- 1Post-processing, Analysis and Model Development, GeoSphere Austria, Vienna, Austria
- 24ward Energy Research GmbH, Graz, Austria
- 3Nowcasting, Analysis and Model Development, GeoSphere Austria, Vienna, Austria
Introduction: With hydropower being the dominant source of renewable energy in Austria and recent years being disproportionally dry, alternative renewable energy sources need to be tapped to compensate for the reduction of fossil fuels and account for dry conditions. This becomes even more important given the current geopolitical situation. Wind power plays an essential role in decarbonizing Austria's electricity system. For local assessments of historic, recent, and future wind conditions, adequate climate data are essential. Reanalysis data, often used for such assessments, have a coarse spatial resolution and could be unable to capture local wind features relevant for wind power modeling. Thus, raw reanalysis data need post-processing, and the results need to be interpreted with care. The purpose of this study is to assess the quality of three reanalysis data sets, such as MERRA-2, ERA5, and COSMO-REA6, for both surface level and hub height wind speed and wind power production at meteorological observation sites and wind farms in flat and mountainous terrain. Furthermore, the study aims at providing a first knowledge baseline toward generating a novel wind speed and wind power atlas at different hub heights for Austria with a spatial resolution of 1 × 1 km and for an experimental region with sub-km resolution. Thus, the study tries to answer (i) the questions if the reanalysis and analysis data can reproduce surface-level wind speed and (ii) if wind power calculations based on these data can be trusted, providing a knowledge base for future wind speed and wind power applications in complex terrain.
Methods: For that purpose, a generalized additive model (GAM) is applied to enable a data-driven gridded surface wind speed analysis as well as extrapolation to hub heights as a first step toward generating a novel wind speed atlas. In addition, to account for errors due to the coarse grid of the re-analysis, the New European Wind Atlas (NEWA) and the Global Wind Atlas (GWA) are used for correction using an hourly correction factor accounting for diurnal variations. For the analysis of wind power, an empirical turbine power curve approach was facilitated and applied to five different wind sites in Austria.
Results and discussion: The results showed that for surface-level wind speed, the GAM outperforms the reanalysis data sets across all altitude levels with a mean average error (MAE) of 1.65 m/s for the meteorological sites. It even outperforms the NEWA wind atlas, which has an MAE of 3.78 m/s. For flat regions, the raw reanalysis matches the production data better than NEWA, also for hub height wind speeds, following wind power. For the mountainous areas, a correction of the reanalysis data based on the NEWA climatology, or even the NEWA climatology itself, significantly improved wind power evaluations. Comparisons between modeled wind power time series and real data show mean absolute errors of 8% of the nominal power in flat terrain and 14 or 17% in mountainous terrain.
1. Introduction
The share of wind energy in energy production is increasing and will continue increasing given the world a wide aim of being as carbon neutral as possible by 2030 (European Commission, 2020). To fulfill this aim, growth rates of renewable energy must increase by at least four times compared with recent growth rates (International Renewable Energy Agency, 2020). With the recent shortage of natural gas in Europe for all sectors ranging from industry and mobility to heating and cooling, a rapid transition to a larger share of renewable energy is needed. However, in contrast to gas-driven energy production, the stochastic nature of the main driving forces of renewable energy systems such as wind and consequently wind energy or cloud cover and solar energy further complicates energy balancing and places an additional load on the grid. As the share of wind energy increases, the impact on the electric system and its infrastructure also increases from local to large scale, sometimes even on a sub-continental scale. Furthermore, energy trading and market volatility are influenced. As a result, an increase in wind energy or other renewable energy sources has large influences across all sectors (Silva et al., 2018) and contributes to the overall vulnerability of the electricity system (Bloomfield et al., 2016). As the atmospheric conditions are the main drivers of power production estimation for both current and future conditions, a good representation of these conditions is needed. An accurate assessment of the potential wind power sites as well as assessment of existing sites in terms of climate change adaptation strategies and wind turbine refactoring is essential to increase production efficiency. Such an assessment is needed for the estimation of future wind power in-feed rates into the power grid and planning of possible storage solutions. To perform such an assessment, historical and recent information on wind speed, wind power production, and demand is needed.
In the past years, the number of resource assessment research studies, especially for wind energy, has increased. The studies and review papers focused on several aspects of improving methods for site assessment (Kelledy, 1982; Troen and Lundtang Petersen, 1989; Bailey et al., 1997; Truhetz et al., 2007; Lackner et al., 2008) and/or prediction of production for both on shore and offshore wind energy such as land-use mapping, social constraints (Schallenberg-Rodriguez, 2013; Höltinger et al., 2016; Jäger et al., 2016; Harper et al., 2019), or improving atmospheric and climate models (Singh et al., 2006; Zhang et al., 2014; Widén et al., 2015; Gualtieri, 2019; Yang et al., 2021).
Recently, McKenna et al. (2022) provided an overview on the different categories of potentials of onshore wind energy assessment (geographical, technical, economical, and feasible) and discussed the state of the art for each of the categories. In this study, we focused on assessing a subcategory of the technical onshore wind energy potential, namely, the meteorological wind power assessment. McKenna et al. (2022) defined two types of requirements in this category, the climatological wind potential assessment and the time-resolved renewable generation variability. While the first relies on wind speed distributions provided by wind atlases, the latter needs time series information from wind masts or reanalysis fields. Often the advantages of these two data sources are combined to bias correct reanalysis-based time series using climatological fields such as the Global Wind Atlas (GWA) (see e.g., Staffell and Pfenninger, 2016; González-Aparicio et al., 2017; Olauson, 2018; Camargo et al., 2019; Koivisto et al., 2021; Davidson and Millstein, 2022; Murcia et al., 2022). This bias correction approach is a well-known and well-used standard approach in this field. It however, only uses one correction factor and does not account for diurnal, monthly, or seasonal variations. We tackle this in our study by using an hourly correction factor. The data availability in this study for two of the wind farm sites is very limited, therefore we did not calculate monthly or seasonal factors.
The goal of this study is to assess the quality of different data sets and correction methods in terms of wind speed and wind power applications in different regions of Austria. The overall aim is to provide a first knowledge baseline toward generating a novel wind speed and wind power atlas at different hub heights for Austria with spatial resolution of 1 × 1 km and for an experimental region with sub-km resolution. Therefore, different data sets, both observations and reanalysis as well as correction methods, need a thorough investigation before finalizing the data to be fed into different (machine learning) algorithms used for generating this novel atlas. More precisely, wind speed and wind power time series computed from ERA5, MERRA2, COSMO-REA6, and NEWA, and a new method to grid observation data (GAM) is corrected with NEWA microclimate and GWA3 and validated against measurements. Various research studies used different reanalysis data sets, numerical weather prediction model data, and wind field atlases and investigated the quality of the data sets themselves as well as correction methods in regions across the world. As relevant studies until 2019 are summarized by Gruber et al. (2019), we provide an update of this summary adding an overview of studies in 2019. Table 1 provides an overview of the most recent studies. The particularity of this study consists of the detailed validation of the wind speed parameter at very local scales in Austria, as well as in complex terrain, and the variety of methods and data sets considered.
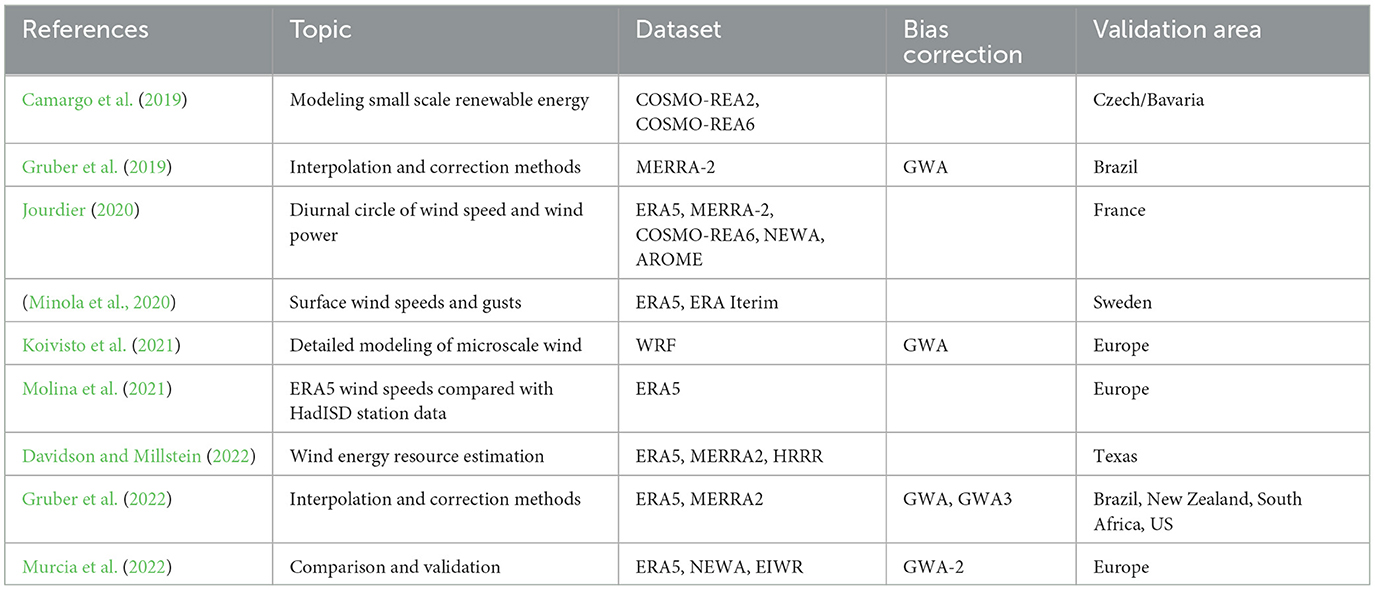
Table 1. Summary of recent studies (younger than 2019) assessing quality of data sets for wind speed and wind power assessment, their scopes, the datasets, bias correction, and validation.
Jourdier (2020) concluded that ERA5 outperforms MERRA-2 but underestimates wind speed, especially in regions with complex terrain. The higher resolved models could decrease the bias in complex terrain but showed other issues such as a wrong diurnal variation in COSMO-REA6. However, the author also found that the NEWA analysis had disappointing results compared with the other models. In contrast to other studies, no bias correction was applied using available local data, or a wind atlas as the GWA, as the general idea was to be able to use the data in regions with no direct observations. A comparable study, which uses bias correction, was carried out by Murcia et al. (2022) who analyzed the performance of ERA5, NEWA, and EIWR across Europe including a bias correction to match the microscale mean wind speed reported in the Global Wind Atlas version 2 (GWA2). Gruber et al. (2022) conducted a similar analysis comparing MERRA-2 and ERA5 combined to bias correction based on GWA2 and GWA3 in Brazil, New Zealand, South Africa, and the United States. All found that (bias corrected) ERA5 yielded the best results.
In addition to studies investigating the usefulness of reanalysis data for wind energy applications, studies also investigated the skills of surface layer wind representation. Minola et al. (2020) compared ERA5 and ERA Interim wind speeds and wind gusts with wind measurements in (i) coastal, (ii) flat, and (iii) mountainous terrain and found low positive bias in (i) and (ii) and an essential negative bias in (iii) for both variables.
In the context of current and future wind power generation and potential, Cai and Bréon (2021) investigated the impact of climate change for French onshore and offshore wind farms including bias corrections. Others such as Ma et al. (2022) investigated future wind power resource using convection permitting simulations for Canada, whereas Akhtar et al. (2021) considered wake loss effects in offshore wind power modeling.
Recently, Davidson and Millstein (2022) investigated the limitations of reanalysis data which are often used as hindcast data for climate modeling, ERA5 and MERRA-2, as well as a high resolution forecasting model HRRR in the context of wind power applications. They conclude that higher spatial resolutions improve the correlation and RMSE. However, although their representation of daily power averages is quite sufficient, the hourly representation is dominated by systematic errors in the diurnal patterns, even after conversion to solar time with worse metrics before sunrise. This has been affecting the generation of synthetic data for hourly wind energy modeling even in the high resolution model as well as when using interpolation of/between model levels to desired hub height. They conclude that research toward improving the nocturnal boundary layer representation in numerical models is needed to account for these issues. A similar study was carried out by Petrik et al. (2021) who investigated the representation of the boundary layer and related sub-daily parameter focusing on application in renewable energy using spatially higher resolved reanalysis (COSMO-REA6, UERRA) and hindcast runs with the COSMO model with different NWP model settings concluding that the model errors are dependent on the topographical representation and suitable error correction of reanalysis data, and dynamical downscaling with NWP or large-eddy simulation should be applied to improve the results of the model.
