Genetic risk factors for postoperative atrial fibrillation—a nationwide genome-wide association study (GWAS)
- 1Department of Surgery and Transplantation, Copenhagen University Hospital, Rigshosptialet, Denmark
- 2Center for Surgical Translational and Artificial Intelligence Research (CSTAR), Copenhagen University Hospital, Rigshospitalet, Denmark
- 3Institute of Clinical Medicine, University of Copenhagen Medical School, Copenhagen, Denmark
Background: Atrial fibrillation (AF) is a major cause of morbidity with a high prevalence among the elderly and has an established genetic disposition. Surgery is a well-known risk factor for AF; however, it is currently not recognized how much common genetic variants influence the postoperative risk. The purpose of this study was to identify Single Nucleotide Polymorphisms associated with postoperative AF.
Methods: The UK Biobank was utilized to conduct a Genome-Wide Association Study (GWAS) to identify variants associated with AF after surgery. An initial discovery GWAS was performed in patients that had undergone surgery with subsequent replication in a unique non-surgical cohort. In the surgical cohort, cases were defined as newly diagnosed AF within 30 days after surgery. The threshold for significance was set at 5 × 10−8.
Results: After quality control, 144,196 surgical patients with 254,068 SNPs were left for analysis. Two variants (rs17042171 (p = 4.86 × 10−15) and rs17042081 (p = 7.12 × 10−15)) near the PITX2-gene reached statistical significance. These variants were replicated in the non-surgical cohort (1.39 × 10−101 and 1.27 × 10−93, respectively). Several other loci were significantly associated with AF in the non-surgical cohort.
Conclusion: In this GWAS-analysis of a large national biobank, we identified 2 variants that were significantly associated with postoperative AF. These variants were subsequently replicated in a unique non-surgical cohort. These findings bring new insight in the genetics of postoperative AF and may help identify at-risk patients and guide management.
Introduction
Atrial fibrillation (AF) is a widespread morbidity and the most common cardiac arrythmia especially affecting the elderly population (1). Overall, the prevalence is around 3% rising to 5.9% percent in individuals over the age of 65 (2, 3). The lifetime risk is estimated to be 1 in 4 for men and women older than the age of 40 (4). Besides increasing age, other important risk factors include male gender, congestive heart failure, hypertension, myocardial infarction, diabetes and hyperthyroidism (5–7).
Genetic risk factors for AF have increasingly been getting attention, and the heritability has been estimated to 22% in the UK Biobank and 62% in a twin study from Denmark (8, 9). Wolff et al. were the first to describe a family with a hereditary AF trait in KCNQ1, coding a Kv7.1 potassium channel (10). Since, several other familial loci have been identified, including autosomal dominant traits (11).
More common genetic variants have since been identified by genome-wide association studies (GWAS), with variants in or near PITX2 showing the most solid associations (12). Moreover, genome-wide polygenic risk scores (GPS), calculated on the basis of identified variants from GWAS-models, have managed to identify patients with a three-fold increased risk of AF—a risk that the authors recognize may justify treatment or screening (13).
Postoperative atrial fibrillation (POAF) constitutes its own entity and is the most common secondary form of AF (14). High rates are especially observed after cardiac surgery, with a prevalence ranging from 20% to 40%, with differences most likely explained by differences in screening rates and monitoring, although factors such as type of procedure, size of the heart and age also may play a major role (15–18). Even though POAF is often transient, and spontaneous return to sinus rhythm is common, there is still a significant association between POAF after cardiac procedures and increased long-term risk of subsequent persistent AF as well as long-term mortality and risk of stroke (19–21). POAF is observed at a lower rate after non-cardiac surgery, although the prevalence is still substantial with rates as high as 18% (22). For non-cardiac surgery, the prevalence is highly variable, most likely due to the heterogeneity of the group, as well as differences in screening protocols, with general rates between 0.5% and 15% (23–25). Earlier research have sought to identify genetic risk factors for POAF (26–28). However, these were candidate gene analyses using candidates from previously identified AF risk loci and were only in patients undergoing cardiac surgery (26–28).
As such, there is currently a lack of studies assessing a potential genetic component of POAF through a standard GWAS approach, as well as to investigate whether genetic variations driving POAF differ from those driving AF in the non-surgical setting. This forms the overall focus of this study.
We hypothesized that common variants may contribute to the risk of 30-day-POAF in a cohort of mixed surgical patients, and that these are overlapping with variants associated with AF in the non-surgical setting.
Methods
Phenotypic data
Access to the UK biobank data was approved by the United Kingdom biobank (UKB) consortium (Study ID #60861). Under Danish law, the study was exempt from ethical board approval due to the de-identified nature of the dataset.
