- 1Department of Animal Science, Shahrekord University, Shahrekord, Iran
- 2Department of Animal Science, University College of Agriculture and Natural Resources, University of Tehran, Karaj, Iran
- 3Department of Animal Life and Environment Sciences, Hankyong National University, Gyeonggi-do, South Korea
- 4Institute for Animal Science and Technology, Universitat Politècnica de València, València, Spain
There has been a growing interest in the genetic improvement of carcass traits as an important and primary breeding goal in the beef cattle industry over the last few decades. The use of correlated traits and molecular information can aid in obtaining more accurate estimates of breeding values. This study aimed to assess the improvement in the accuracy of genetic predictions for carcass traits by using ultrasound measurements and yearling weight along with genomic information in Hanwoo beef cattle by comparing four evaluation models using the estimators of the recently developed linear regression method. We compared the performance of single-trait pedigree best linear unbiased prediction [ST-BLUP and single-step genomic (ST-ssGBLUP)], as well as multi-trait (MT-BLUP and MT-ssGBLUP) models for the studied traits at birth and yearling date of steers. The data comprised of 15,796 phenotypic records for yearling weight and ultrasound traits as well as 5,622 records for carcass traits (backfat thickness, carcass weight, eye muscle area, and marbling score), resulting in 43,949 single-nucleotide polymorphisms from 4,284 steers and 2,332 bulls. Our results indicated that averaged across all traits, the accuracy of ssGBLUP models (0.52) was higher than that of pedigree-based BLUP (0.34), regardless of the use of single- or multi-trait models. On average, the accuracy of prediction can be further improved by implementing yearling weight and ultrasound data in the MT-ssGBLUP model (0.56) for the corresponding carcass traits compared to the ST-ssGBLUP model (0.49). Moreover, this study has shown the impact of genomic information and correlated traits on predictions at the yearling date (0.61) using MT-ssGBLUP models, which was advantageous compared to predictions at birth date (0.51) in terms of accuracy. Thus, using genomic information and high genetically correlated traits in the multi-trait model is a promising approach for practical genomic selection in Hanwoo cattle, especially for traits that are difficult to measure.
Introduction
There is a growing interest in improving economically important traits in beef cattle breeding programs worldwide because it has a substantial effect on the overall profitability of the meat production system. Carcass traits are a comprehensive term that constitutes a major set of target traits for genetic evaluation and selection in beef cattle. In the Korean beef industry, Hanwoo cattle has been intensively bred for meat over the last four decades, and its beef is well known for its marbled fat, tenderness, juiciness, flavor (Jo et al., 2012), and is regarded as a healthy food option (Joo et al., 2017). Presently, the selection index in Hanwoo breeding programs mainly focuses on carcass traits, including backfat thickness (BFT), carcass weight (CW), eye muscle area (EMA), and marbling score (MS), which are major selection criteria in attempts to improve both the quantity and quality of meat (Kim et al., 2017). However, measurement of these traits for genetic evaluation is expensive and difficult, as it requires the animals to be slaughtered to record their phenotypes. An efficient way to evaluate carcass traits is the use of ultrasound measurements at yearling age, which has the potential to improve the rate of genetic progress and reduce the time and expenses of slaughter tests (Jansen et al., 1985; Wilson, 1992). Previous studies have reported high positive genetic correlations between ultrasound measurements in yearling bulls and heifers and the corresponding traits measured in the carcass of progeny (Moser et al., 1998; Reverter et al., 2000; Crews and Kemp, 2002; Kemp et al., 2002; Crews et al., 2003; Lee and Kim, 2004; MacNeil et al., 2010; Yokoo et al., 2015; Elzo et al., 2017). In other words, the evaluation of animals based on ultrasounds of yearlings would be an alternative to anticipate the decision-making about selection for carcass traits. Thus, the inclusion of ultrasound data as correlated traits along with genomic information can result in improved accuracy in the estimation of breeding values for carcass traits.
In recent decades, with the advent of high-throughput single nucleotide polymorphism (SNP) genotyping technologies, genomic selection has become an appealing tool for accelerating genetic improvement in many breeding programs throughout the world (Meuwissen et al., 2001). Genomic selection allows for a better prediction of EBVs than the classical methods, especially for young animals without performance. Several genomic methods have been developed and applied, among which single-step genomic BLUP (ssGBLUP) has been widely used in routine genomic evaluation (Legarra et al., 2009; Misztal et al., 2009; Aguilar et al., 2010; Christensen and Lund, 2010). A single-step genomic BLUP is a modification of the GBLUP method, in which phenotypes of genotyped and non-genotyped individuals are used simultaneously along with an H matrix that combines the pedigree-based numerator relationship matrix (A) and a genomic relationship matrix (G) (Legarra et al., 2009; Aguilar et al., 2010; Christensen and Lund, 2010). Several studies have indicated that this single-step method leads to higher accuracy than the pedigree-based BLUP or genomic BLUP (GBLUP) in Hanwoo cattle (Lee et al., 2017; Mehrban et al., 2019; Lopez et al., 2020; Naserkheil et al., 2020) and other beef cattle (Onogi et al., 2014; Lourenco et al., 2015; Elzo et al., 2017). Most of these studies have been based on single-trait analyses, although this method is suitable for multi-trait analyses. Previously, it has been shown that a multi-trait genomic model for genetically correlated traits can increase the accuracy of breeding values compared to single-trait genomic models using simulated (Calus and Veerkamp, 2011; Guo et al., 2014) and real data (Jia and Jannink, 2012; Ismael et al., 2017; Mehrban et al., 2019; Song et al., 2019). In our previous study (Mehrban et al., 2019), the strong positive genetic correlation between yearling weight and CW resulted in an improvement in the accuracy of genomic prediction for CW in Hanwoo when using multi-trait genetic analyses. Furthermore, based on the literature, it is expected that the use of yearling weight and ultrasound data can improve the accuracy of genomic prediction for carcass traits while simultaneously reducing the generation interval (Elzo et al., 2017; Mehrban et al., 2019). Nonetheless, utilizing all available yearling ultrasound measurements to further increase the accuracy of genomic prediction for corresponding carcass traits in Hanwoo cattle has not yet been investigated. Hence, the objective of this study was to assess the improvement in the accuracy of genetic predictions for carcass traits by using yearling measurements on weight and ultrasound traits as well as genomic information in Hanwoo beef cattle. To this aim four models: pedigree-based single-trait BLUP (ST-BLUP), pedigree-based multi-trait BLUP (MT-BLUP), single-trait single-step genomic BLUP (ST-ssGBLUP), and multi-trait single-step genomic BLUP (MT-ssGBLUP) were compared, based on bias, dispersion, and accuracy of breeding values for carcass traits using a linear regression (LR) method (Legarra and Reverter, 2018).
Materials and Methods
Animals and Phenotypes
The phenotypic measurements used in this study were derived from 10,114 bulls and 5,682 Hanwoo steers born between 1997 and 2017 at Hanwoo Experiment Station (Seosan, South Korea).
The records of body weight at 12 months of age for 15,796 animals, yearling ultrasound traits of 8,945 animals, carcass traits of 5,622 steers, and a pedigree consisting of 54,284 animals were used.
The eight traits analyzed were yearling body weight (YW), ultrasound backfat thickness (UBFT), ultrasound eye muscle area (UEMA), ultrasound intramuscular fat (UIMF), BFT, CW, EMA, and MS. Briefly, carcass traits were measured according to the Korean carcass grading system in steers at approximately 24 months of age, ribbed between the 13th rib and the first lumbar vertebrae after chilling for approximately 24 h postmortem. The MS was graded using a 9-point scale following the Korean Beef Marbling Standard (1 = trace, 9 = very abundant). The ultrasound carcass traits were collected by an experienced technician using a B-mode real-time ultrasound device (HS-2000, FHK Co. Ltd, Japan) with an 18 cm, 3.5 MHz linear probe. The animals were scanned for the longissimus muscle area between the 13th rib and the first lumbar UBFT over the longissimus muscle at point three-fourths the length ventrally of the longissimus muscle area, and UIMF was the percentage intramuscular fat. Phenotypic data for UBFT, UEMA, and UIMF were obtained by analyzing the ultrasonic images with scanning software developed by HIC based on the CUP Lab at Iowa State University1. The yearling weight for each animal was determined from the weight at the termination of the test (body weight at ∼12 months) and the previous weight recorded at a time point before the termination (body weight at ∼6 months), according to the equation described by Park et al. (2013). Descriptive statistics are shown for each trait in Table 1.
