- 1University of Southern California, Los Angeles, CA, United States
- 2Department of Linguistics, University of Michigan, Ann Arbor, MI, United States
This study investigates co-speech movements as a function of the conversational turn exchange type, the type of speech material at a turn exchange, and the interlocutor’s role as speaker or listener. A novel interactive protocol that mixes conversation and (non-read) nursery rhymes works to elicit many speech turns and co-speech movements within dyadic speech interaction. To evaluate a large amount of data, we use the density of co-speech movement as a quantitative measure. Results indicate that both turn exchange type and participant role are associated with variation in movement density for head and brow co-speech movement. Brow and head movement becomes denser as speakers approach overlapping speech exchanges, indicating that speakers increase their movement density as an interruptive exchange is approached. Similarly, head movement generally increases after such overlapping exchanges. Lastly, listeners display a higher rate of co-speech movement than speakers, both at speech turns and remote from them. Brow and head movements generally behave similarly across speech material types, conversational roles, and turn exchange types. On the whole, the study demonstrates that the quantitative co-speech movement density measure advanced here is useful in the study of co-speech movement and turn-taking.
Introduction
The goal of this study is to examine whether and how interacting speakers deploy co-speech movements of the brows and head at speech turn exchanges in a dyadic spoken language interaction. We focus on these movements because they are not directly associated with semantic meaning, and thus might lend themselves to interactional use. We use an interactive, non-read speaking task and a quantitative measure of movement density (velocity peaks per second—first used for co-speech movement in Danner et al. (2018)—to evaluate a large amount of speech turn and kinematic data (Gordon Danner et al., 2021) in addressing our questions.
Experimental linguistics has increasingly attended to an embodied perspective on spoken language interaction. Phonetic research has examined co-speech movements of the hands, head, eyes and facial features (McClave, 2000; Krahmer and Swerts, 2007; Cummins, 2012; Kim et al., 2014; Fuchs and Reichel, 2016) to illuminate prosodic structure, primarily elicited with read speech. A large body of research has examined the informational role of co-speech movement of an individual (Kita and Özyürek, 2003; Özyürek et al., 2005; Gullberg, 2010), but less is known about co-speech movement behaviors in interactional contexts (though see e.g., Nota et al., 2021; Trujillo et al., 2021; Duncan Jr, 1972; Latif et al., 2014; Mondada, 2007).
Research on the human capacity for turn-taking in conversation has observed that turn-taking is remarkably fast and flexible (Duncan Jr, 1972; Stivers et al., 2009). The average gap between speakers in spontaneous speech is ∼200 ms (Levinson and Holler, 2014; Magyari et al., 2014; Garrod and Pickering, 2015; Roberts et al., 2015) and this gap is stable across a variety of languages and cultures (Stivers et al., 2009). As such, researchers have long been interested in what exactly happens at turns that enables the smooth flow of conversation, and research has focused on the ways that turn-end prediction and next-turn preparation are aided by attention to lexical, syntactic, semantic and prosodic content (De Ruiter et al., 2006; Magyari et al., 2014; Bögels and Torreira, 2015; Garrod and Pickering, 2015; Barthel et al., 2017). However, there are reasons to think that co-speech movement might be relevant for conversational turn-taking, in that listeners are sensitive to gestures and that these movements seem to facilitate comprehension (e.g., Kelly et al., 2010; Holler et al., 2014) and facilitate speech production, specifically by reducing cognitive load and facilitating lexical access (Krauss, 1998; Alibali et al., 2000; Goldin-Meadow et al., 2001; Melinger and Kita, 2007; Gillespie et al., 2014). Studies specifically examining co-speech movements at turn-ends or in an interactional context suggest that co-speech movements likely contribute to effective turn-taking; these include movements of the hands, head, face, eye blinking (Duncan Jr, 1972; Hadar et al., 1985; Mondada, 2007; Barkhuysen et al., 2008; Sikveland and Ogden, 2012; Levitan et al., 2015; Holler et al., 2017; Hömke et al., 2018; Zellers et al., 2019; Trujillo et al., 2021) and gaze (Barkhuysen et al., 2008; Bavelas et al., 2002; Stivers et al., 2009). The most examined of these are manual gestures, and it has been suggested that these contribute to the selection of the next speaker, to indicating the end of the turn of the current speaker, and to soliciting help in the interaction, and there is evidence that listeners respond to these gestures by taking up the offered turn (Bavelas et al., 1995; Duncan Jr, 1972). Two studies have specifically investigated how the timing of turn exchanges is affected by the presence of manual co-speech gestures. Holler et al. (2017) find that, at least in question-response pairs, turn exchanges are faster when the question is accompanied by a co-speech gesture. Trujillo et al. (2021) also examine question-response pairs but separate turn exchanges into overlapping exchanges and non-overlapping exchanges and find that both gaps and overlaps between speakers are shorter when questions are accompanied by gestures.
Our study focuses on head and brow movement. Among co-speech body movements, head movement has received significant attention in studies of communicative interaction (Hadar et al., 1985; Munhall et al., 2004; Krahmer and Swerts, 2007; Ishi et al., 2014). Ishi et al. (2014) find that head-motion type differs according to type of dialogue (for example, more head nods in questions than in turn-giving, see also Kendon, 1972) and that the frequency of some head movements is affected by the relationship between conversation partners. Head movement has been found to be more frequent during speaking than during listening (Hadar et al., 1983), but head nods are also known to be part of a listener’s repertoire, e.g., as backchannelling (Duncan Jr, 1972). Listener nods in turn seems to be coupled with speakers’ head nods (McClave, 2000) and can indicate a turn-taking request (Hadar et al., 1985). Nods can also be the first indicator of a response, preceding a verbal response (Stivers et al., 2009).
Beyond (whole) head movement, dynamic aspects of facial features are likely to be relevant to turn-taking and communicative interaction. Eyebrow movement has been studied in non-interactive spoken language (Krahmer and Swerts, 2007; Cvejic et al., 2010; Kim et al., 2014). Goujon (2015) finds that brow movements occur more frequently at the beginning of an utterance than elsewhere in the utterance, while Flecha-Garcia (2010) finds some evidence that such movement occurs at the start of hierarchically high discourse units. The frequency of eyebrow movement is also dependent on the speech material, with expression of personal opinions, for example, being related to more eyebrow movements (Goujon et al., 2015), and eyebrow movements also being more frequent in giving instructions than in asking questions (Flecha-García, 2010). Only a few studies have investigated the role of eyebrow movement in interactions. Guaitella et al. find that brow movements are significantly more likely to occur in the immediate vicinity of the initiation of a new speaking turn than elsewhere in conversations, and the authors link this to the speaker’s intention to communicate (Guaïtella et al., 2009). Borràs-Comes et al. (2014) find that speakers of Catalan and Dutch use more eyebrow raises in questions than in responses. Similarly, Nota et al. (2021) find more eyebrow movements in questions compared to responses for Dutch and that they occur typically early in the utterance (before the onset of speech). Nota et al. (2021) suggest that this might be in order to allow the interlocuter more time to plan the response.
Many of the previous studies examine manual gestures that have an obviously interpretable communicative function, for example, gestures that are iconic, metaphoric, deictic, or pragmatic, and then relate these to the meaning or function in the utterance. It has also been suggested that the cessation of co-speech gestures functions for the listener as a signal for turn completion (Duncan Jr, 1972; Levinson and Torreira, 2015). Thus, together with information from the acoustic speech signal, co-speech gestures could help in predicting the end of the turn and concomitantly help in timing the onset of the next turn (e.g., Barkhuysen et al., 2008; Stivers et al., 2009; Holler et al., 2017; Nota et al., 2021). The general approach of studying the occurrence and placement of individual gestures, largely with meaningful interpretations, contrasts with our approach in that we examine the broad patterning of general movement density in the neighborhood of an interactional event of interest, namely a floor exchange. While our study does not directly test or model predictability of a floor exchange, by evaluating the patterning of co-speech movement at exchanges we lay the ground for future studies of how co-speech movement contributes to the management of interactions and we offer a new empirical strategy for assessing these complex multimodal signals.
The present study examines the rate or density of co-speech head nods and eyebrow raises at speech turn exchanges. Specifically, using non-read conversational interactions with robust opportunities for turn-taking, we examine whether the rate of co-speech movement varies as a function of proximity to a turn exchange, the type of speech turn, or the conversational role. Importantly, we examine any type of movement, regardless of its function. As will be explained in more detail in the next section, our study uses a measure common in articulatory speech production research, namely the rate/density of movement expressed in velocity peaks per second. This measure was first used by Danner et al. (2018), where it has been shown that distinct varieties of movement are used in different kinds of speech tasks. The benefit of this measure is that it can be extracted almost automatically, allowing for a large database of turn exchanges to be examined. Relatedly, Bavelas et al. (2008, 1992) find that the rate of movement of co-speech gestures differs depending on co-speaker visibility and on whether the speaker was alone or part of a dialogue. These findings suggest that the frequency of co-speech movements may aid the human capacity for efficient turn-taking and conversation and thus is a potentially useful measure for our study.
A variety of patterns around floor exchanges are possible from what little we know from the prior literature. Our hypothesis is that the density of co-speech movements will differ depending on the type of floor exchange—whether having overlapping speech or non-overlapping speech—as compared to speech that is non-exchange-adjacent. This is based in particular on findings that turn-end prediction is facilitated by utterance final (prosodic, syntactic, and lexical) information and on the evidence that co-speech movement differs utterance-finally compared to utterance-medially (Duncan Jr, 1972; Barkhuysen et al., 2008; Bögels and Torreira, 2015). We further examine whether the density of co-speech movement will differ as a function of the interlocuter’s immediate role at the exchange, i.e., as “listener” or “speaker”. Previous studies have focused mostly on one participant in an interaction, but a number of findings point to different functions of co-speech movement for the listener and for the speaker. Since our study is among the first to examine the co-speech movements of both participants in a robust sampling of conversational floor exchanges, specific predictions cannot yet be made as to whether listener and speaker will differ in the density of head and brow movement. For example, a speaker may increase the rate of their co-speech movements to indicate an upcoming turn end or to focus phrase edge material, and this may facilitate a listener’s prediction of the end of the turn, thereby facilitating the turn exchange. Furthermore, a listener may increase their movement density as a precursor to interrupting, starting a turn, or as an act of affiliation while listening. These possibilities have motivated the current study of dyads by examining both the speech interval approaching a floor exchange—which we call Turn Approach—and the speech interval after the conversational baton changes hands to the other speaker—which we call Turn Receipt.
