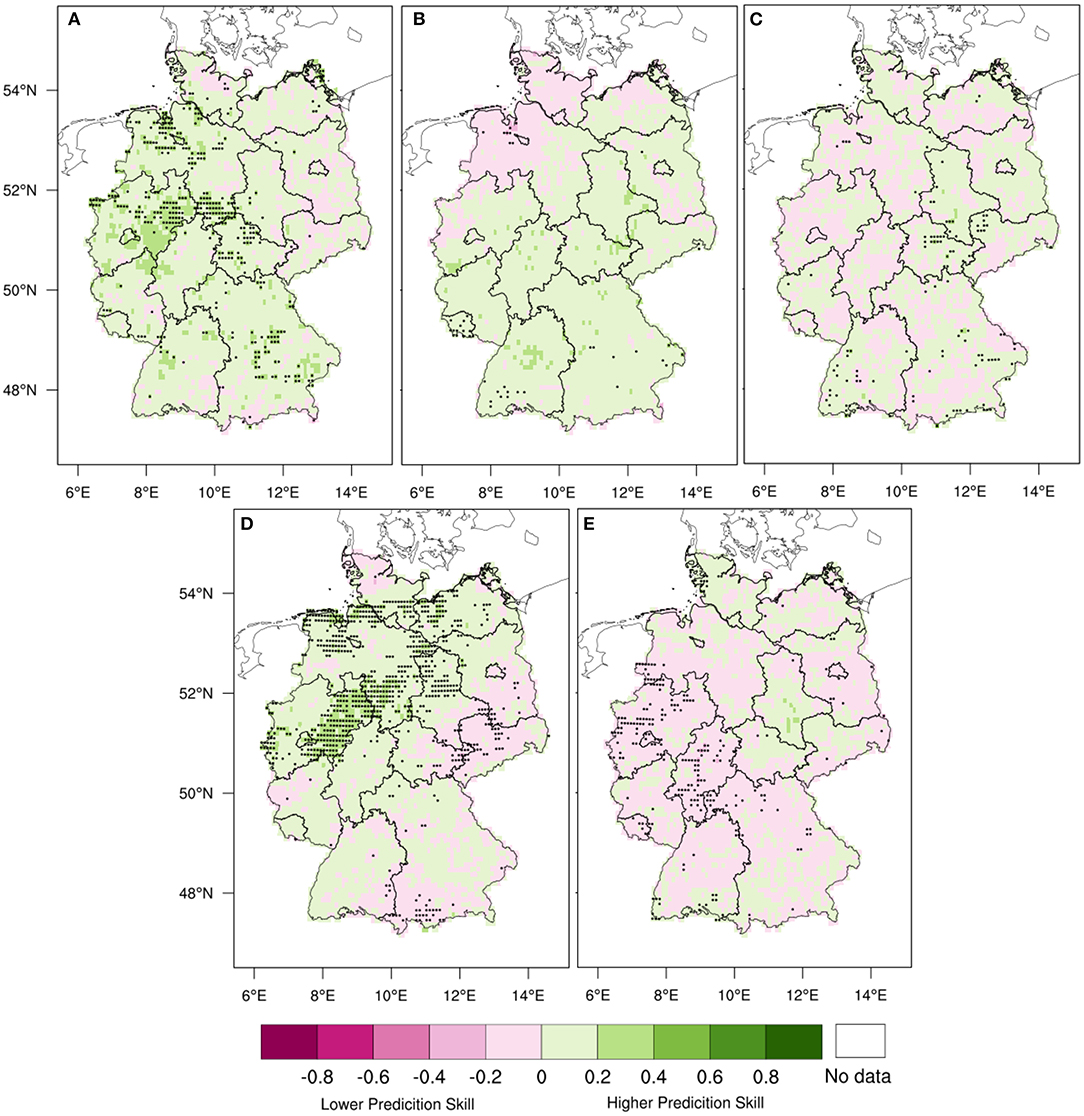
Figure 5. Decadal prediction skill for 3-year SPI of January–December (A), February–April (B), May–July (C), August–October (D) and November–January means (E) for forecast years 1–3 in the evaluation period 1962–2020 (1962–2019 for November–January): RPSS of EPISODES at optimized recalibration compared to the observed HYRAS climatology as reference prediction. Dots indicate significant skill (significance level of 95%).
Concerning the 3-year means of the four hydrological seasons, FMA reveals as well significantly positive correlation coefficients over most of Germany, achieving widespread maxima of 0.4–0.6 in western, central and southern parts and minima in the far north (Figure 4B). Significantly positive correlations in MMJ (Figure 4C) and NDJ (Figure 4E) are restricted to some smaller regions, i.e., mainly in central-eastern and southern Germany, whereas NDJ shows even negative correlations in some western parts. Highest correlations of all seasons are found for ASO (Figure 4D), revealing widespread values of 0.4–0.6 in northern and western Germany and maxima of 0.6–0.8 north-east of the Wupper catchment and at the coastline of the North Sea. Regarding the RPSS, FMA shows widespread positive scores, but significance is only found for single grid boxes in southern Germany which should not be over-interpreted (Figure 5B). MJJ also reveals only some significantly positive RPSS values in southern and central-eastern parts which might also be not robust (see Section Skill assessment) and even more negative scores (Figure 5C). As expected, worst skill results are found for NDJ with widespread negative scores and some significant ones in western parts (Figure 5E). Again, highest and widespread significantly positive scores are found for ASO in northern and western Germany (Figure 5D). However, significantly negative scores are seen in some eastern areas. The Wupper catchment area shows significantly positive correlations in ASO and partly in FMA. The RPSS is significantly positive as well in ASO but might be significantly negative in NDJ.
The FairRPSS can indicate which potential RPSS values could be achieved if the ensemble size grows further, e.g., in considering a larger multi-model ensemble. For the prevailing study, this was not possible since other decadal prediction systems did not provide necessary daily input data for statistical downscaling with EPISODES, but this might change in the future. For all temporal aggregations, the FairRPSS (Figure 6) shows more significantly positive scores and less significantly negative ones than the RPSS of the 16-member ensemble (Figure 5). Thus, a high potential for skill improvements due to a possible future enlargement of the ensemble size is found. For 3-year SPI of annual means, widespread skill over many German regions is found, except in eastern areas (Figure 6A). In FMA (Figure 6B) and MJJ (Figure 6C), significant skill is mainly discovered in southern and eastern parts, whereas ASO reveals widespread significantly positive scores in western and northern regions (Figure 6D). However, skill in NDJ remains limited to some small areas in the far north and south-west, even for larger ensemble sizes (Figure 6E). In the Wupper catchment area, significantly positive FairRPSS scores are computed for annual means, ASO and partly also for FMA (whereas the latter should not be over-interpreted).