Validation of reanalysis, analysis, and also forecast wind fields, especially at heights above the standard measurement height (10 m a.g.l.) is limited by the data availability with a drawback that boundary layer parameterization errors, which are less pronounced in the surface wind field, are hard to detect. Errors found in the diurnal cycle (Jourdier, 2020) could also be attributed to a lack of a sufficient number of ground flux observations and thus may not be able to take different phenoma affecting the atmospheric conditions, taking hub height into account (Millstein et al., 2022). To estimate such effects, especially in wind energy applications, reanalysis data should be validated at both surface level and hub heights. Again, one of the weaknesses, here, is the lack of regular, long-term standard meteorological measurements at different vertical layers above the ground. Recently, a data set consisting of more than 200 measurement sites at tall towers was compiled (Ramon et al., 2020). Unfortunately, such measurement sites are still sparsely distributed and none are found in Austria.
In Austria, most wind farms are located in flat regions with only a few in higher alpine regions. There are many reasons for a lack of wind energy in the remaining parts of Austria; however, in the past years, wind farms are planned (and sometimes built) in more complex topographical regions. This is an essential step to reach the Austrian climate goals of a carbon neutral energy system in 2040. For operational forecasting and the development of climate adoption strategies, historical and prospective data at either wind farm level or even turbine level are needed, also in complex terrain, ideally with a high temporal resolution. Most of the previously mentioned studies validated the suitability of reanalysis data sets for wind power assessments based on conglomeration of wind farms or wind speed measurements, thus not prioritizing the applicability of reanalysis data sets to specific locations in complex terrain.
This study aims at evaluating the applicability of different reanalysis and analysis data sets for wind speed and wind energy production at turbine level for two different regions in Austria at five wind farms (confidential data). Furthermore, a data-driven surface wind analysis based on observations at meteorological sites is generated and validated for surface and hub heights. Different correction methods for the reanalysis data and the data-driven data analysis are evaluated against turbine measurements of wind speed and power as well as wind speed measurements at Austrian measurement sites located close to wind farms. The aims are:
1. assessing the accuracy of reanalysis data and analysis data for wind speed at the surface and hub heights at single sites and turbines;
2. evaluate the skills of a data-driven GAM model-based surface wind speed analysis and its extrapolation to hub height;
3. assess the generated wind power based on raw and corrected reanalysis and the data-driven GAM model analysis for flat and complex topographic located wind farms.
The relevant research questions are as follows:
1. Can an extrapolated and corrected wind analysis be used for hub height wind power estimation?
2. How well do the reanalysis data perform for flat and complex regions?
3. Does the standard approach of data correction suffice in hindsight of possible usage for climate change applications?
This study is structured as follows: in Section 2, the used data, correction methods, and models are described, and the conversion to wind energy production is explained. In addition, the use cases of our study are introduced. In Section 3 the results are discussed, and in Section 4 we draw our conclusions.
2. Materials and methods
The goal of this study is to assess the quality of different data sets and correction methods in terms of wind speeds and wind power. An overview of the used data sets and methods to reach this goal is shown in Figure 1.
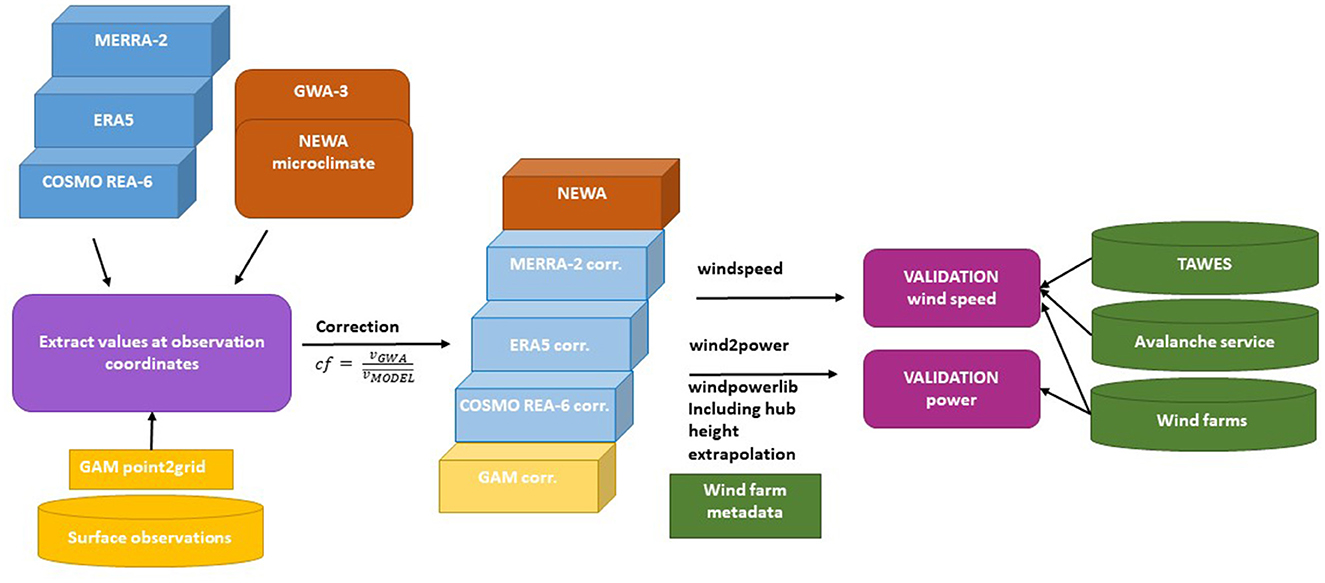
Figure 1. Overview of the approach: wind speed time series obtained from (corrected) reanalysis data sets ERA5, MERRA2, and COSMO-REA6 and processed surface observations from the GAM method are compared with measured data from weather stations (TAWES), avalanche services, and wind farms. In addition, wind power time series computed from wind speeds of the mentioned datasets are compared with wind power production data from five wind farms in Austria.
2.1. Data
As shown in Figure 1, (corrected) reanalysis and analysis data, as well as processed observations from the GAM, are compared with observation data obtained from weather stations (TAWES), avalanche services, and wind farms. An overview of all relevant data sets used in this study is shown in Table 2, and more background information is provided in the following subsections.
2.1.1. Reanalysis and analysis data
Three different reanalysis data sets, ERA5, MERRA-2, and COSMO-6 data, one analysis data set, the Incomprehensible Nowcasting and Analysis model (INCA), and the New European Wind Atlas were used in this study.
The ERA5 atmospheric reanalysis (Hersbach et al., 2020) from the European Center for Medium-range Weather Forecasts (ECMWF) is a long-term gridded data set spanning at least four decades (1979 onwards). The reanalysis is produced using a 4D-Var data assimilation with a fixed model version of the ECMWF IFS forecast model CY41R2. The reanalysis has recently been extended back to the 1950s. The temporal resolution of ERA5 reanalysis data is hourly with the surface parameters being available on a 30 km × 30 km grid. In addition to surface wind components, ERA5 provides wind speed information in 100 m and 200 m above ground level. ERA5 has been widely used for energy applications, with and without corrections (Olauson, 2018; Ramon et al., 2019; van der Wiel et al., 2019; Jourdier, 2020; Bloomfield et al., 2022). Despite its general good skills in representing the atmospheric conditions for wind energy applications, Jourdier (2020) found an issue in the diurnal cycle of ERA5 wind speed at different heights above the ground and in the lower model levels. Gaps at 10:00 UTC and following hours were found with a wind deficit of sometimes even larger than 0.5ms−1. The problem was acknowledged and linked to the data assimilation process (see Jourdier, 2020, for more information). In this study, only ERA5 single level products were used.
Another data set widely used in the wind industry is the MERRA-2 reanalysis (Gelaro et al., 2017), which integrates satellite data assimilation. MERRA-2 has an hourly temporal resolution but a coarser spatial resolution of 0.5° × 0.625° (50 km) compared with ERA5. MERRA-2 is available since 1980. It provides 10 m and 50 m a.g.l. wind components. Thus, extrapolation of wind speed to hub height may contain a higher level of uncertainty than in ERA5. Here, the time-averaged, single-level, and diagnostics data collection, labeled with tavg1_2d_slv_Nx, was used.
Compared with the typical size of a wind farm, both ERA5 and MERRA-2 reanalysis data sets have a low spatial resolution and are not able to capture local and small-scale features such as slope and valley wind systems or turbulence. In spite of other, global higher resolved reanalysis data sets still provide a good baseline, although corrections need to be applied.
In addition to the global reanalysis data sets, regional reanalysis covering parts of Europe with different horizontal resolutions are available. One of those reanalysis is the COSMO reanalysis data set with horizontal resolutions of 12 (as ensemble), 6, and 2 km, respectively. These are part of the Uncertainties in Ensembles of Regional ReAnalysis (UERRA) project. The COSMO-REA models (Bollmeyer et al., 2015; Wahl et al., 2017) use the DWD (German Weather Provider) operational NWP (Numerical Weather Prediction) model COSMO and are forced by the ERA-Interim analysis. In this study, it is decided to use the COSMO-REA6 model, as its domain covers most of Europe including the parts of Austria investigated in this study, which is not the case with COSMO-REA2.
Another analysis data set, based on downscaled ERA5, is recently finished, the New European Wind Atlas (NEWA; Hahmann et al., 2020). It is built using an ensemble of several sub-domains modeled with the Weather Research and Forecasting (WRF) model and has a spatial resolution of 3 km and temporal frequency of 30 min. As NEWA does not assimilate observations, it cannot be considered as a typical reanalysis data set. In contrast to the COSMO-REA6 data, it was driven by ERA5 using spectral nudging. In addition to the 3 km macro-scale NEWA atlas, also a micro-scale data set with 50 m resolution was calculated. However, this data set is currently not available.
For comparison of the newly generated analysis fields (see Section 2.1.2), the Integrated Nowcasting through Comprehensive Analysis (INCA) system (Haiden et al., 2011) was used for the selected avalanche service sites (see Section 2.1.4). Similar to NEWA, INCA is not only a reanalysis data set but also a statistical-dynamical nowcasting and analysis model operating at the Austrian national weather service ZAMG since 2003. It covers a domain consisting of Austria and some neighboring grid points with a horizontal resolution of 1 km and a temporal frequency of 1 h. However, INCA only provides surface data and no vertical levels. Here, it is only used for comparison between the new analysis fields as INCA assimilates observations. Thus, INCA at a grid point representing a site in Vienna would be identical to the observations at that respective site.
2.1.2. Data-driven GAM wind speed analysis
For comparison, data-driven analysis fields using measurements from selected observation sites were produced. Therefore, 10 meter station wind is used and interpolated with a generalized additive model (GAM) to the NEWA grid. GAM allows a combination of non-linear effects to calculate a spatial analysis. The idea is similar to fitting a spatial climatology with more details mentioned in the study by Dabernig et al. (2017) but without any seasonality because the analysis is recalculated at every time step individually. As a result, the reanalysis is a combination of a non-linear spatial effect between latitude and longitude and a linear effect to capture an altitude effect. To ensure a good performance on unseen stations, the degree of freedom for the spatial effect was kept at 20% of number of available stations. For a fair comparison, all the stations in the verification are left out in the reanalysis with GAM. The calculations of the GAM have been performed in R with the package bamlss.
To address the question of a surface-based analysis extrapolated to hub height could be used to assess wind power at different locations or even outperform reanalysis data, the GAM data are extrapolated to hubheight and corrected using GWA and NEWA for comparability (see Section 2.2.1).
2.1.3. Wind farm data
Data of five wind farms located in two Austrian regions were used in this study. Wind farms one, two, and three are located in Eastern Austria, southeast of Vienna, and in a topographical rather flat surrounding north of Lake Neusiedl (BGL hereafter). As they are located very close to each other, one can consider them as a single wind farm. Wind farm four is located in the south of Austria and in the Alpine range on a ridge and can be considered as complex topographical site (CPLX-1 hereafter). The altitude is ~1,900 m. Another wind farm, number five (CPLX-2), is located close to the southern border of Austria with Slovenia, on the border of two Austrian federal states. Here, the altitude is similar to farm four.Due to constraints of the data providers, a detailed description of the locations and turbine types cannot be given, however as much information as possible is shared. Both complex terrain wind farms are located in the vicinity of skiing resorts. Hub heights of the turbines vary from 80 m at the complex sites to 135 m at the BGL sites.
