Image Analysis for Spectroscopic Elemental Dot Maps: P, Al, and Ca Associations in Water Treatment Residuals as a Case Study
- 1Hydro-Geochemistry Laboratory, MIGAL, Galilee Research Institute, Kiryat Shmona, Israel
- 2Department of Environmental Sciences, Faculty of Sciences and Technology, Tel-Hai College, Upper Galilee, Israel
- 3Agrosphere, Institute, IBG-3, Forschungszentrum Jülich GmbH, Jülich, Germany
The associations of elements upon a heterogeneous surface may control nutrients or pollutants sorption and release, having agricultural and environmental implications. This chemical behavior can be elucidated by spatial spectroscopy, followed by image analysis. The purpose of this paper is to present a working procedure for image analysis using the free program ImageJ that can be applied for dot maps of three or more elements produced by solid-state spectroscopy. Detailed step-by-step instructions lead to visual and quantitative information regarding elements associations. The working procedure was demonstrated for P, Al and Ca dot maps produced by scanning electron microscopy energy dispersive spectroscopy (SEM-EDS) for surfaces of Al-based water treatment residuals (Al-WTRs), a by-product of drinking water pretreatment with alum coagulant. Al-WTR was reused to adsorb the macro-nutrient P from polluted soil leach and dairy wastewater (WW). Surficial P onto Al-WTR, SL-Al/O-WTR, and WW-Al/O-WTR (0.56, 0.93, and 2.15%, respectively) displayed sorption dynamics, mostly with Al and Ca. Quantification of the spatial proportions of individual elements and their associations indicated P-Al pool > P-Ca pool (45–24% and 17–7%, respectively). Upon introducing P-rich dairy wastewater, the behavior of P sorption by Al and Ca changed and became more clustered. A ternary phase of P-Al-Ca covered 38% of the area with signal, compared to 4.3 and 4.6% of the area in Al-WTR and SL-Al-WTR, where it was limited to particles edges only. Thus, the presented protocol may promote employing image analysis for geochemical applications, elucidating chemical behavior and affinities. Advantages and pitfalls are discussed.
Introduction
Phosphorus (P) might be an environmental threat for pollution and eutrophication in aquatic settings when impacted by P-rich waste streams (Sims and Sharply, 2005). Yet, those streams can be used as P sources, as P is an essential nutrient for plant growth. Thus, P recycling is of worldwide interest (see review by (Wendling et al., 2013)). Aluminum water treatment residuals (Al-WTR) are known for their great P-sorption capacity (Dayton and Basta, 2005), and a previous study already presented over 90% recovery of P by Al-WTR from waste streams with high potential for subsequent release (Zohar et al., 2017). Identifying major P-binding pools in heterogeneous materials, such as WTRs (Zohar et al., 2020; Zohar et al., 2018) may facilitate its effective recovery. For example, P sequential extraction of Al-WTR and Fe-WTR indicated that major pools to extract P from, were Al and Ca in Al-WTR and Fe and Ca in Fe-WTR and that following mixture with dairy wastewater (WW) the labile P pool grew. However, P surface reactions were emphasized since it was found that P sorption onto WTRs occurs in two steps; the first is rapid sorption onto the surface of WTR, and the second is a slow P diffusion into inner sorption sites (Makris et al., 2004; Massey et al., 2018; Zohar et al., 2018). Thus, it is important to thoroughly examine P dynamics with major pools on top of the materials, as these dynamics have an essential role in P immediate sequestration and partitioning out of the solution.
Spectroscopic solid-state examination of the physio-chemical features and composition of materials surfaces and cross-sections in various scientific fields has increased in recent decades because this approach is less destructive than chemical extraction or more efficient than laborious investigations. In some cases, image analysis, e.g., using ImageJ software, accompanies spectroscopic analysis, contributing to improving understanding of micro and macro processes affecting the materials or the study areas. Many biological and biomedical studies used ImageJ software for image analysis e.g. (Stauffer et al., 2018; Suarez-arnedo et al., 2020; Valente et al., 2017). Geo-physical applications included sedimentary studies (Palma et al., 2019; Fugère et al., 2020) and minerals and salts identification. In the latter case, spectroscopic 2-D dot maps provided spatial information on the distribution and associations of the chemical components, with implications for geology, geomorphology, archeology, hydrology, and construction e.g. (Cardell et al., 2002; El-Gohary, 2016; Kahn et al., 2002; Vázquez et al., 2013). In those cases, the association of two elements provided an indication for the presence of specific salts and minerals, based on their principle ionic chemical composition. However, this approach does not apply when analyzing more complex geochemical situations, like, when the elements of interest are involved in various sorption mechanisms instead of comprising distinct mineral lattices. For example, surface complexes of physical or chemo-adsorption nature and surface complexes initiating precipitation (Avnimelech, 1980; Khare et al., 2005; Ler and Stanforth, 2003), with different pools on heterogeneous surfaces, differently impact the solubility of the sorbed elements and their recovery. Thus, elemental partitioning among significant binding pools may become more relevant than the presence of salts and minerals. This is the case with P, which sequestration includes both adsorption sites onto surfaces and discrete precipitates in poor and in rich P samples (Sø et al., 2011; Zohar et al., 2018).
This paper presents a detailed image analysis procedure of images obtained by scanning electron microscope energy dispersive spectroscopy (SEM-EDS) of Al-WTR materials. This working procedure enables quantification or semi-quantification of elemental associations with two other elements, allowing for affinity determination and deciphering special chemical features and their spatial distribution. In this way, the proposed image analysis is innovative and enables elucidation of more complex chemical interactions than mere association of two elements, which implies the presence of a compound. As a case study, the procedure is demonstrated for P, Al, and Ca in an Al-WTR sample to elucidate P associations with Al and Ca surfaces. The combined analyzed image is compared to the original SEM-EDS dot maps overlay image, emphasizing how they complement each other, adding new insights. The image analysis procedure was applied to two other Al-based WTRs under different treatments and comparison of the final analyzed images enabled a better understanding of P retention trends and processes under various conditions. Advantages and pitfalls of the working scheme are discussed. This working procedure applies to various solid-state examination techniques that yield elemental dot maps when desiring insights regarding the association of several elements. Although the demonstration below consists of 3-element dot maps, it is possible to apply this image analysis working procedure to a larger number of elements.