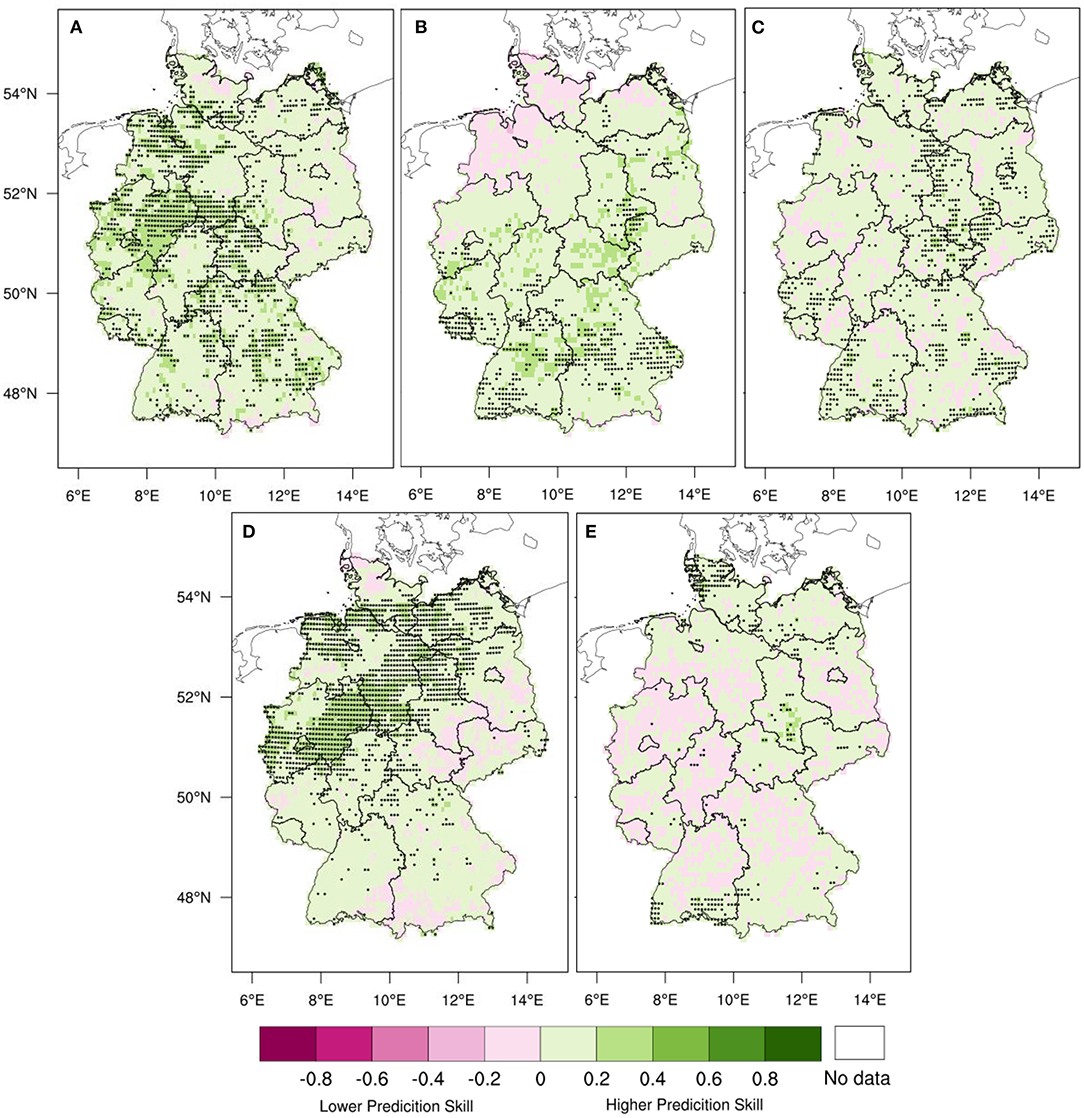
Figure 6. Decadal prediction skill for 3-year SPI of January–December (A), February–April (B), May–July (C), August–October (D) and November–January means (E) for forecast years 1–3 in the evaluation period 1962–2020 (1962–2019 for November–January): FairRPSS of EPISODES at optimized recalibration compared to the observed HYRAS climatology as reference prediction. Dots indicate significant skill (significance level of 95%).
Probabilistic SPI Prediction for 2021–2023
Following user needs the probabilistic SPI prediction for 2021–2023 (Figure 7), i.e., forecast years 1–3 initialized in November of 2020, is shown in combination with the corresponding RPSS prediction skill compared to the reference prediction “observed climatology” (cf., Figure 5). The color of the dots indicates the probabilistic prediction, and the size of the dots signifies the prediction skill. The 3-year SPI of annual means (Figure 7A) shows high probabilities of occurrence (larger than 85%) for dry conditions (negative SPI) in comparison to the characteristics of 1981–2010 in most of the area. The prediction skill is better than applying the observed climatology in several parts of north-western, central and south-eastern Germany (large dots). Again, caution needs to be taken to not over-interpret single grid boxes. The skill and the probability for dry conditions are smaller in the eastern areas and in the far south. In the far north some probability for wet conditions prevails. Dry conditions are predicted for the whole Wupper catchment area, and there might be some significantly positive skill in its eastern parts.
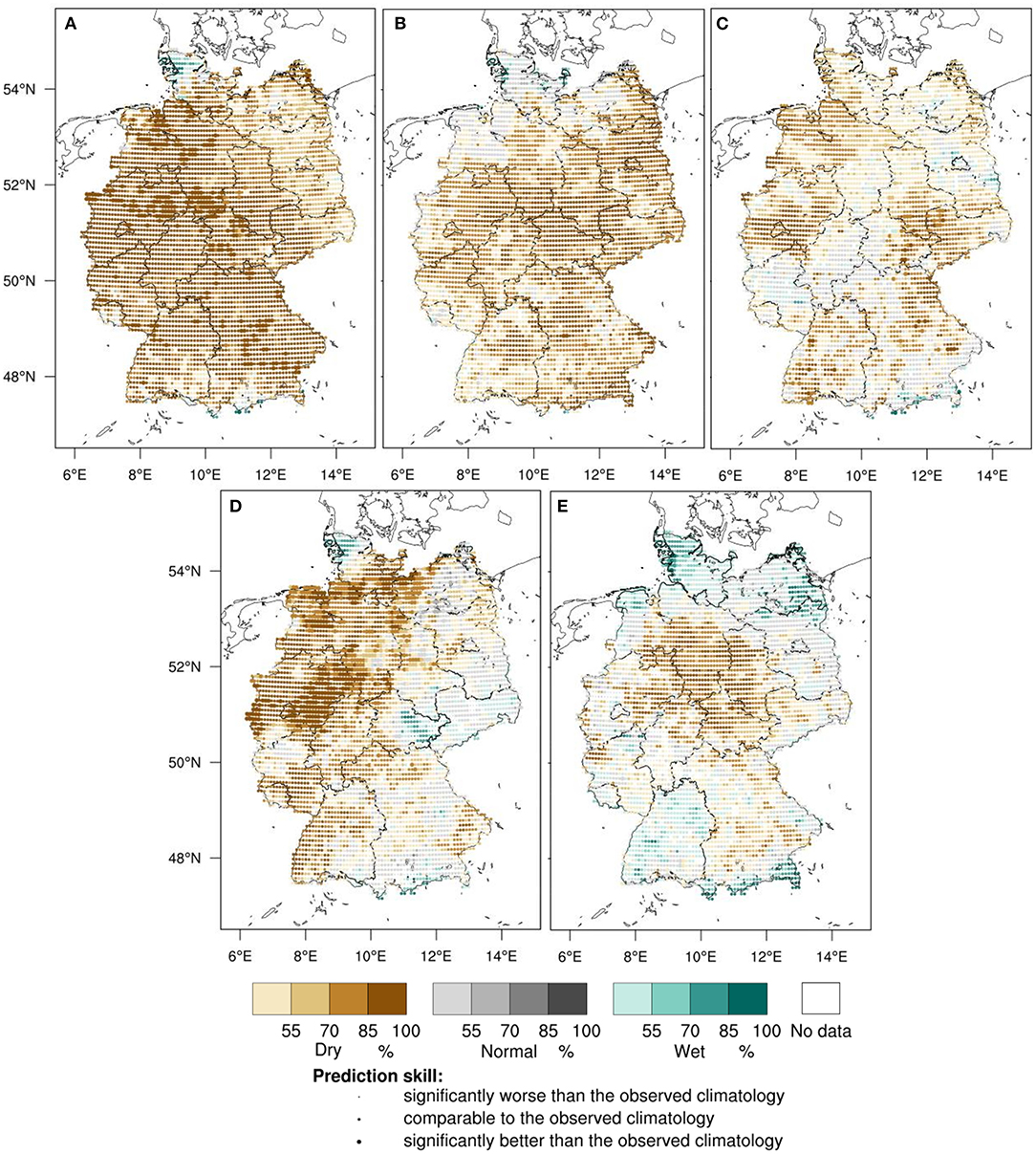
Figure 7. Decadal probabilistic prediction for 3-year SPI of January–December (A), February–April (B), May–July (C), August–October (D) and November–January means (E) for forecast years 1–3: The color represents the most probable category (dry/normal/wet) in comparison to the climate characteristics for 1981–2010. The brightness describes the predicted probability of occurrence of this category. The size of the dots shows the RPSS prediction skill in the evaluation period 1962–2020 (cf., Figure 5).