At the BGL sites, measurements of wind speed and temperature were carried out apart from 2016 with a sampling frequency of 10 minutes. For the CPLX-1 site, the data are available for 2018 and 2019, but in contrast to the BGL sites, only the generated power is available, whereas for CPLX-2, also minimum, maximum, and average wind speed are available. For all selected wind farms, in this study, data of every single wind turbine were used. Thus, in this study, we evaluated the skills and accuracy of the reanalysis data sets on turbine level and not on wind farm level.
2.1.4. Data from avalanche services and weather stations
To assess the skills of the reanalysis and analysis data sets not only on turbine level but also on surface level, wind speed data of the Austrian semi-automatic weather station network (TAWES) were used. A selection of sites across Austria in proximity of existing and planned wind farms was used (see Figure 2, right).
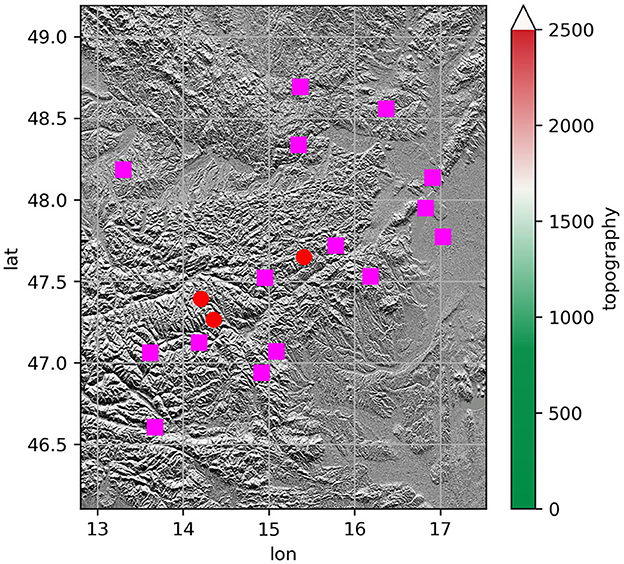
Figure 2. Location of the wind farm sites and selected alternative data of the Austrian avalanche services (red dots). Due to constraints, it is not possible to directly show the wind farm sites. In magenta, the sites of the Austrian Met Service, TAWES, are shown. As background, the EUDEM topography with 25 m resolution is used.
In Austria, most of the complex terrain wind farms are located in a mountainous environment along (gentle) ridges or on plateaus. Often, obtaining such wind farm data is either not possible or underlies very strict non-disclosure agreements. Thus, to verify the accuracy of reanalysis data sets and validate the correction methods in a more mountainous environment, meteorological data of the WMO-conform national meteorological service and/or the Austrian avalanche warning centers (in total seven centers in Austria) are a suitable alternative. However, the distribution of complex terrain WMO-conform measurement sites in complex terrain surroundings similar to wind turbine sites is very sparse. Here, data of the avalanche centers can be an alternative to get an idea of the atmospheric and climatic conditions of such locations. These meteorological observation sites monitor the same parameters as standard World Meteorological Organization (WMO)-conform observation sites and include additional observations such as snow height. They are, similarly as wind turbines located in higher altitudes, more prone to the prevailing atmospheric conditions such as icing, rime, or high wind speeds. Their wind speed and direction measurements are, unlike to standard meteorological sites, not measured at 10 m a.g.l. but at 2.5 m a.g.l, similar to other privately owned measurements such as FieldSense. They provide, however, the most dense high alpine observation network and give an estimate of the prevailing surface conditions. To be able to quantify the reanalysis skills in reproducing atmospheric conditions in more complex terrain at both hub height and surface level, sites in close proximity to the CPLX sites were chosen. One of such avalanche service sites is located within 2 kms of the CPLX-1 site, hereafter named AV-CPLX, to comply with data sharing restrictions. In addition to this site, another site in somewhat closer distance was selected in the northwest of the CPLX-1 wind farm, hereafter AVNW-CPLX. In addition to these two sites, another avalanche service site was chosen, hereafter AVV, which is located close to another high altitude wind farm not used in this study. However, wind speeds and other meteorological parameters recorded at AVV represent the conditions at the mentioned high altitude wind farm to a high accuracy. In Figure 2, AVV is located in the center. A summary of the wind farm and avalanche site specifications is shown in Table 2.
2.2. Methods
For this study, the following steps were taken to derive the results as follows: (i) extraction of relevant meteorological parameters from reanalysis and analysis data at the selected locations; (ii) generation of observation-based data-driven hourly analysis wind speed fields at the NEWA resolution; (iii) extrapolation to the respective hub heights of every turbine; (iv) correction of hub height wind speeds using a correction factor; (v) conversion of wind speeds to power. Steps (i), (iii), and (iv) were carried out using Python using pandas, numpy, metview, matplotlib, and the windpowerlib libraries. Step (ii) was carried out using R and the bamlss package. Both Linux and Windows OS and classical CPU architecture were used. The following subsections describe the methodology in more detail.
2.2.1. Interpolation, extrapolation, and correction
To horizontally match gridded reanalysis data to the exact locations of the observed wind turbines, wind speed, and air density were bilinearly interpolated for ERA5, COSMO6, and MERRA2. Because of the high geographical resolution of the INCA, the new data-driven GAM analysis, and NEWA data sets, the nearest neighbor approach, was sufficient to extract wind speed parameters within those data sets.
Depending on the available vertical heights of the models, wind speed data were extrapolated to the respective hub heights using Hellmann's Law (see Sharp, 2015). As information on the surface roughness is lacking, the Hellmann exponent was set to . This was done to extrapolate the reanalysis data sets as well as the NEWA data. Furthermore, to answer research question 2, we extrapolated the new data-driven GAM analysis fields to the respective hub heights. As this might result in comparatively low wind speed values, a correction was applied (see Section 2.2.2 for more details).
2.2.2. Correction with correction factors
Typically, to correct reanalysis data sets for wind energy applications, correction factors based on the Global Wind Atlas (GWA) are applied (Davis et al., 2023, see e.g., Gruber et al., 2022). Here, we used the latest available version of the GWA, version 3, at the corresponding vertical level of 10 and 100 m, respectively. As stated by Gruber et al. (2019), GWA2 would be superior for our application. However, these data are currently not available for download and usage. However, according to personal communication, these data might again be available in future. The daytime dependent correction factor, cfHH, is calculated using (Equation 1):
where vGWA and vMODEL are the climate mean wind speeds of the respective data sets in m/s. In contrast to other studies, we opted for calculating a correction factor based on the diurnal cycle. Thus, a correction factor cfHH was calculated for every hour of the day to account for systematic diurnal biases of the reanalysis models. Similar to the GWA correction, a correction with the climate fields of the NEWA was carried out. Here, the climate mean wind speed derived from the NEWA macroscale data set was used to calculate the correction coefficient. As follow-up, one could use the microscale data for such a correction for hub height levels. Based on the results of the meteorological evaluation, the corrected reanalysis data based on the hourly correction factors are used for the wind power model.
The data-driven and to hub height extrapolated GAM wind analysis fields were corrected, too. As previous experiments already showed that the hourly correction performs better than the correction based on one mean value, only hourly correction was carried out for the mentioned data set.
2.2.3. Wind to power
An empirical turbine power curve approach was used to evaluate the estimated power output of the wind parks based on wind velocities. In this study, exact location and height, as well as the characteristic turbine curve of each windmill, enter the calculation of the wind farm power output. Turbine power curves are based on constant air density, and density correction (see Ulazia et al., 2019) has been tested but did not improve the power model.
To exclude data from periods of icing or shut-downs caused by overload or maintenance, which distort wind power results, because wind turbines stand still and wind power output is zero despite of high wind speeds, data at time steps where power output was clearly lower than expected from measured wind speed was masked and excluded from the analysis. Unfortunately, this was only possible for two of the three wind farm sites, where both wind power and wind speed measurements were available, namely, BGL and CPLX-2.
The overall modeling approach allowed a good estimation of the power output, but it does not consider wake losses, other environmental losses, or losses introduced by electrical components of the turbines, yielding an overall positive bias of the evaluated power outputs (see Figure 5 in the following chapter).
2.3. Experiments
Here, the use cases of our study are introduced. For the overall evaluation of data sets and methods 2018 was considered as reference year for all sites, except CPLX-1, where data was only available from 10-2018 to 09-2019.
2.3.1. Wind speed
The evaluation of surface wind speed was carried out at 15 measurement stations chosen in close proximity to existing wind farms, namely, the 13 TAWES sites and three LWD sites (see Figure 2 for locations). These sites were not included in the generation of the GAM. In total, 15 experiments were carried out using raw reanalysis, corrected with GWA and NEWA correction factors, corrected hourly GWA and NEWA correction factors, plus NEWA, and the data-driven wind analysis GAM.
The results for the hub height wind speed performance of the three reanalysis models, the NEWA, and the upscaled GAM were carried out for in total 67 wind measurement sites installed at turbines distributed between four windfarms. Wind speed of wind farm CPLX-1 could not be evaluated, and here, only wind power evaluation was carried out.
2.3.2. Wind power
The study on wind power evaluation was conducted at five wind farm sites, namely, BGL (conglomeration of three wind farms), CPLX-1, and CPLX-2. Each of the wind farms consists of at least 10 wind turbines. Wind power was evaluated for each wind turbine—based on interpolated wind speed data—and then summed up to the wind power output of the entire wind farm. On the basis of the experiments carried out with respect to wind speeds, also the wind power evaluation was repeated 13 times with wind speed time series from ERA5, COSMO6, MERRA2, and GAM, as well as hourly corrected versions based on NEWA and GWA3 and the raw NEWA dataset.
3. Results
Here, the results of the use cases wind speed performance in two flat and one complex terrain setting and wind power performance at different hub heights and terrain complexities are presented. For validation, the standard industry metric bias, MAE, and RMSE, is used. Furthermore, we looked at correlation and other metrics (not shown in this study) to validate our results with respect to observations. The experiments, namely, the locations and time period, were selected based on: (i) data length provided by the complex terrain wind farm operators, (ii) overlap with the NEWA macroscale data, and (iii) for the complex terrain site the limitation that so far not many wind farms operate at altitudes above 1,500 m. The selection of the non-complex wind farms is based on previous experiences with those data sets. As for the observation and avalanche sites, the criteria were similar, and temporal availability of avalanche sites and a random selection of sites were left out for validation purposes for the GAM method. A more detailed introduction is given in Section 2.3 .
3.1. Wind speed
3.1.1. Wind speed comparison for wind sites
For hub heights, the model performances can be clearly separated into flat terrain and complex terrain. For the BGL sites (see Figure 3, top row), which are located in a rather flat terrain with only small hills in the direct vicinity, already the raw reanalysis data perform well, similar to the TAWES sites. Here, ERA5 performs best with an MAE of 1.34 m/s and an RMSE of 1.73 m/s. What can be seen here, too, is that for BGL the correction with either of the four factors does not improve the results indicating that for flat regions uncorrected models are preferred. Again, for the flat sites, NEWA performs worst, even worse than the extrapolated GAM or extrapolated and corrected GAM. Similar to the surface wind speed analysis, the NEWA shows a distinct diurnal cycle for the flat terrain sites (see Figure 3, top row purple line), similar to the findings by Jourdier (2020) and others. In addition, the other reanalysis data perform better than NEWA, with COSMO-REA6 showing a shallow diurnal cycle. Concluding, for the reanalysis data, a correction for the flat terrain turbines in BGL did not improve them. On the other hand, the extra data-driven GAM analysis, which exhibits a similar diurnal cycle as NEWA, could be improved using either of the correction methods with the NEWA-hourly correction, resulting in an overall better wind speed product. The behavior of MERRA-2 is similar to findings in other studies.
