GWAS Reveal Targets in Vessel Wall Pathways to Treat Coronary Artery Disease
- 1Center for Public Health Genomics, University of Virginia, Charlottesville, VA, United States
- 2Department of Biochemistry and Molecular Genetics, University of Virginia, Charlottesville, VA, United States
- 3Data Science Institute, University of Virginia, Charlottesville, VA, United States
- 4Department of Public Health Sciences, University of Virginia, Charlottesville, VA, United States
Coronary artery disease (CAD) is the leading cause of mortality worldwide and poses a considerable public health burden. Recent genome-wide association studies (GWAS) have revealed >100 genetic loci associated with CAD susceptibility in humans. While a number of these loci harbor gene targets of currently approved therapies, such as statins and PCSK9 inhibitors, the majority of the annotated genes at these loci encode for proteins involved in vessel wall function with no known drugs available. Importantly many of the associated genes linked to vascular (smooth muscle, endothelial, and macrophage) cell processes are now organized into distinct functional pathways, e.g., vasodilation, growth factor responses, extracellular matrix and plaque remodeling, and inflammation. In this mini-review, we highlight the most recently identified loci that have predicted roles in the vessel wall and provide genetic context for pre-existing therapies as well as new drug targets informed from GWAS. With the development of new modalities to target these pathways, (e.g., antisense oligonucleotides, CRISPR/Cas9, and RNA interference) as well as the computational frameworks to prioritize or reposition therapeutics, there is great opportunity to close the gap from initial genetic discovery to clinical translation for many patients affected by this common disease.
Introduction
Coronary artery disease (CAD) is a maladaptive inflammatory disease of the coronary artery vessel wall that remains one of the leading causes of death worldwide. It involves numerous cell types (smooth muscle cells, endothelial cells, and macrophages) and often manifests in myocardial infarction. Development of CAD is due to a combination of genetic and environmental factors. Early twin studies indicated CAD heritability was ~40-60% (1, 2). Linkage and family-based studies identified genes with now well-established roles in disease pathogenesis, such as the LDL receptor (LDLR) (3), apolipoprotein B (apoB) (4), and proprotein convertase subtilisin/kexin type 9 (PCSK9) (5).
In 2007 the first genome-wide association studies (GWAS) of CAD published the association of the 9p21 locus with both CAD and myocardial infarction (MI) (6–8). The 9p21 locus remains the most robust locus in the genome with respect to CAD association. Many more CAD loci have been discovered in subsequent GWAS over the past decade, leading to the formation of the CARDIoGRAM (9) and C4D (10) consortia and resulting meta-analyses (11–15). The most recent GWAS meta-analysis for CAD has ~300,000 combined cases and controls and identified almost 100 independent loci reaching genome-wide significance (p < 5 × 10−8), and over 300 loci significant at a 5% false discovery rate.
Despite the discovery of many new loci associated with CAD, the current challenges are to validate the causal genes and pathways at CAD loci and to translate this knowledge into new therapies. In this mini-review, we highlight recent GWAS identified non-lipid genes and pathways (with an emphasis on vessel wall pathways) that have the potential to accelerate new treatments for CAD (Figure 1). In addition, we provide some genetic perspective on currently approved and future therapies, as well as the use of genetic risk scores (GRS) to identify high risk patients who may require these novel treatments to augment traditional lipid-lowering therapy.
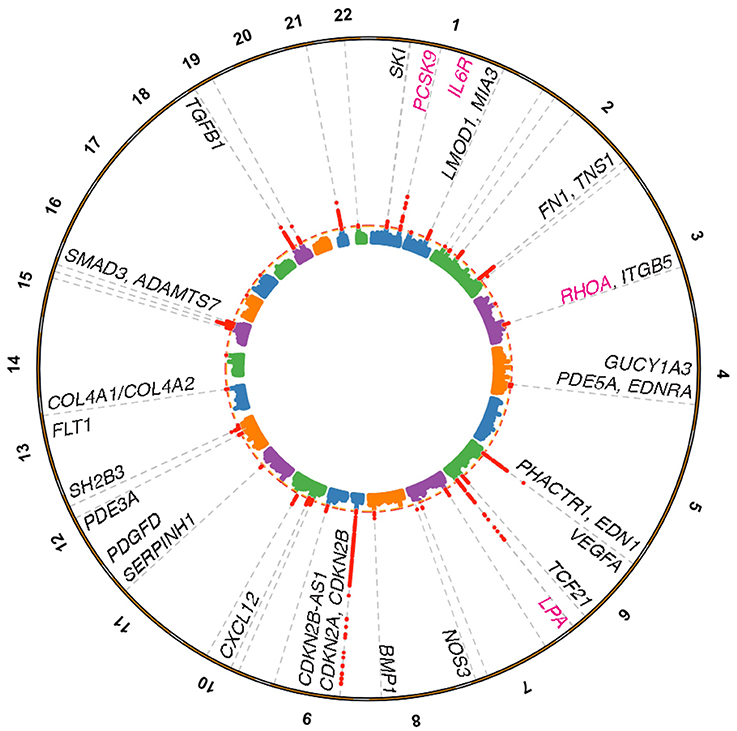
Figure 1. Coronary artery disease loci harboring genes linked to vessel wall functions. Manhattan plot depicting genome-wide significant loci identified from the Nelson et al. (15) meta-analysis for CAD based on a 5% false discovery rate using the pilot UK Biobank data. Loci were annotated through a combination of gene expression, epigenomic features, eQTL, and literature based searches. Vessel wall gene symbols are shown above associations in red, with dotted orange line representing P = 5.0 × 10−8. Pink gene symbols represent loci with either approved or evaluated treatments for CAD.
CAD GWAS Genes and Pathways
Vessel Wall Signaling
Once atherogenic lipoproteins have crossed the endothelium and are taken up by macrophage-derived foam cells, there is a subsequent cascade of complex signaling events in the vessel wall. This involves a tightly orchestrated interplay of vascular smooth muscle cells, endothelial cells, macrophages, cytokines, and extracellular matrix proteins. Reactome pathway gene-set enrichment analysis carried out by the CARDIoGRAM consortium indicated that CAD genes were enriched for pathways involved in NO/cGMP signaling, TGFβ/SMAD signaling, PDGF signaling, extracellular matrix (ECM) integrity/organization, and innate immunity (16). Further integrative analyses of CARDIoGRAM summary data, tissue-specific regulatory networks and gene expression data have revealed interactions across CAD-relevant pathways as well as potential druggable targets such as LUM and STAT3, which serve as key regulators of vessel wall biology (17). Assuming that the genes in these pathways are the most likely causal genes at the associated loci, these results argue that vascular wall pathways have comparable associations to the well-established lipid and lipoprotein mediated pathways (16). In fact up to 75% of the 95 CAD loci (15) appear to be associated independently of classical risk factors. This observation suggests that these risk factors are intrinsic to dysregulated processes in the vessel wall.