A widespread drying for most of Germany is also predicted for FMA, but the probability of occurrence is often smaller, and skill is rarely better than the observed climatology (Figure 7B). Normal conditions are forecasted for the northern parts. In MJJ, the prediction of dry conditions focusses on the north-western, eastern and south-western areas, whereas normal conditions prevail in between (Figure 7C). Skill is found in some central-eastern and southern regions but needs to be interpreted with caution. A stronger drying is again predicted for the whole western part of Germany in ASO with widespread significant skill in north-western areas (Figure 7D). The eastern and the far northern parts show normal or wet conditions but some negative skill scores prevail in the eastern areas (small dots). Finally, the predicted dry conditions are mostly limited to central Germany in NDJ (Figure 7E). Wet conditions are forecasted in the northern and the south-western parts and normal values in between. However, the prediction skill is worse than the observed climatology in some western regions. For the Wupper catchment area, dry conditions are predicted in FMA, MJJ and especially in ASO and rather mixed conditions in NDJ, though, robust skill is only found in ASO.
Product Sheet of the Case Study
The 3-year SPI of annual means was selected for the C3S product sheet (Figure 8) showing widespread high prediction skill. To comply with the limited space given in the product sheet and keep the readability of the user-oriented combined prediction and skill plot, the spatial focus of the product sheet was set on north-western Germany (50.5–53.5°N, 6.5–11°E). This area reveals highest and most significant prediction skill, includes the Wupper catchment area and further addresses similar needs of neighboring water managers as stated on a C3S_34c showcasing event (see Section User Co-production and User Feedback on the Usability of the Product Sheet). The format of the product sheet was developed in cooperation with the scientists of all four C3S_34c case studies considering intensive user feedback (see Section User Co-production and User Feedback on the Usability of the Product Sheet). The first page includes a short description of the goal of the case study and the main prediction message within a prominent red box. Below that main information the combined prediction and skill plot and a corresponding text describe the probabilistic prediction in more detail. Further background information on the needs of the Wupper catchment water board and the data and methods used to compute the prediction is given on the second page. It also includes the RPSS prediction skill, applied to define the dot sizes of the combined prediction and skill plot, and the correlation coefficients. However, please note that the C3S product sheet was published in 2021 (see below) based on 500 bootstraps, whereas the figures of this manuscript use 1,000 bootstraps which might lead to slight differences in the significance of skill.
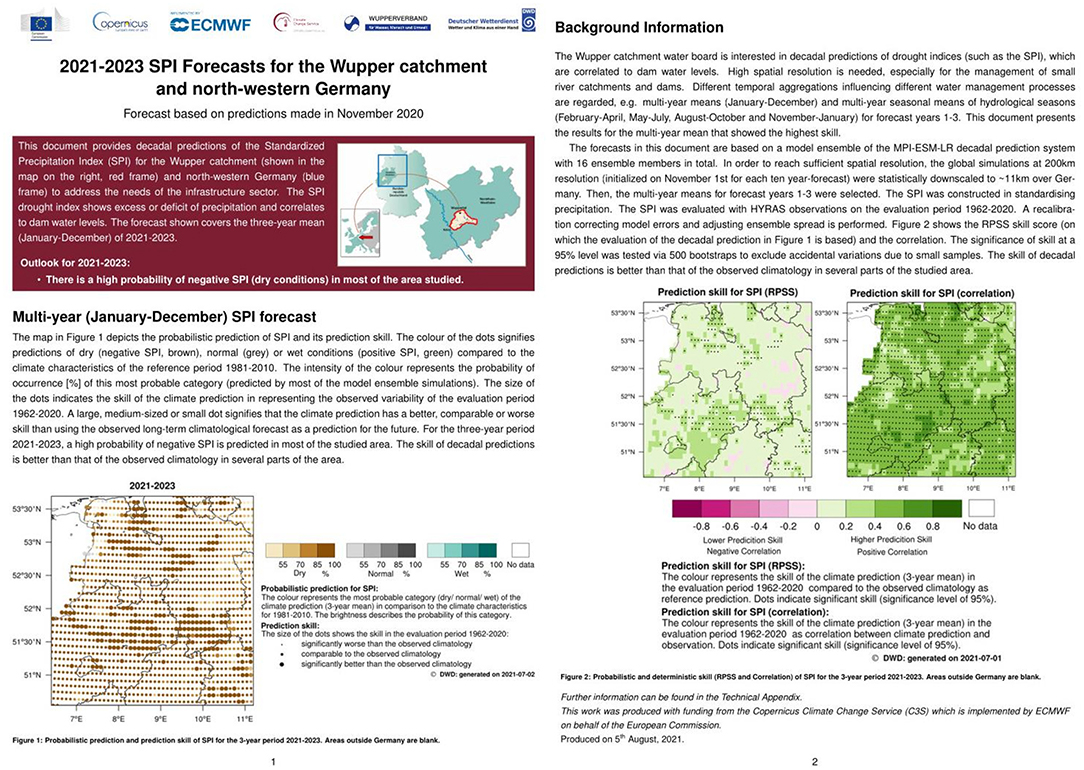
Figure 8. C3S product sheet on decadal predictions for infrastructure: 2021–2023 SPI forecasts for the Wupper catchment and north-western Germany, started in November 2020 (published on https://climate.copernicus.eu/decadal-predictions-infrastructure).
The product sheet was published in the Section “Decadal predictions for infrastructure” (see text footnote 3) on the C3S website on “Sectoral applications of decadal predictions” together with the three other C3S_34c case studies on agriculture, energy and insurance. This website is at a pre-operational state, offering the predictions initialized in November 2019 and 2020. This manuscript describes the case study initialized in 2020 only because that initialized in 2019 covers a shorter evaluation time period, a smaller area (only the Wupper catchment) and a different drought index (the SPEI) based on the old DWD prediction system and is less robust (see Section Conclusions). Further detailed information on model and observational data, post-processing and evaluation methods and the analysis protocol for all four case studies was published in a common technical appendix7.
User Co-production and User Feedback on the Usability of the Product Sheet
The 3-year SPI product sheet and corresponding analyses of hydrological seasons were generated in close co-production with the Wupper catchment water board. They computed the SPI following their usual workflow based on the downscaled and recalibrated decadal predictions of DWD. After standardization, the skill analysis, computation of the probabilistic prediction and design of the product sheet was done by DWD. The development of the climate service included several feedback loops, but the close co-development guarantees that the resulting climate service matches the needs of the Wupper catchment water board in terms of content and format to be applied in their working routine. They consider the skill of the high-resolution SPI predictions for annual means and ASO to be promising and would be interested in receiving similar skillful products for the other seasons (to cover the whole annual water management cycle: water storage in winter with regard to flood protection and water usage in dryer seasons) and the SPEI as well. The latter describes also the possible losses from the water surface of greater reservoirs than the SPI. Thus, it correlates better to water levels of dams of bigger sizes (Lorza-Villegas et al., 2021), and would further improve the applicability of this climate service. Analyses of SPEI predictions were also performed in co-production and were part of the product sheet initialized in November 2019. However, they proved to be less robust and skillful after a model update for the predictions initialized in 2020 (see Section Conclusions). Nevertheless, first user needs of the Wupper catchment water board could be clearly fulfilled.