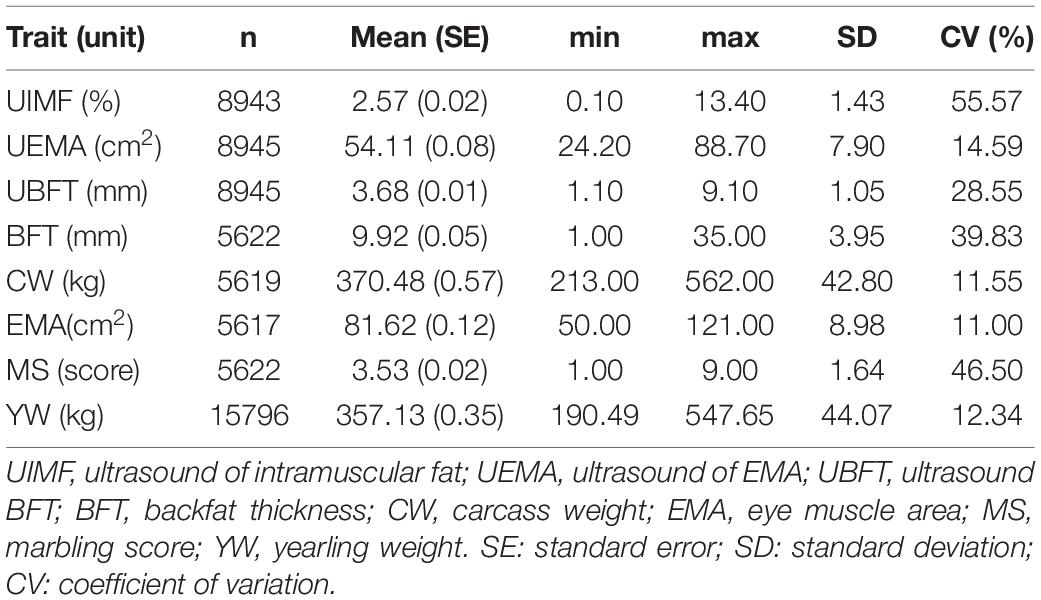
Table 1. Descriptive statistics for the ultrasound at 12 months of age, carcass traits, and yearling weight in Hanwoo cattle.
Genotypes
A total of 6,616 animals were genotyped (animal and SNP call rate > 90%) with the Illumina Bovine SNP50 BeadChip (Illumina, San Diego, CA, United States). These genotyping data were from animals (4,284 steers and 2,332 bulls) with at least one observation for the interest of traits or as a sire in the pedigree. Missing genotypes of SNPs were imputed using FImpute V3 software (Sargolzaei et al., 2014), and 52,791 SNPs on the 29 chromosomes were obtained. Markers with minor allele frequencies lower than 0.01 (8,819 SNPs), and a maximum difference between the observed and expected frequency of 0.15, as a departure of heterozygous from the Hardy-Weinberg equilibrium (23 SNPs) were excluded. After quality control, the number of SNPs remaining for subsequent analyses was 43,949.
Statistical Analyses
Estimation of Variance-Covariance Components
The Bayesian multi-trait pedigree-based animal model was applied to obtain variance-covariance components for YW, ultrasound, and carcass traits using the gibbsf90test software (Misztal et al., 2015) as follows:
where y is the vector of observations for the trait of interest; b is the vector of fixed effects, including batch-sex-technician (108 levels), birth place (103 levels), and age of recording as a covariate for ultrasound traits; batch-sex (87 levels) and birth place (109 levels) for YW, slaughter date (274 levels), and slaughter age (days from birth to slaughter) was considered as a covariate for carcass traits; u is the vector of random genetic additive effects; e is the vector of random residual effects; X and Z are incidence matrices related to fixed and random genetic additive effects, respectively. Var(u) = G⊗A and Var(e) = R⊗I were assumed, where A is the numerator relationship matrix, I is the identity matrix, and G and R are additive genetic and residual covariance, respectively, for the eight traits.
The Markov Chain Monte Carlo (MCMC) method was used to estimate variance components and heritabilities in 550,000 cycles with a thinning interval of 50 and 50,000 iterations as burn-in. The convergence of the chain was verified by visual inspection of trace plots. The variance components and correlations were estimated as the posterior means of the corresponding sampled values. Furthermore, the estimated variance components from the multi-trait model were compared with those obtained using a single-trait animal model, which assumes zero for genetic and residual covariance among the traits.
Modeling Methods
Pedigree-Based BLUP
Pedigree-based evaluations were performed to estimate breeding values for each trait based on ST-BLUP and MT-BLUP models. The model is defined in equation (1). Furthermore, the genetic and residual covariances for the eight traits were assumed to be zero for the ST-BLUP model.
Single-Step GBLUP
The ssGBLUP analyses using both ST-ssGBLUP and MT-ssGBLUP models were applied for genetic evaluations, which combined pedigree and genomic information. The ssGBLUP has the same model as BLUP, except for the inverse of numerator relationship matrix A – 1, which was replaced by matrix H – 1. This matrix was obtained using the following equation (Aguilar et al., 2010, 2011) with preGSf90 software (Aguilar et al., 2014):
where A is the numerator relationship matrix, G is the genomic relationship matrix (VanRaden, 2008), and A22 is the numerator relationship matrix for genotyped animals.
Variance components used in the MT-BLUP (ST-BLUP) and MT-ssGBLUP (ST-ssGBLUP) were estimated using the pedigree-based multi-trait (single-trait) animal model (Equation 1). Breeding values in all models were obtained using BLUPF90 software (Misztal et al., 2015).
Genetic Evaluation at Birth and Yearling Date
Genetic evaluation of animals can be performed at two stages of birth or yearling dates (Kim et al., 2017), regardless of whether single- or multi-trait models are considered and with or without genomic information. Note that the phenotype data of steers obtained at slaughter date (around two years of age), but the body weight and ultrasound measurements were also recorded at the yearling date for each animal. In traditional BLUP evaluations, the EBV of carcass traits for a steer can be expressed as the parent average EBV at birth date and the composition of parent average and the yield deviation (due to correlated traits) at the yearling date (Mrode, 2014). In addition, genomic information contains direct genomic value and pedigree prediction derived from A22 (Aguilar et al., 2010). Hence, the extra source of information to estimate breeding values at the yearling date relative to the birth date is the part of the yield deviation, regardless of the model. By comparing the two evaluations at birth and yearling dates, increases in accuracy due to including correlated traits (yearling measurement of weight and ultrasound) of focal animals in the models can be observed.
Assessment of Accuracy, Bias, and Dispersion
There are several validation methods to evaluate genetic prediction (Legarra and Reverter, 2018); however, a validation method that can support any model and any data structure is preferable. Recently, Legarra and Reverter (2018) described the ability of the LR method to validate the results obtained from genetic evaluations. In this method, estimates of bias, dispersion, and accuracy of EBV/GEBV derived from a dataset containing old (“partial”) compared with a dataset containing both old and new records (“whole”) for the same individuals. The validation population was defined as animals born in 2016 and 2017; therefore, the EBV/GEBV were estimated for focal animals in either the whole and partial dataset using different models. In the partial dataset, the phenotypes of focal animals were assumed to be unknown, and only the genotypes and pedigree information were retained. The number of steers in the focal population was 803 for carcass traits, 815 for ultrasound traits, and 817 for YW. The expectation of EBV/GEBV accuracy from the partial dataset is , where is the average inbreeding coefficient, 2 is the average relationship between individuals, is the genetic variance at equilibrium in a population under selection, which is estimated using Gibbs sampling technique described by Sorensen et al. (2001), and () is the vector of estimated breeding value for focal animals using the whole (partial) dataset (Legarra and Reverter, 2018). Estimator represents the relative increase in accuracy from the ith method to MT-ssGBLUP in the partial data (Legarra and Reverter, 2018). Thus, is the superiority of accuracy using MT-ssGBLUP compared to the ith method in all traits under study when partial data were used. This value confirms the extent to which the inclusion of genomic information and correlated traits in the MT-ssGBLUP method increases the accuracy of predictions for steers.
The expected bias is estimated from the difference between the mean estimated breeding value in partial and whole data, . The expected value of this estimator is zero if the evaluation is unbiased. The estimator of dispersion of the breeding value is the regression of on , . In the absence of over- or under-dispersion of breeding values, the expected value of this estimator is one (Legarra and Reverter, 2018).