NO/cGMP Signaling
NO/cGMP signaling is fundamental to diverse cardiovascular physiological responses and emerging evidence suggests that activation of this pathway is defective in the setting of atherosclerosis and CAD. Nitric oxide (NO) is an important gas that is synthesized by endothelial nitric oxide synthase (eNOS), which upon activation results in paracrine signaling through the myoendothelial junction to smooth muscle cells, subsequent activation of soluble guanylate cyclase, cGMP production, and cGMP-dependent protein kinase (protein kinase G; PKG) mediated phosphorylation of downstream targets involved in vasodilation. The 1000 Genomes based CARDIoGRAMplusC4D (12) and recent UK Biobank-CARDIoGRAMplusC4D meta-analysis (15) identified an association for rs3918226 at NOS3, the gene which encodes eNOS, implicating a role in endothelial dysfunction. An intronic variant rs7692387 in GUCY1A3, encoding the alpha1-subunit of sGC, was associated with CAD (11), while another variant rs13139571 was associated with systolic (SBP) and diastolic (DBP) blood pressure (18). Recent functional studies identified a mechanism by which the non-risk allele at rs7692387 preferentially binds the ZEB1 transcription factor leading to increased GUCY1A3 expression and sGC levels, which correlated with reduced atherosclerosis severity in mice (19). Other members of this pathway that have been linked to CAD include recently identified PDE5A (rs7678555) (15) and PDE3A, previously associated at 5% FDR (11), suggesting alterations in vascular wall signaling could be rescued with existing therapies (e.g., sildenafil, ciloztasol).
TGFβ and PDGF Signaling
The CARDIoGRAM GWAS studies have implicated several components of the transforming growth factor beta (TGFβ) signaling pathway in CAD. Activated TGFβ receptor I phosphorylates receptor-regulated SMAD proteins (SMAD3 or SMAD2). These are transcriptional mediators of TGFβ signaling that along with SMAD4 translocate to the nucleus to regulate transcription of TGFβ target genes. The TGFβ1 and SMAD3 genes are both associated with CAD in addition to bone morphogenic protein 1 (BMP1), a member of the TGF beta superfamily (20). Mechanistic studies have implicated a functional intronic SNP in SMAD3 (rs17293632) that disrupts binding of the AP-1 transcription factor complex underlying this association (21, 22). The genetic association of rs36096196 at the SKI locus suggests a role for SKI, a co-repressor of SMAD3/SMAD2 signaling in CAD (23).
The rs150512726 SNP [proxy for the recently reported SNP rs142695226 (15)] results in a 3 amino acid deletion in the integrin beta 5 (ITGB5) protein. ITGB5 has been shown to play a role in activation of the latent TGFβ precursor protein outside the cell (24). The TGFβ pathway also regulates gene expression at the 9p21 locus. SNPs at this locus disrupt TEAD factor binding and the TEAD3-dependent TGF beta induction of p16 in human aortic smooth muscle cells (25).
The CARDIoGRAM studies have also identified SNPs at the platelet-derived growth factor D (PDGFD) locus associated with CAD at genome-wide significance. This PDGF mediated pathway may involve many other risk-associated genes. Preliminary work by our group has provided evidence of cross-talk with smooth muscle cell enriched pathways using genome-wide profiling of these cells. For example, the expression of TCF21, a transcription factor which determines the fate of epicardial progenitor cells during development, is increased in individuals carrying the risk alleles, rs121902987 and rs12524865 (26). Its expression was positively regulated by PDGF-BB-PDGFRB stimulation in human coronary artery smooth muscle cells (26). TCF21 dysregulation likely increases CAD risk by altering coronary artery smooth muscle cell responses to vascular injury during plaque remodeling (27, 28). Another vessel wall gene, LMOD1, an actin filament nucleator, was shown to be downregulated in vascular smooth muscle cells in response to PDGF treatment and serves as a potent marker of smooth muscle cell phenotypic modulation (29).
Extracellular Matrix Remodeling Pathways
The CARDIoGRAM consortium has highlighted numerous extracellular matrix and basement membrane genes involved in the pathogenesis of atherosclerosis, including COL4A1/COL4A2, ITGB5, and FN1. A COL4A2 variant, rs4773144, was associated with both COL4A1 and COL4A2 expression, as well as smooth muscle cell survival, and plaque stability (30). The authors suggest type IV collagen levels affect SMC proliferation, migration, extracellular matrix remodeling, apoptosis, and infiltration of immune cells through plaque remodeling. The CAD locus MIA3 is involved in the endoplasmic reticulum export of large cargo such as pre-chylomicrons/VLDL (31) and collagens (including Col4a1 and Col4a2 in mice) (32). The CAD locus SERPINH1 encodes heat-shock protein 47 (Hsp47) (33), a molecular chaperone involved in the collagen secretion pathway. FN1 encodes fibronectin, a glycoprotein with established roles in cell adhesion, migration, growth, and differentiation. Though increased in atherosclerotic regions, the role of fibronectin in development of CAD remains unclear, with postulated roles in atherogenic lipoprotein retention, direct adverse effects on endothelial cell function, or roles in plaque stability (34). The TNS1 gene encodes for the tensin-1 protein that attaches the plasma membrane to the extracellular matrix and positively regulates the small Rho GTPase, RhoA (35). The RHOA gene itself was identified as a genome-wide significant locus in the latest CARDIoGRAMplusC4D meta-analysis (15) and is predicted to interact with several other CAD genes/pathways in smooth muscle cells and endothelial cells including TGFβ/SMAD3 and ECM proteins, such as collagens and fibronectin (36). RhoA also cooperates with Rac1 and cadherin to regulate barrier function in mural and endothelial cells (37). RhoA activation coincides with endothelial cell inflammation, permeability, and disturbed flow as a result of reduced PPAP2B (itself associated with CAD and ischemic stroke) (38). Lastly, the ADAMTS7 gene, encoding a metalloproteinase, is proatherogenic based on mouse studies, with a direction of effect consistent with the human genetic association data (39). In the context of its association with CAD, it has been proposed that ADAMTS7 alters smooth muscle cell migration and extracellular matrix composition (40).