Materials and Methods
Sample Preparation
Al-WTR formation and organic composites’ production, Al/O-WTRs, are described in detail in (Zohar et al., 2017). In brief, Al-WTR, the residue of surface water clarification treatment (Eshkol reservoir, Israel; 0.002–0.04 mg P L−1 (IOLR, 2016)) using alum coagulant (Al2(SO4)3·4H2O) was air-dried and crushed to < 2 mm grain size. Being the residue of surface water clarification, it contains Al hydroxides (forming following alum application to water), deposited suspended solids (e.g., clay colloids and calcium carbonate), organic particulates, etc. Subsequently, the dried residual sludge was reused to recover P from two organic waste streams: contaminated soil leach (SL) and dairy WW. Both SL and WW streams were pre-clarified from organic solids by centrifugation before mixture with Al-WTR. The soluble inorganic P was measured in the clear liquor (11.5 and 43 mg P L−1, respectively). Al-WTR recovered phosphorus by shaking a mixture containing a ratio of 9 g to 1 L waste liquid (SL or WW) for three days to ensure solid-solution equilibrium; the enriched solid products of this process, namely SL-Al/O-WTR and WW-Al/O-WTR, were each separated from the solution by centrifugation; P recovery level was about 95% (Zohar et al., 2017).
The original Al-WTR and the two products, SL-Al/O-WTR and WW-Al/O-WTR, were examined by SEM equipped with EDS (JEOL JCM-6000 Bench-top, Japan). Analysis performed by SEM-EDS includes information regarding the very thin layer (∼1 μm deep) at the surface of the examined material, in agreement with the focus of the study of surface sorption reactions. Consistently, SEM-EDS analyses were performed on noncoated particles, directly mounted on double-sided adhesive copper conducting tape (3 M®). Analyses used a 15 kV accelerating voltage at 2000 fold magnification, with back-scattered electrons collected under low vacuum. In the SEM-EDS program, distinct characteristic X-rays emitted by each element, following the bombardment of the specimen with high energy electrons (for technique overview and analysis, see (Friel, 2003)), is translated to a 2D spatial image of the sample (i.e., dot maps), representing the counts of the identified X-ray energy emissions. For each of the three materials, dot maps of different elements were produced, including P, Al, and Ca and their overlay maps were produced.
Image Analysis Working Procedure
The working procedure of image analysis was developed for ImageJ software (ImageJ 1.05i, Wayne Rashband, National Institute of Health, United States) and was applied to the dot maps of each of the three elements (namely, P, Al, and Ca) in each of the materials: Al-WTR, SL-Al/O-WTR, and WW-Al/O-WTR. The image processing procedure consists of first-level segmentation by binary transformation of the dot maps of each of the three elements, followed by a second-level segmentation by combining the three elements' binary images using rational operators. Figure 1 and the technical notes that follow describe the image analysis working procedure in detail, including the use of menus and sub-menus of ImageJ software (see selected menus in Supplementary Figure S1).
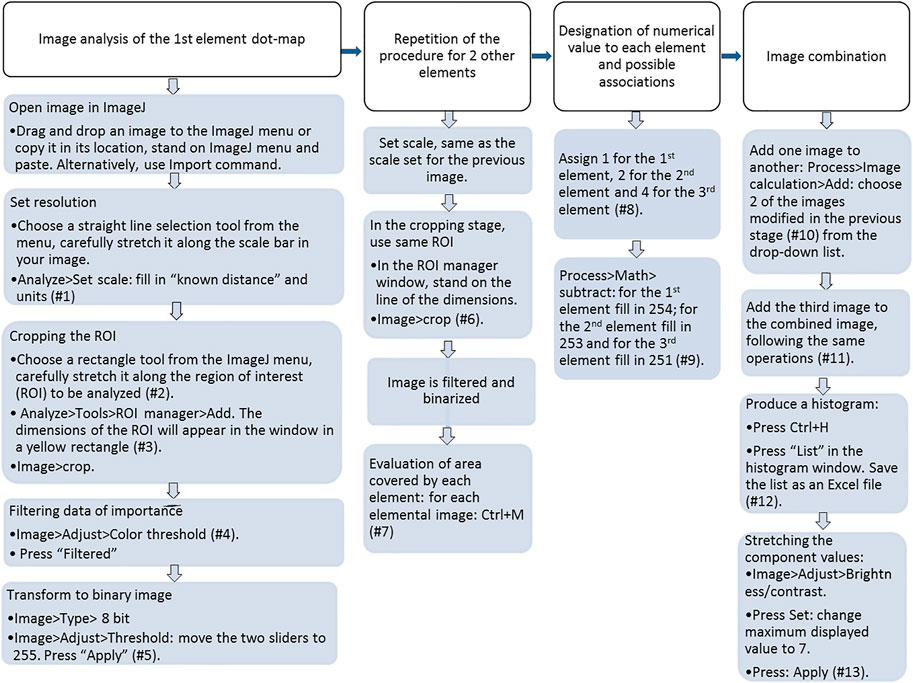
FIGURE 1. Flow-chart diagram of the image analysis working procedure; demonstration of a system with three elements. Numbers refer to listed notes.