Our instrumental setup allows us to examine kinematic data for both listener and speaker simultaneously. And while most prior research on co-speech movement in interactions has focused on manual gestures (and to a lesser extent on head movement), our study quantifies and examines both whole-head and eyebrow movement. In comparison to the more studied manual gestures, these articulators are less directly associated with semantic meaning (other than the agreement and disagreement of head nods) and may lend themselves to interactional use. The experimental approach of the current study of dyads thus advances a more complete understanding of co-speech movement patterning with the goal of broadening our empirical knowledge of the interactional process taking place between conversing partners.
Strategies for Experimental Design
While motion-capture technology and other tools for detecting movement from video have existed for some years in speech and linguistic research (Levelt et al., 1985; Munhall et al., 1985; Yehia et al., 1998; Barbosa et al., 2008), recent advances have enabled researchers to examine conversational interaction in a variety of novel ways: from empirically quantifying movement in video recordings (Barbosa et al., 2012; Voigt et al., 2016), to directly tracking the kinematics of speech-accompanying movements (Ishi et al., 2014; Kim et al., 2014; Danner et al., 2018), to considering the speech articulator kinematics of two interacting speakers (Scobbie et al., 2013; Lee et al., 2018). The present study combines several of these tools with the goal of examining co-speech movement density at conversational turn exchanges for pairs of speakers in naturalistic, face-to-face conversation. By capitalizing on a dual-magnetometer setup described in the Methods section below (see also previous work from our laboratory e.g. Lee et al., 2018), we are able to collect time-aligned audio and kinematic signals from pairs of conversing speakers. The conversing speakers were seated facing each other, able to see one another’s heads, arms/hands and torsos. This experimental setting offers the rare opportunity to collect kinematic data for two interacting speakers in a relatively natural setting, enabling the study of participants in their roles as both speakers and listeners during an interaction. While the exchange of these conversational roles has of course been extensively observed (Rochet-Capellan and Fuchs, 2014), the consideration of empirical data for the co-speech movements of interacting pairs in conversation, with annotation of conversational role (speaker and listener), has rarely been undertaken with kinematic data for both participants in a dyadic interaction.
A further advantage of our experimental protocol is that the rich kinematic data can be analyzed quantitatively, as described in detail in the methods section below. Specifically, using a method developed in Danner et al. (2018), a measure of movement rate (density) is algorithmically derived from brow and head movement kinematic velocity profiles (see for other uses of velocity profiles: Leonard & Cummins, 2011; Munhall et al., 1985; Ostry et al., 1987). This differs from manually annotating multiple gestural landmarks from video recordings and classifying them according to their communicative function, a technique used in many foundational studies of co-speech movement behavior (see overviews in Danner et al., 2018; Wagner et al., 2014). This method has given invaluable results but it has always, by necessity, been limited to a very small set of data. For example, Bavelas et al. (1995) was based on 88 gestures (selected from a larger corpus of 464 gestures), Holler et al. (2017) examined 281 question response sequences, Loehr (2004) is based on 164s of data and 147 gestures, and seminal work Kendon’s (1972) was based on 90 s of data. While our method does not explicitly assess the communicative function of co-speech movement, it automatically detects movement occurrence, thereby enabling the examination of a much larger set of data (>85 min of speech, 3,110 exchanges and thousands of individual movements) than the earlier gesture annotation method. In order to elicit structured turn-taking that is not read speech, we developed a speech elicitation paradigm in which dyads cooperatively undertake a spoken language task that promotes significant interaction between the interlocutors (see also Danner, 2017; Geluykens & Swerts, 1992; Lee et al., 2018). Crucially, by not relying on reading, our study allows participants the opportunity to interact with one another in a visually engaged way that promotes naturalistic speech and co-speech behavior with ample opportunities for floor exchanges. This protocol was achieved by leveraging familiar nursery rhymes in a collaborative task, as described in detail below. While many studies use conversational interactions and non-read speech to examine co-speech movement, the short, easily predicted phrases of the present task provide many opportunities for participants to exchange speaking turns both related and unrelated to the nursery rhyme at hand. The prosodic and rhythmic structure of the nursery rhymes, along with the engaging collaborative nature of the task, promote speech-accompanying movements of the brow, head, and hands, as well as non-speech communication like smiling and laughing, as participants cooperate to complete a rhyme; for these reasons this data collection protocol is particularly suitable to examining our question of co-speech movement patterning in the approach and receipt of dyadic floor exchanges.
Taken together, the instrumental set-up utilized within the collaborative speech task allows for a robust quantified view of the speech and co-speech movements that are integral to human conversational interaction. This empirical data alongside the innovative experimental data elicitation paradigm offers a new window for advancing the understanding of the cognitive and linguistic processes that underlie the elegant human ability for conversation.
Methods
Experiment Design and Stimuli
In order to study how fluctuating conversational conditions might affect co-speech movement behavior, we created a cooperative turn-taking task in which participants were asked to work together to recite fairly well-known English nursery rhymes. Nursery rhymes were selected for use in this task in order to elicit naturalistic interactions between two participants that were conducive to numerous speech turn exchanges. Nursery rhymes were suited to this goal due to their prosodic structure and the fact that, as somewhat familiar speech material, they could (after prompting) be recalled and produced with relative ease without being read. Participants were asked to complete the rhymes by taking turns and helping one another to complete the rhyme if either speaker were to forget the next portion of the rhyme. If the participants working together got irrevocably stuck trying to complete a rhyme, they could decide to give up and move on to the next rhyme, but this occurred quite rarely. These nursery rhymes are suited to the elicitation goals of this project because they are many in number and commonly known, they tend to be short (typically around 15–30 s for a solo production of one well-known verse), and they have a simple rhythmic and phrasal structure and accessible rhyme patterns (Fuchs and Reichel, 2016). It was useful for our elicitation purposes that most native speakers have been exposed to nursery rhymes as children but that the rhymes are not regularly encountered by older children and adults without children. We expected that our participants would have a baseline level of familiarity with nursery rhymes but may not remember a given nursery rhyme in exact detail.
This then provided an excellent opportunity for interaction between the participants, given that they were likely to need each other’s help to remember and complete the rhyme or negotiate with each other as to when to give up and move on. It’s important to note that the recorded conversational interaction for each dyad included a great deal of speech well beyond the production of nursery rhymes themselves, as the participants navigated the task they had been given to cooperate in. This meant that free conversational material—chatting between the speakers—was intermingled with the nursery rhyme production material. In fact a coding of the immediately turn final material (See Exchange Types, Conversational Roles and Speech Content Types section below) indicates that roughly 40% of the exchanges were conversational and not strictly nursery-rhyme production. All the speech material in the entire session was included in the analysis below, which is to say that the analyzed material included both free conversation and nursery rhyme production. Critically, the task elicited many floor exchanges with a variety of speech material, as was intended.1
To construct the nursery rhyme stimuli set for this experiment, we selected 24 common nursery rhymes found on the website nurseryrhymes.org (Granum, 2017). From this database of 202 unique nursery rhymes, we excluded rhymes that are not primarily in English (e.g., Frère Jacques), rhymes requiring stylized melodies or “dances” (e.g., I’m a Little Teapot), rhymes introduced within the last century (e.g., Miss Suzy/Hello Operator), rhymes of a religious nature (e.g., Now I Lay Me Down to Sleep), and rhymes longer than three stanzas (e.g., Little Bunny Foo). The titles of the 24 remaining rhymes were submitted to the Corpus of Contemporary English (Davies, 2008) and a Google search to norm for frequency of appearance (COCA count frequency ranged between 0 and 134 appearances, mean = 18.46; Google frequency ranged between 99,800–843 M hits; mean = 134.9 M). The two least frequent rhymes, Peter Pumpkin Eater and There Was an Old Woman Who Lived in a Shoe, were chosen for use as practice trials.
Subjects
Six pairs of previously unacquainted2 speakers, henceforth dyads, participated in the experiment. The first two dyads recorded were used as pilot data to refine our data collection procedure and were excluded from further analysis, leaving four analyzed dyads, henceforth referred to as Dyads 1–4. Dyads 1 and 3 are composed of two female participants, while Dyads 2 and 4 are composed of one male and one female participant. Participants range in age between 19 and 40 (mean age: 27.75) and are native speakers of American English. All participants voluntarily completed the entire experiment, which lasted approximately 1.5–2.5 h, and all participants were naïve to the purpose of the study.
Data Acquisition
Participants were seated at facing desks approximately 2 m apart in a sound-insulated room in the University of Southern California Phonetics Laboratory. Each participant had a Wave (Northern Digital, Inc.) electromagnetic articulography (EMA) system positioned beside their head, a tabletop microphone and a computer monitor on their desk, and a tripod and video camera positioned in front of the desk, angled down toward the speaker. The monitor and microphone were placed to allow each participant an unobstructed view of the other participant’s head and upper body. Figure 1A shows a schematic of the experiment room setup. Prior to the beginning of the experiment, participants were given the stimulus set of 24 nursery rhymes, each one printed on an individual sheet of paper, and were instructed to have a quick read-through of each rhyme only once, before putting that sheet of paper face-down on their desk. After both participants finished reading the set of nursery rhymes once through, the study personnel removed the papers from the participants’ desks and commenced with EMA sensor placement.