We conducted a two-stage GWAS for the risk of POAF. First, a unique cohort of all patients in the UKB with a history of minimally invasive or open surgery was identified. POAF was defined as a new diagnosis (no recorded AF diagnoses prior to surgery) of atrial fibrillation within 30 days after surgery. Subsequently, a second GWAS was conducted in a unique non-surgical cohort to validate variants identified in the surgical cohort, as well as to assess whether an overlap between variants identified in the surgical and non-surgical cohorts could be identified.
UKB is a large, national biobank of more than 500,000 individuals between the age of 40 to 69. All patients were recruited in the years 2006 to 2010 and subsequently followed prospectively for a planned duration of 30 years or longer. All patients currently in the UKB were invited from National Health Service registries and encouraged to complete a comprehensive survey on lifestyle, habits, and medical history. Written consent for participation was given for all participants before inclusion.
Identification of surgical cohort
Surgical history was identified using OPCS4-codes from UK Biobank data field 41,200. Patients with a new diagnosis of AF thirty days post-surgery were classified as cases and those without as controls. POAF was identified using International Statistical Classification of Diseases, 10th revision (ICD-10) and ICD-9 codes. The identifying ICD-10 codes for POAF were I48, I480, I481, I483, I484, I489 and the identifying ICD-9 was 4,273. Comorbidities were identified using the appropriate data fields in the UKB and or ICD-10 codes: Heart failure; ICD-10 codes, diabetes; data field 2,443.
Cardiac procedures were identified as all OPCS4-codes included for analysis in the K-category.
Identification of non-surgical cohort
All patients with available genomic data excluding the surgical patients were included for analysis. Cases were defined as patients with a history of AF using the same ICD-10 and ICD-9 codes as defined in the surgical cohort and controls as those without.
By defining each cohort with the above stated criteria no overlapping occurred.
Quality control of genomic data
Two different arrays were used in the genotyping of patients in UKB. Fifty thousand participants were genotyped using the Affymetrix UK BiLEVE Axiom array while 450,000 were genotyped using the Affymetrix UK Biobank Axiom array. No significant differences exist between the utilized arrays with each genotyping around 850,000 Single Nucleotide Polymorphisms (SNPs).
Quality control was performed using PLINK v1.90b6.16 (Shaun Purcell, MA, US) with a standard approach (29, 30). Patients with cryptic relatedness, sex discrepancies and outlying heterozygosity rates were excluded. With the purpose of only including patients with high quality genomic information, all individuals with a genotype rate under 98% were excluded.
To account for data quality, markers with a missingness rate of more than or equal to 2% were excluded. Further, markers not in Hardy-Weinberg equilibrium, with a threshold set at p < 1 × 10−6, were excluded for controls and only variants with a Minor Allele Frequency (MAF) > 5% were included for analysis. In total, 530,188 variants were excluded the quality control process. Restricting to >5% MAF removed the bulk (338,813 SNPs).
Analyses were rerun with the same parameters on imputed genotypic data. The data was imputed by The Wellcome Centre for Human Genetics (WTCHG).
Statistics
Both GWAS-analyses in the surgical and non-surgical cohort were analyzed with a mixed linear model approach using fastGWA with a sparse genetic relationship matrix (GRM) using GCTA version 1.93 beta for Windows. A p-value of 5 × 10−8 was considered statistically significant. Both additive, recessive, and dominant effects were tested. Additive effects were tested in GCTA, while a model including additive as well as recessive and dominant effects was created using PLINK 1.9 for Windows. Figures (Manhattan and QQ-plots) were created with qqman (R version 4.0.2) (31). All SNPs are symbolized using the dbSNP Reference (rs) number.
Results
Surgical cohort
Overall, 488,377 patients were available in the UBK with genomic data. Following quality control and restriction to surgical patients, 144,196 participants (42,197 excluded from quality control) with 254,068 SNPs were left for analysis. Of these, 1,190 (0.83%) patients had a newly confirmed AF diagnosis up to 30 days after the surgical procedure. Baseline characteristics are listed in Table 1.
