- 1Department of Biological Sciences, Research Center for Precision Medicine, Xi'an Jiaotong-Liverpool University, Suzhou, China
- 2Institute of Integrative Biology, University of Liverpool, Liverpool, United Kingdom
- 3Institute of & Chronic Disease, University of Liverpool, Liverpool, United Kingdom
- 4Key Laboratory of Information Fusion Technology of Ministry of Education, School of Automation, Northwestern Polytechnical University, Xi'an, China
- 5Department of Epidemiology and Biostatistics, University of Texas Health San Antonio, San Antonio, TX, United States
- 6Department of Electrical and Computer Engineering, University of Texas at San Antonio, San Antonio, TX, United States
Recent studies have revealed that the RNA N6-methyladenosine (m6A) modification plays a critical role in a variety of biological processes and associated with multiple diseases including cancers. Till this day, transcriptome-wide m6A RNA methylation sites have been identified by high-throughput sequencing technique combined with computational methods, and the information is publicly available in a few bioinformatics databases; however, the association between individual m6A sites and various diseases are still largely unknown. There are yet computational approaches developed for investigating potential association between individual m6A sites and diseases, which represents a major challenge in the epitranscriptome analysis. Thus, to infer the disease-related m6A sites, we implemented a novel multi-layer heterogeneous network-based approach, which incorporates the associations among diseases, genes and m6A RNA methylation sites from gene expression, RNA methylation and disease similarities data with the Random Walk with Restart (RWR) algorithm. To evaluate the performance of the proposed approach, a ten-fold cross validation is performed, in which our approach achieved a reasonable good performance (overall AUC: 0.827, average AUC 0.867), higher than a hypergeometric test-based approach (overall AUC: 0.7333 and average AUC: 0.723) and a random predictor (overall AUC: 0.550 and average AUC: 0.486). Additionally, we show that a number of predicted cancer-associated m6A sites are supported by existing literatures, suggesting that the proposed approach can effectively uncover the underlying epitranscriptome circuits of disease mechanisms. An online database DRUM, which stands for disease-associated ribonucleic acid methylation, was built to support the query of disease-associated RNA m6A methylation sites, and is freely available at: www.xjtlu.edu.cn/biologicalsciences/drum.
Introduction
Epigenetic regulation, such as, RNA methylation, DNA methylation and post-translational modification (PTM), participates in a variety of important cellular processes, including embryonic development, maintenance of chromosome stability and X-chromosome inactivation (Wu and Zhang, 2014). Over the past decade, DNA methylation has been considered to play a critical key role in gene expression regulation to moderate various biological functions. It has been found that dysregulated DNA methylation is associated with various diseases. For example, epigenetic defects, like the global genomic hypo-methylation or locus-specific hyper-methylation is one of the cancer hallmarks (Gopalakrishnan et al., 2008). To date, there have been a number of works seeking to unveil the functional relevance of epigenetic modifications to various diseases. DiseaseMeth (Xiong et al., 2016) contains aberrant DNA methylation in 679602 disease-gene association collected from 32701 samples; MethyCancer (He et al., 2007) and MethHC (Huang et al., 2014) supports the query of cancer and disease related DNA methylation profiles. ActiveDriverDB (Huang et al., 2014), CaspNeuroD (Kumar and Cieplak, 2016), dbPTM (Huang et al., 2019) and PTMSNP (Kim Y. et al., 2015) investigated human disease mutations that potentially functional through post-translational modifications. Recently, Xu and Wang investigated the disease-associated phosphorylation sites of protein from a multi-layer heterogeneous network using the random walk algorithm (Xu and Wang, 2016). These studies greatly advanced our understanding of the role epigenetic modifications play in disease pathology. However, the study of biochemical modifications have been dominated by DNA methylation and post-translation protein modifications, until recently, RNA methylation emerged as important layer for gene expression regulation.
Firstly identified more 40 years ago (Wei et al., 1976), more than 100 different types of RNA modifications have also been discovered in cell as epigenetic mark recognized by other regulators for modulating the genetic information (Cantara et al., 2011; Boccaletto et al., 2017), among which, N6-methyladenosine is the most abundant in mRNA (Fu et al., 2014; Meyer and Jaffrey, 2014). A series of studies reveal that, RNA methylation plays a crucial role in the regulation of circadian clock (Fustin et al., 2013), RNA stability (Wang et al., 2014), cell differentiation (Geula et al., 2015), translation efficiency (Wang et al., 2015), as well as DNA damage response (Xiang et al., 2017) and cortical neurogenesis (Yoon et al., 2017). It has been shown that RNA methylation may be central in disease pathology especially in various cancers, including breast cancer (Cai et al., 2018), myeloid leukemia (Barbieri et al., 2017; Kwok et al., 2017; Li Z. et al., 2017; Vu et al., 2017), liver cancer (Chen M. et al., 2017), carcinoma (Li et al., 2017a), glioma (Visvanathan et al., 2017; Zhang et al., 2017), etc. (Hsu et al., 2017; Stojković and Fujimori, 2017; Wang S. et al., 2017). Recent studies revealed the impacts of m6A modification on specific diseases. E.g., N6-methyladenosine (m6A) modification of mRNA plays a role in regulating the self-renewal and tumorigenesis of glioblastoma stem cell (GSC). Studies report the knockdown of RNA methyltransferase complex METTL3 or METTL14 can dramatically decrease abundance of m6A methylation and alter mRNA expression of genes (e.g., ADAM19, EPHA3, KLF4), thereby promoting human GSC growth (Cui et al., 2017). Meanwhile, the up-regulation of RNA m6A demethylase ALKBH5 can also induce the proliferation of GSCs (Zhang et al., 2017). It is found that FOXM1, the cell cycle regulator, is the downstream target of m6A modification through inhibition of ALKBH5 by shRNA. Importantly, the hypo-methylation of target mRNA promotes the binding of RNA binding protein HuR, resulting in increased FOXM1 expression and the development of glioma (Zhang et al., 2017). Additionally, the RNA m6A demethylase FTO is found to be an oncogene of the Acute Myeloid Leukemia (Li Z. et al., 2017). studies show that reduced m6A levels in some mRNA transcripts, such as ASB2 and RARA, can enhance leukemic oncogene-mediated cell transformation, leukemogenesis, and inhibit AML cell differentiation (Li Z. et al., 2017). Furthermore, Zhang et al. found that the breast cancer cells stimulated by hypoxia can cause upregulation of m6A demethylase ALKBH5 expression, which is mediated by hypoxic induction factor (HIF). Consequently, it results in the demethylation of the multipotent factor NANOG's mRNA, and hypomethylation increases the stability of mRNA so as to causes high expression of NANOG, further inducing the maintenance and metastasis of tumor stem cells (Zhang et al., 2016a).
Despite the growing interests in m6A RNA modification and its potential regulatory role in various diseases, the study of m6A methylation under the context of diseases has been restricted. The experimental approaches are mostly limited to the study of m6A mediator genes, i.e., the RNA methyltransferase (writer), demethylase (eraser) and RNA binding protein (reader). For instance, the RNA m6A demethylase FTO is also found to play an important role in neurogenesis, as well as in learning and memory. Hence, m6A modification is regarded to be related to Alzheimer's disease (Li L. et al., 2017). And, another study reports RNA m6A demethylase ALKBH5 can relate to the major depressive disorder in Chinese Han population (Du et al., 2015). These studies are often less detailed in genomic resolution and could not unveil the disease relevance of a specific RNA methylation site. Comparing with the research dedicated to the experimental investigation of m6A site regulatory functions, bioinformatics is a possible method to identify the putative disease association of the m6A sites, thereby urgently needed at present. Till this day, the computational approaches for studying the association between m6A methylation and diseases have been limited to the disease-associated mutations that may potentially disrupt or form an m6A-containing motif, which may be regulated through epitranscriptome layer. Works of this category include m6AVar (Zheng et al., 2018), which contains a number of functional variants involved in m6A modification, and m6ASNP (Jiang et al., 2018; Mo et al., 2018; Zhang et al., 2019), which is a tool for annotating genetic variants from the perspective of impact on m6A modification. Although generated fruitful results (Mo et al., 2018,a,b), SNP-based approaches are limited to existing GWAS analysis results and cannot predict previously unknown novel associations between m6A sites and diseases. Other disease association study of the epitranscriptome focuses on a specific mediator gene of the epitranscriptome, which could cover the disease association of the epitranscriptome for only a limited number of diseases (Zhang et al., 2016b, 2019), but not yet an arbitrary disease.
The accumulation of epitranscriptome high-throughput sequencing data has provided numerous possibilities for epitranscriptome analysis. Nowadays, the most widely used approach for profiling transcriptome-wide RNA methylation is methylated RNA immunoprecipitation sequencing (m6A-seq or MeRIP-seq) (Wan et al., 2015), and the technique has been used in various studies to profile the condition-specific RNA methylation (Liu H. et al., 2018; Xuan et al., 2018). The m6A RNA methylation sites has been more accurately identified in human, mouse and other species with the machine learning approaches. It is possible and solely needed to develop computational approaches for understanding the disease relevance of individual RNA methylation sites by taking advantage of the large amount of epitranscriptome data accumulated from existing studies (Chen X. et al., 2017; Chen et al., 2019). Random walk on a multi-layer network has been used previously to uncover the important role of RNA molecules under a pathologic context, including disease-related long non-coding RNAs (lncRNA) (Zhou et al., 2015) and miRNAs (Mendell and Olson, 2012). In the field of epitranscriptome analysis, random walk with start (RWR) algorithm has been implemented to study the functional protein-protein network driven by RNA methylation enzymes through the regulation of epitranscriptome layer (Zhang et al., 2016b).