Inflammation and Immune Pathways
The role of inflammation in CAD pathogenesis is now well-established, yet the number of inflammatory genes mapping to CAD-associated loci is under-represented. One of the main CAD loci involved in inflammation is the interleukin 6 receptor (IL6R), which binds the pro-inflammatory cytokine IL-6 and its pathways have been causally linked to CAD using Mendelian randomization analyses (41). Another example is the CAD-associated CXCL12 gene, which encodes an anti-inflammatory cytokine (also known as stromal derived factor 1; SDF-1) that binds the chemokine (C-X-C motif) receptor CXCR4, a G-protein coupled receptor. Given that CXCL12 is induced immediately after vessel injury and specifically expressed in atherosclerotic lesions, this gene has potential to serve as a biomarker for early detection (42). The CAD-associated SH2B3 gene encoding an adapter protein known as LNK is involved in hematopoiesis and suppression of cytokines and thrombopoietin signaling (43). In mice, loss of Sh2b3 was shown to promote both atherosclerosis and thrombosis only under the setting of hypercholesterolemia, suggesting an involvement in platelet/leukocyte activation during atherogenesis (44). It may also serve as an inflammatory link between vascular endothelial cells and immune cells and therapeutic target for hypertension and end-organ inflammation (45). Finally, the ligand VEGFA and the VEGF receptor (FLT1) loci both associate with CAD; inflammatory conditions in the plaque promote the release of angiogenic factors that result in neovascularization, plaque remodeling, and plaque instability (46).
Current Therapies for CAD
Current therapies for CAD primarily focus on alleviating the symptoms of ischemic events as well as preventing thrombosis from ruptured plaque. Here we review the current treatments for CAD and also provide a genetically informed perspective on these drug targets (Table 1).
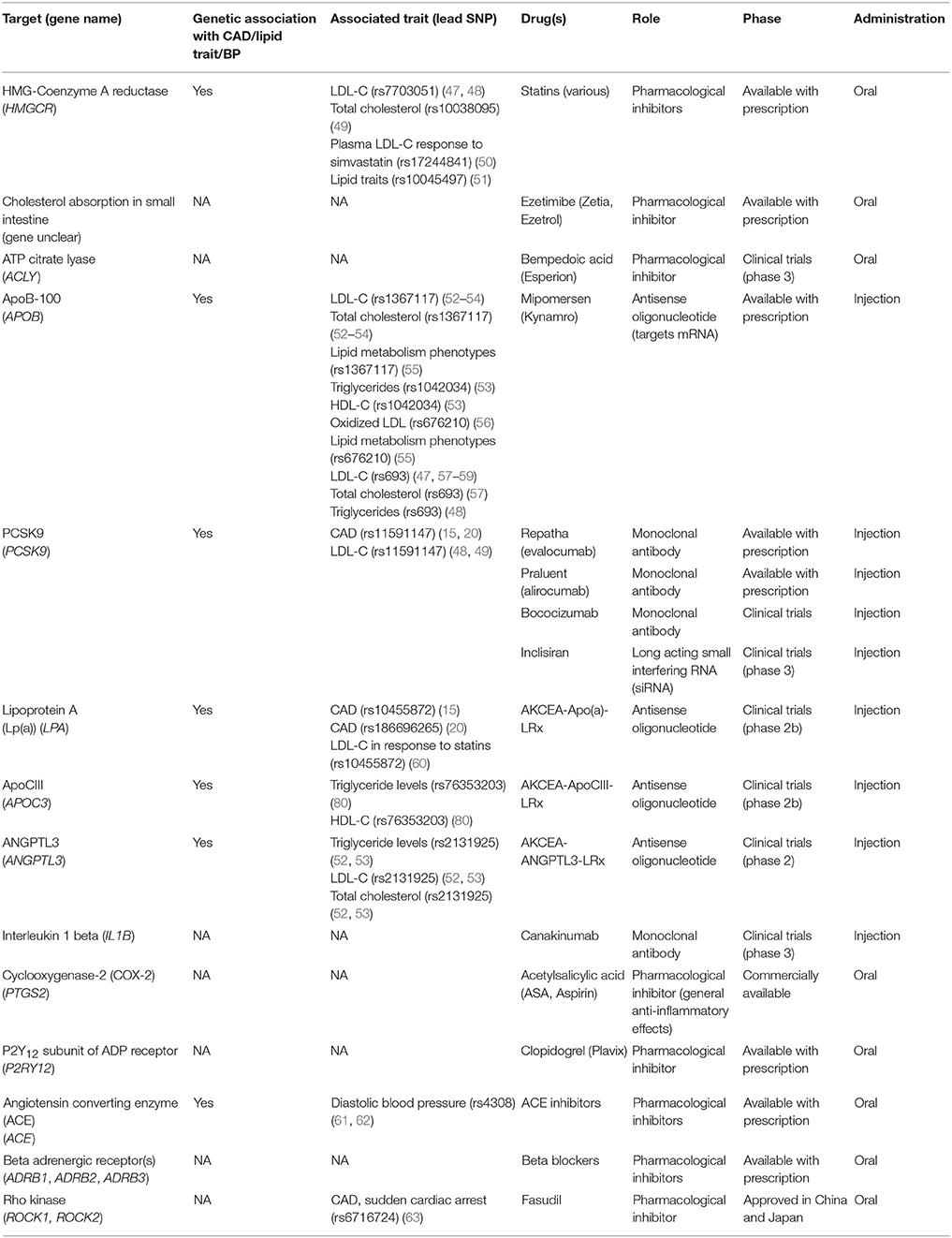
Table 1. List of current target genes for management of coronary artery disease and their genetic associations.
Statins
Statins represent the first line of treatment for elevated LDL-cholesterol levels associated with hyperlipidemia and CAD. By inhibiting HMG-coA (hydroxy-3-methylglutaryl-coenzyme A) reductase, statins decrease the production of cholesterol in the liver, thereby reducing its concentration in the circulation. Statins exhibit a pleiotropic effect by attenuating other risk factors for CAD (64). Genetic studies have identified variations in the HMGCR gene (rs12916) consistently associated with both blood lipids and LDL-cholesterol (52, 53), while an intergenic variant near HMGCR is also associated with CAD in the combined CARDIoGRAMplusC4D and UK Biobank analysis (20).
Anti-Platelet Therapies
As a prophylactic measure against thrombosis, antiplatelet drugs are utilized to reduce the risk of myocardial infarction. Two of the more popular antiplatelet drugs are acetylsalicylic acid (ASA) and clopidogrel. ASA is a COX inhibitor that prevents platelet activation by inhibiting the synthesis of thromboxane A2. On the other hand, clopidogrel is an ADP receptor (P2Y12) antagonist that prevents platelet aggregation and further amplification of the activation signal through the downregulation of glycoprotein IIb/IIIa receptor on its surface (65). While the gene targets of these drugs (PTSG2 and P2Y12) do not harbor variants specifically associated with CAD, some of the effector signaling molecules in the pathway (RHOA, ITGB5, and SH2B3) indeed have CAD associations, as described above. This may represent an opportunity to understand some of the heterogeneity in responses to these commonly used agents by using a pathway approach.
