- 1Heilongjiang Bayi Agricultural University, Daqing, China
- 2Heilongjiang Academy of Agricultural Sciences, Harbin, China
- 3Crop Resources Institute, Heilongjiang Academy of Agricultural Sciences, Harbin, China
- 4Keshan Branch, Heilongjiang Academy of Agricultural Sciences, Qiqihar, China
Uncovering the genetic architecture for grain yield (GY)–related traits is important for wheat breeding. To detect stable loci for GY-related traits, a genome-wide association study (GWAS) was conducted in a diverse panel, which included 251 elite spring wheat accessions mainly from the Northeast of China. In total, 52,503 single nucleotide polymorphisms (SNPs) from the wheat 55 K SNP arrays were used. Thirty-eight loci for GY-related traits were detected and each explained 6.5–16.7% of the phenotypic variations among which 12 are at similar locations with the known genes or quantitative trait loci and 26 are likely to be new. Furthermore, six genes possibly involved in cell division, signal transduction, and plant development are candidate genes for GY-related traits. This study provides new insights into the genetic architecture of GY and the significantly associated SNPs and accessions with a larger number of favorable alleles could be used to further enhance GY in breeding.
Background
Common wheat is the most important food crop worldwide and provides nearly 20% of the total caloric input to the global population. Grain yield (GY) improvement is one of the challenging goals in wheat breeding due to the complex genetic architecture and low heritability (He et al., 2010; Tester and Langridge, 2010; Gao et al., 2017; Wang et al., 2019). Heilongjiang and Jilin of China are the major spring wheat-producing regions, and yield potential in this region has been improved largely in the past decades (He et al., 2010; Zhou et al., 2014; Qin et al., 2015). However, the wheat production in this region is facing various threats, such as decreased groundwater, resulting in declining growing area, the irrigation frequency of wheat, and the bottleneck for yield potential of new cultivars through conventional breeding (He et al., 2010; Tester and Langridge, 2010).
Grain yield is a complex trait and influenced by many factors, particularly the genetic factors. GY-related traits included the spike number per unit area (SN), kernel number per spike (KNS), and thousand-kernel weight (TKW) (Ellis et al., 2005; Zhou et al., 2007; Rasheed et al., 2016; Gao et al., 2017; Li et al., 2018). Marker-assisted selection (MAS) is an effective tool for the further improvement of yield potential. Also, MAS is a key technique to increase the yield of wheat (He et al., 2010; Rasheed et al., 2016).
The effectiveness and reliability of MAS depends on the number of available genes and tightly linked markers for target traits. Until now, more than 70 genes have been cloned in wheat, among which 40 are associated with GY and related traits (Cui et al., 2014; Rasheed et al., 2016; Nadolska-Orczyk et al., 2017; Li et al., 2018; Wang et al., 2019). For all the cloned genes, about 150 functional markers or kompetitive allele-specific PCR (KASP) were developed (Rasheed et al., 2016). Besides this, more than 100 loci identified by genome-wide association study (GWAS) or biparental linkage mapping for GY-related traits are reported (Cui et al., 2014; Gao et al., 2017; Würschum et al., 2017; Li et al., 2018). However, identifying the novel genes or loci for GY is still important for wheat production.
Single nucleotide polymorphisms (SNP) provide an effective way to identify candidate genes for various traits (Zhu et al., 2008; Wang et al., 2014; Rasheed et al., 2016). Recently, the wheat 55, 90, and 660 K SNP arrays are gradually replacing simple sequence repeats (SSR) and diversity array technology (DArT) markers in genetic analysis in yield, disease resistance, end-use quality, and biotic or abiotic stress tolerance–related traits (Jin et al., 2016; Li et al., 2016; Liu et al., 2016, 2017; Valluru et al., 2017; Quan et al., 2021). Linkage analysis based on biparental populations and association mapping based on natural populations are two main ways to uncover the genetic analysis of complex traits (Zhu et al., 2008; Liu et al., 2017). Compared with linkage analysis, association mapping is based on linkage disequilibrium (LD) and offers an effective and reliable approach to uncover the genetic architecture of complex traits (Zhu et al., 2008; Liu et al., 2017). GWAS uses the natural germplasms, including wild types, landraces, released cultivars, and improved accessions, as materials and bypasses the time of developing biparental populations (Sela et al., 2014; Shi et al., 2017). Furthermore, traditional linkage analysis focuses on specific traits, whereas association mapping can be used to analyze various traits based on the same genotype data (Zhu et al., 2008). Nowadays, GWAS is commonly applied in genetic analysis of complex traits in wheat, such as GY-related traits, disease-related traits (stripe rust, leaf rust, or powdery mildew), and biotic and abiotic stress (Cui et al., 2011, 2014; Azadi et al., 2015; Beyer et al., 2019; Quan et al., 2021).
In China, Heilongjiang and Jilin are the main zones for spring wheat. In this study, 251 spring wheat accessions mainly originating from Heilongjiang and Jilin (1930–2020s) were selected. The aims of this study were to (1) detect the loci for GY and related traits in spring wheat, and (2) search for candidate genes for GY-related traits for further study.
Materials and Methods
Plant Materials and Field Trials
The diverse panel used in the present study contained 251 varieties mainly from Heilongjiang or Jilin province of China (Supplementary Table 1). The diverse panel was grown at Haerbin and Keshan in Heilongjiang province during the 2019 and 2020 cropping seasons. A randomized complete block design with three replicates was employed in field trials. Each plot comprised four 2.0-m rows spaced 20 cm apart with 40 seeds in each row. Agronomic management was performed according to local practices at each location.
Phenotyping and Statistical Analysis
Six phenotypic traits related to GY were evaluated in all four environments, including the GY, spike number per unit area (SNU), SN, spike length (SL), KNS, and TKW. The middle two lines of plants were harvested in each plot at physiological maturity, and GY was measured after the seed water content dried to 14% and was expressed as kg ha–1. The investigation of the other five traits and statistical analysis were according to Li et al. (2018). BLUP estimation for six traits among four environments was calculated using the MIXED procedure (PROCMIXED) in SAS v9.3 (SAS Institute)1 following the formula:
Of these, y is the observed phenotype, Xb is the fixed effects (environment), Zu is the random effect (genotype), and e is the residual effect.
Genotyping, Population Structure, and Linkage Disequilibrium
The 251 accessions were genotyped using the wheat 55 K SNP arrays. SNP markers with missing data > 20% and minor allele frequency (MAF) < 0.05 were removed to avoid spurious alleles. The physical position for subsequent GWAS analysis followed the IWGSC RefSeq v2.12.
