- 1Institute of Life Sciences, Scuola Superiore Sant’Anna, Pisa, Italy
- 2Department of Excellence in Robotics and Artificial Intelligence, The BioRobotics Institute, Scuola Superiore Sant’Anna, Pisa, Italy
- 3Bertarelli Foundation Chair in Translational Neural Engineering, Center for Neuroprosthetics, Institute of Bioengineering, Ecole Polytechnique Federale de Lausanne, Lausanne, Switzerland
- 4Fondazione Toscana Gabriele Monasterio, Pisa, Italy
- 5Department of Physiology, Cardiovascular Research Center, Lewis Katz School of Medicine at Temple University, Philadelphia, PA, United States
The autonomic nervous system exerts a fine beat-to-beat regulation of cardiovascular functions and is consequently involved in the onset and progression of many cardiovascular diseases (CVDs). Selective neuromodulation of the brain-heart axis with advanced neurotechnologies is an emerging approach to corroborate CVDs treatment when classical pharmacological agents show limited effectiveness. The vagus nerve is a major component of the cardiac neuroaxis, and vagus nerve stimulation (VNS) is a promising application to restore autonomic function under various pathological conditions. VNS has led to encouraging results in animal models of CVDs, but its translation to clinical practice has not been equally successful, calling for more investigation to optimize this technique. Herein we reviewed the state of the art of VNS for CVDs and discuss avenues for therapeutic optimization. Firstly, we provided a succinct description of cardiac vagal innervation anatomy and physiology and principles of VNS. Then, we examined the main clinical applications of VNS in CVDs and the related open challenges. Finally, we presented preclinical studies that aim at overcoming VNS limitations through optimization of anatomical targets, development of novel neural interface technologies, and design of efficient VNS closed-loop protocols.
Introduction
Cardiovascular diseases (CVDs) still represent a major disease burden worldwide, despite advances in pharmacological treatments (1). Therefore, new therapeutical strategies are currently being investigated as an alternative to classical schemes. Among those, the solutions offered by Bioelectronic Medicine (BM) (2–5)—a new, highly interdisciplinary field incorporating neuroscience, engineering, and molecular medicine (4, 6)—are emerging as appealing candidates. The development of BM was inspired by the growing comprehension of the autonomic nervous system (ANS), which plays a key role in the control of whole-body homeostasis. Dysfunctions of the ANS are consequently implicated in the development and progression of many diseases, including those affecting the cardiovascular system (4, 7–13). BM utilizes this body of knowledge as a reference for the design of implantable devices (5) that modulate signals of the peripheral nervous system to visceral organs for therapeutic purposes (14–16).
The neural control of cardiovascular functions involves multiple interactions among central and peripheral components of the so-called “cardiac neuraxis” (17), which comprises the intrinsic cardiac nervous system, vagus nerves (VNs), intrathoracic sympathetic ganglia, spinal cord, brain stem, and multiple central regions up to the insular cortex. Acting together, these hierarchically organized functional units coordinate and regulate cardiac activity to preserve an adequate match between cardiac output and blood flow demand (18). Autonomic dysregulation at various levels of the cardiac neuroaxis, from central nuclei to peripheral effectors, is now recognized as a fundamental contributor to the progression of CVDs (1, 19). For instance, altered neural signals, such as the pathological activation of cardiac afferent neurons by acute myocardial ischemia and reperfusion, induce maladaptive responses such as sympathetic overdrive and parasympathetic withdrawal (autonomic imbalance) that in turn contribute to the development of systemic cardiovascular alterations (1, 19, 20). Therefore, the selective modulation of the cardiac neuraxis to achieve targeted control of cardiovascular functions has been proposed as a potentially impactful application of BM (21, 22). Peripheral nerves and ganglia of the ANS are attractive targets for BM for their favorable location for surgical interventions compared with deep and anatomically less characterized ANS centers of the CNS like the periaqueductal gray matter of the midbrain or hypothalamic nuclei (11, 23). In this perspective, the most widely studied intervention is represented by the electrical stimulation of the VN (VNS) (24, 25).
The VN (or X cranial nerve) is a paired asymmetric and the most extensively distributed nerve in the body, as well as a major component of the cardiac neuraxis (26). Sensory signaling through the VN plays a critical role in maintaining homeostasis of feeding, digestion, respiration, and cardiovascular functions (27). For this reason, VN neuromodulation is being tested as a potential therapeutical strategy for many pathological conditions, including CVDs (28). However, despite promising results achieved in preclinical studies (29), VNS is accompanied by side effects and still needs to be optimized for better exploitation of its full potential in the clinical setting. Herein we will review the state of the art of VNS for CVDs and discuss the current perspectives of VNS optimization. First, we will summarize the anatomy and physiology of the cardiac vagal system; then, we will describe the principles of VNS for CVDs and its main clinical applications, with related open challenges. Finally, we will review preclinical studies aimed at overcoming VNS limitations through optimization of anatomical targets, development of novel neural interface technologies, and design of efficient VNS closed-loop protocols. In the latter case, we will particularly focus on neural decoding strategies that aim at the identification of timely- and spatially selective feedback signals to drive VNS for CVDs properly (8, 30–32).
Anatomy and Physiology of Vagus Nerve in the Cardiovascular System
Gross Anatomy
The VN originates bilaterally in the medulla as multiple filaments that extend toward the jugular foramen and then converge to form a single trunk. Within or just caudal to the jugular foramen are located the superior (jugular) and inferior (nodose) ganglia of the VN (Figure 1A) (26). At its emergence from the nodose ganglion, the VN can be anatomically divided into three segments along the rostro-caudal direction: cervical, thoracic and abdominal (26). The cervical VN generates multiple branches, including the superior cardiac and aortic branches (Figure 1A) (26, 33–36). Leaving the carotid sheath at the neck base, the VN enters the thorax, and it is referred to as the thoracic VN. Vagal cardiac branches include the superior and inferior cervical cardiac branches and the inferior or thoracic cardiac branch that originates from the thoracic VN (Figures 1A,B) (26, 37). The aortic branch or depressor nerve from the left VN contains afferent fibers innervating the aortic arch, while the one from the right VN innervates the bifurcation of the right brachio-cephalic trunk (35, 38). Within the mediastinum, the thoracic VN provides thoracic cardiac branches that are mainly observed between the aortic arch and the pulmonary arterial trunk and innervate the heart along the coronary arteries (37). Together with cardiac nerves from the sympathetic trunk, they contribute to form the cardiac plexus, which is usually divided into a superficial and a deep portion (Figure 1B). From a functional perspective, the right-sided nerves innervate mostly the sinoatrial node, while the left-sided nerves innervate mostly the atrioventricular node (36).
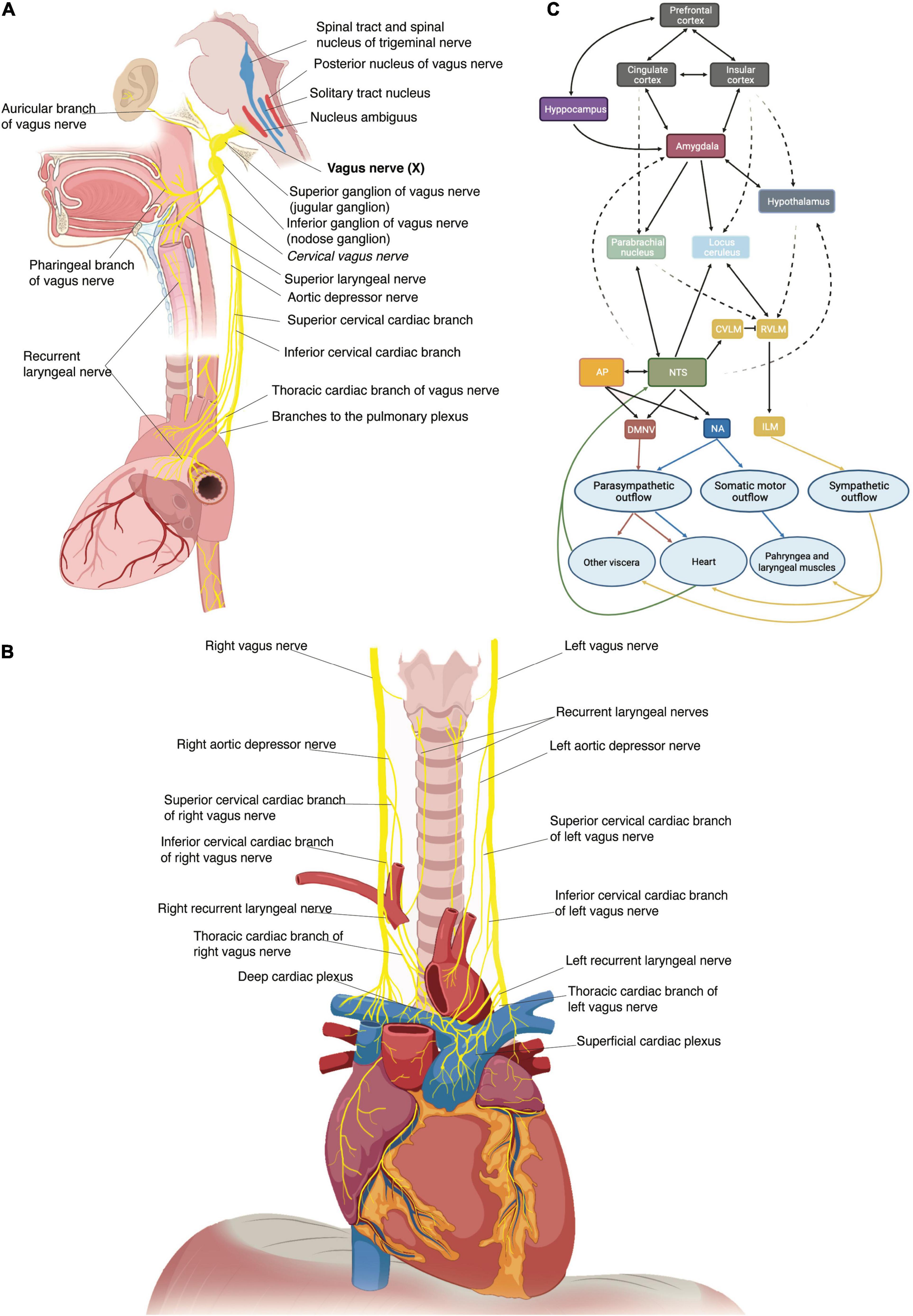
Figure 1. (A) Schematic representation of the origin of the vagus nerve from the medulla, its ganglia and its major branches at the cervical and thoracic levels. (B) Thoracic vagus nerves with cervical and thoracic cardiac branches to the deep and superficial cardiac plexi and recurrent laryngeal nerves. (C) Schematic representation of the central autonomic network with internuclei connections. An autonomic vagovagal loop comprises visceral inputs to the nucleus tractus solitarii (NTS) which then sends outputs to the dorsal motor nucleus (DMNV), rostral ventrolateral medullary (RVLM), and intermediate lateral medulla (ILM) to adapt autonomic balance to physiological demands. The cross-talk between the NTS and brain regions (hypothalamus, amygdala, cingulate cortex, insula, prefrontal cortex) engaged in neuroendocrine, affective, and cognitive regulation of behavior modulates this autonomic forebrain loop. AP, area postrema; NA, nucleus accumbens.
Microscopic Anatomy
Similar to other peripheral nerves, vagal fibers are grouped into a variable number of fascicles (39) with high variability among different species and even within the same species (40, 41). Several studies in human cadavers found the mean fascicles number of the cervical VN to oscillate between 5 and 10 (33, 40, 42, 43). The cervical VN in mice, rats, canines and non-human primates displays a less complex fascicular organization than in humans, typically consisting of 1–2 fascicles (43, 44). The porcine VN displays more fascicles than the human, containing 46 ± 10 and 43 ± 8 bundles at cervical and abdominal level, respectively (43, 44). In the somatic nervous system, all fascicles seem to conform a somatotopic organization (45, 46) and whether this occurs also in the ANS needs still to be assessed even if it is highly possible in complex nerves such as the VN (47). In fact, Settell et al. described a distinct bimodal organization of fascicles in the pig cervical VN. Specifically, they observed pseudounipolar cells aggregated in a large “fascicle” in nodose ganglion cross-sections and found a distinct group of fascicles arising from that large “fascicle” in caudal cross-sections of the cervical VN (Figure 2). This distinct organization of fascicles disappeared beyond the recurrent laryngeal nerve branching point; thus, they were identified as fascicles pertaining to the recurrent laryngeal nerve, separately from those coming from other visceral organs (Figure 2) (44). The precise distribution of fibers from the other visceral organs, especially fibers from peripheral cardiovascular targets, remains a matter of study, along with the definition of VN anatomical-functional models to guide the design of future VNS devices and protocols (48).
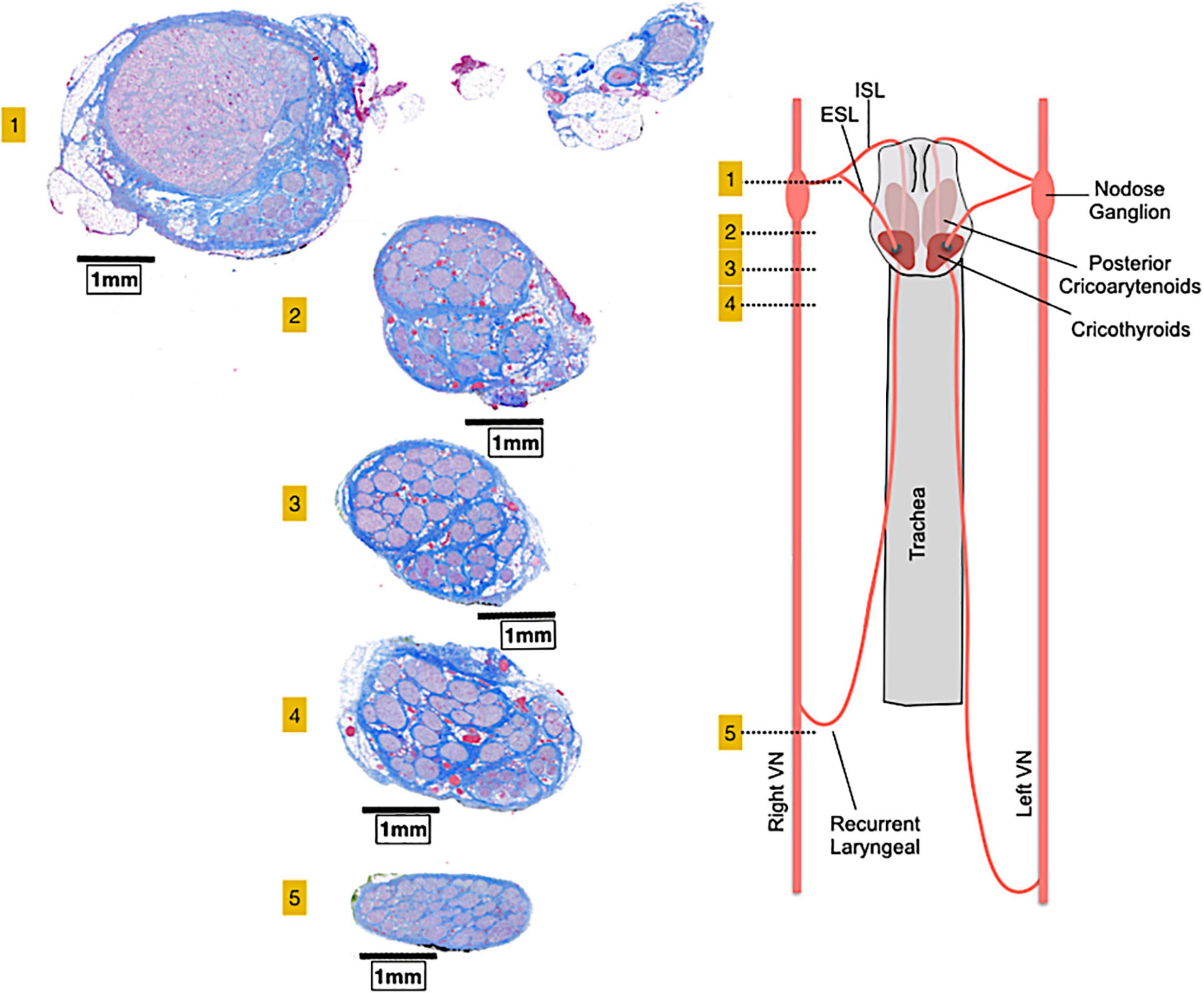
Figure 2. A bimodal arrangement, or vagotopy, can be observed in histological sections collected at various levels along the pig vagus nerve. Section 1 comprises the nodose ganglion and the superior laryngeal branch and show pseudo-unipolar cells grouped in a huge “fascicle” from which arises a smaller group of fascicles in sections 2, 3, and 4. Caudally to the recurrent laryngeal nerve (section 5), the bimodal architecture is no longer visible. ESL, external superior laryngeal nerve; ISL, internal superior laryngeal nerve, VN, vagus nerve. Adapted from Settell et al. (44).