In this work, we for the first time extracted disease-associated m6A sites through a multi-layer heterogeneous network using random walk with restart (RWR) algorithm, and provided with a more specific regulatory circuit that functions at epitranscriptome layer. Specifically, a novel multi-layer heterogeneous network was constructed from gene expression and RNA methylation data. The nodes of the network are corresponding to the diseases, the genes and the m6A RNA methylation sites. The network contains both cross-layer associations, such as gene-m6A site association, disease-gene association, as well as the with-layer associations, i.e., gene-gene association, m6A site-m6A site association and disease-disease association. Depending on the known gene-disease network and gene-m6A site network that link the m6A site and disease layers together, the potential relationships of the m6A sites and diseases are both implicated (Tong et al., 2008). The within-layer association networks (e.g., disease-disease association) can further enhance the confidence of interactions.
To evaluate the performance of the proposed approach, a 10-fold cross-validation was implemented. Our RWR-based predictor achieved a reliable prediction performance and the area under the receiver operating characteristic curve (AUC) is equal to 0.83, compared with an alternative hypergeometric test-based approach (AUC: 0.73) and a random predictor (AUC: 0.48). A website DRUM, which stands for disease-related ribo-nucleic acid methylation, is built to support the query of the RNA methylation sites most probable related to 705 diseases. The DRUM website is freely available at: www.xjtlu.edu.cn/biologicalsciences/drum.
Materials and Methods
To infer disease-associated RNA methylation site, a multi-layer heterogeneous network was constructed, which consists of three types of nodes, i.e., the diseases, genes and m6A sites, and five types of associations, i.e., gene-gene association, gene-disease association, gene-m6A site association, disease-disease association, and m6A site- m6A site association (see Figure 1). The network was constructed by integrating the RNA methylation profiles, the RNA expression profiles and gene-disease associations, which will be detailed in the next.
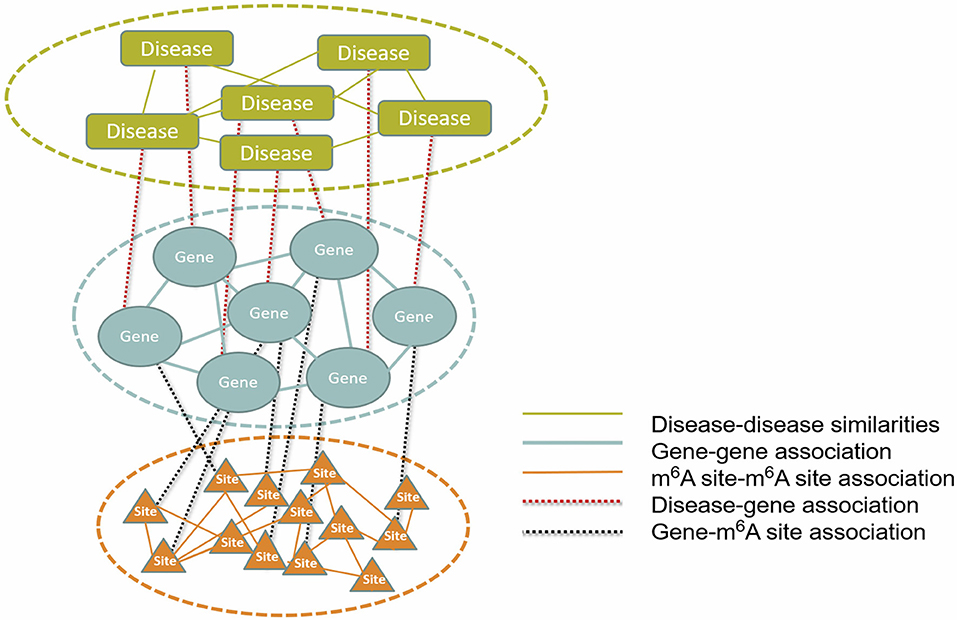
Figure 1. The constructed multi-layer heterogeneous network. To infer disease-m6A site association, a multi-layer heterogeneous network was constructed, which consists of three types of nodes, i.e., the disease, gene and m6A site, and five types of associations, i.e., gene-gene, gene-disease, gene-m6A site, disease-disease, and m6A site-m6A site.
RNA Methylation Data
The locus information of 477,452 m6A RNA methylation sites in human was extracted from RMBase V2 (Xuan et al., 2018), which collected the m6A RNA methylation sites reported by multiple techniques including m6A-seq, miCLIP, m6A-CLIP, and PA-m6A-seq (Li et al., 2017b). In the site filtering stage, 182,358 sites, which are supported by more than 10 experiments, are kept. To further select the most robust m6A methylation signal, we selected the methylation sites with average methylation level within the 70 percentile. Additionally, the m6A sites with the variance of methylation level ranked in the top 80 percentiles were retained, which represent the most actively regulated set of m6A sites, whose functional relevance may be more reliably inferred. In the end, 28278 RNA methylation sites were retained for further analysis.
Although there exists base-resolution m6A profiling techniques, technique either cannot be used for methylation level quantification (e.g., miCLIP and m6A-CLIP), or the limited number of available samples is insufficient to infer reliably the associations (e.g., PA-m6A-seq). Instead of using data generated from base-resolution techniques, the RNA methylation levels of each m6A sites were estimated from MeRIP-seq data, which profiled the m6A epitranscriptome under 38 different experimental conditions (see Table 1). The raw data was downloaded from GEO and aligned to human reference genome hg19 with HISAT2 (Kim D. et al., 2015). The reads associated with each RNA methylation sites were counted under R enrironment, and the methylation status were quantified using the M-value, which is essentially the log2 fold change of reads in the IP sample compared to the input control sample of MeRIP-seq data, as is shown in (1):
where, RPKMIP and RPKMInput represent the reads abundance of a specific m6A site (101 bp flanked region) in the IP and Input control sample of MeRIP-seq data, respectively. The reads abundance was measured in terms of the Reads Per Kilobase of transcript per Million mapped reads (RPKM). When multiple biological replicates from the same experimental conditions were available, they were merged during the data processing stage. Quantile normalization was then performed to remove potential batch effect.
Gene Expression Data
The gene expression profiles under the same 38 experimental conditions, (matched with the RNA methylation data) were extracted from the input control samples of the MeRIP-seq data, which measures the expression level of genes. Similar to the processing of RNA methylation data, the gene expression levels were measured in RPKM, multiple biological replicates were merged, and the quantile normalization was performed to reduce batch effect.
Disease-Gene Association
The human gene-disease associations used in our analysis were directly collected from OUGene, which collects the over- and under-expressed genes under a specific disease condition (Pan and Shen, 2016). A total of 41,269 associations between 705 human diseases and 1080 genes from OUGene were integrated into our multi-layer heterogeneous network.
Disease-Disease Similarities
Since similar diseases are often associated with similar gene sets, the association between diseases was also considered (Xu and Wang, 2016). The disease-disease similarity network was constructed based on MeSH (medical subject headings vocabulary) terms (Lowe and Barnett, 1994), and the diseases share significant number of MeSH terms are considered more associated. Specifically, the similarity of two diseases Vij is denoted by the number of shared MeSH terms panelized by the total number of terms in their disease titles, as shown in the following
where, di and dj strand for all the MeSH terms of the disease i and j , respectively. And |*| denotes the total number of terms. Please note that the OUGENE database does not contain the MeSH terms information. The MeSH terms associated with various diseases was extracted from the semantically integrated database of disease SIDD (Liang et al., 2013). No additional cut-off threshold was further applied. All the pair-wise associations between diseases were kept for the analysis.
Association Between m6A Sites
The association between m6A RNA methylation sites was inferred from RNA methylation profiles. We speculate that the functions of two m6A sites are related if their methylation profiles are highly correlated across different experimental conditions. Fisher's asymptotic test was implemented to calculate the Pearson correlation coefficient (Pcc) P-Values for each m6A site pairs, and then Bonferroni multiple test correction was used for adjusting the P-Values. Only the m6A site pairs with the adjusted P < 0.05 cut-off and the homologous Pcc value ranked in the top or bottom 10 percentile were considered as associated in our network (Liao et al., 2011). Positive and negative correlations were not distinguished in the association network, which is because that the regulatory impact of m6A RNA methylation is complex. It may both enhances or decreases transcriptional expression level for different genes, making it difficult to distinguish the functional consequences of positive or negative correlation at epitranscriptome layer.
Gene-Gene Association
We constructed the gene-gene association networks from RNA expression data. The genes that exhibit strong positive or negative correlation are considered functionally related in our multi-layer heterogeneous network. And it followed the same procedure of building the associations between m6A RNA methylation sites.
Association Between m6A Sites and Genes
Similar to gene-association or m6A site-m6A site association, the association between m6A sites and genes was constructed from the correlation of their expression and methylation levels. If the methylation level of an m6A site and the expression level of a gene are highly correlated across different experimental conditions, we assume that the two are functionally related. The construction of gene-m6A site network follows the same procedure of m6A site-m6A site network.
