Atmospheric drivers affect crop yields in Mozambique
- Center of Plant Sciences, Scuola Universitaria Superiore Sant'Anna, Pisa, Italy
Climate change has been inducing variations in the statistics of both the large-scale weather patterns and the local weather in many regions of the world, and these variations have been affecting several human activities, including agriculture. In this study, we look at the links between large-scale weather patterns and local weather as well as agriculture, with a specific regional focus on Mozambique between 1981 and 2019. First, we investigated linear trends and links between large-scale weather patterns and local weather in the region using the ERA5 dataset. We used the same data to investigate how climate change has been affecting the statistics of large-scale weather patterns. Then, we derived Mozambique country-level cereal yield data from FAO and linked it up with climate and weather data to assess what is the relationship between large-scale patterns and local agronomic outputs using a multiple linear regression (MLR) model with crop yield as the response variable and climate drivers as predictors. The results indicate that in Mozambique, the crop season warmed substantially and consistently with climate change-induced global warming, and the rainy season had become drier and shorter, with precipitation concentrated in fewer, more intense events. These changes in the local weather have been linked to variations in the statistics of large-scale weather patterns that characterize the (large-scale) atmospheric flow over the region. Our results indicate a negative impact on yield associated with climate change, with average yield losses of 20% for rice and 8% for maize over the analyzed period (1981–2019). This negative impact suggests that, at the country scale, further future warming during the growing season may offset some of the cereal yield gains from technological advances.
1. Introduction
Climate variability, in particular the frequency and intensity of extreme weather conditions, has a profound impact on agricultural systems. Understanding how climate variability affects agricultural systems is a necessary step to be able to assess the resilience of agricultural systems to changing climate conditions, to issue valuable predictions, and thus to be able to identify adequate and effective adaptation measures (Moore and Lobell, 2014).
According to the International Panel on Climate Change (IPCC) Sixth Assessment Report (AR6), climate warming is unequivocal, and it is the result of anthropogenic activities. Changes in the land biosphere since 1970 are consistent with global warming; climate zones have shifted, and crop-growing seasons are changed in both hemispheres. Warming has resulted in an increased frequency, intensity, and duration of heat-related events, including heatwaves in most land regions, and in an increase in the intensity of heavy precipitation events at a global scale (IPCC, 2021).
The impact of climate change on crops depends on agricultural systems: in particular, in large parts of the lower latitude regions, yields of key crops including maize and wheat are affected negatively by observed climate shifts; the same crops, in some parts of the higher-latitude regions, may see positive yield changes (IPCC, 2019). Large-scale atmospheric weather patterns have a prominent role in driving the year-to-year variability in crop production by influencing the local weather, including the surface parameters that most affect crop production, such as rainfall, temperature, humidity, and wind. Quantifying yield loss anomalies and understanding their climatic drivers are a prerequisite to assessing vulnerabilities, developing prediction systems, and designing adaptation measures to increase the resilience of food systems.
In sub-Saharan Africa (SSA), ~95% of the farming systems are rainfed (Calzadilla et al., 2013), and half of the cultivated land can be classified as a dry land ecosystem (Cervigni and Morris, 2016). Since spatial and temporal rainfall patterns and rainfall amounts are highly variable, and temperature and evapotranspiration stresses are high, water deficit can have very large negative impacts on crop growth and development (Testa et al., 2016). If we look into the future, the warming in the SSA continent is projected to be greater than the global average, with a projected increased average temperature of 3–4°C in 2100 over the historical average (Thompson et al., 2010). This, in combination with low adaptive capacity in connection to the acute levels of poverty and the limited facilities needed to mitigate and/or adapt to the changing climate, makes the SSA region very vulnerable to the climate crisis (Ringler et al., 2010; Ramirez and Thornton, 2015). Crop-climate models indicate that maize (−5 %), sorghum (−14.5 %), and millet (−9.6 %) yields are set to decline significantly in future climates in the SSA (Knox et al., 2012). Mozambique is among the African countries that is most exposed to climate-related risks (Mavume et al., 2021). Its agricultural production is characterized by moderately low productivity of a wide selection of staple crops, a diverse variety of annual and perennial horticultural products, and cash crops (Silva and Matyas, 2014). Rainfed agriculture, which is practiced by smallholder farmers, accounts for most of the cropped area, a feature common to other SSA countries. Maize and rice are the most widely grown crops in Mozambique, and as of 2019, they account for more than 90% of the total cereal production (FAOSTAT, 2021).
In SSA smallholder agriculture, crop yields are highly dependent on local weather. Local weather conditions influence crop growth and development by affecting the availability of water and the day and night temperature, resulting in a differential agronomic output that also depends on agronomic practices and types of crops and varieties (Karl et al., 1999). Hence, farmers rely on the knowledge of climate patterns and, when available, the weather forecast to determine which crops to cultivate and which management options to choose.
Local weather conditions are affected by large-scale weather patterns, and thus, changes in the statistics can impact local weather and crop yield. Climate change affects the statistics of large-scale weather patterns, making some patterns more/less frequent, and/or more/less intense; climate change can also impact the structure of the large-scale patterns. Since predicting large-scale weather patterns is, in general, easier than predicting local weather (van Oldenborgh and Burgers, 2005; Buizza and Leutbecher, 2015; Salinger et al., 2020; Buizza, 2021), there is value in characterizing the relation existing between large-scale weather patterns and the crop yield (Ceglar et al., 2016, 2017; Zhao et al., 2017).
In this study, we focus on Mozambique as a case study to investigate whether (a) in general, variations in the crop yield can be linked to variations in local weather and then (b) whether these variations can be linked to variations in the statistics of large-scale weather patterns due to climate change. If this was the case, then if we can predict how climate warming will affect the variations in the large-scale weather patterns, we can also predict the variations in the local weather and their perspective impact on the crop yield. Our results show that cereal yields in Mozambique decreased due to warming and that if this trend continues, it may hamper yield gains from technological development.