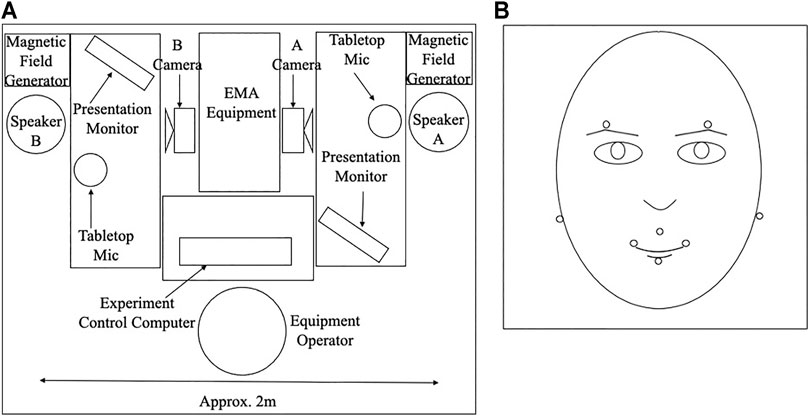
FIGURE 1. Panel (A) shows the experiment room setup; Panel (B) shows EMA sensor placement schematic: right/left/center reference sensors, right/left brow sensors, right/left lip corner sensors, and lower lip sensor.
Following standard EMA protocol, head reference EMA sensors were adhered externally at participants’ left and right mastoid processes and internally on the gum above the upper incisor, using a temporary adhesive. An occlusal plane measure was then taken for each participant, after which study personnel placed the remaining EMA sensors on the lower lip (mid-sagitally on the vermillion border), the right and left brows (placed above the most mobile part of the brow), and on the right and left upper lip corners as close to where the upper and lower lips meet as possible. Figure 1B shows the sensor placement schematic. An XML-based Matlab tool for stimulus presentation and experiment management called Marta (custom software written by Mark Tiede at Haskins Laboratories, New Haven, CT) was used to present stimuli to participants via separate monitors on their desks, and to save and organize recorded data by participant and trial for later analysis. The EMA sensor movement was sampled at 400 Hz, and speech audio was sampled at 44.1 kHz. GoPro cameras were used for video capture, using 1080 PPI resolution and a medium field of view in H.264 encoding; secondary GoPro audio was recorded in stereo at 48 kHz. (GoPro video and audio are not analyzed for the present study).
After sensor placement was complete, participants were jointly briefed on their tasks and the experiment began. The first task in the experiment was a brief mutual introduction between the two participants lasting 2 min, which served to familiarize the dyad members with one another and help them adapt to speaking with the EMA sensors. The next task was the primary experimental task of collaboratively completing nursery rhymes. The specific instructions that subjects received are described in Supplementary Table S1; the experimenters also verbally instructed the subjects that they will work as a team taking turns to say a nursery rhyme, going back and forth and helping one another finish the rhyme if someone gets stuck. On their screen, participants saw the first phrase of each rhyme, and for each rhyme, a graphic “star” was displayed on one participant’s screen serving to denote who would start that particular rhyme; this alternated between speakers. The rhyme presentation order was randomized for each dyad, and before each trial, a beep sound was played as a go-signal (and to facilitate future alignment of video with EMA/audio). The dyads completed practice trials of two nursery rhymes that were not repeated in the main experiment, after which, experiment personnel answered any participant questions and provided feedback on whether the practice trial was performed in accordance with the instructions. The participants then proceeded to complete one block of the nursery rhyme task (24 nursery rhymes). After the conclusion of the entire first block, the speakers completed another 2 min conversation period in which they were asked to find out what they had in common with one another; this provided a rest from the semi-structured task (and possibly helped sustain a friendly affiliative atmosphere between the participants). Following this, the participants were again given the chance to briefly read through the printed pages of nursery rhymes. The second repetition of the nursery rhyme task subsequently commenced with stimuli presented in the same randomized presentation order as in the first block for that dyad. The completion of the second round of the nursery rhyme task concluded the experiment, after which sensors were removed. The conversational and commonalities tasks were not included in the data analysis portion of the study; only blocks 1 and 2 of the nursery rhyme task were analyzed. That said, in addition to the nursery rhyme material itself, the design elicited a substantial amount of conversational material not related to the rhymes within those blocks as the dyads conversed and collaborated on the task.
This protocol yielded a large database of over 85 min of actual speech audio (Dyad S5S6: 33.67 min; Dyad S7S8: 19.59 min; Dyad S9S10: 12.61 min; Dyad S11S12: 19.43 min).
Data Processing
The kinematic trajectories of the EMA sensors were used to calculate gestural density of head and brow gestures as follows. EMA sensor trajectory data was prepared for use with the MATLAB-based analysis program Mview (custom software written by Mark Tiede at Haskins Laboratories, New Haven, CT) by interpolating missing data and extracting three-dimensional sensor trajectories from raw data. As is standard, EMA sensor data was rotated to a coordinate system aligned with the speaker’s occlusal plane, and brow and lip-corner sensors were corrected for headmovement. Custom Python scripts were created to extract head and eyebrow movement data from the EMA sensor trajectory data.
Head movement data was derived as follows: the three-dimensional movement of the plane formed by the three head reference sensors (left and right external mastoid processes and just above the upper incisor [UI]) rotating around the projected EMA system origin was calculated at each sample. This head movement data was subsequently detrended and low-pass filtered at 5 Hz (Tiede et al., 2010). (Instantaneous) angular velocity derived from all three available dimensions of movement was then computed. Angular velocity peaks (in three dimensions) were extracted from all data for a given participant (as is common in EMA-derived signal analysis, the minimum velocity threshold for a given speaker was computed using 5% of the maximum observed value across all of that speaker’s trials; below-threshold head velocity peaks were not considered). Figure 2 shows an illustration of the head movement measure.
Brow movement was derived as follows: the y-dimensional Euclidean distance from the right brow sensor to the (fixed) upper incisor [UI] sensor was calculated. Brow movements were detrended and low-pass filtered at 12 Hz, and their instantaneous velocity was computed from the change in y-dimensional distance from the brow sensor to the fixed mandibular UI sensor. Positive instantaneous velocities, associated with upward-going brow movements, were used for all subsequent data analysis. Negative instantaneous velocities, associated with downward-going brow movements were not analyzed, as brow raising but not brow lowering has been observed to co-occur with discourse-relevant and with prosodically relevant acoustic events in speech (Flecha-García, 2010; Prieto et al., 2015). In the same manner as the head movement data, a minimum velocity peak threshold was computed for each participant’s brow data using 5% of the maximum value across all of a given participant’s trials, and only velocity peaks above this threshold were used.
For both head and brow movement data, the primary measure of interest was co-speech gesture density, measured as velocity peaks per second (PPS). Prior research has suggested that co-speech gestural density depends on speech and interlocutor context (Ishi et al., 2014; Danner et al., 2018). Gestural PPS is a time-normalized rate measure calculated for a variety of conversationally relevant regions, as described in detail below in Exchange Types, Conversational Roles and Speech Content Types.
The first author along with two trained research assistants produced a word-level transcription of the recorded speech. These transcriptions and the associated audio files were then submitted to the Penn Forced Aligner (Yuan and Liberman, 2008) for automatic text alignment, resulting in the production of Praat TextGrids (Boersma and Weenink, 2016) for each file. This implementation of forced alignment cannot attribute parts of a transcription to multiple speakers, so a subsequent annotation step was performed to check/correct the automatic alignment and to attribute speech to each of the two recorded speakers in a trial. After a transcription was produced using a two-channel audio file (one channel per speaker), speaker attribution was performed by separating the audio files into two mono channels, each of which was associated with only one speaker’s microphone. The final TextGrids contain the automatic force-aligned transcriptions at phone and word levels, a tier for each of the two speakers in a given file containing only the speech attributed to a given speaker, and a point tier where the acoustic onset and offset of each participant’s speech was annotated; the last three of these tiers are shown in the examples in Table 1. The annotations were used to demarcate participants’ speech turns, with the acoustic onset and offset of each participant’s speech corresponding to turn start and end points, respectively. Speech turn exchange events are described further below. All transcriptions, annotations, and turn start/end points were cross-checked by the first author and assistants for accuracy.
Exchange Types, Conversational Roles and Speech Content Types
The TextGrids described above were coded for different types of floor exchanges, the conversational role held by each speaker at each exchange, and whether the content of speech at the end of turns was rhyme-related or not. These coding decisions were made in the context of analysis windows. To determine the duration of the analysis window (which was dyad-dependent), the average inter-turn interval (ITI) for each dyad was computed as the average interval duration from the acoustic offset of a speech turn to the acoustic onset of the next speech turn across every trial of that dyad. The ITI was determined separately for each dyad to account for dyad-specific factors such as differences in conversational rate. The ITI duration was used only to determine the duration of the analysis window local to a floor exchange over which co-speech movement density was calculated (see Turn Approach and Turn Receipt Analyses below and Figure 3); ITI was not itself analyzed.
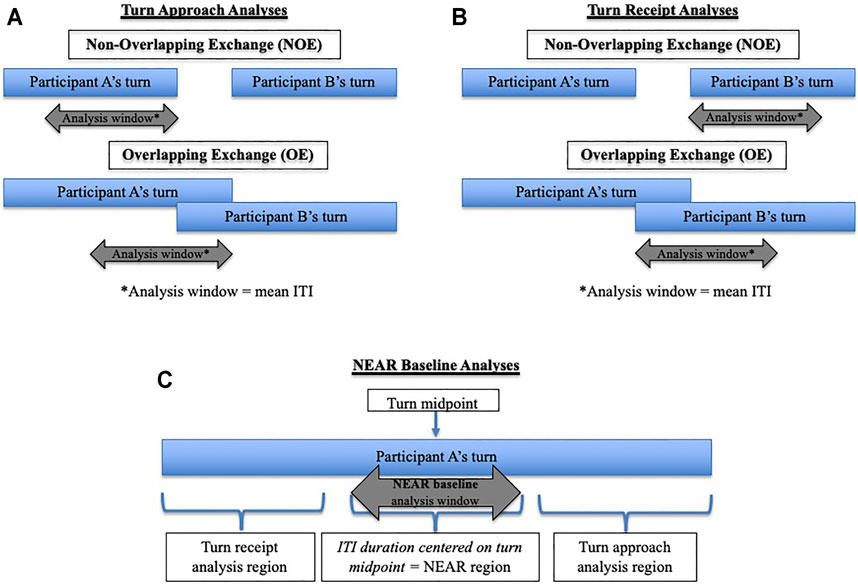
FIGURE 3. Panel (A) shows the Turn Approach analysis schematic; Panel (B) shows the Turn Receipt analysis schematic; Panel (C) shows the NEAR region schematic.