The Multi-Layered Heterogeneous Network
As shown in Figure 1, the multi-layer heterogeneous network incorporates three types of nodes and five types of associations, from which, it is possible to infer disease-associated m6A RNA methylation sites. We use D{d1, d2, ⋯ , dN},S{s1, s2, ⋯sM} and G{g1, g2, ⋯ , gT} successively to represent three types of nodes within network: the diseases, the m6A sites and the genes. And N , M and T denote the total number of diseases, m6A sites and genes, respectively. The associations within the disease, the gene and the site layer can then be represented by DD{dij:i, j = 1, 2, ⋯ , N}, GG{gi, j:i, j = 1, 2, ⋯ , T}and SS{sij:i, j = 1, 2, ⋯ , M}, respectively. While the other two types of connection between different types of nodes are represented by DG{dg:i = 1, 2, ⋯ , N; j = 1, 2, ⋯ , T} and SG {sgij:i = 1, 2, ⋯ , M; j = 1, 2, ⋯ , T}. Please note that the missing information of m6A site-disease association is substituted by DS { dsij:i = 1, 2, ⋯ , M; j = 1, 2, ⋯ , N}, which is a null network and used to complement the integrity of the adjacency matrix of the multi-layer heterogeneous network.
Construct the Adjacency Matrix of the Overall Network
In RWR algorithm, the multi-layer heterogeneous network is represented by the W matrix. It is a column-normalized adjacency matrix and comprises of nine sub matrixes, which respectively reflects diverse relationships among the nodes (i.e., disease, gene, and m6A site). Among them, MDS, MSG,and MDG strands for the probabilities of nodes transmitting between different type of nodes, and their transpose matrixes are denoted by MSD,MGS, and MGD, respectively. While MDD, MSS and MGG represent the transition probabilities among the same type of nodes. MGS,MGD,MDD, MSS, and MGG were estimated previously; while MSD is set to be 0, as it is unknown. Due to the different weights used in various types of networks, the adjacency matrix were further normalized with
where, all the 5 sub networks were assigned with the equal weight, despite that their relative importance may be further optimized (Xu and Wang, 2016).
Random Walk With Restart (RWR) Algorithm
Random walk with start (RWR) algorithm, as an iterative network propagation method, was used for inference of disease-associated RNA methylation site on our multi-layer heterogeneous network. RWR algorithm is defined that a random walker starts from a specific node and iteratively transmits to its neighbor nodes. The pump flow of random workers is proportional to the weights of edge, and it is synchronously recycled to the initial position with the certain proportion. Compared to the conventional random walk approach, RWR algorithm allows the return of the random walkers, so that it can avoid all random walkers assembling at a single node location. When applied to multi-layer heterogeneous networks, another notable strength of RWR is that it does not restrict movement of the random walker among nodes of the same type, and allows walking among all the three layers of the network via the five types of edges. In the end, when the terminated condition is satisfied, all the reachable positions can obtain a steady-state probability, and the nodes are ranked according to the proportion that random walker reaches. Here, we assume the Ps is the stopping probability of random walker at each position after the s-th iteration, which can be calculated as following:
where, r is the restart probability, indicating the proportion of random walkers being recycled at step, and is set to 0.75 arbitrarily. And P0 refers to the initial probability vector of seed node and W is a matrix that consists of transition probabilities of movement through different types nodes (discussed in the next). Here, the stopping criterion for iteration is the difference of probabilities between the (S+1)-th iteration and its prior iteration falls below a predefined threshold 10−10. We can have the disease node di as the seed node with initial probability 1, while the remaining disease nodes are assigned with an initial probability of 0. With the implementation of RWR algorithm, we can rank the disease-associated m6A sites according to the stable probability that the random walker di reaches each m6A site node.
The overall RWR algorithm is summarized in the following (Figure 2).
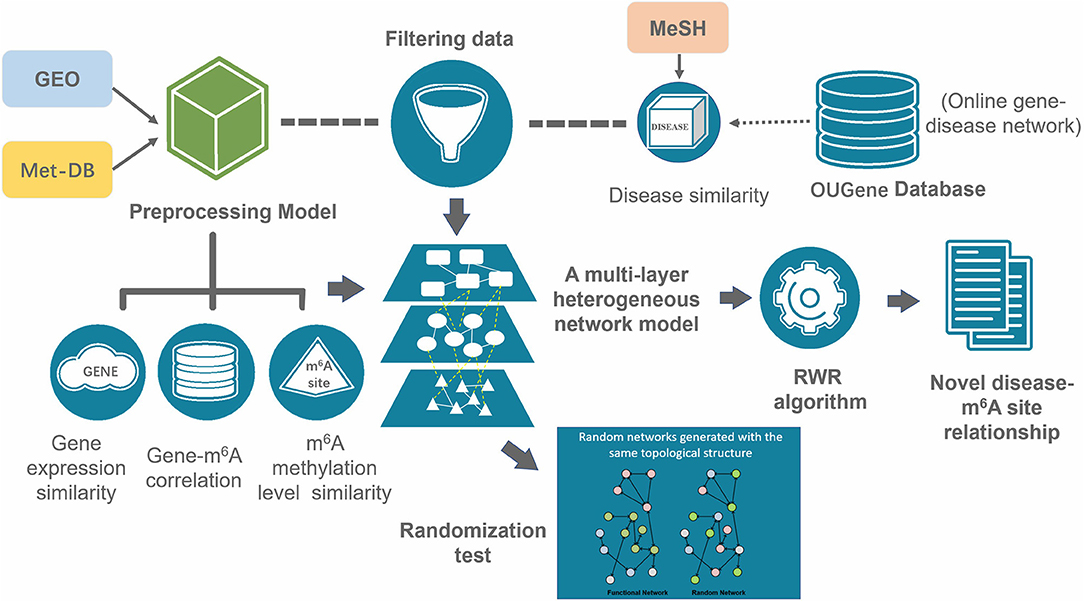
Figure 2. Overall workflow of the prediction. A multi-layer consists of three types of nodes (disease, gene and m6A site) was constructed from gene expression data, RNA methylation data, disease-gene association data and disease similarities. The disease-associated m6A RNA methylation sites were inferred with the RWR algorithm.
Evaluate the Statistical Significance of Prediction by Random Permutation
In general, of interests are the nodes with highest probabilities in RWR result, as they are regarded as highly accessible from the initial node, and thus denotes the association. To evaluate the statistical significance of the prediction results, a randomization-based estimation (Jia and Zhao, 2014) is implemented. Specifically, we generated 100 random networks by building random edges within the multi-layer heterogeneous network but still maintaining its original topology characteristics (Liao et al., 2011). This randomization chose two arbitrary edges (e.g., a-b and c-d) and exchanged them (e.g., with a-d and c-b), if the new links generated not already exist in the network after the node exchange. Then, for each of 100 random networks, RWR algorithm is applied and ranks all the m6A sites according to the probabilities of association to the disease. These probabilities represent the observed probabilities of a negative association between a disease and an m6A site, with which the statistical significance of a prediction from the real network can be assessed (Jia and Zhao, 2014).
Determine the Direction of the Predicted Association
Given an m6A site is predicted to be associated with a disease, we would like to know whether we should expect a hyper or hypo-methylation of this site under disease condition. Conceivably, if the methylation level of this site is positively correlated to the genes that are overexpressed under disease condition, or anti-correlated to genes that are under expressed under disease condition, the site is likely to be hyper-methylated under disease condition; and vice versa. The median of the correlations of this site to all the disease-associated genes was used to infer the direction of the association, and has been provided at our website.
An Alternative Approach for Performance Comparison
To evaluate the performance of this approach, we also considered a naïve hypergeometric test-based approach, which assesses the association between a disease and an m6A sites by checking whether they are simultaneously linked to a significant number of genes in the constructed multi-layer heterogeneous network (see Figure 3). The statistical significance (P-Value) of the association can be assessed with a hypergeometric test, with
where, m denotes the total number of genes in the analysis. n denotes the number of genes linked to a specific disease in the gene-disease association sub network, x denotes the number of genes linked to a specific m6A site in the gene-m6A site sub network; and y denotes the number of genes associated with both the disease and the m6A site. With the P-Values, it is then possible to predict the disease-associated RNA methylation sites given a specific significance level. Please note that the above alternative approach takes advantage of only two out of the five types of associations: the gene-m6A site associations and disease-gene associations.
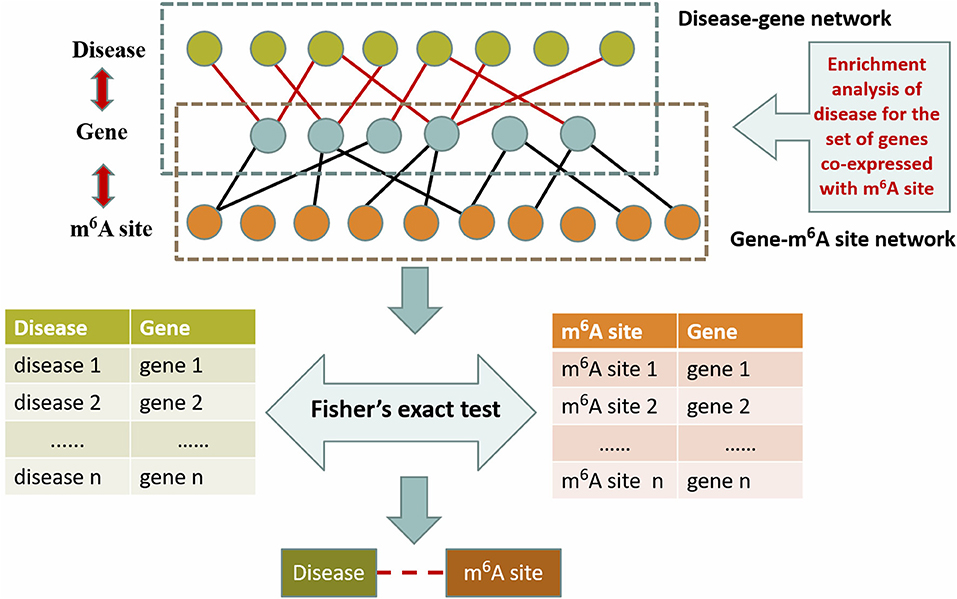
Figure 3. Hypergeometric test-based approach. This method is based on the disease-gene association from OUGene database and the gene-m6A site association networks derived from the gene expression profiles and RNA methylation profiles. The statistical significance is assessed with the hypergeometric test.