Results
Estimates of Genetic Parameters
The variance components and heritability estimates were similar for both single and multi-traits except for CW, in which the magnitude of genetic variance in the multi-trait model was significantly higher than in the single-trait, which led to increasing heritability in the former (0.42) relative to the latter (0.31) model. The estimated heritabilities were moderate for the ultrasound and YW ranged from 0.19 to 0.33, while the heritabilities were high for BFT, EMA, and MS traits ranged from 0.48 to 0.59 (Supplementary Table 1). The lowest and highest heritability values were obtained for UIMF and MS, respectively, regardless of whether a single- or multi-trait model was used.
Favorable and high genetic correlations were estimated between UIMF and MS (0.78), UBFT and BFT (0.63), and UEMA and EMA (0.65). In addition, the estimated genetic correlation between YW and CW was positive and high (0.84) and relatively moderate between YW and EMA (0.43) (Supplementary Table 2). The phenotypic correlations were generally lower than the genetic correlations between UIMF and MS (0.42), UBFT and BFT (0.40), UEMA and EMA (0.48), and YW and CW (0.78) (Supplementary Table 2).
Genetic Evaluation of Steers
Predictive accuracies for carcass traits at birth and yearling dates of steers obtained with the four models (ST-BLUP, MT-BLUP, ST-ssGBLUP, and MT-SSBLUP) are presented in Figures 1 and 2. Our results indicate that averaged across all traits, the accuracy of ssGBLUP methods (0.52) was higher than that of pedigree-based BLUP (0.34), regardless of the use of single- or multi-trait models. On average, the accuracy of prediction can be further improved by implementing yearling weight and ultrasound data in the MT-ssGBLUP model (0.56) for the corresponding carcass traits compared to the ST-ssGBLUP model (0.49).
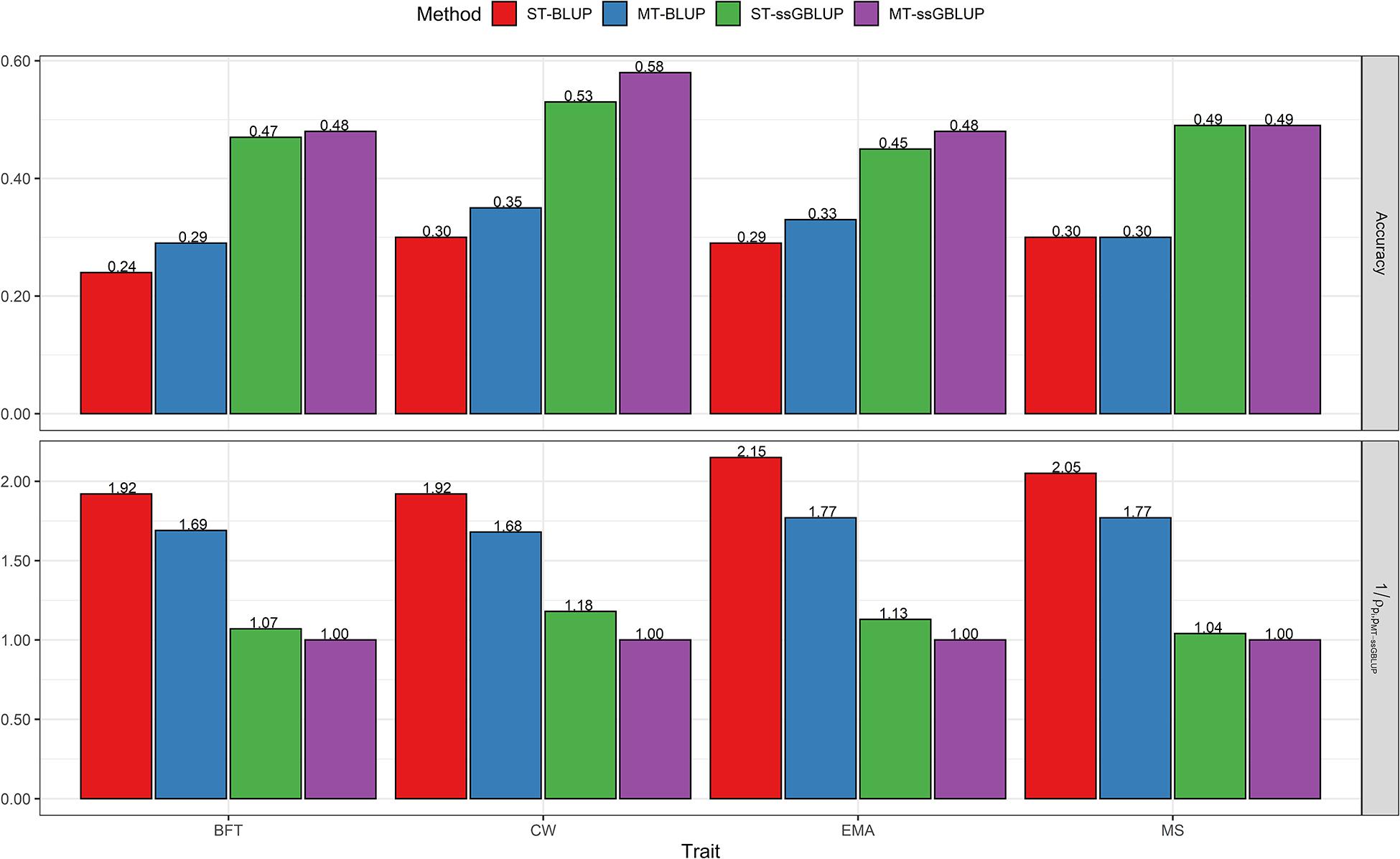
Figure 1. Accuracy of breeding values obtained using BLUP (ST-BLUP, MT-BLUP) and ssGBLUP (ST-ssGBLUP, MT-ssGBLUP) models. : the relative increase of accuracy from ith model to MT-ssGBLUP in the partial data for steers at birth date. ST and MT are single-trait and multi-trait analyses, respectively. BFT, backfat thickness; CW, carcass weight; EMA, eye muscle area; MS, marbling score; YW, yearling weight.
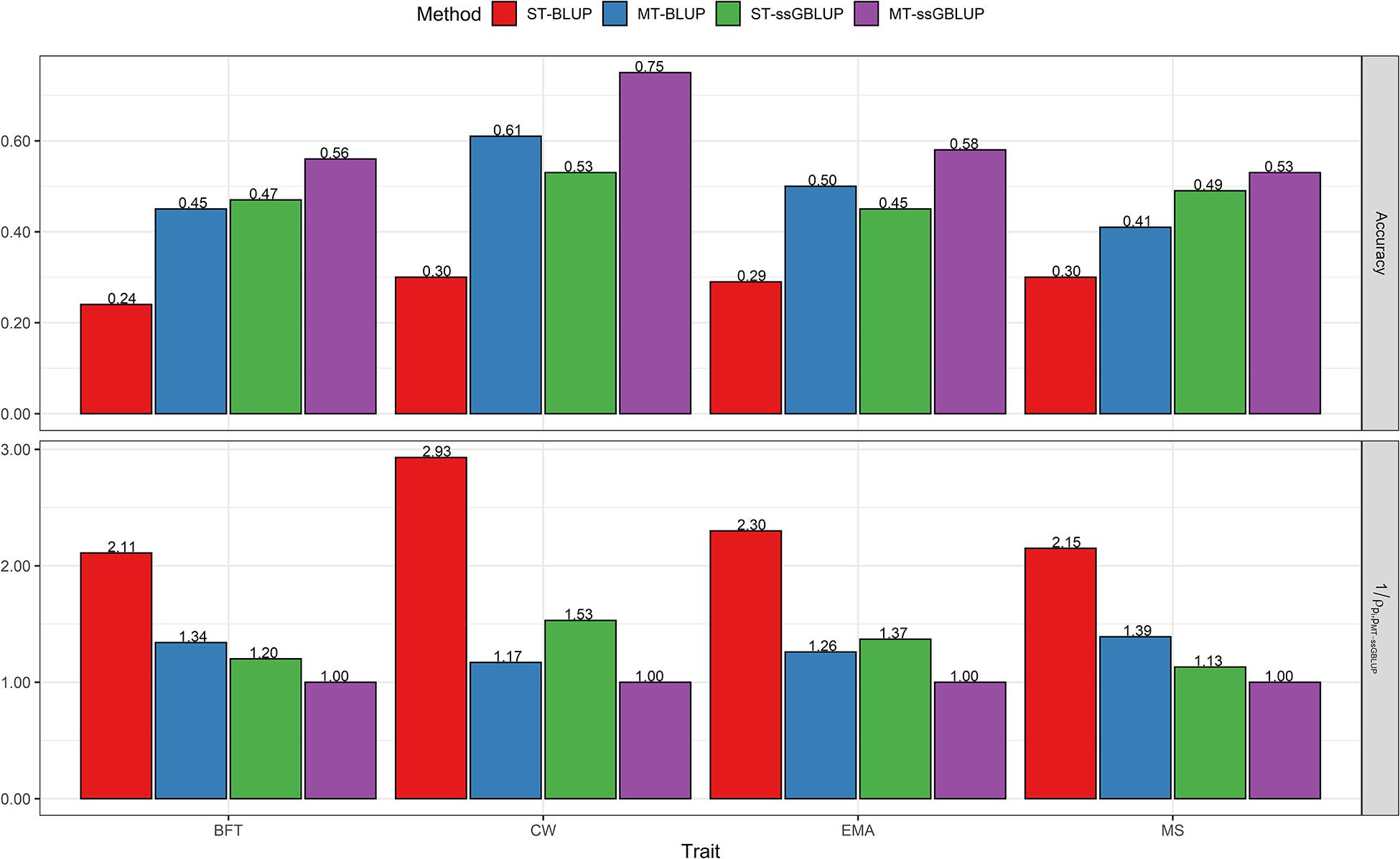
Figure 2. Accuracy of breeding values obtained using BLUP (ST-BLUP, MT-BLUP) and ssGBLUP (ST-ssGBLUP, MT-ssGBLUP) models. : the relative increase of accuracy from ith model to MT-ssGBLUP in the partial data for steers at yearling date. ST and MT are single-trait and multi-trait analyses, respectively. BFT, backfat thickness; CW, carcass weight; EMA, eye muscle area; MS, marbling score; YW, yearling weight.