2. Data and methods
2.1. Crop yield and climate data
Crop yield in Mozambique is measured by the country's average annual yield for rice, maize, and cereals. Maize and rice are widely grown crops in Mozambique. Based on the 2019 statistics from FAOSTAT (https://www.fao.org/faostat/en/#data/QCL), the two crops make up the large majority of the total cereal production. Country-level annual crop production and harvested area data were obtained from the Food and Agriculture Organization (FAO) of the United Nations (FAOSTAT, 2021). Country-level cereal yield values were calculated from total production and harvested area. Data from 1981 to 2019 have been used in this study.
To identify the large-scale weather patterns, the mean sea level pressure (MSLP) and 850 mb level meridional (u850) and zonal (v850) wind were obtained from ERA5, the fifth generation of the ECMWF reanalysis for the global climate and weather for the past 4 to 7 decades (Hersbach et al., 2020). To analyze the local weather conditions, the daily gridded weather dataset from the AgERA5 dataset was used. AgERA5 is a dataset designed to make the ERA5 data available in an easy-to-use format to users in the agricultural sector. AgERA5 variables used in this study are maximum air temperature, minimum air temperature, rainfall, and vapor pressure, obtained from the European Copernicus Program (AgERA5, 2021).
2.2. Methods of analysis
Crop yields often show an increasing trend over time, which can be attributed to technological improvements such as the introduction of new varieties or enhanced crop management. Therefore, to properly identify the effects of climate on crop yield, impact from other factors such as technological improvements must be removed. A time trend analysis using a linear regression model is one of the most common methods used to detect non-weather-related impacts on yields over time. A simple linear regression model has been applied to de-trend the crop yield time series (Lu et al., 2017).
The crop growing season summary of rainfall, average temperature, and vapor pressure deficit are computed to describe the local climate in Mozambique. Local weather is driven by large-scale weather patterns. Large-scale weather patterns are weather developments over a large area, which do not significantly change over several days. The weather itself can change during a large-scale weather pattern, but the main characteristics of the respective regional weather remain the same. The regional differentiation and the typical sequence of large-scale weather patterns define the climate of a region. Large-scale weather patterns may include synoptic-scale pressure systems and wind patterns such as monsoon systems and convergent and divergent regions. Mozambique's rainfall climatology is determined by seasonal changes in such large-scale weather patterns (see Supplementary Figure 2), part of which involves a series of variations:
❖Southward migration of the Inter-Tropical Convergence Zone (ITCZ).
❖Variation in the strength of Angola Low (AL).
❖Changes in the Mozambique Channel Trough (MCT), characterized by a low-pressure area over the central and southern Mozambique Channel.
❖Variations in position and intensity of the Mascarene High (MH) pressure systems and perturbations in the associated southern hemisphere mid-latitude westerly circulation.
The MSLP and the 850 mb meridional and zonal wind speed were used to describe the large-scale weather patterns. Within the area of interest (latitude from 40°S to 40°N and longitude from 5°E to 80°E), a Pearson product moment correlation analysis was used to explore the relationship between variations in the large-scale weather patterns and in the country-level crop yields. The target region within large-scale atmospheric circulation fields that drives Mozambique's interannual yield variation was determined by computing the simultaneous correlation with respect to fields of de-trended crop yields and November-to-March average MSLP, 850 hPa meridional winds, and zonal winds. Over the geographic area of interest, regions of significant influence, defined as the ones with an anomaly correlation coefficient >20% with a 90% confidence level (i.e., p < 0.1), were identified from the point-to-field correlation map. Points with a correlation lower than 20% have been masked.
The role of large-scale drivers in recent yield trends was investigated following the method described by Lobell and Field (2007), Lobell et al. (2011) and Iizumi et al. (2018). We applied a multiple linear regression model with crop yield as the response variable and climate drivers (large-scale weather patterns and local climate) as the predictor variables. Symbolically, we have assessed the existence of a linear relationship between the weather variables and yield:
While the existence of a linear relationship cannot attribute directions of causality, as in Kukal et al.'s study (Kukal and Irmak, 2018), we can confidently assume that climate variations may cause yield changes and not vice versa. A multiple linear regression (MLR) model was used to estimate the role of climate in recent yield progress. Assuming that the observed trend in the climate fields is attributed to the anthropogenic effect (due to climate change), two yield estimations were performed using the MLR model: one using the actual observed climate fields (Yfactual) as predictors and a second one using the de-trended climate fields (Ycounterfactual) as predictors. Then, the climate impact on the crop yield was estimated by comparing the factual and counterfactual yield estimations:
where the suffices “t” and “c” indicate the year and crop type, ΔY is the estimated yield impact, Yfactual is the estimated yield under the factual climate condition, Ycounterfactual is the estimated yield under the counterfactual climate condition, and Yfactual, 1981:2019 is the estimated average yield over the historical period estimated using factual climate data.
This approach was followed to identify the proportion of changes in yields due to climate trends relative to the historical average yield and to assess whether the climate impact on yield was positive or negative. While an MLR model does not attempt to capture details of plant physiology or crop management, they do capture the net effect of the entire range of processes by which climate affects yields, including the effects of poorly modeled processes. Thus, such estimates of climate impacts can be viewed as an upper bound on the impacts of recent trends (Lobell and Field, 2007; McLachlan et al., 2020).
3. Result and discussion
3.1. Rainfall trends during the cropping season
The rainfall season in Mozambique lasts from October to May (see Supplementary Figure 1 for a map showing monthly rainfall climatology). The rainfed cropping season for major cereals relies on the months with the highest rainfall intensity and typically covers November to April or May, depending on the crop.
Monthly rainfall totals show a decreasing trend since 1981 in critical moments of the cropping seasons, particularly in November (planting month) and February (reproductive stage for the cereals) (Figure 1). The decreasing trend of the November rainfall totals is especially critical as it may cause an erratic onset of the rainy season over time, which creates unfavorable conditions for sowing during the planting window. The results also indicate that the January rainfall totals have been increasing significantly during the last 40 years (Figure 1). In addition, throughout Mozambique, very heavy rain days have been increasing during January, whereas light-to-medium rain days have been decreasing (see Supplementary Figure 4). Thus, in summary, observed trends indicate that the rainy season has been drier and has been shortening, with an increased frequency of very intense rainy days in January.