Result
Constructed Multi-Layer Heterogeneous Network
Utilizing the aforementioned approaches, a multi-layer heterogeneous network was constructed to incorporate three types of nodes (m6A site, gene, and disease) and five types of associations. The numbers of nodes and edges in each layer of the network were summarized in Table 2.
Performance Evaluation
We employed the 10-fold cross-validation to evaluate the performance of the proposed RWR algorithm. During each iteration, 10% of disease-gene associations were deleted from the original multi-layer heterogeneous network and reserved as the testing data, while the remaining 90% of associations were used as training dataset.
The proposed approach was also compared to a random predictor, which is constructed by random permutation of the multi-layer heterogeneous network, and an alternative hypergeometric test-based approach.
To compare the performances of the different methods, the receiver operating characteristics (ROC) curve was implemented to illustrate the true positive rate (TPR) vs. the false positive rate (FPR) at different stringency cut-offs, and the performance of different methods can be measured by the area under the ROC curve (AUC).
As is shown in Figure 4, the RWR method achieved an AUC of 0.827, outperformed the hypergeometric test-based approach (AUC: 0.733) and the random predictor (AUC: 0.550), which is close to the theoretical random performance (Figure 4A). Additionally, we also calculated the AUCs of each individual disease. As is shown in Figure 4B, RWR algorithm achieved superior performance on most of the diseases (average/median AUC: 0.867/0.913), compared to the other two methods: Hypergeometric test-based approach (average/median AUC: 0.723/0.772) and random predictor (average/median AUC: 0.486/0.479). This suggested that the multi-layer network model coupled with RWR algorithm could effectively predict the disease-m6A site associations, or potentially unveil the disease circuits regulated at epitranscriptome layer.
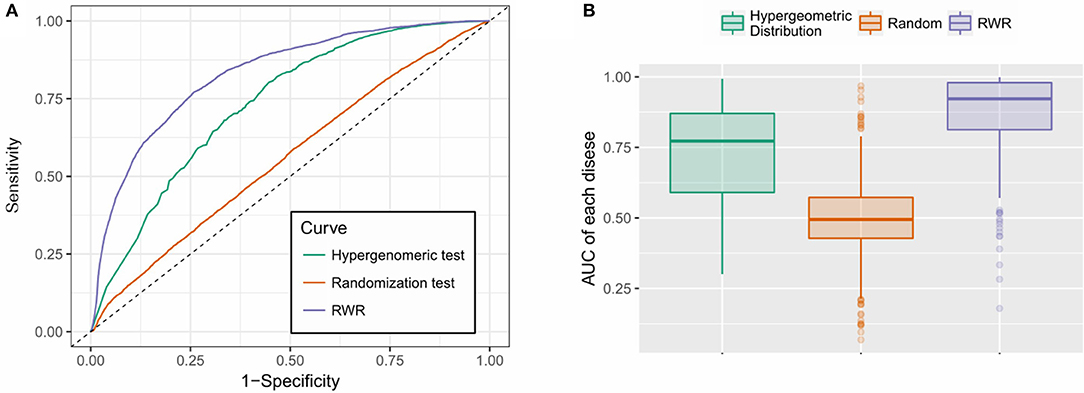
Figure 4. Performance evaluation. (A) RWR method achieved an AUC of 0.827, outperformed the hypergeometric test-based approach (AUC: 0.733) and the random predictor (AUC: 0.550); (B) RWR algorithm achieved superior performance on most of the diseases (average and median AUC: 0.867 and 0.913), compared to the other two methods: Hypergeometric test-based approach (average and median AUC: 0.723 and 0.772) and random predictor (average and median AUC: 0.486 and 0.479).
The prediction results are relatively reliable on the following diseases (Table 3), and they may be more relevant to epitranscriptome regulation.
Case of Study: Cancer-Related m6A Sites
We further examined the prediction performance of several common diseases. For top 100 predictions, the proposed approach achieved reasonable performance in all the 5 diseases tested (Table 4). As is shown in Figure 5, the cancer-related m6A site prediction achieved relatively steady performance. Indeed, recent studies suggest that m6A RNA methylation plays a crucial role in the pathologies of breast cancer, myeloid leukemia, liver cancer, carcinoma, glioma, etc. (Hsu et al., 2017; Stojković and Fujimori, 2017; Wang S. et al., 2017). Additionally, the model works better on cancer may partially due to the samples used are mostly related to cancer and tumor (see Table 1). As cancer samples were used, cancer-specific functions are more easily inferred from the data available. However, the samples were collected unbiasedly from all the published studies. The collection only reflects that most existing m6A-seq studies are either based on cancer cell lines or related to cancer. It suggests that inferring cancer-associated m6A sites may be more feasible than other diseases with the data cumulated from existing studies. We thus used cancer-related m6A sites in the next for a case study by checking whether our predictions are supported by existing literatures. Interestingly, many of our predicted associations are supported (see Table 5).
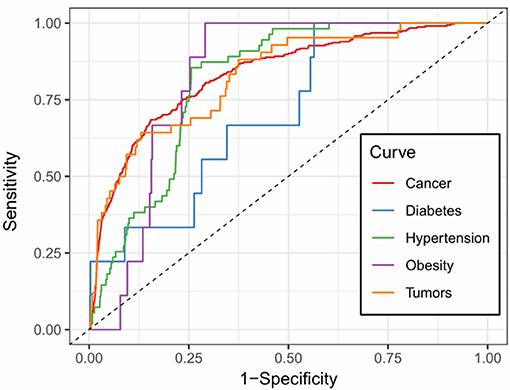
Figure 5. Prediction accuracy of five common m6A site-associated diseases. Figure shows the accuracy of disease associated m6A sites for five common diseases, including cancer (AUC: 0.832), diabetes (AUC: 0.717), hypertension (AUC: 0.812), obesity (AUC: 0.828) and tumors (AUC: 0.825), respectively. Among them, the prediction of cancer-related m6A sites achieved relatively stable performance.
Additionally, there are cases when dysregulated RNA methylation status is observed but does not lead to RNA level differential expression. Such associations may still be predicted by the proposed approach. DRUM works directly with RNA methylation data, and can thus detect associations that are observable at epitranscriptome layer only (see Table 6).
To gain more insights, the m6A-seq data from Non-Small Cell Lung Cancer (NSCLC) cell line (A549) and the normal control cell line (H1299) were obtained (Lin et al., 2016). Differential RNA methylation analysis and differential expression analysis were performed using exomePeak R/Bioconductor package and the Cuffdiff software, respectively, with their default settings. The results are then compared to the predictions from the proposed approach. In the end, 9 sites predicted to be associated with NSCLC were validated (Please see Supplementary Materials for more details), including one site located on the gene ING5) that shows no differential expression (log2 fold change = 0.07, FDR = 0.999) but significant differential methylation (log2 fold change = 0.762 and FDR = 0.027) (see Figure S3).
Altogether, our case studies indicate that the proposed method is effective in uncovering putative disease-m6A site associations, especially cancer-related m6A sites. The approach we developed may be useful to unveil the molecular pathologies regulated at epitranscriptome layer and provide potentially new perspective for effective therapeutic strategies of cancer and other diseases.
DRUM: Database for Disease-Associated RNA Methylation
To facilitate the exploration and direct query of our predicted results by the research community of RNA epigenetics, we developed an online database DRUM, which stands for disease-associated ribonucleic acid methylation. The website hosts the top 100 m6A sites predicted to be associated to 705 diseases at significance level of 0.1, and supports queries that may be a disease or the host gene of m6A site (see Figure 6). Additionally, the prediction results can be downloaded in batch for large-scale automated analysis such as result comparison. The DRUM website is freely available at: www.xjtlu.edu.cn/biologicalsciences/drum.