ACE Inhibitors and Beta Blockers
Two classes of drugs, angiotensin converting enzymes (ACE) inhibitors and beta blockers both function in the maintenance of normal blood pressure. In the endothelium, ACE catalyzes the conversion of angiotensin I to angiotensin II where the latter is a potent vasoconstrictor. Additionally, ACE upregulation results in the degradation of bradykinin, a vasodilatory factor involved in the upregulation of nitric oxide and prostaglandins (66). Given the numerous CAD associations within the NO/cGMP pathway, the efficacy or toxicity profile of these drugs may be influenced by individual genetic variation. Beta blockers exert their cardioprotective effects by intervening in the adrenergic nervous system as competitive antagonists in both the myocardium and vasculature, depending on their selectivity for beta1 or beta2-adrenergic receptors. Clinically, reduced catecholamine stimulation results in decreased cardiac stress leading to decreased heart rate and blood pressure (67, 68). The third generation of beta blockers were shown to have more potent blood pressure lowering effects. Although it may be reasonable to speculate that NO mediated signaling is involved, it was recently demonstrated that nebivolol (compared to metoprolol) suppresses ET-1 mediated vasoconstriction to lower BP (69). This is important given that variation at the ET-1 gene EDN1 (rs1629862) and the ET-1 receptor type A gene EDNRA (rs6841581) were recently identified as CAD loci (20).
Anti-Inflammatory Therapies
Therapies targeting inflammatory pathways have been extensively explored in cardiovascular disease. Two recent studies investigating the role of clonal expansion of hematopoietic cells as a potential driver for age-related onset of atherosclerosis have provided evidence that IL1β secretion from TET2 deficient macrophages plays a role in the acceleration of disease (70, 71). TET2 is an epigenetic modifier that negatively regulates the expression of IL1β. Thus, loss of function of TET2 results in the upregulation of IL1β and IL-6 secretion from lesional macrophages (70). This elevated level of proinflammatory cytokines was positively correlated with increased plaque size in the aorta as well as severity of coronary artery calcification in mice and human patients, respectively(70, 71). Studies such as these underscore the potential of targeting the IL1β pathway in slowing down atherosclerosis progression.
The CANTOS (NCT01327846) clinical trial provided critical evidence that targeting IL1β alone with the monoclonal antibody canakinumab can reduce major cardiovascular events along with proinflammatory cytokines (IL-6) and high sensitivity C reactive protein in patients with atherosclerosis. Although the intermediate dose (150 mg) met the primary endpoint of reducing nonfatal myocardial infarction, nonfatal stroke, or cardiovascular death, a significant risk of fatal infection relative to placebo was observed (72). In addition to the high pricing and safety concerns, the marginal clinical benefits demand more development in this area. Given that IL-6 is a causal risk factor for CAD (73), anti-inflammatory therapies remain an attractive therapeutic approach for patients that do not respond to standard lipid lowering medication.
New CAD Therapies Informed From Genetic Studies
PCSK9 Inhibitors and Antisense Oligonucleotides
One example of newly approved drug targets that have origins in genetic studies is the development of monoclonal antibodies against PCSK9. PCSK9 is a liver protease that targets LDL receptors for lysosomal degradation. The therapeutic potential of targeting PCSK9 was validated through Mendelian randomization studies that correlated a deleterious mutation in this gene with decreased risk (74). Large clinical trials [e.g., FOURIER (NCT01764633), ODYSSEY (NCT01623115)] demonstrated that inhibition of this enzyme reduced systemic LDL levels to a greater extent than maximum statin therapy, with the most recent ODYSSEY trial (NCT01663402) reporting a reduction in both cardiovascular events and all-cause mortality for the first time. In addition to monoclonal antibodies, antisense oligonucleotides have also been developed against PCSK9, which should be evaluated for clinical outcomes in the near future.
Lipoprotein A and APOC3 Antisense Oligonucleotides
A high level of circulating lipoprotein A [Lp(a)] is considered a risk factor for cardiovascular disease. Two SNPs, rs3798220 and rs10455872, located within the lipoprotein A (LPA) gene correlate with increased levels of Lp(a) and are associated with increased risk for CAD. As one of the first therapies targeting lipoprotein A, AKCEA-APO(a)-LRx is an antisense oligonucleotide that binds LPA mRNA leading to its degradation. Phase 2 clinical trial data has suggested that this approach is well tolerated and significantly reduced Lp(a) plasma concentrations (65, 66). Similarly, an antisense therapy was developed targeting APOC3, a gene involved in regulating plasma triglyceride levels. The antisense oligonucleotide therapy, volanesorsen was shown to reduce cellular levels of APOC3 and led to an overall reduction of triglyceride levels in phase 3 clinical trials (70, 71).
RhoA-ROCK Inhibition
The RhoA-Rock signaling pathway offers another avenue for CAD therapeutic targets. Aberrant activation of this signaling cascade has been implicated in vasoconstriction and endothelial dysfunction. Given the recent CAD association (rs7623687) at RHOA, further investigation is warranted to determine how to specifically target this gene. One opportunity is to target the downstream effectors, Rho-associated protein kinases (ROCK1, ROCK2), which control actin cytoskeleton arrangement, cell migration and contractility (75). In particular, a Rock2 inhibitor, Fasudil, has already been tested in clinical trials as a possible therapeutic for CAD as a vasodilatory agent through the upregulation of nitric oxide. It is also noteworthy to mention that Fasudil has been approved as a treatment option for cerebral vasospasm in Japan and China (75).
Perspectives and Future Directions
Genetic Risk Scores
Besides the 9p21 locus, most loci uncovered from GWAS of CAD have small effects with odds ratios between 1.05 and 1.30. Nonetheless, GWAS results can be utilized to generate genetic risk scores for individuals based on the number of risk alleles they harbor. Therefore, in addition to traditional drug treatments such as statins, individuals that fall within the high CAD risk range based on their genetic risk score can be selected for more aggressive therapies and/or novel CAD treatments as mentioned above. With more data from sources such as the UK Biobank, the Million Veterans Project, and the NIH-funded All of Us project on the horizon, genetic risk scores will have more clinically-relevant predictive utility (76).
Feasible vs. Difficult Drug Targets
Since GWAS has highlighted the role of vessel wall genes and signaling pathways in the pathogenesis of CAD, it will be critical to apply this knowledge toward vessel wall therapeutic development. Strategies include non-specific targeting of the vessel wall (through upstream or downstream effector molecules), specifically targeting plaque vasculature, or specific cellular phenotypes (e.g., activated resident macrophages or phenotypically modulated smooth muscle cells).