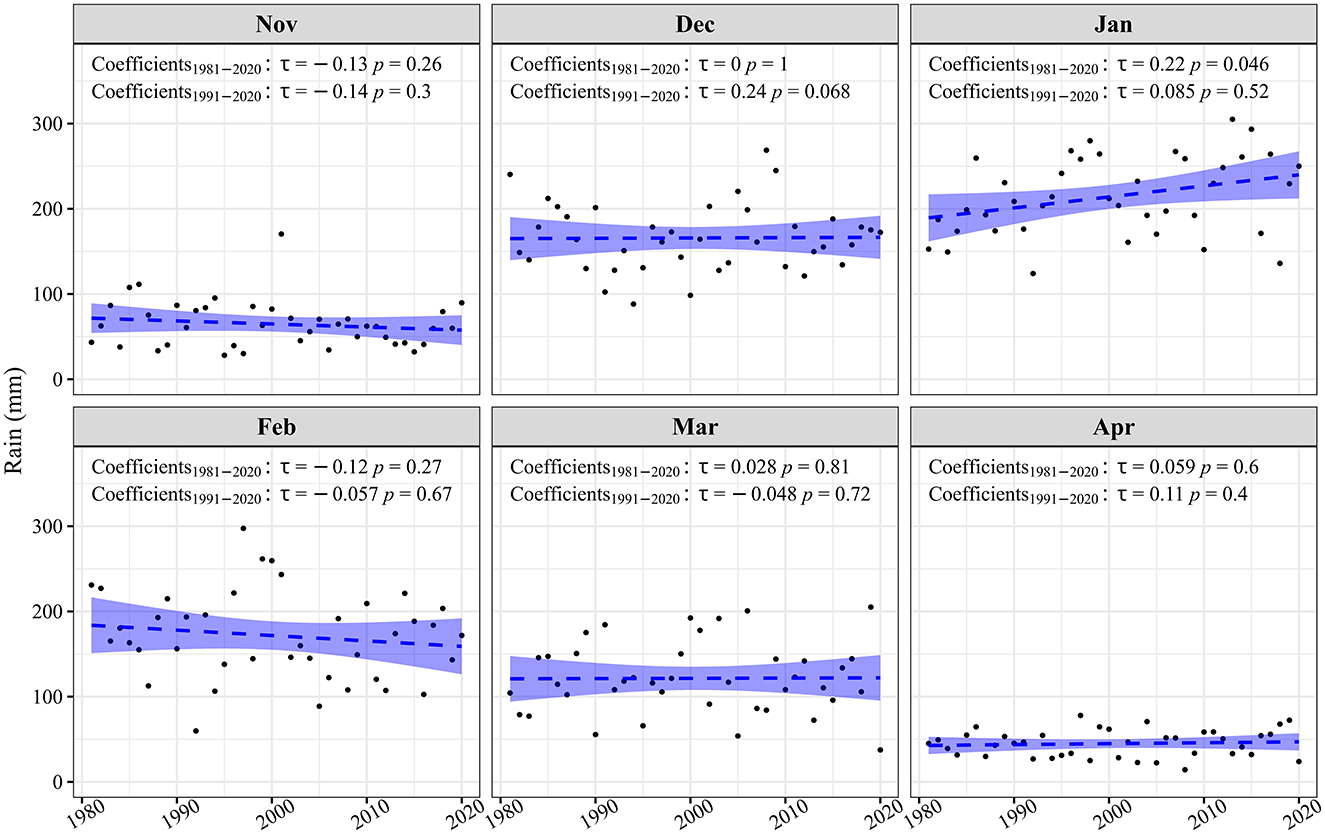
Figure 1. Linear trend (broken lines) of Mozambique averaged monthly rainfall totals (on the Y-axis) from 1981 to 2020. The blue shade represents the 95% confidence region. The correlation coefficients Kendall tau (τ) and the p-value (p) for the 1981–2020 and 1991–2020 periods are given at the top of each panel.
3.2. Warming of the cropping season
The temperature anomaly during the November to March season across the past 40 years shows a clear warming trend, which becomes more evident after 2002 (Figure 2). It is also worth noticing a quasi-decadal variability in the growing season temperature that can be detected in the 5-year centered running average (black line in Figure 2: for each year Y, the line shows the mean between Y-2 and Y+2 years), with lower relative values in 1989, 2003, and 2012. The trend analyses conducted between 1981 and 2020 have indicated an increase in the seasonal average temperature of 1.9°C per decade in Mozambique. This is higher than the global average, which is approximately 1.5°C since the pre-industrial period (Allen et al., 2018). The scientific community agrees on continuous warming in the years to come. Mavume et al. (2021) projected that mean annual temperatures in Mozambique are likely to increase by 1.0–1.5°C from the historical by the 2040s and between 1.5 and 4°C by the end of the 21st century. The projected rise in temperatures and the associated rate of warming will increase evapotranspiration and result in more frequent and intense extreme heat events (INGC, 2009). Higher temperatures during the cropping season can have dramatic impacts on productivity, farm incomes, and food security (Battisti and Naylor, 2009).
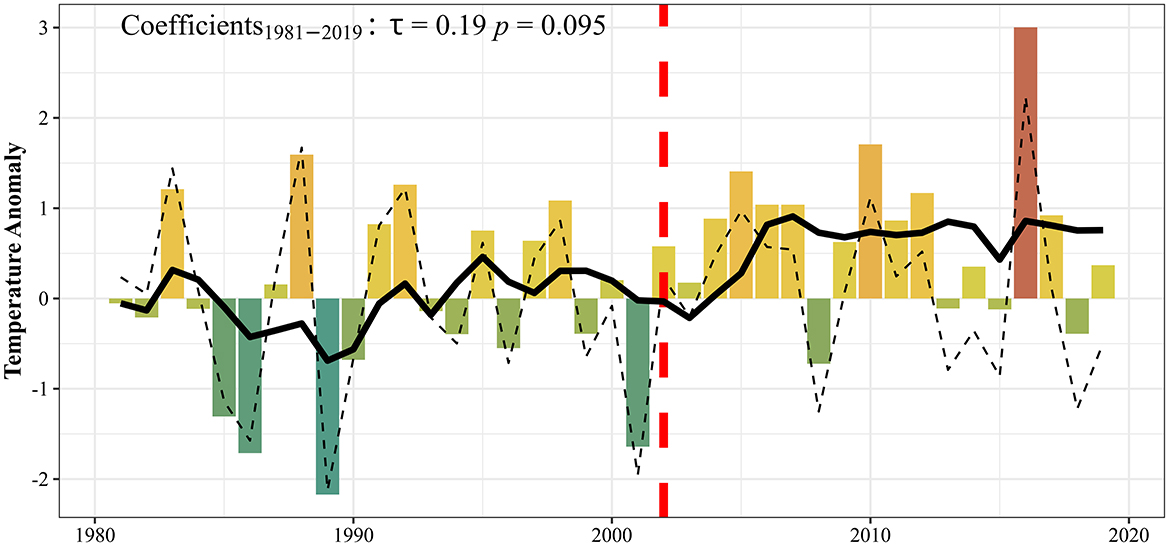
Figure 2. Standardized daily average temperature anomaly relative to 1981–2000: average over November to March. The black line shows the 5-year running mean. The broken black lines show the de-trended temperature anomaly. The bold broken red line marks the value for 2001.