The VN is a mixed nerve with fibers carrying sensory, motor, and visceral information. It entails mostly afferent nerve fibers (80–90%) with fewer efferent fibers (10–20%) in the majority of mammalian species (26, 49). VN fibers are classified as “A-fibers,” “B-fibers” and “C-fibers” in accordance with the classical Erlanger/Gasser classification (50). Among the afferents, C-fibers are thin unmyelinated, Aδ-fibers are thin myelinated and Aβ-fibers are thicker myelinated. Among the efferents, Aα-fibers are the thickest myelinated axons of α-motoneurons that innervate pharyngeal and laryngeal muscles, while B-fibers are tiny, myelinated and carry parasympathetic inputs to visceral organs (51, 52). The diameters of the unmyelinated and myelinated fibers of the VN are in the range of 0.25–1.0 μm and 1–4 μm, respectively, in most animal species (53). Nearly all the large (above 10 μm) and 40–50% of the small (below 4μm) myelinated fibers are efferent (49).
Preganglionic parasympathetic fibers originate from the dorsal motor nucleus of the vagus in the brain stem, branch out of the VN main trunk to join several autonomic plexuses and synapse at cell bodies of postganglionic neurons, generally located in the wall of the target organ. Afferent fibers consist of the T-shaped axons of pseudounipolar sensory neurons, with their neuronal soma residing in the nodose and jugular ganglia (52–54). In the brainstem, central processes of jugular ganglion neurons project to the trigeminal nucleus through the spinal trigeminal tract (26), while the primary relay of vagal visceral inputs from nodose ganglion neurons is the nucleus tractus solitarii in the medulla (55, 56). The nucleus tractus solitarii has direct and indirect connections with a wide range of neural structures, thus endowing the VN with the control of a broad array of processes (Figure 1C) (55, 57, 58). An autonomic vagovagal loop encompasses visceral inputs to secondary neurons in the nucleus tractus solitarii, which then contact efferent neurons of the dorsal motor nucleus and sympathetic neurons of the rostral ventrolateral medulla to adapt the autonomic balance to physiological demands (Figure 1C) (3).
Vagal Baroreceptors and Chemoreceptors
Vagal sensory neurons densely innervate great thoracic vessels and they include Piezo2 + /TTN3 + mechanosensory fibers, functioning as baroreceptors, and chemosensory fibers that detect arterial blood gas changes in the aortic bodies (Figure 3) (59). Afferent fibers from the left nodose ganglion innervate the apex of the aortic arch, while afferents from the right nodose ganglion innervate the right subclavian artery, near its branching from the innominate artery. These fibers run within the aortic depressor nerves forming fascicles that include both high-threshold mechanosensory and chemosensory afferents (59). The majority of vagal baroreceptors are myelinated fibers that convey information on stretch magnitude, pulse frequency and mean arterial pressure (59, 60) and, together with the carotid sinus innervated by the glossopharyngeal nerve, they represent the afferent arm of the arterial baroreflex (61–63).
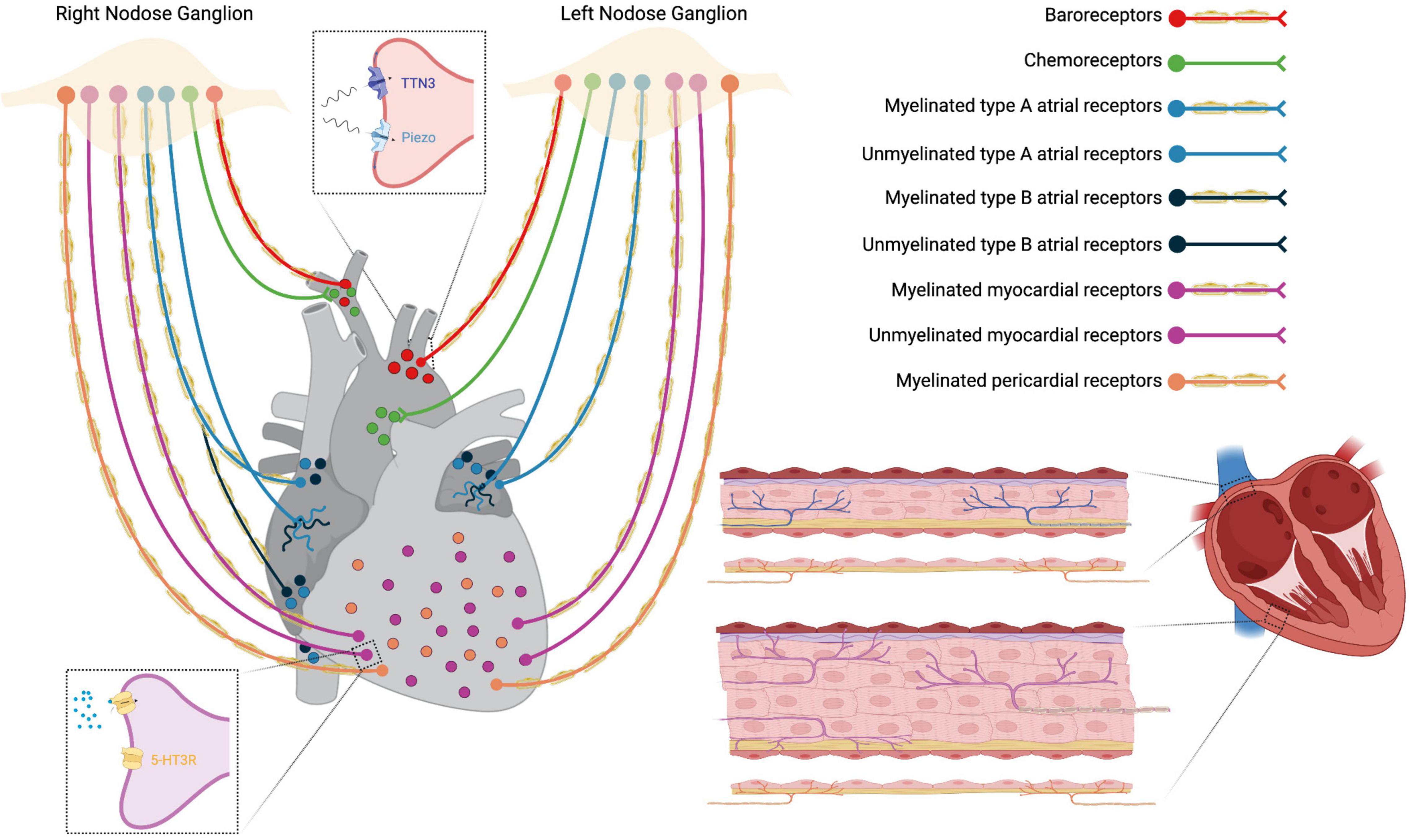
Figure 3. Schematic representation of thoracic cardiovascular afferent fibers running in the vagus nerve. Insets: top, baroreceptors Piezo2 + and TTN3 + terminals; down, unmyelinated 5-HT3R + myocardial receptors.
Vagal Cardiac Receptors
The VN provides both sensory and parasympathetic innervation to the heart via cardiac branches. Nodose neurons terminate with chemoreceptors and/or low-threshold mechanoreceptors in cardiac atria, ventricles, and major veins (Figure 3). Under normal circumstances, cardiac receptors are necessary for fine-tuning of the cardiovascular system. In CVDs like heart failure (HF), sensory endings undergo pathological activation that causes autonomic imbalance, with sympathetic excitation prevailing over vagal excitation (21, 22, 64).
There are at least two different types of atrial receptors belonging to the VN system of several mammalian species: type B receptors, that fire in response to increased volume (stretch receptors), and type A, that respond to atrial contraction. Both receptor endings correspond to slightly myelinated/unmyelinated fibers located mainly in the endocardium at the pulmonary veins-atrium and caval-atrium junctions and, to a lesser extent, in the free wall and appendage of both atria (65). They function as slowly adapting stretch receptors with low-frequency firing (66). Other vagal afferent fibers from the atria are unmyelinated C-fibers with a diffuse distribution and activity patterns similar to those described for type A or type B receptors (64).
Two types of sensory vagal endings have been described in both cardiac ventricles: myocardial and epicardial receptors (Figure 3). Myelinated myocardial receptors are mechanosensitive fibers working as tension/pressure-sensitive receptors and fire at the onset of left ventricular contraction (59, 64). On the other hand, unmyelinated myocardial receptors include 5-HT3R + C-fibers, predominantly functioning as mechanoreceptors (Figure 3) (64, 67), and C-fibers predominantly functioning as chemoreceptors which transduce the pain sensation that characterizes angina pectoris (21). Finally, vagal afferent fibers innervating the parietal pericardium are finely myelinated and sensitive to pericardium distension (64).
Vagal Cardiac Efferent Fibers
The VN provides parasympathetic innervation to the heart via preganglionic cardioinhibitory neurons mainly located in the nucleus accumbens and to a lesser extent in the caudal dorsal motor nucleus of the vagus (21). Neurons of the nucleus accumbens possess thin myelinated axons with a diameter comprised in the B-fibers range (conduction velocity range 3–15 m/s) (68, 69) and exert strong respiratory- and cardio-modulatory and chronotropic effects. Efferent fibers from the nucleus accumbens of the right VN synapse with postganglionic cholinergic neurons that innervate the sino-atrial node, while fibers of the left VN project to postganglionic cholinergic neurons that innervate the atrioventricular node (21, 63). In contrast, neurons of the dorsal motor nucleus have unmyelinated axons, show little or no respiratory and cardiac modulation, exert smaller effects on HR and possibly stronger dromotropic and inotropic effects, as they project to postganglionic neurons innervating the left ventricle (70, 71). Cardiac neural control is realized via tonic interaction between sympathetic and parasympathetic limbs of the ANS, particularly in the mammalian heart, where many terminal fibers lie close to each other and exert reciprocal inhibitory effects at the synaptic level (21, 22, 72).
Vagus Nerve Stimulation for Cardiovascular Diseases
Vagus nerve stimulation was first developed for the treatment of drug-resistant epilepsy and depression, obtaining FDA approval in 1994 (73–77) and 2005 (24, 78–81), respectively. Based on prior animal experiments, the first clinical studies defined the therapeutic range of VNS, the algorithm for stimulation titration up to the threshold of patient tolerance, and the safety and tolerability profile (73, 82). With the discovery of the inflammatory reflex in the early 2000s (83) and the evidence that VNS could attenuate inflammation normalizing the expression of proinflammatory cytokines (such as TNF-α and IL-6), VNS studies increased exponentially (28). At present, research labs worldwide are studying the effects of VNS in a multitude of conditions, spanning from neurological to inflammatory disorders, both in animal models and in patients (84).
The VN can be stimulated in different ways and at different levels. The classical VNS consists in an invasive procedure that is performed as a day case procedure under general anesthesia. The FDA-approved VNS device comprises a spiral anchor and two bipolar helical electrodes with a platinum ribbon functioning as an anode and a cathode. They wrap approximately 270° around the left cervical VN, below the origin of the superior and inferior cervical cardiac branches, and are connected via a cable tunneled subcutaneously to a pulse generator that is most commonly positioned in a infra-clavicular pocket (24, 85–90). Despite the fact that VNS is a minimally invasive treatment, surgery remains inherently risky and comes with a number of possible side effects (91, 92). Therefore, alternative non-surgical methods have been developed, such as cervical non-invasive or transcutaneous VNS directed to the auricular branch of the VN (Figure 4) (91, 93–95). In the current standard practice, VNS parameters are set individually and tuned periodically for each patient. An “adequate” stimulation is generally set between a minimum level of perception by the patient to a maximum level of intolerability due to side effects, both of which are subjective and variable (96).
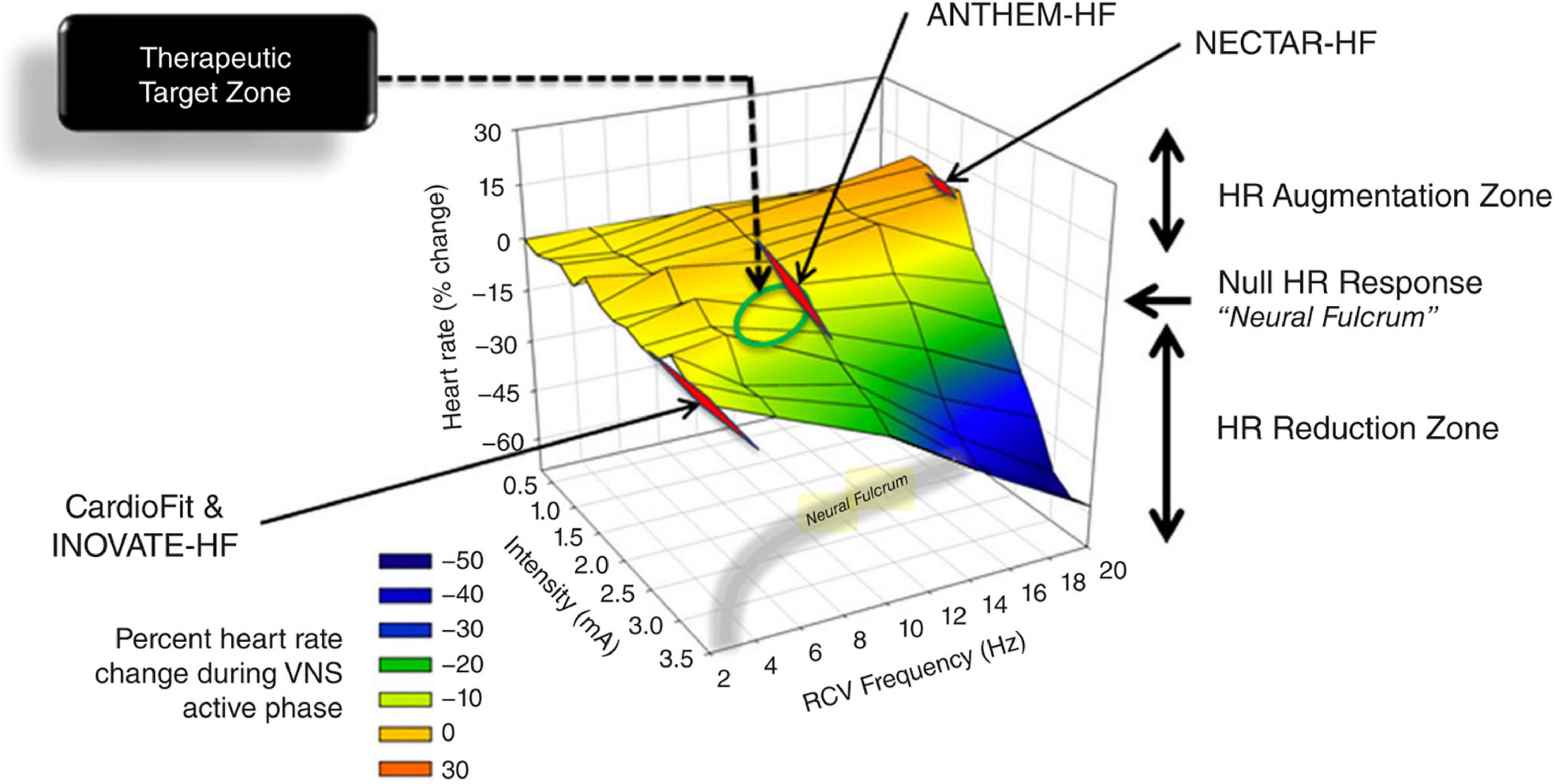
Figure 4. 3D plot of percent change in heart rate against right VNS intensity and frequency, with representation of the neural fulcrum in the yellow-shaded region. Operational points used in the NECTAR-HF, INOVATE-HF, and the ANTHEM-HF studies. Adapted from Ardell et al. (17).