In addition, a common C3S_34c event was organized to showcase the four case studies on sectoral decadal predictions. Several German water managers were present to discuss the developed product sheet (the old version initialized in November 2019). They found it well-structured and understandable and could use it in their work. It shouldn't include any technical terms but the technical appendix could do so. The combined plot of prediction and skill is very interesting but probably needs some further explanation, e.g., more information on the thresholds of the categories of the probabilistic prediction (see below). Overall, the product sheet is important for water managers in communicating the probabilistic prediction and its skill. In addition, hydrological modelers would be interested in information on further atmospheric variables relevant for impact modeling for different German regions or temporal aggregations and would also need the downscaled data for modeling. An operational product sheet could be accessible via the C3S website or even sent per e-mail.
Some feedback could be considered already within the project and some is part of the outlook (see Section Outlook): we modified the product sheet with respect to technical terms and explanations of the combined plot and enlarged the study area of the (old) product sheet from the Wupper catchment area to whole north-western Germany (as shown in this paper) to fulfill similar needs of neighboring water managers. A map of the 33 and 66% terciles of observations in the reference period 1981–2010, defining the thresholds between low and normal SPI as well as normal and high SPI, respectively, was added to the technical appendix (Figure 9). In the north-eastern and south-eastern part of the study area the lower SPI threshold is below zero and the upper threshold above zero as expected. However, in the north-western, south-western and central parts both thresholds are above zero, indicating that the reference period reveals higher SPI values, i.e., wetter conditions, than the long-term evaluation period 1962–2020 chosen for standardization. This is especially true for the Wupper catchment area. Thus, the probabilistic SPI prediction for 2021–2023 in the product sheet showing widespread drying conditions for north-western Germany needs to be interpreted in the context that the reference period was clearly wetter than the long-term standardization period in several parts of the study area.
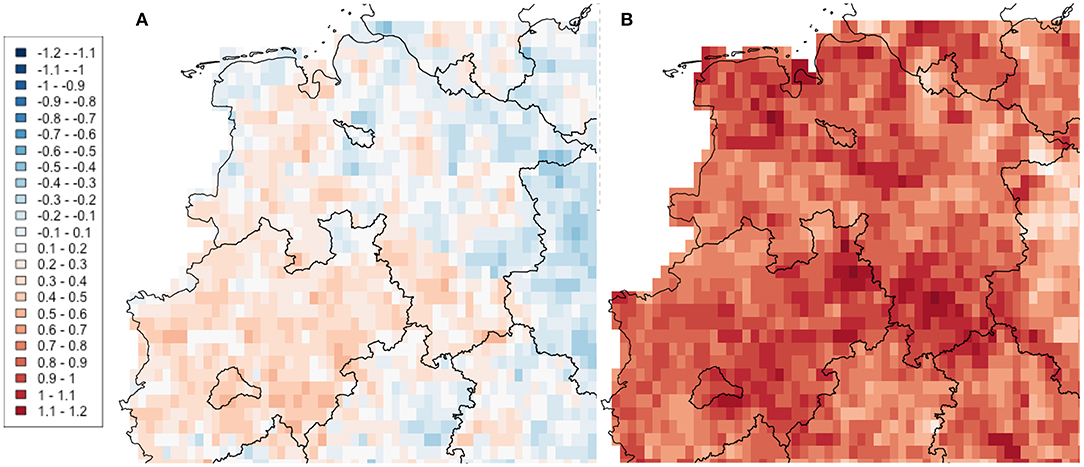
Figure 9. Thresholds between the three categories of the decadal probabilistic prediction for 3-year SPI of January–December for forecast years 1–3, based on the 33% (A) and the 66% (B) terciles of observations in the reference period 1981–2010 (published on https://climate.copernicus.eu/sites/default/files/2021-09/Technical_appendix_2020.pdf, modified).
Discussion
This final section provides a summary of the key findings of this study, discusses the major conclusions drawn from the results and gives a final outlook for future research.
Summary
In this study we present user-oriented high-resolution decadal drought predictions for German water boards, with focus on the Wupper catchment. To reach the desired horizontal resolution of ~11 km the global decadal climate predictions of MPI-ESM-LR are statistically downscaled by EPISODES. This procedure succeeds in preserving the prevailing MPI-ESM-LR prediction skill at higher resolution. The skill assessment is performed applying (anomaly) correlation coefficients and the RPSS against the reference prediction “observed climatology.” For the downscaled predictions, the standard recalibration version with a linear trend along start years does not produce the expected skill improvements as achieved with global predictions. However, an optimized recalibration version using a third order polynomial along start years is able to clearly enhance correlation and RPSS in many German regions. After standardizing precipitation predictions, the SPI drought index reveals similar or higher skill than unstandardized precipitation.
The 3-year SPI of annual means for forecast years 1–3 shows widespread positive RPSS skill in many parts of Germany, achieving significance in several north-western, central and south-eastern regions. Concerning 3-year means of hydrological seasons, the skill of ASO predictions is significantly positive in many northern and western areas. However, the positive skill in FMA and MJJ achieves significance only in some limited areas (which should not be over-interpreted), whereas significantly negative skill is found in western Germany in NDJ. The FairRPSS shows higher scores, thus indicating that there is a clear potential of further skill improvement with increasing ensemble size. Widespread significantly positive scores are found in all temporal aggregations, except for NDJ where skill remains limited to some smaller areas.
A user-oriented plot combining prediction and skill is applied for the probabilistic SPI prediction for 2021–2023, initialized in November 2020. The 3-year SPI of annual means results in dry conditions compared to 1981–2010 in most of Germany. The predicted drying is similarly widespread in FMA but less extensive in MJJ and ASO, leaving some smaller areas for which wet and normal conditions are forecasted. In NDJ, the prediction of dry conditions is limited to central Germany, and wet conditions are computed for the northern and south-western parts. However, significantly positive skill is mainly found for annual means and ASO. The Wupper catchment area is a typical example in western Germany: Mixed conditions are predicted in NDJ and dry conditions in all other temporal aggregations, but skill might only be prominent for ASO and partly for annual means (which should be interpreted with caution).
A 2-page product sheet was designed including the main message, information on the probabilistic prediction and background information on data and methods used and resulting skills. The 3-year SPI of annual means was selected showing widespread high skill. Due to the limited space of the product sheet, the study area is focused on north-western Germany including the Wupper catchment area (instead of showing whole Germany). The product sheet based on predictions initialized in November 2020 is published on the C3S website (see text footnote 3), together with three other sectoral case studies. Detailed additional information on data and methods can be found in the C3S technical appendix (see text footnote 7).
Co-production of the product sheet with the Wupper catchment water board guarantees its usability. Their feedback and that of further German water managers was gathered at a C3S_34c showcasing event. They stated that the product sheet can be used in their work and considered the skill to be promising. Further product sheets and data for hydrological modeling would be useful. Following their needs, the study area in the product sheet was enlarged from the Wupper catchment area (old version) to north-western Germany, and a map of the observed tercile-based thresholds of the categories of the probabilistic prediction was included in the technical appendix. It shows that the reference period 1981–2010 was wetter than the long-term SPI standardization period in several regions. This needs to be considered in interpreting the widespread drying in north-western Germany in the product sheet.
Conclusions
(1) High spatial resolution of decadal predictions is needed by many users, especially water managers of small river catchments, such as the Wupper catchment water board. We find that the cost-efficient empirical-statistical downscaling procedure EPISODES is able to preserve the skill of the global prediction system at higher resolution of ~11 km. This observed conservation of skill at higher resolution confirms also former findings of applying EPISODES to seasonal predictions of hydropower production in Germany and Portugal (Ostermöller et al., 2021).
(2) The optimized recalibration version applying third order polynomial parameters along start years can adjust high-resolution EPISODES precipitation to observed statistics and clearly improve correlation and RPSS in most of Germany. The standard recalibration version using a linear trend along start years (cf., Pasternack et al., 2018, 2021) is sufficient for global predictions at coarser resolution, e.g., for global drought indices at 5° or 2° resolution (Paxian et al., 2019). However, the variability of high-resolution precipitation in Germany makes the use of higher order polynomials, e.g., of the third order, necessary. This study reveals that statistical approaches can improve dynamical models which has also been shown by Sahastrabuddha and Ghosh (2021) applying multi-variate singular spectrum analysis, a computationally inexpensive data-driven model addressing oscillations and trends. The choice of approaches depends on variable, time and space under consideration and needs to be carefully considered in product development.