3.3. The link between large-scale climatic drivers, local weather, and crop yield
Large-scale climatic drivers relevant to the wet season in Mozambique are most prominent from November to March, whereas they start to weaken in April and May when the dry season system takes over (Supplementary Figure 2). We identified the drivers in this window looking at the simultaneous correlation between de-trended fields of MSLP and 850mb meridional and zonal wind and country-level yield. The correlation map between country-level yield and atmospheric variables (Figure 3) illustrates the links between large-scale climatic drivers and yield. There is a negative MSLP signal in the northern Indian Ocean including the Arabian Peninsula (10°N to 30°N, 50°E to 70°E; MSLP index), an area dominated by the Arabian high-pressure system which intensifies in strength and extent during November to March. There is a positive meridional wind signal over the southern part of Africa (10°S to 18°S, 10°E to 20°E; u850-index). This region is dominated by the Angola low which intensifies during this period (Crétat et al., 2019; Barimalala et al., 2020). There is a distinct negative surface zonal wind correlation in the region located south of Madagascar (30°S to 38°S, 23°E to 37E; v850-index).
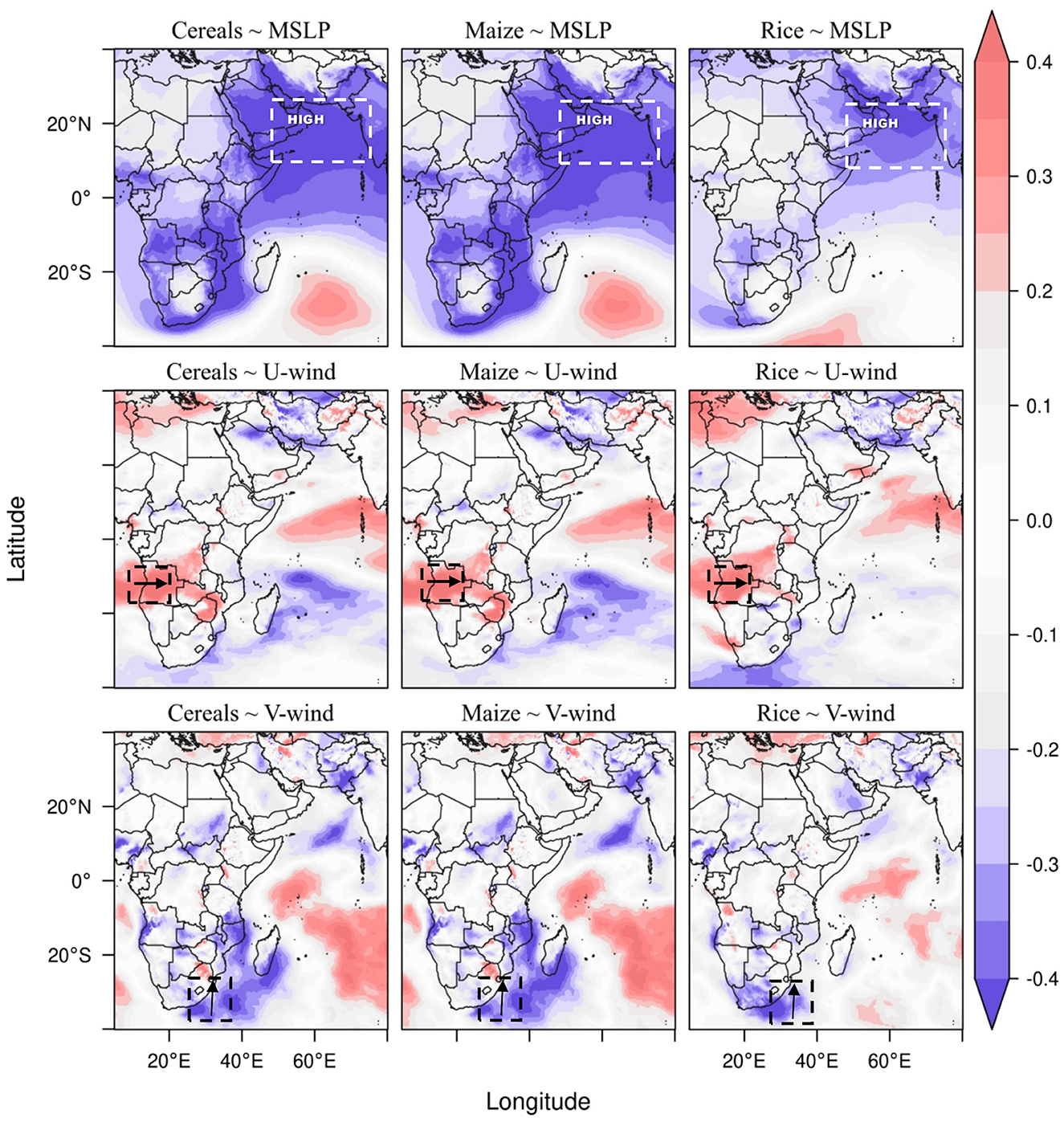
Figure 3. Correlation (positive shades of red and negative shades of blue) of continuous Mozambique yield anomalies: (first column) cereals, (second column) maize, and (third column) rice, with simultaneous synoptic-scale meteorological drivers: (first row) MSLP, (second row) U-wind @850 hPa, (third row) V-wind @850 hPa. Target area and key features are highlighted.