Polymorphic and evenly distributed markers (one marker/LD block) were used to conduct population structure analysis. In this study, after filtering by MAF and missing data, 3000 polymorphic SNP markers (about 5 Mb for the whole genome of common wheat according to previous studies) evenly distributed on 21 chromosomes were analyzed in Structure v2.3.4 (Pritchard et al., 2000)3.
To verify the result, PCA and neighbor-jointing (NJ) trees were also estimated using the software Tassel v5.0 (Breseghello and Sorrells, 2006), respectively. After filtering using the Tassel v5.0, 5000 evenly distributed SNP markers were chosen to calculate LD for whole genomes using the full matrix and sliding window options in Tassel v5.0 (Breseghello and Sorrells, 2006). The details for population structure and LD decay analysis followed Liu et al. (2017). Variance components were used to calculate broad sense heritability (hb2) of GY-related traits as hb2 = σg2/(σg2 + σge2/r + σε 2/re), where σg2, σge2, and σε 2 represent the genotype, genotype × environment interaction, and residual error variances, respectively, and e and r are the numbers of environments and replicates per environment, respectively.
Genome-Wide Association Mapping
To control background variation and eliminate the spurious marker-trait associations (MTAs), the mixed linear model (MLM, PCA + K model) in Tassel v5.0 was used in consideration of the kinship matrix and population structure as follows:
Of these, y is the vector of observed phenotype, μ is the mean, x is the genotype, β is the effect of the SNP, u is the random effects due to genetic relatedness with Var (u) = σ2g K and Var (e) = σ2e, and K is the kinship matrix across all genotypes. The kinship matrix was treated as a random-effect factor and calculated by the software Tassel v5.0, whereas the PCA was considered as a fixed-effect factor and inferred by the Tassel V5.0 in MLM analysis. Due to Bonferroni–Holm correction for multiple testing (α = 0.05) being too conserved, and no significant MTAs were detected with this criterion, markers with an adjusted -log10 (P-value) ≥ 3.0 were regarded as significant markers. Manhattan plots and Q-Q plots were drawn based on R language (R 3.6.5).
The Identification of Candidate Genes
Loci existing in two or more environments are considered stable. To identify candidate genes, the flanking sequences corresponding to the SNP markers (including the SNPs located in the LD decay interval for peak markers) significantly associated with GY-related traits are used in BLASTn and BLASTx searches against NCBI4 and ENA databases. Also, the annotation information for IWGSC 2.1 was also used to identify candidate genes.
Results
Phenotypic Evaluation
Significant and continuous variations of GY and related traits were observed in the diverse panel (Supplementary Table 1 and Supplementary Figure 1). The mean values of SNU, SN, SL, KNS, TKW, and GY were 131.1 (88.7–177.9), 16.6 (12.9–20.7), 10.6 (6.8–13.8), 36.0 (23.1–50.6), 32.4 g (20.5–41.2 g), and 4623.5 kg.hm–1 (2233.0–6987.4 kg.hm–1). ANOVA showed highly significant effects (P < 0.01) of genotypes, environments, and genotype × environment interactions on all traits (Table 1) that were exhibited. The broad sense heritability (h2) for SNU, SN, SL, KNS, TKW, and GY were 0.64, 0.52, 0.65, 0.71, 0.63, and 0.49, respectively, which indicates most of these traits are stable and mainly determined by genetic factors.
In this study, GY showed significant (P < 0.01) and positive correlations with SNU, SN, SL, KNS, and TKW (r ranged from 0.18 to 0.75). SNU showed significant (P < 0.01) and positive correlations with GY (r = 0.18), whereas significant and negative correlations with SN, SL, KNS, and TKW (r ranged from 0.19 to 0.24); SN showed significant (P < 0.01) and positive correlations with SL, KNS, and TKW (r ranged from 0.10 to 0.82); SL exhibited significant (P < 0.01) and positive correlations with KNS and TKW (r ranged from 0.21 to 0.71) (Supplementary Table 2).
Marker Coverage and Genetic Diversity of the Physical Map
After filtering unqualified markers, 52,503 polymorphic SNPs were employed for construction of physical map and GWAS analysis (Supplementary Table 3 and Supplementary Figure 2). Among the polymorphic SNP markers, 34.9, 35.6, and 29.6% were from the A, B, and D genomes, respectively, indicating that the D genome has the lowest polymorphism (Supplementary Table 3). Of these, the chromosome 6B had more SNPs at 2691, whereas the 4D possessed only 1118 SNPs. The total length of the physical map is 14,058.8 Mb with an average marker density of 0.273 Mb per marker.
Population Structure and Linkage Disequilibrium
Population analysis indicates that all 251 wheat accessions were divided into three subgroups: subgroups I, II, and III. Of these, subgroup I contained 126 varieties mainly from Heilongjiang province ranging from the 1950s to 1980s, such as Kehong, Kehan 8, Kejian, and Xinshuguang 5; subgroup II had 75 varieties mainly from Heilongjiang province ranging from the 1990s to 2010s, such as Kechun 2, Kechun 9, Longmai 2, and Longmai 8; and subgroup III comprised 50 varieties mainly from Jilin province of China and foreign counties (United States, Canada, and Japan) (Figure 1). Furthermore, the average LD for the whole genome was 8 Mb (Supplementary Figure 3).