Three floor exchange types3 (factor: exchange type) were designated for data analysis, as follows (see Figure 3):
• Non-Overlapping Exchange (NOE): Exchanges in which one member of a dyad stops speaking, and after a pause, the other dyad member begins speaking.
• Overlapping Exchange (OE): Exchanges in which one dyad member begins speaking prior to the time when the other dyad member has stopped speaking.
• Non-Exchange-Adjacent Region (NEAR): A region of participant speech that does not fall within any other analysis window and which is not interrupted by the speech of the other dyad member. NEAR regions are considered a baseline region to which the other exchange-proximate regions of interest are compared; this level therefore serves as the reference level for the exchange type factor.
Example TextGrids for each of the analyzed exchange types (NOE, OE and NEAR) are shown in Table 1, and a summary of exchange types for each dyad can be found in Table 2.
In addition to floor exchange types, the data were coded for two conversational roles (factor: role):
• Speaker: leading up to a NOE (non-overlapping exchange), “speaker” is the dyad member speaking prior to the pause; after an NOE has just occurred, “speaker” is the person who takes the floor and begins speaking. At an OE (overlapping exchange), the “speaker” is the dyad member who is initially speaking before the other dyad member begins speaking. Speaker is used as the reference level of the roles factor.
• Listener: This is the dyad member who is not speaking during the analysis window, with the exception of the analysis region following an OE, in which case “listener” is the dyad member who is initially not speaking but who then begins speaking during the ongoing speech turn of the other dyad member.
Finally, both overlapping and non-overlapping exchanges were coded as being rhyme-related or non-rhyme-related (speech content). To perform this analysis, ITI durations described above were used to create an analysis window whose right edge aligned with the right edge of exchanges for each dyad. The text transcriptions of recorded speech occurring within this turn-approach analysis window were extracted and, to define the factor speech content obtaining at the floor exchange, the three coauthors coded the extracted transcriptions as being either:
• Rhyme-related: Primarily lexical material associated with the nursery rhyme that is underway (whether correct words or not).4 Rhyme-related speech was used as the reference level for the speech content factor
• Non-Rhyme-related: Primarily lexical material that is not associated with the nursery rhyme that is underway
Among overlapping (OE) and non-overlapping (NOE) exchanges (n = 1706), 38% of speech content was coded as non-rhyme-related and 62% was coded as rhyme-related. Average pairwise rater agreement was very strong at 93.24%; Fleiss’ κ = 0.852 (interrater reliability was assessed using ReCal3 (Freelon, 2013) and R package irr (Gamer et al., 2019)).
Turn Approach and Turn Receipt Analyses
Two regions of analysis of co-speech movement are considered—the region immediately leading up to a floor exchange, denoted the Turn Approach, and the region immediately following a floor exchange, denoted the Turn Receipt.
In the Turn Approach Analysis for non-overlapping exchanges (NOE), the right edge of the analysis window is aligned with the right edge—or end—of a participant’s speech turn, such that the analysis window covers the speech interval leading up to the floor exchange. For overlapping exchanges (OE), the right edge of the analysis window is aligned with the right edge (end) of the initial speaker’s turn. Schematic representations of analysis window placement and length for each Turn Approach exchange type are given in Figure 3A.
Specifically, to compute the average inter-turn interval (ITI) for each dyad, we took the following steps. For non-overlapping exchanges (NOE), the ITI value is a positive number. For overlapping exchanges (OE), we considered the ITI value to simply be zero since there is no inter-turn interval or delay between when one speaker stops speaking and the other begins. We summed all the ITI values for a dyad and divided by the total number of exchanges for that dyad5. This procedure for calculating average ITI yielded analysis windows of: 858 ms for Dyad 1, 763 ms for Dyad 2, 485 ms for Dyad 3, and 730 ms for Dyad 4. Note that ITI was used only to define the duration of the analysis windows and was not itself the object of any analysis.
In the Turn Receipt analysis, the analysis window duration for each dyad is computed in the same manner as in the Turn Approach analysis. This analysis is complementary to the Turn Approach analysis, in that the Turn Receipt analysis focuses on the opposite “side” of speech turns from the Turn Approach analysis. Therefore, the placement of the analysis window is now aligned to the left edge (or onset) of a speech turn, such that the analysis window covers the portion of a turn immediately following a speaker exchange. In the case of an overlapping exchange (OE) this corresponds with the onset of the second speaker’s turn. A schematic representation of the placement of analysis windows used in the Turn Receipt analysis is shown in Figure 3B.
Finally, the reference level for comparing movement density at floor exchanges was specified to be the NEAR (non-exchange adjacent region); see Figure 3C. The NEAR region is equivalent to the ITI duration centered on the midpoint of a turn, when turns were sufficiently long such that the NEAR region did not interfere with any other speaker’s speech or any other possible analysis region (either Turn Approach or Turn Receipt). If there was not enough duration in a given speech turn to guarantee that the NEAR region did not overlap any other analysis region, the NEAR was not calculated for that turn.
Results
The co-speech movement density results presented here comprise visualization, descriptive analysis, and linear mixed effects modeling. Data processing was performed in MATLAB (MATLAB, 2018), and statistical analyses were performed in R version 4.1.1 (R Core Team, 2021). Data manipulation and organization was performed in R using package dplyr version 1.0.7 (Wickham et al., 2019). Visualizations were produced using R package ggplot2 version 3.3.5 (Wickham et al., 2019). Linear mixed effects models and associated statistics were produced using R packages lme4 version 1.1–27.1 (Bates et al., 2015), lmerTest version 3.1–3 (Kuznetsova et al., 2014), and afex version 1.0–1 (Singmann et al., 2021). Each Turn Approach and Turn Receipt analysis includes violin graph visualizations of mean velocity peaks per second (PPS) for brow and head movements by individual participant, descriptive statistics for the PPS measure (summarized over all participants), and linear mixed effects models detailed in the Supplementary Materials.
The first analysis probes the effect of speech content during Turn Approach (the spoken material immediately preceding speech offset) on co-speech movement density (in peaks per second)6. We used the lmer() function in R package lme4 (Bates et al., 2015) to create a model containing the fixed effect of speech content and random effects of item and participant nested within dyad. We estimated significance using the χ2 tests and F-tests in the mixed() function of afex (Singmann et al., 2021).
Next, the analysis shifts to the primary goal of illuminating how conversational role and speech exchange type affect co-speech gesturing rate for movements of the brow and head in both the Turn Approach and Turn Receipt analyses. The Turn Approach region considers co-speech movement behavior leading up to a floor exchange. The Turn Receipt analysis concerns co-speech behavior immediately after a speaker exchange has occurred. The fixed effects are conversational role and speech exchange type and their interaction, with random effects of item (where each item is a particular nursery rhyme) and of participants nested within dyads. These models do not include random slopes because introduction of random slopes created convergence issues. The linear mixed effects models in these analyses all used the same fixed and random effects structure (PPS ∼ role * exchange type + (1|dyad/participant) + (1|item). We used the lmer() function in R package lme4 (Bates et al., 2015) to create an initial (treatment-coded) model containing all effects of interest. Then, using the function mixed() in the R package afex (Singmann et al., 2021), we estimated the significance of all fixed effects entered in the interaction analyses using F-tests with the Kenward-Roger method7 for approximating degrees of freedom. Finally, for each role * exchange type analysis, we created two parallel models with contrast coding to examine the main effects of exchange type and role. All models are reported in the Supplementary Materials section.
Speech Content Analysis
Previous research suggests that lexical/semantic content is useful to speakers in predicting the end of a speech turn (De Ruiter et al., 2006; Garrod and Pickering, 2015), and while our design reflects no prediction regarding whether the speech content at floor exchanges is associated with unique movement behavior responses, it was prudent to determine whether the nature of the lexical content at the floor exchange (coded as rhyme-related or non-rhyme-related based on the immediately preceding lexical material) had an association with co-speech movement density. Recall that a large portion, more than a third, of the speech content defined in this way was conversational and not specific to nursery rhyme production.
First, a 2 × 2 contingency table was created comparing type of speech content (rhyme-related or non-rhyme-related) and exchange type (overlapping exchange or non-overlapping exchange), and a χ2 analysis was performed to statistically assess the distributions. We found that speech content was indeed non-randomly associated with exchange type; χ2 (df = 1, N = 2,720) = 52.627 (p < 0.001). Specifically, rhyme-related speech content was more likely to be found at non-overlapping exchanges than at overlapping exchanges (see Figure 4), though qualitatively, the strength of this association varied by speaker (see Figure 5).
To statistically test the speech content analysis, we specified linear mixed effects models with the same structure for both brow and head movement signals8; we only consider the Turn Approach region in this speech content analysis, as lexical material in only this region was the basis for the coding for speech content. We included speech content as a fixed effect; random intercepts were fitted for each of two random effects, item and participant (participants are nested within dyad)9. The speech content model for brow movements was found to differ significantly from a model without the speech content effect (χ2(1) = 4.835, p = 0.028). Non-rhyme-related speech was associated with significantly denser Brow movements than baseline rhyme-related speech (β = 0.203, SE = 0.091, t = 2.229, p = 0.026). The speech content model for the head also differed from a model without the speech content effect (χ2(1) = 12.565, p < 0.001). Non-rhyme-related speech was also associated with significantly denser Head movements than baseline rhyme-related speech (β = 0.299, SE = 0.084, t = 3.585, p < 0.001). See Supplementary Tables S2, S3 for model formula and complete model summaries.