A preliminary principal step in the analysis is filtering the image by the automated Brightness threshold. Brightness represents the amount of white in each pixel, making this parameter most appropriate for thresholding SEM-EDS images, since Brightness is predominantly determined by the intensity (relative concentration) of the element in a specific location. Hence, areas in the physical sample with a higher concentration of the element, will show higher intensity (“more white”) in the SEM-EDS image and be given a higher Brightness value. The automated threshold value varies among images (i.e., different dot maps). The ‘cut-off’ value is based on the IsoData method, also known as iterative intermeans (Ridler and Calvard, 1978): the procedure divides the image into object and background by taking an initial threshold and computing the averages of both the pixels at or below the threshold and pixels above it. The average (mid-value) of those two values is computed, and the threshold is incremented accordingly. The process is repeated until the threshold is greater than the composite average. The resulting filtered image is binarized for object and background (255, 0, respectively), in the next step.
Segmentation is crucial; however, its error is unknown as there is no reference to compare it with (Iassonov et al., 2009). Other than concentration, Brightness may also be affected by the angle of incident radiation, the reflectance of the sample and the nature of the analyzed element. The SEM image is sensitive to morphology of the particle, i.e., its microtopography since distance influences X-ray photons’ counting and, therefore, intensity (Bauer et al., 1983). This is a pitfall in unpolished samples or non-smoothed plain samples. To partly overcome this, only multiple-count localities in the image were chosen for investigation. The automated threshold changes from image to image, reflecting the program's sensitivity (Vázquez et al., 2013); however, in cases that include microtopography, the nature of the results may be defined only semi-quantitatively. This is further elaborated on in the case study demonstrated below.
The following segmentation level consists of operations based on elementary arithmetics, including designating a specific value to each image object and subtracting and adding functions. The result is one image that sums the information from 3-elemental images and which obtains eight different segments. The segments include "empty" areas, areas occupied by individual elements, and areas with varying combinations of the three elements. Enabling differentiation of those segments and their semi-quantification can potentially contribute to deciphering geochemical and physical features, like chemical affinity, distribution of chemical entities and physical porosity.
Notes by numbers in the flow chart (Figure 1).
#1 The calculated resolution will be written in pixels per units, as you reported. The “Set scale” box can be used to set the same scale for image processing of the following elements.
#2 Zooming in (Ctrl+↑) can improve accuracy. The region of interest (ROI) should contain all dots that express the object’s presence, but not any other parts of the image (e.g., the scale bar, frame, technical details). Thus, the rectangle that defines the ROI should not include them (Supplementary Figure S1A).
#3 Keep the ROI manager window open for later use (Supplementary Figure S1A).
#4 Note that the diagrams should be in the HSB method (Hue, Saturation, Brightness). Change if needed in the “Color space”. Note that the Threshold color is “white” and that the “Thresholding method” is “Default” (Supplementary Figure S1B). Note the value chosen for minimum in the “Brightness” diagram. The Hue and Saturation ranges should be 0 to 255. Following filtration, the object will appear white, while the below-the-threshold background will appear dark in color. Pressing Ctrl + H will yield a histogram window presenting some statistical details and a histogram with the threshold and background proportion mark. When placing the pointer at the end of the scale, on the value “255”, the count of object pixels is displayed, and likewise, when placing the pointer on the visible peak of the signals below the threshold.
#5 Binary transformation menus are displayed in Supplementary Figure S1C. After the binary transformation, when moving the pointer over the image, the value in the results bar (in the main menu) is either 0 (white) or 255 (black). It is recommended to duplicate the image (Ctrl + shift + D) and save it to your files as this is the final image of a specific element before all elements’ images are added.
#6 A yellow rectangle will appear on the activated image upon selecting the ROI dimensions from the ROI manager window. Those are the dimensions set in the first image analyzed.
#7 The “Results” window will open, showing the total area of the element. When repeating this operation for another element’s image, the new value will appear in the "Results" window. After completing this for the three elements, it is advised to save the results as an Excel file. Alternatively, you can derive this information from the final Excel results file obtained for the list of values (0–7) described in comment #12, below. Note that the three elements' percentage does not sum to 100% as the ROI contains both overlapping elements and empty areas.
#8 Assigning a number to each element (in your records) will enable identifying single elements and their associations in the combined final image by a specific tone and a numerical value. Adjusting the assigned values will allow considering more than three elements. See also comment #9.
#9 A subtraction operation leads to the object in each of the elemental binary images receiving an assigned value, namely, 1 (1st element, very light-toned image), 2 (2nd element), or 4 (3rd element). Further summation of the three images will result in a combined image with segmented areas with values from 0 to 7 (Table 1). For example, in case four elements are of interest, the 4th element should be designated 8, and further combinations will include 9 (1st +4th elements), 10 (2nd +4th elements), 11 (1st +2nd+4th elements), and so on.
#10 The box “Create new window” should be checked. The resulting combined image is named “Result of..” and will have very light gray tones.
#11 The resulting combined image (named “Result of Result of … ”) will be in light gray tones as the tones range from 0 to 7 out of 255.
#12 The list will include the area covered by any of the components, with values 0 to 7. Save the list for further analysis.
#13 Now the image will show the various areas in more visible darker gray tones. This is because each of the tone values (Xi; Xi = 0–7) now has a tone value of Xi*(255/7), i.e., Xi*36.4. Save the final image for further qualitative analysis.

TABLE 1. Possible values for theoretical identity (1–7) in a combined image for three elements and results of the elemental images combination.