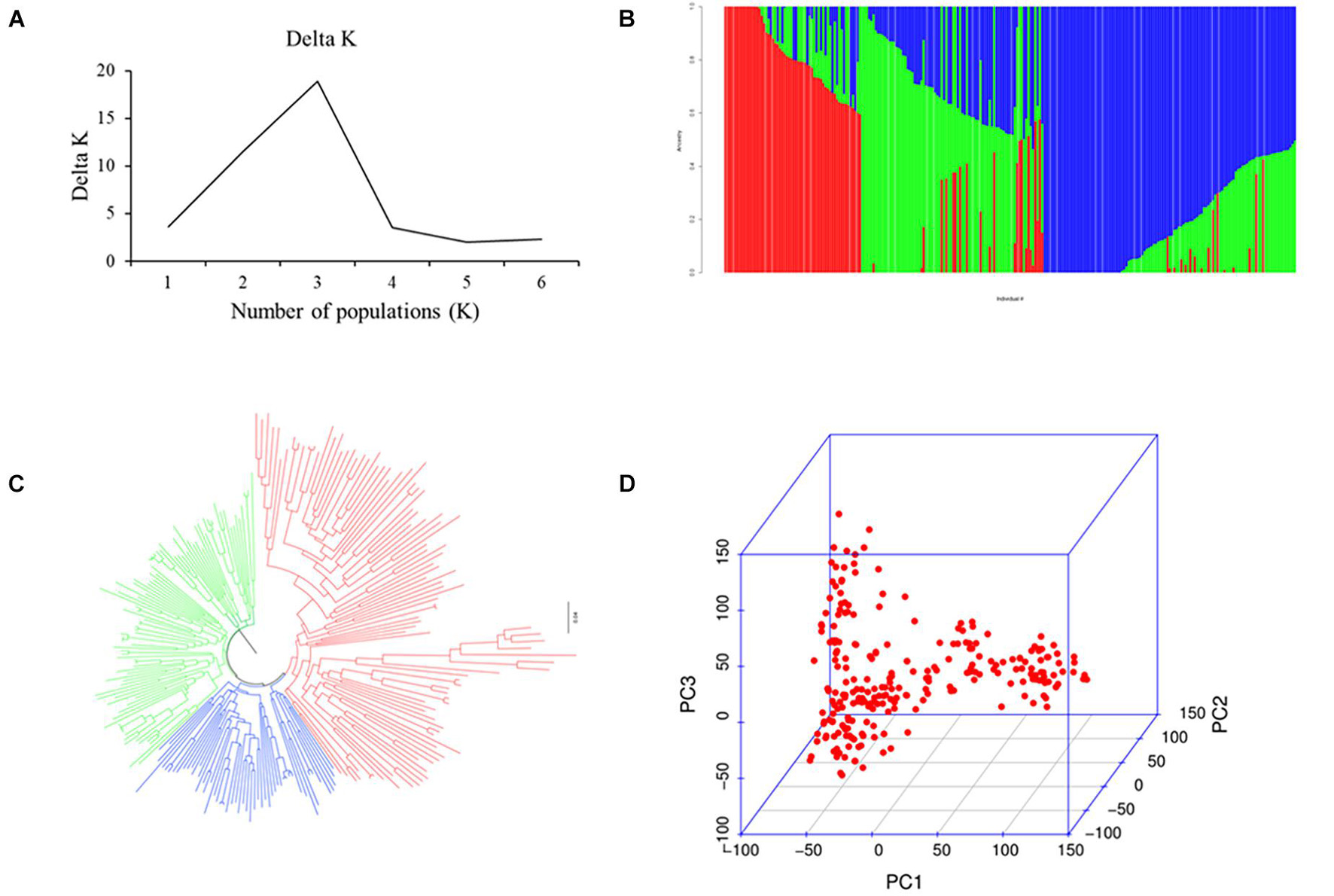
Figure 1. Population analysis for the 251 spring wheat accessions. (A) Delta K for structure analysis. (B) Population structure analysis. (C) Neighbor-joining (NJ) tree. (D) Principal components analysis (PCA) plots.
GWAS
In total, 38 loci (162 MTAs) were identified associated with GY-related traits in this study (Table 2, Figure 2, Supplementary Table 4, and Supplementary Figure 4). Among these, 6 (1D, 3D, 5A, 6A, 6D, and 7B), 7 (2D, 3A, 3B, 5A, and 6A), 21 (1A, 1D, 2D, 3A, 3B, 3D, 4A, 4B, 4D, 5A, 5B, 6A, 6B, and 6D), 7 (1B, 2B, 5A, 6B, and 7D), 5 (1A, 4A, 4B, 1A, 4A, 1A, and 4A), and 12 (2B, 3A, 3D, 5D, 6A, 6D, 3D, 4A, 1D, and 2A) loci were detected for SNU, SN, SL, KNS, TKW, and GY, respectively and each explaining 7.7–9.3% of the phenotypic variances. Of these loci, two on chromosome 3D (22.8 Mb) and 6D (482.3–482.6 Mb) showed pleiotropic effects on SNU and GY; a locus on chromosome 5A (517.5–529.3 Mb) controlled both the SL and SNU; four loci on chromosome 2D (23.2–37.9 Mb), 3A (10.9–21.9 and 595.6 Mb), and 5A (20.7 Mb) had pleiotropic effects on SL and SN. Besides this, five loci on chromosome 1A (28.6–30.7, 307.1–321.1, and 338.9–346.0 Mb), 4A (653.4–687.1 Mb), and 4B (15.8–17.3 Mb) had pleiotropic effects on SL and TKW; two loci on chromosome 1D (252.4–284.1 Mb) and 3D (600.0–600.4 Mb) had significant effects on GY and KNS; and a locus on chromosome 2B (592.8 Mb) controlled both the KNS and GY. Another locus on chromosome 6A (2.5–4.0 Mb) had pleiotropic effects on GY, SN, and SL, whereas the loci of 6B (132.4–133.0 Mb), showed pleiotropic effects on SL and KNS.