Vagus Nerve Stimulation Mechanisms on the Cardiovascular System and Cardiovascular Diseases
Vagus nerve stimulation impacts cardiovascular control at multiple levels (97) via activation of afferent and efferent pathways and, depending on the frequency, pulse-width, and current intensity, of diverse fibers populations (43, 98).
In general, VNS of efferent cardiac fibers causes a reduction in heart rate (negative chronotropic effect on the sinoatrial node), in atrioventricular conduction (negative dromotropic effect on the atrioventricular node), and in ventricular contractility (negative inotropic effect on ventricular myocardium) (28, 99), with right VNS having mostly chronotropic effects while left VNS mostly dromotropic effects (100). VNS modulates left ventricular function increasing both action potential duration and the effective refractory period, either of which decreases intracellular calcium and ventricular contractility and wall motion (28, 89, 101). Activation of descending efferent projections can also mitigate sympathoexcitation via neural interactions within the intrinsic cardiac nervous system, modulate cardio-cardiac reflexes, and impart cardioprotection via direct effects on cardiomyocytes (1).
Vagus nerve stimulation of afferent fibers can impact central reflexes, including those that involve sympathetic and parasympathetic efferent outflows to the heart (1). For instance, VNS of vagal baroreceptors reflexively activates vagal cardioinhibitory efferent fibers to reduce heart rate and concurrently inhibits sympathetic efferent activity and down-regulates the renin-angiotensin-aldosterone system (28, 102).
Vagus nerve stimulation effects demonstrated beneficial effects in different animal models of CVDs. First of all, VNS has shown antiarrhythmic effects in several conditions, probably via multifactorial mechanisms that include a decrease in heart rate, the release of nitric oxide, anti-inflammatory effects, and antagonism of the sympathetic nervous system (28, 103). It was shown that VNS increases the threshold for ventricular arrhythmias via reduction in ventricular excitability and repolarization heterogeneity (effect on ventricular conduction system) (28, 99). In animal models of atrial fibrillation, VNS exhibited antifibrillatory effects by shortening atrial fibrillation duration and prolonging the atrial fibrillation cycle length (19, 28). Moreover, a VNS delivered below the threshold of bradycardia induction can effectively suppress atrial fibrillation in anesthetized dogs (89). VNS effects on the sympathetic nervous system contribute to the prevention of arrhythmias also during cardiopulmonary resuscitation (28). VNS-induced decrease in cardiac motion reduces cardiac metabolic demands during the vulnerable period of ventricular fibrillation, making VNS a potential intervention to improve the efficacy of defibrillation (101).
Vagus nerve stimulation was also shown to decrease infarct size and to halt post-myocardial infarction phenomena such as the remodeling of both the myocytes and the intrinsic cardiac neuronal system. These cardioprotective mechanisms include anti-inflammatory effects, prevention of Connexin 40 and Connexin 43 loss, antioxidative effects, and antiapoptotic effects such as decrease in cytochrome c release and in the proapoptotic Bcl-2-associated X protein levels (28, 89, 103–106). When applied during myocardial reperfusion, VNS was shown to improve ventricular function and reduce arrhythmic episodes via antagonization of the cardiac sympathetic outflow, reduction of reactive oxygen species and of ventricular excitability (28, 103–106). VNS improves left ventricular ejection fraction post-myocardial infarction restoring subcellular levels of calcium-binding proteins (such as SERCA2a, NCX1, and PLB) and can reestablish baroreceptor reflex to the pre-infarction baseline (28).
Vagus nerve stimulation can slow the progression of myocardial remodeling and atrial and ventricular dysfunction in animal models of chronic HF with reduced ejection fraction (28). VNS beneficial effects in HF can be attributed to improvements in left ventricular mechanics, attenuation of the sympathetic drive, down-regulation of the renin-angiotensin-aldosterone system, reduction of proinflammatory cytokines, normalization of the nitric oxide pathway, increase in myocardial expression of gap junction proteins and capillary density and tempering of myocardial interstitial fibrosis (1, 28, 104, 106–113). Optogenetic stimulation of cardioinhibitory neurons in the dorsal motor nucleus of the vagus can reduce myocardial expression of G-protein-coupled receptor kinase 2 (GRK2) and b-arrestin 2, which both contribute to the progressive decline of myocardial contractile function in HF (104).
Vagus nerve stimulation can ameliorate poststroke recovery via enhancement of motor cortex plasticity during rehabilitation, likely favoring the release of acetylcholine, norepinephrine, GABA, and brain-derived neurotrophic factor (28). VNS can also attenuate cerebral edema after brain injury by reducing cerebral blood flow, glutamate excitotoxicity, and inflammation (28).
Finally, VNS in hypertensive rats showed a significant blood pressure reduction, with static stimulation clinically more effective than pulsatile stimulation (28).
Vagus Nerve Stimulation in the Clinical Scenario
To date, despite the vast assortment of CVDs investigated in the pre-clinical scenario, VNS clinical applications in the cardiovascular field have been mostly focused on HF. This syndrome provides a strong rationale for ANS modulation, as its genesis and progression are heavily influenced by autonomic imbalance (21, 22, 114, 115). Preclinical studies have shown that VNS can exert very positive effects on the progression of HF, but clinical trials failed to achieve the same results. Complete clinical trials of VNS for HF with reduced ejection fraction include two randomized controlled trials, i.e., the INOVATE-HF (116) and the NECTAR-HF (117), and two open-label studies, i.e., the ANTHEM-HF (118) and the study by De Ferrari et al. (119). In the study by De Ferrari et al. (119) and in the INOVATE-HF, VNS was delivered using the CardioFit system that senses heart rate (via an intracardiac electrode) and delivers asymmetric stimulation at a variable delay (70–325 ms) from the R-wave (85, 87, 119, 120). The stimulation lead is an asymmetric bipolar multi-contact cuff electrode specifically designed for cathodic induction of action potentials while simultaneously applying asymmetrical anodal blocks, thereby reducing the activation of A-fibers while preferentially activating efferent B-fibers (85, 87, 105). In the NECTAR-HF (117) and the ANTHEM-HF (118) trials the investigators utilized the Boston Scientific VNS device that activates VN fibers bidirectionally with no synchronization with the cardiac cycle (118). All trials recruited similar NYHA class II-III patients with reduced left ventricular ejection fraction and receiving optimal medical therapy (121). These trials did not raise safety issues but showed variable efficacy (116, 118, 121, 122). As highlighted by a recent meta-analysis, these trials showed significant improvement in the functional NYHA class, quality of life, 6-min walking test, and NT-proBNP levels, but VNS did not have any impact on mortality (123). The subjective measures that improved in all trials should be cautiously taken as subjects were not totally blind to the therapeutic procedures, notwithstanding the sham-controlled design (116, 118, 124). Only the two uncontrolled studies (ANTHEM-HF and the study by De Ferrari et al.) showed a positive effect of VNS on cardiac remodeling (118, 119), despite the success of preclinical experiments (118, 120). These trials employed diverse stimulation parameters, and subsequent analyses showed that the ANTHEM-HF study was the only one to achieve the therapeutic stimulation corresponding to the “neural fulcrum” (17). The neural fulcrum is defined as the combination of VNS parameters such as frequency–amplitude–pulse width that results in no heart rate response (Figure 4), and it corresponds to a dynamic equilibrium where VNS activates cardiac neural circuits while keeping reflex control of cardiovascular functions. In fact, VNS normally modifies cardiac neural circuits pushing them in one direction that tends to be physiologically counterbalanced by cardiovascular reflexes (17). For instance, low intensity/high frequency (20 Hz or more) stimulation preferentially activates afferent fibers causing tachycardia that leads to secondary activation of both central pathways and vagovagal, vagosympathetic, or vagoadrenal reflexes. On the other hand, higher intensity/lower frequency (10–15 Hz) stimulation activates parasympathetic neurons resulting in bradycardia and mitigation of sympatho-excitation via neural interactions within the intrinsic cardiac nervous system (1). Finally, low intensity/very low frequencies (1–2 Hz) stimulation determines little to no cardiomotor effects, as the afferent-driven decreases in central parasympathetic outflow are equivalently counteracted by direct activation of cardiac parasympathetic neurons (125).
ANTHEM-HF utilized the principle of neural fulcrum by determining the autonomic engagement via an automatic beat-to-beat pattern analysis throughout the initial phase of VNS titration (122). In the NECTAR-HF trial, the high frequency stimulation provoked patients intolerance and impeded titration to a therapeutic dose; in the INOVATE-HF study, not all patients received adequate stimulation levels (116, 121, 122, 126–128). On the basis of the results of ANTHEM-HF, a large, randomized, controlled trial of right VNS with the use of the same system is now underway (ANTHEM-HFrEF PIVOTAL trial, NCT03425422) (18), along with a novel study in patients suffering from HF with preserved and mid-range ejection fraction (127).
Unresolved Issues in Clinical Vagus Nerve Stimulation for Cardiovascular Diseases
The exploitation of VNS full potential and its transformation into a simple and cost-effective therapy for a wide range of conditions requires the completion of some major steps. A general problem is the still limited knowledge of VN function, with an ensuing lack of understanding of the mechanisms responsible for already established VNS treatments of diseases such as drug-resistant epilepsy (121, 126, 129). Consequently, optimum stimulation parameters tailored for patient-specific clinical characteristics and precise timing remain a matter of debate (74, 75, 79, 128, 129). VNS is normally up-titrated through a series of follow-up visits until a therapeutic dosage is attained without adverse effects and up to the tolerance threshold of the patients (73, 94). Given subjectivity of patients’ tolerance and the uniqueness of the electrode-nerve interface, no standard therapeutic dose exist, the effectiveness of parameters adjustment during titration remains dubious, and the response prediction uncertain (92, 121, 122, 129, 130).
Another related problem is the definition of the target population using adequate “predictors” to discriminate between responders and non-responders. These predictors could be markers of autonomic imbalance represented by physiological parameters such as heart rate variability or innovative markers such as those derived from neural decoding of specific ANS circuits (78, 118, 129, 131).
While technology advances at a quick pace, neuromodulation’s ultimate potential can be realized when the relationship between nerve activity and physiological function is thoroughly known (4, 6), thus allowing translation of biological information into appropriate engineering specifications (16, 132). The knowledge gap of vagal physiology, functional anatomy and neuromodulation mechanisms inevitably also affects the selectivity of neural interfaces and of neuromodulation protocols (133). First of all, most VNS systems lack functional selectivity, that is stimulation of distinct functional classes of fibers, and are far from mimicking patterns of action potential occurring in healthy nerve fibers (32, 133). Secondly, most VNS systems lack spatial selectivity, that is selective modulation of fibers in the specific anatomical territory innervated by a given fascicle (32). Electrodes commonly used for VNS are not selective enough to achieve targeted neuromodulation in a complex fasciculate nerve like the VN (133). The direct consequences are the failure to achieve therapeutical effects and the onset of side effects that include hoarseness, throat pain, voice alteration, difficulty swallowing, coughing, abdominal and chest pain, nausea, dyspnea, and bradycardia (36, 85–88, 90). The inadvertent stimulation of somatic nerve branches such as the superior and recurrent laryngeal nerve has been addressed as one of the main causes of VNS side effects (36). Selective stimulation of vagal cardiac B and C fibers can be challenging given that their thresholds are 2–100 times greater than A fibers, as those branching to the laryngeal nerves (89). To achieve such selectiveness, several authors tried the combination of different stimulation parameters or to modify the pulse shape using different techniques such as the anodal block, slowly rising pulses or depolarizing pre-pulses (89). Other strategies are the modification of electrode design to allow preferential activation of efferent fibers (such as in the case of the CardioFit system) or the development of multicontact electrodes that exploit the topographical architecture of human nerves to target organ-specific fascicles (36, 88–90). This last approach would provide better spatial resolution and consequently improve the selectivity both for recordings and stimulation (36, 89). Therefore, new neural interfaces with higher electrode counts and spatial selectivity should be implemented (8, 30), along with advanced signal processing techniques and the use of hybrid models (134, 135) with extensive validation in experimental animals (136). With such technologies, the development of closed-loop VNS based on the combination of selective nerve stimulation and biosensing technologies could be one of the best solutions to overcome the aforementioned limitations emerged during VNS clinical trials (7, 133).
Closed-Loop Strategies for Vagus Nerve Stimulation
The current VNS systems provide stimulation in an open-loop fashion, meaning that parameters are pre-set and are not automatically adjusted according to the patient’s clinical characteristics (137). Closed-loop stimulation strategies offer the advantage of providing treatment only in response to detection of altered biomarkers of disease, thus tuning the stimulation according to the patient’s condition (138, 139). This approach potentially improves the efficacy of open-loop interventions and decreases the associated side effects (140). Closed-loop devices should continuously monitor internal biological variables to adjust therapy to individual conditions (7), thus allowing not only automatic but also adaptive neurostimulation that could maintain its efficacy over time and overcome the intrinsic plasticity of biological systems (8, 11, 141). In fact, plasticity and memory are crucial characteristics of the cardiac neuraxis, which undergoes profound alterations in chronic CVDs, causing the disruption of homeostatic cardiovascular functional responses (19).
Closed-loop VNS relies heavily on the precise selection and processing of physiological inputs (32, 142). To date, in the clinical scenario, only macro-biosignals like heart rate have been employed as input data for control loops. This concept is well illustrated in the work by Tosato et al. who achieved heart rate regulation with a closed-loop control system that continuously measured the RR interval, recalculated the difference between the measured and the target value and fed it back to the stimulator accordingly (100). Multiple other indirect and non-invasive measures can be used as indexes of cardiac VN activity (115), for instance heart rate variability, baroreflex sensitivity or respiratory sinus arrhythmia (21, 115). However, such clinical vagal indexes should be used with caution as they represent gross markers of the final net effect of parasympathetic and sympathetic action on the heart (72). On the other hand, local control loops can be obtained by recording feedback bio-signals from the same spot where the stimulus is delivered (142). This approach optimizes the spatial and temporal distribution of the local stimuli (142) but requires high-fidelity feedback signals that are not clinically obtainable due to current technological limitations (142, 143). A good example is represented by the lack of instruments to properly follow and locally evaluate in real-time the myriad of metabolic signals or the fluctuating levels of inflammatory stimuli. Nevertheless, all this information is collected by the peripheral sensors of the ANS and converted into electrical signals (neural encoding), a sort of “neural footprints” of physiological processes that can be recorded and decoded (Figure 5) (8, 30). Deciphering the neural language through decoding techniques would be essential to understand the underlying mechanisms of many diseases and to develop new methods and technologies that better engage with neural circuities (141). In the case of VNS, the copresence of afferent and efferent fibers in the VN offers the opportunity to build a feedback loop on the same anatomical site, that is to record from and then to stimulate the VN (Figure 5) (144, 145). Vagal sensory neurons are equipped with a vast arsenal of receptors to sense and respond to a huge variety of stimuli (27). Consequently, neural signals traveling through the VN represent a peerless source of information, and this helps the implementation of neural decoding strategies that usually benefit from an adequate number of signals (30, 32). Moreover, vagal neural signals offer the advantage of high temporal and spatial resolution (146), helping in the identification and classification of patterns difficult to see using other biosignals (8, 30) and potentially allowing devices to diagnose various conditions before symptoms presentation (32). New neural interfaces with higher electrode densities and spatial selectivity, such as intraneural electrodes, (147) and advanced signal processing techniques (8) will be necessary to take full advantage of the information traveling along with the VN. In the next section, we present the main preclinical studies that aim at the development of VNS closed-loop approaches based on different neural recording and decoding strategies for CVDs.