Genetic Evaluation of Steers at Birth Date
The results showed that the accuracies of estimated breeding values from single-step genomic BLUP methods were substantially higher than those of conventional BLUP for all traits, regardless of the single or multi-trait models. When information on the correlated traits was included in the prediction of breeding values in the multi-trait analyses, the accuracy increased for BFT, CW, and EMA, whereas a trivial gain in accuracy was observed for MS by changing from a single-trait to a multi-trait model (Figure 1). Our results show that the MT-ssGBLUP approximately doubled (100 % improvement) the accuracy of breeding values compared with the ST-BLUP model, and the superiority over MT-BLUP was obvious for BFT (69%), CW (68%), EMA (77%), and MS (77%) in the present study. Moreover, the accuracy was improved by 7% for BFT, 18% for CW, 13% for EMA, and 4% for MS by using the MT-ssGBLUP method rather than the ST-ssGBLUP (Figure 1). Concerning the bias, for genetic predictions of validation animals, pedigree BLUP methods showed less bias than predictions from ssGBLUP methods for BFT, while bias for MS was small and similar in pedigree and genomic methods. The smallest and largest biases for CW were observed with the MT-BLUP and MT-ssGBLUP methods, respectively. The prediction obtained with ST-BLUP for EMA was marginally more biased compared with the other three models (Figure 3). For all traits, the average value for the estimator of dispersion varied from 0.72 to 1.13 (average absolute deviation 0.99) for ST-BLUP, from 0.85 to 0.99 (average absolute deviation 0.92) for MT-BLUP, from 0.92 to 1.15 (average absolute deviation 0.98) for ST-ssGBLUP, and from 0.85 to 1.12 (average absolute deviation 0.94) for the MT-ssGBLUP method (Figure 3).
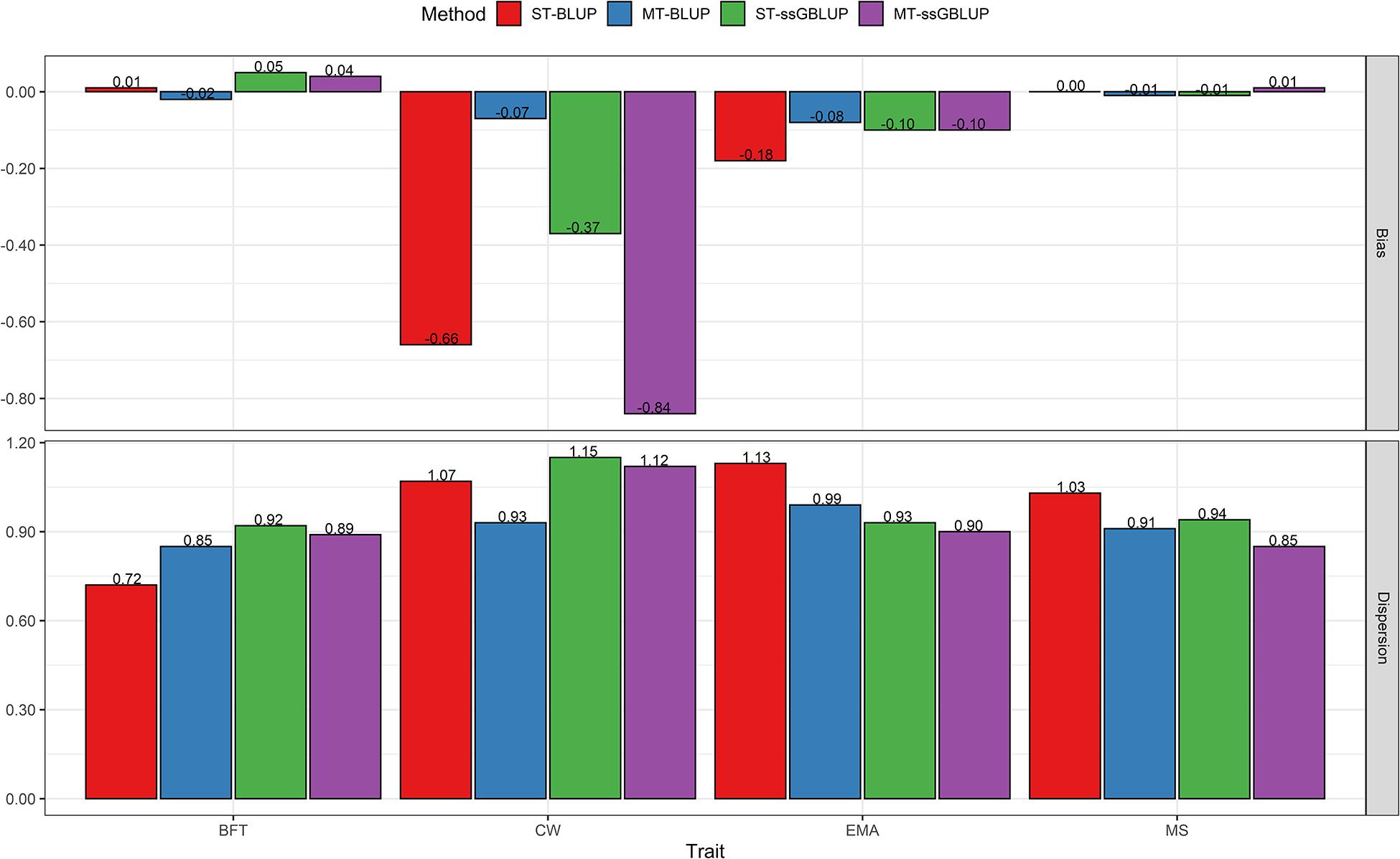
Figure 3. Bias and Dispersion of breeding values obtained using BLUP (ST-BLUP, MT-BLUP) and ssGBLUP (ST-ssGBLUP, MT-ssGBLUP) models for steers at birth date. ST and MT are single-trait and multi-trait analyses, respectively. BFT, backfat thickness; CW, carcass weight; EMA, eye muscle area; MS, marbling score; YW, yearling weight.