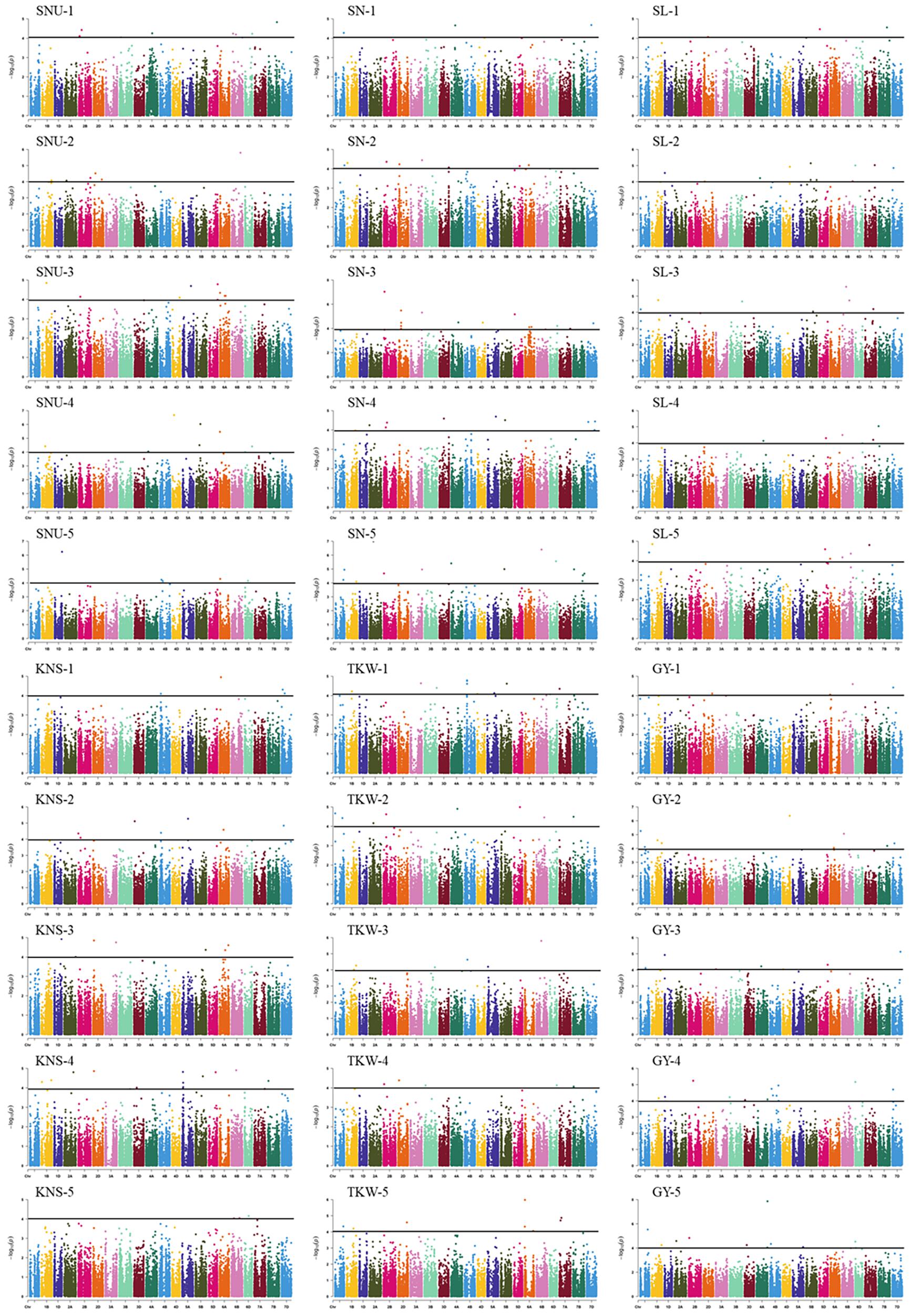
Figure 2. Manhattan plot for GY-related traits in 251 wheat accessions analyzed by the MLM in Tassel v5.0. SNU, spike number per unit area; SN, spikelet number; SL, spike length; KNS, kernel number per spike; TKW, thousand-kernel weight; GY, grain yield. The 1, 2, 3, 4, and 5 indicated the Haerbin, 2018, 2019; Keshan, 2018, 2019, and the best linear unbiased prediction (BLUP).
Of the loci identified in this study, 28 were identified in the BLUP. Besides this, five loci (249.7–250.3 Mb on 1D, 10.7–21.7 Mb on 3A, 640.8–652.0 Mb on 4A, 516.7–528.5 Mb on 5A, and 460.8–464.8 Mb on 6D) existed in two or more environments and could not be detected in the BLUP. Besides this, four loci (30.2 Mb on 2A, 544.2–544.9 Mb on 5D, 615.2 Mb on 6A, 291.1 Mb on 6D, and 580.8–581.0 Mb on 7B) with strong environmental specificity and only identified in specific environment. Also, we observed a new QTL at 536.4–536.8 Mb on chromosome 6A, which is only identified in BLUP and not observed by analyzing individual environments.
Candidate Genes
In this study, six candidate genes for GY traits were identified (Table 3). Two cytokinin ribosides (TraesCS2B02G397600 and TraesCS3B02G281000) were identified in the LD decay of the loci on 2B (592.8 Mb) and 3B (480.8–481.4 Mb). Another gene encoding an E3 ubiquitin transferase (TraesCS3A02G344600) was identified in the LD decay of the loci on chromosome 3A (596.0 Mb). For the loci on chromosome 3A (696.4–700.9 Mb) and 6A (2.5–4.0 Mb), candidate gene (TraesCS3A02G459800) for F-box proteins and serine/threonine-protein kinases (TraesCS7B02G328800) were identified, respectively. Besides this, the gene TraesCS6A02G301800 encoding trehalose 6-phosphatase (T6P) was identified as the candidate gene for the loci 7B (586.4 Mb).
Discussion
The 251 spring wheat accessions were divided into three subgroups (Figure 1), and the characterization of the subgroups was largely consistent with geographic origins, released years, and pedigrees. For example, most of the accessions from Heilongjiang province ranging from the 1950s to 1980s belonged to subgroup 1, the accessions from Heilongjiang province ranging from the 1990s to 2010s belonged to subgroup 2, and subgroup 3 mainly included the accessions from the Jilin province and foreign counties. A significant population structure existed in the diverse panel, and previous studies indicate that the lack of appropriate correction for population structure can lead to spurious MTAs (Zhu et al., 2008). Thus, to eliminate spurious MTAs, an MLM model with subpopulation data (Q) (fixed-effect factors) and kinship matrix (random-effect factor) were conducted. Also, the LD decay influenced several factors, such as population structure, allele frequency, recombination rate, and selection, and seriously affects the precision of association mapping. In this panel, the LD decay for the whole genome was about 8 Mb, consistent with previous reports (Liu et al., 2017), and indicates that the number of markers is enough for the subsequent association analysis.
Comparison With the QTL or Gene in Previous Studies
The genes or loci associated with GY-related traits were extensively reported previously. In this study, association of GY and related traits were performed based on the wheat high-density physical map. GY is a typical quantitative inheritance complex trait and significantly influenced by various environments (Gao et al., 2015; Li et al., 2018). The GY-related loci (gene) is distributed on all 21 chromosomes in wheat (Kumar et al., 2007; Reif et al., 2011; Lee et al., 2014; Lopes et al., 2015; Sukumaran et al., 2015; Gao et al., 2017; Li et al., 2018). Azadi et al. (2015) report a QTL for GY-related traits on chromosome 1A, which is tightly linked with the SSR marker gwm357 and located between the two GY QTL mapped by Cuthbert et al. (2008) and Huang et al. (2004). Also, a locus for KNS at chromosome 1A (around 26.8–40.5 Mb) is identified (Wang et al., 2011; Li et al., 2019). According to the IWGSC V2.0 and the consensus map by Maccaferri et al. (2015), the loci in 1A identified by Azadi et al. (2015) and Li et al. (2019) are overlapped with the 1A locus (28.6–30.7 Mb) for TKW and SL identified in the present study. Thus, these loci in 1A (AX-108817901 307.1–321.1 Mb) for TKW or SL (AX-109971512 338.9–346.0 Mb) for SL (AX-108850659 370.7–370.8 Mb) for SL and (AX-110363533 587.8–594.7 Mb) for SN were novel. Besides this, the loci for YM and NS identified in the 1B chromosome (AX-110953049 100.8–103.2 Mb) and two loci in 1D chromosome for YM and NS (AX-110953049 252.4–284.1 Mb) and SL (AX-94767476 430.1–430.3 Mb) may be novel.