Target New Cell Types (e.g., Endothelial Cells, Smooth Muscle Cells, Macrophages)
A CAD protective variant upstream of ADAMTS7 confers greater protection against CAD for never-smokers compared to those that have smoked 100 or more cigarettes in their lifetime (77). This example highlights the importance of taking into account environmental factors in managing treatments. Other potential targets include the receptors for endothelin-1 on smooth muscle cells. Many of these potential vessel wall target proteins affect smooth muscle cell proliferation and migration, originally believed to drive atherogenesis. The current view suggests smooth muscle cell proliferation and migration could be reparative and promote plaque stability (78). Once the roles and timing of smooth muscle cell proliferation and migration are clarified, the TGF beta and PDGF pathways may be attractive targets due to their role in the regulation of smooth muscle cell genes.
Machine Learning/Systems Approaches
While GWAS has uncovered invaluable insights into potential therapies and validated existing ones, these associations require extensive follow-up to pinpoint causal variants, genes, pathways. More advanced algorithms such as machine learning can be leveraged to prioritize targets with diverse data inputs such as electronic health records, clinical notes, and -omics. These approaches can help to systematically decrease noise, reduce features, and identify gene sets of interest in addition to common GWAS methods of odds ratios, p-value statistics, and chi-square comparisons. Unsupervised learning algorithms have the capability to provide researchers and clinicians with an unbiased network of candidate genes that account for the greatest variance in CAD related phenotypes. A specific example is the use of machine learning for drug repurposing based on finding patterns from multi-dimensional datasets. Specific tools have been developed to provide an out-of-the-box approach for understanding diverse text, biological, and medical record data for non-data scientists. One such tool, RepurposeDB, combines drug and disease information to create a reference database for drug repositioning research (79). With the rapidly growing costs of drug discovery/development, such data-informed approaches can offer significant progress for the field.
Concluding Remarks
In summary, in this brief review we bring attention to the genetic loci discovered over the past decade which play critical roles in the vessel wall. Many of these genes are organized into distinct functional pathways, which will help redefine some of the pathogenic mechanisms and prioritize those pathways for future drug development or repurposing strategies.
Author Contributions
AT and CM conceived of the manuscript. AT, DW, CD, and CM wrote the manuscript.
Funding
Funding support provided by the National Institutes of Health (R00 HL125912) to CM.
Conflict of Interest Statement
The authors declare that the research was conducted in the absence of any commercial or financial relationships that could be construed as a potential conflict of interest.
References
1. Zdravkovic S, Wienke A, Pedersen NL, Marenberg ME, Yashin AI, De Faire U. Heritability of death from coronary heart disease: a 36-year follow-up of 20 966 Swedish twins. J Intern Med. (2002) 252:247–54. doi: 10.1046/j.1365-2796.2002.01029.x
2. Lloyd-Jones DM, Nam B-H, D'Agostino RB Sr, Levy D, Murabito JM, Wang TJ, et al. Parental cardiovascular disease as a risk factor for cardiovascular disease in middle-aged adults. JAMA (2004) 291:2204. doi: 10.1001/jama.291.18.2204
3. Watts GF, Lewis B, Sullivan DR. Familial hypercholesterolemia: a missed opportunity in preventive medicine. Nat Clin Pract Cardiovasc Med. (2007) 4:404–5. doi: 10.1038/ncpcardio0941
4. Innerarity TL, Mahley RW, Weisgraber KH, Bersot TP, Krauss RM, Vega GL, et al. Familial defective apolipoprotein B-100: a mutation of apolipoprotein B that causes hypercholesterolemia. J Lipid Res. (1990) 31:1337–49.
5. Abifadel M, Varret M, Rabès J-P, Allard D, Ouguerram K, Devillers M, et al. Mutations in PCSK9 cause autosomal dominant hypercholesterolemia. Nat Genet. (2003) 34:154–6. doi: 10.1038/ng1161
6. McPherson R, Pertsemlidis A, Kavaslar N, Stewart A, Roberts R, Cox DR, et al. A common allele on chromosome 9 associated with coronary heart disease. Science (2007) 316:1488–91. doi: 10.1126/science.1142447
7. Helgadottir A, Thorleifsson G, Manolescu A, Gretarsdottir S, Blondal T, Jonasdottir A, et al. A common variant on chromosome 9p21 affects the risk of myocardial infarction. Science (2007) 316:1491–3. doi: 10.1126/science.1142842
8. Samani NJ, Erdmann J, Hall AS, Hengstenberg C, Mangino M, Mayer B, et al. Genomewide association analysis of coronary artery disease. N Engl J Med. (2007) 357:443–53. doi: 10.1056/NEJMoa072366
9. Schunkert H, König IR, Kathiresan S, Reilly MP, Assimes TL, Holm H, et al. Large-scale association analysis identifies 13 new susceptibility loci for coronary artery disease. Nat Genet. (2011) 43:333–8. doi: 10.1038/ng.784
10. Artery Disease (C4D) Genetics Consortium. A genome-wide association study in Europeans and South Asians identifies five new loci for coronary artery disease. Nat Genet. (2011) 43:339–44. doi: 10.1038/ng.782
11. CARDIoGRAMplusC4D Consortium, Deloukas P, Kanoni S, Willenborg C, Farrall M, Assimes TL, et al. Large-scale association analysis identifies new risk loci for coronary artery disease. Nat Genet. (2013) 45:25–33. doi: 10.1038/ng.2480
12. Nikpay M, Goel A, Won H-H, Hall LM, Willenborg C, Kanoni S, et al. A comprehensive 1,000 Genomes-based genome-wide association meta-analysis of coronary artery disease. Nat Genet. (2015) 47:1121–30. doi: 10.1038/ng.3396