Two variants reached statistical significance (p < 5 × 10−8). The variants were rs17042171 (p = 4.86 × 10−15) and rs17042081 (p = 7.12 × 10−15). The most significant effects were additive in both SNPs. A dominant effect was also found to be significant for the same variants, although less than the additive (p = 9.3 × 10−11 and 4.7 × 10−10, respectively). No recessive effects were statistically significant. Both SNPs are downstream variants of PITX2, a gene previously described in association with AF in other patient populations. Similar results were reached in the analysis using imputed data with rs17042171 and rs17042081 both reaching statistical significance (p = 4.2 × 10−15 and 6.1 × 10−15, respectively). The most significant variant was the imputed SNP rs35323767 (p = 1.0 × 10−16), which is also a variant near PITX2. No other regions reached statistical significance in the imputed or non-imputed analyses. The genomic inflation factor, λ, was calculated to be 1.02. The Manhattan plot for this regression is illustrated in Figure 1A and the Quantile-Quantile-plot (QQ-plot) is illustrated in Figure 2A. A list of the 10 most significant SNPs are listed in Table 2.
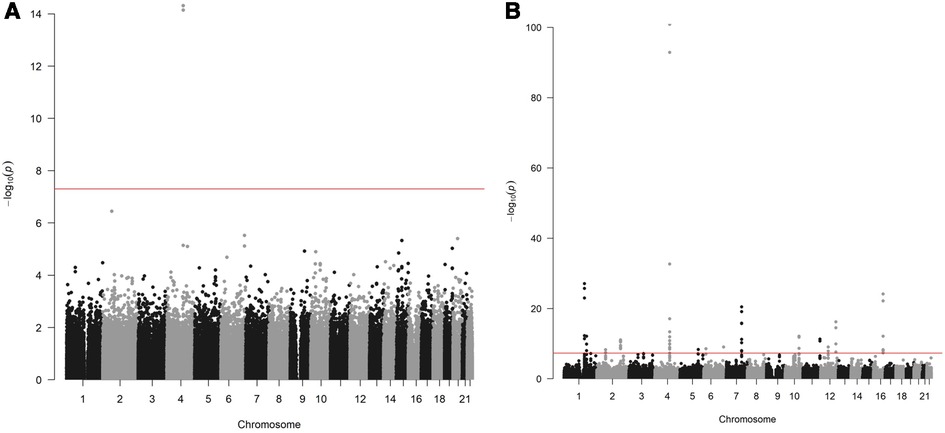
Figure 1. (A) Manhattan plot for discovery genome wide association study for new-onset atrial fibrillation 30-days post-surgery. (B) Manhattan plot for replication genome wide association study for atrial fibrillation in the general population.
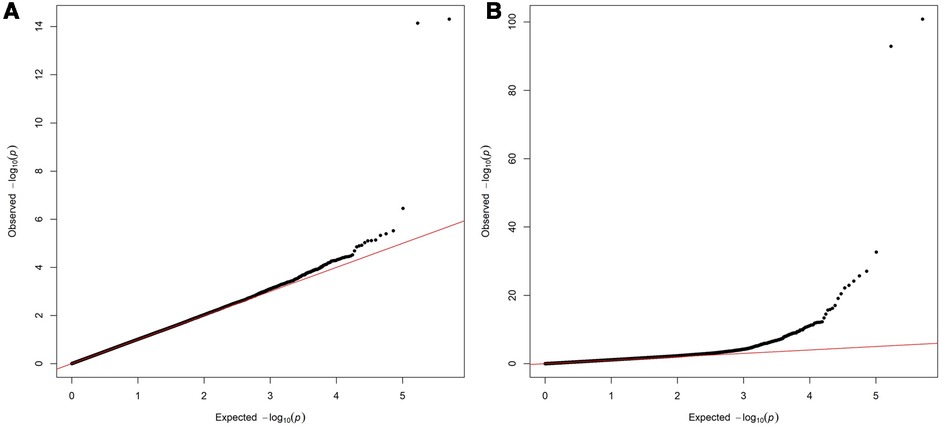
Figure 2. (A) Quantile-Quantile plot for discovery genome wide association for new-onset atrial fibrillation 30-days post-surgery. (B) Quantile-Quantile plot for replication genome wide association for atrial fibrillation in the general population.
A subgroup analysis stratifying by sex was conducted. For females, 80,606 individuals were included for analysis. Of these, 336 (0.42%) had a newly confirmed AF diagnosis up to 30 days after the surgical procedure. No variants reached statistical significance. The variants rs17042171 and rs17042081 were had the highest association (p = 2.36 × 10−7 and p = 5.77 × 10−6, respectively). For males, 63,590 individuals were included for analysis. Of these, 854 (1.36%) had a newly confirmed AF diagnosis up to 30 days after the surgical procedure. The same variants as in the non-stratified analysis reached statistical significance (4.38 × 10−10 and 3.86 × 10−9 for rs17042171 and rs17042081, respectively).