Li et al. (2019) identified a locus for GY at chromosome 2A (33.3–34.9 Mb) and 3A (21.2–26.9 and 702.6–712.2 Mb) by association mapping in 166 wheat accessions. Also, we identified few loci for GY-related traits in chromosome 2A and 3A. Of these loci identified by Li et al. (2019), the locus at 2A (32.3–33.9 Mb) was overlapped with the QTL for GY at 2A (34.7 Mb) in this study; the locus at 3A (22.9–39.3 Mb) were nearly with the QTL for GY at 3A (10.9–21.9 Mb), whereas the locus at 3A (700.1–705.1 Mb) coincides with the locus for KNS on 3A (696.4–700.9 Mb) in our study. Besides this, Azadi et al. (2015) and Gao et al. (2015) identified a locus at the 3A chromosome nearly the 3A locus (696.4–700.9 Mb) identified in this study. On chromosome 3D, Li et al. (2019) identified a locus for KNS by GWAS in 166 common wheat accessions (574.8–576.8 Mb) and explained 7.1–9.9% of the phenotypic variations, which overlapped with the loci for NS and SL (3D, 600.0–600.4 Mb) identified in our study. The loci on chromosome 2B (AX-111470278 592.8 Mb) for SN and YM, 2D (AX-86163393 23.9–37.9 Mb) for SL and NSS, and AX-110090611 (556.2–556.3 Mb) for SL are likely to be new loci for GY-related traits. Besides this, the loci identified in chromosome 3A (AX-110922897 3A 596.0 Mb) for NSS and SL, 3B (AX-109379472 480.4–481.4 Mb) for NSS, AX-108791993 (589.9 Mb) for SL and 3D (AX-94879852 22.8 Mb) for NS and YM appear to be new. Also, the loci identified in chromosome 4A (AX-111662342 4A 640.2 Mb) for YM, AX-110577792 (653.4–687.1 Mb) for TKW and SL, 4B (AX-109901470 15.8–17.3 Mb) for SL and TKW, and 4D (AX-109294476 13.5 Mb) are new loci for GY-related traits.
The 5A chromosome enriched a series of loci for GY-related traits. Reif et al. (2011) identified a GY QTL on 5A linked with SSR marker barc151. Liu et al. (2014) also mapped a SL QTL on 5A. Li et al. (2018) identified a QTL QSL.caas-5AL.2 for SL in the Gaocheng 8901 × Zhou 8425B population. Besides this, Li et al. (2019) identified a locus for TKW by GWAS in 166 common wheat accessions at 5A (709.4–711.3) and explained phenotypic variations 8.2–13.0%. The locus for SL identified in this study at chromosome 5A (705.2 Mb) is overlapped with the loci discussed above. Azadi et al. (2015) detected a QTL for KNS on 5B, linked with DArT markers wPt-3661, whereas the 5B QTL is around the loci for SL (677.9–697.5 Mb) identified in the present study, and these might be the same. Also, the SL locus (677.9–697.5 Mb) on 5B is nearly the loci for KL, KW, and TKW detected by Chen et al. (2016); Mohler et al. (2016), and Sun et al. (2017), respectively, indicating that this should be an important locus in determining kernel weight. Li et al. (2019) identified a locus for TKW and SN by GWAS in 166 wheat accessions at 5B (696.5–699.7 Mb) and explained 8.2–13.0% of the phenotypic variations, which are nearly the loci at chromosome 5B identified in our study. Also, Li et al. (2019) detected a locus for TKW and SN by GWAS at 6D (482.3–486.3 Mb). We also have identified a locus for NS and GY on chromosome 6D (482.3–486.3 Mb). Thus, the loci on chromosome 5D (AX-110510530 547.6–548.3 Mb) for YM and 6A (AX-94478216 2.5–4.0 Mb) for YM, NSS, and SL and the loci (AX-110172686 538.9–539.3 Mb) for NS and (AX-109862690 619.4 Mb) for YM are likely to be new. The loci for GY-related traits identified in chromosome 6B (AX-111569068 132.4–133.0 Mb) for SL and SN, 6D (AX-94621559 8.6 Mb) for SL and SN, and AX-111694627 (312.8 Mb) for YM were novel. Besides this, we identified 3 loci on the 7B and 7D chromosomes for GY-related traits. The loci on chromosome 7B (AX-109321162 585.4–586.4 Mb) for NS and 7D (AX-111622533 21.9 Mb; AX-109984815 138.9–148.9 Mb) for SN appear to be new.
Among the 38 loci for GY and related traits, 10 loci discussed above (1A: 28.6–30.7 Mb, 2A: 30.2 34.7 Mb, 3A: 10.9–21.9 Mb, 3A: 696.4–700.9 Mb, 3D: 600.0–600.4 Mb, 5A: 10.3–20.7 Mb, 5A: 517.5–529.3 Mb, 5A: 702.6–705.2 Mb, 5B: 677.9–697.5 Mb, and 6D: 482.3–486.3 Mb) should be the same as the QTL reported in previous studies. The stable loci validated by both GWAS and QTL mapping between ours and previous studies indicate that they are widespread in varieties and may be more powerful and stable in various varieties. Moreover, the methods of GWAS used in the present study are proven to be reliable and efficient in detecting loci for GY and related traits.
Different QTL should be used in different regions. According to the results from association analysis, most of the loci (28) identified in this study existed on two or more environments (including BLUP), indicating high stability, and could be applied in various regions; these loci are only identified in an individual environment (30.2 Mb on 2A, 544.2–544.9 Mb on 5D, 615.2 Mb on 6A, 291.1 Mb on 6D, and 580.8–581.0 Mb on 7B) could be used in a specific environment, whereas those loci (536.4–536.8 Mb on chromosome 6A) only detected in BLUP may provide new insights into the genetic mechanism of GY-related traits.