13. Webb TR, Erdmann J, Stirrups KE, Stitziel NO, Masca NGD, Jansen H, et al. Systematic evaluation of pleiotropy identifies 6 further loci associated with coronary artery disease. J Am Coll Cardiol. (2017) 69:823–36. doi: 10.1016/j.jacc.2016.11.056
14. Howson JMM, Zhao W, Barnes DR, Ho W-K, Young R, Paul DS, et al. Fifteen new risk loci for coronary artery disease highlight arterial-wall-specific mechanisms. Nat Genet. (2017) 49:1113–9. doi: 10.1038/ng.3874
15. Nelson CP, Goel A, Butterworth AS, Kanoni S, Webb TR, Marouli E, et al. Association analyses based on false discovery rate implicate new loci for coronary artery disease. Nat Genet. (2017) 49:1385–91. doi: 10.1038/ng.3913
16. Ghosh S, Vivar J, Nelson CP, Willenborg C, Segrè AV, Mäkinen V-P, et al. Systems genetics analysis of genome-wide association study reveals novel associations between key biological processes and coronary artery disease. Arterioscler Thromb Vasc Biol. (2015) 35:1712–22. doi: 10.1161/ATVBAHA.115.305513
17. Zhao Y, Chen J, Freudenberg JM, Meng Q, Rajpal DK, Yang X. Network-based identification and prioritization of key regulators of coronary artery disease loci. Arterioscler Thromb Vasc Biol. (2016) 36:928–41. doi: 10.1161/ATVBAHA.115.306725
18. International Consortium for Blood Pressure Genome-Wide Association Studies, Ehret GB, Munroe PB, Rice KM, Bochud M, Johnson AD, et al. Genetic variants in novel pathways influence blood pressure and cardiovascular disease risk. Nature (2011) 478:103–9. doi: 10.1038/nature10405
19. Kessler T, Wobst J, Wolf B, Eckhold J, Vilne B, Hollstein R, et al. Functional characterization of the GUCY1A3 coronary artery disease risk locus. Circulation (2017) 136:476–89. doi: 10.1161/CIRCULATIONAHA.116.024152
20. van der Harst P, Verweij N. Identification of 64 novel genetic loci provides an expanded view on the genetic architecture of coronary artery disease. Circ Res. (2018) 122:433–43. doi: 10.1161/CIRCRESAHA.117.312086
21. Turner AW, Martinuk A, Silva A, Lau P, Nikpay M, Eriksson P, et al. Functional analysis of a novel genome-wide association study signal in SMAD3 that confers protection from coronary artery disease. Arterioscler Thromb Vasc Biol. (2016) 36:972–83. doi: 10.1161/ATVBAHA.116.307294
22. Miller CL, Pjanic M, Wang T, Nguyen T, Cohain A, Lee JD, et al. Integrative functional genomics identifies regulatory mechanisms at coronary artery disease loci. Nat Commun. (2016) 7:12092. doi: 10.1038/ncomms12092
23. Xu W, Angelis K, Danielpour D, Haddad MM, Bischof O, Campisi J, et al. Ski acts as a co-repressor with Smad2 and Smad3 to regulate the response to type beta transforming growth factor. Proc Natl Acad Sci USA. (2000) 97:5924–9. doi: 10.1073/pnas.090097797
24. Worthington JJ, Klementowicz JE, Travis MA. TGFβ: a sleeping giant awoken by integrins. Trends Biochem Sci. (2011) 36:47–54. doi: 10.1016/j.tibs.2010.08.002
25. Almontashiri NAM, Antoine D, Zhou X, Vilmundarson RO, Zhang SX, Hao KN, et al. 9p21.3 Coronary artery disease risk variants disrupt TEAD transcription factor-dependent transforming growth factor β regulation of p16 expression in human aortic smooth muscle cells. Circulation (2015) 132:1969–78. doi: 10.1161/CIRCULATIONAHA.114.015023
26. Miller CL, Anderson DR, Kundu RK, Raiesdana A, Nürnberg ST, Diaz R, et al. Disease-related growth factor and embryonic signaling pathways modulate an enhancer of TCF21 expression at the 6q23.2 coronary heart disease locus. PLoS Genet. (2013) 9:e1003652. doi: 10.1371/journal.pgen.1003652
27. Nürnberg ST, Cheng K, Raiesdana A, Kundu R, Miller CL, Kim JB, et al. Coronary artery disease associated transcription factor TCF21 regulates smooth muscle precursor cells that contribute to the fibrous cap. PLoS Genet. (2015) 11:e1005155. doi: 10.1371/journal.pgen.1005155
28. Sazonova O, Zhao Y, Nürnberg S, Miller C, Pjanic M, Castano VG, et al. Characterization of TCF21 downstream target regions identifies a transcriptional network linking multiple independent coronary artery disease loci. PLoS Genet. (2015) 11:e1005202. doi: 10.1371/journal.pgen.1005202
29. Perisic Matic L, Rykaczewska U, Razuvaev A, Sabater-Lleal M, Lengquist M, Miller CL, et al. Phenotypic modulation of smooth muscle cells in atherosclerosis is associated with downregulation of LMOD1, SYNPO2, PDLIM7, PLN, and SYNM. Arterioscler Thromb Vasc Biol. (2016) 36:1947–61. doi: 10.1161/ATVBAHA.116.307893
30. Yang W, Ng FL, Chan K, Pu X, Poston RN, Ren M, et al. Coronary-heart-disease-associated genetic variant at the COL4A1/COL4A2 locus affects COL4A1/COL4A2 expression, vascular cell survival, atherosclerotic plaque stability and risk of myocardial infarction. PLoS Genet. (2016) 12:e1006127. doi: 10.1371/journal.pgen.1006127
31. Santos AJM, Nogueira C, Ortega-Bellido M, Malhotra V. TANGO1 and Mia2/cTAGE5 (TALI) cooperate to export bulky pre-chylomicrons/VLDLs from the endoplasmic reticulum. J Cell Biol. (2016) 213:343–54. doi: 10.1083/jcb.201603072
32. Wilson DG, Phamluong K, Li L, Sun M, Cao TC, Liu PS, et al. Global defects in collagen secretion in a Mia3/TANGO1 knockout mouse. J Cell Biol. (2011) 193:935–51. doi: 10.1083/jcb.201007162
33. Nagata K. Hsp47: a collagen-specific molecular chaperone. Trends Biochem Sci. (1996) 21:22–6. doi: 10.1016/S0968-0004(06)80023-X
34. Moore KJ, Fisher EA. The double-edged sword of fibronectin in atherosclerosis. EMBO Mol Med. (2012) 4:561–3. doi: 10.1002/emmm.201200238
35. Shih Y-P, Sun P, Wang A, Lo SH. Tensin1 positively regulates RhoA activity through its interaction with DLC1. Biochim Biophys Acta (2015) 1853:3258–65. doi: 10.1016/j.bbamcr.2015.09.028