The country-level climate indices (Rain, Tavg, and VPD), together with identified atmospheric indices (MSLP index, u850-index, and v850-index) from the area of influence, which drive the Mozambique cereal yield, were used to describe the influences of large-scale atmospheric drivers on local weather that affect year-to-year variations of country-level yield progress.
There is a strong association between large-scale weather patterns and the local climate that influences crop yield (see Supplementary Figure 5). An intensification of the high-pressure system over the Arabian subcontinent (as indicated, e.g., by the MSLP index) leads to a reduction in the growing season rainfall and an increased vapor pressure deficit in Mozambique, which induce a lower crop yield due to the reduced moisture availability during the growing season (Figure 4A). At times of stronger meridional winds over the southern Africa region, especially around the west coast of Angola (as indicated by the u850-index), Mozambique seasonal rainfall totals are enhanced and vapor pressure deficit (moisture demand) is reduced, increasing cereal yields. Weaker zonal wind over the south of Madagascar (v850-index), which enhances growing season temperature and the associated vapor pressure deficit, may result in reduced cereal yields (Figure 4).
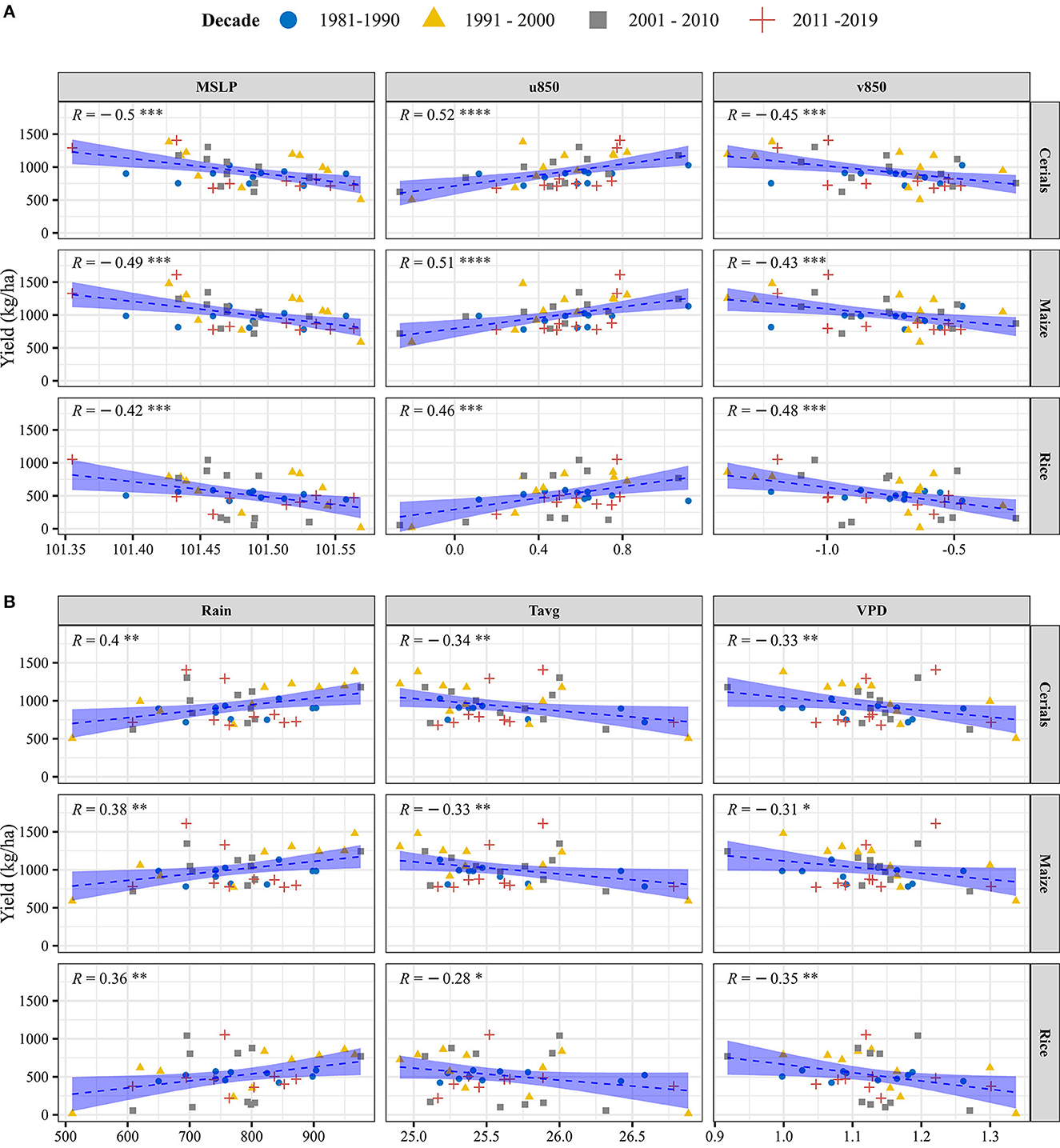
Figure 4. Relationship between crop yield (kg ha−1) and climatic drivers. (A) Crop yield ~ large-scale drivers; (B) crop yield ~ local climate. Each decade is shown with a different color and shape, indicating that the relationships do not appear to change through time. Significance level: *P < 0.1, **P < 0.05, ***P < 0.01, ****P < 0.001.
3.4. Impacts of climate on crop yield
Yields of total cereals and grain maize increased in Mozambique in the last 40 years (see Supplementary Figure 3). In the same period, rice yields show a decreasing trend although yield values between 1981 and 1986 and from 2015 onward show some leveling off. We explored the potential influence of large-scale weather patterns on local climate, in turn affecting crop production, using an MLR model. The vapor pressure deficit (VPD) parameter was left out from the MLR models due to co-linearity with average temperature which may inflate the variance factor (Supplementary Table 1). At least 48% (cereals), 46% (maize), and 43% (rice) of the variance in year-to-year yield changes were explained by the MLR models.