Turn Approach Analysis
As described above, the Turn Approach analysis was designed to consider speaker and listener behavior at and just before the offset of a speech turn (Figure 3A). This provides insight into the ways that speakers and listeners may pattern their movement behavior in anticipation of an upcoming floor exchange. The brow movement data is considered first and then the head movement data.
Turn Approach Brow PPS
Figure 6 shows a violin plot of Turn Approach Brow PPS data in the two factors of interest, conversational role and floor exchange type. Descriptive statistics are summarized in Table 3. Recall that NEAR regions (non-exchange-adjacent regions) are utilized as a reference level for exchange type and Speaker is the reference level for role. Including the predictors role and exchange type and their interaction improved model fit (F (2) = 37.01, p < 0.001). A model summary for the final model is available in Supplementary Table S4. In the model testing the main effect of role, Listeners were found to show significantly denser brow movements than Speakers (β = 1.165, SE = 0.089, t = 13.039, p < 0.001). In the model testing the main effect of exchange type, significantly denser brow movement was attested in the OE region than in the NEAR region (β = 0.614, SE = 0.127, t = 4.850, p < 0.001). Conversely, significantly less dense brow movement was attested in the NOE region than in the NEAR region (β = −0.275, SE = 0.117, t = -2.342, p = 0.019). See Supplementary Table S5 for summaries of the contrast-coded models used to report main effects. Finally, a significant interaction of Listener and OE region was observed, indicating that PPS values are affected by both the exchange type and a dyad member’s role as speaker or listener (β = −1.570, SE = 0.250, t = −6.287, p < 0.001). The PPS parameter estimate for Listeners at OE (3.127 PPS) is qualitatively higher than that of Speakers at OE (2.934 PPS) and the PPS value for Speakers’ brow movements at overlapping exchanges is more dense than their movements at NEAR (1.534), while this difference did not exist for Listeners (see regression table in Supplementary Table S6). The distinction in listener and speaker brow movement behavior in OE and NEAR regions drives the observed significant interaction value.
In sum, these results suggest that brow movements in Turn Approach regions are substantially more frequent for listeners than speakers, that brow movements are more dense at overlapping exchanges than in non-exchange adjacent regions (NEAR) of speech and less dense at non-overlapping exchanges than during NEAR speech. Additionally, exchange type and role jointly affect brow movement density driven by the fact that Speakers’ co-speech brow movements are denser at overlapping exchanges in Turn Approach than they are in non-exchange adjacent regions.
Turn Approach Head PPS
Turning to head movement density at Turn Approach, key patterns are shown in Figure 7. Descriptive statistics are summarized in Table 4. Including the predictors exchange type and role and their interaction improved model fit (F (2) = 23.99, p < 0.0001). A summary of the full model is presented in Supplementary Table S7. In a contrast-coded model testing the main effect of role, Listeners were found to show significantly denser head movements than Speakers (β = 0.927, SE = 0.081, t = 11.387, p < 0.001). In a contrast-coded model testing the main effect of exchange type, OE regions are associated with significantly greater head movement density than NEAR regions (β = 0.838, SE = 0.115, t = 7.256, p < 0.001). See Supplementary Table S8 for summaries of the contrast-coded models used to report main effects. A significant interaction of Listener and OE is obtained in the treatment-coded Turn Approach head movement model (β = −0.937, SE = 0.227, t = −4.125, p < 0.001). The PPS parameter estimate for Listeners at OE (2.951 PPS) is qualitatively greater than that of Speakers at OE (2.683 PPS), and the PPS value for Speakers at OEs is qualitatively denser than in NEAR intervals (1.376 PPS) but such a difference is not apparent for Listeners (see regression table in Supplementary Table S9), which drives the observed significant interaction value.
In sum, these results suggest that head movements in Turn Approach are, like brow movements, substantially more frequent for listeners than speakers, and that head movements in OE regions are more dense than those in NEAR regions. Also as with brow, exchange type and role jointly affect brow movement density with Speakers’ head movements being more dense at overlapping exchanges than at non-exchange adjacent regions.
Turn Receipt Analysis
The Turn Receipt analysis is complementary to the Turn Approach analysis, considering speaker and listener behavior at the onset and in the early moments of an initiated speech turn, when a new speaker has just begun speaking (see Figure 3B). The brow movement data is considered first, followed by the head movement data.
Turn Receipt Brow PPS
A graphical representation of Turn Receipt Brow PPS results is shown in Figure 8. Descriptive statistics are summarized in Table 5. Including the predictors exchange type and role and their interaction improved model fit (F (2) = 7.18, p < 0.001). In a contrast-coded model testing the main effect of role, Listeners’ brow movements at Turn Receipt were significantly denser than speakers’ movements (β = 1.736, SE = 0.090, t = 19.260, p < 0.001). In a contrast-coded model testing the main effect of exchange type, OE regions are associated with significantly greater head movement density than NEAR regions (β = 0.372, SE = 0.128, t = 2.916, p = 0.004). No significant interactions between levels of exchange type and role were observed for the Turn Receipt Brow model. See Supplementary Table S10 for a summary of the full model, Supplementary Table S11 for summaries of the contrast-coded models used to report main effects, and Supplementary Table S12 for the regression table for this model.
In sum, for the Turn Receipt Brow model, we again observe significantly denser listener brow movement compared with speakers. As was seen in the Turn Approach models, brow movements in OE regions at Turn Receipt are significantly denser than brow movements in NEAR regions. No significant interactions between the two fixed effects in this model were observed.
Turn Receipt Head PPS
Turning to the head movement data at Turn Receipt, a graphical representation of PPS for individual participants is shown in Figure 9. Descriptive statistics are summarized in Table 6. Including the predictors exchange type and their interaction improved model fit marginally (F (2) = 2.84, p = 0.058). In a contrast-coded model testing the main effect of role, Listeners were found to show denser head movements at turn approach than Speakers (β = 1.458, SE = 0.079, t = 18.472, p < 0.001). Similar to all the models discussed so far, significantly more head movement was attested in the OE Turn Receipt region than in the NEAR region (β = 0.461, SE = 0.112, t = 4.122, p > 0.001). A significant interaction of Listener and NOE is also observed in this model (β = 0.468, SE = 0.204, t = 2.289, p = 0.022). Note that the model fit only marginally improves when including the interaction term, so these model results should not be over-interpreted. See Supplementary Table S13 for a summary of the full Turn Receipt Head model, Supplementary Table S14 for summaries of the contrast-coded models used to report main effects, and Supplementary Table S15 for the full model regression table.
These results suggest that head movements in Turn Receipt regions are more frequent for listeners than speakers, and more frequent in OE regions than NEAR regions, as also observed for brow and head in Turn Approach and brow in Turn Receipt. A significant crossover interaction in NOE*Listener obtained, a result that is unique to the Turn Receipt head model.
Results Summary
The experiment protocol successfully provided a rich database of speech for four interacting dyads, with a variety of floor exchange types, speech both related and unrelated to the nursery rhyme prompts, and participants acting both as speakers and as listeners.
The analyses of the brow and head movement density signals revealed several similarities. Non-rhyme related speech content was associated with greater movement density than rhyme-related speech content, for Turn Approach head and brow movements. Listeners consistently produced higher movement density than speakers for both brow and head movement across all turn types, both approaching and following a floor exchange. Overlapping exchange regions were consistently associated with denser movements of both brow and head in Turn Approach and Turn Receipt than non-overlapping exchanges. Finally, approaching an overlapping floor exchange, speakers but not listeners displayed more dense movements of both brow and head relative to movement during speech remote from the floor exchange.
Discussion
Consistency Across Movement Signals
One of the current findings that warrants highlighting is the similarities in behavior across brow and head movements measured in this research.10 While a few researchers have considered both brow and head movements in the same study (e.g., Bolinger, 1983; Hadar et al., 1983; McClave, 2000; Clark and Krych, 2004; Munhall et al., 2004; Krahmer and Swerts, 2007; Kita, 2009; Kim et al., 2014; Prieto et al., 2015), previous research has not illuminated whether different effectors of co-speech movements pattern similarly or differently at floor exchanges. In the present study, there is a remarkable similarity in how brow and head behave in the vicinity before, after, and remote from a floor exchange. Given the inherent differences in range of motion, degrees of freedom and velocity of the signal types (and the known role of head movement in signaling semantic content such as agreement), this finding of systematic and similar patterning across the brow and head modalities stands to inform future investigations.
Speech Content and Movement Behavior
The central role of semantic and lexical content in successful conversational interaction is clear (De Ruiter et al., 2006). In this study we did not embark on a rigorous analysis of lexico-semantic characteristics of speech; we simply noted whether the spoken material immediately at the floor exchange was related or unrelated to the nursery rhyme verse and we tested whether that coded content had an association with movement behavior. A substantial number of studies have found that co-speech movement facilitates speech production—whether by facilitating thinking, reducing cognitive load, or facilitating lexical access (Alibali et al., 2000; Gillespie et al., 2014; Goldin-Meadow et al., 2001; Krauss, 1998; Melinger & Kita, 2007, though see Hoetjes et al., 2014 for possible evidence against this view). We would therefore have expected interlocutors to have higher co-speech movement density when executing the challenge of the rhyme task material, and furthermore, the rhythmic nature of the task (producing nursery rhymes) could have contributed to an increase of movement as well (for example an increase in movement associated with beat gestures). Instead, the reverse transpired for the Turn Approach region. It may be that the topical content of the non-rhyme related material was sufficiently concerned with the challenges of the collaborative task that it exhibited an uptick in co-speech movement density associated with heightened affect or load.