36. Collins C, Osborne LD, Guilluy C, Chen Z, O'Brien ET, Reader JS, et al. Haemodynamic and extracellular matrix cues regulate the mechanical phenotype and stiffness of aortic endothelial cells. Nat Commun. (2014) 5:3984. doi: 10.1038/ncomms4984
37. Alimperti S, Mirabella T, Bajaj V, Polacheck W, Pirone DM, Duffield J, et al. Three-dimensional biomimetic vascular model reveals a RhoA, Rac1, and N-cadherin balance in mural cell-endothelial cell-regulated barrier function. Proc Natl Acad Sci USA. (2017) 114:8758–63. doi: 10.1073/pnas.1618333114
38. Wu C, Huang R-T, Kuo C-H, Kumar S, Kim CW, Lin Y-C, et al. Mechanosensitive PPAP2B regulates endothelial responses to atherorelevant hemodynamic forces. Circ Res. (2015) 117:e41–53. doi: 10.1161/CIRCRESAHA.117.306457
39. Bauer RC, Tohyama J, Cui J, Cheng L, Yang J, Zhang X, et al. Knockout of Adamts7, a novel coronary artery disease locus in humans, reduces atherosclerosis in mice. Circulation (2015) 131:1202–13. doi: 10.1161/CIRCULATIONAHA.114.012669
40. Pu X, Xiao Q, Kiechl S, Chan K, Ng FL, Gor S, et al. ADAMTS7 cleavage and vascular smooth muscle cell migration is affected by a coronary-artery-disease-associated variant. Am J Hum Genet. (2013) 92:366–74. doi: 10.1016/j.ajhg.2013.01.012
41. IL6R Genetics Consortium Emerging Risk Factors Collaboration, Sarwar N, Butterworth AS, Freitag DF, Gregson J, Willeit P, et al. Interleukin-6 receptor pathways in coronary heart disease: a collaborative meta-analysis of 82 studies. Lancet (2012) 379:1205–13. doi: 10.1016/S0140-6736(11)61931-4
42. van der Vorst EPC, Döring Y, Weber C. MIF and CXCL12 in cardiovascular diseases: functional differences and similarities. Front Immunol. (2015) 6:373. doi: 10.3389/fimmu.2015.00373
43. Norddahl GL, Wahlestedt M, Gisler S, Sigvardsson M, Bryder D. Reduced repression of cytokine signaling ameliorates age-induced decline in hematopoietic stem cell function. Aging Cell (2012) 11:1128–31. doi: 10.1111/j.1474-9726.2012.00863.x
44. Wang W, Tang Y, Wang Y, Tascau L, Balcerek J, Tong W, et al. LNK/SH2B3 loss of function promotes atherosclerosis and thrombosis. Circ Res. (2016) 119:e91–103. doi: 10.1161/CIRCRESAHA.116.308955
45. Saleh MA, McMaster WG, Wu J, Norlander AE, Funt SA, Thabet SR, et al. Lymphocyte adaptor protein LNK deficiency exacerbates hypertension and end-organ inflammation. J Clin Invest. (2015) 125:1189–202. doi: 10.1172/JCI76327
46. Camaré C, Pucelle M, Nègre-Salvayre A, Salvayre R. Angiogenesis in the atherosclerotic plaque. Redox Biol. (2017) 12:18–34. doi: 10.1016/j.redox.2017.01.007
47. Burkhardt R, Kenny EE, Lowe JK, Birkeland A, Josowitz R, Noel M, et al. Common SNPs in HMGCR in micronesians and whites associated with LDL-cholesterol levels affect alternative splicing of exon13. Arterioscler Thromb Vasc Biol. (2008) 28:2078–84. doi: 10.1161/ATVBAHA.108.172288
48. Kathiresan S, Melander O, Guiducci C, Surti A, Burtt NP, Rieder MJ, et al. Six new loci associated with blood low-density lipoprotein cholesterol, high-density lipoprotein cholesterol or triglycerides in humans. Nat Genet. (2008) 40:189–97. doi: 10.1038/ng.75
49. Surakka I, Horikoshi M, Mägi R, Sarin A-P, Mahajan A, Lagou V, et al. The impact of low-frequency and rare variants on lipid levels. Nat Genet. (2015) 47:589–97. doi: 10.1038/ng.3300
50. Medina MW, Gao F, Ruan W, Rotter JI, Krauss RM. Alternative splicing of 3-hydroxy-3-methylglutaryl coenzyme A reductase is associated with plasma low-density lipoprotein cholesterol response to simvastatin. Circulation (2008) 118:355–62. doi: 10.1161/CIRCULATIONAHA.108.773267
51. Zhou L, He M, Mo Z, Wu C, Yang H, Yu D, et al. A genome wide association study identifies common variants associated with lipid levels in the Chinese population. PLoS ONE (2013) 8:e82420. doi: 10.1371/journal.pone.0082420
52. Willer CJ, Schmidt EM, Sengupta S, Peloso GM, Gustafsson S, Kanoni S, et al. Discovery and refinement of loci associated with lipid levels. Nat Genet. (2013) 45:1274–83. doi: 10.1038/ng.2797
53. Teslovich TM, Musunuru K, Smith AV, Edmondson AC, Stylianou IM, Koseki M, et al. Biological, clinical and population relevance of 95 loci for blood lipids. Nature (2010) 466:707–13. doi: 10.1038/nature09270
54. Spracklen CN, Chen P, Kim YJ, Wang X, Cai H, Li S, et al. Association analyses of East Asian individuals and trans-ancestry analyses with European individuals reveal new loci associated with cholesterol and triglyceride levels. Hum Mol Genet. (2017) 26:1770–84. doi: 10.1093/hmg/ddx062
55. Chasman DI, Pare G, Mora S, Hopewell JC, Peloso G, Clarke R, et al. Forty-three loci associated with plasma lipoprotein size, concentration, and cholesterol content in genome-wide analysis. PLoS Genet. (2009) 5:e1000730. doi: 10.1371/journal.pgen.1000730
56. Mäkelä K-M, Seppälä I, Hernesniemi JA, Lyytikäinen L-P, Oksala N, Kleber ME, et al. Genome-wide association study pinpoints a new functional apolipoprotein B variant influencing oxidized low-density lipoprotein levels but not cardiovascular events: AtheroRemo Consortium. Circ Cardiovasc Genet. (2013) 6:73–81. doi: 10.1161/CIRCGENETICS.112.964965
57. Aulchenko YS, Ripatti S, Lindqvist I, Boomsma D, Heid IM, Pramstaller PP, et al. Loci influencing lipid levels and coronary heart disease risk in 16 European population cohorts. Nat Genet. (2009) 41:47–55. doi: 10.1038/ng.269
58. Sabatti C, Service SK, Hartikainen A-L, Pouta A, Ripatti S, Brodsky J, et al. Genome-wide association analysis of metabolic traits in a birth cohort from a founder population. Nat Genet. (2009) 41:35–46. doi: 10.1038/ng.271
59. Diabetes Genetics Initiative of Broad Institute of Harvard and MIT, Lund University and Novartis Institutes of BioMedical Research, Saxena R, Voight BF, Lyssenko V, Burtt NP, de Bakker PIW, et al. Genome-wide association analysis identifies loci for type 2 diabetes and triglyceride levels. Science (2007) 316:1331–6. doi: 10.1126/science.1142358