Results and Discussion
The Al-Based Water Treatment Residual and the Organic Composites, Al/O-WTRs
The main P pools on the Al-WTR surface, before and after reaction with SL or with dairy WW, were Al oxides and Ca minerals (Zohar et al., 2020). Obviously, these pools compete for P sorption when Al-WTR is mixed with P-rich streams. Interestingly, this competition was further emphasized when Al/O-WTR was allowed to re-equilibrate in KCl solution for 62 days, resulting in Ca enhanced solubility and P-Al enhanced re-sorption after about 14 days, demonstrating P greater affinity to Al (Zohar et al., 2017). The mechanisms governing P sequestration were studied thoroughly previously and were found to consist of adsorption onto Al hydr(oxides) and CaCO3 surfaces, as well as co-precipitation with Al and Ca (Massey et al., 2018; Zohar et al., 2020; Zohar et al., 2018). It is important to emphasize that the Al/O-WTR materials are not pure, being residues of organic and inorganic environmental waste materials, hence they display high heterogeneity. Thus, P sequestration may also involve relatively weak physical adsorption onto low surface affinity constituents (e.g., silica surfaces (Zohar et al., 2018)). Additionally, P may sequester to constituents of strong adsorption, which are of low abundance in WTR and thus their effect is negligible. For example, content of iron (hydr)oxides in Al-WTR was 4.4%, decreasing to 4.2% after mixture with WW (Zohar et al., 2017), iron surficial coverage in Al-WTR was very low (data not shown) and consistently, pool of P-sorbed to Fe (hydr)oxides in the final WW-Al/O-WTR was less than 5% (Zohar et al., 2020). It follows, the current study focuses on P association with the components that displayed the most important role in P sorption, namely, Al and Ca surfaces.
The produced Al/O-WTRs were enriched with P, while the Al and Ca proportions mainly remained unchanged (Table 2). Since Zohar et al. (2017) stressed the importance of the Al-WTR active surfaces for various P sorption mechanisms, SEM-EDS images were acquired for non-polished particles so that P affinity to Al and Ca surfaces could be studied. However, the image analysis results, in this case, are semi-quantitative. The working scheme is demonstrated for the SL-Al/O-WTR surficial dot maps of P, Al, and Ca. The resulting summed image is compared to segmented images of Al-WTR and WW-Al/O-WTR to emphasize trends and processes in elements' distribution and association.

TABLE 2. P, Al and Ca oxide proportions on surfaces and in whole samples of Al-WTR, SL-Al/O-WTR and WW-Al/O-WTR. Modified from (Zohar et al., 2017).
Image Analysis of the SL-Al/O-WTR Scanning Electron Microscopy Energy Dispersive Spectroscopy Images
The original SEM-EDS dot maps of P, Al, and Ca and their overlay images of a 40 m width particle surface of SL-Al/O-WTR are presented in Figure 2 The specific color combinations recognize P’s associations with Al and, Ca: P associations with Al or Ca are marked in yellow or magenta, respectively, while the ternary association (P, Al, and Ca) is marked in white.
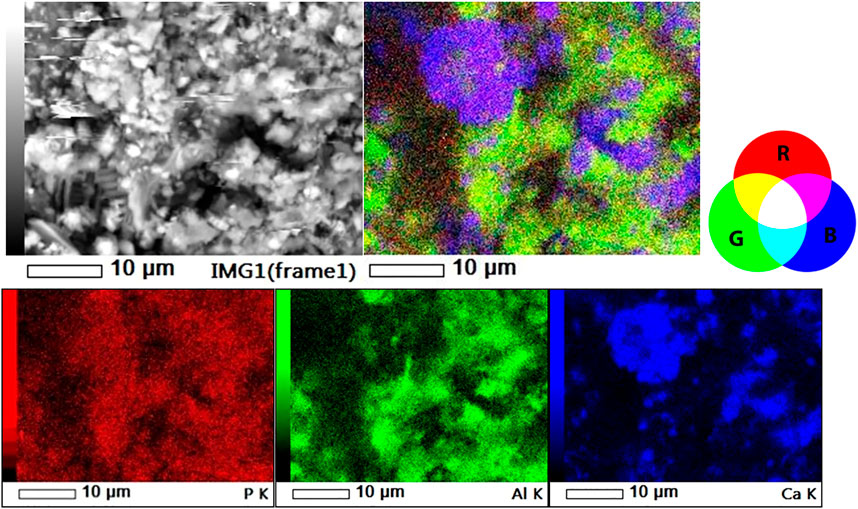
FIGURE 2. SL-Al/O-WTR in the back-scattered image (top left), 3-element overlay image (top right) and individual dot maps of P, Al and Ca (lower left, center and right, respectively).
Upon performing the image analysis protocol, the dot maps of P, Al, and Ca were each cropped and filtered according to the automated color threshold for Brightness. Interestingly, the P threshold (102/255) was lower than those of Al and Ca (121, 122/255, respectively). This trend repeated itself for P, Al, and Ca dot maps of Al-WTR and WW-Al/O-WTR, possibly because of differences in elemental intensities or due to technical artifacts. Areas with microtopography of “deep trenches” in the physical specimen displayed a low signal in the back-scattered image, i.e., in P dot map (Figure 2), and were consistently filtered out of the P, Al, and Ca images (Figures 3, 4) performing a mutual effect. The difference between the P threshold value and those of Al and Ca thus appeared to account for the low P concentrations.
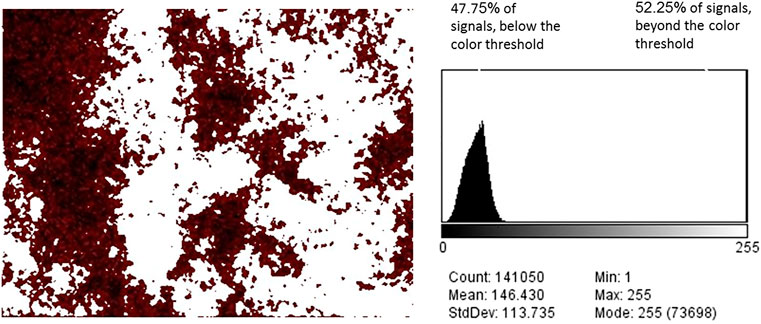
FIGURE 3. P in SL-Al/O-WTR, after color threshold filtration (brightness threshold 102/255). All signals beyond the color threshold turned white (left). The signals below the color threshold constitute 47.75% of the total signals (right).