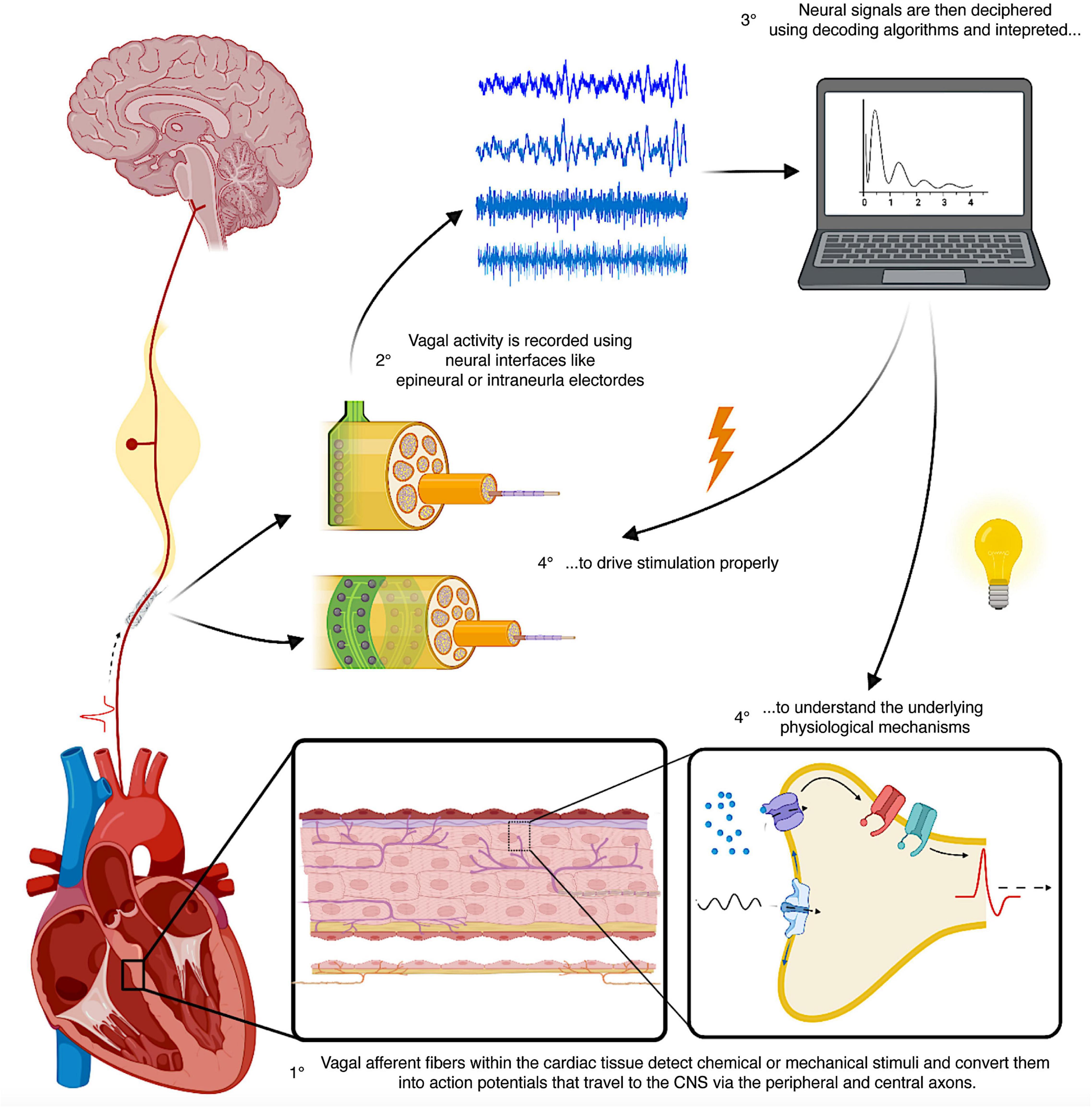
Figure 5. Schematic representation of the principles of closed-loop neuromodulation using neural recordings and decoding strategies. The heart is densely innervated by vagal afferent fibers, which detect chemical and mechanical stimuli, convert them into electrical signals (action potential) and send them to the CNS via their peripheral and central axons. Using neural interfaces placed on the vagus nerve, it is possible to record and decode these electrical signals and identify the underlying physiological processes and set the spatiotemporal characteristics of stimulation. CNS, central nervous system.
Decoding Techniques for Physiological Fiber Firing
Neural interfaces used for stimulation can also be used to record neural signals and monitor ANS activity in real-time (13). While innovative neural interfaces with multiple contacts are designed to improve the quality and information content of neural recordings (148–151), comparable efforts are being made to develop advanced signal processing and data analysis methods (152, 153). Several studies recently focused on the extrapolation of neural markers from spontaneous or physiologically enhanced VN activity, employing various decoding techniques. One technique is the coherent electroneurogram averaging that aims at the isolation of the neural activity of interest from the random noise by taking the average of N snippets from a recorded signal in correspondence to an external or an internal trigger, for instance a biological change (154). Using multicontact cuff electrodes, Plachta et al. employed the ECG rising edge as a trigger to remove stochastic noise, isolate baroreceptors activity from VN recordings and perform selective VNS reducing blood pressure without producing side effects in rats (145). Sevcencu et al. recorded signals from the porcine left VN to extract intraneural and extraneural profiles resembling the temporal evolution of blood pressure during baseline activity. In particular, systolic peaks and dicrotic waves characterizing blood pressure were reflected by the neural counterpart (144, 155). Rozman and Ribarič employed 33-electrode spiral cuff to record from the left VN of a dog during stimulation of cardiovascular or respiratory stimulations and identified the channel that was best correlated with heart activity using the spectrum estimation technique (156).
Another common approach to extract physiological information from VN electrical activity consists of decoding fiber spike patterns using spikes sorting techniques (157–162). Typically, raw neural signals are band-passed from 200 Hz to a maximum of 10 kHz, robustly denoised, spikes are then detected using thresholding methods and clustered using feature waveforms (46, 163). In general, there is not a universally adopted low-frequency cutoff as it usually depends on the quality and the nature of the signals. For instance, 200 Hz low-frequency cutoff was used in the context of intrafascicular sciatic nerve recordings (164), 1 kHz for intraneural recordings from the pig VN (136), 700 Hz (165) and 300 Hz (166) in the case of microneurographic recordings from the peroneal nerve and from the human VN, respectively. In the murine VN, spike sorting techniques within decoding frameworks have been employed to decode the activity of different fiber types enhanced by inflammatory stimuli such as particular cytokines (153) or metabolic ones like hypoglycemia/hyperglycemia (167). Spike sorting techniques are potentially usable in human patients as we recently obtained single-fiber recordings from the human cervical VN identifying tonically active neurons that discharged synchronously with the respiratory and cardiac cycles (166). Spike-like signals, as they reflect the activity of individual fibers, are preferable to cumulative signals to obtain maximal functional selectivity (162). Real-time implementations of complex spike sorting algorithms onto low-power off-the-shelf digital signal processors are currently available, as in the case of neuroprosthetic applications where the power consumption enabled more than 24 h processing at the maximum load (162). In the case of closed-loop VNS protocols, this would allow longer operational time scales, such as Holter-like monitoring at a neural level, i.e., a “Neural Holter” with biomarkers extracted directly from neural activity. As pointed out in Raspopovic et al. (136), signals recorded with intraneural electrodes can be classified as a hybrid category between cumulative and single-unit signals. This characteristic allows the development of more robust recording schemes and processing algorithms via a combination of decoding strategies developed on both cumulative and single-unit signals (136).
The identification of neural signals elicited by specific physiological stimuli could be extremely useful to distinguish among VN fibers coming from different cardiovascular sites and carrying information on multiple functional parameters that can vary over short time windows, such as cardiac output and blood pressure. Such distinction could be further appreciated using neural multielectrode devices with high spatial selectivity to better interface the potential topographical architecture of the VN (44, 46). However, cuff electrodes can only sense compound nerve action potentials and multi-unit activity and they do not allow access to single-fiber action potentials contrary to intraneural electrodes (89, 168). Intraneural electrodes offer the advantage of higher signal-to-noise ratio and higher spatial specificity compared to epineural electrodes and could allow more effective closed-loop decoding methods to be used (8, 139, 169). Intraneural electrodes such as the Longitudinal Intra-Fascicular Electrode (LIFE) or the Transversal Intra-Fascicular Multi-channel Electrodes (TIME) have provided rich and valuable sensory feedback in human amputees and detailed information from decoding hand movements in somatic nerves (139, 157, 159, 170). The LIFE is a flexible electrode consisting of 25–50 μm diameter Pt or Pt-Ir wires insulated with Teflon or metalized Kevlar fibers insulated with medical-grade silicone. The wire is surgically inserted into the nerve along the fascicle and then pinched out of the nerve again. The recording sites are areas of 0.5–1.5 mm long which are left uninsulated (149). A more recent version of LIFEs is the thin-filmLIFEs (tfLIFE), based on a thin highly flexible micropatterned polyimide substrate filament that can host eight contact sites (46, 149). The TIME consists of a thin, strip-like polyimide substrate with platinum electrode sites. The substrate is folded to align several electrodes and the folded substrate is threaded transversely through the nerve between the fascicles (149). The original design contained 10 sites with interelectrode spacing of 230 μm (148). The TIME was developed to achieve good contact with nerve fibers, selectively addresses several fascicles in a nerve with a single implant, and minimizes the mechanical mismatch between the implanted material and nerve tissue (168). The TIME has shown higher selectivity at low stimulation intensities than the single LIFE and multipolar cuffs (46, 148, 149). Our group combined the use of multichannel intrafascicular electrodes, machine learning principles and hybrid models (136) to study high frequency (>1,500 Hz) VN activity of anesthetized pigs during artificially produced alterations of physiological parameters, simulating increases in respiratory rate, tidal volume and arterial blood pressure (Figure 6A) (152). Using a new decoding algorithm that combines wavelet decomposition, dimensionality reduction, and ensemble learning classifiers, we could associate VN signals to specific functional changes (Figure 6B). Our approach was a machine learning-driven approach to find informative feature vectors for reliable decoding of cardio-respiratory alterations regardless of their precise nature, and future analysis will serve to get more interpretable features related to units and aggregate activities. Different from epineural electrodes that can only provide a global picture of neural signal trafficking (147, 171), we employed intraneural electrodes to enhance selectivity (147, 169) and to map a possible spatial functional organization of VN fascicles. Thus, we employed a hybrid modeling framework based on histological analysis combined with electrode discrimination ability properties measured via a novel quantitative measure called Discriminative Field Potential (DFP) and we obtained distinct spatial configurations of discriminative patterns generated by fascicles during the various functional challenges (Figure 6C) (152). This is extremely important for the development not only of timely, but also spatially selective stimulation protocols in a complex nerve with multiple fascicles like the human or porcine VN (152). In this perspective, the precise knowledge of cardiovascular fibers arrangement within the cervical vagal trunk would be crucial to establish effective VNS protocols for the treatment of CVDs. Anatomical models and non-invasive tests should be developed and used to better anticipate which site for neuromodulation would give the best outcomes and to overcome the anatomical variability that limits clinical VNS applications (116, 120, 129).
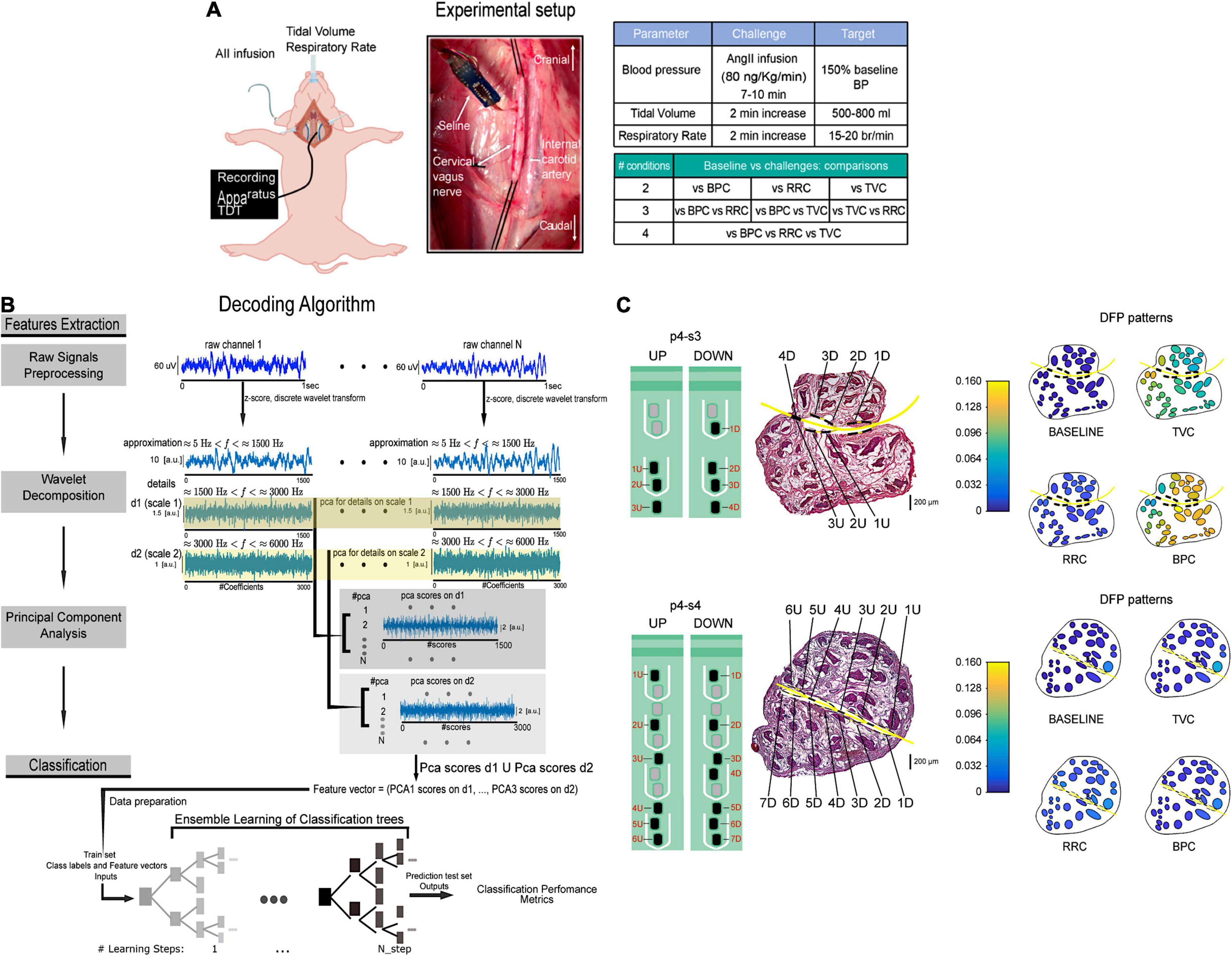
Figure 6. Schematic representation of the experimental setup and decoding algorithm from Vallone et al. (152). (A) Recording apparatus, electrode implantation and summary of the in vivo protocol and functional challenges comparisons. (B) Decoding algorithm. Feature extraction was performed on 1 s portions of raw intraneural signals (dark blue traces) by applying principal component analysis on wavelet details relative to two different scales (1,500 Hz < f < 3,000 Hz and 3,000 Hz < f < 6,000 Hz). Neural signals recorded with intraneural electrodes were a combination of single-unit and compound action potentials. Decoding performed with ensemble learning based on classification trees combined with random undersampling and boosting procedure was applied on feature vectors. Decoding performance was assessed by means of confusion matrices and accuracy level. (C) Schematic description of two intraneural electrodes implantation in the VN of one pig and hybrid model simulations of fascicles activation at baseline and in response to functional challenges. The two intraneural electrodes are represented on a VN histological section (central panels). DFP patterns of activity during baseline and each functional challenge (left panels). DFP, discriminative field potentials. Adapted from Vallone et al. (152) with permission.