These inferred impacts reflect the influences of large-scale climatic drivers that were captured by the MLR models. Negative yield impacts since 2002 indicate that recent climate trends have suppressed Mozambique's cereal yield progress and should be addressed through adaptation measures. The estimated average yield impacts in the historical period (1981–2019) due to historical climate change, relative to a counterfactual climate condition, were observed to be negative for all major crops (Figure 5). Rice is more affected by climate change than maize. The MLR model estimates average yield loss of 20% for rice, 8% for maize, and 9% for major cereals over the historical period.
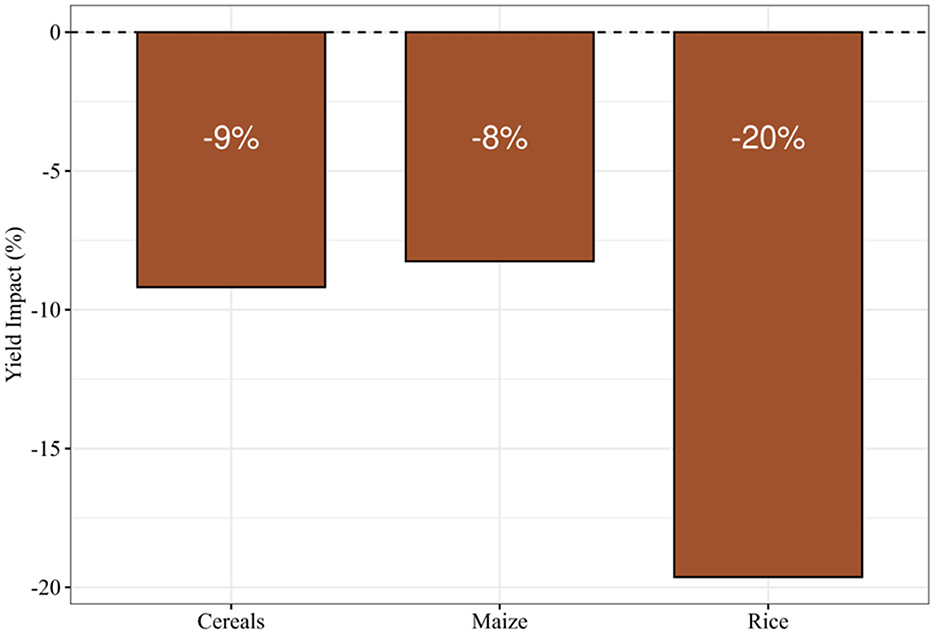
Figure 5. Estimated impacts of large-scale climate drivers on average yields for 1981–2019. Positive values indicate that the climate has increased the yields, and negative values indicate that climate has decreased the yields relative to what would have occurred without the anthropogenic effect.
This result confirms the impact of anthropogenic climate change on crop yields, by linking the influence of changes in the variability of the large-scale weather patterns on the local weather variability, affecting crop production. The results also indicate that the increased warming during the growing season affects water availability and thus crop production. Lesk et al. (2021) discussed that rising air temperature is a leading risk to global crop production and emphasized the critical role of moisture availability in regulating crop responses to heat and the importance of temperature–moisture couplings. Despite the underlying uncertainty of climate projections in regard to the precise amount of warming, the expected rise in temperature is likely to increase the adverse impacts on cereal production and impact the food supply in Mozambique.
4. Conclusion
Using weather data from ERA5 and AgERA5 and crop yield data from FAOSTAT, we have investigated how trends in rainfall and temperature during the crop growing season have been affecting crop yields in Mozambique.
We found that rainfall totals during November and February have been decreasing since 1981, while rainfall totals during January have been increasing. In other words, the rainfall season has been becoming drier and shorter, with more extreme precipitation events concentrated in January. The seasonal average temperature of the crop season has also been warming significantly from 1981 to 2020, with the warming being more pronounced after 2002. We observed that interannual yield anomalies in Mozambique could be linked to variations in the large-scale systems that control the regional climate with quasi-periodic fluctuations. The results indicate that recent large-scale climate trends, attributed to human activity, have a negative impact on the country's yield progress. On average, since 1981, the yield of maize and rice was reduced by 20% and 8% due to climate change alone.
These findings could likely be generalized to other regions of Southern Africa. More detailed, local data would reinforce our results and allow a finer discussion of the effects of climate on crop yield. For this study, we could use only access country-level crop production data, and this did not allow us to account for the variation of cropping practices across different locations in different agro-ecologies as well as for the separation of irrigated and rainfed cropping areas. Further research may benefit from disaggregated crop production to explore localized impacts of climate variability. Another opportunity to further improve our results would be to investigate in detail the impact of climate change on the frequency and intensity of extreme weather conditions, such as floods, storms, cyclones, and droughts, and their impact on crop yields. Currently, there is limited availability of data on the occurrence of natural disasters. Finally, we could not consider shifts of cropping areas and changes in agricultural policies that could have affected cultural cropping practices at a large scale. Those changes may introduce an artificial temporary trend to crop production progress and bias the regression estimator used to de-trend yield to remove non-climatic factors. However, our factual vs. counterfactual approach allowed us to attribute a decrease in yield to climate change regardless of these background factors. Following our results, we can confidently state that the historical large-scale climate drivers and cereal yield relationships indicate that, at the country scale, warming from 2001 has very likely offset some of the cereal yield gains from technological advances.
These results may be relevant also in a prediction framework; since predicting large-scale weather patterns is easier than predicting the local weather. In addition, understanding how climate change could affect the statistics of the large-scale weather patterns would contribute to a better early warning system centered on yield forecasts at longer timescales. The impacts of anthropogenic warming on agriculture can be offset by innovating management practices and leveraging the capacity for local adaptation of crop varieties (Nobre et al., 2019; Gomez-Zavaglia et al., 2020; Henry, 2020). Adaptation strategies for the agriculture sector in areas affected by climate change, such as Mozambique, could be developed by combining studies similar to this one, assessing how climate variability has been affecting crop yields, with studies that identify which crop varieties and management practices can adapt better to the ongoing and projected climates. The existing linkage between large-scale weather patterns, local climate, and crop yields examined and described in this study can also be a basis for the development of crop production monitoring and prediction systems capable of providing indication and early warning on the performance of crop production season and the expected yield before harvest, thus supporting food security in the wake of the climate crisis.
Data availability statement
The original contributions presented in the study are included in the article/Supplementary material, further inquiries can be directed to the corresponding author/s.