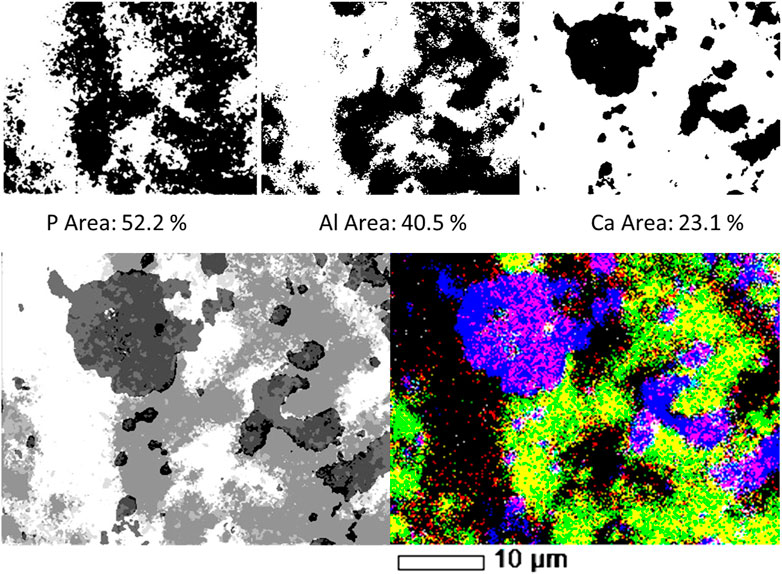
FIGURE 4. Top row: P, Al and Ca individual filtered, binary images, and the areas occupied by each element on SL-Al/O-WTR surface. Bottom row: Combination of three elemental (P, Al and Ca) images of SL-Al/O-WTR. Segmented image, processed by the image analysis working procedure (left) compared to the SEM-EDS overlay map displayed in 100% contrast (right).
Further transformation to the binary format enabled quantifying the area of each of the elements. Although the P content of the sample was substantially lower than that of Al or Ca (0.93%, compared with 20.9 and 9.4%, respectively, Table 2), it covered the largest area in SL-Al/O-WTR image (52.2% compared with 40.5 and 23.1%, respectively, Figure 4). As all pixels with the above threshold values received the exact value of 255 regardless of their different intensities, the spatial results demonstrate how each element’s distribution pattern, rather than its concentration, is emphasized by this working procedure. Elemental distributions suggest a scenario where P introduction upon mixing with SL to Al-WTR triggered rapid sorption by all the potential sorbing components and mainly by the dominant constituents, Al and Ca, finally yielding substantial cover. The presence of Al in the Al-WTRs was shown to be governed by amorphous Al (hydr)oxides, probably precipitated upon Al-WTR formation following the addition of the alum coagulant to the surface water (Zohar et al., 2020). This can explain the high cover of Al (hydr)oxides on the surface. Calcium (associated with C, data not shown) was predominated by calcite, CaCO3 (Zohar et al., 2020), and characterized by a particulate nature.
The objects in the P, Al, and Ca images attained a value of 255 following binarization; thus, to assign each object a different value, each image was subjected to a different subtraction value. A value of 254 was subtracted from the P image, resulting in the object obtaining a value of 1. Similarly, the objects in the Al and Ca images obtained values of 2 and 4, respectively, after 253 and 251 were subtracted from their respective images. An arithmetic operation of adding in two stages resulted in a combined image of the three elements. According to their numerical values, the chemical information was displayed in segments of 8 tones, as presented in Table 1 and Figure 4 (bottom row, left image). The SEM-EDS 3-element overlay map of SL-Al/O-WTR is shown at 100% contrast for comparison (Figure 4, bottom row, right image), to improve the emphasis of elemental associations and features. It should be noted that a color image (the SEM-EDS, right) is superior to the gray-scaled image (analyzed image, left) for directly depicting the chemical components. This can be partly compensated by inspecting the segmented image while activated in ImageJ, allowing for dynamic identification of the various segments (presenting the 0–7 values in the results pane while hovering over the image with the pointer).
The two images in Figure 4 (bottom row) are comparable, and much of the chemical information can be obtained from both. However, the image analysis added semi-quantitative information, which allowed determining the predominating pool for P sorption. Quantification was improved by normalizing P, Al, and Ca to the fraction of the occupied area (i.e., total area minus the empty area). The association of P with Al was almost three times greater than its association with Ca (45.1 and 17.3%, respectively, Table 1), although Al cover was less than twice that of Ca (40.5 and 23.1%, respectively, Figure 4), indicating P highest affinity to Al (see further discussion in the following section). The processed image also strongly emphasized the ternary phase of P, Al, and Ca (black in Figure 4, bottom row, left) and the nature of its spatial distribution. Interestingly, the ternary phase was primarily found at Al and Ca bordering surfaces in the presence of P. This preliminary information can contribute to the study of mechanisms and processes involved in the formation of this mutual phase.
This image analysis working procedure can improve our understanding of the elements' distributions and associations; however, presenting the segmented image can be aided by being coupled to the SEM-EDS overlay map for rapid chemical identification. Thus, those two means of image presentation can be complementary.