Candidate Gene Analysis
To identify the candidate genes for GY-related traits, the flanking sequences of SNP markers (including the markers from the LD decay interval) significantly associated with GY-related traits were used as queries to BLAST against the National Center for Biotechnology Information (NCBI) and European Nucleotide Archive (ENA) databases. In total, six candidate genes were identified (Table 3) for further research. Of these, two cytokinin ribosides (TraesCS2B02G397600 and TraesCS3B02G281000) were identified in the LD decay of the loci on chromosome 2B (592.8 Mb) and 3B (480.4–481.4 Mb). The cytokinin is a positive regulator of shoot growth and negative regulator of root growth (Han et al., 2014) and has been strongly implicated in many aspects affecting GY-related traits, particularly the kernel number and size (Yamburenko et al., 2017). Besides this, cytokinin also plays crucial roles in the response to biotic and abiotic stressors (Cortleven et al., 2019). TraesCS3A02G344600, coded an E3 ubiquitin transferase, which were identified for the loci on chromosome 3A (596.0 Mb). Previous studies report that the E3-Ubiquitin protein ligases are a large protein family that is important in plant growth and development (Craig et al., 2009; Park et al., 2010). Wang et al. (2020) indicates that a E3 ligase gene, TaSDIR1-4A, contributes to the determination of grain size in common wheat. For the loci on chromosome 3A (696.4–700.9 Mb) and 6A (2.5–4.0 Mb), candidate genes for F-box proteins (TraesCS3A02G459800) and serine/threonine-protein kinases (TraesCS7B02G328800) were identified, respectively. F-box proteins are a large protein family and plays crucial roles in cell-cycle progression, transcriptional regulation, flower formation, signal transduction, and many other cellular processes in plants (Hong et al., 2012; Kaur et al., 2017; Marcotuli et al., 2018; Guérin et al., 2021). Furthermore, AX-108951749 on 2B and IWA2223 on 5AL encodes the serine/threonine-protein kinases. The serine/threonine-protein kinases play crucial roles in cell-cycle progression, transcriptional regulation, flower formation, and signal transduction (Rehman et al., 2019; Jia et al., 2020). The gene TraesCS6A02G301800 encodes trehalose 6-phosphatase (T6P) on the loci 7B (585.6 Mb), which regulates carbon assimilation and sugar status in plants. In addition, previous studies report that T6P has also been demonstrated to play an essential role in plant development under abiotic stress (Diab et al., 2013; Gahlaut et al., 2019).
Potential Implications in Wheat Breeding
The conventional breeding approach has led to improved GY on wheat, and breeding selection is time-consuming and not very efficient (He et al., 2010). Significant additive effects are reported between GY traits and a number of favorable alleles, indicating that pyramiding favorable alleles will improve GY traits (Li et al., 2018). The markers associated with GY detected should facilitate MAS. Loci with pleiotropic and consistent effects across each environment should be amenable to MAS. Besides this, the loci validated between ours and previous studies by QTL-mapping or GWAS indicated these loci are stable in various varieties and should be applied in further study. In this study, accessions with agronomic characters and more favorable alleles, such as Kefeng 6, Beimai 15, Kehan 18, Longfumai 6, Longfumai 196, Kechun 7, Longmai 23, Kechun 1, Longfumai 4, and Longmai 13, are recommended as parental lines for improvement of GY-related traits in wheat breeding.
Conclusion
In this study, association mapping for GY and related traits (SNU, SL, SN, KNS, and TKW) were conducted in a diverse panel, including 251 spring wheat varieties mainly from China. In total, 38 loci were identified, and each explained 6.5–16.7% of the phenotypic variations. Of these, 12 are overlapped with known genes or QTL, and 26 are likely to be new. Besides this, six candidate genes for GY-related traits involved in grain development, plant hormone signal transduction, and starch biosynthesis were identified. The stable loci and associated markers and varieties with favorable traits and alleles could be used in further wheat breeding. These new loci provide a new sight in genetic architecture of GY-related traits.
Data Availability Statement
The original contributions presented in the study are included in the article/Supplementary Material, further inquiries can be directed to the corresponding author/s.
Author Contributions
YL and LY designed the research. JT analyzed the physiology data. YL and WY drafted the manuscript. YL, YS, JC, and CT performed the experiment. HZ and LY revised the manuscript. All authors have read, edited, and approved the current version of the manuscript.
Funding
This work was funded by the “Key Projects of the Ministry of Science and Technology: Mutation Breeding of Main Crops” (2016YFD0102101). The Heilongjiang Agricultural Innovation Project (HNK2019CX04-06).
Conflict of Interest
The authors declare that the research was conducted in the absence of any commercial or financial relationships that could be construed as a potential conflict of interest.
Publisher’s Note
All claims expressed in this article are solely those of the authors and do not necessarily represent those of their affiliated organizations, or those of the publisher, the editors and the reviewers. Any product that may be evaluated in this article, or claim that may be made by its manufacturer, is not guaranteed or endorsed by the publisher.
Supplementary Material
The Supplementary Material for this article can be found online at: https://www.frontiersin.org/articles/10.3389/fgene.2021.728472/full#supplementary-material
Abbreviations
BLUE, best linear unbiased estimation; GY, grain yield; GWAS, genome-wide association study; h2, broad-sense heritability; KASP, kompetitive allele-specific PCR; KNS, kernel number per spike; LD, linkage disequilibrium; MAS, marker-assisted selection; QTL, quantitative trait loci; R2, phenotypic variance explained; RIL, recombinant inbred line; SL, spike length; SN, spike number per unit area; SNP, single nucleotide polymorphism; TKW, thousand-kernel weight.