Genetic Evaluation of Steers at Yearling Date
Figure 2 compares the results obtained with ST-BLUP, MT-BLUP, ST-ssGBLUP, and MT-ssGBLUP for carcass traits when predictions were based on data of the correlated traits at the yearling age of steers. Accuracies ranged from 0.24 to 0.30 (average 0.28) for ST-BLUP, from 0.41 to 0.61 (average 0.49) for MT-BLUP, from 0.45 to 0.53 (average 0.49) for ST-ssGBLUP, and from 0.53 to 0.75 (average 0.61) for the MT-ssGBLUP method. Across the studied traits, the multi-trait models substantially outperformed the single-trait models in both pedigree and genomic evaluations. The highest accuracies were obtained when using MT-ssGBLUP for all traits at the yearling date. The results show that the relative gain in accuracy of GEBV using MT-ssGBLUP was twice as high as that estimated with ST-BLUP for BFT, EMA, and MS, whereas for CW it was approximately triple. In addition, the MT-ssGBLUP method was superior to MT-BLUP with an increase or relative gain of 34% for BFT, 17% for CW, 26% for EMA, and 39% for MS. In addition, using the MT-ssGBLUP model led to an increase in accuracy of 20, 53, 37, and 13% for BFT, CW, EMA, and MS, respectively, compared with the ST-ssBLUP model (Figure 2). In terms of the GEBV bias of the validation individuals at the yearling date, the MT-ssGBLUP had the lowest bias for CW and EMA, while the pedigree-based BLUP methods showed the lowest bias for the BFT trait (Figure 4). The bias estimates for MS were not very different among the models except for MT-BLUP, in which the highest bias was observed (Figure 4). Across the studied traits, the value of dispersion ranged from 0.72 to 1.13 (average absolute deviation 0.99) for ST-BLUP, from 0.95 to 1.08 (average absolute deviation 1.02) for MT-BLUP, from 0.92 to 1.15 (average absolute deviation 0.98) for ST-ssGBLUP, and from 0.90 to 1.06 (average absolute deviation 0.98) for MT-ssGBLUP method (Figure 4).
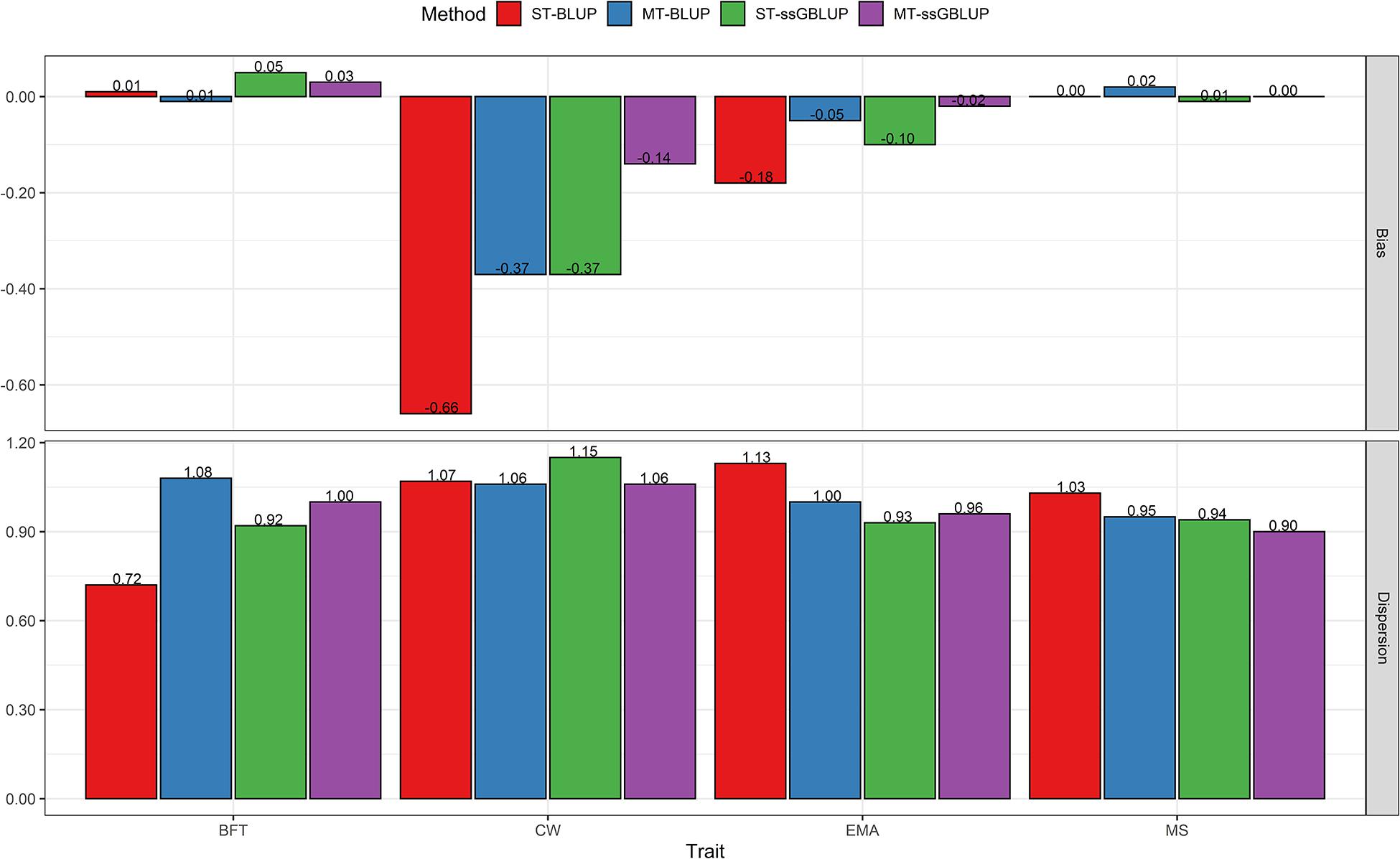
Figure 4. Bias and Dispersion of breeding values obtained using BLUP (ST-BLUP, MT-BLUP) and ssGBLUP (ST-ssGBLUP, MT-ssGBLUP) models for steers at yearling date. ST and MT are single-trait and multi-trait analyses, respectively. BFT, backfat thickness; CW, carcass weight; EMA, eye muscle area; MS, marbling score; YW, yearling weight.
Comparisons of Accuracy, Bias, and Dispersion Between Evaluation at Birth and Yearling Date of Steers
The results of evaluations at birth date were comparable with those obtained from the yearling date in terms of accuracy, bias, and dispersion of predictions. When additional information of the correlated traits at the yearling date was used, prediction accuracies were higher than those of birth date for all traits (Figures 1, 2). The results showed that the accuracies of EBV/GEBV at the yearling date were higher than those derived from multi-trait models at birth. The population accuracy of GEBVs at yearling date compared with birth date were 0.56 vs. 0.48 for BFT, 0.75 vs. 0.58 for CW, 0.58 vs. 0.48 for EMA, and 0.53 vs. 0.49 for MS using the MT-ssGBLUP method. In other words, this study showed the impact of genomic information and the correlated traits on predictions at yearling date (average 0.61) using the MT-ssGBLUP model, which was advantageous compared to predictions at birth date (average 0.51) in terms of accuracy. In addition, the results of the MT-BLUP model at yearling (birth) date were 0.45 (0.29), 0.61 (0.35), 0.50 (0.33), and 0.41 (0.30) for BFT, CW, EMA, and MS, respectively (Figures 1, 2). Regarding the bias, predictions based on genomic information and the correlated traits at the yearling date using multi-trait models appeared to have less bias than predictions at birth date, except for CW in the MT-BLUP model (Figures 3, 4). The results of dispersion using multi-trait models showed values close to 1 at the yearling date for all traits when compared to birth date (Figures 3, 4).
Discussion
Based on the observed results, the yearling ultrasound and carcass traits were moderate to high heritable traits, which were comparable to those reported in Brangus (Moser et al., 1998), Angus (Reverter et al., 2000), Simmental (Crews et al., 2003), Nellore (Yokoo et al., 2015; Silva et al., 2021), multi-breed Angus-Brahman (Elzo et al., 2017), and Hanwoo cattle (Lee and Kim, 2004; Hwang et al., 2014; Choi et al., 2015). Moreover, the heritability estimates for carcass traits and yearling weight in our study corresponded to those obtained by Mehrban et al. (2019) in Hanwoo cattle. Furthermore, our results showed moderate to strong positive genetic correlations between yearling ultrasound and corresponding carcass traits, which were within the range of values estimated in the literature (Moser et al., 1998; Reverter et al., 2000; Kemp et al., 2002; Crews et al., 2003; Lee and Kim, 2004; Elzo et al., 2017; Su et al., 2017). These results indicate that better responses to selection for carcass traits can be expected when the corresponding carcass traits are measured by ultrasound at yearling age.