Comparison Between Al-Based Water Treatment Residual, SL-Al/O-WTR, and WW-Al/O-WTR Based on Their Image Analyses
The Al-WTR, SL-Al/O-WTR, and WW-Al/O-WTR were each produced from three different solutions, with varying concentrations of P (the Sea of Galilee with 0.002–0.04 mg P L−1, (IOLR, 2016), directly affecting the original Al-WTR, SL (11.5 mg P L−1), and dairy WW solution (43 mg P L−1), respectively). Therefore, the materials differ in their P content. Comparing the segmented images of Al-WTR, SL-Al/O-WTR, and WW-Al/O-WTR can elucidate trends in P associations and distributions (Figure 5 and Table 3). The proportion of the empty area (i.e., area with a value of 0) increased in the order Al-WTR < SL-Al/O-WTR < WW-Al/O-WTR (27.9, 32.6, and 40.9%, respectively, Table 3A). Normalization of the elemental proportions is essential when comparing different samples because of the potential impact of sample morphology and local microtopography and the presence of other elements; thus, the proportions of P, Al, and Ca were normalized to the occupied area in each of the three Al-WTRs (Table 3B). Table 3A presents the original data for comparison, yet the discussion below refers to the normalized data.
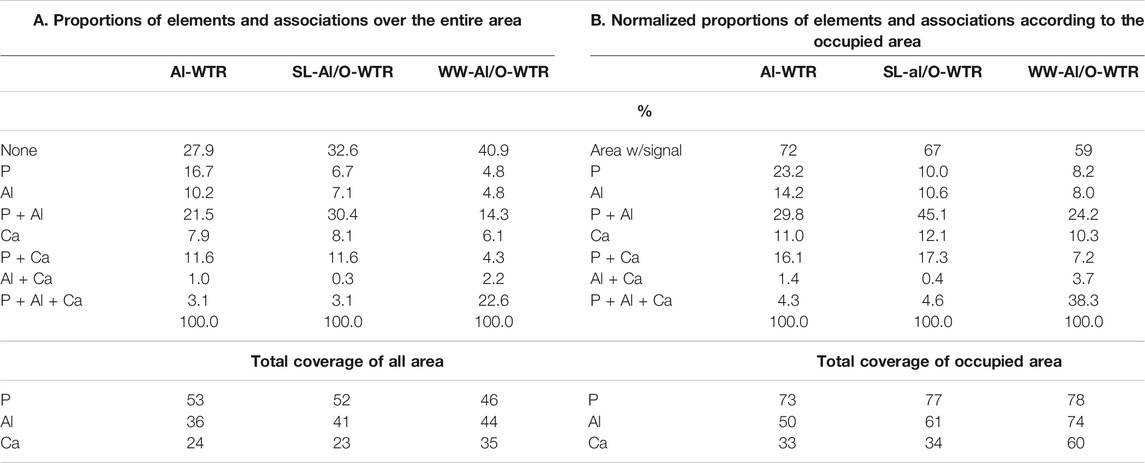
TABLE 3. Elements and their associations, A. the results of the image analysis, B. the results normalized to the total occupied area.
In terms of P associations, the Al pool appears to be more significant than the Ca pool, in all three Al-WTRs (45–24% and 17–7%, respectively, Table 3B), consistent with previous studies of Al-WTRs (Ippolito et al., 2003; Makris et al., 2004). Nevertheless, in contrast to previous studies, Ca was an essential pool for P, potentially affecting P sorption and recovery. Calcium in the Israeli Al-WTRs is attributed to the treated water: hard surface water of the Sea of Galilee (IOLR, 2016).
The proportion of standalone elements followed a decreasing trend with increasing P content of the materials, suggesting that excess P concentrations promoted clustering of P, Al, and Ca on the surface. Moreover, the segmented images of the three Al-WTRs reveal interesting information regarding the presence of the ternary phase of P, Al, and Ca. This phase may be stable where P, Al, and Ca are abundant (Allen and Hajek, 1989). The proportion of the P-Al-Ca phase was very similar for Al-WTR and SL-Al/O-WTR but increased substantially in the WW-Al/O-WTR sample (4.3, 4.6, and 38.3%, respectively, Table 3B). Since P content was the main variable factor in the chemical compositions of SL-Al/O-WTR and WW-Al/O-WTR following introducing Al-WTR with SL and WW, respectively, the formation of the ternary phase may have required exceptionally high P concentrations.
Furthermore, Table 2 indicates that P content on the surface of SL-Al/O-WTR was 0.93%, increasing from 0.56% following the introduction of SL to Al-WTR, while introducing the P-rich dairy WW to Al-WTR increased P surficial content to 2.70%. In fact, the increase in surficial P content with higher concentrations of introduced P exceeded the growth rate for the XRF-whole-sample P content. Zohar et al. (2017) suggested that exceeding concentrations of P promoted its preferential accumulation on top of the WW-Al/O-WTR particles rather than in inner pores. The current image analysis also suggests that this accumulation is mainly in the form of the P-Al-Ca phase. Additionally, in both Al-WTR and SL-Al/O-WTR, the P-Al-Ca phase appears at Ca surfaces' edges (probably small CaCO3 particles) when bordering Al and P surfaces. In contrast, in WW-Al/O-WTR, the ternary phase commonly appears in rather large collections, not limited to particle edges (Figure 5). Complexes with organic moieties in the WW (Zohar et al., 2017) probably contributed to enhanced solubility of Ca and Al; subsequently, the metals re-precipitated (similar to (Ler and Stanforth, 2003)) with the excessive concentrations of introduced P in WW, on top WW-Al/O-WTR surfaces.