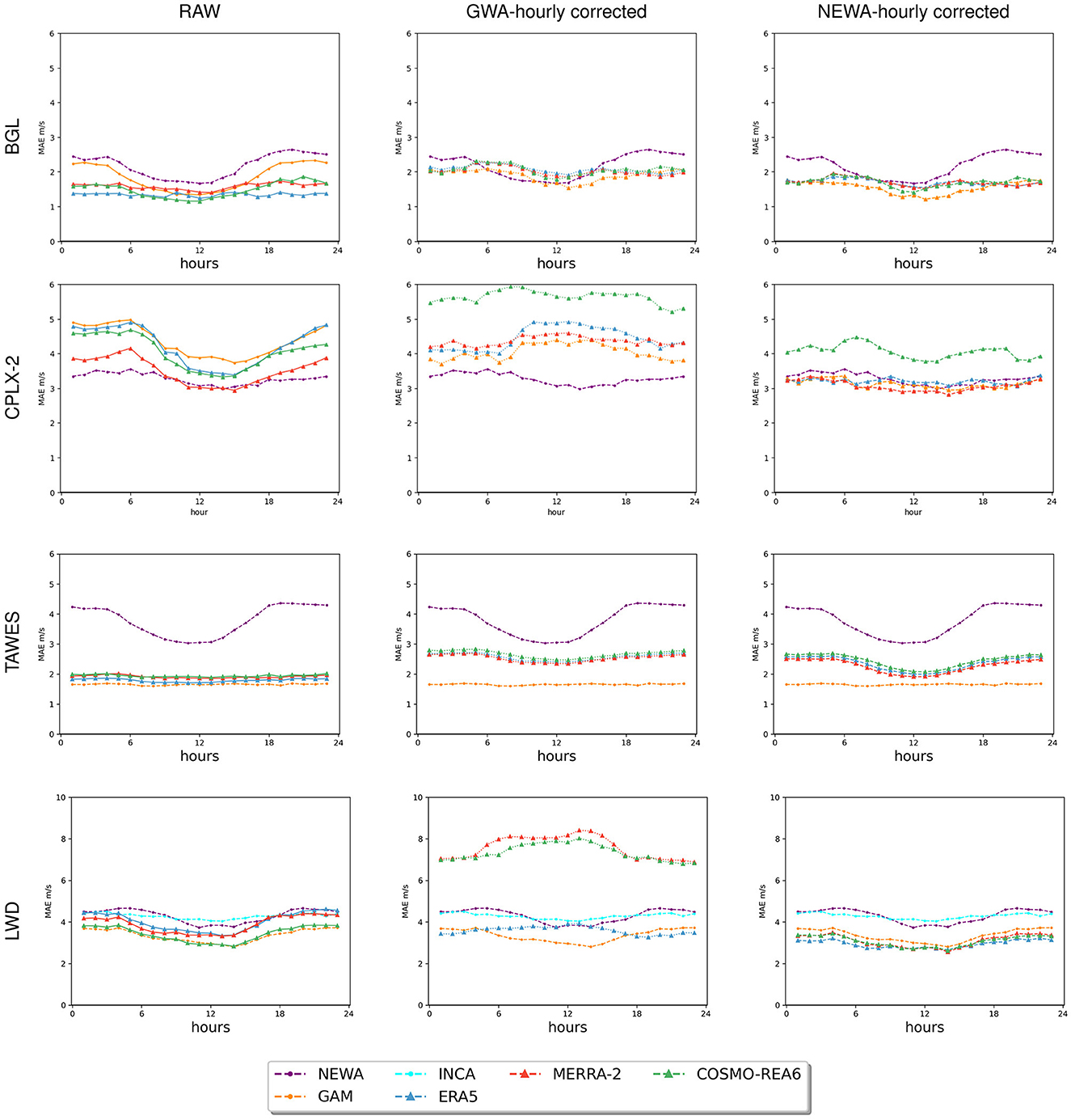
Figure 3. Mean absolute error [m/s] of the wind speed intercomparison for two of the three wind farm locations, BGL, which contains three wind farms very closely located to each other, and CPLX-2 (top two rows), for the standard meteorological observations sites TAWES (third row), and for the avalanche service sites (LWD). For the LWD sites also the mean absolute error of the INCA data set is shown for comparison (cyan line). Please note the different y-axis for LWD and the other three evaluations.
The results for turbines in CPLX-2 results show that in contrast to the flat terrain turbines the corrections using NEWA-hourly did improve the scores except for the COSMO-REA6 model. Here, MERRA-2 corrected with NEWA-hourly performs best with a mean absolute error of 3.07 m/s and a bias of −0.52 m/s. All reanalysis and analysis models show a rather distinct diurnal cylce. However, NEWA only exhibits small diurnal cycle. The uncorrected reanalysis data are outperformed by NEWA with a mean absolute error of 3.27 m/s, and not surprisingly, the raw GAM has the largest error for all uncorrected models with 4.36 m/s and a high bias of −4.08 m/s, indicating an underestimation of the wind speed. Correcting the GAM with NEWA-hourly resulted in a bias of −0.62 m/s and a mean absolute error of 3.13 m/s exhibiting better metrics than in the corrected ERA5 model. However, given that the extrapolation to hub height was rather simplistic, the results are still comparable to the reanalysis data. Most likely, adapting the extrapolation methodology and adding additional features to the interpolation method would result in an even better performance.
While this wind-speed analysis is limited by a comparatively small number of higher altitude surface and wind farm sites, the results are valuable as they present different aspects of surface and hub height wind speed, corrections, and reanalysis data skills. Overall, the differences between raw and the corrected data (Figure 4) show that at some sites, the corrections did improve the metrics while at other sites the correction had the opposite effects. NEWA overestimates wind speed, especially at the TAWES surface sites and the BGL wind farm located in flat terrain, whereas the reanalysis and GAM analysis underestimate wind speed. For the complex sites, the results of NEWA are good, and the correction also improves other reanalysis data. Thus, the overestimation in flat terrain produces comes along with a better representation of wind speed along ridges in more complex terrain. Although the NEWA results themselves do not show a good performance at some of the locations compared with other reanalysis data, using their climatic fields for a simple correction did improve the other reanalysis data. This might be due to two main reasons, one could be a balancing effect of under/overestimation and the other reason could be related to the fact that the selection of the target year, though predefined by the wind farm data, might not have been a good choice in terms of NEWA performance. The corrections with GWA-hourly did not improve the bias and turned a negative bias into, partially, strong bias. Corrections using NEWA resulted in a stronger overestimation of wind speed at sites that already exhibited overestimated wind speed without correction and resulted in a reduction of negative bias at the other sites.
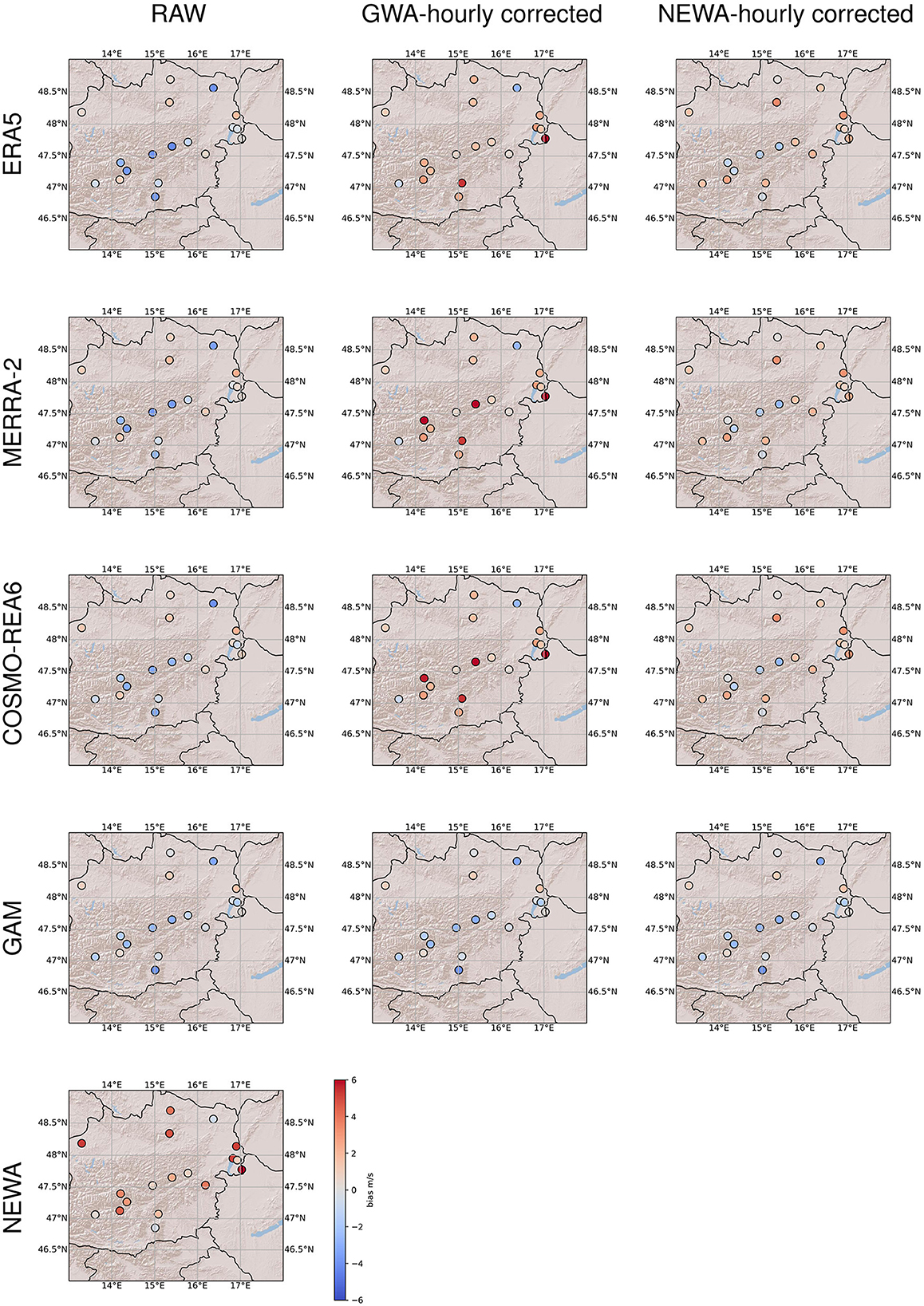
Figure 4. Overall bias [m/s] of surface and hub height wind speed for all locations and the corrections using the hourly correction factors (left column: raw, middle: GWA hourly corrected, right: NEWA hourly corrected). The four wind farm locations are each denoted by a single dot. Top row for ERA5, second row for MERRA-2, middle row for COSMO-REA6, and the two bottom rows for GAM and NEWA. Notably, GAM was only corrected for hub height wind speeds, thus differences might be hard to see.
3.1.2. Wind speed comparison for meteorological stations
The results of the diurnal cycle in Figure 3 (only raw, GWA-hourly corrected, and NEWA-hourly corrected shown), the two bottom rows, show that, unsurprisingly, the GAM (orange) performs best for the standard meteorological sites, TAWES, with an overall MAE of 1.65 m/s. However, also the raw reanalysis data (ERA5 blue, MERRA-2 red, and COSMO-REA6 green) perform well-given that the sites were chosen across several altitude ranges. Surprisingly, the NEWA (purple) performs worst with a mean absolute error of 3.78 m/s and an RMSE of 4.92 m/s for the surface wind speed and exhibits a strong diurnal cycle with the nocturnal mean absolute errors being worse than daytime mean absolute errors which are also found by Jourdier (2020). This might be related to the fact that the NEWA simulations had issues with the nocturnal boundary layer representation. Given the results of NEWA, an improvement in the raw reanalysis using NEWA climate for correction was not expected. However, the corrections using GWA did not improve performances but the opposite occurred. This might be related to the fact that although some sites were chosen in complex topographical areas (results not shown) where such a correction is able to improve due to the poor representation of topography in the reanalysis data set, the overall results are dominated by more flat region sites. In general, the ERA5 performed best for the TAWES sites and even outperforms the COSMO-REA6 (see Table 3 for more details). Using NEWA for, e.g., small, urban, or privately owned wind turbine site exploration, which are below 50 m above the ground, extrapolation of surface wind speed is not recommended. Here, distinct errors would be introduced.