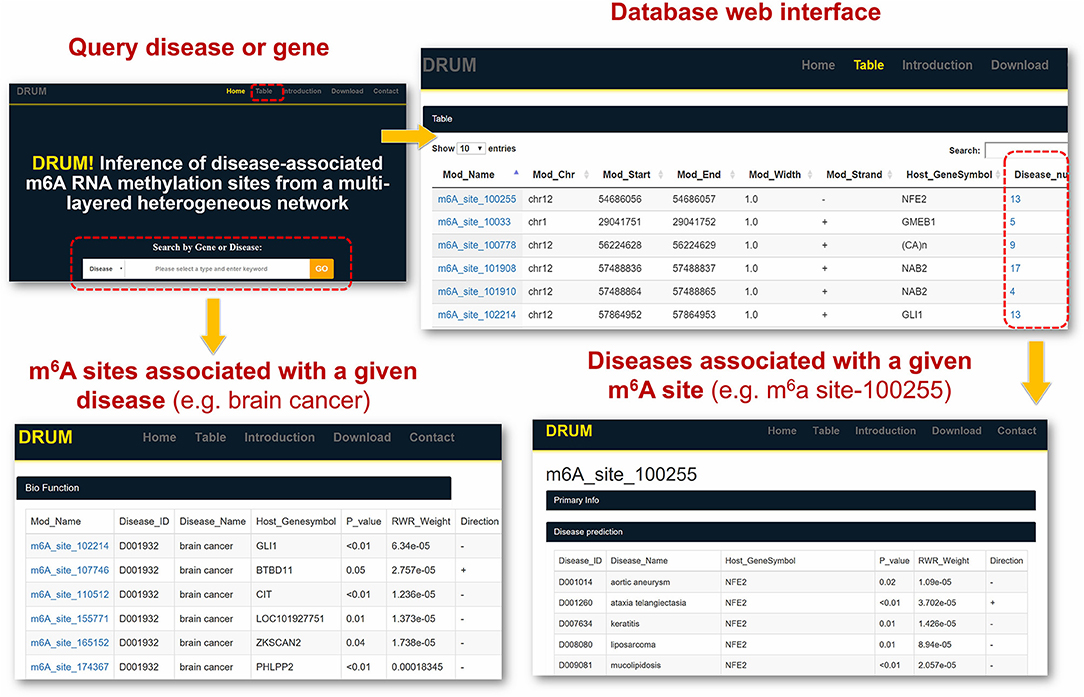
Figure 6. DRUM Database. DRUM is a public online database for disease-associated m6A sites. It integrates the m6A sites predicted to be associated with 705 diseases. The statistical significance of the prediction was assessed by random permutation. Users can access the data via the name of disease or the hosting gene of m6A site. It also supports the download of the entire prediction results for automated large-scale analysis.
Conclusions
Investigation of N6-methyladenosine (m6A) RNA modification over the past 4 decade, especially since 2012, has uncovered its critical biological functions in various cellular processes. It has been clearly shown that RNA modifications directly or indirectly contribute to disease development and play a critical role in the many diseases such as cancers (Deng et al., 2017; Wang S. et al., 2017) and virus infections (Gokhale and Horner, 2017). It is solely needed to cover the epitranscriptome perspective of disease pathology or unveil the regulatory circuit of diseases regulated from epitranscriptome layer.
We presented here a multi-layer heterogeneous network model coupled with the RWR algorithm, which effectively incorporated five types of association among the diseases, genes and m6A sites, to unveil the disease association of individual m6A RNA methylation sites. To evaluate the performance of the proposed approach, a ten-fold cross-validation was performed. Superior performance is achieved by our approach (overall AUC: 0.827, average AUC 0.867) compared with the hypergeometric test-based approach (overall AUC: 0.7333 and average AUC: 0.723) and the random predictor (overall AUC: 0.550 and average AUC: 0.486). And a number of cancer-related RNA methylation sites are validated from existing studies. At last, an online database DRUM was constructed to enable the query of top m6A sites related to 705 different diseases.
It is worth noting that, as indicated in equation (1), the calculation of RNA methylation profiles partially relies on the expression data, which inevitably induces dependency between them. Ideally, we want to use independent datasets that profile RNA methylation and expression, respectively. Additionally, the detectability of methylated molecule depends on the abundance of transcripts, i.e., if the expression level of a specific transcript is low, it is not possible to accurately determine the methylation level (M-value) of the m6A sites on it. The current formulation of methylation level, as shown in equation (1), will penalize those very lowly expressed transcripts, and reports an M-value close to 0, which may induces additional bias to methylation profiles (as shown in Figure 7A).
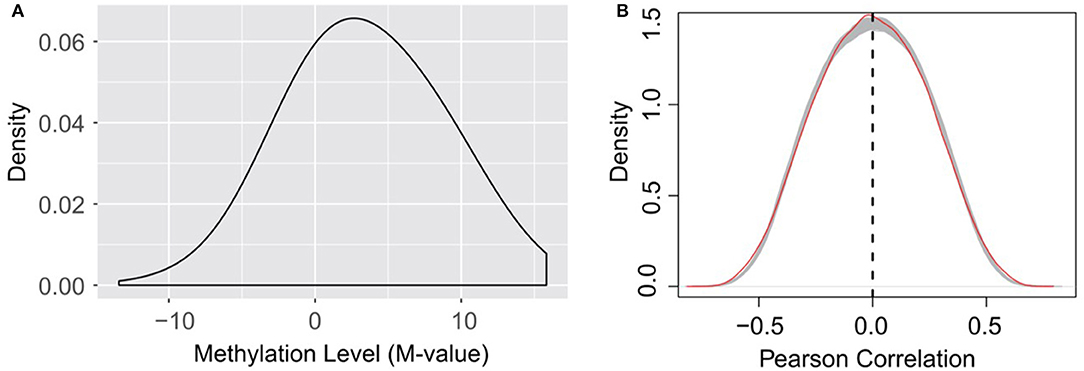
Figure 7. (A) Distribution of RNA methylation level (M-value). The estimated methylation levels are not strictly centered around 0, suggesting that the formation of M-value, which penalize lowly expressed transcripts as suggested by equation (1), may induce bias to the estimated methylation profiles on very lowly expressed transcripts. (B) Little linear correlation is observed between gene expression and RNA methylation profiles. The red line indicates the self-gene Pearson correlation coefficients, which are the correlation between the methylation level of a site and the expression level of its hosting gene. The gray lines indicate the Pearson correlation between the methylation level of a site and the expression level of a random gene under the 38 experimental conditions, when the methylation data and expression data are strictly separated, and thus independent from each other. A total of 1,000 gray lines were obtained from 1,000 random permutations, and serve as a null model of Pearson correlation distribution. The methylation level of an m6A site is not more linearly correlated (or anti-correlated) to the expression level of its host gene than a random gene.
Nevertheless, dispute of the bias and dependency that may be induced to the data, we didn't observe linear correlation (or anti-correlated) between the expression of the methylation level of an m6A site and the expression level of its host gene. The methylation level of an m6A site is not more linearly correlated (or anti-correlated) to the expression level of its host gene than a random gene (see Figure 7B). As suggested by a previous study, the epitranscriptome regulation changes only the percentage of methylated molecule, while transcriptional regulation changes only the abundance (Meng et al., 2014). Although slightly affecting each other, the two regulation mechanisms are observed to be largely independent and simultaneously regulate the transcriptome and epitranscriptome, which is consistent with our observation. As little linear correlation is observed between RNA methylation and gene expression profiles, and the association network was built based on Pearson correlation that relies on linear correlation (see section Materials and Methods), the predicted patterns associated with m6A sites are not likely to be dominated by gene expression profiles.
It is also worth noting that, by starting from the methylation profiles of individual m6A sites, our work focused specifically on the disease circuits that are potentially regulated at epitranscriptome layer at the resolution of individual m6A sites (see Figure 8). This work is substantially different from general disease-gene association prediction, where the gene and disease may interact at any layer of gene expression regulation, such as at transcriptional or post- transcriptional layer (Chen and Yan, 2013). The work is also quite different from existing works (Zhang et al., 2016b, 2019) that aimed to predict diseases that may be significantly regulated at epitranscriptome layer, because these studies unveiled only the potential association between diseases and m6A RNA methylation, but didn't answer specifically which m6A sites are involved in the regulation process. Compared to existing works, our computational framework provided a more specific resolution for the study of disease mechanism functions at the epitranscriptome layer.
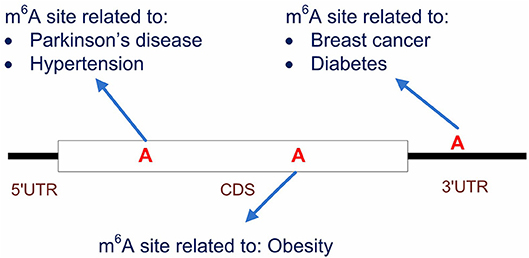
Figure 8. Predicting the disease-associated m6A sites. Our computational framework aims to predict disease-associated m6A sites. It is possible that multiple sites located on the same transcripts are associated to different diseases. Compared to general disease-gene association prediction, the proposed framework provides a more specific circuit of disease mechanism that functions at epitranscriptome layer.
This presented computational scheme can be easily extended in the future by incorporating additional data sources such as disease-related functional variants involved in m6A modification (Jiang et al., 2018; Zheng et al., 2018), the regulatory specificity of the RNA methyltransferases and demethylases (Liu H. et al., 2018), or the associations between m6A site to other biomolecules (Xuan et al., 2018), so as to further improve prediction accuracy. Additionally, the method can be conveniently applied to other RNA modification types such as m1A (Dominissini et al., 2016) and Pseudouridine (Cabili et al., 2011) as well in other species such as mouse and yeast when sufficient amount of data is available.
Data Availability
Publicly available datasets were analyzed in this study. This data can be found here: http://www.csbio.sjtu.edu.cn/bioinf/OUGene/.
Author Contributions
JM and YT conceived the idea and initialized the project. ZW, YT, and BS processed the raw data. YT and XW constructed the network. YT implemented prediction, the performance evaluation and the case studies. KC built the website. YT drafted the manuscript. All authors read, critically revised, and approved the final manuscript.