A subgroup analysis stratifying patients by cardiac vs. non-cardiac procedures was conducted. For the cardiac group, 4,162 patients were included. Of these, 522 had POAF diagnosed. No variants reached statistical significance. For the non-cardiac group, 140,034 patients were included. Of these, 668 had POAF diagnosed. Baseline characteristics are listed in Table 3. The same variants as in the main analysis reached statistical significance (6.30 × 10−17 and 1.80 × 10−16 for rs17042171 and rs17042081, respectively).
Non-surgical cohort
Overall, 488,377 patients were available in the UKB with genomic data. Following quality control and exclusion of surgical patients, 301,984 participants (42,192 excluded from quality control) and 254,068 SNPs were left for analysis. Baseline characteristics are listed in Table 4. The variants identified in the discovery surgical cohort, rs17042171 and rs17042081, were also significantly associated with AF (1.39 × 10−101 and 1.27 × 10−93, respectively). Several other loci were found to be associated with AF in this non-surgical cohort, however, none of these loci were found to be associated with POAF. The genomic inflation factor, λ, was calculated to be 1.14. The Manhattan plot for this regression is illustrated in Figure 1B and the QQ-plot is illustrated in Figure 2B. A list of the 10 most significant SNPs are listed in Table 5.
Discussion
We describe the findings of a locus associated with 30-day-POAF in a large GWAS-analysis of a surgical population. Further, this locus was replicated and found to be associated with AF in a cohort of non-surgical patients, as well as having been previously associated with AF in other patient populations (12). Moreover, several loci were found to be associated with AF in the general population without showing any association in the surgical cohort. This could be due to a lack of statistical power but may also be an indicator that fewer genes contribute to the overall risk of POAF compared with general AF, as well as a potential greater contribution of non-genetic factors that we were unable to adequately control for. As some of the pathophysiological mechanisms for POAF differ from general AF, with local inflammation being a contributor to the pathogenesis of POAF, it seems reasonable to assume that the genetic composition of risk factors is also different (32). However, since POAF is a major risk factor for AF, it is indicative that overlapping factors are also present (19).
The variants found to be associated with both POAF and AF in the general population are downstream variants near PITX2 on chromosome 4. The gene has previously been associated with AF in various cohorts, including Icelandic, Swedish and Chinese populations (12, 33). Further, a small candidate gene analysis found an association of the same variants in patients undergoing Coronary Artery Bypass Graft (CABG) Surgery (26). Additionally, the two variants identified in our study are in very high Linkage Disequilibrium (LD) with the primary risk SNP (rs2200733) identified in this study, which emphasizes the replication of this region as a risk factor. To our knowledge, our analysis is the first large-scale GWAS analysis to describe this association in a heterogenous surgical cohort.
The top variant rs17042171_A near PITX2 (position: GRCh38.p13: 110787131) is highly prevalent with an overall global frequency of around 16% and a European prevalence of around 13%. The highest prevalence is seen the Asian population, with a prevalence around 50% (34). The top variant in the imputed analysis, rs35323767, is an imputed SNP also near PITX2. No other regions were found to be statistically significant in the imputed analysis, and all the variants found in the PITX2 are likely to be in LD with the risk variant and not carry inherent risk.
PITX2 and its genetic product, the paired-like homeodomain transcription factor 2, is a well-described gene and is known to have an essential function in cardiac development including left-right asymmetry, pulmonary vein morphogenesis and shortening of the left atrial action potential (35–37). The implication in pulmonary vein development is especially interesting as the veins are often the source of ectopic electrical activity involved in the pathogenesis of AF, and often response to radio-frequency ablation (38). Interestingly, a recent study (39) found that a reduced left atrial expression of PITX2 and elevated plasma concentrations of the PITX2-regulated gene BMP10 predicted recurrence of AF after catheter-based ablation procedures, further highlighting the role of PITX2 in the pathogenesis of AF and the importance of large whole-genome studies in identifying potential biomarkers potentially useful in a clinical setting. Moreover, mice studies have shown a promise in PITX2-guided pharmacotherapy, as it was indicated that flecainide was superior to sotalol in suppressing AF, explained by PITX2-mRNA modulation of atrial membrane resting potential (37). Although the closest gene to the identified variants is PITX2, this analysis does not conclude that PITX2 is the gene modified by the variants associated with POAF as they are intergenic. However, given the role of PITX2 in cardiogenesis and the other well-described effects on AF modulation, and the fact that other nearby genes are microRNA and non-functional, it is deemed the most plausible. Moreover, this analysis cannot conclude that the variants identified are the functional modifiers of transcription, as other non-identified SNPs in LD may be.