The biocompatibility and longevity of intraneural electrodes was demonstrated in animal models and preliminarily confirmed in human experiments with long-term stimulation for sensory feedback and chronic neural recordings (139, 172). However, the experience in chronic implantations in humans indicates that there is frequently a reduction in the number of functioning electrode active sites, an in- crease in the stimulation threshold, and a decrease of the signal-to-noise ratio along time. To further improve the usability of the neural electrodes, considerable efforts are being devoted in the engineering field for increasing robustness and flexibility at the same time of miniaturizing the electrodes and in the biological field to increase biocompatibility of the substrates and to modulate the foreign body reaction (46). On the computational side, since the drift in the amplitudes of signals and changes in the signal-to-noise ratio greatly hampers chronic neural recordings and decoding, new algorithms for drift compensation have been developed (173). In the case of the VN, carbon nanotube yarn electrodes have been used to make the first direct chronic measurements of vagal tone in freely moving rats (174). Thanks to their small size, high flexibility, and low impedance, carbon nanotube yarn electrodes have provided stable, high-signal-to-noise chronic recordings in rats VN with high-quality signals continuing up to 4 months after implantation (174).
Decoding Fiber Activity in Electrically Stimulated Nerves
Recording and decoding the spontaneous activity of vagal fibers would be useful to determine the precise timing for VNS delivery, but may prove limited for the definition of precise dosing (133). In this regard, recording and decoding fiber activity during VNS may represent a complementary method to better define the relationship between stimulation and physiological effects.
The standard VNS dosing method does not rely on any measurement of fiber activation, since the commercial implants lack the capability to record from nerves during stimulation. Consequently, factors like electrode interface and nerve sensitivity are not controlled and VNS effects in a patient are neither uniquely determined over time nor comparable with other patients (133). The ability to distinguish between stimulated fibers could aid in correlating neural activity to external variables, thus increasing the ability to achieve targeted stimulation (175), with decreased variability in therapeutic responses and increased response rate (96).
In studies that employ extraneural interfaces, nerve activity is frequently analyzed in terms of evoked compound activity by electrical stimulation (eCAP) (176). The diverse conduction velocities of various fiber types, which ultimately depends on fiber caliber and degree of myelination, determine typical patterns and shapes with distinctive latencies and peaks in the eCAP (177), as shown in Figure 7. A sophisticated analysis of VN eCAPs would help in the assessment of the relationship between stimulus dose, neural recruitment and physiological effects (178).
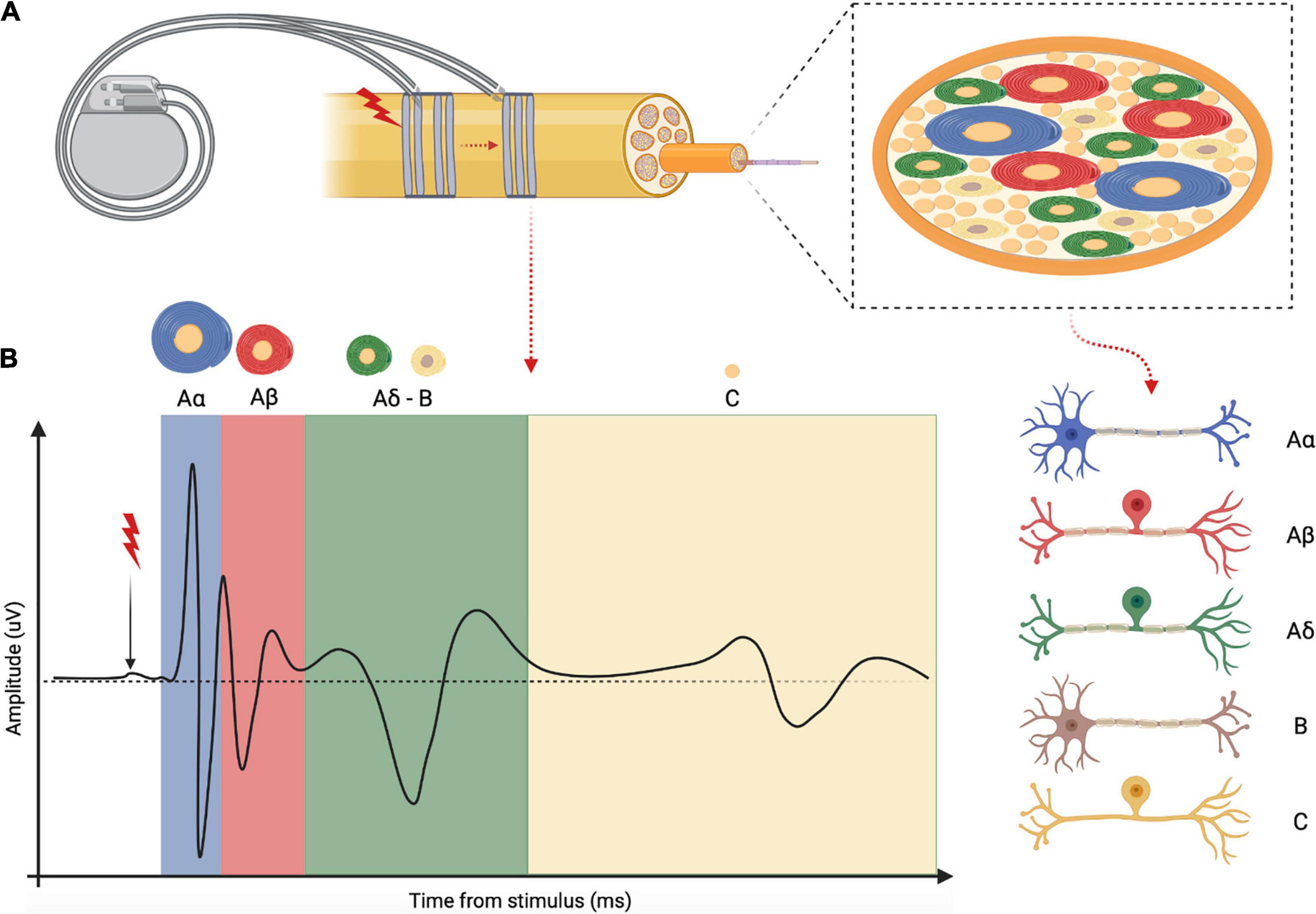
Figure 7. Schematic representation and explanation of the electrically evoked compound action potential (eCAP). (A) Example of a VNS device that electrically stimulate the vagus nerve at one site while recording evoked activity at another site. Inset: vagal fascicle containing different fiber types distinguished by myelination degree and fiber caliber. (B) Example of a complete vagus nerve eCAP obtained with supra-threshold stimulation of all vagal fibers subtypes. Each peak of the eCAP corresponds to different fiber subtypes as indicated by the different color codes.
Tahry et al. obtained, for the first time, VN eCAPs recordings after implanting the Advanced Nerve Stimulator version 300 (ADNS-300, Neurotech SA, Louvain-La-Neuve, Belgium) in the human VN (179). In preclinical studies, VNS-eCAP were used to optimize stimulation parameters and electrode design during VNS in dogs (176), pigs (180), and rodents (96), showing a strong correlation with the physiological effects of stimulation. For instance, vagal B-type fiber eCAP amplitude was correlated with changes in heart activity (176), indicating that parasympathetic B-fibers are the best predictors of cardiac activity during VNS (96, 177). Ordelman et al. found an indirect component in pig vagal eCAPs during VNS protocols, and they showed that it correlated with the state of the cardiovascular system (181).
Conclusion
In conclusion, the VN represents a key component of the cardiac neuraxis and VNS has shown a great potential for the treatment of a wide range of CVDs in the preclinical setting. Results in experimental animals may not be immediately translated into clinical applications, yet they are paving the way for fine-tuning and customized VNS applications. VNS represents a cheaper alternative/complementary solution to pharmacological remedies that lack of efficacy and present significant side effects and astronomical costs (4, 7, 182, 183). Closed-loop VNS would guide earlier, more accurate diagnosis and enable more effective, less costly prevention and intervention compared to pharmacological treatments (183). Moreover, access to personalized bioelectronic data would facilitate greater patient understanding of their conditions and greater engagement with their treatments, higher levels of health literacy, and greater communication and trust between patients and physicians (183). A plethora of promising research is advancing to overcome VNS limitations and develop closed-loop modalities. Advanced neural decoding strategies represent a major candidate. However, automatic closed-loop modalities will require not only advancements in biotechnologies but also improvements in the basic understanding of fundamental biological mechanisms (16). Progress in neural interface technology, big-data analysis methods, and signal processing techniques will accelerate biological breakthroughs that, in turn, will inform additional advancements in technology and methodology, creating a synergistic loop that ensues cross-disciplinary collaboration (Figure 8) (8).
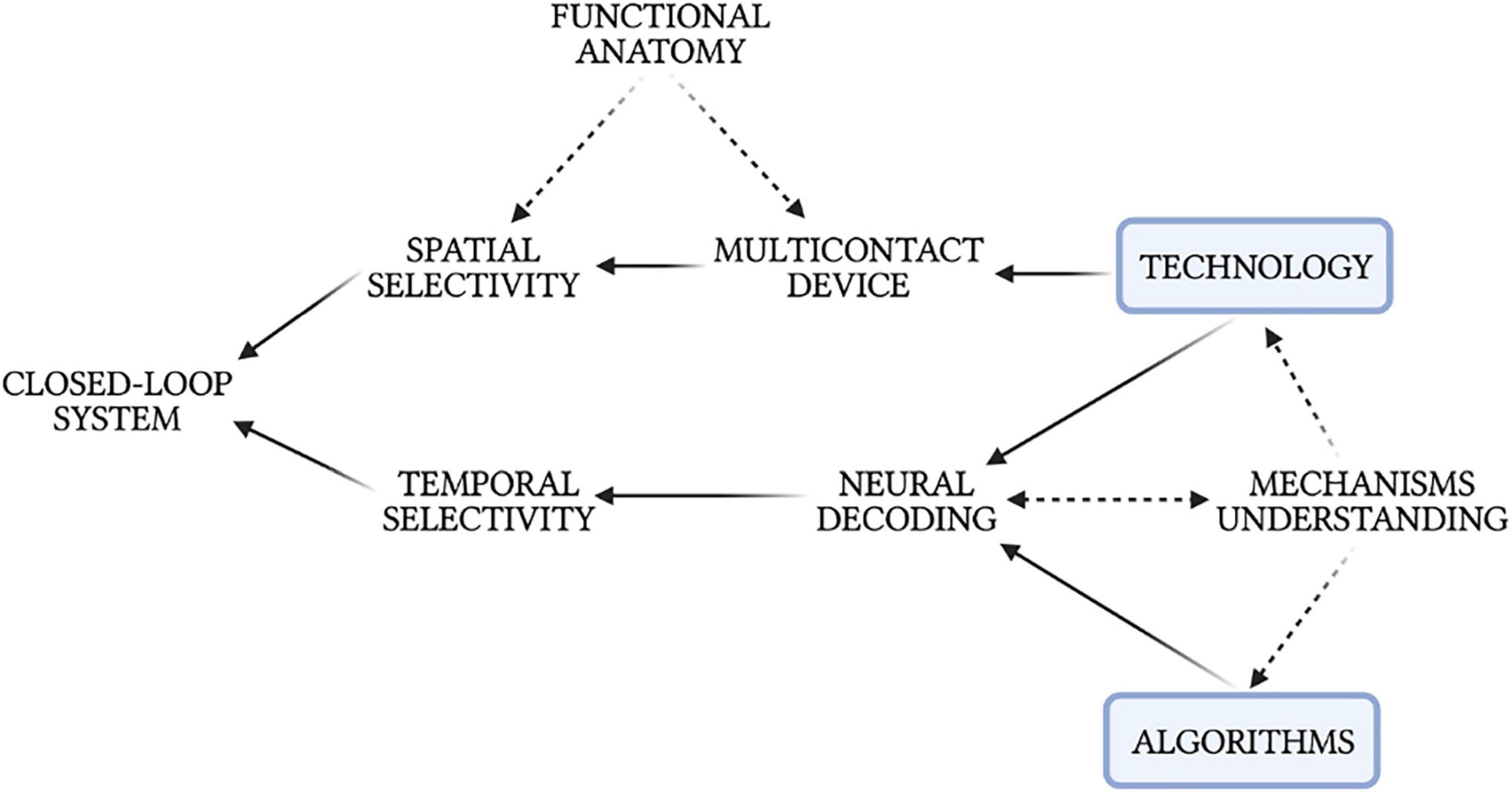
Figure 8. Intercorrelations between neural decoding, technology, algorithms, physiological mechanisms, and the development of a closed-loop neuromodulation system.
Author Contributions
MMO conceptualized, wrote the manuscript, and prepared the figures. FV wrote and revised the manuscript. SM and FR conceptualized and revised the manuscript. All authors authorized the submission of the manuscript.
Funding
This work was supported in part by the European Commission H2020-FETPROACT-2018-01 NeuHeart Project (#824071) (SM) and European Union’s Horizon 2020 Research and Innovation Program under grant agreement number 874764 (FR).
Conflict of Interest
The authors declare that the research was conducted in the absence of any commercial or financial relationships that could be construed as a potential conflict of interest.
Publisher’s Note
All claims expressed in this article are solely those of the authors and do not necessarily represent those of their affiliated organizations, or those of the publisher, the editors and the reviewers. Any product that may be evaluated in this article, or claim that may be made by its manufacturer, is not guaranteed or endorsed by the publisher.