Exchange Types and Movement Behavior
This study sought to determine whether different types of conversational floor exchange events are associated with empirically distinct head or brow movement density. One clear result emerged across both analyses and signal types: overlapping exchanges were associated with speakers having substantially more dense head and brow movements than they did in non-exchange-adjacent regions of speech (Figures 5, 6). Figures 6–9 This finding can be considered in line with Duncan’s suggestion that termination of manual co-speech gestures on the part of the speaker is a turn-yielding signal and the continuation of a manual co-speech gesture an “attempt suppressing” signal (Duncan Jr, 1972). While our approach differs from Duncan’s, in that we do not analyze the timing of the end or the continuation of co-speech gestures but rather the density of movement, we think this increase in movement at overlapping exchanges by the speaker can be seen as further supporting this finding through a different measure, and now for brow and head movement. Alternatively, this increase in movement could be also due to the speaker and listener interacting concurrently and the speaker signaling cooperation in yielding the turn.
Movements at non-overlapping exchanges (NOEs) showed no consistent difference from non-exchange-adjacent (NEAR) speech for brow or head movements in the Turn Approach or Receipt regions. It is not entirely clear why movement behavior around non-overlapping exchange (NOE) speech is similar to baseline because co-speech movements could conceivably help with smooth turn-exchanges (e.g., Stivers et al., 2009; Trujillo et al., 2021). Nevertheless, our results indicate that interlocutors generally negotiate a NOE without an increase in their co-speech movement. (Cf. Duncan Jr, 1972 who finds the end of a manual gesture to be a signal for the end of the turn.) A question for future research is whether listeners actually use these movement signals to help predict the end of a current speaker’s turn. An additional topic for future research concerns the functional role(s) of movement during the Turn Receipt, and whether qualitative rather than quantitative changes in movement behavior are meaningful.
Conversational Roles and Movement Behavior
Previous research has focused predominantly on co-speech movement behavior of a speaker. While there are a few previous works that have focused on the co-speech behavior of listeners versus speakers (Hilton, 2018), relatively little is known about listener movement behavior or simply the behavior associated with silent listening. Our study offers a novel consideration of empirical kinematic data collected simultaneously from both a speaker and a listener during interaction. One of the most consistent observations in the co-speech movement in our study was the more frequent head and brow gestures of listeners as compared to speakers. When participants were in the role of listeners, they moved their head and brow more frequently than they did when in the role of speakers, an observation that held true for both Turn Approach and Turn Receipt analysis regions. There are a number of interpretations of why listener co-speech movement is more frequent than speaker movement in the present study. The uptick in co-speech movement density may indicate attentiveness or affiliation with the speaker (Clark and Krych, 2004; Latif et al., 2014), or it may indicate a listener’s intent to start speaking (Duncan Jr, 1972; Hadar et al., 1985; Lee and Narayanan, 2010), or simply help the listener initiate their turn in some way (Hadar et al., 1983). Certainly, the across-the-board higher density of listener co-speech movement could be due to backchanneling that helps regulate turn-taking (McClave, 2000), but it may also be one way for the listener to engage in the interaction that does not intrude on the spoken contribution currently underway.
Summary and Conclusion
Using a novel interactive protocol designed to elicit many conversational floor exchanges within a structured, non-read dyadic speech interaction, this study examines hypotheses that the density of co-speech movements differs depending on exchange type and the participant’s role as speaker or listener in the interaction. The results support the specific hypotheses. In brief, we find that co-speech movements of the brow and head are more dense for listeners as opposed to speakers, and that this is the case in both Turn Approach and Turn Receipt regions. Additionally, listeners display a higher rate of co-speech movement than speakers both at floor exchanges and remote from them. This patterning may be related to a listener’s desire to signal interest, engagement or attention to the speaker without actually intruding on their interlocutor’s speech signal, as well as possibly facilitating conversational turn-taking (Hadar et al., 1985; Holler et al., 2017).
Movement behavior is increased for speakers approaching overlapping exchanges (interruptions). Conversational role interacts with the type of floor exchange in its association with co-speech movement. Speakers who are approaching an interruptive exchange show an increase in their co-speech movement, possibly attempting to keep the floor or possibly creating a visual scenario that listeners see as ripe for interruption.
Overall, a high level of activation of interactional management and negotiation is exhibited in this dataset. We conclude that this interactional navigation may be facilitated in part by the patterning of co-speech movement across interlocutors that this study is able to analyze quantitatively for the first time. Furthermore, with the ability to examine both brow and head movements in conjunction, the kinematic data indicate that brow and whole-head movement densities tend to behave similarly across exchange types and conversational roles. Lastly, our findings based on large quantities of (non-read) dyadic speech have implications for the likelihood of any role of co-speech (non-manual) gesture in facilitating turn end prediction in that when approaching a floor exchange as the sole talker, no reliable changes in the amount of co-speech movement on the part of speakers are observed. Taken together, the study is an initial step in characterizing how speakers’ and listeners’ co-speech movements jointly pattern in dyadic conversational interaction.
Data Availability Statement
The datasets presented in this study can be found in online repositories. The names of the repository/repositories and accession number(s) can be found below: http://dx.doi.org/10.17632/jy5t72fd32.4 Gordon Danner, Samantha (2021), “Dataset for Co-speech Movement in Conversational Turntaking,” Mendeley Data, V4, doi: 10.17632/jy5t72fd32.4.
Ethics Statement
The studies involving human participants were reviewed and approved by the University of Southern California Institutional Review Board. The patients/participants provided their written informed consent to participate in this study.
Author Contributions
SD: Conceptualization (supporting); Writing—original draft (lead), revisions (co-lead); Data curation (lead); Formal analysis (lead); Investigation (equal); Methodology (equal); Project administration (supporting); Resources (supporting); Software (lead); Supervision (supporting); Validation (lead); Visualization (lead). JK: Conceptualization (lead); Writing—original draft (supporting), revisions (co-lead); Formal analysis (supporting); Investigation (equal); Methodology (equal); Visualization (supporting). DB: Conceptualization (lead); Writing—original draft (supporting), revisions (co-lead); Formal analysis (supporting); Funding acquisition (lead); Investigation (equal); Methodology (equal); Project administration (lead); Resources (lead); Supervision (lead); Visualization (supporting).
Funding
This work was supported by NIH DC003172 (Byrd).
Conflict of Interest
The authors declare that the research was conducted in the absence of any commercial or financial relationships that could be construed as a potential conflict of interest.
Publisher’s Note
All claims expressed in this article are solely those of the authors and do not necessarily represent those of their affiliated organizations, or those of the publisher, the editors and the reviewers. Any product that may be evaluated in this article, or claim that may be made by its manufacturer, is not guaranteed or endorsed by the publisher.
Acknowledgments
We thank Mathias Barthel, James P. Trujillo, Nynaeve Perkins Booker, Lena Foellmer, Louis Goldstein, Sarah Harper, Cheyenne LaRoque, and Sungbok Lee for their assistance.
Supplementary Material
The Supplementary Material for this article can be found online at: https://www.frontiersin.org/articles/10.3389/fcomm.2021.779814/full#supplementary-material
Footnotes
1While our task elicits both nursery rhyme material and spontaneous speech, it can be assumed that other types of interaction would give different results, as different tasks and contexts elicit different conversational strategies and different co-speech gesture behavior (see for example Danner et al., 2018; Dideriksen et al., 2019).
2Lack of familiarity between participants in a dyad was the only constraint we placed on their pairing. Although speaker age, gender, race or other perceived or real demographic information may affect some aspects of interaction, we have no reason to believe that these details impact the fine-grained movement behavior that is the object of this study, nor was this study designed to probe such myriad socio-linguistic variables.
3Two additional exchange types were identified in the dataset but were not included in further analysis. The first of these is a turn-within-turn, in which one dyad member’s speech turn occurs entirely within the other dyad member’s speech turn. The second exchange type excluded from analysis is a non-consummated exchange, in which one dyad member stops speaking and after a long pause (>500 ms) during which the other dyad member remains silent, the same dyad member begins speaking once again. These excluded exchange types are challenging to interpret as turns-within-turns could represent either backchanneling or a failed floor exchange, and non-consummated exchanges could represent a failed floor exchange or an exceptionally long pause.
4Coders also had available for reference the canonical text of each of the 24 nursery rhymes. In cases where both speakers were speaking during the analysis window, the instructions to the coders stated that speech should be coded as ‘rhyme-related’ if either one of the two speakers’ transcriptions were primarily lexical material associated with the ongoing rhyme
5In addition to NOE and OE exchange types, non-consummated exchanges (as described in Exchange Types, Conversational Roles and Speech Content Types) are included in the ITI calculations so as to include all potential floor exchanges
6Note that because speech content type was evaluated based only on the words at the offset of speech turns, this was analyzed only for Turn Approach and not for Turn Receipt analyses (because Turn Receipt analyses consider speech turn onset).
7This method provides good control against Type I errors in smaller datasets like the one presented here.
8We use treatment coding to report results for the single fixed effect in the model.
9The specification of random slopes caused convergence issues and were therefore not included; though it would be ideal to have enough data to estimate random slopes, the random effects structure described here is a very good representation of the experiment as performed.
10We can be sure in our study that the movement of the brow is not merely a consequence of the movement of the head, because head movement correction was performed on the brow movement trajectories (but not on the head movement trajectories). It is plausible that other future measures of the brow and head movements, such as displacement or duration, could yield differences.
References
Alibali, M. W., Kita, S., and Young, A. J. (2000). Gesture and the Process of Speech Production: We Think, Therefore We Gesture. Lang. Cogn. Process. 15, 593–613. doi:10.1080/016909600750040571
Barbosa, A. v., Yehia, H. C., and Vatikiotis-Bateson, E. (2008). “Linguistically Valid Movement Behavior Measured Non-invasively,” in Auditory Visual Speech Processing. Editors R. Gucke, P. Lucey, and S. Lucey (Queensland, Australia), 173–177.