Conclusion
The presented working procedure for image analysis of three-element dot maps consists of signal filtering, binary image transformation, segmentation to distinguished phases, and associations’ quantification/semi-quantification. Indeed, the primary outcome is quantifying the spatial cover of individual elements and their associations, overcoming an otherwise vague visual impression. Nevertheless, the final segmented image may elucidate and emphasize chemical and physical features, like elemental spatial distribution and porosity. Rapid identification of elements can benefit from comparing the segmented gray-scale image to SEM-EDS color overlay map. In case surface chemical behavior is of interest, the use of unpolished specimens is mandatory, as surface reactions are the initial sequestration step. The proposed image analysis procedure becomes helpful as it overcomes potential biases by microtopography (porosity) by using automated Brightness Thresholding. Yet, while elemental distribution (and thus, chemical affinity) is emphasized, the processed image does not reflect elemental concentration. The working procedure was demonstrated for dot maps produced by SEM-EDS, yet it can be applied to dot maps produced by any solid-state spectroscopic technique. Moreover, the working procedure was set up to allow modifications to analyze dot maps of more than three elements.
Application of the image analysis working procedure to the dot maps of Al-WTR, SL-Al/O-WTR, and WW-Al/O-WTR yielded semi-quantitative data indicating that P had a higher affinity to Al than to Ca in the three materials. In SL-Al/O-WTR, P spatial distribution was more extensive than Al and Ca, although P content was much lower, suggesting scattered sorption on both weak and strong binding sites upon SL mixture with Al-WTR. On the other hand, with increasing P content in the WW solution, the elements were more clustered in WW-Al/O-WTR. P-Al-Ca’s ternary association was recognized to play an important role in WW-Al/O-WTR, as its areal cover grew substantially from ca. 4.5% in Al-WTR and SL-Al/O-WTR (limited to bordering Ca and Al surfaces) to 38% cover in the P-enriched WW-Al/O-WTR. Knowing P chemical affinity and occurrence can contribute to the formulation of P recovery and recycling strategies.
Data Availability Statement
The original contributions presented in the study are included in the article/Supplementary Material, further inquiries can be directed to the corresponding author.
Author Contribution
Conceptualization: IZ and PH; methodology: PH; SEM-EDS images production, data analysis and writing: IZ.
Funding
This research was supported by Research Grant No. IS-4870-15 R from BARD, the United States-Israel Binational Agricultural Research and Development Fund and by a research grant, No. 596-0550-14 from the Israel Department of Agriculture.
Conflict of Interest
The authors declare that the research was conducted in the absence of any commercial or financial relationships that could be construed as a potential conflict of interest.
Acknowledgments
We are grateful to the Petro-physical Laboratory in the Marine Geosciences Department, at the University of Haifa, Israel, for using the SEM and highly appreciate all assistance and consultancy given by Nimer Taha and Or Bialik.
Supplementary Material
The Supplementary Material for this article can be found online at: https://www.frontiersin.org/articles/10.3389/fenvc.2021.719300/full#supplementary-material
Abbreviations
Al-WTR, aluminum-based water treatment residuals; SL-Al/O-WTR, soil leach-reacted aluminum/organic water treatment residuals; WW-Al/O-WTR, wastewater-reacted aluminum/organic water treatment residuals; XRF, x-ray fluorescence; SEM-EDS, scanning Electron Microscopy-Energy Dispersive Spectroscopy; SL, soil leach; WW, wastewater.
References
Allen, B. L., and Hajek, B. F. (1989). Mineral Occurrence in Soil Environments. Miner. Soil Environ. 1, 199–278.
Avnimelech, Y. (1980). Calcium-Carbonate-Phosphate Surface Complex in Calcareous Systems. Nature 288, 255–257. doi:10.1038/288255a0
Bauer, B., Schwarz, H., and Thanh, N. (1983). Digital Image Processing of Combined SEM and EDX Signals. J. Microsc. 130, 325–330. doi:10.1111/j.1365-2818.1983.tb04552.x
Cardell, C., Yebra, A., and Van Grieken, R. E. (2002). Applying Digital Image Processing to SEM-EDX and BSE Images to Determine and Quantify Porosity and Salts With Depth in Porous Media. Microchim. Acta 140, 9–14. doi:10.1007/s006040200063
Dayton, E. A., and Basta, N. T. (2005). A Method for Determining the Phosphorus Sorption Capacity and Amorphous Aluminum of Aluminum-Based Drinking Water Treatment Residuals. J. Environ. Qual. 34, 1112–1118. doi:10.2134/jeq2004.0230
El-Gohary, M. A. (2016). A Holistic Approach to the Assessment of the Groundwater Destructive Effects on Stone Decay in Edfu temple Using AAS, SEM-EDX and XRD. Environ. Earth Sci. 75, 1–11. doi:10.1007/s12665-015-4849-x
Friel, J. J. (2003). X-ray and Image Analysis in Elcetron Microscopy. Princeton, NJ: Princeton Gamma-Tech, Inc.
Fugère, V., Lostchuck, E., and Chapman, L. J. (2020). Litter Decomposition in Afrotropical Streams: Effects of Land Use, Home-field Advantage, and Terrestrial Herbivory. Freshw. Sci. 39, 497–507. doi:10.1086/709807
Iassonov, P., Gebrenegus, T., and Tuller, M. (2009). Segmentation of X-ray Computed Tomography Images of Porous Materials: A Crucial Step for Characterization and Quantitative Analysis of Pore Structures. Water Resour. Res. 45, 1–12. doi:10.1029/2009WR008087
IOLR (2016). Israel Oceanographic and Limnological Research LTD. Lake Kinneret Water Quality Monitoring Center [WWW Document]. URL http://kinneret.ocean.org.il/text/ann_report/Ann_report_2015.pdf.