References
1. Buckley U, Shivkumar K, Ardell JL. Autonomic regulation therapy in heart failure. Curr Heart Fail Rep. (2015) 12:284–93. doi: 10.1007/s11897-015-0263-7
2. Wilks SJ, Hara SA, Ross EK, Nicolai EN, Pignato PA, Cates AW, et al. Non-clinical and pre-clinical testing to demonstrate safety of the barostim neo electrode for activation of carotid baroreceptors in chronic human implants. Front Neurosci. (2017) 11:438. doi: 10.3389/fnins.2017.00438
3. Bonaz B, Sinniger V, Pellissier S. Anti-inflammatory properties of the vagus nerve: potential therapeutic implications of vagus nerve stimulation. J Physiol. (2016) 594:5781–90. doi: 10.1113/JP271539
4. Pavlov VA, Tracey KJ. Neural circuitry and immunity. Immunol Res. (2015) 63:38–57. doi: 10.1007/s12026-015-8718-1
6. Chang EH. Changing the tune using bioelectronics. Bioelectron Med. (2021) 7:2. doi: 10.1186/s42234-021-00063-x
7. Olofsson PS, Tracey KJ. Bioelectronic medicine: technology targeting molecular mechanisms for therapy. J Intern Med. (2017) 282:3–4. doi: 10.1111/joim.12624
8. Bouton C. Cracking the neural code, treating paralysis and the future of bioelectronic medicine. J Intern Med. (2017) 282:37–45. doi: 10.1111/joim.12610
9. Karemaker JM. An introduction into autonomic nervous function. Physiol Meas. (2017) 38:R89–118. doi: 10.1088/1361-6579/aa6782
10. Cheng ZJ, Wang R, Chen QH. Autonomic regulation of the cardiovascular system: diseases, treatments, and novel approaches. Neurosci Bull. (2019) 35:1–3. doi: 10.1007/s12264-019-00337-0
11. Horn CC, Ardell JL, Fisher LE. Electroceutical targeting of the autonomic nervous system. Physiology. (2019) 34:150–62. doi: 10.1152/physiol.00030.2018
12. Guarino D, Nannipieri M, Iervasi G, Taddei S, Bruno RM. The role of the autonomic nervous system in the pathophysiology of obesity. Front Physiol. (2017) 8:665. doi: 10.3389/fphys.2017.00665
13. Debnath S, Levy TJ, Bellehsen M, Schwartz RM, Barnaby DP, Zanos S, et al. A method to quantify autonomic nervous system function in healthy, able-bodied individuals. Bioelectron Med. (2021) 7:1–38. doi: 10.1186/s42234-021-00075-7
15. Tracey KJ. Physiology and immunology of the cholinergic antiinflammatory pathway. J Clin Invest. (2007) 117:289–96. doi: 10.1172/JCI30555
16. Won SM, Song E, Reeder JT, Rogers JA. Emerging modalities and implantable technologies for neuromodulation. Cell. (2020) 181:115–35. doi: 10.1016/j.cell.2020.02.054
17. Ardell JL, Nier H, Hammer M, Southerland EM, Ardell CL, Beaumont E, et al. Defining the neural fulcrum for chronic vagus nerve stimulation: implications for integrated cardiac control. J Physiol. (2017) 595:6887–903. doi: 10.1113/JP274678
18. Herring N, Kalla M, Paterson DJ. The autonomic nervous system and cardiac arrhythmias: current concepts and emerging therapies. Nat Rev Cardiol. (2019) 16:707–26. doi: 10.1038/s41569-019-0221-2
19. Dusi V, Ardell JL. Brain-heart afferent-efferent traffic. In: Govoni S, Politi P, Vanoli E editors. Brain and Heart Dynamics. Cham: Springer International Publishing (2020). p. 3–24. doi: 10.1007/978-3-030-28008-6_2
20. Amiya E, Watanabe M, Komuro I. The relationship between vascular function and the autonomic nervous system. Ann Vasc Dis. (2014) 7:109–19. doi: 10.3400/avd.ra.14-00048
21. Olshansky B, Sabbah HN, Hauptman PJ, Colucci WS. Parasympathetic nervous system and heart failure pathophysiology and potential implications for therapy. Circulation. (2008) 118:863–71. doi: 10.1161/CIRCULATIONAHA.107.760405
22. Schwartz PJ, De Ferrari GM. Sympathetic-parasympathetic interaction in health and disease: abnormalities and relevance in heart failure. Heart Fail Rev. (2011) 16:101–7. doi: 10.1007/s10741-010-9179-1
23. Basiago A, Binder DK. Effects of deep brain stimulation on autonomic function. Brain Sci. (2016) 6:33. doi: 10.3390/brainsci6030033
24. Bonaz B, Sinniger V, Pellissier S. The vagus nerve in the neuro-immune axis: implications in the pathology of the gastrointestinal tract. Front Immunol. (2017) 8:1452. doi: 10.3389/fimmu.2017.01452
25. Pavlov VA, Tracey KJ. Bioelectronic medicine: updates, challenges and paths forward. Bioelectron Med. (2019) 5:1. doi: 10.1186/s42234-019-0018-y
26. Câmara R, Griessenauer CJ. Anatomy of the vagus nerve. Nerve Nerve Inj. (2015) 1:385–97. doi: 10.1016/B978-0-12-410390-0.00028-7
27. Kupari J, Häring M, Agirre E, Castelo-Branco G, Ernfors P. An atlas of vagal sensory neurons and their molecular specialization. Cell Rep. (2019) 27:2508–23.e4. doi: 10.1016/j.celrep.2019.04.096
28. Capilupi MJ, Kerath SM, Becker LB. Vagus nerve stimulation and the cardiovascular system. Cold Spring Harb Perspect Med. (2020) 10:a034173. doi: 10.1101/cshperspect.a034173
29. Marmerstein JT, McCallum GA, Durand DM. Direct measurement of vagal tone in rats does not show correlation to HRV. Sci Rep. (2021) 11:1–12. doi: 10.1038/s41598-020-79808-8
30. Bouton C. Neural decoding and applications in bioelectronics medicine. Bioelectron Med. (2015) 2:20–4. doi: 10.15424/bioelectronmed.2014.00012
31. Ganzer PD, Sharma G. Opportunities and challenges for developing closed-loop bioelectronic medicines. Neural Regen Res. (2019) 14:46–50. doi: 10.4103/1673-5374.243697
32. Zanos TP. Recording and decoding of vagal neural signals related to changes in physiological parameters and biomarkers of disease. Cold Spring Harb Perspect Med. (2019) 9:a034157. doi: 10.1101/cshperspect.a034157
33. Hammer N, Löffler S, Cakmak YO, Ondruschka B, Planitzer U, Schultz M, et al. Cervical vagus nerve morphometry and vascularity in the context of nerve stimulation - a cadaveric study. Sci Rep. (2018) 8:1–9. doi: 10.1038/s41598-018-26135-8
34. Hammer N, Glätzner J, Feja C, Kühne C, Meixensberger J, Planitzer U, et al. Human vagus nerve branching in the cervical region. PLoS One. (2015) 10:e0118006. doi: 10.1371/journal.pone.0118006
35. Berthoud HR, Neuhuber WL. Functional and chemical anatomy of the afferent vagal system. Auton Neurosci Basic Clin. (2000) 85:1–17. doi: 10.1016/S1566-0702(00)00215-0
36. Thompson N, Mastitskaya S, Holder D. Avoiding off-target effects in electrical stimulation of the cervical vagus nerve: neuroanatomical tracing techniques to study fascicular anatomy of the vagus nerve. J Neurosci Methods. (2019) 325:108325. doi: 10.1016/j.jneumeth.2019.108325
37. Kawashima T. The autonomic nervous system of the human heart with special reference to its origin, course, and peripheral distribution. Anat Embryol (Berl). (2005) 209:425–38. doi: 10.1007/s00429-005-0462-1
38. Green JH, Heffron PF. The origin of the right aortic nerve in the rabbit. Quart J Exper Physiol. (1966) 51:276–83. doi: 10.1113/expphysiol.1966.sp001863
39. Cohen ML, Georgievskaya Z. Histopathology of the stimulated vagus nerve: primum non nocere. Heart Fail Rev. (2011) 16:163–9. doi: 10.1007/s10741-010-9182-6
40. Seki A, Green HR, Lee TD, Hong L, Tan J, Vinters HV, et al. Sympathetic nerve fibers in human cervical and thoracic vagus nerves. Heart Rhythm. (2010) 14:384–99.
41. Bassi GS, Kanashiro A, Coimbra NC, Terrando N, Maixner W, Ulloa L. Anatomical and clinical implications of vagal modulation of the spleen. Neurosci Biobehav Rev. (2020) 112:363–73. doi: 10.1016/j.neubiorev.2020.02.011
42. Verlinden TJM, Rijkers K, Hoogland G, Herrler A. Morphology of the human cervical vagus nerve: implications for vagus nerve stimulation treatment. Acta Neurol Scand. (2016) 133:173–82. doi: 10.1111/ane.12462
43. Stakenborg N, Gomez-Pinilla PJ, Verlinden TJM, Wolthuis AM, D’Hoore A, Farré R, et al. Comparison between the cervical and abdominal vagus nerves in mice, pigs, and humans. Neurogastroenterol Motil. (2020) 32:e13889. doi: 10.1111/nmo.13889
44. Settell ML, Knudsen BE, Dingle AM, Mcconico AL, Nicolai EN, Trevathan JK, et al. Functional vagotopy in the cervical vagus nerve of the domestic pig : implications for vagus nerve stimulation. J Neural Eng. (2020) 17:026022. doi: 10.1088/1741-2552/ab7ad4
45. Micera S, Navarro X, Carpaneto J, Citi L, Tonet O, Rossini PM, et al. On the use of longitudinal intrafascicular peripheral interfaces for the control of cybernetic hand prostheses in amputees. IEEE Trans Neural Syst Rehabil Eng. (2008) 16:453–72. doi: 10.1109/TNSRE.2008.2006207
46. Raspopovic S, Cimolato A, Panarese A, Vallone F, del Valle J, Micera S, et al. Neural signal recording and processing in somatic neuroprosthetic applications: a review. J Neurosci Methods. (2020) 337:108653. doi: 10.1016/j.jneumeth.2020.108653
47. Thompson N, Ravagli E, Mastitskaya S, Iacoviello F, Aristovich K, Perkins J, et al. MicroCT optimisation for imaging fascicular anatomy in peripheral nerves. J Neurosci Methods. (2020) 338:108652. doi: 10.1101/818237
48. Ravagli E, Mastitskaya S, Thompson N, Welle EJ, Chestek CA, Aristovich K, et al. Fascicle localisation within peripheral nerves through evoked activity recordings: a comparison between electrical impedance tomography and multi-electrode arrays. J Neurosci Methods. (2021) 358:109140. doi: 10.1016/j.jneumeth.2021.109140
49. Evans DHL, Murray JG. Histological and functional studies on the fibre composition of the vagus nerve of the rabbit. J Anat. (1954) 88:320–37.
50. Agostoni E, Chinnock JE, De Daly MB, Murray JG. Functional and histological studies of the vagus nerve and its branches to the heart, lungs and abdominal viscera in the cat. J Physiol. (1957) 135:182–205. doi: 10.1113/jphysiol.1957.sp005703
51. Ruffoli R, Giorgi FS, Pizzanelli C, Murri L, Paparelli A, Fornai F. The chemical neuroanatomy of vagus nerve stimulation. J Chem Neuroanat. (2011) 42:288–96. doi: 10.1016/j.jchemneu.2010.12.002
52. Carr MJ, Undem BJ. Bronchopulmonary afferent nerves. Respirology. (2003) 8:291–301. doi: 10.1046/j.1440-1843.2003.00473.x
53. Zhuo H, Ichikawa H, Helke CJ. Neurochemistry of the nodose ganglion. Prog Neurobiol. (1997) 52:79–107. doi: 10.1016/S0301-0082(97)00003-8
54. Mazzone SB, Undem BJ. Vagal afferent innervation of the airways in health and disease. Physiol Rev. (2016) 96:975–1024. doi: 10.1152/physrev.00039.2015
55. Thayer JF, Loerbroks A, Sternberg EM. Inflammation and cardiorespiratory control: the role of the vagus nerve. Respir Physiol Neurobiol. (2011) 178:387–94. doi: 10.1016/j.resp.2011.05.016
56. Travagli RA, Anselmi L. Vagal neurocircuitry and its influence on gastric motility. Nat Rev Gastroenterol Hepatol. (2016) 13:87–92. doi: 10.1016/j.coviro.2015.09.001.Human
57. Chang EH, Chavan SS, Pavlov VA. Cholinergic control of inflammation, metabolic dysfunction, and cognitive impairment in obesity-associated disorders: mechanisms and novel therapeutic opportunities. Front Neurosci. (2019) 13:263. doi: 10.3389/fnins.2019.00263
58. Rajendran PS, Challis RC, Fowlkes CC, Hanna P, Tompkins JD, Jordan MC, et al. Identification of peripheral neural circuits that regulate heart rate using optogenetic and viral vector strategies. Nat Commun. (2019) 10:1–13. doi: 10.1038/s41467-019-09770-1
59. Min S, Chang RB, Prescott SL, Beeler B, Joshi NR, Strochlic DE, et al. Arterial baroreceptors sense blood pressure through decorated aortic claws. Cell Rep. (2019) 29:2192–201.e3. doi: 10.1016/j.celrep.2019.10.040
60. Paintal AS. The conduction velocities of respiratory and cardiovascular afferent fibres in the vagus nerve. J Physiol. (1953) 121:341–59. doi: 10.1113/jphysiol.1953.sp004950
61. Allen AM, Lewis SJ, Verberne AJM, Mendelsohn FAO. Angiotensin receptors and the vagal system. Clin Exp Hypertens Part A Theory Pract. (1988) 10:1239–49. doi: 10.1080/07300077.1988.11878914
62. Zeng WZ, Marshall KL, Min S, Daou I, Chapleau MW, Abboud FM, et al. PIEZOs mediate neuronal sensing of blood pressure and the baroreceptor reflex. Science. (2018) 362:464–7. doi: 10.1126/science.aau6324
63. Lumbers BYER, Mccloskey DI, Potter EK. Inhibition by angiotensin II of baroreceptor-evoked activity in cardiac vagal efferent nerves in the dog. J Physiol. (1979) 294:69–80. doi: 10.1113/jphysiol.1979.sp012915
64. Longhurst JC. Cardiac receptors: their function in health and disease. Prog Cardiovasc Dis. (1984) 27:201–22. doi: 10.1016/0033-0620(84)90005-7
65. Paintal AS. A study of right and left atrial receptors. J Physiol. (1953) 120:596–610. doi: 10.1113/jphysiol.1953.sp004920
66. Campbell T, Shenton F, Lucking E, Pyner S, Jones J. Electrophysiological characterisation of atrial volume receptors using ex−vivo models of isolated rat cardiac atria. Exp Physiol. (2020) 105:2190–206. doi: 10.1113/ep088972
67. Kashihara K, Kawada T, Yanagiya Y, Uemura K, Inagaki M, Takaki H, et al. Bezold-Jarisch reflex attenuates dynamic gain of baroreflex neural arc. Am J Physiol Heart Circ Physiol. (2003) 285:5–7. doi: 10.1152/ajpheart.01082.2002
68. McAllen RM, Spyer KM. Two types of vagal preganglionic motoneurones projecting to the heart and lungs. J Physiol. (1978) 282:353–64. doi: 10.1113/jphysiol.1978.sp012468
69. Jordan D, Khalid MEM, Schneiderman N, Spyer KM. The location and properties of preganglionic vagal cardiomotor neurones in the rabbit. Pflügers Arch Eur J Physiol. (1982) 395:244–50. doi: 10.1007/BF00584817
70. Machhada A, Marina N, Korsak A, Stuckey DJ, Lythgoe MF, Gourine AV. Origins of the vagal drive controlling left ventricular contractility. J Physiol. (2016) 594:4017–30. doi: 10.1113/JP270984