Author contributions
RT, RB, and MD conceived and designed the study. RT performed the analysis and drafted the manuscript and the figures. All authors contributed to revisions, discussion of results, and approved the submitted manuscript.
Funding
This work was supported by the EU H2020 Focus Africa Project GA 869575 and the Doctoral Programme in Agrobiodiveristy at Scuola Superiore Sant'Anna.
Conflict of interest
The authors declare that the research was conducted in the absence of any commercial or financial relationships that could be construed as a potential conflict of interest.
Publisher's note
All claims expressed in this article are solely those of the authors and do not necessarily represent those of their affiliated organizations, or those of the publisher, the editors and the reviewers. Any product that may be evaluated in this article, or claim that may be made by its manufacturer, is not guaranteed or endorsed by the publisher.
Supplementary material
The Supplementary Material for this article can be found online at: https://www.frontiersin.org/articles/10.3389/fclim.2023.1214703/full#supplementary-material
References
AgERA5 (2021). “Copernicus Climate Change Service (C3S), Fifth generation of ECMWF atmospheric reanalysis of the global climate for agriculture and ago-ecological studies,” in Copernicus Climate Change Service Climate Data Store (CDS), July-2021.
Allen, M. R., Dube, O. P., Solecki, W., Aragón-Durand, F., Cramer, W., et al. (2018). “Framing and Context,” in Global Warming of 1.5°C. An IPCC Special Report on the Impacts of Global Warming of 1.5°C Above Pre-Industrial Levels and Related Global Greenhouse Gas Emission Pathways, in the Context of Strengthening the Global Response to the Threat of Climate Change, Sustainable Development, and Efforts to Eradicate Poverty, Masson-Delmotte, V., Zhai, P., Pörtner, H. O., Roberts, D., Skea, J., Shukla, P. R., et al. (eds.). Cambridge, UK and New York, NY, USA: Cambridge University Press, Cambridge, 49–92.
Barimalala, R., Blamey, R. C., Desbiolles, F., and Reason, C. J. C. (2020). Variability in the mozambique channel trough and impacts on Southeast African rainfall. J. Clim. 33, 749–765. doi: 10.1175/JCLI-D-19-0267.1
Battisti, D. S., and Naylor, R. L. (2009). Historical warnings of future food insecurity with unprecedented seasonal heat. Science 323, 240–244. doi: 10.1126/science.1164363
Buizza, R. (2021). “Chapter 4 - Predictability,” in Uncertainties in Numerical Weather Prediction, eds H. Ólafsson and J.-W Bao (Elsevier), 119146. doi: 10.1016/B978-0-12-815491-5.00004-5
Buizza, R., and Leutbecher, M. (2015). The forecast skill horizon. Q. J. Roy. Meteorol. Soc. 141, 3366–3382. doi: 10.1002/qj.2619
Calzadilla, A., Rehdanz, K., Betts, R., Falloon, P., Wiltshire, A., and Tol, R. S. J. (2013). Climate change impacts on global agriculture. Clim. Chan. 120, 357–374. doi: 10.1007/s10584-013-0822-4
Ceglar, A., Toreti, A., Lecerf, R., Van der Velde, M., and Dentener, F. (2016). Impact of meteorological drivers on regional inter-annual crop yield variability in France Agric. Forest Meteorol. 216, 58–67 doi: 10.1016/j.agrformet.2015.10.004
Ceglar, A., Turco, M., Toreti, A., and Doblas-Reyes, F. J. (2017). Linking crop yield anomalies to large-scale atmospheric circulation in Europe. Agric For Meteorol. 240–241, 35–45. doi: 10.1016/j.agrformet.2017.03.019
Cervigni, R., and Morris, M. (2016). Confronting Drought in Africa's Drylands: Opportunities for Enhancing Resilience. © Washington, DC: World Bank; and Agence Française de Développement.
Crétat, J., Pohl, B., Dieppois, B., Berthou, S., and Pergaud, J. (2019). The Angola Low: relationship with southern African rainfall and ENSO. Clim. Dyn. 52, 1783–1803. doi: 10.1007/s00382-018-4222-3
FAOSTAT (2021). FAOSTATStatistical Database, Food and Agriculture Organization of the United Nations (FAO). Rome: FAOSTATDatabase. Available online at: https://www.fao.org/faostat/en/#data/QCL (accessed September, 2021).
Gomez-Zavaglia, A., Mejuto, J. C., and Simal-Gandara, J. (2020). Mitigation of emerging implications of climate change on food production systems. Food Res. Int. 134, 109256. doi: 10.1016/j.foodres.2020.109256
Henry, R. J. (2020). Innovations in plant genetics adapting agriculture to climate change. Curr. Opin. Plant Biology. 56, 168–173. doi: 10.1016/j.pbi.2019.11.004
Hersbach, H., Bell, B., Berrisford, P., and Hirahara, S. (2020). The ERA5 global reanalysis. Q. J. R. Meteorol. Soc. 146, 1999–2049. doi: 10.1002/qj.3803
Iizumi, T., Shiogama, H., Imada, Y., Hanasaki, N., Takikawa, H., and Nishimori, M. (2018). Crop production losses associated with anthropogenic climate change for 1981–2010 compared with preindustrial levels. Int. J. Climatol. 38, 5405–5417. doi: 10.1002/joc.5818
INGC (2009). Main report: INGC Climate Change Report: Study on the Impact of Climate Change on Disaster Risk in Mozambique, Asante, K., Brundrit, G., Epstein, P., Fernandes, A., Marques, M. R., Mavume, A., et al. (eds.). Mozambique: INGC, Mozambique
IPCC (2019). “Summary for policymakers,” in Climate Change and Land: an IPCC Special Report on Climate Change, Desertification, Land Degradation, Sustainable Land Management, Food Security, and Greenhouse Gas Fluxes in Terrestrial Ecosystems, eds P. R. Shukla, J. Skea, E. Calvo Buendia, V. Masson-Delmotte, H. O. Pörtner, D. C. Roberts, et al.