Interestingly, variants near PITX2 were the only significant locus in the surgical cohort. Meanwhile, several loci were associated with AF in the general population. Other interesting loci associated with AF in this cohort include rs6666258, an intron variant in KCNN3, which encodes a potassium channel protein which is important for atrial repolarization and has previously been associated with AF in other patient populations (40), as well as the very common rs11773845, an intron variant in CAV1 encoding caveolin-1 which has a great variety of functions and has also been associated with AF in other patient populations (41). While a lower power may explain the lack of significance of these loci in the surgical cohort, it is also possible that PITX2 contribute to a greater proportion of the genetic risk of POAF compared with general AF. This is further emphasized by the fact that none of the significant loci from the non-surgical cohort had a suggestive p-value of less than 5 × 10−5, which is generally considered the cut-off (42). However, we utilized a large cohort, and with a disease prevalence of around 3%, an allele relative risk of 1.5, 1,190 cases and around 140,000 controls, a statistical power of >80% would be expected. With these conditions, it would be expected that our analysis would miss common variants with minor contribution to the overall genetic risk, again suggesting that variants near PITX2 may be more important in the POAF phenotype compared with general AF.
When stratifying the cohorts by sex, the most associated variants remained unchanged in all subgroups. However, the variants failed to reach statistical significance in the surgical female cohort. While this is most likely due to lacking power in this cohort, since the incidence of POAF was only 0.42% with 336 events, it cannot be ruled out to be a consequence of effect modification by sex. When stratifying by cardiac vs. non-cardiac procedure, only a minority of the cohort had a cardiac procedure (4,162 patients, 2.9%). However, roughly half of alle the diagnosed cases of POAF happened in this group, demonstrating that cardiac surgery is a major risk factor for POAF. No variants in the cardiac group reached statistical significance, which we believe to be due to a low number of controls. The non-cardiac group showed similar results as the main, combined analysis.
Genotyping for AF or POAF is not currently common in clinical practice, except for rare familial clusters. Given the morbidity associated with POAF a future approach may include genotyping in preoperative risk assessment, however, this analysis does not currently present an indication. First, replication needs to be done in an independent surgical cohort, secondly, it is currently unknown if genotyping for POAF will decrease the associated morbidity and mortality.
Limitations
This analysis has limitations. First, this is a retrospective case-control study, and hence comes with the inherent limitations, including the inability to assess causation. Secondly, the diagnoses of AF and POAF were made with ICD-9 and ICD-10 codes which may be inaccurate and underreported. Asymptomatic cases are not reported, and cases of POAF may have had AF before the procedure, but not given the diagnosis until after, and therefore not as a direct result of the surgery. Consequently, if any true differences in the genomic risk factors for AF and POAF exist, this may have been influenced by this limitation. Further, the data lacked information on postoperative medication, which may confound the results. Also, other factors such as copy number variations (CNV) could be of importance. Including these in future analyses could potentially add value and identify novel associations. Additionally, the study may have been underpowered to detect variants with minor contributions to the overall genetic risk. Our power calculation assumed a relative risk of 1.5 of the variants in question, however, to detect variants with a relative risk of 1.1 more than 20,000 cases would be needed for a power of 80%. Further, the replication cohort originated from the from the same original dataset as the surgical cohort, as well as being non-surgical, which makes this cohort not a replication set in the truest sense. Ideally, additional research will replicate our findings in an independent, surgical cohort, which will add more value to our proposed results.
Conclusion
In conclusion, we report the association of intergenic variants downstream of PITX2 with postoperative atrial fibrillation in a GWAS-study of a large, national cohort. This finding was replicated in a unique non-surgical cohort with AF. Further, variants associated with AF in the general population were not associated with POAF, indicating that the genomic landscape of AF and POAF may differ.
Data availability statement
Data is not publicly available but can be applied for at https://www.ukbiobank.ac.uk/enable-your-research/apply-for-access. Analytic methods will be made public at github.com at request. Requests to access these datasets should be directed to https://www.ukbiobank.ac.uk/enable-your-research/apply-for-access.
Ethics statement
The studies involving human participants were reviewed and approved by UK Biobank has approval from the North West Multi-centre Research Ethics Committee (MREC) as a Research Tissue Bank (RTB) approval. This approval means that researchers do not require separate ethical clearance and can operate under the RTB approval (there are certain exceptions to this which are set out in the Access Procedures, such as re-contact applications). The patients/participants provided their written informed consent to participate in this study.