71. Chapleau MW, Sabharwal R. Methods of assessing vagus nerve activity and reflexes. Heart Fail Rev. (2010) 14:384–99.
72. Grossman P, Taylor EW. Toward understanding respiratory sinus arrhythmia: relations to cardiac vagal tone, evolution and biobehavioral functions. Biol Psychol. (2007) 74:263–85. doi: 10.1016/j.biopsycho.2005.11.014
73. Penry JK, Dean JC. Prevention of intractable partial seizures by intermittent vagal stimulation in humans: preliminary results. Epilepsia. (1990) 31:S40–3. doi: 10.1111/j.1528-1157.1990.tb05848.x
74. George R, Sonnen A, Upton A, Salinsky M, Ristanovic R, Bergen D, et al. A randomized controlled trial of chronic vagus nerve stimulation for treatment of medically intractable seizures. Neurology. (1995) 45:224–30. doi: 10.1212/WNL.45.2.224
75. DeGiorgio C, Heck C, Bunch S, Britton J, Green P, Lancman M, et al. Vagus nerve stimulation for epilepsy: randomized comparison of three stimulation paradigms. Neurology. (2005) 65:317–9. doi: 10.1212/01.wnl.0000168899.11598.00
76. Klinkenberg S, Aalbers MW, Vles JSH, Cornips EMJ, Rijkers K, Leenen L, et al. Vagus nerve stimulation in children with intractable epilepsy: a randomized controlled trial. Dev Med Child Neurol. (2012) 54:855–61. doi: 10.1111/j.1469-8749.2012.04305.x
77. Salinsky MC, Uthman BM, Ristanovic RK, Wernicke JF, Tarver WB. Vagus nerve stimulation for the treatment of medically intractable seizures. Arch Neurol. (1996) 53:1176–80. doi: 10.1001/archneur.1996.00550110128021
78. Rush AJ, George MS, Sackeim HA, Marangell LB, Husain MM, Giller C, et al. Vagus nerve stimulation (VNS) for treatment-resistant depressions: a multicenter study. Biol Psychiatry. (2000) 47:276–86. doi: 10.1016/S0006-3223(99)00304-2
79. Rush AJ, Marangell LB, Sackeim HA, George MS, Brannan SK, Davis SM, et al. Vagus nerve stimulation for treatment-resistant depression: a randomized, controlled acute phase trial. Biol Psychiatry. (2005) 58:347–54. doi: 10.1016/j.biopsych.2005.05.025
80. Ryvlin P, Gilliam FG, Nguyen DK, Colicchio G, Iudice A, Tinuper P, et al. The long-term effect of vagus nerve stimulation on quality of life in patients with pharmacoresistant focal epilepsy: the PuLsE (open prospective randomized long-term effectiveness) trial. Epilepsia. (2014) 55:893–900. doi: 10.1111/epi.12611
81. George MS, Rush AJ, Marangell LB, Sackeim HA, Brannan SK, Davis SM, et al. A one-year comparison of vagus nerve stimulation with treatment as usual for treatment-resistant depression. Biol Psychiatry. (2005) 58:364–73. doi: 10.1016/j.biopsych.2005.07.028
82. Tyler R, Cacace A, Stocking C, Tarver B, Engineer N, Martin J, et al. Vagus nerve stimulation paired with tones for the treatment of tinnitus: a prospective randomized double-blind controlled pilot study in humans. Sci Rep. (2017) 7:1–11. doi: 10.1038/s41598-017-12178-w
83. Tracey Kevin J. The inflammatory reflex. Nature. (2005) 257:122–5. doi: 10.1111/j.1365-2796.2004.01440.x
84. Fitchett A, Mastitskaya S, Aristovich K. Selective neuromodulation of the vagus nerve. Front Neurosci. (2021) 15:685872. doi: 10.3389/fnins.2021.685872
85. Farmer AD, Albu-Soda A, Aziz Q. Vagus nerve stimulation in clinical practice. Br J Hosp Med. (2016) 77:645–51. doi: 10.12968/hmed.2016.77.11.645
86. Beekwilder JP, Beems T. Overview of the clinical applications of vagus nerve stimulation. J Clin Neurophysiol. (2010) 27:130–8. doi: 10.1097/WNP.0b013e3181d64d8a
87. Yuan H, Silberstein SD. Vagus nerve and vagus nerve stimulation, a comprehensive review: part II. Headache. (2016) 56:259–66. doi: 10.1111/head.12650
88. Nicolai EN, Settell ML, Knudsen BE, McConico AL, Gosink BA, Trevathan JK, et al. Sources of off-target effects of vagus nerve stimulation using the helical clinical lead in domestic pigs. J Neural Eng. (2020) 17:046017. doi: 10.1088/1741-2552/ab9db8
89. Guiraud D, Andreu D, Bonnet S, Carrault G, Couderc P, Hagège A, et al. Vagus nerve stimulation: state of the art of stimulation and recording strategies to address autonomic function neuromodulation. J Neural Eng. (2016) 13:041002. doi: 10.1088/1741-2560/13/4/041002
90. Settell ML, Skubal AC, Chen RCH, Kasole M, Knudsen BE, Nicolai EN, et al. In vivo visualization of pig vagus nerve “vagotopy” using ultrasound. Front Neurosci. (2021) 15:676680. doi: 10.3389/fnins.2021.676680
91. Aihua L, Lu S, Liping L, Xiuru W, Hua L, Yuping W. A controlled trial of transcutaneous vagus nerve stimulation for the treatment of pharmacoresistant epilepsy. Epilepsy Behav. (2014) 39:105–10. doi: 10.1016/j.yebeh.2014.08.005
92. Yap JYY, Keatch C, Lambert E, Woods W, Stoddart PR, Kameneva T. Critical review of transcutaneous vagus nerve stimulation : challenges for translation to clinical practice. Front Neurosci. (2020) 14:284. doi: 10.3389/fnins.2020.00284
93. Silberstein SD, Mechtler LL, Kudrow DB, Calhoun AH, McClure C, Saper JR, et al. Non–invasive vagus nerve stimulation for the ACute treatment of cluster headache: findings from the randomized, double-blind, sham-controlled ACT1 study. Headache. (2016) 56:1317–32. doi: 10.1111/head.12896
94. Stefan H, Kreiselmeyer G, Kerling F, Kurzbuch K, Rauch C, Heers M, et al. Transcutaneous vagus nerve stimulation (t-VNS) in pharmacoresistant epilepsies: a proof of concept trial. Epilepsia. (2012) 53:115–8. doi: 10.1111/j.1528-1167.2012.03492.x
95. Henssen DJHA, Derks B, van Doorn M, Verhoogt NC, Staats P, Vissers K, et al. Visualizing the trigeminovagal complex in the human medulla by combining ex-vivo ultra-high resolution structural MRI and polarized light imaging microscopy. Sci Rep. (2019) 9:1–11. doi: 10.1038/s41598-019-47855-5
96. Qing KY, Wasilczuk KM, Ward MP, Phillips EH, Vlachos PP, Goergen CJ, et al. B fibers are the best predictors of cardiac activity during vagus nerve stimulation. Bioelectron Med. (2018) 4:5. doi: 10.1186/s42234-018-0005-8
97. Kaniusas E, Kampusch S, Tittgemeyer M, Panetsos F, Gines RF, Papa M, et al. Current directions in the auricular vagus nerve stimulation I - a physiological perspective. Front Neurosci. (2019) 13:854. doi: 10.3389/fnins.2019.00854
98. de Lartigue G. Role of the vagus nerve in the development and treatment of diet-induced obesity. J Physiol. (2016) 594:5791–815. doi: 10.1113/JP271538
99. Shen MJ. The cardiac autonomic nervous system: an introduction. Herzschrittmachertherapie Elektrophysiologie. (2021) 32:295–301. doi: 10.1007/s00399-021-00776-1
100. Tosato M, Yoshida K, Toft E, Nekrasas V, Struijk JJ. Closed-loop control of the heart rate by electrical stimulation of the vagus nerve. Med Biol Eng Comput. (2006) 44:161–9. doi: 10.1007/s11517-006-0037-1
101. Naggar I, Nakase K, Lazar J, Salciccioli L, Selesnick I, Stewart M. Vagal control of cardiac electrical activity and wall motion during ventricular fibrillation in large animals. Auton Neurosci Basic Clin. (2014) 183:12–22. doi: 10.1016/j.autneu.2014.01.009
102. Komisaruk BR, Frangos E. Vagus nerve afferent stimulation: projection into the brain, reflexive physiological, perceptual, and behavioral responses, and clinical relevance. Auton Neurosci Basic Clin. (2022) 237:102908. doi: 10.1016/j.autneu.2021.102908
103. Yamakawa K, So EL, Rajendran PS, Hoang JD, Makkar N, Mahajan A, et al. Electrophysiological effects of right and left vagal nerve stimulation on the ventricular myocardium. AJP Heart Circ Physiol. (2014) 307:H722–31. doi: 10.1152/ajpheart.00279.2014
104. Machhada A, Hosford PS, Dyson A, Ackland GL, Mastitskaya S, Gourine AV. Optogenetic stimulation of vagal efferent activity preserves left ventricular function in experimental heart failure. JACC Basic to Transl Sci. (2020) 5:799–810. doi: 10.1016/j.jacbts.2020.06.002
105. De Ferrari GM, Schwartz PJ. Vagus nerve stimulation: from pre-clinical to clinical application: challenges and future directions. Heart Fail Rev. (2011) 16:195–203. doi: 10.1007/s10741-010-9216-0
106. Zhao S, Dai Y, Ning X, Tang M, Zhao Y, Li Z, et al. Vagus nerve stimulation in early stage of acute myocardial infarction prevent ventricular arrhythmias and cardiac remodeling. Front Cardiovasc Med. (2021) 8:648910. doi: 10.3389/fcvm.2021.648910
107. Beaumont E, Southerland EM, Hardwick JC, Wright GL, Ryan S, Li Y, et al. Vagus nerve stimulation mitigates intrinsic cardiac neuronal and adverse myocyte remodeling postmyocardial infarction. Am J Physiol Heart Circ Physiol. (2015) 309:H1198–206. doi: 10.1152/ajpheart.00393.2015
108. Li M, Zheng C, Sato T, Kawada T, Sugimachi M, Sunagawa K. Vagal nerve stimulation markedly improves long-term survival after chronic heart failure in rats. Circulation. (2004) 109:120–4. doi: 10.1161/01.CIR.0000105721.71640.DA
109. Zhang Y, Popović ZB, Bibevski S, Fakhry I, Sica DA, Van Wagoner DR, et al. Chronic vagus nerve stimulation improves autonomic control and attenuates systemic inflammation and heart failure progression in a canine high-rate pacing model. Circ Heart Fail. (2009) 2:692–9. doi: 10.1161/CIRCHEARTFAILURE.109.873968
110. Uitterdijk A, Yetgin T, te Lintel Hekkert M, Sneep S, Krabbendam-Peters I, van Beusekom HMM, et al. Vagal nerve stimulation started just prior to reperfusion limits infarct size and no-reflow. Basic Res Cardiol. (2015) 110:508. doi: 10.1007/s00395-015-0508-3
111. Shinlapawittayatorn K, Chinda K, Palee S, Surinkaew S, Kumfu S, Kumphune S, et al. Vagus nerve stimulation initiated late during ischemia, but not reperfusion, exerts cardioprotection via amelioration of cardiac mitochondrial dysfunction. Heart Rhythm. (2014) 11:2278–86. doi: 10.1016/j.hrthm.2014.08.001
112. Nuntaphum W, Pongkan W, Wongjaikam S, Thummasorn S, Tanajak P, Khamseekaew J, et al. Vagus nerve stimulation exerts cardioprotection against myocardial ischemia/reperfusion injury predominantly through its efferent vagal fibers. Basic Res Cardiol. (2018) 113:1–20. doi: 10.1007/s00395-018-0683-0
113. Shinlapawittayatorn K, Chinda K, Palee S, Surinkaew S, Thunsiri K, Weerateerangkul P, et al. Low-amplitude, left vagus nerve stimulation significantly attenuates ventricular dysfunction and infarct size through prevention of mitochondrial dysfunction during acute ischemia-reperfusion injury. Heart Rhythm. (2013) 10:1700–7. doi: 10.1016/j.hrthm.2013.08.009
114. Menuet C, Connelly AA, Bassi JK, Melo MR, Le S, Kamar J, et al. Prebötzinger complex neurons drive respiratory modulation of blood pressure and heart rate. Elife. (2020) 9:1–30. doi: 10.7554/eLife.57288
115. Thayer JF, Lane RD. The role of vagal function in the risk for cardiovascular disease and mortality. Biol Psychol. (2007) 74:224–42. doi: 10.1016/j.biopsycho.2005.11.013
116. Gold MR, Veldhuisen DJ, Hauptman PJ, Borggrefe M, Kubo SH, Lieberman RA, et al. Vagus nerve stimulation for the treatment of heart failure: the INOVATE-HF trial. J Am Coll Cardiol. (2016) 68:149–58. doi: 10.1016/j.jacc.2016.03.525
117. De Ferrari GM, Tuinenburg AE, Ruble S, Brugada J, Klein H, Butter C, et al. Rationale and study design of the neurocardiac therapy for heart failure study: NECTAR-HF. Eur J Heart Fail. (2014) 16:692–9. doi: 10.1002/ejhf.80
118. Zannad F, De Ferrari GM, Tuinenburg AE, Wright D, Brugada J, Butter C, et al. Chronic vagal stimulation for the treatment of low ejection fraction heart failure: results of the neural cardiac therapy for heart failure (NECTAR-HF) randomized controlled trial. Eur Heart J. (2015) 36:425–33. doi: 10.1093/eurheartj/ehu345
119. De Ferrari GM, Crijns HJGM, Borggrefe M, Milasinovic G, Smid J, Zabel M, et al. Chronic vagus nerve stimulation: a new and promising therapeutic approach for chronic heart failure. Eur Heart J. (2011) 32:847–55. doi: 10.1093/eurheartj/ehq391
120. Schwartz PJ, De Ferrari GM, Sanzo A, Landolina M, Rordorf R, Raineri C, et al. long term vagal stimulation in patients with advanced heart failure first experience in man. Eur J Heart Fail. (2008) 10:884–91. doi: 10.1016/j.ejheart.2008.07.016
121. De Ferrari GM, Stolen C, Tuinenburg AE, Wright DJ, Brugada J, Butter C, et al. Long-term vagal stimulation for heart failure: eighteen month results from the neural cardiac therapy for heart failure (NECTAR-HF) trial. Int J Cardiol. (2017) 244:229–34. doi: 10.1016/j.ijcard.2017.06.036
122. Nearing BD, Libbus I, Amurthur B, Kenknight BH, Verrier RL. Acute autonomic engagement assessed by heart rate dynamics during vagus nerve stimulation in patients with heart failure in the ANTHEM-HF trial. J Cardiovasc Electrophysiol. (2016) 27:1072–7. doi: 10.1111/jce.13017
123. Sant’Anna LB, Couceiro SLM, Ferreira EA, Sant’Anna MB, Cardoso PR, Mesquita ET, et al. Vagal neuromodulation in chronic heart failure with reduced ejection fraction: a systematic review and meta-analysis. Front Cardiovasc Med. (2021) 8:766676. doi: 10.3389/fcvm.2021.766676
124. Hauptman PJ, Schwartz PJ, Gold MR, Borggrefe M, Van Veldhuisen DJ, Starling RC, et al. Rationale and study design of the increase of vagal tone in heart failure study: INOVATE-HF. Am Heart J. (2012) 163:954–62.e1. doi: 10.1016/j.ahj.2012.03.021
125. Yamaguchi N, Yamakawa K, Rajendran PS, Takamiya T, Vaseghi M. Antiarrhythmic effects of vagal nerve stimulation after cardiac sympathetic denervation in the setting of chronic myocardial infarction. Heart Rhythm. (2018) 15:1214–22. doi: 10.1016/j.hrthm.2018.03.012
126. Tran N, Asad Z, Elkholey K, Scherlag BJ, Po SS, Stavrakis S. Autonomic neuromodulation acutely ameliorates left ventricular strain in humans. J Cardiovasc Transl Res. (2019) 12:221–30. doi: 10.1007/s12265-018-9853-6
127. DiCarlo LA, Libbus I, Kumar HU, Mittal S, Premchand RK, Amurthur B, et al. Autonomic regulation therapy to enhance myocardial function in heart failure patients: the ANTHEM-HFpEF study. ESC Heart Fail. (2018) 5:95–100. doi: 10.1002/ehf2.12241
128. Libbus I, Nearing BD, Amurthur B, KenKnight BH, Verrier RL. Autonomic regulation therapy suppresses quantitative T-wave alternans and improves baroreflex sensitivity in patients with heart failure enrolled in the ANTHEM-HF study. Heart Rhythm. (2016) 13:721–8. doi: 10.1016/j.hrthm.2015.11.030
129. Klein HU, De Ferrari GM. Vagus nerve stimulation: a new approach to reduce heart failure. Cardiol J. (2010) 17:638–43.