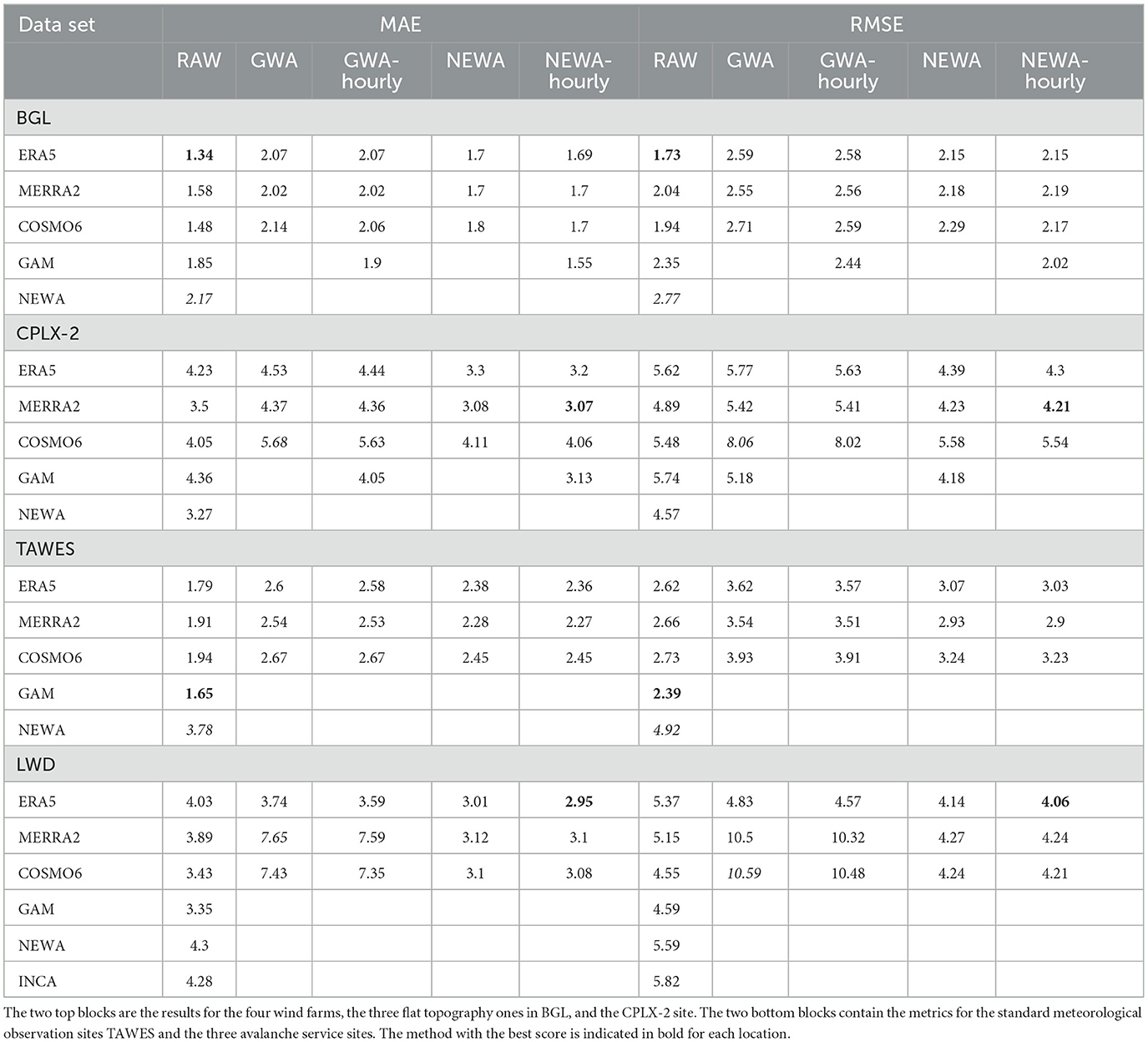
Table 3. Mean absolute error and RMSE of the raw, GWA corrected, and NEWA corrected reanalysis fields plus NEWA and GAM analysis metrics.
The evaluation results of the three non-standard meteorological sites of the avalanche service, which operate under similar harsh climatic conditions as the complex topographic wind farms, are mixed. Here, the corrections with an hourly cf using the NEWA climatological fields, the NEWA-hourly corrected data, perform better than the raw reanalysis data and even NEWA, as a bias of 3.03 m/s and a mean absolute error of 4.3 m/s. Without using a correction, the COSMO-REA6 exhibits a mean absolute error of 3.43 m/s and GAM of 3.35 m/s outperforms the other experiments. With the hourly NEWA correction, however, the bias of ERA5 could be reduced from −3.64 to 1.4 m/s (MAE from 4.03 m/s uncorrected to 2.95 m/s with hourly NEWA corrected). The results of the INCA analysis show that also INCA has a strong positive bias of 1.83 m/s and overestimates wind speed at those locations. Thus, even though the reanalysis has a rather coarse spatial resolution in contrast to the analysis data, using a climatological and hourly correction factor of NEWA was able to improve the results in terms of mean absolute error and RMSE. Here, it is beneficial for using the hourly correction factor of NEWA, in contrast to the standard meteorological sites, as it is able to decrease the strong negative bias.
In general, for both surface station types, the uncorrected reanalysis data have a negative bias which is turned into a strong positive bias when applying the corrections using the GWA.
3.2. Wind power
3.2.1. Validation of the power model
The quality of the wind power model itself could be estimated at two windfarms, where both wind speed measurements at hub height and wind power data were available. However, the wind power resulting from simulations based on measured wind speed (approach see Section 2.2.3) could be compared with real wind power data. As these data are available in a time resolution of 10 min, also the effects of using hourly averaged windspeeds could be investigated. Maintenance, grid availability, and environmental effects such as icing lead to shut downs of wind farms, when wind speeds would be suitable for wind power production. Therefore, wind power is only compared with time steps where measured wind power is above zero and measured wind speed is >3 m/s. The results of bias of the power calculation are shown in Figure 5. It is shown that the model accuracy decreases in the winter months in the alpine wind farm CPLEX- 2.
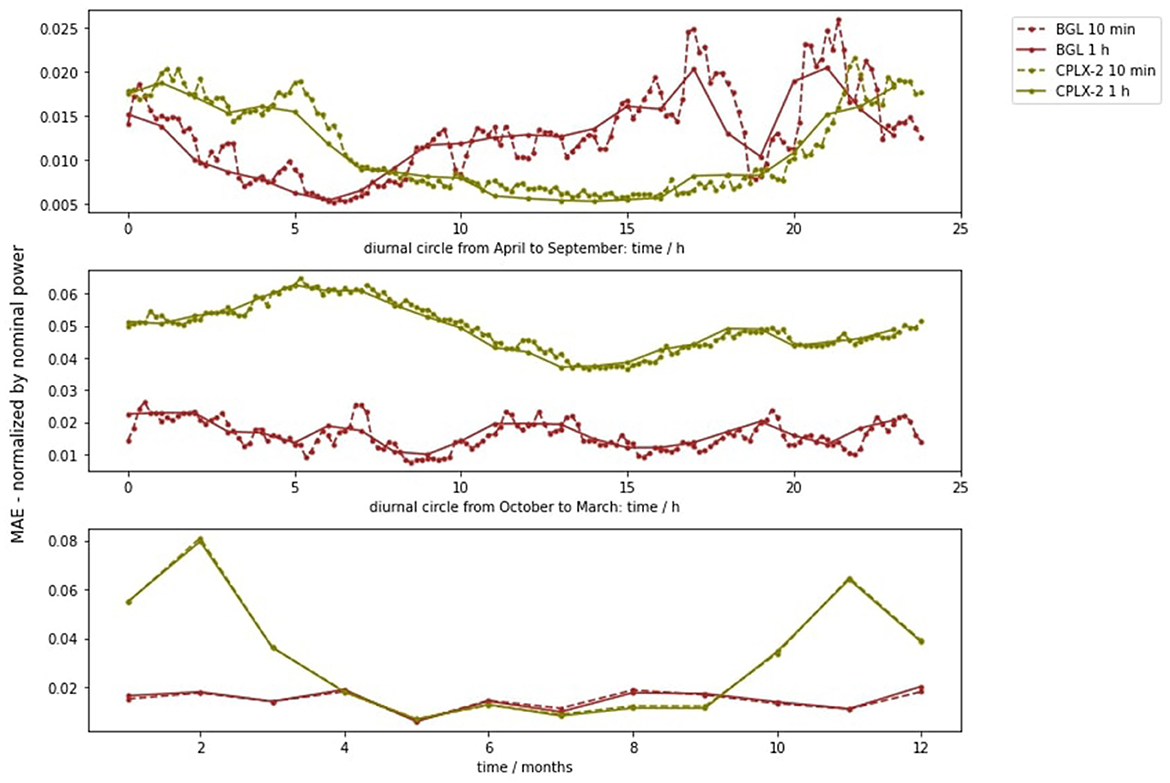
Figure 5. Mean absolute error of the wind power model (when compared with measured data) at each hour of the day from April to September (top), from October to March (middle), and at each month of the year (bottom)—evaluated at two wind farm sites: BGL (red) and CPLEX-2 (green) based on original 10 min resolution data (dotted line) as well as hourly averaged data (solid line).
The hourly simulations lead to a mean average error (MAE) of 1.5% (BGL) and 2% (CPLX-2), a bias of 0.1% (BGL) and 1% (CPLX-2), and a root mean square error of 5% (BGL) and 4% (CPLX-2) of the nominal power, respectively. The main take away from this comparison is that the wind power model itself introduces inaccuracy, especially in winter in the alpine regions and leads to an MAE in the range of ~1–2 % of the nominal power, and an RMSE of roughly 4–5% of the nominal power. These errors are related to the windpower model described in Section 2.2.3 and add up to uncertainties introduced by the use of wind speed input from analysis and reanalysis data sets (details in the previous Section 3.1), errors introduced by the vertical interpolation of these wind speed data, and a bias resulting from not considering wake loss effects.
3.2.2. Wind power comparison
Diurnal representations of wind power outputs are shown in Figure 6, according to the three wind farm sites and 13 experiments. Moreover, MAE is indicated in diurnal representation (Figure 8) and monthly representation (Figure 7). Scores of all experiments are summarized for all locations in Table 4. Evaluation of wind power is based on the model described in Section 2.2.3. The focus of this study is to investigate the credibility of different reanalysis and analysis data sets for wind power potential assessment. However, in addition to inaccuracy of those data sets, the power model itself introduces uncertainties as described in Section 3.2.1. Moreover, wake losses which are not captured at all by the wind power model lead to an overestimation of power production in all cases, justifying positive bias of compared results. Wake losses are strongly related to local conditions, and their assessment shows a large variability between different studies, but they make up roughly 5–15% of the total power production (Lee and Fields, 2021), which corresponds to a decrease of 0.01–0.06 in the capacity factor. In Figure 6, one can see, that the overestimation introduced by NEWA and hourly based corrections of GWA-3 is in the order of 0.1–0.25 of the capacity factor, and can therfore not be explained by the wake loss effect alone.
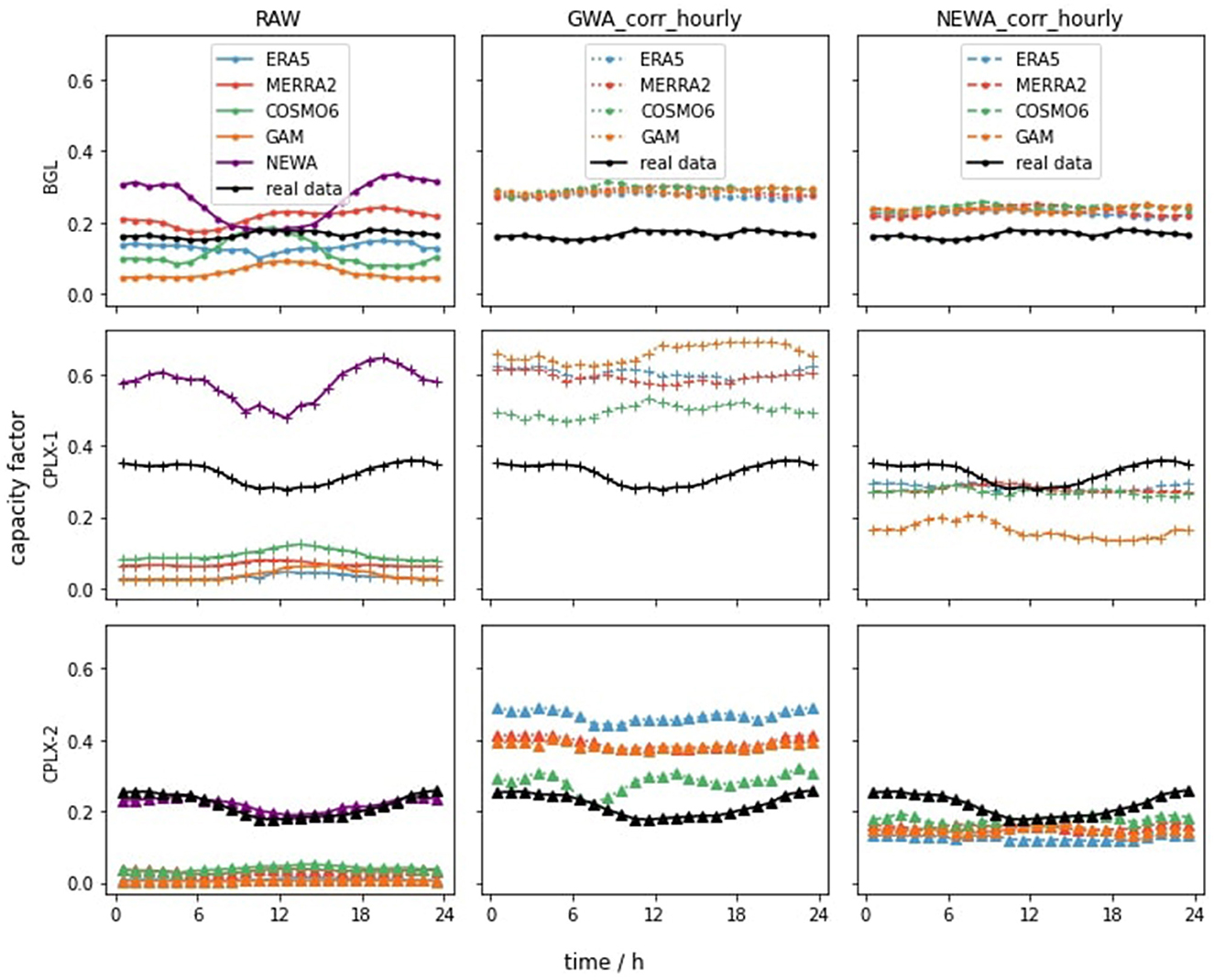
Figure 6. Diurnal presentation of hourly average wind power using different datasets (colors) and different correction methods (columns) at the three investigated locations (rows). The wind power is normalized by the nominal power of the respective wind farm. In other words, the diurnal variation of the capacity factor is shown.