60. Postmus I, Trompet S, Deshmukh HA, Barnes MR, Li X, Warren HR, et al. Pharmacogenetic meta-analysis of genome-wide association studies of LDL cholesterol response to statins. Nat Commun. (2014) 5:5068. doi: 10.1038/ncomms6068
61. Warren HR, Evangelou E, Cabrera CP, Gao H, Ren M, Mifsud B, et al. Genome-wide association analysis identifies novel blood pressure loci and offers biological insights into cardiovascular risk. Nat Genet. (2017) 49:403–15. doi: 10.1038/ng.3768
62. Wain LV, Vaez A, Jansen R, Joehanes R, van der Most PJ, Erzurumluoglu AM, et al. Novel blood pressure locus and gene discovery using genome-wide association study and expression data sets from blood and the kidney. Hypertension (2017) 70:e4–19. doi: 10.1161/HYPERTENSIONAHA.117.09438
63. Aouizerat BE, Vittinghoff E, Musone SL, Pawlikowska L, Kwok P-Y, Olgin JE, et al. GWAS for discovery and replication of genetic loci associated with sudden cardiac arrest in patients with coronary artery disease. BMC Cardiovasc Disord. (2011) 11:29. doi: 10.1186/1471-2261-11-29
64. Finkel JB, Duffy D. 2013 ACC/AHA cholesterol treatment guideline: paradigm shifts in managing atherosclerotic cardiovascular disease risk. Trends Cardiovasc Med. (2015) 25:340–7. doi: 10.1016/j.tcm.2014.10.015
65. Knight CJ. Antiplatelet treatment in stable coronary artery disease. Heart (2003) 89:1273–8. doi: 10.1136/heart.89.10.1273
66. Ferrari R, Guardigli G, Ceconi C. Secondary prevention of CAD with ACE inhibitors: a struggle between life and death of the endothelium. Cardiovasc Drugs Ther. (2010) 24:331–9. doi: 10.1007/s10557-010-6244-x
67. Andreasen C, Andersson C. Current use of beta-blockers in patients with coronary artery disease. Trends Cardiovasc Med. (2018). doi: 10.1016/j.tcm.2017.12.014. [Epub ahead of print].
68. Barrese V, Taglialatela M. New advances in beta-blocker therapy in heart failure. Front Physiol. (2013) 4:323. doi: 10.3389/fphys.2013.00323
69. Diehl KJ, Stauffer BL, Dow CA, Bammert TD, Brunjes DL, Greiner JJ, et al. Chronic nebivolol treatment suppresses endothelin-1-mediated vasoconstrictor tone in adults with elevated blood pressure. Hypertension (2016) 67:1196–204. doi: 10.1161/HYPERTENSIONAHA.115.06979
70. Gaudet D, Alexander VJ, Baker BF, Brisson D, Tremblay K, Singleton W, et al. Antisense inhibition of apolipoprotein C-III in patients with hypertriglyceridemia. N Engl J Med. (2015) 373:438–47. doi: 10.1056/NEJMoa1400283
71. Gouni-Berthold I. The role of antisense oligonucleotide therapy against apolipoprotein-CIII in hypertriglyceridemia. Atheroscler Suppl. (2017) 30:19–27. doi: 10.1016/j.atherosclerosissup.2017.05.003
72. Ridker PM, Everett BM, Thuren T, MacFadyen JG, Chang WH, Ballantyne C, et al. Antiinflammatory therapy with canakinumab for atherosclerotic disease. N Engl J Med. (2017) 377:1119–31. doi: 10.1056/NEJMoa1707914
73. Interleukin-6 Receptor Mendelian Randomisation Analysis (IL6R MR) Consortium, Swerdlow DI, Holmes MV, Kuchenbaecker KB, Engmann JEL, Shah T, et al. The interleukin-6 receptor as a target for prevention of coronary heart disease: a mendelian randomisation analysis. Lancet (2012) 379:1214–24. doi: 10.1016/S0140-6736(12)60110-X
74. Kastelein JJP. Decade in review–dyslipidaemia: resurgence of targets and compounds to treat dyslipidaemia. Nat Rev Cardiol. (2014) 11:629–31. doi: 10.1038/nrcardio.2014.132
75. Lock FE, Ryan KR, Poulter NS, Parsons M, Hotchin NA. Differential regulation of adhesion complex turnover by ROCK1 and ROCK2. PLoS ONE (2012) 7:e31423. doi: 10.1371/journal.pone.0031423
76. Abraham G, Havulinna AS, Bhalala OG, Byars SG, De Livera AM, Yetukuri L, et al. Genomic prediction of coronary heart disease. Eur Heart J. (2016) 37:3267–78. doi: 10.1093/eurheartj/ehw450
77. Saleheen D, Zhao W, Young R, Nelson CP, Ho W, Ferguson JF, et al. Loss of cardioprotective effects at the ADAMTS7 Locus as a result of gene-smoking interactions. Circulation (2017) 135:2336–53. doi: 10.1161/CIRCULATIONAHA.116.022069
78. Bennett MR, Sinha S, Owens GK. Vascular smooth muscle cells in atherosclerosis. Circ Res. (2016) 118:692–702. doi: 10.1161/CIRCRESAHA.115.306361
79. Shameer K, Glicksberg BS, Hodos R, Johnson KW, Badgeley MA, Readhead B, et al. Systematic analyses of drugs and disease indications in RepurposeDB reveal pharmacological, biological and epidemiological factors influencing drug repositioning. Brief Bioinformatics (2017) 375:4. doi: 10.1093/bib/bbw136
Keywords: genome-wide association study (GWAS), coronary artery disease (CAD), drug targets, smooth muscle cells, vascular wall
Citation: Turner AW, Wong D, Dreisbach CN and Miller CL (2018) GWAS Reveal Targets in Vessel Wall Pathways to Treat Coronary Artery Disease. Front. Cardiovasc. Med. 5:72. doi: 10.3389/fcvm.2018.00072
Received: 22 March 2018; Accepted: 29 May 2018;
Published: 25 June 2018.
Edited by:
Jeanette Erdmann, Universität zu Lübeck, GermanyReviewed by:
Yun Fang, University of Chicago, United StatesHsiao-Huei Chen, University of Ottawa, Canada
Copyright © 2018 Turner, Wong, Dreisbach and Miller. This is an open-access article distributed under the terms of the Creative Commons Attribution License (CC BY). The use, distribution or reproduction in other forums is permitted, provided the original author(s) and the copyright owner are credited and that the original publication in this journal is cited, in accordance with accepted academic practice. No use, distribution or reproduction is permitted which does not comply with these terms.
*Correspondence: Clint L. Miller, clintm@virginia.edu