Funding
This work has been supported by National Natural Science Foundation of China [31671373]; Jiangsu University Natural Science Program [16KJB180027]; XJTLU Key Program Special Fund [KSF-T-01]; Jiangsu Six Talent Peak Program [XYDXX-118].
Conflict of Interest Statement
The authors declare that the research was conducted in the absence of any commercial or financial relationships that could be construed as a potential conflict of interest.
Supplementary Material
The Supplementary Material for this article can be found online at: https://www.frontiersin.org/articles/10.3389/fgene.2019.00266/full#supplementary-material
Abbreviations
m6A, N6-methyladenosine; MeRIP-Seq: methylated RNA immunoprecipitation sequencing; IP, immunoprecipitation; DRUM, disease-associated ribonucleic acid methylation; ROC, receiver operating characteristics; AUC, area under the ROC curve; Pcc, Pearson correlation coefficient.
References
Bansal, H., Yihua, Q., Iyer, S. P., Ganapathy, S., Proia, D. A., Proia, D., et al. (2014). WTAP is a novel oncogenic protein in acute myeloid leukemia. Leukemia 28, 1171–1174. doi: 10.1038/leu.2014.16
Barbieri, I., Tzelepis, K., Pandolfini, L., Shi, J., Millán-Zambrano, G., Robson, S. C., et al. (2017). Promoter-bound METTL3 maintains myeloid leukaemia by m6A-dependent translation control. Nature 552, 126–131. doi: 10.1038/nature24678
Batista Pedro, J., Molinie, B., Wang, J., Qu, K., Zhang, J., Li, L., et al. (2014). m6A RNA modification controls cell fate transition in mammalian embryonic stem cells. Cell Stem Cell 15, 707–719. doi: 10.1016/j.stem.2014.09.019
Berry, W. L., and Janknecht, R. (2013). KDM4/JMJD2 histone demethylases: epigenetic regulators in cancer cells. Cancer Res. 73, 2936–2942. doi: 10.1158/0008-5472.CAN-12-4300
Boccaletto, P., Machnicka, M. A., Purta, E., Piatkowski, P., Baginski, B., Wirecki, T. K., et al. (2017). MODOMICS: a database of RNA modification pathways. update. Nucl. Acids Res. 2017:gkx1030-gkx1030. doi: 10.1093/nar/gkx1030
Burnatowska-Hledin, M. A., Kossoris, J. B., Van Dort, C. J., Shearer, R. L., Zhao, P., Murrey, D. A., et al. (2004). T47D breast cancer cell growth is inhibited by expression of VACM-1, a cul-5 gene. Biochem. Biophys. Res. Commun. 319, 817–825. doi: 10.1016/j.bbrc.2004.05.057
Cabili, M. N., Trapnell, C., Goff, L., Koziol, M., Tazon-Vega, B., Regev, A., et al. (2011). Integrative annotation of human large intergenic noncoding RNAs reveals global properties and specific subclasses. Genes Dev. 25, 1915–1927. doi: 10.1101/gad.17446611
Cai, X., Wang, X., Cao, C., Gao, Y., Zhang, S., Yang, Z., et al. (2018). HBXIP-elevated methyltransferase METTL3 promotes the progression of breast cancer < em>via < /em> inhibiting tumor suppressor let-7g. Cancer Lett. 415:11–19. doi: 10.1016/j.canlet.2017.11.01
Cantara, W. A., Crain, P. F., Rozenski, J., McCloskey, J. A., Harris, K. A., Zhang, X., et al. (2011). The RNA modification database, RNAMDB: 2011 update. Nucl. Acids Res. 39, D195–D201. doi: 10.1093/nar/gkq1028
Cao, M. D., Cheng, M., Rizwan, A., Lu, J., Krishnamachary, B., Bhujwalla, Z. M., et al. (2016). Targeting choline phospholipid metabolism: GDPD5 and GDPD6 silencing decrease breast cancer cell proliferation, migration, and invasion. NMR Biomed. 29, 1098–1107. doi: 10.1002/nbm.3573
Carpenter, R. L., and Lo, H. W. (2012). Hedgehog pathway and GLI1 isoforms in human cancer. Discov. Med. 13, 105–113.
Chan, D. W., Chan, C. Y., Yam, J. W., Ching, Y. P., and Ng, I. O. (2006). Prickle-1 negatively regulates Wnt/beta-catenin pathway by promoting Dishevelled ubiquitination/degradation in liver cancer. Gastroenterology 131, 1218–1227. doi: 10.1053/j.gastro.2006.07.020
Chen, K., Wu, X., Zhang, Q., Wei, Z., Rong, R., Lu, Z., et al. (2019). WHISTLE: a high-accuracy map of the human N6-methyladenosine (m6A) epitranscriptome predicted using a machine learning approach. Nucl. Acids Res. gkz074. doi: 10.1093/nar/gkz074
Chen, M., Wei, L., Law, C. T., Tsang, F. H., Shen, J., Cheng, C. L., et al. (2017). RNA N6-methyladenosine methyltransferase METTL3 promotes liver cancer progression through YTHDF2 dependent post-transcriptional silencing of SOCS2. Hepatology. 67, 2254–2270. doi: 10.1002/hep.29683
Chen, X., Sun, Y. Z., Liu, H., Zhang, L., Li, J. Q., and Meng, J. (2017). RNA methylation and diseases: experimental results, databases, Web servers and computational models. Brief. Bioinformatics doi: 10.1093/bib/bbx142. [Epub ahead of print].
Chen, X., and Yan, G.-Y. (2013). Novel human lncRNA–disease association inference based on lncRNA expression profiles. Bioinformatics 29, 2617–2624. doi: 10.1093/bioinformatics/btt426
Cong, T., Fan, Q., Ping, W., Chi, Y., Wang, W., Ni, S., et al. (2016). DIXDC1 activates the Wnt signaling pathway and promotes gastric cancer cell invasion and metastasis. Mol. Carcinog. 55, 397–408. doi: 10.1002/mc.22290
Cui, Q., Shi, H., Ye, P., Li, L., Qu, Q., Sun, G., et al. (2017). m 6 A RNA methylation regulates the self-renewal and tumorigenesis of glioblastoma stem cells. Cell Rep. 18, 2622–2634. doi: 10.1016/j.celrep.2017.02.059
Das, S., Harris, L. G., Metge, B. J., Liu, S., Riker, A. I., Samant, R. S., et al. (2009). The hedgehog pathway transcription factor GLI1 promotes malignant behavior of cancer cells by up-regulating osteopontin. J. Biol. Chem. 284:22888. doi: 10.1074/jbc.M109.021949
Daulat, A. M., Bertucci, F., Audebert, S., Finetti, P., Josselin, E., et al. (2016). PRICKLE1 Contributes to cancer cell dissemination through its interaction with mTORC2. Dev. Cell 37, 311–325. doi: 10.1016/j.devcel.2016.04.011
Deng, X., Su, R., Feng, X., Wei, M., and Chen, J. (2017). Role of N6-methyladenosine modification in cancer. Curr. Opin. Genet. Dev. 48:1–7. doi: 10.1016/j.gde.2017.10.005
Dominissini, D., Moshitch-Moshkovitz, S., Schwartz, S., Salmon-Divon, M., Ungar, L., Osenberg, S., et al. (2012). Topology of the human and mouse m6A RNA methylomes revealed by m6A-seq. Nature 485, 201–206. doi: 10.1038/nature11112
Dominissini, D., Nachtergaele, S., Moshitch-Moshkovitz, S., Peer, E., Kol, N., Ben-Haim, M. S., et al. (2016). The dynamic N(1)-methyladenosine methylome in eukaryotic messenger RNA. Nature 530, 441–446. doi: 10.1038/nature16998
Du, T., Rao, S., Wu, L., Ye, N., Liu, Z., Hu, H., et al. (2015). An association study of the m6A genes with major depressive disorder in Chinese Han population. J. Affect. Disord. 183:279–286. doi: 10.1016/j.jad.2015.05.025
Fay, M. J., Longo, K. A., Karathanasis, G. A., Shope, D. M., Mandernach, C. J., Leong, J. R., et al. (2003). Analysis of CUL-5 expression in breast epithelial cells, breast cancer cell lines, normal tissues and tumor tissues. Mol. Cancer 2:40. doi: 10.1186/1476-4598-2-40
Feng, Y., Feng, L., Yu, D., Zou, J., and Huang, Z. (2016). srGAP1 mediates the migration inhibition effect of Slit2-Robo1 in colorectal cancer. J. Exp. Clin. Cancer Res. 35:191. doi: 10.1186/s13046-016-0469-x
Fu, Y., Dominissini, D., Rechavi, G., and He, C. (2014). Gene expression regulation mediated through reversible m(6)A RNA methylation. Nat. Rev. Genet. 15, 293–306. doi: 10.1038/nrg3724
Fustin, J. M., Doi, M., Yamaguchi, Y., Hida, H., Nishimura, S., Yoshida, M., et al. (2013). RNA-methylation-dependent RNA processing controls the speed of the circadian clock. Cell 155, 793–806. doi: 10.1016/j.cell.2013.10.026
Geula, S., Moshitch-Moshkovitz, S., Dominissini, D., Mansour, A. A., Kol, N., Salmon-Divon, M., et al. (2015). m6A mRNA methylation facilitates resolution of naïve pluripotency toward differentiation. Science 347:1261417. doi: 10.1126/science.1261417
Gokhale, N. S., and Horner, S. M. (2017). RNA modifications go viral. PLoS Pathog. 13:e1006188. doi: 10.1371/journal.ppat.1006188