The results of this study showed that the accuracies of breeding values obtained from ssGBLUP were substantially higher than those of the traditional BLUP model for all traits at birth and yearling date, regardless of using single- or multi-trait genomic models. The observed gain in accuracy is due to additional variation in genomic information capture in Mendelian sampling by a realized relationship matrix (Christensen et al., 2012). Hence, it was noted that the gain in accuracy from pedigree to genomic predictions can be explained by better relationships (VanRaden, 2008; Hayes et al., 2009). Consequently, the inclusion of genomic information in the single-trait analyses led to an average increase of accuracy from 0.28 to 0.49 by changing the method from ST-BLUP to ST-ssGBLUP across all carcass traits at birth or yearling date. Similarly, results of the multi-trait methods showed an increase in accuracy of 0.32 to 0.51 at birth date and from 0.49 to 0.61 at yearling date by moving the method from MT-BLUP to MT-ssGBLUP for all the carcass traits. Many studies have reported the superiority of ssGBLUP over pedigree-based methods in both single (Tsuruta et al., 2011; Carillier et al., 2014; Onogi et al., 2014; Koivula et al., 2015; Lourenco et al., 2015; Lee et al., 2017; Teissier et al., 2018; Oget et al., 2019; Lopez et al., 2020; Mehrban et al., 2021) and multi-trait models (Mehrban et al., 2019; Karaman et al., 2020; Lopez et al., 2020).
The accuracies of ST-BLUP models for carcass traits were similar to those previously reported for Hanwoo beef cattle (Lee et al., 2017; Mehrban et al., 2019; Lopez et al., 2020; Mehrban et al., 2021); however, the accuracies derived from ST-ssGBLUP models were lower than those obtained by Lopez et al. (2020) and higher than those obtained by Lee et al. (2017) and Mehrban et al. (2019). One of the main reasons for this difference between the studies could be the difference in the proportion of genotyped individuals as a reference population (Goddard and Hayes, 2009; VanRaden et al., 2009). The number of genotyped steers for carcass traits in previous studies in the same breed was 988 (Lee et al., 2017), 1,151 (Mehrban et al., 2019), and 16,892 (Lopez et al., 2020), while in this study it was 4,284. Moreover, the accuracies of the ST-ssGBLUP model for carcass traits were higher than those obtained by Mehrban et al. (2021), except for CW using the same dataset. This difference between the results of the two studies is due to the cross-validation method. Mehrban et al. (2021) used fold-cross validation with precorrected data that could be biased (Legarra and Reverter, 2018). The LR method was addressed in this study, without pre-corrected data. This method is independent of heritability and errors in estimates of fixed effects (Legarra and Reverter, 2018), in addition to the application of any statistical model and any data structure (Misztal et al., 2020). For instance, Bermann et al. (2021) applied the LR method to analyze mortality in broiler chickens using a threshold model and showed that this method is a useful tool for predicting improvement in the accuracy of breeding values due to the inclusion of genomic information.
One effective strategy to improve the accuracy of evaluations for carcass traits that are difficult or expensive to measure is the use of a multi-trait model with indicator traits that are easier or cheaper to measure. One of the main benefits of the multi-trait model is the simultaneous use of information from relatives and genetically correlated traits, mostly for the candidate animals and their offspring without any phenotypes (e.g., the pre-selection of young bulls for progeny testing).
The results demonstrated that multi-trait models generally yielded higher accuracies than single-trait models in both pedigree and genomic evaluations. Based on our results, the average improvement of the accuracies for carcass traits was +4 percentage points (from 0.28 to 0.32%) using MT-BLUP instead of ST-BLUP, and +2 percentage points (from 0.49 to 0.51) by changing the model from ST-ssGBLUP to MT-ssGBLUP when using the information of correlated traits from relatives at the birth date of steers. Similar to predictions at the birth date, accuracies using multi-trait models were higher than single-trait models for all traits at the yearling date because of the additional information of correlated traits (yearling weight and ultrasound traits) recorded from steers. In other words, when the data for yearling ultrasound of steers as correlated traits were available at yearling age, the average gain in accuracy for carcass traits obtained +21 percentage points (from 0.28 to 0.49) by changing the model from ST-BLUP to MT-BLUP, and +12 percentage points (from 0.49 to 0.61) by moving the model from ST-ssGBLUP to MT-ssGBLUP. Consistent with our results, several previous studies have shown that multi-trait genomic prediction is superior to single-trait genomic analyses using simulated (Calus and Veerkamp, 2011; Guo et al., 2014) and real data (Jia and Jannink, 2012; Schulthess et al., 2016; Ismael et al., 2017; Mehrban et al., 2019; Song et al., 2019; Karaman et al., 2020).
In general, the advantage of using multi-trait models for improving the prediction accuracies at the yearling date was more obvious than those obtained at the birth date for all the considered traits. These results can be attributed to the use of phenotypic records from YW and ultrasound traits of steers (in the validation population) in addition to their relatives’ information at the yearling date, whereas information from the correlated traits (YW and ultrasound traits) is not available for focal animals at birth, and only information from their relatives was included in the multi-trait models. In this line, (Ismael et al., 2017) revealed that using a multi-trait model improved the reliability of breeding values for an interval from calving to first high activity, while no gain was observed for calving to first insemination, resulting in the number of phenotypic records being higher for those calving to first insemination (1,472,313) than those calving to first high activity (36,504). Similarly, Song et al. (2019) reported that the ssGBLUP model performed better than both the pedigree-based BLUP or GBLUP for seven body measurement traits in pigs. They demonstrated an improvement in accuracy from a two-trait ssGBLUP model compared to ST-ssGBLUP when a high genetic correlation between traits was observed. Moreover, a similar conclusion was drawn by Sun et al. (2010), who reported that the reliability of EBV for fertility traits (low heritability) was increased with the multi-trait traditional BLUP model when information regarding milk yield traits (high heritability) as a genetically correlated trait was used in Danish Holstein cattle. Similar to the findings of Mehrban et al. (2019), the prediction accuracy was improved for CW and EMA by switching from a single-trait to multi-trait model; however, for two other traits (BFT and MS), the results of that study disagree with our findings using the multi-trait model. This difference can be explained by the existence of a high genetic correlation between BFT and UBFT (0.63), and MS and UIMF (0.78), along with the inclusion of ultrasound measurements on the candidate animals in the multi-trait model. Lopez et al. (2020) indicated that accuracies from single-trait and multi-trait models were similar for carcass traits, which is inconsistent with the results of the present study. However, they did not consider YW and ultrasound traits in the model and found low to moderate genetic correlations among carcass traits. Consequently, the results of the current study demonstrated that the genetic gain in carcass traits can be improved at birth and yearling date when yearling weight and ultrasound traits are included as correlated traits in a multi-trait model.
Conclusion
The findings revealed that using genomic information along with including high genetically correlated traits, such as yearling weight and ultrasound traits, in the multi-trait model can be useful in ongoing Hanwoo cattle breeding programs, especially for traits that are difficult to measure, such as carcass traits.
Data Availability Statement
The data analyzed in this study is subject to the following licenses/restrictions: This dataset was generated to select proven bulls by Hanwoo Improvement center under the national government rule of Hanwoo breeding schemes. Requests to access these datasets should be directed to DL.
Ethics Statement
All animals used in this study were cared for slaughtered according to animal health and welfare guidelines approved by the Animal Care and Use Committee of the National Institute of Animal Science (NIAS), Rural Development Administration of South Korea. Genotype and phenotype data were collected by Hanwoo Improvement Center (HIC) of National Agricultural Cooperative Federation.
Author Contributions
HM and DL conceived and designed the study. HM conducted statistical analyses. HM and MN interpreted and discussed the results and drafted the manuscript. DL was responsible for phenotypic and genotyping data collection. NI-E conceived the study, evaluated the experiments, interpreted, and discussed the results, and edited the manuscript. All authors read and approved the final manuscript.
Funding
This study was supported by the Cooperative Research Program for Agriculture Science and Technology Development (Project No. PJ01260601), Rural Development Administration (RDA), South Korea.
Conflict of Interest
The authors declare that the research was conducted in the absence of any commercial or financial relationships that could be construed as a potential conflict of interest.
Publisher’s Note
All claims expressed in this article are solely those of the authors and do not necessarily represent those of their affiliated organizations, or those of the publisher, the editors and the reviewers. Any product that may be evaluated in this article, or claim that may be made by its manufacturer, is not guaranteed or endorsed by the publisher.
Acknowledgments
We are grateful to the staff of the Korean Hanwoo Improvement Center of the National Agricultural Cooperative Federation for supplying data and blood samples from Hanwoo cattle.