Ippolito, J. A., Barbarick, K. A., Heil, D. M., Chandler, J. P., and Redente, E. F. (2003). Phosphorus Retention Mechanisms of a Water Treatment Residual. J. Environ. Qual. 32, 1857–1864. doi:10.2134/jeq2003.1857
Kahn, H., Mano, E. S. M., Tassinari, M. M. M. L., and Tassinari, M. L. (2002). Image Analaysis Coupled with A SEM-EDS Applied to the Characaterization of A Zn-Pb Partially Weathered Ore. Jmmce 01, 1–9. doi:10.4236/jmmce.2002.11001
Khare, N., Hesterberg, D., and Martin, J. D. (2005). XANES Investigation of Phosphate Sorption in Single and Binary Systems of Iron and Aluminum Oxide Minerals. Environ. Sci. Technol. 39, 2152–2160. doi:10.1021/es049237b
Ler, A., and Stanforth, R. (2003). Evidence for Surface Precipitation of Phosphate on Goethite. Environ. Sci. Technol. 37, 2694–2700. doi:10.1021/es020773i
Makris, K. C., Harris, W. G., O'Conno, G. A., and Obreza, T. A. (2004). Phosphorus Immobilization in Micropores of Drinking-Water Treatment Residuals: Implications for Long-Term Stability. Environ. Sci. Technol. 38, 6590–6596. doi:10.1021/es049161j
Massey, M. S., Zohar, I., Ippolito, J. A., and Litaor, M. I. (2018). Phosphorus Sorption to Aluminum‐based Water Treatment Residuals Reacted with Dairy Wastewater: 2. X‐Ray Absorption Spectroscopy. J. Environ. Qual. 47, 546–553. doi:10.2134/jeq2017.10.0407
Palma, A., Di, Capozzi, F., Spagnuolo, V., Giordano, S., and Adamo, P. (2019). Atmospheric Particulate Matter Intercepted by moss-bags: Relations to moss Trace Element Uptake and Land Use. Chemosphere 176, 361–368. doi:10.1016/j.chemosphere.2017.02.120
Ridler, T. W., and Calvard, S. (1978). Picture Thresholding Using an Iterative Slection Method. IEEE Trans. Syst. Man. Cybern. 8, 630–632. doi:10.1109/TSMC.1978.4310039
Sims, J. T., and Sharply, A. N. (2005). Phosphorus: Agriculture and the Environment. Madison, USA: Agronomy monograph 46.
Sø, H. U., Postma, D., Jakobsen, R., and Larsen, F. (2011). Sorption of Phosphate Onto Calcite; Results From Batch Experiments and Surface Complexation Modeling. Geochim. Cosmochim. Acta 75, 2911–2923. doi:10.1016/j.gca.2011.02.031
Stauffer, W., Sheng, H., and Lim, H. N. (2018). EzColocalization: An ImageJ Plugin for Visualizing and Measuring Colocalization in Cells and Organisms. Sci. Rep. 8, 1–13. doi:10.1038/s41598-018-33592-8
Suarez-arnedo, A., Torres Figueroa, F., Clavijo, C., Arbeláez, P., Cruz, J. C., Muñoz-Camargo, C., et al. (2020). An Image J Plugin for the High Throughput Image Analysis of In Vitro Scratch Wound Healing Assays. PLoS One 15, e0232565–14. doi:10.1371/journal.pone.0232565
Vázquez, M. A., Galán, E., Ortiz, P., and Ortiz, R. (2013). Digital Image Analysis and EDX SEM as Combined Techniques to Evaluate Salt Damp on Walls. Constr. Build. Mater. 45, 95–105. doi:10.1016/j.conbuildmat.2013.03.067
Valente, A. J., Maddalena, L. A., Robb, E. L., Moradi, F., and Stuart, J. A. (2017). A Simple ImageJ Macro Tool for Analyzing Mitochondrial Network Morphology in Mammalian Cell Culture. Acta Histochem. 119, 315–326. doi:10.1016/j.acthis.2017.03.001
Wendling, L. A., Blomberg, P., Sarlin, T., Priha, O., and Arnold, M. (2013). Phosphorus Sorption and Recovery Using mineral-based Materials: Sorption Mechanisms and Potential Phytoavailability. Appl. Geochem. 37, 157–169. doi:10.1016/j.apgeochem.2013.07.016
Zohar, I., Ippolito, J. A., Massey, M. S., and Litaor, I. M. (2017). Innovative Approach for Recycling Phosphorous from Agro-Wastewaters Using Water Treatment Residuals (WTR). Chemosphere 168, 234–243. doi:10.1016/j.chemosphere.2016.10.041
Zohar, I., Massey, M. S., Ippolito, J. A., and Litaor, M. I. (2018). Phosphorus Sorption Characteristics in Aluminum-Based Water Treatment Residuals Reacted with Dairy Wastewater: 1. Isotherms, XRD, and SEM-EDS Analysis. J. Environ. Qual. 47, 538–545. doi:10.2134/jeq2017.10.0405
Keywords: image analysis, element dot maps, scanning electron microscopy-energy dispersive spectroscopy, phosphorus sorption, water treatment residuals
Citation: Zohar I and Haruzi P (2021) Image Analysis for Spectroscopic Elemental Dot Maps: P, Al, and Ca Associations in Water Treatment Residuals as a Case Study. Front. Environ. Chem. 2:719300. doi: 10.3389/fenvc.2021.719300
Received: 02 June 2021; Accepted: 08 July 2021;
Published: 19 July 2021.
Edited by:
Lingxin Chen, Yantai Institute of Coastal Zone Research, Chinese Academy of Sciences (CAS), ChinaReviewed by:
Dawei Pan, Yantai Institute of Coastal Zone Research, Chinese Academy of Sciences (CAS), ChinaGuoqing Wang, Ocean University of China, China
Feng Tan, Dalian University of Technology, China
Copyright © 2021 Zohar and Haruzi. This is an open-access article distributed under the terms of the Creative Commons Attribution License (CC BY). The use, distribution or reproduction in other forums is permitted, provided the original author(s) and the copyright owner(s) are credited and that the original publication in this journal is cited, in accordance with accepted academic practice. No use, distribution or reproduction is permitted which does not comply with these terms.
*Correspondence: Iris Zohar, irisz2910@gmail.com