(3) Overall, skillful high-resolution decadal predictions for 3-year SPI of annual means are possible for several north-western, central and south-eastern parts of Germany, exceeding correlation coefficients of 0.6 and/or revealing significantly positive RPSS against the reference prediction “observed climatology.” This also holds for 3-year mean ASO predictions in northern and western Germany. The skill of these high-resolution results is mostly similar to decadal drought predictions of former studies: Paxian et al. (2019) find similar skill for 4-year mean SPI predictions in different areas of the globe. Four-year mean SPEI predictions based on the Thornthwaite (1948) parametrization for PET achieve higher skills in the tropics due to large temperature trends but cannot be applied to colder seasons in Germany. Solaraju-Murali et al. (2021) find as well positive RPSS for 5-year mean SPEI predictions for the 6 months preceding the wheat harvest month in several regions worldwide, based on a multi-model. For northern Germany and Scandinavia, Solaraju-Murali et al. (2019) detect multi-model skill of five-year mean summer SPI. Finally, similar correlations and probabilistic scores are found for a NAO-based multi-model prediction of the 10-year mean winter precipitation for regional means of Spanish and Italian river catchments (see text footnote 1).
(4) The close co-production with the Wupper catchment water board in developing the product sheet is essential to guarantee that it is understandable, matches user needs and that format and content can be used in their working routine. They computed the drought index following their standard procedure because they use statistical relationships between drought index values and dam water levels based on this method. In addition, feedback of the Wupper catchment water board and further German water managers at the C3S_34c showcasing event was gathered. The usability of the product sheet in their work was confirmed, first feedback could be considered within the project, and we aim at developing further required products to consider the residual feedback in the future. This confirms similar experiences in the development of the DWD climate predictions website8. Users are involved in the product development via individual meetings, surveys and workshops, and such feedback loops strongly improve the understandability and applicability of the final climate service.
(5) This case study was part of the C3S_34c contract developing sector-specific applications. A close scientific exchange between the developers took place improving the prevailing product sheet. The first case study using the decadal predictions initialized in November 2019 applied the downscaling of the old MPI-ESM version. The focus was set on the 3-year mean high-resolution SPEI of the FMA season, closely following the user need and applying the Penman-Monteith parametrization (Allen et al., 1998) for PET. Unfortunately, high-resolution observations for wind and radiation in Germany are only available for a short evaluation period of 1995–2012. However, after a model update to the new MPI-ESM-LR version the first case study was not skillful any more. The developers of the other case studies recommended to use a longer evaluation period to be more robust, more ensemble members from different models to be more resilient against model updates and to improve skill in Europe considering the signal-to-noise paradox of weak predictable model signals (Scaife and Smith, 2018) and skillful large-scale teleconnections to improve the skill (see text footnote 1)9. In the second case study based on the downscaling of the new model version initialized in November 2020 (and presented now in this paper) the 3-year mean high-resolution SPI is chosen, allowing for a long evaluation period and robust statistical recalibration due to available high-resolution precipitation observations. The FairRPSS results (cf., Figure 6) indicate a potential skill improvement with increasing ensemble size. However, a multi-model cannot be downscaled because the daily input data for EPISODES is not (yet) available (see Section Outlook). In addition, large-scale teleconnections between a multi-model NAO prediction (based on the four global models of the scientific partners) and high-resolution SPI and SPEI observations in Germany were analyzed but the link is not strong enough to improve skill (see Section Outlook). Nevertheless, a skillful high-resolution SPI prediction is found, highlighting the benefit of close scientific exchange in product development.
(6) The final conclusion is the most important one and directly results from the experiences described in conclusion (5). User needs and scientific capabilities need to be weighed against each other. Users often ask for very specific products, considering complex variables, high spatial resolution and short time periods. However, decadal prediction skill is mostly found for large-scale variables over large regions and time periods. Thus, if no skill is detected for a certain user need, a “compromise solution” might be found in analyzing other variables, but of a similar kind, larger areas or longer time periods as described in conclusion (5). Within the co-production of a climate service such alternative products need to be defined in cooperation with the user, of course.
Outlook
This study motivates further research to improve decadal prediction skill of high-resolution drought predictions for German water boards: first, the statistical downscaling EPISODES should be applied to more ensemble members of a multi-model ensemble. To reach this goal daily temperature, relative humidity and geopotential height fields at different levels need to be available from decadal prediction systems. In addition, EPISODES was developed to downscale climate projections and is thus, optimized to reduce bias but not to enhance skill. Thus, the downscaling should not only consider the best relationship between a large-scale input variable and a high-resolution output variable but also the best skill of the large-scale input. More skillful large-scale teleconnection patterns in a larger region of the North Atlantic/European sector need to be considered in statistical downscaling. A first analysis shows that the teleconnection of the NAO to Germany is not strong enough, but further teleconnections need to be investigated.
Second, the C3S_34c showcasing event revealed that German water boards are interested in decadal predictions of high-resolution droughts and further atmospheric variables relevant for water management and hydrological impact modeling. Concerning more robust SPEI predictions, high-resolution observations for radiation and wind with long time periods would be needed for the Penman-Monteith parameterization (Allen et al., 1998) of PET. In this context, it would be interesting to test the parameterization of Hargreaves and Samani (1985), requiring less input data than Penman-Monteith and thus, simplifying the search for high-resolution observations in Germany. Instead, the parameterization of Thornthwaite (1948) cannot be computed for cold seasons in Germany. Overall, DWD plans to publish operational predictions for high-resolution drought conditions in Germany for the next weeks, months and years based on sub-seasonal, seasonal and decadal climate predictions on the DWD climate predictions website (see text footnote 8). Further relevant variables such as wind, humidity or radiation might be added as well to cover the needs of German water boards and hydrological modelers. In addition, the access to the downscaled prediction data should be enabled. Regular exchange in user workshops, surveys and individual user meetings supports the development of this climate service to ensure that the presented operational prediction products are understandable and can be applied in the working routines of the users.
Data Availability Statement
The raw data supporting the conclusions of this article will be made available by the authors, without undue reservation.
Author Contributions
APax designed the methodological concept of the case study assisted by BF, KP, and MS. KP generated the global model data. AH and PL generated the downscaled data. APas and BM conducted the recalibration. ML-V, KR, and KP conducted the calculation of the SPI (and the SPEI in the first case study). KR and APax assessed the prediction skill, estimated probabilistic predictions, designed the product sheet, and considered the user feedback. APas executed a SPI (and SPEI) prediction based on a multi-model NAO prediction. APax wrote the manuscript and considered revisions of all coauthors. All authors contributed to the article and approved the submitted version.
Funding
The authors acknowledge funding from the C3S_34c contract on the development of prototype climate services based on decadal predictions (contract number: ECMWF/COPERNICUS/2019/C3S_34c_DWD) of the Copernicus Climate Change Service (C3S) operated by the European Centre of Medium-Range Weather Forecasts (ECMWF). In addition, several authors were funded by the Deutscher Wetterdienst (DWD) and the Wupper catchment water board (Wupperverband).
Conflict of Interest
The authors declare that the research was conducted in the absence of any commercial or financial relationships that could be construed as a potential conflict of interest.
Publisher's Note
All claims expressed in this article are solely those of the authors and do not necessarily represent those of their affiliated organizations, or those of the publisher, the editors and the reviewers. Any product that may be evaluated in this article, or claim that may be made by its manufacturer, is not guaranteed or endorsed by the publisher.