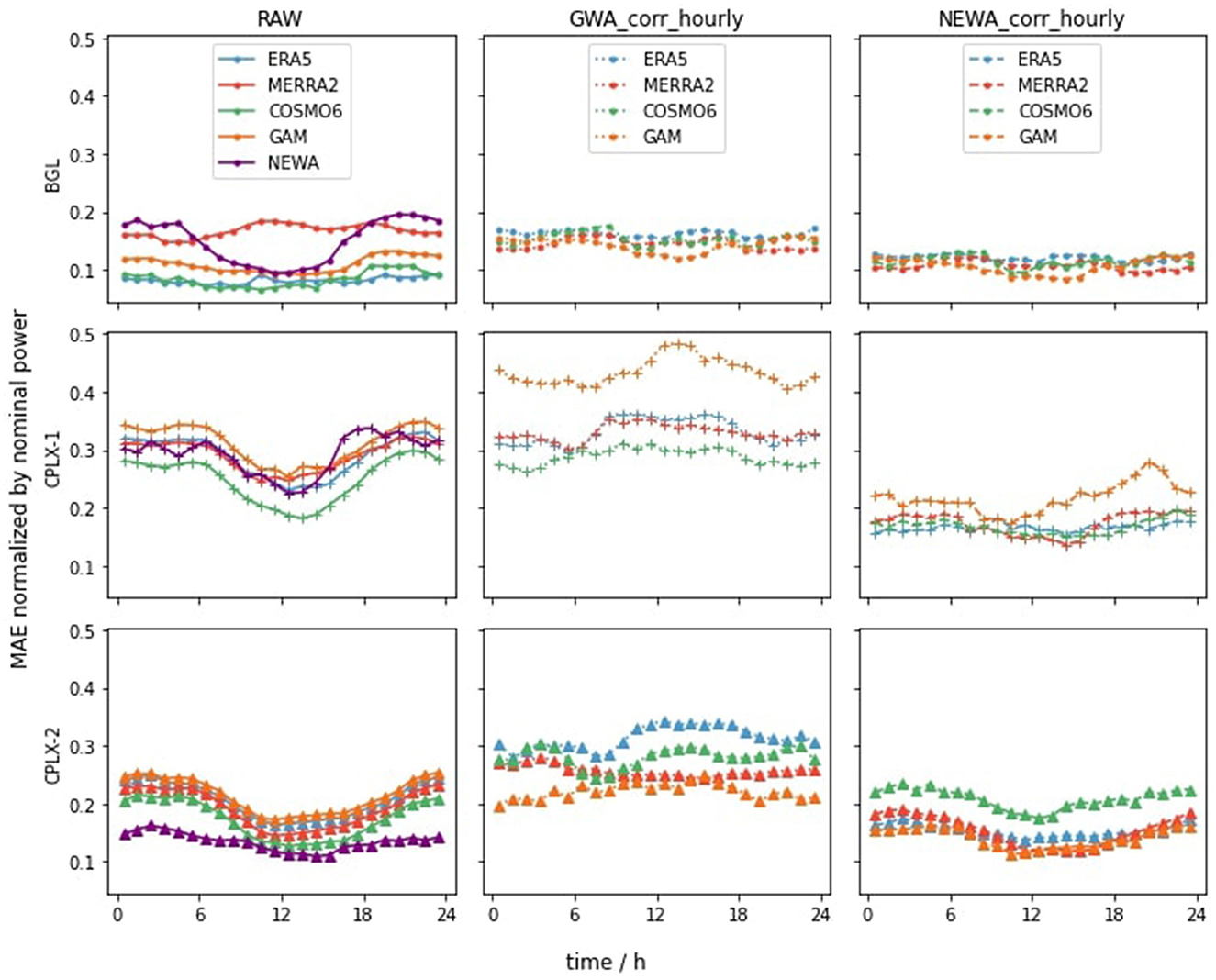
Figure 7. Diurnal presentation of the mean absolute error of the wind power using different datasets (colors) and different correction methods (columns) at the three investigated locations (rows). The mean absolute error is normalized by the nominal power of the respective wind farm.
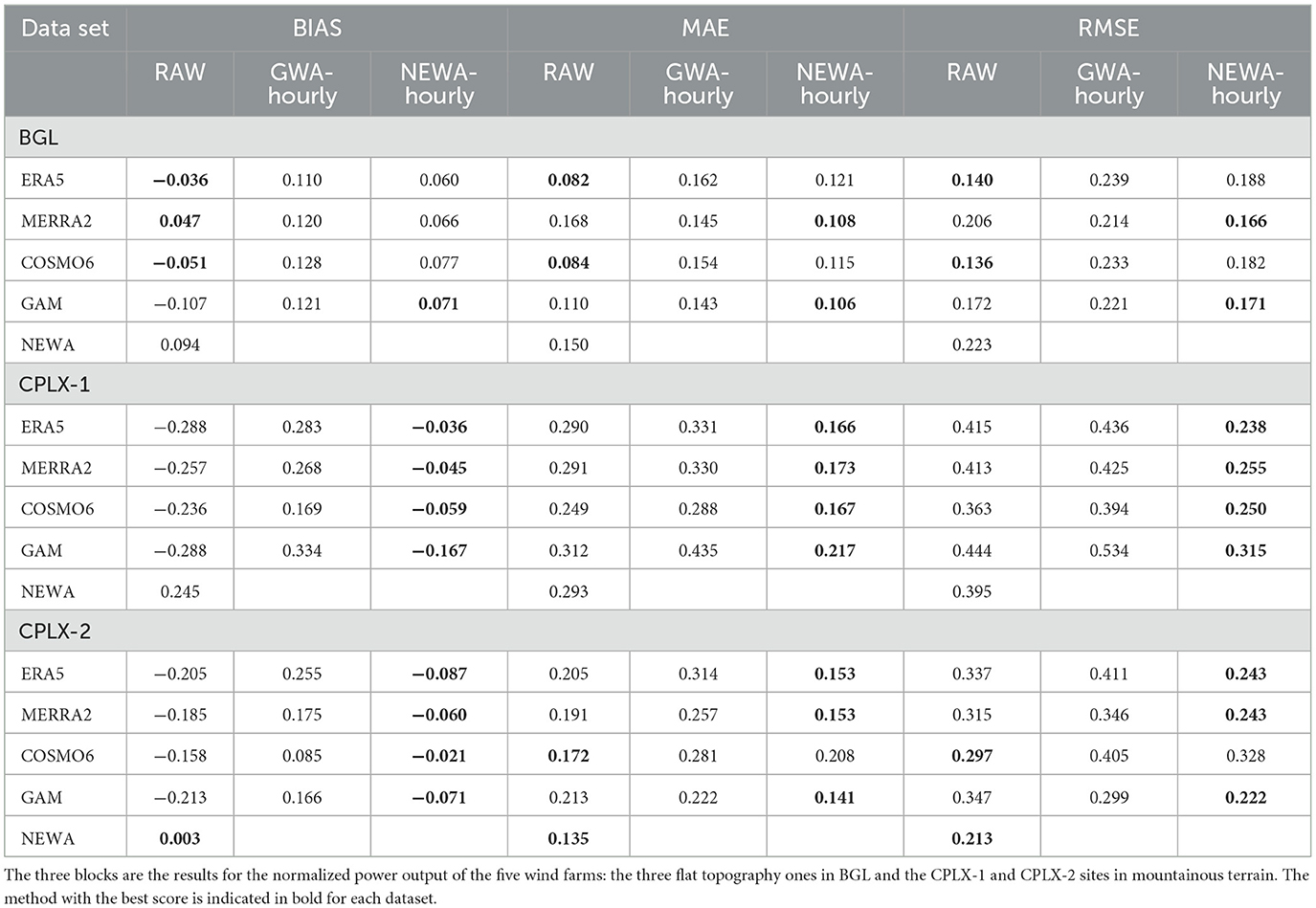
Table 4. Bias, mean absolute error, and RMSE of the raw, GWA corrected, and NEWA corrected reanalysis fields plus NEWA and GAM analysis metrics.
In comparison to diurnal circles of normalized wind power production (Figure 6), one can see that power evaluations based on hourly GWA3 corrections overestimate real data at all sites. The raw NEWA data set also overestimates the actual data in case of CPLX-1, but matches the actual data surprisingly well in case of CPLX-2. Raw reanalysis datasets, in contrast, tend to underestimate production, especially in complex terrain. This backs up the findings by Minola et al. (2020).
For the wind power sites in mountainous area, namely, CPLX-1 and CPLX-2 (see Figures 6, 7, second row and third row), the hourly corrected data based on NEWA clearly outperforms the raw reanalysis data. Despite of an obvious bias in the case of CPLX-1, the course of NEWA matches the course of the original data quite well, making it interesting for hourly based correction. In addition, the positive bias of NEWA is able to counteract to the negative bias of the raw reanalysis data sets. For CPLX-1, hourly correction of ERA5 based on NEWA shows the best performance with a bias of −0.04-, a mean absolute error of −0.17-, and an RMSE of 0.24 times the nominal power. For CPLX-2, already the raw NEWA data outperforms other datasets with a bias of 0.003-, an MAE of 0.14-, and an RMSE of 0.21 times the nominal power. In addition, hourly based correction based on NEWA improved RMSE and mean absolute error of GAM, MERRA2, and ERA5, as well as the bias of all considered data. Hourly correction based on GWA3 rarely improved the performance of raw data, in most cases it lead to poor results.
The ranking of reanalysis and analysis data sets is different when comparing the results from the wind speed analysis (Section 3.1) with the results from wind power analysis at the CPLX-2 site: raw NEWA and hourly NEWA-based correction of GAM perform slightly better than hourly NEWA-based corrections of MERRA2 and ERA5 when it comes to wind power. However, when comparing the analysis (Figure 3: 3rd graph in the second row, and Figure 7: 3rd graph in the third row) only small differences between the data sets occur, and a similar overall performance is reached for wind speeds and wind power, respectively.
In complex terrain, all datasets perform better in summer than in winter. This can be clearly seen in Figure 8, where the mean absolute error is higher in the winter months in the second (CPLX-1) row and third (CPLX-2) row, for nearly all datasets. This can only be partially explained by an increased bias of the wind power model in winter in complex terrain (see Figure 5).