Author contributions
Conceptualization: MC, AB and MS. Data Curation: MC, AB. Formal Analysis: MC, AB. Funding Acquisition: MS. Methodology: MC, AB and MS. Resources: MS. Software: MC and AB. Supervision: AB and MS. Visualization: MC and AB. Writing—Original Draft Preparation: MC. Writing—Review & Editing: AB and MS. All authors contributed to the article and approved the submitted version.
Funding
Funded by a grant from the Novo Nordisk Foundation to MS (Grant #NNF20SA0062879).
Conflict of interest
The authors declare that the research was conducted in the absence of any commercial or financial relationships that could be construed as a potential conflict of interest.
Publisher's note
All claims expressed in this article are solely those of the authors and do not necessarily represent those of their affiliated organizations, or those of the publisher, the editors and the reviewers. Any product that may be evaluated in this article, or claim that may be made by its manufacturer, is not guaranteed or endorsed by the publisher.
References
1. Chugh SS, Havmoeller R, Narayanan K, Singh D, Rienstra M, Benjamin EJ, et al. Worldwide epidemiology of atrial fibrillation: a global burden of disease 2010 study. Circulation. (2014) 129(8):837–47. doi: 10.1161/CIRCULATIONAHA.113.005119
2. Ball J, Carrington MJ, McMurray JJ, Stewart S. Atrial fibrillation: profile and burden of an evolving epidemic in the 21st century. Int J Cardiol. (2013) 167(5):1807–24. doi: 10.1016/j.ijcard.2012.12.093
3. Feinberg WM, Blackshear JL, Laupacis A, Kronmal R, Hart RG. Prevalence, age distribution, and gender of patients with atrial fibrillation. Analysis and implications. Arch Intern Med. (1995) 155(5):469–73. doi: 10.1001/archinte.1995.00430050045005
4. Kornej J, Borschel CS, Benjamin EJ, Schnabel RB. Epidemiology of atrial fibrillation in the 21st century: novel methods and new insights. Circ Res. (2020) 127(1):4–20. doi: 10.1161/CIRCRESAHA.120.316340
5. Stewart S, Hart CL, Hole DJ, McMurray JJ. Population prevalence, incidence, and predictors of atrial fibrillation in the Renfrew/Paisley study. Heart. (2001) 86(5):516–21. doi: 10.1136/heart.86.5.516
6. Kannel WB, Wolf PA, Benjamin EJ, Levy D. Prevalence, incidence, prognosis, and predisposing conditions for atrial fibrillation: population-based estimates. Am J Cardiol. (1998) 82(8A):2N–9N. doi: 10.1016/S0002-9149(98)00583-9
7. Kerr CR, Klein GJ. Atrial fibrillation–future directions. Can J Cardiol. (1996) 12(Suppl A):58A–61A.8598004
8. Weng LC, Choi SH, Klarin D, Smith JG, Loh PR, Chaffin M, et al. Heritability of atrial fibrillation. Circ Cardiovasc Genet. (2017) 10(6). doi: 10.1161/CIRCGENETICS.117.001838
9. Christophersen IE, Ravn LS, Budtz-Joergensen E, Skytthe A, Haunsoe S, Svendsen JH, et al. Familial aggregation of atrial fibrillation: a study in Danish twins. Circ Arrhythm Electrophysiol. (2009) 2(4):378–83. doi: 10.1161/CIRCEP.108.786665
10. Wolff L. Familial auricular fibrillation. N Engl J Med. (1943) 229(10):396–8. doi: 10.1056/NEJM194309022291002
11. Brugada R, Tapscott T, Czernuszewicz GZ, Marian AJ, Iglesias A, Mont L, et al. Identification of a genetic locus for familial atrial fibrillation. N Engl J Med. (1997) 336(13):905–11. doi: 10.1056/NEJM199703273361302
12. Gudbjartsson DF, Arnar DO, Helgadottir A, Gretarsdottir S, Holm H, Sigurdsson A, et al. Variants conferring risk of atrial fibrillation on chromosome 4q25. Nature. (2007) 448(7151):353–7. doi: 10.1038/nature06007
13. Khera AV, Chaffin M, Aragam KG, Haas ME, Roselli C, Choi SH, et al. Genome-wide polygenic scores for common diseases identify individuals with risk equivalent to monogenic mutations. Nat Genet. (2018) 50(9):1219–24. doi: 10.1038/s41588-018-0183-z
14. Lubitz SA, Yin X, Rienstra M, Schnabel RB, Walkey AJ, Magnani JW, et al. Long-term outcomes of secondary atrial fibrillation in the community: the Framingham Heart study. Circulation. (2015) 131(19):1648–55. doi: 10.1161/CIRCULATIONAHA.114.014058