130. Murray K, Reardon C. The cholinergic anti-inflammatory pathway revisited. Neurogastroenterol Motil. (2018) 30:1–6. doi: 10.1111/nmo.13288
131. Aaronson ST, Carpenter LL, Conway CR, Reimherr FW, Lisanby SH, Schwartz TL, et al. Vagus nerve stimulation therapy randomized to different amounts of electrical charge for treatment-resistant depression: acute and chronic effects. Brain Stimul. (2013) 6:631–40. doi: 10.1016/j.brs.2012.09.013
132. Famm K, Litt B, Tracey KJ, Boyden ES, Slaoui M. A jump-start for electroceuticals. Nature. (2013) 496:159–61. doi: 10.1038/496159a
133. Cracchiolo M, Ottaviani MM, Panarese A, Strauss I, Vallone F, Mazzoni A, et al. Bioelectronic medicine for the autonomic nervous system: clinical applications and perspectives. J Neural Eng. (2021) 18. doi: 10.1088/1741-2552/abe6b9
134. Raspopovic S, Capogrosso M, Micera S. A computational model for the stimulation of rat sciatic nerve using a transverse intrafascicular multichannel electrode. IEEE Trans Neural Syst Rehabil Eng. (2011) 19:333–44. doi: 10.1109/TNSRE.2011.2151878
135. Romeni S, Valle G, Mazzoni A, Micera S. Tutorial: a computational framework for the design and optimization of peripheral neural interfaces. Nat Protoc. (2020) 15:3129–53. doi: 10.1038/s41596-020-0377-6
136. Raspopovic S, Capogrosso M, Badia J, Navarro X, Micera S. Experimental validation of a hybrid computational model for selective stimulation using transverse intrafascicular multichannel electrodes. IEEE Trans Neural Syst Rehabil Eng. (2012) 20:395–404. doi: 10.1109/TNSRE.2012.2189021
137. Sun FT, Morrell MJ. Closed-loop Neurostimulation: the clinical experience. Neurotherapeutics. (2014) 11:553–63. doi: 10.1007/s13311-014-0280-3
138. Romero-Ugalde HM, Le Rolle V, Bonnet J-L, Henry C, Mabo P, Carrault G, et al. Closed-loop vagus nerve stimulation based on state transition models. IEEE Trans Biomed Eng. (2018) 65:1630–8. doi: 10.1109/TBME.2017.2759667
139. Cracchiolo M, Valle G, Petrini F, Strauss I, Granata G, Stieglitz T, et al. Decoding of grasping tasks from intraneural recordings in trans-radial amputee. J Neural Eng. (2020) 17:026034. doi: 10.1088/1741-2552/ab8277
140. Jastrzebska-Perfect P, Chowdhury S, Spyropoulos GD, Zhao Z, Cea C, Gelinas JN, et al. Translational neuroelectronics. Adv Funct Mater. (2020) 1909165:1–31. doi: 10.1002/adfm.201909165
141. Ereifej ES, Shell CE, Schofield JS, Charkhkar H, Cuberovic I, Dorval AD, et al. Neural engineering: the process, applications, and its role in the future of medicine. J Neural Eng. (2019) 16:063002. doi: 10.1088/1741-2552/ab4869
142. Kaniusas E, Kampusch S, Tittgemeyer M, Panetsos F, Gines RF, Papa M, et al. Current directions in the auricular vagus nerve stimulation II - an engineering perspective. Front Neurosci. (2019) 13:772. doi: 10.3389/fnins.2019.00772
143. Dirr EW, Urdaneta ME, Patel Y, Johnson RD, Campbell-Thompson M, Otto KJ. Designing a bioelectronic treatment for type 1 diabetes: targeted parasympathetic modulation of insulin secretion. Bioelectron Med. (2020) 3:17–31. doi: 10.2217/bem-2020-0006
144. Sevcencu C, Nielsen TN, Struijk JJ. An intraneural electrode for bioelectronic medicines for treatment of hypertension. Neuromodulation. (2018) 21:777–86. doi: 10.1111/ner.12758
145. Plachta DTT, Gierthmuehlen M, Cota O, Espinosa N, Boeser F, Herrera TC, et al. Blood pressure control with selective vagal nerve stimulation and minimal side effects. J Neural Eng. (2014) 11:036011. doi: 10.1088/1741-2560/11/3/036011
146. Gonzalez AG, Etienne-cummings R, Georgiou P. Closed-loop bioelectronic medicine for diabetes management. Bioelectron Med. (2020) 6:4. doi: 10.1186/s42234-020-00046-4
147. Navarro X, Krueger TB, Lago N, Micera S, Stieglitz T, Dario P. A critical review of interfaces with the peripheral nervous system for the control of neuroprotheses and hybrid bionic systems. J Peripher Nerv Syst. (2005) 10:229–58. doi: 10.1111/j.1085-9489.2005.10303.x
148. Boretius T, Badia J, Pascual-Font A, Schuettler M, Navarro X, Yoshida K, et al. A transverse intrafascicular multichannel electrode (TIME) to interface with the peripheral nerve. Biosens Bioelectron. (2010) 26:62–9. doi: 10.1016/j.bios.2010.05.010
149. Rijnbeek EH, Eleveld N, Olthuis W. Update on peripheral nerve electrodes for closed-loop neuroprosthetics. Front Neurosci. (2018) 12:350. doi: 10.3389/fnins.2018.00350
150. Cutrone A, Valle J, Del, Santos D, Badia J, Filippeschi C, Micera S, et al. A three-dimensional self-opening intraneural peripheral interface ({SELINE}). J Neural Eng. (2015) 12:16016. doi: 10.1088/1741-2560/12/1/016016
151. Strauss I, Niederhoffer T, Giannotti A, Panarese AM, Bernini F, Gabisonia K, et al. The Q-PINE: a quick-to-implant peripheral intraneural electrode. J Neural Eng. (2020) 17. doi: 10.1088/1741-2552/abc52a
152. Vallone F, Ottaviani MM, Dedola F, Cutrone A, Romeni S, Macrì Panarese A, et al. Simultaneous decoding of cardiovascular and respiratory functional changes from pig intraneural vagus nerve signals. J Neural Eng. (2021) 18. doi: 10.1088/1741-2552/ac0d42
153. Zanos TP, Silverman HA, Levy T, Tsaava T, Battinelli E, Lorraine PW, et al. Identification of cytokine-specific sensory neural signals by decoding murine vagus nerve activity. Proc Natl Acad Sci USA. (2018) 115:E4843–52. doi: 10.1073/pnas.1719083115
154. Plachta DTT, Espinosa N, Gierthmuehlen M, Cota O, Herrera TC, Stieglitz T. Detection of baroreceptor activity in rat vagal nerve recording using a multi-channel cuff-electrode and real-time coherent averaging. Proc Annu Int Conf IEEE Eng Med Biol Soc EMBS. (2012) 2012:3416–9. doi: 10.1109/EMBC.2012.6346699
155. Sevcencu C, Nielsen TN, Struijk JJ. A neural blood pressure marker for bioelectronic medicines for treatment of hypertension. Biosens Bioelectron. (2017) 98:1–6. doi: 10.1016/j.bios.2017.06.031
156. Rozman J, Ribarič S. Selective recording of electroneurograms from the left vagus nerve of a dog during stimulation of cardiovascular or respiratory systems. Chin J Physiol. (2007) 50:240–50.
157. Citi L, Carpaneto J, Yoshida K, Hoffmann KP, Koch KP, Dario P, et al. On the use of wavelet denoising and spike sorting techniques to process electroneurographic signals recorded using intraneural electrodes. J Neurosci Methods. (2008) 172:294–302. doi: 10.1016/j.jneumeth.2008.04.025
158. Rossini PM, Micera S, Benvenuto A, Carpaneto J, Cavallo G, Citi L, et al. Double nerve intraneural interface implant on a human amputee for robotic hand control. Clin Neurophysiol. (2010) 121:777–83. doi: 10.1016/j.clinph.2010.01.001
159. Micera S, Citi L, Rigosa J, Carpaneto J, Raspopovic S, Di Pino G, et al. Decoding information from neural signals recorded using intraneural electrodes: toward the development of a neurocontrolled hand prosthesis. Proc IEEE. (2010) 98:407–17. doi: 10.1109/JPROC.2009.2038726
160. Musick KM, Rigosa J, Narasimhan S, Wurth S, Capogrosso M, Chew DJ, et al. Chronic multichannel neural recordings from soft regenerative microchannel electrodes during gait. Sci Rep. (2015) 5:1–9. doi: 10.1038/srep14363
161. Tombini M, Rigosa J, Zappasodi F, Porcaro C, Citi L, Carpaneto J, et al. Combined analysis of cortical (EEG) and nerve stump signals improves robotic hand control. Neurorehabil Neural Repair. (2012) 26:275–81. doi: 10.1177/1545968311408919
162. Pani D, Barabino G, Citi L, Meloni P, Raspopovic S, Micera S, et al. Real-time neural signals decoding onto off-the-shelf DSP processors for neuroprosthetic applications. IEEE Trans Neural Syst Rehabil Eng. (2016) 24:993–1002. doi: 10.1109/TNSRE.2016.2527696
163. Rey HG, Pedreira C, Quian Quiroga R. Past, present and future of spike sorting techniques. Brain Res Bull. (2015) 119:106–17. doi: 10.1016/j.brainresbull.2015.04.007
164. Kamavuako EN, Jensen W, Yoshida K, Kurstjens M, Farina D. A criterion for signal-based selection of wavelets for denoising intrafascicular nerve recordings. J Neurosci Methods. (2010) 186:274–80. doi: 10.1016/j.jneumeth.2009.11.022
165. Diedrich A, Charoensuk W, Brychta RJ, Ertl AC, Shiavi R. Analysis of raw microneurographic recordings based on wavelet de-noising technique and classification algorithm: wavelet analysis in microneurography. EEE Trans Biomed Eng. (2003) 50:41–50. doi: 10.1109/TBME.2002.807323.Analysis
166. Ottaviani MM, Wright L, Dawood T, Macefield VG. In vivo recordings from the human vagus nerve using ultrasound−guided microneurography. J Physiol. (2020) 598:3569–76. doi: 10.1113/JP280077
167. Battinelli E, Levy T, Tsaava T, Bouton CE, Tracey KJ, Chavan SS, et al. Identification of hypoglycemia-specific neural signals by decoding murine vagus nerve. Bioelectron Med. (2018) 115:E4843–52.
168. Spearman BS, Desai VH, Mobini S, McDermott MD, Graham JB, Otto KJ, et al. Tissue-engineered peripheral nerve interfaces. Adv Funct Mater. (2018) 28:1–18. doi: 10.1002/adfm.201701713
169. Micera S, Rossini PM, Rigosa J, Citi L, Carpaneto J, Raspopovic S, et al. Decoding of grasping information from neural signals recorded using peripheral intrafascicular interfaces. J Neuroeng Rehabil. (2011) 8:53. doi: 10.1186/1743-0003-8-53
170. Badia J, Raspopovic S, Carpaneto J, Micera S, Navarro X. Spatial and functional selectivity of peripheral nerve signal recording with the transversal intrafascicular multichannel electrode (TIME). IEEE Trans Neural Syst Rehabil Eng. (2016) 24:20–7. doi: 10.1109/TNSRE.2015.2440768
171. Raspopovic S, Carpaneto J, Udina E, Navarro X, Micera S. On the identification of sensory information from mixed nerves by using single-channel cuff electrodes. J Neuroeng Rehabil. (2010) 7:1–15. doi: 10.1186/1743-0003-7-17
172. Wurth S, Capogrosso M, Raspopovic S, Gandar J, Federici G, Kinany N, et al. Long-term usability and bio-integration of polyimide-based intra-neural stimulating electrodes. Biomaterials. (2017) 122:114–29. doi: 10.1016/j.biomaterials.2017.01.014
173. Davey CE, Soto-Breceda A, Shafton A, McAllen RM, Furness JB, Grayden DB, et al. A new algorithm for drift compensation in multi-unit recordings of action potentials in peripheral autonomic nerves over time. J Neurosci Methods. (2020) 338:108683. doi: 10.1016/j.jneumeth.2020.108683
174. Marmerstein JT, Mccallum GA, Durand DM. Decoding vagus-nerve activity with carbon nanotube sensors in freely moving rodents. Biosensors. (2022) 12:114. doi: 10.3390/bios12020114
175. Vespa S, Stumpp L, Bouckaert C, Delbeke J, Smets H, Cury J, et al. Vagus nerve stimulation-induced laryngeal motor evoked potentials: a possible biomarker of effective nerve activation. Front Neurosci. (2019) 13:880. doi: 10.3389/fnins.2019.00880
176. Yoo PB, Liu H, Hincapie JG, Ruble SB, Hamann JJ, Grill WM. Modulation of heart rate by temporally patterned vagus nerve stimulation in the anesthetized dog. Physiol Rep. (2016) 4:1–10. doi: 10.14814/phy2.12689
177. Chang YC, Cracchiolo M, Ahmed U, Mughrabi I, Gabalski A, Daytz A, et al. Quantitative estimation of nerve fiber engagement by vagus nerve stimulation using physiological markers. Brain Stimul. (2020) 13:1617–30. doi: 10.1016/j.brs.2020.09.002
178. Parker JL, Shariati NH, Karantonis DM. Electrically evoked compound action potential recording in peripheral nerves. Bioelectron Med. (2018) 1:71–83. doi: 10.2217/bem-2017-0005
179. El Tahry R, Raedt R, Mollet L, De Herdt V, Wyckuys T, Van Dycke A, et al. A novel implantable vagus nerve stimulation system (ADNS-300) for combined stimulation and recording of the vagus nerve: pilot trial at Ghent university hospital. Epilepsy Res. (2010) 92:231–9. doi: 10.1016/j.eplepsyres.2010.10.007
180. Rozman J, Pečlin P, Ribarič S, Godec M, Burja J. An improved method of crafting a multi-electrode spiral cuff for the selective stimulation of peripheral nerves. Sci Rep. (2018) 8:915. doi: 10.1038/s41598-018-19318-w
181. Ordelman SCMA, Kornet L, Cornelussen R, Buschman HPJ, Veltink PH. An indirect component in the evoked compound action potential of the vagal nerve. J Neural Eng. (2010) 7:066001. doi: 10.1088/1741-2560/7/6/066001
182. Stieglitz T. Of man and mice: translational research in neurotechnology. Neuron. (2020) 105:12–5. doi: 10.1016/j.neuron.2019.11.030
Keywords: autonomic nervous system, vagus nerve stimulation, cardiovascular diseases, neural decoding, closed-loop
Citation: Ottaviani MM, Vallone F, Micera S and Recchia FA (2022) Closed-Loop Vagus Nerve Stimulation for the Treatment of Cardiovascular Diseases: State of the Art and Future Directions. Front. Cardiovasc. Med. 9:866957. doi: 10.3389/fcvm.2022.866957
Received: 31 January 2022; Accepted: 14 March 2022;
Published: 07 April 2022.
Edited by:
Deborah Hunt, Mercy College, United StatesReviewed by:
Ankit Gilani, Cornell University, United StatesCharles C. Horn, University of Pittsburgh, United States
Copyright © 2022 Ottaviani, Vallone, Micera and Recchia. This is an open-access article distributed under the terms of the Creative Commons Attribution License (CC BY). The use, distribution or reproduction in other forums is permitted, provided the original author(s) and the copyright owner(s) are credited and that the original publication in this journal is cited, in accordance with accepted academic practice. No use, distribution or reproduction is permitted which does not comply with these terms.
*Correspondence: Fabio A. Recchia, ZmFiaW8ucmVjY2hpYUBzYW50YW5uYXBpc2EuaXQ=