Barkhuysen, P., Krahmer, E., and Swerts, M. (2008). The Interplay between the Auditory and Visual Modality for End-Of-Utterance Detection. The J. Acoust. Soc. America 123, 354–365. doi:10.1121/1.2816561
Barthel, M., Meyer, A. S., and Levinson, S. C. (2017). Next Speakers Plan Their Turn Early and Speak after Turn-Final "Go-Signals". Front. Psychol. 8, 1–10. doi:10.3389/fpsyg.2017.00393
Bates, D., Mächler, M., Bolker, B., and Walker, S. (2015). Fitting Linear Mixed-Effects Models Using lme4. J. Stat. Soft. 67 (1), 1–48. doi:10.18637/jss.v067.i01
Bavelas, J. B., Chovil, N., Coates, L., and Roe, L. (1995). Gestures Specialized for Dialogue. Pers Soc. Psychol. Bull. 21, 394–405. doi:10.1177/0146167295214010
Bavelas, J. B., Chovil, N., Lawrie, D. A., and Wade, A. (1992). Interactive Gestures. Discourse Process. 15, 469–489. doi:10.1080/01638539209544823
Bavelas, J. B., Coates, L., and Johnson, T. (2002). Listener Responses as a Collaborative Process: The Role of Gaze. J. Commun. 52, 566–580. doi:10.1111/j.1460-2466.2002.tb02562.x
Bavelas, J., Gerwing, J., Sutton, C., and Prevost, D. (2008). Gesturing on the Telephone: Independent Effects of Dialogue and Visibility. J. Mem. Lang. 58, 495–520. doi:10.1016/j.jml.2007.02.004
Boersma, P., and Weenink, D. (2016). Praat: Doing Phonetics by Computer. Available at: http://www.praat.org/.
Bögels, S., and Torreira, F. (2015). Listeners Use Intonational Phrase Boundaries to Project Turn Ends in Spoken Interaction. J. Phonetics 52, 46–57. doi:10.1016/j.wocn.2015.04.004
Borràs-Comes, J., Kaland, C., Prieto, P., and Swerts, M. (2014). Audiovisual Correlates of Interrogativity: A Comparative Analysis of Catalan and Dutch. J. Nonverbal Behav. 38, 53–66. doi:10.1007/s10919-013-0162-0
Clark, H. H., and Krych, M. A. (2004). Speaking while Monitoring Addressees for Understanding. J. Mem. Lang. 50, 62–81. doi:10.1016/j.jml.2003.08.004
Cummins, F. (2012). Gaze and Blinking in Dyadic Conversation: A Study in Coordinated Behaviour Among Individuals. Lang. Cogn. Process. 27, 1525–1549. doi:10.1080/01690965.2011.615220
Cvejic, E., Kim, J., Davis, C., and Gibert, G. (2010). “Prosody for the Eyes: Quantifying Visual Prosody Using Guided Principal Component Analysis,” in Proceedings of the 11th Annual Conference of the International Speech Communication Association, Makuhari, Chiba, Japan, September 26-30, 2010, 1433–1436.
Danner, S. G. (2017). Effects of Speech Context on Characteristics of Manual Gesture. Doctoral dissertation Los Angeles, (CA): University of Southern California in Los Angeles.
Danner, S. G., Barbosa, A. V., and Goldstein, L. (2018). Quantitative Analysis of Multimodal Speech Data. J. Phonetics 71, 268–283. doi:10.1016/j.wocn.2018.09.007
Davies, M. (2008). The Corpus of Contemporary American English: 450 Million Words, 1990-present. Available at: http://corpus.byu.edu/coca/.
Dideriksen, C., Fusaroli, R., Tylén, K., Dingemanse, M., and Christiansen, M. H. (2019). “Contextualizing Conversational Strategies: Backchannel, Repair and Linguistic Alignment in Spontaneous and Task-Oriented Conversations,” in Proceedings of the 41st Annual Conference of the Cognitive Science Society : Creativity + Cognition + Computation. Editors A. K. Goel, C. M. Seifert, and C. Freksa (Canada: Montreal), 261–267. doi:10.31234/osf.io/fd8y9
Duncan, S. (1972). Some Signals and Rules for Taking Speaking Turns in Conversations. J. Personal. Soc. Psychol. 23, 283–292. doi:10.1037/h0033031
Flecha-García, M. L. (2010). Eyebrow Raises in Dialogue and Their Relation to Discourse Structure, Utterance Function and Pitch Accents in English. Speech Commun. 52, 542–554. doi:10.1016/j.specom.2009.12.003
Freelon, D. (2013). ReCal OIR: Ordinal, Interval, and Ratio Intercoder Reliability as a Web Service. Int. J. Internet Sci. 8, 10–16. Available at: http://www.ijis.net/ijis8_1/ijis8_1_freelon_pre.html.
Fuchs, S., and Reichel, U. D. (2016). “On the Relationship between Pointing Gestures and Speech Production in German Counting Out Rhymes : Evidence from Motion Capture Data and Speech Acoustics,” in Proceedings of P&P 12. Editors C. Draxler, and F. Kleber Munich: Ludwig Maximilian University, 1–4.
Gamer, M., Lemon, J., Fellows, I., and Singh, P. (2019). Various Coefficients of Interrater Reliability and Agreement. Available at: https://cran.r-project.org/package=irr.
Garrod, S., and Pickering, M. J. (2015). The Use of Content and Timing to Predict Turn Transitions. Front. Psychol. 6, 1–12. doi:10.3389/fpsyg.2015.00751
Geluykens, R., and Swerts, M. (1992). Prosodic Topic- and Turn-Finality Cues. Proceedings of the IRCS Workshop on Prosody in Natural Speech, Netherlands, 63–70.
Gillespie, M., James, A. N., Federmeier, K. D., and Watson, D. G. (2014). Verbal Working Memory Predicts Co-speech Gesture: Evidence from Individual Differences. Cognition 132, 174–180. doi:10.1016/j.cognition.2014.03.012
Goldin-Meadow, S., Nusbaum, H., Kelly, S. D., and Wagner, S. (2001). Explaining Math: Gesturing Lightens the Load. Psychol. Sci. 12, 516–522. doi:10.1111/1467-9280.00395
Gordon Danner, S., Krivokapić, J., and Byrd, D. (2021). Dataset for Co-speech Movement in Conversational Turn-Taking. V. doi:10.17632/jy5t72fd32.1
Goujon, A., Bertrand, R., and Tellier, M. (2015). Eyebrows in French Talk-In-Interaction Nantes, France.
Granum, M. (2017). NurseryRhymes.org - Nursery Rhymes with Lyrics and Music. Available at: https://www.nurseryrhymes.org/(Accessed November 6, 2018).
Guaïtella, I., Santi, S., Lagrue, B., and Cavé, C. (2009). Are Eyebrow Movements Linked to Voice Variations and Turn-Taking in Dialogue? an Experimental Investigation. Lang. Speech 52, 207–222. doi:10.1177/0023830909103167
Gullberg, M. (2010). Language-specific Encoding of Placement Events in Gestures. Event Representation Lang. Cogn. 11, 166–188. doi:10.1017/CBO9780511782039.008
Hadar, U., Steiner, T. J., and Clifford Rose, F. (1985). Head Movement during Listening Turns in Conversation. J. Nonverbal Behav. 9, 214–228. doi:10.1007/bf00986881
Hadar, U., Steiner, T. J., Grant, E. C., and Rose, F. C. (1983). Kinematics of Head Movements Accompanying Speech during Conversation. Hum. Movement Sci. 2, 35–46. doi:10.1016/0167-9457(83)90004-0
Hoetjes, M., Krahmer, E., and Swerts, M. (2014). Does Our Speech Change when We Cannot Gesture? Speech Commun. 57, 257–267. doi:10.1016/j.specom.2013.06.007
Holler, J., Kendrick, K. H., and Levinson, S. C. (2017). Processing Language in Face-To-Face Conversation: Questions with Gestures Get Faster Responses. Psychon. Bull. Rev. 25, 1900–1908. doi:10.3758/s13423-017-1363-z
Holler, J., Schubotz, L., Kelly, S., Hagoort, P., Schuetze, M., and Özyürek, A. (2014). Social Eye Gaze Modulates Processing of Speech and Co-speech Gesture. Cognition 133, 692–697. doi:10.1016/j.cognition.2014.08.008
Hömke, P., Holler, J., and Levinson, S. C. (2018). Eye Blinks Are Perceived as Communicative Signals in Human Face-To-Face Interaction. PLoS ONE 13, e0208030–13. doi:10.1371/journal.pone.0208030
Ishi, C. T., Ishiguro, H., and Hagita, N. (2014). Analysis of Relationship between Head Motion Events and Speech in Dialogue Conversations. Speech Commun. 57, 233–243. doi:10.1016/j.specom.2013.06.008
Kelly, S. D., Özyürek, A., and Maris, E. (2010). Two Sides of the Same Coin. Psychol. Sci. 21, 260–267. doi:10.1177/0956797609357327
Kendon, A. (1972). “Some Relationships between Body Motion and Speech,” in Studies In Dyadic Communication. Editors A. W. Siegman, and B. Pope (New York: Pergamon Press), 177–210. doi:10.1016/b978-0-08-015867-9.50013-7
Kim, J., Cvejic, E., and Davis, C. (2014). Tracking Eyebrows and Head Gestures Associated with Spoken Prosody. Speech Commun. 57, 317–330. doi:10.1016/j.specom.2013.06.003
Kita, S. (2009). Cross-cultural Variation of Speech-Accompanying Gesture: A Review. Lang. Cogn. Process. 24 (2), 145–167. doi:10.1080/01690960802586188
Kita, S., and Özyürek, A. (2003). What Does Cross-Linguistic Variation in Semantic Coordination of Speech and Gesture Reveal?: Evidence for an Interface Representation of Spatial Thinking and Speaking. J. Mem. Lang. 48, 16–32. doi:10.1016/S0749-596X(02)00505-3
Krahmer, E., and Swerts, M. (2007). The Effects of Visual Beats on Prosodic Prominence: Acoustic Analyses, Auditory Perception and Visual Perception. J. Mem. Lang. 57, 396–414. doi:10.1016/j.jml.2007.06.005
Krauss, R. M. (1998). Why Do We Gesture when We Speak? Curr. Dir. Psychol. Sci. 7, 54. doi:10.1111/1467-8721.ep13175642
Kuznetsova, A., Bruun Brockhoff, P., and Haubo Bojesen Christensen, R. (2014). lmerTest: Tests in Linear Mixed Effects Models. Available at: http://cran.r-project.org/package=lmerTest.