Acknowledgments
We thank the Wupper catchment water board for providing the algorithm for SPI calculation, and we are grateful to the MiKlip project for providing the FREVA software tools including recalibration, skill assessment and probabilistic prediction tools. We especially acknowledge the developers of the other C3S_34c case studies, Jens Grieger (Freie Universität Berlin), Clementine Dalelane and Markus Ziese (DWD) for discussions to improve the methodological concept of this study.
Footnotes
1. ^https://climate.copernicus.eu/decadal-predictions-energy
2. ^https://climate.copernicus.eu/sectoral-applications-decadal-predictions
3. ^https://climate.copernicus.eu/decadal-predictions-infrastructure
4. ^https://esgf.dwd.de/projects/esgf-dwd/
5. ^https://opendata.dwd.de/climate_environment/CDC/grids_germany/daily/hyras_de/precipitation/
7. ^https://climate.copernicus.eu/sites/default/files/2021-09/Technical_appendix_2020.pdf
8. ^www.dwd.de/climatepredictions
9. ^https://climate.copernicus.eu/decadal-predictions-insurance
References
Agresti, A., and Hitchcock, D. B. (2005). Bayesian inference for categorical data analysis. Statist. Meth. Applicat. 14, 297–330. doi: 10.1007/s10260-005-0121-y
Allen, R., Pereira, L., Raes, D., and Smith, M. (1998). Crop Evapotranspiration – Guidelines for Computing Crop Water Requirements. FAO Irrigation and drainage paper 56. Rome: Food and Agriculture Organization of the United Nations (FAO). Available online at: http://www.fao.org/docrep/X0490E/x0490e00.htm#Contents (accessed January 26, 2022)
Athanasiadis, P. J., Yeager, S., Kwon, Y. O., Bellucci, A., Smith, D. W., and Tibaldi, S. (2020). Decadal predictability of North Atlantic blocking and the NAO. NPJ Clim. Atmos. Sci. 3, 20. doi: 10.1038/s41612-020-0120-6
Bellucci, A., Haarsma, R., Bellouin, N., Booth, B., Cagnazzo, C., van den Hurk, B., et al. (2015). Advancements in decadal climate predictability: the role of nonoceanic drivers. Rev. Geophys. 53, 165–202. doi: 10.1002/2014RG000473
Benson, C., and Clay, E. J (1998). The Impact of Drought on Sub-Saharan Economies. World Bank Tech. Paper No. 401. London: Overseas Development Institute, Regent's College. doi: 10.1596/0-8213-4180-4
Bosseler, B., Salomon, M., Schlüter, M., and Rubinato, M. (2021). Living with urban flooding: a continuous learning process for local municipalities and lessons learnt from the 2021 events in Germany. Water 13, 2769. doi: 10.3390/w13192769
Brune, S., Nerger, L., and Baehr, J. (2015). Assimilation of oceanic observations in a global coupled Earth system model with the SEIK filter. Ocean Modell. 96, 254–264. doi: 10.1016/j.ocemod.2015.09.011
Bruno Soares, M., Alexander, M., and Dessai, S. (2018). Sectoral use of climate information in Europe: a synoptic overview. Clim. Serv. 9, 5–20. doi: 10.1016/j.cliser.2017.06.001
Buontempo, C., Hanlon, H. M., Bruno Soares, M., Christel, I., Soubeyroux, J. M., Viel, C., et al. (2018). What have we learnt from EUPORIAS climate service prototypes? Clim. Serv. 9, 21–32. doi: 10.1016/j.cliser.2017.06.003
Buontempo, C., Hutjes, R., Beavis, P., Berckmans, J., Cagnazzo, C., Vamborg, F., et al. (2019). Fostering the development of climate services through Copernicus climate change service (C3S) for agricultural applications. Weath. Clim. Extr. 27, 100226. doi: 10.1016/j.wace.2019.100226
Changnon, S. D.. (2003). Measure of economic impacts of weather extremes. Bull. Amer. Meteor. Soc. 84, 1231–1235. doi: 10.1175/BAMS-84-9-1231
Chikamoto, Y. A, Timmermann, S., Stevenson, P., Dinezio, S., and Langford (2015). Decadal predictability of soil water, vegetation, and wildfire frequency over North America. Clim. Dyn. 45, 2213–2235. doi: 10.1007/s00382-015-2469-5
Dunstone, N., Lockwood, J., Solaraju-Murali, B., Reinhardt, K., Tsartsali, E., et al. (2022). Towards useful decadal climate services. Bull. Amer. Met. Soc. (in press). doi: 10.1175/BAMS-D-21-0190.1
Eyring, V., Bony, S., Meehl, G. A., Senior, C. A., Stevens, B., Stouffer, R. J., et al. (2016). Overview of the coupled model Intercomparison project phase 6 (CMIP6) experimental design and organization. Geosc. Model Develop. 9, 1937–1958. doi: 10.5194/gmd-9-1937-2016
Feldmann, H., Pinto, J. G., Laube, N., Uhlig, M., Moemken, J., Früh, B., et al. (2019). Skill and added value of the MiKlip regional decadal prediction system for temperature over Europe. Tellus A 71, 1618678. 10.1080/16000870.2019.1618678
Ferro, C. A. T.. (2013). Fair scores for ensemble forecasts. Q. R. J. Meteorol. Soc. 140, 1917–1923. doi: 10.1002/qj.2270
Ferro, C. A. T., Richardson, D. S., and Weigel, A. P. (2008). On the effect of ensemble size on the discrete and continuous ranked probability scores. Meteor. Appl. 15, 19–24. doi: 10.1002/met.45
Frick, C., Steiner, H., Mazurkiewicz, A., Riediger, U., Rauthe, M., Reich, T., et al. (2014). Central European high-resolution gridded daily data sets (HYRAS): mean temperature and relative humidity. Meteorol. Z. 23, 15–32. doi: 10.1127/0941-2948/2014/0560
Fricko, O., Havlik, P., Rogelj, J., Klimont, Z., Gusti, M., and Johnson, N. (2017). The marker quantification of the Shared Socioeconomic Pathway 2: a middle-of-the-road scenario for the 21st century. Glob. Env. Change. 42, 251–267, doi: 10.1016/j.gloenvcha.2016.06.004
Gangstø, R., Weigel, A. P., Liniger, M. A., and Appenzeller, C. (2013). Methodological aspects of the validation of decadal predictions. Clim. Res. 55, 181–200. doi: 10.3354/cr01135
Good, S. A., Martin, M. J., and Rayner, N. A. (2013). EN4: Quality controlled ocean temperature and salinity profiles and monthly objective analyses with uncertainty estimates. J. Geophys. Res. Oceans. 118, 6704–6716. doi: 10.1002/2013JC009067
Hargreaves, G. H., and Samani, Z. A. (1985). Reference crop evapotranspiration from temperature. Appl. Engin. Agricult. 1, 96–99. doi: 10.13031/2013.26773
Hermanson, L., Eade, R., Robinson, N. H., Dunstone, N. J., Andrews, M. B., Knight, J. R., et al. (2014). Forecast cooling of the Atlantic subpolar gyre and associated impacts. Geophys. Res. Lett. 41, 5167–5174. doi: 10.1002/2014GL060420
Hermanson, L., Smith, D., Seabrook, M., Bilbao, R., Doblas-Reyes, F., Tourigny, E., et al. (2022). WMO global annual to decadal climate update: a prediction for 2021–2025. Bull. Am. Met. Soc. 103, E1117–E1129. doi: 10.1175/BAMS-D-20-0311.1
Hersbach, H., Bell, B., Berrisford, P., Hirahara, S., Horányi, A., Muñoz-Sabater, J., et al. (2020). The ERA5 global reanalysis. Q. J. R. Meteorol. Soc. 146, 1999–2049. doi: 10.1002/qj.3803
Illing, S., Kadow, C., Kunst, O., and Cubasch, U. (2014). MurCSS: a tool for standardized evaluation of decadal hindcast systems. J. Open Res. Softw. 2, e24. doi: 10.5334/jors.bf
Jungclaus, J. H., Fischer, N., Haak, H., Lohmann, K., Marotzke, J., Matei, D., et al. (2013). Characteristics of the ocean simulations in MPIOM, the ocean component of the MPI-Earth system model. J. Adv. Model. Earth Syst. 5, 422–446. doi: 10.1002/jame.20023