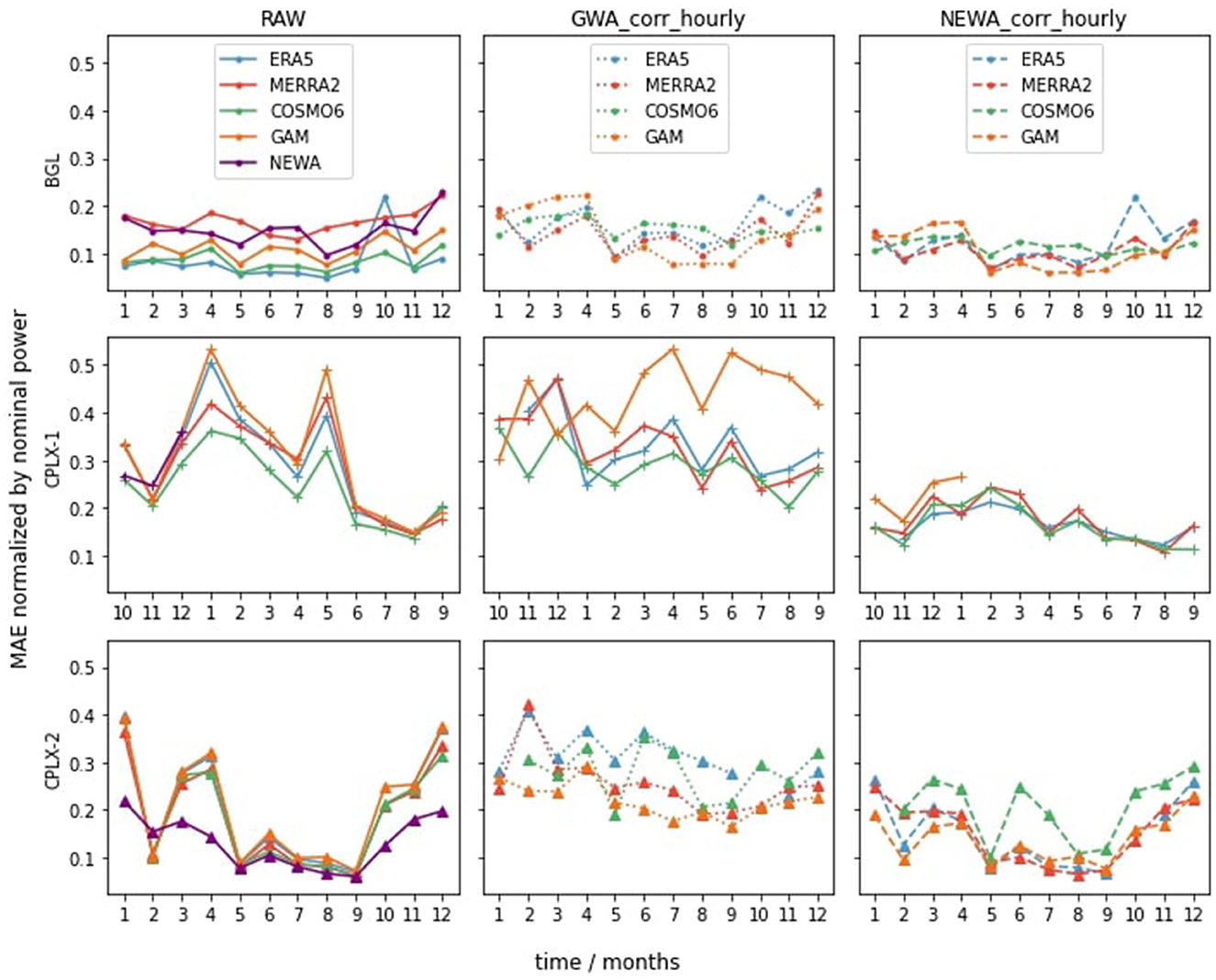
Figure 8. Monthly presentation of the mean absolute error of the wind power using different datasets (colors) and different correction methods (columns) at the three investigated locations (rows). The mean absolute error is normalized by the nominal power of the respective wind farm. Data availability restricted the analysis of CPLX-1 to the reference year 10-2018 to 09-2019, CPLX-2, and BGL were observed in 2018. There are data gaps of ERA5 in October 2018, of NEWA in 2019 and GAM apart from February 2019.
For the BGL sites (see Figures 6, 7, top row), which are located in a rather flat terrain with only small hills in the direct vicinity, the raw reanalysis data already performed well. This matches with the results obtained in the analysis of wind speeds in the previous chapter. Here, ERA5 performs best with an MAE of 0.08, a bias of −0.04, and an RMSE of 0.14 times the nominal power. However, a dip at 10:00 a.m. can be found in the diurnal variation (Figure 6, top row, first columns), which is reflected as top in the mean absolute error (Figure 7, top row, first columns). This is in line with the findings by Jourdier (2020) and can be explained with data gathering issues of ERA5. It can be observed that ERA5 performs slightly better in summer than in winter. This becomes clear when observing the upper left picture in Figure 8, where the MAE of raw ERA5-based power calculations (blue) is slightly lower in summer months. As before (Section 3.1), NEWA performs worst at the flat sites (BGL), even worse than the extrapolated GAM or extrapolated and corrected GAM. Hourly correction based on NEWA slightly improves the MAE and RMSE of MERRA2 and GAM at the BGL site. Moreover, as no wake effects are taken into account in the wind power simulation model, an overestimation, as introduced by the hourly correction based on NEWA, seems appropriate. However, in general, the results indicate that for flat regions, raw models perform roughly as well as the NEWA-corrected ones.
4. Conclusion
So far, Austrian wind power potentials in mountainous areas remain unused for various reasons. However, in the context of the european goal to decarbonize the electricity sector, wind power expansion in mountainous terrain in Austria will gain relevance. This makes prestudies indispensable, and the underlying data need to be used and interpreted with care.
In this study, three reanalysis data sets (ERA5, MERRA-2, and COSMO-REA6), one analysis data set (NEWA), and one data-driven surface wind speed analysis data set (GAM) were evaluated to answer the questions how good reanalysis and analysis data represent the surface and hub height wind speed and generated wind power in Austria in flat and complex topography, if the standard correction approaches are applicable to all sites. Overall, the best scores for wind power applications have been obtained with hourly based NEWA correction of ERA5 data, with bias ranging from −0.09 to 0.06, MAE ranging from 0.12 to 0.17, and RMSE ranging from 0.19 to 0.24. Although, the results showed that for flat regions, the raw reanalysis data outperform the NEWA. The extrapolated raw data-driven GAM is able to outperform NEWA with an MAE between 1.65 and 4.36/2.17 and 4.28 and an RMSE ranging from 2.35 and 5.74/2.77 to 5.82, respectively, for wind speed. This might be related to the chosen parameterizations in the underlying WRF model (Witha et al., 2019) and the nocturnal boundary layer representation in the numerical models. However, the results at the topographical complex sites show that NEWA is better than the raw models and the GWA-hourly corrected models, indicating that not always the classical and adapted-classical correction factor with GWA is beneficial. However, correction using the NEWA-hourly factor showed the added value of such a correction, except for the COSMO-REA6 data. Overall, the results of the reanalysis show an overestimation of wind speed in flat terrain and an underestimation of wind speed in more complex terrain. The results of this study are in accordance with other findings (see Jourdier, 2020, and references herein).
In general, one can conclude that the corrections of wind speed using the NEWA-hourly correction factor improved the overall metrics of the reanalysis and analysis data and are preferred over the GWA and GWA-hourly corrections. Furthermore, the results show that there is not one true model and data for all but a careful selection and processing of meteorological data is needed based on individual requirements and characteristics of the considered location. Especially, when generating synthetic data for extending an existing data set or generating data for site exploitation, caution and careful selection are needed. However, when aiming to use NEWA for statistical-machine learning-based downscaling of climate scenarios, the overestimation of wind speed in surface and hub height of NEWA should be accounted for.
Shortcomings of the proposed strategy are that, in general, for wind energy estimations in regions with complex terrain higher spatial resolutions are beneficial to account for slope and valley wind systems or turbulence, even a resolution of 1 km might be too coarse. In addition, observations at hub height or in mountainous regions are sparse; thus, verification is based solely on data provided by the wind farm operators and might be prone to systematic errors. Extrapolation of surface or reanalysis of 100 m wind speeds to hub height need to be done with care, and often, surface roughness can only be taken from land-use data sets and might not reproduce the conditions at the respective sites properly.
The goal of this study was to provide a first knowledge baseline toward generating a novel wind speed and wind power atlas at different hub heights for Austria with spatial resolution of 1 × 1 km and for an experimental region with sub-km resolution. Future studies could include the integration of wind direction sectors and surface roughness based on the CORINE land-use data to account for varying surface roughness in the extrapolation procedure or use machine learning methods for the extrapolation. In addition, monthly or seasonal hourly correction factors for correcting the coarse resolution reanalysis data could be considered. Another topic for future studies would include combining different reanalysis sources and adding the most recent ECMWF reanalysis data set CERRA (Schimanke et al., 2021) and using the CERRA forecast for the intermediate time steps between the 3-hourly analysis. In addition to these steps, one could either dynamically downscale ERA5 or CERRA to very high 1 km or sub-km scale resolutions and use these high resolution information for future applications. Moreover, the wind2power model can be improved by considering wake loss effects in the evaluation.
Data availability statement
The original contributions presented in the study are included in the article/supplementary material, further inquiries can be directed to the corresponding author.
Author contributions
IS and JG conceptualized the research and carried out the project administration. IS carried out the meteorological and reanalysis data retrieval, preparation, formal analysis, investigation, programming, validation, visualization, and writing of the manuscript. JG carried out the wind power data retrieval, preparation, formal analysis, investigation, programming, validation, visualization, and writing of the manuscript. MD prepared the data-driven wind analysis data set and contributed to result discussion and writing of the manuscript. TN discussed and revised the study. All authors contributed to the article and approved the submitted version.
Funding
Part of the resources for this study was funded within the Austrian Climate and Research Programme (ACRP) under the project name MEDEA (KR19AC0K17614), to further research on renewable energy and meteorologically induced extreme events. Another part of the resources as well as the publication fees was funded within the Programme IKT der Zukunft/AI for Green vom Bundesministerium für Klimaschutz, Umwelt, Energie, Mobilität, Innovation und Technologie (BMK) under the project name AI4wind (FO999892230), to investigate the suitability of reanalysis data sets for wind power modeling.
Acknowledgments
The authors would like to acknowledge valuable discussions with colleagues at both institutions as well as the two funding schemes which brought them together. In addition, the authors want to thank the developers of wind power lib (Haas et al., 2021) for their valuable open source implementations and the ImWind Gruppe and wind power operators of the consortium of the AI4wind project for providing valuable wind power data.
Conflict of interest
JG and TN were employed by 4ward Energy Research, GmbH.
The remaining authors declare that the research was conducted in the absence of any commercial or financial relationships that could be construed as a potential conflict of interest.
Publisher's note
All claims expressed in this article are solely those of the authors and do not necessarily represent those of their affiliated organizations, or those of the publisher, the editors and the reviewers. Any product that may be evaluated in this article, or claim that may be made by its manufacturer, is not guaranteed or endorsed by the publisher.
References
Akhtar, N., Geyer, B., Rockel, B., Sommer, P., and Schrum, C. (2021). Accelerating deployment of offshore wind energy alter wind climate and reduce future power generation potentials. Sci. Rep. 11:11826. doi: 10.1038/s41598-021-91283-3
Bailey, B. H., McDonald, S. L., Bernadett, D. W., Markus, M. J., and Elsholz, K. V. (1997). Wind Resource Assessment Handbook: Fundamentals for Conducting a Successful Monitoring Program. Technical report, National Renewable Energy Lab, Golden, CO. doi: 10.2172/486127
Bloomfield, H., Brayshaw, D., Deakin, M., and Greenwood, D. (2022). Hourly historical and near-future weather and climate variables for energy system modelling. Earth Syst. Sci. Data 14, 2749–2766. doi: 10.5194/essd-2021-436
Bloomfield, H. C., Brayshaw, D. J., Shaffrey, L. C., Coker, P. J., and Thornton, H. E. (2016). Quantifying the increasing sensitivity of power systems to climate variability. Environ. Res. Lett. 11:124025. doi: 10.1088/1748-9326/11/12/124025
Bollmeyer, C., Keller, J. D., Ohlwein, C., Wahl, S., Crewell, S., Friederichs, P., et al. (2015). Towards a high-resolution regional reanalysis for the European cordex domain. Q. J. R. Meteorol. Soc. 141, 1–15. doi: 10.1002/qj.2486
Cai, Y., and Bréon, F.-M. (2021). Wind power potential and intermittency issues in the context of climate change. Energy Convers. Manage. 240:114276. doi: 10.1016/j.enconman.2021.114276
Camargo, L. R., Gruber, K., and Nitsch, F. (2019). Assessing variables of regional reanalysis data sets relevant for modelling small-scale renewable energy systems. Renew. Energy 133, 1468–1478. doi: 10.1016/j.renene.2018.09.015
Dabernig, M., Mayr, G. J., Messner, J. W., and Zeileis, A. (2017). Spatial ensemble post-processing with standardized anomalies. Q. J. R. Meteorol. Soc. 143, 909–916. doi: 10.1002/qj.2975
Davidson, M. R., and Millstein, D. (2022). Limitations of reanalysis data for wind power applications. Wind Energy 25, 1646–1653. doi: 10.1002/we.2759
Davis, N., Badger, J., Hahmann, A. N., Hansen, B. O., Olsen, B. T., Mortensen, N. G., et al. (2023). The Global Wind Atlas: A high-resolution dataset of climatologies and associated web-based application. Bull. Amer. Meteor. Soc. doi: 10.1175/BAMS-D-21-0075.1
European Commission (2020). Communication From the Commission to the European Parliament, the Council, the European Economic and Social Committee and the Committee of the Regions - Stepping Up europe's 2030 Climate Ambition. European Commission.