Gopalakrishnan, S., Van Emburgh, B. O., and Robertson, K. D. (2008). DNA methylation in development and human disease. Mutat. Res. 647, 30–38. doi: 10.1016/j.mrfmmm.2008.08.006
He, X., Chang, S., Zhang, J., Zhao, Q., Xiang, H., Kusonmano, K., et al. (2007). MethyCancer: the database of human DNA methylation and cancer. Nucl. Acids Res. 36 (suppl_1), D836–D841. doi: 10.1093/nar/gkm730
Hsu, P. J., Shi, H., and He, C. (2017). Epitranscriptomic influences on development and disease. Genome Biol. 18:197. doi: 10.1186/s13059-017-1336-6
Huang, K.-Y., Lee, T.-Y., Kao, H.-J., Ma, C.-T., Lee, C.-C., Lin, T.-H., et al. (2019). dbPTM in 2019: exploring disease association and cross-talk of post-translational modifications. Nucl. Acids Res. 47, D298–D308. doi: 10.1093/nar/gky1074
Huang, W.-Y., Hsu, S.-D., Huang, H.-Y., Sun, Y.-M., Chou, C.-H., Weng, S.-L., et al. (2014). MethHC: a database of DNA methylation and gene expression in human cancer. Nucleic Acids Res. 43, D856–D861. doi: 10.1093/nar/gku1151
Jia, P., and Zhao, Z. (2014). VarWalker: personalized mutation network analysis of putative cancer genes from next-generation sequencing data. PLoS Comput. Biol. 10:e1003460. doi: 10.1371/journal.pcbi.1003460
Jiang, S., Xie, Y., He, Z., Zhang, Y., Zhao, Y., Chen, L., et al. (2018). m6ASNP: a tool for annotating genetic variants by m6A function. Gigascience. 7:giy035. doi: 10.1093/gigascience/giy035
Kim, D., Langmead, B., and Salzberg, S. L. (2015). HISAT: a fast spliced aligner with low memory requirements. Nat. Methods 12, 357–360. doi: 10.1038/nmeth.3317
Kim, Y., Kang, C., Min, B., and Yi, G.-S. (2015). Detection and analysis of disease-associated single nucleotide polymorphism influencing post-translational modification. BMC Med. Genomics 8:S7. doi: 10.1186/1755-8794-8-S2-S7
Kumar, S., and Cieplak, P. (2016). CaspNeuroD: a knowledgebase of predicted caspase cleavage sites in human proteins related to neurodegenerative diseases. Database 2016:baw142. doi: 10.1093/database/baw142
Kwok, C.-T., Marshall, A. D., Rasko, J. E. J., and Wong, J. J. L. (2017). Genetic alterations of m6A regulators predict poorer survival in acute myeloid leukemia. J. Hematol. Oncol. 10:39. doi: 10.1186/s13045-017-0410-6
Li, L., Duan, T., Wang, X., Zhang, R. H., Zhang, M., Wang, S., et al. (2016). KCTD12 regulates colorectal cancer cell stemness through the ERK pathway. Sci. Rep. 6:20460. doi: 10.1038/srep20460
Li, L., Zang, L., Zhang, F., Chen, J., Shen, H., Shu, L., et al. (2017). Fat mass and obesity-associated (FTO) protein regulates adult neurogenesis. Hum. Mol. Genet. 26, 2398–2411. doi: 10.1093/hmg/ddx128
Li, X., Tang, J., Huang, W., Wang, F., Li, P., Qin, C., et al. (2017a). The M6A methyltransferase METTL3: acting as a tumor suppressor in renal cell carcinoma. Oncotarget 8, 96103–96116. doi: 10.18632/oncotarget.21726
Li, X., Xiong, X., and Yi, C. (2017b). Epitranscriptome sequencing technologies: decoding RNA modifications. Nat. Methods 14, 23–31. doi: 10.1038/nmeth.4110
Li, Z., Weng, H., Su, R., Weng, X., Zuo, Z., Li, C., et al. (2017). FTO plays an oncogenic role in acute myeloid leukemia as a N 6-methyladenosine RNA demethylase. Cancer Cell 31, 127–141. doi: 10.1016/j.ccell.2016.11.017
Liang, C., Wang, G., Li, J., Zhang, T., Xu, P., and Wang, Y. (2013). SIDD: a semantically integrated database towards a global view of human disease. PLoS ONE 8:e75504. doi: 10.1371/journal.pone.0075504
Liao, Q., Liu, C., Yuan, X., Kang, S., Miao, R., Xiao, H., et al. (2011). Large-scale prediction of long non-coding RNA functions in a coding–non-coding gene co-expression network. Nucleic Acids Res. 39, 3864–3878. doi: 10.1093/nar/gkq1348
Lin, S., Choe, J., Du, P., Triboulet, R., and Gregory, R. I. (2016). The m 6 A methyltransferase METTL3 promotes translation in human cancer cells. Mol. Cell 62, 335–345. doi: 10.1016/j.molcel.2016.03.021
Liu, H., Wang, H., Wei, Z., Zhang, S., Hua, G., Zhang, S.-W., et al. (2018). MeT-DB V2.0: elucidating context-specific functions of N6-methyl-adenosine methyltranscriptome. Nucl. Acids Res. 46, D281–D287. doi: 10.1093/nar/gkx1080
Liu, J. A., Eckert, M., T. Harada, B., Liu, S., Lu, Z., Yu, K., M. Tienda, S., et al. (2018). m6A mRNA methylation regulates AKT activity to promote the proliferation and tumorigenicity of endometrial cancer. Nat. Cell Biol. 20, 1074–1083. doi: 10.1038/s41556-018-0174-4
Lowe, H. J., and Barnett, G. O. (1994). Understanding and using the medical subject headings (MeSH) vocabulary to perform literature searches. JAMA 271, 1103–1108. doi: 10.1001/jama.1994.03510380059038
Mendell, J. T., and Olson, E. N. (2012). MicroRNAs in stress signaling and human disease. Cell 148, 1172–1187. doi: 10.1016/j.cell.2012.02.005
Meng, J., Lu, Z., Liu, H., Zhang, L., Zhang, S., Chen, Y., et al. (2014). A protocol for RNA methylation differential analysis with MeRIP-Seq data and exomePeak R/Bioconductor package. Methods 69, 274–281. doi: 10.1016/j.ymeth.2014.06.008
Mesak, F. M., Osada, N., Hashimoto, K., Liu, Q. Y., and Cheng, E. N. (2003). Molecular cloning, genomic characterization and over-expression of a novel gene, XRRA1 identified from human colorectal cancer cell HCT116 Clone2_XRR and macaque testis. BMC Genomics 4:32. doi: 10.1186/1471-2164-4-32
Meyer, K. D., and Jaffrey, S. R. (2014). The dynamic epitranscriptome: N6-methyladenosine and gene expression control. Nat. Rev. Mol. Cell Biol. 15, 313–326. doi: 10.1038/nrm3785
Meyer, K. D., Saletore, Y., Zumbo, P., Elemento, O., Mason, C. E., and Jaffrey, S. R. (2012). Comprehensive analysis of mRNA methylation reveals enrichment in 3′ UTRs and near stop codons. Cell 149, 1635–1646. doi: 10.1016/j.cell.2012.05.003
Mo, X., Lei, S., Zhang, Y., and Zhang, H. (2018). Genome-wide enrichment of m6 A-associated single-nucleotide polymorphisms in the lipid loci. Pharmacogenomics J. 1. doi: 10.1038/s41397-018-0055-z. [Epub ahead of print].
Mo, X., Zhang, Y., and Lei, S. (2018a). Genome-wide identification of m 6 A-associated SNPs as potential functional variants for bone mineral density. Osteoporosis Int. 29, 2029–2039. doi: 10.1007/s00198-018-4573-y
Mo, X., Zhang, Y.-H., and Lei, S.-F. (2018b). Genome-wide identification of N6-methyladenosine (m6A) SNPs associated with rheumatoid arthritis. Front. Genet. 9:299. doi: 10.3389/fgene.2018.00299
Ohshio, I., Kawakami, R., Tsukada, Y., Nakajima, K., Kitae, K., Shimanoe, T., et al. (2016). ALKBH8 promotes bladder cancer growth and progression through regulating the expression of survivin. Biochem. Biophys. Res. Commun. 477, 413–418. doi: 10.1016/j.bbrc.2016.06.084
Pan, X., and Shen, H.-B. (2016). OUGENE. a disease associated over-expressed and under-expressed gene databaseOUGene. Sci. Bull. 61, 752–754. doi: 10.1007/s11434-016-1059-1
Schmalhofer, O., and Brabletz, S. T (2009). E-cadherin, beta-catenin, and ZEB1 in malignant progression of cancer. Cancer Metas. Rev. 28, 151–166. doi: 10.1007/s10555-008-9179-y
Schwartz, S., Mumbach, M. R., Jovanovic, M., Wang, T., Maciag, K., Bushkin, G. G., et al. (2014). Perturbation of m6A writers reveals two distinct classes of mRNA methylation at internal and 5' sites. Cell Rep. 8, 284–296. doi: 10.1016/j.celrep.2014.05.048
Shimada, K., Nakamura, M., Anai, S., De, V. M., Tanaka, M., Tsujikawa, K., et al. (2009). A novel human AlkB homologue, ALKBH8, contributes to human bladder cancer progression. Cancer Res. 69:3157. doi: 10.1158/0008-5472.CAN-08-3530
Shyamasundar, S., Lim, J. P., and Bay, B. H. (2016). miR-93 inhibits the invasive potential of triple-negative breast cancer cells in vitro via protein kinase WNK1. Int. J. Oncol. 49:2629. doi: 10.3892/ijo.2016.3761
Soini, Y., Kosma, V. M., and Pirinen, R. (2015). KDM4A, KDM4B and KDM4C in non-small cell lung cancer. Int. J. Clin. Exp. Pathol. 8, 12922–12928.