Supplementary Material
The Supplementary Material for this article can be found online at: https://www.frontiersin.org/articles/10.3389/fgene.2021.692356/full#supplementary-material
Abbreviations
HIC, Hanwoo Improvement Center; ST-BLUP, Single-trait pedigree best linear unbiased prediction; MT-BLUP, Multi-trait pedigree best linear unbiased prediction; GBLUP, Genomic best linear unbiased prediction; ssGBLUP, Single-step genomic best linear unbiased prediction; SNP, Single nucleotide polymorphism; GEBV, Genomic estimated breeding value; BFT, Backfat thickness; CW, Carcass weight; EMA, Eye muscle area; MS, Marbling score; YW, Yearling body weight; UBFT, Ultrasound backfat thickness; UEMA, Ultrasound eye muscle area; UIMF, Ultrasound intramuscular fat; LR, Linear regression.
Footnotes
References
Aguilar, I., Misztal, I., Johnson, D. L., Legarra, A., Tsuruta, S., and Lawlor, T. J. (2010). Hot topic: a unified approach to utilize phenotypic, full pedigree, and genomic information for genetic evaluation of Holstein final score. J. Dairy Sci. 93, 743–752. doi: 10.3168/jds.2009-2730
Aguilar, I., Misztal, I., Legarra, A., and Tsuruta, S. (2011). Efficient computation of the genomic relationship matrix and other matrices used in single-step evaluation. J. Anim. Breed. Genet. 128, 422–428. doi: 10.1111/j.1439-0388.2010.00912.x
Aguilar, I., Misztal, I., Tsuruta, S., Legarra, A., and Wang, H. (2014). “PREGSF90 – POSTGSF90: computational tools for the implementation of single-step genomic selection and genome-wide association with ungenotyped individuals in BLUPF90 programs,” in Proceedings of the 10th World Congress on Genetics Applied to Livestock Production (WCGALP) (Vancouver: American Society of Animal Science).
Bermann, M., Legarra, A., Hollifield, M. K., Masuda, Y., Lourenco, D., and Misztal, I. (2021). Validation of single-step GBLUP genomic predictions from threshold models using the linear regression method: an application in chicken mortality. J. Anim. Breed. Genet. 138, 4–13. doi: 10.1111/jbg.12507
Calus, M. P., and Veerkamp, R. F. (2011). Accuracy of multi-trait genomic selection using different methods. Genet. Sel. Evol. 43:26. doi: 10.1186/1297-9686-43-26
Carillier, C., Larroque, H., and Robert-Granié, C. (2014). Comparison of joint versus purebred genomic evaluation in the French multi-breed dairy goat population. Genet. Sel. Evol. 46:67. doi: 10.1186/s12711-014-0067-3
Choi, T. J., Alam, M., Cho, C. I., Lee, J. G., Park, B., Kim, S., et al. (2015). Genetic parameters for yearling weight, carcass traits, and primal-cut yields of Hanwoo cattle. J. Anim. Sci. 93, 1511–1521. doi: 10.2527/jas.2014-7953
Christensen, O. F., and Lund, M. S. (2010). Genomic prediction when some animals are not genotyped. Genet. Sel. Evol. 42:2. doi: 10.1186/1297-9686-42-2
Christensen, O. F., Madsen, P., Nielsen, B., Ostersen, T., and Su, G. (2012). Single-step methods for genomic evaluation in pigs. Animal 6, 1565–1571. doi: 10.1017/S1751731112000742
Crews, D. H. Jr., and Kemp, R. A. (2002). Genetic evaluation of carcass yield using ultrasound measures on young replacement beef cattle. J. Anim. Sci. 80, 1809–1818. doi: 10.2527/2002.8071809x
Crews, D. H. Jr., Pollak, E. J., Weaber, R. L., Quaas, R. L., and Lipsey, R. J. (2003). Genetic parameters for carcass traits and their live animal indicators in Simmental cattle. J. Anim. Sci. 81, 1427–1433. doi: 10.2527/2003.8161427x
Elzo, M. A., Mateescu, R. G., Johnson, D. D., Scheffler, T. L., Scheffler, J. M., Carr, C., et al. (2017). Genomic-polygenic and polygenic predictions for nine ultrasound and carcass traits in Angus-Brahman multibreed cattle using three sets of genotypes. Livest. Sci. 202, 58–66. doi: 10.1016/j.livsci.2017.05.027
Goddard, M. E., and Hayes, B. J. (2009). Mapping genes for complex traits in domestic animals and their use in breeding programmes. Nat. Rev. Genet. 10, 381–391. doi: 10.1038/nrg2575
Guo, G., Zhao, F., Wang, Y., Zhang, Y., Du, L., and Su, G. (2014). Comparison of single-trait and multiple-trait genomic prediction models. BMC Genet. 15:30. doi: 10.1186/1471-2156-15-30
Hayes, B. J., Visscher, P. M., and Goddard, M. E. (2009). Increased accuracy of artificial selection by using the realized relationship matrix. Genet. Res. 91, 47–60. doi: 10.1017/S0016672308009981
Hwang, J. M., Cheong, J. K., Kim, S. S., Jung, B. H., Koh, M. J., Kim, H. C., et al. (2014). Genetic analysis of ultrasound and carcass measurement traits in a regional Hanwoo steer population. Asian Australas. J. Anim. Sci. 27, 457–463. doi: 10.5713/ajas.2013.13543
Ismael, A., Løvendahl, P., Fogh, A., Lund, M. S., and Su, G. (2017). Improving genetic evaluation using a multitrait single-step genomic model for ability to resume cycling after calving, measured by activity tags in Holstein cows. J. Dairy Sci. 100, 8188–8196. doi: 10.3168/jds.2017-13122
Jansen, J., Andersen, B. B., Bergström, P. L., Busk, H., Lagerweij, G. W., and Oldenbroek, J. K. (1985). In vivo estimation of body composition in young bulls for slaughter. 2. The prediction of carcass traits from scores, ultrasonic scanning and body measurements. Livest. Prod. Sci. 12, 231–240. doi: 10.1016/0301-6226(85)90053-3
Jia, Y., and Jannink, J. L. (2012). Multiple-trait genomic selection methods increase genetic value prediction accuracy. Genetics 192, 1513–1522. doi: 10.1534/genetics.112.144246
Jo, C., Cho, S. H., Chang, J., and Nam, K. C. (2012). Keys to production and processing of Hanwoo beef: a perspective of tradition and science. Anim. Front. 2, 32–38. doi: 10.2527/af.2012-0060
Joo, S. T., Hwang, Y. H., and Frank, D. (2017). Characteristics of Hanwoo cattle and health implications of consuming highly marbled Hanwoo beef. Meat Sci. 132, 45–51. doi: 10.1016/j.meatsci.2017.04.262
Karaman, E., Lund, M. S., and Su, G. (2020). Multi-trait single-step genomic prediction accounting for heterogeneous (co) variances over the genome. Heredity 124, 274–287. doi: 10.1038/s41437-019-0273-4
Kemp, D. J., Herring, W. O., and Kaiser, C. J. (2002). Genetic and environmental parameters for steer ultrasound and carcass traits. J. Anim. Sci. 80, 1489–1496. doi: 10.2527/2002.8061489x
Kim, S., Alam, M., and Park, M. (2017). Breeding initiatives for Hanwoo cattle to thrive as a beef industry–A review study. J. Anim. Breed. Genet. 1, 102–124.