Kadow, C., Illing, S., Lucio-Eceiza, E. E., Bergemann, M., Ramadoss, M., Sommer, P. S., et al. (2021). Introduction to Freva – a free evaluation system framework for Earth system modeling. J. Open Res. Softw. 9, 13. doi: 10.5334/jors.253
Kalnay, E., Kanamitsu, M., Kistler, R., Collins, W., Deaven, D., Gandin, L., et al. (1996). The NCEP/NCAR 40-year reanalysis project. Bull. Am. Meteorol. Soc. 77, 437–471. doi: 10.1175/1520-0477(1996)077<0437:TNYRP>2.0.CO
Kharin, V. V., Boer, G. J., Merryfield, W. J., Scinocca, J. F., and Lee, W.-S. (2012). Statistical adjustment of decadal predictions in a changing climate. Geophys. Res. Lett. 39, L19705. doi: 10.1029/2012GL052647
Klein, W., Lewis, B., and Enger, I. (1959). Objective prediction of five-day mean temperatures during winter. J. Meteorol. 16, 672–682. doi: 10.1175/1520-0469(1959)016<0672:OPOFDM>2.0.CO
Koks, E., Van Ginkel, K., Van Marle, M., and Lemnitzer, A. (2021). Brief Communication: critical infrastructure impacts of the 2021 mid-July western European flood event. Nat. Hazards Earth Syst. Sci. Discuss. (Preprint, in review). doi: 10.5194/nhess-2021-394. Available online at: https://nhess.copernicus.org/preprints/nhess-2021-394/
Kreienkamp, F., Lorenz, P., and Geiger, T. (2020). Statistically downscaled CMIP6 projections show stronger warming for Germany. Atmosphere 11, 1245. doi: 10.3390/atmos11111245
Kreienkamp, F., Paxian, A., Früh, B., Lorenz, P., and Matulla, C. (2018). Evaluation of the empirical-statistical downscaling method EPISODES. Clim. Dyn. 52, 991–1026 (2019). doi: 10.1007/s00382-018-4276-2
Kreienkamp, F., Philip, S. Y., Tradowsky, J. S., Kew, S. F., Lorenz, P., Arrighi, J., et al. (2021). Rapid Attribution of Heavy Rainfall Events Leading to the Severe Flooding in Western Europe During July 2021. Available online at: https://www.worldweatherattribution.org/wp-content/uploads/Scientific-report-Western-Europe-floods-2021-attribution.pdf (accessed January 26, 2022)
Kruschke, T., Rust, H. W., Kadow, C., Leckebusch, G. C., and Ulbrich, U. (2014). Evaluating decadal predictions of northern hemispheric cyclone frequencies. Tellus A 66, 22830. doi: 10.3402/tellusa.v66.22830
Kruschke, T., Rust, H. W., Kadow, C., Müller, W. A., Pohlmann, H., Leckebusch, G. C., et al. (2015). Probabilistic evaluation of decadal prediction skill regarding Northern Hemisphere winter storms. Meteorol. Z. 25, 721–738. doi: 10.1127/metz/2015/0641
Kushnir, Y., Scaife, A. A., Arritt, R., Balsamo, G., Boer, G., Doblas-Reyes, F., et al. (2019). Towards operational predictions of the near-term climate. Nat. Clim. Chang. 9, 94–101. doi: 10.1038/s41558-018-0359-7
Lloyd-Hughes, B., and Saunders, M. A (2002). A drought climatology for Europe. Int. J. Climatol. 22, 1571–1592. doi: 10.1002/joc.846
Lorza-Villegas, M. P., Becker, R., Scheibel, M., aus der Beek, T., and Roehrig, J. (2021). Assessing short-term climate change impacts on water supply at the Wupper catchment area, Germany. Nat. Hazards Earth Syst. Sci. (Preprint, in review). doi: 10.5194/nhess-2020-429. Available online at: https://nhess.copernicus.org/preprints/nhess-2020-429/
Matei, D., Pohlmann, H., Jungclaus, J., Müller, W., Haak, H., and Marotzke, J. (2012). Two tales of initializing decadal climate prediction experiments with the ECHAM5/MPI-OMmodel. J. Climate. 25, 8502–8523. doi: 10.1175/JCLI-D-11-00633.1
McKee, T. B., Doesken, N. J., and Kliest, J. (1993). “The relationship of drought frequency and duration to time scales”, in Proceedings of the 8th Conference of Applied Climatology, 17–22 January (Anaheim, CA: Boston, MA: Am. Met. Soc.), 179–184.
Meehl, G. A., Goddard, L., Murphy, J., Stouffer, R. J., Boer, G., Danabasoglu, G., et al. (2009). Decadal prediction – can it be skilful? Bull. Amer. Meteor. Soc. 90, 1467–1485. doi: 10.1175/2009BAMS2778.1
Moemken, J., Feldmann, H., Pinto, J. G., Buldmann, B., Laube, N., Kadow, C., et al. (2020). The regional MiKlip decadal prediction system for Europe: hindcast skill for extremes and user-oriented variables. Int. J. Climatol. 2020, 1–14. doi: 10.1002/joc.6824
Moemken, J., Reyers, M., Buldmann, B., and Pinto, J. G. (2016). Decadal predictability of regional scale wind speed and wind energy potentials over Central Europe. Tellus A 68, 29199. doi: 10.3402/tellusa.v68.29199
Ostermöller, J., Lorenz, P., Fröhlich, K., Kreienkamp, F., and Früh, B. (2021). Downscaling and evaluation of seasonal climate data for the european power sector. Atmosphere 12, 304. doi: 10.3390/atmos12030304
Paeth, H., Paxian, A., Sein, D., Jacob, D., Panitz, H.-J., Warscher, M., et al. (2017). Decadal and multi-year predictability of the West African monsoon and the role of dynamical downscaling. Meteorol. Z. 26, 363–377. doi: 10.1127/metz/2017/0811
Palmer, W. C.. (1965). Meteorological Drought. Research paper no. 45. Washington, DC: U.S. Weather Bureau. Available online at: http://www.ncdc.noaa.gov/temp-and-precip/drought/docs/palmer.pdf (accessed January 26, 2022).
Pasternack, A., Bhend, J., Liniger, M. A., Rust, H. W., Müller, W. A., and Ulbrich, U. (2018). Parametric decadal climate forecast recalibration (DeFoReSt 1.0). Geosci. Model Dev. 11, 351–368. doi: 10.5194/gmd-2017-162
Pasternack, A., Grieger, J., Rust, H. W., and Ulbrich, U. (2021). Recalibrating decadal climate predictions – what is an adequate model for the drift? Geosci. Model Dev. 14, 4335–4355. doi: 10.5194/gmd-14-4335-2021
Paxian, A., Sein, D., Panitz, H.-J., Warscher, M., Breil, M., Engel, T., et al. (2016). Bias reduction in decadal predictions of West African monsoon rainfall using regional climate models. J. Geophys. Res. Atmos. 121, 1715–1735. doi: 10.1002/2015JD024143
Paxian, A., Ziese, M., Kreienkamp, F., Pankatz, K., Brand, S., and Pasternack, A. (2019). User-oriented global predictions of the GPCC drought index for the next decade. Meteorol. Z. 28, 3–21. doi: 10.1127/metz/2018/0912
Pohlmann, H., Müller, W. A., Kulkarni, K., Kameswarrao, M., Matei, D., Vamborg, F. S. E., et al. (2013). Improved forecast skill in the tropics in the new MiKlip decadal climate predictions. Geophys. Res. Lett. 40, 5798–5802. doi: 10.1002/2013GL058051
Rauthe, M., Steiner, H., Riediger, U., Mazurkiewicz, A., and Gratzki, A. (2013). A Central European precipitation climatology – part I: generation and validation of a high-resolution gridded daily data set (HYRAS). Meteorol. Z. 22, 235–256. doi: 10.1127/0941-2948/2013/0436
Razafimaharo, C., Krähenmann, S., Höpp, S., Rauthe, M., and Deutschländer, T. (2020). New high-resolution gridded dataset of daily mean, minimum, and maximum temperature and relative humidity for Central Europe (HYRAS). Theor. Appl. Climatol. 142, 1531–1553. doi: 10.1007/s00704-020-03388-w
Reyers, M., Feldmann, H., Mieruch, S., Pinto, J. G., Uhlig, M., Ahrens, B., et al. (2019). Development and prospects of the regional MiKlip decadal prediction system over Europe: predictive skill, added value of regionalization, and ensemble size dependency. Earth Syst. Dyn. 10, 171–187. doi: 10.5194/esd-10-171-2019
Richling, A., Kadow, C., and Illing, S. (2017). Problems. Version from March 10, 2017. Available online at: https://www.xces.dkrz.de/about/problems/ (accessed January 26, 2022).