Footnotes
- ^ http://www.sas.com
- ^ https://urgi.versailles.inra.fr/blast_iwgsc/blast.php
- ^ http://pritchardlab.stanford.edu/structure.html
- ^ http://www.ncbi.nlm.nih.gov/
References
Azadi, A., Mardi, M., Hervan, E. M., Mohammadi, S. A., Moradi, F., Tabatabaee, M. T., et al. (2015). QTL mapping of yield and yield components under normal and salt-stress conditions in bread wheat (Triticum aestivum L.). Plant Mol. Biol. Rep. 33, 102–120. doi: 10.1007/s11105-014-0726-0
Beyer, S., Daba, S., Tyagi, P., Bockelman, H., Brown-Guedira, G., and Mohammadi, M. (2019). Loci and candidate genes controlling root traits in wheat seedlings-a wheat root GWAS. Funct. Integr. Genomics 19, 91–107. doi: 10.1007/s10142-018-0630-z
Breseghello, F., and Sorrells, M. E. (2006). Association mapping of kernel size and milling quality in wheat (Triticum aestivum L.) cultivars. Genetics 172, 1165–1177. doi: 10.1534/genetics.105.044586
Chen, G. F., Zhang, H., Deng, Z. Y., Wu, R. G., Li, D. M., Wang, M. Y., et al. (2016). Genome-wide association study for kernel weight-related traits using SNPs in a Chinese winter wheat population. Euphytica 212, 173–185. doi: 10.1007/s10681-016-1750-y
Cortleven, A., Leuendorf, J. E., Frank, M., Pezzetta, D., Bolt, S., and Schmülling, T. (2019). Cytokinin action in response to abiotic and biotic stresses in plants. Plant Cell Environ. 42, 998–1018. doi: 10.1111/pce.13494
Craig, A., Ewan, R., Mesmar, J., Gudipati, V., and Sadanandom, A. (2009). E3 ubiquitin ligases and plant innate immunity. J Exp. Bot. 60, 1123–1132.
Cui, F., Li, J., Ding, A., Zhao, C., Wang, L., Wang, X., et al. (2011). Conditional QTL mapping for plant height with respect to the length of the spike and internode in two mapping populations of wheat. Theor. Appl. Genet. 122, 1517–1536.
Cui, F., Zhao, C., Ding, A., Li, J., Wang, L., Li, X., et al. (2014). Construction of an integrative linkage map and QTL mapping of grain yield-related traits using three related wheat RIL populations. Theor. Appl. Genet. 127, 659–675. doi: 10.1007/s00122-013-2249-8
Cuthbert, J. L., Somers, D. J., Brûlé-Babel, A. L., Brown, P. D., and Crow, G. H. (2008). Molecular mapping of quantitative trait loci for yield and yield components in spring wheat (Triticum aestivum L.). Theor. Appl. Genet. 117, 595–608. doi: 10.1007/s00122-008-0804-5
Diab, A. A., Nada, A. M., and Ashoub, A. (2013). Molecular cloning, expression, sequence analysis and in silico comparative mapping of trehalose 6-phosphate gene from Egyptian durum wheat. Int. J. Biotechnol. Mol. Biol. Res. 4, 9–23. doi: 10.5897/ijbmbr2012.0147
Ellis, M. H., Rebetzke, G. J., Azanza, F., Richards, R. A., and Spielmeyer, W. (2005). Molecular mapping of gibberellin-responsive dwarfing genes in bread wheat. Theor. Appl. Genet. 111, 423–430. doi: 10.1007/s00122-005-2008-6
Gahlaut, V., Jaiswal, V., Singh, S., Balyan, H. S., and Gupta, P. K. (2019). Multi-locus genome wide association mapping for yield and its contributing traits in hexaploid wheat under different water regimes. Sci. Rep. 9:19486.
Gao, F., Ma, D., Yin, G., Rasheed, A., Dong, Y., Xiao, Y., et al. (2017). Genetic progress in grain yield and physiological traits in Chinese wheat cultivars of Southern Yellow and Huai Valley since 1950. Crop Sci. 57, 760–773. doi: 10.2135/cropsci2016.05.0362
Gao, F., Wen, W., Liu, J., Rasheed, A., Yin, G., Xia, X., et al. (2015). Genome-wide linkage mapping of QTL for yield components, plant height and yield-related physiological traits in the Chinese wheat cross Zhou 8425B/Chinese Spring. Front. Plant Sci. 6:1099. doi: 10.3389/fpls.2015.01099
Guérin, C., Mouzeyar, S., and Roche, J. (2021). The landscape of the genomic distribution and the expression of the f-box genes unveil genome plasticity in hexaploid wheat during grain development and in response to heat and drought stress. Int. J. Mol. Sci. 22:3111. doi: 10.3390/ijms22063111
Han, Y., Zhang, C., Yang, H., and Jiao, Y. (2014). Cytokinin pathway mediates APETALA1 function in the establishment of determinate floral meristems in Arabidopsis. Proc. Natl. Acad. Sci. U.S.A. 111, 6840–6845. doi: 10.1073/pnas.1318532111
He, Z. H., Xia, X. C., and Bonjean, A. P. A. (2010). Wheat Improvement in China. Mexico: CIMMYT, 51–68.
Hong, M. J., Kim, D. Y., Kang, S. Y., Kim, D. S., Kim, J. B., and Seo, Y. W. (2012). Wheat F-box protein recruits proteins and regulates their abundance during wheat spike development. Mol. Biol. Rep. 39, 9681–9696. doi: 10.1007/s11033-012-1833-3
Huang, X. Q., Kempf, H., Ganal, M. W., and Röder, M. S. (2004). Advanced backcross QTL analysis in progenies derived from a cross between a German elite winter wheat variety and a synthetic wheat (Triticum aestivum L.). Theor. Appl. Genet. 109, 933–943. doi: 10.1007/s00122-004-1708-7
Jia, H., Li, M., Li, W., Liu, L., Jian, Y., Yang, Z., et al. (2020). A serine/threonine protein kinase encoding gene KERNEL NUMBER PER ROW6 regulates maize grain yield. Nat. Commun. 11:988.
Jin, H., Wen, W., Liu, J., Zhai, S., Zhang, Y., Yan, J., et al. (2016). Genome-wide QTL mapping for wheat processing quality parameters in a Gaocheng 8901/Zhoumai 16 recombinant inbred line population. Front. Plant Sci. 7:1032. doi: 10.3389/fpls.2016.01032
Kaur, S., Dhugga, K. S., Beech, R., and Singh, J. (2017). Genome-wide analysis of the cellulose synthase-like (Csl) gene family in bread wheat (Triticum aestivum L.). BMC Plant Biol. 17:193. doi: 10.1186/s12870-017-1142-z
Kumar, N., Kulwal, P. L., Balyan, H. S., and Gupta, P. K. (2007). QTL mapping for yield and yield contributing traits in two mapping populations of bread wheat. Mol. Breeding 19, 163–177. doi: 10.1007/s11032-006-9056-8
Lee, H. S., Jung, J. U., Kang, C. S., Heo, H. Y., and Park, C. S. (2014). Mapping of QTL for yield and its related traits in a doubled haploid population of Korean wheat. Plant. Biotechnol. Rep. 8, 443–454. doi: 10.1007/s11816-014-0337-0
Li, C., Bai, G., Chao, S., Carver, B., and Wang, Z. (2016). Single nucleotide polymorphisms linked to quantitative trait loci for grain quality traits in wheat. Crop J. 4, 1–11.