IPCC (2021). “Summary for policymakers,” in Climate Change 2021: the Physical Science Basis. Contribution of Working Group I to the Sixth Assessment Report of the Intergovernmental Panel on Climate Change, eds V. Masson-Delmotte, P. Zhai, A. Pirani, S. L. Connors, C. Péan, S. Berger, N. Caud, Y. Chen, L. Goldfarb, M. I. Gomis, M. Huang, K. Leitzell, E. Lonnoy, J. B. R. Matthews, T. K. Maycock, T. Waterfield, O. Yelekçi, R. Yu, and B. Zhou (Cambridge; New York, NY: Cambridge University Press), 3–32. doi: 10.1017/9781009157896.001
Karl, T. R., Nicholls, N., and Ghazi, A. (1999). “CLIVAR/GCOS/WMO workshop on indices and indicators for climate extremes workshop summary,” in Weather and Climate Extremes: Changes, Variations and a Perspective from the Insurance Industry, Karl, T. R., Nicholls, N., and Ghazi, A.(eds.). Amsterdam: Springer Netherlands, 3–7.
Knox, J., Hess, T., Daccache, A., and Wheeler, T. (2012). Climate change impacts on crop productivity in Africa and South Asia. Environm. Res. Lett. 7, 34032 doi: 10.1088/1748-9326/7/3/034032
Kukal, M. S., and Irmak, S. (2018). Climate-driven crop yield and yield variability and climate change impacts on the U.S. great plains agricultural production. Sci Rep. 8, 3450. doi: 10.1038/s41598-018-21848-2
Lesk, C., Coffel, E., Winter, J., Ray, D., Zscheischler, J., Seneviratne, S. I., et al. (2021). Stronger temperature–moisture couplings exacerbate the impact of climate warming on global crop yields. Nat Food. 2, 683–691. doi: 10.1038/s43016-021-00341-6
Lobell, D. B., and Field, C. B. (2007). Global scale climate–crop yield relationships and the impacts of recent warming. Environm. Res. Lett. 2, 014002. doi: 10.1088/1748-9326/2/1/014002
Lobell, D. B., Schlenker, W., and Costa-Roberts, J. (2011). Climate trends and global crop production since 1980. Science 333, 616–620. doi: 10.1126/science.1204531
Lu, J., Carbone, G. J., and Gao, P. (2017). Detrending crop yield data for spatial visualization of drought impacts in the United States, 1895–2014. Agric. For. Meteorol. 237–238, 196-208. doi: 10.1016/j.agrformet.2017.02.001
Mavume, A. F., Banze, B. E., Macie, O. A., and Queface, A. J. (2021). Analysis of climate change projections for Mozambique under the representative concentration pathways. Atmosphere 12, 588. doi: 10.3390/atmos12050588
McLachlan, B. A., van Kooten, G. C., and Zheng, Z. (2020). Country-level climate-crop yield relationships and the impacts of climate change on food security. SN Appl. Sci. 2, 1650. doi: 10.1007/s42452-020-03432-4
Moore, F., and Lobell, D. (2014). Adaptation potential of European agriculture in response to climate change. Nature Clim. Chan. 4, 610–614. doi: 10.1038/nclimate2228
Nobre, G. G., Hunink, J. E., Baruth, B., Aerts, J. C. J. H., and Ward, P. J. (2019). Translating large-scale climate variability into crop production forecast in Europe. Sci. Rep. 9, 1277. doi: 10.1038/s41598-018-38091-4
Ramirez, J., and Thornton, P. K. (2015). “Climate change impacts on African crop production,” in CCAFS Working Paper no. 119. CGIAR Research Program on Climate Change, Agriculture and Food Security (CCAFS). Copenhagen. Available online at: https://ccafs.cgiar.org/resources/publications/climate-change-impacts-african-crop-production (accessed July 17, 2022).
Ringler, C., Zhu, T., Cai, X., Koo, J., and Wang, D. (2010). Climate Change Impacts on Food Security in Sub-Saharan Africa: Insights from Comprehensive Climate Change Scenarios. Washington, D.C.: International Food Policy Research Institute.
Salinger, M. J., Marta, A. D., Dalu, G., Messerri, A., Baldi, M., Messeri, G., et al. (2020). Linking crop yields in Tuscany, Italy, to large-scale atmospheric variability, circulation regimes and weather types. J. Agricult. Sci. 158, 606–623. doi: 10.1017/S0021859620001021
Silva, J. A., and Matyas, C. J. (2014). Relating rainfall patterns to agricultural income: implications for rural development in Mozambique. Weather Clim Soc. 6, 218–237. doi: 10.1175/WCAS-D-13-00012.1
Testa, G., Reyneri, A., and Blandino, M. (2016). Maize grain yield enhancement through high plant density cultivation with different inter-row and intra-row spacings. Eur. J. Agron. 72, 28–37. doi: 10.1016/j.eja.2015.09.006
Thompson, H. E., Berrang-Ford, L., and Ford, J. D. (2010). Climate change and food security in Sub-Saharan Africa: a systematic literature review. Sustainability 2, 2719–2733. doi: 10.3390/su2082719
van Oldenborgh, G. J., and Burgers, G. (2005). Searching for decadal variations in ENSO precipitation teleconnections. Geophys. Res. Lett. 32, L15701. doi: 10.1029/2005GL023110
Keywords: agriculture, cereal yields, climate change, climatic drivers, multiple linear regression, production losses, warming
Citation: Takele R, Buizza R and Dell'Acqua M (2023) Atmospheric drivers affect crop yields in Mozambique. Front. Clim. 5:1214703. doi: 10.3389/fclim.2023.1214703
Received: 30 April 2023; Accepted: 09 August 2023;
Published: 14 September 2023.
Edited by:
Michael Bliss Singer, Cardiff University, United KingdomReviewed by:
Thong Nguyen-Huy, University of Southern Queensland, AustraliaDagmawi Teklu Asfaw, University of Bristol, United Kingdom
Copyright © 2023 Takele, Buizza and Dell'Acqua. This is an open-access article distributed under the terms of the Creative Commons Attribution License (CC BY). The use, distribution or reproduction in other forums is permitted, provided the original author(s) and the copyright owner(s) are credited and that the original publication in this journal is cited, in accordance with accepted academic practice. No use, distribution or reproduction is permitted which does not comply with these terms.
*Correspondence: Robel Takele, robeltakele.miteku@santannapisa.it