Risbey, J. S., Squire, D. T., Black, A. S., et al. (2021). Standard assessments of climate forecast skill can be misleading. Nat. Commun. 12, 4346. doi: 10.1038/s41467-021-23771-z
Sahastrabuddha, R., and Ghosh, S. (2021). Does statistical model perform at par with computationally expensive general circulation model for decadal prediction? Environ. Res. Lett. 16, 064028. doi: 10.1088/1748-9326/abfeed
Salvi, K., Villarini, G., and Vecchi, G. A. (2017). High resolution decadal precipitation predictions over the continental United States for impacts assessment. J. Hydrol. 553, 559–573. doi: 10.1016/j.jhydrol.2017.07.043
San-Martín, D., Manzanas, R., Brands, S., Herrera, S., and Gutiérrez, J. M. (2017). Reassessing model uncertainty for regional projections of precipitation with an ensemble of statistical downscaling methods. J. Clim. 30, 203–223. doi: 10.1175/JCLI-D-16-0366.1
Scaife, A. A., and Smith, D. (2018). A signal-to-noise paradox in climate science. NPJ Clim. Atmos. Sci. 1, 28. doi: 10.1038/s41612-018-0038-4
Schuldt, B., Buras, A., Arend, M., Vitasse, Y., Beierkuhnlein, C., Damm, A., et al. (2020). A first assessment of the impact of the extreme 2018 summer drought on Central European forests. Basic Appl. Ecol. 45, 86–103. doi: 10.1016/j.baae.2020.04.003
Sheen, K. L., Smith, D. M., Dunstone, N. J., Eade, R., Rowell, D. P., and Vellinga, M. (2017). Skilful prediction of Sahel summer rainfall on inter-annual and multi-year timescales. Nat. Comm. 8, 14966. doi: 10.1038/ncomms14966
Siegert, S.. (2014). SpecsVerification: Forecast Verification Routines for Ensemble Forecasts of Weather and Climate. Available online at: http://CRAN.R-project.org/package=SpecsVerification (accessed January 28, 2022).
Smith, D. M., Eade, R., Scaife, A. A., Caron, L.-P., Danabasoglu, G., DelSole, T. M., et al. (2019). Robust skill of decadal climate predictions. NPJ Clim. Atmos. Sci. 2, 1–10. doi: 10.1038/s41612-019-0071-y
Smith, D. M., Scaife, A. A., Eade, R., Athanasiadis, P., Bellucci, A., Bethke, I., et al. (2020). North Atlantic climate far more predictable than models imply. Nature 583, 796–800. doi: 10.1038/s41586-020-2525-0
Sohn, S.-J., Ahn, J.-B., and Tam, C.-Y. (2013). Six month–lead downscaling prediction of winter to spring drought in South Korea based on a multimodel ensemble. Geophys. Res. Lett. 40, 579–583. doi: 10.1002/grl.50133
Solaraju-Murali, B., Caron, L.-P., Gonzalez-Reviriego, N., and Doblas-Reyes, F. J. (2019). Multi-year prediction of European summer drought conditions for the agricultural sector. Environ. Res. Lett. 14, 124014. doi: 10.1088/1748-9326/ab5043
Solaraju-Murali, B., Gonzalez-Reviriego, N., Caron, L.-P., Ceglar, A., Toreti, A., Zampieri, M., et al. (2021). Multi-annual prediction of drought and heat stress to support decision making in the wheat sector. NPJ Clim. Atmos. Sci. 4, 34. doi: 10.1038/s41612-021-00189-4
Stevens, B., Giorgetta, M., Esch, M., Mauritsen, T., Crueger, T., Rast, S., et al. (2013). Atmospheric component of the MPI-M Earth system model: ECHAM6. J. Adv. Model. Earth Sys. 5, 146–172. doi: 10.1002/jame.20015
Thornthwaite, C.. (1948). An approach towards a rational classification of climate. Geogr. Rev. 38, 55–94. doi: 10.2307/210739
Toreti, A., Belward, A., Perez-Dominguez, I., Naumann, G., Luterbacher, J., Cronie, O., et al. (2019). The exceptional 2018 European water seesaw calls for action on adaptation. Earth's Future 7, 652–663. doi: 10.1029/2019EF001170
Uppala, S. M., KÅllberg, P. W., Simmons, A. J., Andrae, U., Da Costa Bechtold, V., Fiorino, M., et al. (2005). The ERA-40 re-analysis. Q. J. R. Meteorol. Soc. 131, 2961–3012. doi: 10.1256/qj.04.176
Van Oldenborgh, G. J., Doblas-Reyes, F. J., Wouters, B., and Hazeleger, W. (2012). Decadal prediction skill in a multi-model ensemble. Clim. Dyn. 38, 1263–1280. doi: 10.1007/s00382-012-1313-4
Vicente-Serrano, S. M., Begueria, S. A. S., and Lopez-Moreno, J. I. (2010). A multiscalar Drought Index sensitive to global warming: the standardized precipitation evapotranspiration index. J. Clim. 23, 1696–1718. doi: 10.1175/2009JCLI2909.1
Wieland, M., and Martinis, S. (2020). Large-scale surface water change observed by Sentinel-2 during the 2018 drought in Germany. Int. J. Rem. Sens. 41, 4742–4756. doi: 10.1080/01431161.2020.1723817
Wilks, D.. (2011). Statistical Methods in the Atmospheric Sciences. Oxford; Amsterdam: Elsevier Academic Press.
Keywords: decadal prediction, drought, high resolution, statistical downscaling, recalibration, water management, user co-production, case study
Citation: Paxian A, Reinhardt K, Pankatz K, Pasternack A, Lorza-Villegas MP, Scheibel M, Hoff A, Mannig B, Lorenz P and Früh B (2022) High-Resolution Decadal Drought Predictions for German Water Boards: A Case Study for the Wupper Catchment. Front. Clim. 4:867814. doi: 10.3389/fclim.2022.867814
Received: 01 February 2022; Accepted: 24 June 2022;
Published: 19 July 2022.
Edited by:
Fai Fung, Met Office, United KingdomReviewed by:
Subimal Ghosh, Indian Institute of Technology Bombay, IndiaDougal Squire, Commonwealth Scientific and Industrial Research Organisation (CSIRO), Australia
Copyright © 2022 Paxian, Reinhardt, Pankatz, Pasternack, Lorza-Villegas, Scheibel, Hoff, Mannig, Lorenz and Früh. This is an open-access article distributed under the terms of the Creative Commons Attribution License (CC BY). The use, distribution or reproduction in other forums is permitted, provided the original author(s) and the copyright owner(s) are credited and that the original publication in this journal is cited, in accordance with accepted academic practice. No use, distribution or reproduction is permitted which does not comply with these terms.
*Correspondence: Andreas Paxian, andreas.paxian@dwd.de