15. Aranki SF, Shaw DP, Adams DH, Rizzo RJ, Couper GS, VanderVliet M, et al. Predictors of atrial fibrillation after coronary artery surgery. Current trends and impact on hospital resources. Circulation. (1996) 94(3):390–7. doi: 10.1161/01.CIR.94.3.390
16. Mathew JP, Parks R, Savino JS, Friedman AS, Koch C, Mangano DT, et al. Atrial fibrillation following coronary artery bypass graft surgery: predictors, outcomes, and resource utilization. MultiCenter study of perioperative ischemia research group. JAMA. (1996) 276(4):300–6. doi: 10.1001/jama.1996.03540040044031
17. Kowey PR, Stebbins D, Igidbashian L, Goldman SM, Sutter FP, Rials SJ, et al. Clinical outcome of patients who develop PAF after CABG surgery. Pacing Clin Electrophysiol. (2001) 24(2):191–3. doi: 10.1046/j.1460-9592.2001.00191.x
18. Melduni RM, Schaff HV, Bailey KR, Cha SS, Ammash NM, Seward JB, et al. Implications of new-onset atrial fibrillation after cardiac surgery on long-term prognosis: a community-based study. Am Heart J. (2015) 170(4):659–68. doi: 10.1016/j.ahj.2015.06.015
19. Ahlsson A, Fengsrud E, Bodin L, Englund A. Postoperative atrial fibrillation in patients undergoing aortocoronary bypass surgery carries an eightfold risk of future atrial fibrillation and a doubled cardiovascular mortality. Eur J Cardiothorac Surg. (2010) 37(6):1353–9. doi: 10.1016/j.ejcts.2009.12.033
20. Lahtinen J, Biancari F, Salmela E, Mosorin M, Satta J, Rainio P, et al. Postoperative atrial fibrillation is a major cause of stroke after on-pump coronary artery bypass surgery. Ann Thorac Surg. (2004) 77(4):1241–4. doi: 10.1016/j.athoracsur.2003.09.077
21. Lee SH, Kang DR, Uhm JS, Shim J, Sung JH, Kim JY, et al. New-onset atrial fibrillation predicts long-term newly developed atrial fibrillation after coronary artery bypass graft. Am Heart J. (2014) 167(4):593–600.e1. doi: 10.1016/j.ahj.2013.12.010
22. Amar D, Roistacher N, Burt M, Reinsel RA, Ginsberg RJ, Wilson RS. Clinical and echocardiographic correlates of symptomatic tachydysrhythmias after noncardiac thoracic surgery. Chest. (1995) 108(2):349–54. doi: 10.1378/chest.108.2.349
23. Christians KK, Wu B, Quebbeman EJ, Brasel KJ. Postoperative atrial fibrillation in noncardiothoracic surgical patients. Am J Surg. (2001) 182(6):713–5. doi: 10.1016/S0002-9610(01)00799-1
24. Batra GS, Molyneux J, Scott NA. Colorectal patients and cardiac arrhythmias detected on the surgical high dependency unit. Ann R Coll Surg Engl. (2001) 83(3):174–6.11432135
25. Dobrev D, Aguilar M, Heijman J, Guichard J-B, Nattel S. Postoperative atrial fibrillation: mechanisms, manifestations and management. Nat Rev Cardiol. (2019) 16(7):417–36. doi: 10.1038/s41569-019-0166-5
26. Body SC, Collard CD, Shernan SK, Fox AA, Liu KY, Ritchie MD, et al. Variation in the 4q25 chromosomal locus predicts atrial fibrillation after coronary artery bypass graft surgery. Circ Cardiovasc Genet. (2009) 2(5):499–506. doi: 10.1161/CIRCGENETICS.109.849075
27. Kolek MJ, Muehlschlegel JD, Bush WS, Parvez B, Murray KT, Stein CM, et al. Genetic and clinical risk prediction model for postoperative atrial fibrillation. Circ Arrhythm Electrophysiol. (2015) 8(1):25–31. doi: 10.1161/CIRCEP.114.002300
28. Voudris KV, Apostolakis S, Karyofillis P, Doukas K, Zaravinos A, Androutsopoulos VP, et al. Genetic diversity of the KCNE1 gene and susceptibility to postoperative atrial fibrillation. Am Heart J. (2014) 167(2):274–80.e1. doi: 10.1016/j.ahj.2013.09.020
29. Purcell S, Neale B, Todd-Brown K, Thomas L, Ferreira MA, Bender D, et al. PLINK: a tool set for whole-genome association and population-based linkage analyses. Am J Hum Genet. (2007) 81(3):559–75. doi: 10.1086/519795
30. Chang CC, Chow CC, Tellier LC, Vattikuti S, Purcell SM, Lee JJ. Second-generation PLINK: rising to the challenge of larger and richer datasets. GigaScience. (2015) 4(1). doi: 10.1186/s13742-015-0047-8