Spaderna, S., Schmalhofer, O., Wahlbuhl, M., Dimmler, A., Bauer, K., Sultan, A., et al. (2008). The transcriptional repressor ZEB1 promotes metastasis and loss of cell polarity in cancer. Cancer Res. 68:537. doi: 10.1158/0008-5472.CAN-07-5682
Stojković, V., and Fujimori, D. G. (2017). Mutations in RNA methylating enzymes in disease. Curr. Opin. Chem. Biol. 41, 20–27. doi: 10.1016/j.cbpa.2017.10.002
Tong, H., Faloutsos, C., and Pan, J. (2008). Random walk with restart: fast solutions and applications. Knowl. Inf. Syst. 14, 327–346. doi: 10.1007/s10115-007-0094-2
Visvanathan, A., Patil, V., Arora, A., Hegde, A. S., Arivazhagan, A., Santosh, V., et al. (2017). Essential role of METTL3-mediated m6A modification in glioma stem-like cells maintenance and radioresistance. Oncogene 37, 522–533. doi: 10.1038/onc.2017.351
Vu, L. P., Pickering, B. F., Cheng, Y., Zaccara, S., Nguyen, D., Minuesa, G., et al. (2017). The N6-methyladenosine (m6A)-forming enzyme METTL3 controls myeloid differentiation of normal hematopoietic and leukemia cells. Nat. Med. 23, 1369–1376. doi: 10.1038/nm.4416
Wan, Y., Tang, K., Zhang, D., Xie, S., Zhu, X., Wang, Z., et al. (2015). Transcriptome-wide high-throughput deep m(6)A-seq reveals unique differential m(6)A methylation patterns between three organs in Arabidopsis thaliana. Genome Biol. 16:272. doi: 10.1186/s13059-015-0839-2
Wang, L., Cao, X. X., Chen, Q., Zhu, T. F., Zhu, H. G., and Zheng, L. (2009). DIXDC1 targets p21 and cyclin D1 via PI3K pathway activation to promote colon cancer cell proliferation. Cancer Sci. 100, 1801–1808. doi: 10.1111/j.1349-7006.2009.01246.x
Wang, S., Sun, C., Li, J., Zhang, E., Ma, Z., Xu, W., et al. (2017). Roles of RNA methylation by means of N(6)-methyladenosine (m(6)A) in human cancers. Cancer Lett. 408, 112–120. doi: 10.1016/j.canlet.2017.08.030
Wang, W., Guo, M., Xia, X., Chao, Z., Yuan, Z., and Wu, S. (2017). XRRA1 Targets ATM/CHK1/2-Mediated DNA repair in colorectal cancer. Biomed Res. Int. 2017, 1–11. doi: 10.1155/2017/5718968
Wang, X., Lu, Z., Gomez, A., Hon, G. C., Yue, Y., Han, D., et al. (2014). N6-methyladenosine-dependent regulation of messenger RNA stability. Nature 505, 117–120. doi: 10.1038/nature12730
Wang, X., Zhao, B. S., Roundtree, I. A., Lu, Z., Han, D., Ma, H., et al. (2015). N(6)-methyladenosine modulates messenger RNA translation efficiency. Cell 161, 1388–1399. doi: 10.1016/j.cell.2015.05.014
Wei, C. M., Gershowitz, A., and Moss, B. (1976). 5'-Terminal and internal methylated nucleotide sequences in HeLa cell mRNA. Biochemistry 15, 397-401. doi: 10.1021/bi00647a024
Wijnen, J. P., Jiang, L., Greenwood, T. R., Cheng, M., Döpkens, M., Cao, M. D., et al. (2014). Silencing of the glycerophosphocholine phosphodiesterase GDPD5 alters the phospholipid metabolite profile in a breast cancer model in vivo as monitored by 31P magnetic resonance spectroscopy. NMR Biomed. 27, 692–699. doi: 10.1002/nbm.3106
Wu, H., and Zhang, Y. (2014). Reversing DNA methylation: mechanisms, genomics, and biological functions. Cell 156, 45–68. doi: 10.1016/j.cell.2013.12.019
Xiang, Y., Laurent, B., Hsu, C. H., Nachtergaele, S., Lu, Z., Sheng, W., et al. (2017). RNA m6A methylation regulates the ultraviolet-induced DNA damage response. Nature 543, 573–576. doi: 10.1038/nature21671
Xiong, Y., Wei, Y., Gu, Y., Zhang, S., Lyu, J., Zhang, B., et al. (2016). DiseaseMeth version 2.0: a major expansion and update of the human disease methylation database. Nucleic Acids Res. 45, D888–D895. doi: 10.1093/nar/gkw1123
Xu, X., and Wang, M. (2016). Inferring Disease associated phosphorylation sites via random walk on multi-layer heterogeneous network. IEEE/ACM Trans. Comput. Biol. Bioinformatics 13, 836–844. doi: 10.1109/TCBB.2015.2498548
Xuan, J.-J., Sun, W.-J., Lin, P.-H., Zhou, K.-R., Liu, S., Zheng, L.-L., et al. (2018). RMBase v2.0: deciphering the map of RNA modifications from epitranscriptome sequencing data. Nucl. Acids Res. 46, D327–D334. doi: 10.1093/nar/gkx934
Yao, L., Chi, Y., Hu, X., Li, S., Qiao, F., Wu, J., et al. (2016). Elevated expression of RNA methyltransferase BCDIN3D predicts poor prognosis in breast cancer. Oncotarget 7, 53895–53902. doi: 10.18632/oncotarget.9656
Yoon, K. J., Ringeling, F. R., Vissers, C., Jacob, F., Pokrass, M., Jimenez-Cyrus, D., et al. (2017). Temporal control of mammalian cortical neurogenesis by m6A Methylation. Cell 171, 877-889 e817. doi: 10.1016/j.cell.2017.09.003
Zhang, C., Samanta, D., Lu, H., Bullen, J. W., Zhang, H., Chen, I., et al. (2016a). Hypoxia induces the breast cancer stem cell phenotype by HIF-dependent and ALKBH5-mediated m6A-demethylation of NANOG mRNA. Proc. Natl. Acad. Sci. U.S.A. 113, E2047–E2056. doi: 10.1073/pnas.1602883113
Zhang, S., Zhang, S., Liu, L., Meng, J., and Huang, Y. (2016b). m6A-driver: identifying context-specific mRNA m6A methylation-driven gene interaction networks. PLoS Comput. Biol. 12:e1005287. doi: 10.1371/journal.pcbi.1005287
Zhang, S., Zhao, B. S., Zhou, A., Lin, K., Zheng, S., Lu, Z., et al. (2017). m(6)A Demethylase ALKBH5 Maintains Tumorigenicity of Glioblastoma Stem-like Cells by Sustaining FOXM1 Expression and Cell Proliferation Program. Cancer Cell 31, 591-606 e596. doi: 10.1016/j.ccell.2017.02.013
Zhang, S. Y., Zhang, S. W., Fan, X. N., Meng, J., Chen, Y., Gao, S. J., et al. (2019). Global analysis of N6-methyladenosine functions and its disease association using deep learning and network-based methods. PLoS Comput. Biol. 15:e1006663. doi: 10.1371/journal.pcbi.1006663
Zheng, Y., Nie, P., Peng, D., He, Z., Liu, M., Xie, Y., et al. (2018). m6AVar: a database of functional variants involved in m6A modification. Nucl. Acids Res. 46, D139–D145. doi: 10.1093/nar/gkx895
Keywords: disease, RWR, random walk with restart, m6A modification, Co-expression, network analysis
Citation: Tang Y, Chen K, Wu X, Wei Z, Zhang S-Y, Song B, Zhang S-W, Huang Y and Meng J (2019) DRUM: Inference of Disease-Associated m6A RNA Methylation Sites From a Multi-Layer Heterogeneous Network. Front. Genet. 10:266. doi: 10.3389/fgene.2019.00266
Received: 15 November 2018; Accepted: 11 March 2019;
Published: 03 April 2019.
Edited by:
Emanuele Buratti, International Centre for Genetic Engineering and Biotechnology, ItalyReviewed by:
Zhixiang Zuo, Sun Yat-sen University, ChinaJernej Ule, University College London, United Kingdom
Copyright © 2019 Tang, Chen, Wu, Wei, Zhang, Song, Zhang, Huang and Meng. This is an open-access article distributed under the terms of the Creative Commons Attribution License (CC BY). The use, distribution or reproduction in other forums is permitted, provided the original author(s) and the copyright owner(s) are credited and that the original publication in this journal is cited, in accordance with accepted academic practice. No use, distribution or reproduction is permitted which does not comply with these terms.
*Correspondence: Jia Meng, jia.meng@xjtlu.edu.cn