Gelaro, R., McCarty, W., Suárez, M. J., Todling, R., Molod, A., Takacs, L., et al. (2017). The modern-era retrospective analysis for research and applications, version 2 (MERRA-2). J. Clim. 30, 5419–5454. doi: 10.1175/JCLI-D-16-0758.1
González-Aparicio, I., Monforti, F., Volker, P., Zucker, A., Careri, F., Huld, T., and Badger, J. (2017). Simulating European wind power generation applying statistical downscaling to reanalysis data. Appl. Energy 199, 155–168. doi: 10.1016/j.apenergy.2017.04.066
Gruber, K., Klöckl, C., Regner, P., Baumgartner, J., and Schmidt, J. (2019). Assessing the Global Wind Atlas and local measurements for bias correction of wind power generation simulated from MERRA-2 in Brazil. Energy 189:116212. doi: 10.1016/j.energy.2019.116212
Gruber, K., Regner, P., Wehrle, S., Zeyringer, M., and Schmidt, J. (2022). Towards global validation of wind power simulations: a multi-country assessment of wind power simulation from MERRA-2 and ERA-5 reanalyses bias-corrected with the global wind atlas. Energy 238:121520. doi: 10.1016/j.energy.2021.121520
Gualtieri, G. (2019). A comprehensive review on wind resource extrapolation models applied in wind energy. Renew. Sustain. Energy Rev. 102, 215–233. doi: 10.1016/j.rser.2018.12.015
Haas, S., Krien, U., Schachler, B., Bot, S., Zeli, V., Shivam, K., et al. (2021). Wind-python/windpowerlib: silent improvements.
Hahmann, A. N., Sīle, T., Witha, B., Davis, N. N., Dörenkämper, M., Ezber, Y., et al. (2020). The making of the new European wind atlas-part 1: model sensitivity. Geosci. Model Dev. 13, 5053–5078. doi: 10.5194/gmd-13-5053-2020
Haiden, T., Kann, A., Wittmann, C., Pistotnik, G., Bica, B., and Gruber, C. (2011). The integrated nowcasting through comprehensive analysis (INCA) system and its validation over the eastern alpine region. Weath. Forecast. 26, 166–183. doi: 10.1175/2010WAF2222451.1
Harper, M., Anderson, B., James, P., and Bahaj, A. (2019). Assessing socially acceptable locations for onshore wind energy using a GIS-MCDA approach. Int. J. Low-Carbon Technol. 14, 160–169. doi: 10.1093/ijlct/ctz006
Hersbach, H., Bell, B., Berrisford, P., Hirahara, S., Horányi, A., Muñoz-Sabater, J., et al. (2020). The ERA5 global reanalysis. Q. J. R. Meteorol. Soc. 146, 1999–2049. doi: 10.1002/qj.3803
Höltinger, S., Salak, B., Schauppenlehner, T., Scherhaufer, P., and Schmidt, J. (2016). Austria's wind energy enAustria's participatory modeling approach to assess socio-political and market acceptance. Energy Policy 98, 49–61. doi: 10.1016/j.enpol.2016.08.010
International Renewable Energy Agency (2020). 10 Years Progress to Action. International Renewable Energy Agency.
Jäger, T., McKenna, R., and Fichtner, W. (2016). The feasible onshore wind energy potential in Baden-Württemberg: a bottom-up methodology considering socio-economic constraints. Renew. Energy 96, 662–675. doi: 10.1016/j.renene.2016.05.013
Jourdier, B. (2020). Evaluation of ERA5, MERRA-2, COSMO-REA6, NEWA and AROME to simulate wind power production over France. Adv. Sci. Res. 17, 63–77. doi: 10.5194/asr-17-63-2020
Kelledy, E. (1982). “Wind resource evaluation in Ireland,” in Energy for Rural and Island Communities, ed J. Twidell (Pergamon Press), 117–120. doi: 10.1016/B978-0-08-027606-9.50016-7
Koivisto, M., Plakas, K., Hurtado Ellmann, E. R., Davis, N., and Sørensen, P. (2021). Application of microscale wind and detailed wind power plant data in large-scale wind generation simulations. Electr. Power Syst. Res. 190:106638. doi: 10.1016/j.epsr.2020.106638
Lackner, M. A., Rogers, A. L., and Manwell, J. F. (2008). Uncertainty analysis in MCP-based wind resource assessment and energy production estimation. J. Solar Energy Eng. 130:031006. doi: 10.1115/1.2931499
Lee, J. C. Y., and Fields, M. J. (2021). An overview of wind-energy-production prediction bias, losses, and uncertainties. Wind Energy Sci. 6, 311–365. doi: 10.5194/wes-6-311-2021
Ma, X., Li, Y., and Li, Z. (2022). The projection of Canadian wind energy potential in future scenarios using a convection-permitting regional climate model. Energy Rep. 8, 7176–7187. doi: 10.1016/j.egyr.2022.05.122
McKenna, R., Pfenninger, S., Heinrichs, H., Schmidt, J., Staffell, I., Bauer, C., et al. (2022). High-resolution large-scale onshore wind energy assessments: a review of potential definitions, methodologies and future research needs. Renew. Energy 182, 659–684. doi: 10.1016/j.renene.2021.10.027
Millstein, D., Bolinger, M., and Wiser, R. (2022). What can surface wind observations tell us about interannual variation in wind energy output? Wind Energy 25, 1142–1150. doi: 10.1002/we.2717
Minola, L., Zhang, F., Azorin-Molina, C., Pirooz, A., Flay, R., Hersbach, H., et al. (2020). Near-surface mean and gust wind speeds in ERA5 across Sweden: towards an improved gust parametrization. Clim. Dyn. 55, 887–907. doi: 10.1007/s00382-020-05302-6
Molina, M. O., Gutiérrez, C., and Sánchez, E. (2021). Comparison of ERA5 surface wind speed climatologies over Europe with observations from the HADISD dataset. Int. J. Climatol. 41, 4864–4878. doi: 10.1002/joc.7103
Murcia, J. P., Koivisto, M. J., Luzia, G., Olsen, B. T., Hahmann, A. N., Sørensen, P. E., et al. (2022). Validation of European-scale simulated wind speed and wind generation time series. Appl. Energy 305:117794. doi: 10.1016/j.apenergy.2021.117794
Olauson, J. (2018). ERA5: the new champion of wind power modelling? Renew. Energy 126, 322–331. doi: 10.1016/j.renene.2018.03.056
Petrik, R., Geyer, B., and Rockel, B. (2021). On the diurnal cycle and variability of winds in the lower planetary boundary layer: evaluation of regional reanalyses and hindcasts. Tellus A 73, 1–28. doi: 10.1080/16000870.2020.1804294
Ramon, J., Lledó, L., Pérez-Zanón, N., Soret, A., and Doblas-Reyes, F. J. (2020). The tall tower dataset: a unique initiative to boost wind energy research. Earth Syst. Sci. Data 12, 429–439. doi: 10.5194/essd-12-429-2020
Ramon, J., Lledó, L., Torralba, V., Soret, A., and Doblas-Reyes, F. J. (2019). What global reanalysis best represents near-surface winds? Q. J. R. Meteorol. Soc. 145, 3236–3251. doi: 10.1002/qj.3616
Schallenberg-Rodriguez, J. (2013). A methodological review to estimate techno-economical wind energy production. Renew. Sustain. Energy Rev. 21, 272–287. doi: 10.1016/j.rser.2012.12.032
Schimanke, S., Ridal, M., Le Moigne, P., Berggren, L., Undén, P., Randriamampianina, R., et al. (2021). CERRA Sub-Daily Regional Reanalysis Data for Europe on Single Levels From 1984 to Present. Copernicus Climate Change Service (C3S) Climate Data Store (CDS). doi: 10.24381/cds.622a565a
Sharp, R. (2015). Spatiotemporal Disaggregation of GB Scenarios Depicting Increased Wind Capacity and Electrified Heat Demand in Dwellings, Vol. 38. International Association for Energy Economics (IAEE). Available online at: http://www.iaee15.org/ (accessed June, 2022).
Silva, V., López-Botet Zulueta, M., Wang, Y., Fourment, P., Hinchliffe, T., Burtin, A., et al. (2018). “Anticipating some of the challenges and solutions for 60% renewable energy sources in the european electricity system,” in Renewable Energy: Forecasting and Risk Management, Vol. 254, eds P. Drobinski, M. Mougeot, D. Picard, R. Plougonven, and P. Tankov (Cham: Springer International Publishing), 169–184. doi: 10.1007/978-3-319-99052-1_9
Singh, S., Bhatti, T. S., and Kothari, D. P. (2006). A review of wind-resource-assessment technology. J. Energy Eng. 132, 8–14. doi: 10.1061/(ASCE)0733-9402(2006)132:1(8)
Staffell, I., and Pfenninger, S. (2016). Using bias-corrected reanalysis to simulate current and future wind power output. Energy 114, 1224–1239. doi: 10.1016/j.energy.2016.08.068
Troen, I., and Lundtang Petersen, E. (1989). European Wind Atlas. Risø National Laboratory. Available online at: https://backend.orbit.dtu.dk/ws/portalfiles/portal/112135732/European_Wind_Atlas.pdf (accessed June 2, 2022).
Truhetz, H., Gobiet, A., and Kirchengast, G. (2007). Evaluation of a dynamic-diagnostic modeling approach to generate highly resolved wind climatologies in the alpine region. Meteorol. Zeitschrift. 16, 191–201. doi: 10.1127/0941-2948/2007/0192
Ulazia, A., Ibarra-Berastegi, G., Sáenz, J., Carreno-Madinabeitia, S., and González-Rojí, S. J. (2019). Seasonal correction of offshore wind energy potential due to air density: case of the Iberian peninsula. Sustainability 11:3648. doi: 10.3390/su11133648
van der Wiel, K., Bloomfield, H., Lee, R., Stoop, L., Blackport, R., Screen, J., and Selten, F. (2019). The influence of weather regimes on European renewable energy production and demand. Environ. Res. Lett. 14:094010. doi: 10.1088/1748-9326/ab38d3
Wahl, S., Bollmeyer, C., Crewell, S., Figura, C., Friederichs, P., Hense, A., et al. (2017). A novel convective-scale regional reanalysis COSMO-REA2: improving the representation of precipitation. Meteorol. Zeitsch. 26, 345–361. doi: 10.1127/metz/2017/0824
Widén, J., Carpman, N., Castellucci, V., Lingfors, D., Olauson, J., Remouit, F., et al. (2015). Variability assessment and forecasting of renewables: a review for solar, wind, wave and tidal resources. Renew. Sustain. Energy Rev. 44, 356–375. doi: 10.1016/j.rser.2014.12.019
Witha, B., Hahmann, A., Sile, T., Dörenkämper, M., Ezber, Y., García-Bustamante, E., et al. (2019). WRF model sensitivity studies and specifications for the NEWA mesoscale wind atlas production runs.
Yang, B., Zhong, L., Wang, J., Shu, H., Zhang, X., Yu, T., et al. (2021). State-of-the-art one-stop handbook on wind forecasting technologies: an overview of classifications, methodologies, and analysis. J. Clean. Prod. 283:124628. doi: 10.1016/j.jclepro.2020.124628
Keywords: wind power simulation, reanalysis, data-driven analysis, spatio-temporal-analysis, representativeness
Citation: Schicker I, Ganglbauer J, Dabernig M and Nacht T (2023) Wind power estimation on local scale—A case study of representativeness of reanalysis data and data-driven analysis. Front. Clim. 5:1017774. doi: 10.3389/fclim.2023.1017774
Received: 12 August 2022; Accepted: 13 July 2023;
Published: 09 August 2023.
Edited by:
Matthew Collins, University of Exeter, United KingdomReviewed by:
ZhaoYang Dong, Nanyang Technological University, SingaporeJingbo Wang, University of Liverpool, United Kingdom
Copyright © 2023 Schicker, Ganglbauer, Dabernig and Nacht. This is an open-access article distributed under the terms of the Creative Commons Attribution License (CC BY). The use, distribution or reproduction in other forums is permitted, provided the original author(s) and the copyright owner(s) are credited and that the original publication in this journal is cited, in accordance with accepted academic practice. No use, distribution or reproduction is permitted which does not comply with these terms.
*Correspondence: Irene Schicker, aXJlbmUuc2NoaWNrZXJAZ2Vvc3BoZXJlLmF0