31. Turner SD. Qqman: an R package for visualizing GWAS results using Q-Q and manhattan plots. bioRxiv. (2014):005165.
32. Gaudino M, Andreotti F, Zamparelli R, Di Castelnuovo A, Nasso G, Burzotta F, et al. The -174G/C interleukin-6 polymorphism influences postoperative interleukin-6 levels and postoperative atrial fibrillation. Is atrial fibrillation an inflammatory complication? Circulation. (2003) 108(Suppl 1):II195–9. doi: 10.1161/01.cir.0000087441.48566.0d
33. Kaab S, Darbar D, van Noord C, Dupuis J, Pfeufer A, Newton-Cheh C, et al. Large scale replication and meta-analysis of variants on chromosome 4q25 associated with atrial fibrillation. Eur Heart J. (2009) 30(7):813–9. doi: 10.1093/eurheartj/ehn578
34. Phan YJ L, Zhang H, Qiang W, Shekhtman E, Shao D, Revoe D, et al. ALFA: Allele Frequency Aggregator. National Center for Biotechnology Information. U.S. National Library of Medicine. (2020).
35. Hill MC, Kadow ZA, Li L, Tran TT, Wythe JD, Martin JF. A cellular atlas of Pitx2-dependent cardiac development. Development. (2019) 146(12). doi: 10.1242/dev.180398
36. Mommersteeg MT, Brown NA, Prall OW, de Gier-de Vries C, Harvey RP, Moorman AF, et al. Pitx2c and Nkx2-5 are required for the formation and identity of the pulmonary myocardium. Circ Res. (2007) 101(9):902–9. doi: 10.1161/CIRCRESAHA.107.161182
37. Syeda F, Holmes AP, Yu TY, Tull S, Kuhlmann SM, Pavlovic D, et al. PITX2 Modulates atrial membrane potential and the antiarrhythmic effects of sodium-channel blockers. J Am Coll Cardiol. (2016) 68(17):1881–94. doi: 10.1016/j.jacc.2016.07.766
38. Haissaguerre M, Jais P, Shah DC, Takahashi A, Hocini M, Quiniou G, et al. Spontaneous initiation of atrial fibrillation by ectopic beats originating in the pulmonary veins. N Engl J Med. (1998) 339(10):659–66. doi: 10.1056/NEJM199809033391003
39. Reyat JS, Chua W, Cardoso VR, Witten A, Kastner PM, Kabir SN, et al. Reduced left atrial cardiomyocyte PITX2 and elevated circulating BMP10 predict atrial fibrillation after ablation. JCI Insight. (2020) 5(16). doi: 10.1172/jci.insight.139179
40. Ellinor PT, Lunetta KL, Glazer NL, Pfeufer A, Alonso A, Chung MK, et al. Common variants in KCNN3 are associated with lone atrial fibrillation. Nat Genet. (2010) 42(3):240–4. doi: 10.1038/ng.537
41. Liu L, Ebana Y, Nitta JI, Takahashi Y, Miyazaki S, Tanaka T, et al. Genetic variants associated with susceptibility to atrial fibrillation in a Japanese population. Can J Cardiol. (2017) 33(4):443–9. doi: 10.1016/j.cjca.2016.10.029
Keywords: atrial fibrillation, GWAS, SNP, postoperative, personalized medicine, PITX2
Citation: Christensen MA, Bonde A and Sillesen M (2023) Genetic risk factors for postoperative atrial fibrillation—a nationwide genome-wide association study (GWAS). Front. Cardiovasc. Med. 10:1040757. doi: 10.3389/fcvm.2023.1040757
Received: 9 September 2022; Accepted: 29 May 2023;
Published: 19 June 2023.
Edited by:
Sandro Gelsomino, Maastricht University, Netherlands© 2023 Christensen, Bonde and Sillesen. This is an open-access article distributed under the terms of the Creative Commons Attribution License (CC BY). The use, distribution or reproduction in other forums is permitted, provided the original author(s) and the copyright owner(s) are credited and that the original publication in this journal is cited, in accordance with accepted academic practice. No use, distribution or reproduction is permitted which does not comply with these terms.
*Correspondence: Martin Sillesen martin.hylleholt.sillesen@regionh.dk