Koivula, M., Strandén, I., Pösö, J., Aamand, G. P., and Mäntysaari, E. A. (2015). Single-step genomic evaluation using multitrait random regression model and test-day data. J. Dairy Sci. 98, 2775–2784. doi: 10.3168/jds.2014-8975
Lee, D. H., and Kim, H. C. (2004). Genetic relationship between ultrasonic and carcass measurements for meat qualities in Korean steers. Asian Australas. J. Anim. Sci 17, 7–12. doi: 10.5713/ajas.2004.7
Lee, J., Cheng, H., Garrick, D., Golden, B., Dekkers, J., Park, K., et al. (2017). Comparison of alternative approaches to single-trait genomic prediction using genotyped and non-genotyped Hanwoo beef cattle. Genet. Sel. Evol. 49:2. doi: 10.1186/s12711-016-0279-9
Legarra, A., Aguilar, I., and Misztal, I. (2009). A relationship matrix including full pedigree and genomic information. J. Dairy Sci. 92, 4656–4663. doi: 10.3168/jds.2009-2061
Legarra, A., and Reverter, A. (2018). Semi-parametric estimates of population accuracy and bias of predictions of breeding values and future phenotypes using the LR method. Genet. Sel. Evol. 50:53. doi: 10.1186/s12711-018-0426-6
Lopez, B. I., Lee, S.-H., Shin, D.-H., Oh, J.-D., Chai, H.-H., Park, W., et al. (2020). Accuracy of genomic evaluation using imputed high-density genotypes for carcass traits in commercial Hanwoo population. Livest. Sci. 241:104256. doi: 10.1016/j.livsci.2020.104256
Lourenco, D. A., Tsuruta, S., Fragomeni, B. O., Masuda, Y., Aguilar, I., Legarra, A., et al. (2015). Genetic evaluation using single-step genomic best linear unbiased predictor in American Angus. J. Anim. Sci. 93, 2653–2662. doi: 10.2527/jas.2014-8836
MacNeil, M. D., Nkrumah, J. D., Woodward, B. W., and Northcutt, S. L. (2010). Genetic evaluation of Angus cattle for carcass marbling using ultrasound and genomic indicators. J. Anim. Sci. 88, 517–522. doi: 10.2527/jas.2009-2022
Mehrban, H., Lee, D. H., Naserkheil, M., Moradi, M. H., and Ibáñez-Escriche, N. (2019). Comparison of conventional BLUP and single-step genomic BLUP evaluations for yearling weight and carcass traits in Hanwoo beef cattle using single trait and multi-trait models. PLoS One 14:e0223352. doi: 10.1371/journal.pone.0223352
Mehrban, H., Naserkheil, M., Lee, D. H., Cho, C., Choi, T., Park, M., et al. (2021). Genomic prediction using alternative strategies of weighted single-step genomic BLUP for yearling weight and carcass traits in Hanwoo beef cattle. Genes 12:266. doi: 10.3390/genes12020266
Meuwissen, T. H. E., Hayes, B. J. B., and Goddard, M. E. M. (2001). Prediction of total genetic value using genome-wide dense marker maps. Genetics 157, 1819–1829.
Misztal, I., Legarra, A., and Aguilar, I. (2009). Computing procedures for genetic evaluation including phenotypic, full pedigree, and genomic information. J. Dairy Sci. 92, 4648–4655. doi: 10.3168/jds.2009-2064
Misztal, I., Lourenco, D., and Legarra, A. (2020). Current status of genomic evaluation. J. Anim. Sci. 98:skaa101. doi: 10.1093/jas/skaa101
Misztal, I., Tsuruta, S., Lourenco, D., Aguilar, I., Legarra, A., and Vitezica, Z. (2015). Manual for BLUPF90 Family of Programs. Athens: University of Georgia 199.
Moser, D. W., Bertrand, J. K., Misztal, I., Kriese, L. A., and Benyshek, L. L. (1998). Genetic parameter estimates for carcass and yearling ultrasound measurements in Brangus cattle. J. Anim. Sci. 76, 2542–2548. doi: 10.2527/1998.76102542x
Naserkheil, M., Lee, D. H., and Mehrban, H. (2020). Improving the accuracy of genomic evaluation for linear body measurement traits using single-step genomic best linear unbiased prediction in Hanwoo beef cattle. BMC Genet. 21:144. doi: 10.1186/s12863-020-00928-1
Oget, C., Teissier, M., Astruc, J. M., Tosser-Klopp, G., and Rupp, R. (2019). Alternative methods improve the accuracy of genomic prediction using information from a causal point mutation in a dairy sheep model. BMC Genomics 20:719. doi: 10.1186/s12864-019-6068-4
Onogi, A., Ogino, A., Komatsu, T., Shoji, N., Simizu, K., Kurogi, K., et al. (2014). Genomic prediction in Japanese Black cattle: application of a single-step approach to beef cattle. J. Anim. Sci. 92, 1931–1938. doi: 10.2527/jas.2014-7168
Park, B., Choi, T., Kim, S., and Oh, S. H. (2013). National genetic evaluation (system) of Hanwoo (Korean native cattle). Asian Australas. J. Anim. Sci. 26, 151–156. doi: 10.5713/ajas.2012.12439
Reverter, A., Johnston, D. J., Graser, H. U., Wolcott, M. L., and Upton, W. H. (2000). Genetic analyses of live-animal ultrasound and abattoir carcass traits in Australian Angus and Hereford cattle. J. Anim. Sci. 78, 1786–1795. doi: 10.2527/2000.7871786x
Sargolzaei, M., Chesnais, J. P., and Schenkel, F. S. (2014). A new approach for efficient genotype imputation using information from relatives. BMC Genomics 15:478. doi: 10.1186/1471-2164-15-478
Schulthess, A. W., Wang, Y., Miedaner, T., Wilde, P., Reif, J. C., and Zhao, Y. (2016). Multiple-trait- and selection indices-genomic predictions for grain yield and protein content in rye for feeding purposes. Theor. Appl. Genet. 129, 273–287. doi: 10.1007/s00122-015-2626-6
Silva, R. P., Espigolan, R., Berton, M. P., Lôbo, R. B., Magnabosco, C. U., Pereira, A. S. C., et al. (2021). Genomic prediction ability for carcass composition indicator traits in Nellore cattle. Livest. Sci. 245:104421. doi: 10.1016/j.livsci.2021.104421
Song, H., Zhang, J., Zhang, Q., and Ding, X. (2019). Using different single-step strategies to improve the efficiency of genomic prediction on body measurement traits in pig. Front. Genet. 9:730. doi: 10.3389/fgene.2018.00730
Sorensen, D., Fernando, R., and Gianola, D. (2001). Inferring the trajectory of genetic variance in the course of artificial selection. Genet. Res. 77, 83–94. doi: 10.1017/s0016672300004845
Su, H., Golden, B., Hyde, L., Sanders, S., and Garrick, D. (2017). Genetic parameters for carcass and ultrasound traits in Hereford and admixed Simmental beef cattle: accuracy of evaluating carcass traits. J. Anim. Sci. 95, 4718–4727. doi: 10.2527/jas2017.1865
Sun, C., Madsen, P., Lund, M. S., Zhang, Y., Nielsen, U. S., and Su, G. (2010). Improvement in genetic evaluation of female fertility in dairy cattle using multiple-trait models including milk production traits. J. Anim. Sci. 88, 871–878. doi: 10.2527/jas.2009-1912
Teissier, M., Larroque, H., and Robert-Granié, C. (2018). Weighted single-step genomic BLUP improves accuracy of genomic breeding values for protein content in French dairy goats: a quantitative trait influenced by a major gene. Genet. Sel. Evol. 50:31. doi: 10.1186/s12711-018-0400-3
Tsuruta, S., Misztal, I., Aguilar, I., and Lawlor, T. J. (2011). Multiple-trait genomic evaluation of linear type traits using genomic and phenotypic data in US Holsteins. J. Dairy Sci. 94, 4198–4204. doi: 10.3168/jds.2011-4256
VanRaden, P. M. (2008). Efficient methods to compute genomic predictions. J. Dairy Sci. 91, 4414–4423. doi: 10.3168/jds.2007-0980
VanRaden, P. M., Van Tassell, C. P., Wiggans, G. R., Sonstegard, T. S., Schnabel, R. D., Taylor, J. F., et al. (2009). Invited review: reliability of genomic predictions for North American Holstein bulls. J. Dairy Sci. 92, 16–24. doi: 10.3168/jds.2008-1514
Wilson, D. E. (1992). Application of ultrasound for genetic improvement. J. Anim. Sci. 70, 973–983. doi: 10.2527/1992.703973x
Keywords: single-step GBLUP, genomic prediction, accuracy, ultrasound measurement, genetic correlation, Hanwoo
Citation: Mehrban H, Naserkheil M, Lee D and Ibáñez-Escriche N (2021) Multi-Trait Single-Step GBLUP Improves Accuracy of Genomic Prediction for Carcass Traits Using Yearling Weight and Ultrasound Traits in Hanwoo. Front. Genet. 12:692356. doi: 10.3389/fgene.2021.692356
Received: 08 April 2021; Accepted: 02 July 2021;
Published: 30 July 2021.
Edited by:
Fabyano Fonseca Silva, Universidade Federal de Viçosa, BrazilReviewed by:
Marielle Moura Baena, Universidade Federal de Lavras, BrazilHao Cheng, University of California, Davis, United States
Copyright © 2021 Mehrban, Naserkheil, Lee and Ibáñez-Escriche. This is an open-access article distributed under the terms of the Creative Commons Attribution License (CC BY). The use, distribution or reproduction in other forums is permitted, provided the original author(s) and the copyright owner(s) are credited and that the original publication in this journal is cited, in accordance with accepted academic practice. No use, distribution or reproduction is permitted which does not comply with these terms.
*Correspondence: Deukhwan Lee, ZGhsZWVAaGtudS5hYy5rcg==
†These authors have contributed equally to this work and share first authorship