Latif, N., Barbosa, A. v., Vatiokiotis-Bateson, E., Castelhano, M. S., and Munhall, K. G. (2014). Movement Coordination during Conversation. PLoS ONE 9, e105036. doi:10.1371/journal.pone.0105036
Lee, C.-C., and Narayanan, S. (2010). “Predicting Interruptions in Dyadic Spoken Interactions,” in Proceedings of the 2010 IEEE International Conference on Acoustics, Speech and Signal Processing, Dallas, TX, USA, 14-19 March 2010 (Dallas, Texas: IEEE), 5250–5253. doi:10.1109/ICASSP.2010.5494991
Lee, Y., Gordon Danner, S., Parrell, B., Lee, S., Goldstein, L., and Byrd, D. (2018). Articulatory, Acoustic, and Prosodic Accommodation in a Cooperative Maze Navigation Task. PLOS ONE 13, e0201444. doi:10.1371/journal.pone.0201444
Leonard, T., and Cummins, F. (2011). The Temporal Relation between Beat Gestures and Speech. Lang. Cogn. Process. 26, 1457–1471. doi:10.1080/01690965.2010.500218
Levelt, W. J. M., Richardson, G., and la Heij, W. (1985). Pointing and Voicing in Deictic Expressions. J. Mem. Lang. 24, 133–164. doi:10.1016/0749-596X(85)90021-X
Levinson, S. C., and Holler, J. (2014). The Origin of Human Multi-Modal Communication. Phil. Trans. R. Soc. B 369, 20130302. doi:10.1098/rstb.2013.0302
Levinson, S. C., and Torreira, F. (2015). Timing in Turn-Taking and its Implications for Processing Models of Language. Front. Psychol. 6, 731. doi:10.3389/fpsyg.2015.00731
Levitan, R., Beňuš, Š., Gravano, A., and Hirschberg, J. (2015). Entrainment and Turn-Taking in Human-Human Dialogue. Turn-taking Coord. Human- Machine Interaction: Pap. 2015 AAAI Spring Symp., 44–51.
Magyari, L., Bastiaansen, M. C. M., de Ruiter, J. P., and Levinson, S. C. (2014). Early Anticipation Lies behind the Speed of Response in Conversation. J. Cogn. Neurosci. 26, 2530–2539. doi:10.1162/jocn_a_00673
McClave, E. Z. (2000). Linguistic Functions of Head Movements in the Context of Speech. J. Pragmatics 32, 855–878. doi:10.1016/S0378-2166(99)00079-X
Melinger, A., and Kita, S. (2007). Conceptualisation Load Triggers Gesture Production. Lang. Cogn. Process. 22, 473–500. doi:10.1080/01690960600696916
Mondada, L. (2007). Multimodal Resources for Turn-Taking. Discourse Stud. 9, 194–225. doi:10.1177/1461445607075346
Munhall, K. G., Jones, J. A., Callan, D. E., Kuratate, T., and Vatikiotis-Bateson, E. (2004). Visual Prosody and Speech Intelligibility. Psychol. Sci. 15, 133–137. doi:10.1111/j.0963-7214.2004.01502010.x
Munhall, K. G., Ostry, D. J., and Parush, A. (1985). Characteristics of Velocity Profiles of Speech Movements. J. Exp. Psychol. Hum. Perception Perform. 11, 457–474. doi:10.1037/0096-1523.11.4.457
Nota, N., Trujillo, J. P., and Holler, J. (2021). Facial Signals and Social Actions in Multimodal Face-To-Face Interaction. Brain Sci. 11, 1017. doi:10.3390/brainsci11081017
Ostry, D. J., Cooke, J. D., and Munhall, K. G. (1987). Velocity Curves of Human Arm and Speech Movements. Exp. Brain Res. 68, 37–46. doi:10.1007/BF00255232
Özyürek, A., Kita, S., Allen, S. E. M., Furman, R., and Brown, A. (2005). How Does Linguistic Framing of Events Influence Co-speech Gestures? Gest 5, 219–240. doi:10.1075/gest.5.1.15ozy
Prieto, P., Puglesi, C., Borràs-Comes, J., Arroyo, E., and Blat, J. (2015). Exploring the Contribution of Prosody and Gesture to the Perception of Focus Using an Animated Agent. J. Phonetics 49, 41–54. doi:10.1016/j.wocn.2014.10.005
R Core Team (2021). R: A Language and Environment for Statistical Computing. Available at: https://www.gbif.org/tool/81287/r-a-language-and-environment-for-statistical-computing. doi:10.1007/978-3-540-74686-7
Roberts, S. n. G., Torreira, F., and Levinson, S. C. (2015). The Effects of Processing and Sequence Organization on the Timing of Turn Taking: a Corpus Study. Front. Psychol. 6, 509. doi:10.3389/fpsyg.2015.00509
Rochet-Capellan, A., and Fuchs, S. (2014). Take a Breath and Take the Turn: How Breathing Meets Turns in Spontaneous Dialogue. Phil. Trans. R. Soc. B 369, 20130399. doi:10.1098/rstb.2013.0399
Ruiter, J.-P. d., Mitterer, H., and Enfield, N. J. (2006). Projecting the End of a Speaker's Turn: A Cognitive Cornerstone of Conversation. Language 82, 515–535. doi:10.1353/lan.2006.0130
Scobbie, J. M., Turk, A., Geng, C., King, S., Lickley, R., and Richmond, K. (2013). “The edinburgh Speech Production Facility Doubletalk Corpus,” in Proceedings of the Annual Conference of the International Speech Communication Association, August 2013, 764–766.
Sikveland, R. O., and Ogden, R. (2012). Holding Gestures across Turns. Gest 12, 166–199. doi:10.1075/gest.12.2.03sik
Singmann, H., Bolker, B., Westfall, J., Aust, F., and Ben-Shachar, M. S. (2021). Afex: Analysis of Factorial Experiments. R package version 1.0-1. Available at: https://CRAN.R-project.org/package=afex.
Stivers, T., Enfield, N. J., Brown, P., Englert, C., Hayashi, M., Heinemann, T., et al. (2009). Universals and Cultural Variation in Turn-Taking in Conversation. Proc. Natl. Acad. Sci. 106, 10587–10592. doi:10.1073/pnas.0903616106
Tiede, M., Bundgaard-Nielsen, R., Kroos, C., Gibert, G., Attina, V., Kasisopa, B., et al. (2010). Speech Articulator Movements Recorded from Facing Talkers Using Two Electromagnetic Articulometer Systems Simultaneously. J. Acoust. Soc. America 128, 1–9. doi:10.1121/1.3508805
Trujillo, J. P., Levinson, S. C., and Holler, J. (2021). “Visual Information in Computer-Mediated Interaction Matters: Investigating the Association between the Availability of Gesture and Turn Transition Timing in Conversation,” in Human-Computer Interaction. Design and User Experience Case Studies. HCII 2021. Lecture Notes in Computer Science. Editor M. Kurosu (Berlin/Heidelberg, Germany: Springer), 12764, 643–657. doi:10.1007/978-3-030-78468-3_44
Vilela Barbosa, A., Déchaine, R.-M., Vatikiotis-Bateson, E., and Camille Yehia, H. (2012). Quantifying Time-Varying Coordination of Multimodal Speech Signals Using Correlation Map Analysis. J. Acoust. Soc. America 131, 2162–2172. doi:10.1121/1.3682040
Voigt, R., Eckert, P., Jurafsky, D., and Podesva, R. J. (2016). Cans and Cants: Computational Potentials for Multimodality with a Case Study in Head Position. J. Sociolinguistics 20, 677–711. doi:10.1111/josl.12216
Wagner, P., Malisz, Z., and Kopp, S. (2014). Gesture and Speech in Interaction: An Overview. Speech Commun. 57, 209–232. doi:10.1016/j.specom.2013.09.008
Wickham, H., Averick, M., Bryan, J., Chang, W., McGowan, L., François, J., et al. (2019). Welcome to the Tidyverse. J. Open Source Softw. 4 (43), 1686. doi:10.21105/joss.01686
Yehia, H., Rubin, P., and Vatikiotis-Bateson, E. (1998). Quantitative Association of Vocal-Tract and Facial Behavior. Speech Commun. 26, 23–43. doi:10.1016/s0167-6393(98)00048-x
Yuan, J., and Liberman, M. (2008). Speaker Identification on the SCOTUS Corpus. Proc. Acoust. ’08, 6–9. doi:10.1121/1.2935783
Keywords: turn-taking, multimodal speech, head movement, brow movement, conversational interaction
Citation: Danner SG, Krivokapić J and Byrd D (2021) Co-Speech Movement in Conversational Turn-Taking. Front. Commun. 6:779814. doi: 10.3389/fcomm.2021.779814
Received: 19 September 2021; Accepted: 17 November 2021;
Published: 16 December 2021.
Edited by:
Martine Grice, University of Cologne, GermanyReviewed by:
James P. Trujillo, Radboud University Nijmegen, NetherlandsMathias Barthel, Humboldt University of Berlin, Germany
Copyright © 2021 Danner, Krivokapić and Byrd. This is an open-access article distributed under the terms of the Creative Commons Attribution License (CC BY). The use, distribution or reproduction in other forums is permitted, provided the original author(s) and the copyright owner(s) are credited and that the original publication in this journal is cited, in accordance with accepted academic practice. No use, distribution or reproduction is permitted which does not comply with these terms.
*Correspondence: Samantha Gordon Danner, sgdanner@usc.edu