Li, F., Wen, W., He, Z., Liu, J., Jin, H., Cao, S., et al. (2018). Genome-wide linkage mapping of yield related traits in three Chinese bread wheat populations using high-density SNP markers. Theor. Appl. Genet. 131, 1903–1924. doi: 10.1007/s00122-018-3122-6
Li, F., Wen, W., Liu, J., Zhang, Y., Cao, S., He, Z., et al. (2019). Genetic architecture of grain yield in bread wheat based on genome-wide association studies. BMC Plant Biol. 19:168. doi: 10.1186/s12870-019-1781-3
Liu, G., Jia, L., Lu, L., Qin, D., Zhang, J., Guan, P., et al. (2014). Mapping QTLs of yield-related traits using RIL population derived from common wheat and Tibetan semi-wild wheat. Theor. Appl. Genet. 127, 2415–2432.
Liu, J., He, Z., Rasheed, A., Wen, W., Yan, J., Zhang, P., et al. (2017). Genome-wide association mapping of black point reaction in common wheat (Triticum aestivum L.). BMC Plant Biol. 17, 1–12.
Liu, J., He, Z., Wu, L., Bai, B., Wen, W., Xie, C., et al. (2016). Genome-wide linkage mapping of QTL for black point reaction in bread wheat (Triticum aestivum L.). Theor. Appl. Genet. 129, 2179–2190. doi: 10.1007/s00122-016-2766-3
Lopes, M. S., Dreisigacker, S., Peña, R. J., Sukumaran, S., and Reynolds, M. P. (2015). Genetic characterization of the wheat association mapping initiative (WAMI) panel for dissection of complex traits in spring wheat. Theor. Appl. Genet. 128, 453–464. doi: 10.1007/s00122-014-2444-2
Maccaferri, M., Ricci, A., Salvi, S., Milner, S. G., Noli, E., Martelli, P. L., et al. (2015). A high-density, SNP-based consensus map of tetraploid wheat as a bridge to integrate durum and bread wheat genomics and breeding. Plant Biotechnol. J. 13, 648–663. doi: 10.1111/pbi.12288
Marcotuli, I., Colasuonno, P., Blanco, A., and Gadaleta, A. (2018). Expression analysis of cellulose synthase-like genes in durum wheat. Sci. Rep. 8:15675.
Mohler, V., Albrecht, T., Castell, A., Diethelm, M., Schweizer, G., and Hartl, L. (2016). Considering causal genes in the genetic dissection of kernel traits in common wheat. J. Appl. Genet. 57, 467–476. doi: 10.1007/s13353-016-0349-2
Nadolska-Orczyk, A., Rajchel, I. K., Orczyk, W., and Gasparis, S. (2017). Major genes determining yield-related traits in wheat and barley. Theor. Appl. Genet. 130, 1081–1098. doi: 10.1007/s00122-017-2880-x
Park, Y. Y., Lee, S., Karbowski, M., Neutzner, A., Youle, R. J., and Cho, H. (2010). Loss of MARCH5 mitochondrial E3 ubiquitin ligase induces cellular senescence through dynamin-related protein 1 and mitofusin 1. J. Cell Sci. 123, 619–626.
Pritchard, J. K., Stephens, M., Rosenberg, N. A., and Donnelly, P. (2000). Association mapping in structured populations. Am. J. Hum. Genet. 67, 170–181. doi: 10.1086/302959
Qin, X., Zhang, F., Liu, C., Yu, H., Cao, B., Tian, S., et al. (2015). Wheat yield improvements in China: past trends and future directions. Field Crop. Res. 177, 117–124. doi: 10.1016/j.fcr.2015.03.013
Quan, X., Dong, L. J., Zhang, N., Xie, C., Li, H., Xia, X., et al. (2021). Genome-wide association study uncover the genetic architecture of salt tolerance-related traits in common wheat (Triticum aestivum L.). Front. Genet. 12:663941. doi: 10.3389/fgene.2021.663941
Rasheed, A., Wen, W., Gao, F., Zhai, S., Jin, H., Liu, J., et al. (2016). Development and validation of KASP assays for genes underpinning key economic traits in bread wheat. Theor. Appl. Genet. 129, 1843–1860. doi: 10.1007/s00122-016-2743-x
Rehman, S. U., Wang, J., Chang, X., Zhang, X., Mao, X., and Jing, R. (2019). A wheat protein kinase gene TaSnRK2.9-5A associated with yield contributing traits. Theor. Appl. Genet 132, 907–919. doi: 10.1007/s00122-018-3247-7
Reif, J. C., Maurer, H. P., Korzun, V., Ebmeyer, E., Miedaner, T., and Würschum, T. (2011). Mapping QTLs with main and epistatic effects underlying grain yield and heading time in soft winter wheat. Theor. Appl. Genet. 123, 283–292. doi: 10.1007/s00122-011-1583-y
Sela, H., Ezrati, S., Ben-Yehuda, P., Manisterski, J., Akhunov, E., Dvorak, J., et al. (2014). Linkage disequilibrium and association analysis of stripe rust resistance in wild emmer wheat (Triticum turgidum ssp. dicoccoides) population in Israel. Theor. Appl. Genet. 127, 2453–2463. doi: 10.1007/s00122-014-2389-5
Shi, W., Hao, C., Zhang, Y., Cheng, J., Zhang, Z., Liu, J., et al. (2017). A combined association mapping and linkage analysis of kernel number per spike in common wheat (Triticum aestivum L.). Front. Plant Sci. 8:1412. doi: 10.3389/fpls.2017.01412
Sukumaran, S., Dreisigacker, S., Lopes, M., Chavez, P., and Reynolds, M. P. (2015). Genome-wide association study for grain yield and related traits in an elite spring wheat population grown in temperate irrigated environments. Theor. Appl. Genet. 128, 353–363. doi: 10.1007/s00122-014-2435-3
Sun, C. W., Zhang, F. Y., Yan, X. F., Zhang, X. F., Dong, Z. D., Cui, D. Q., et al. (2017). Genome-wide association study for 13 agronomic traits reveals distribution of superior alleles in bread wheat from the Yellow and Huai Valley of China. Plant Biotechnol. J. 15, 953–969. doi: 10.1111/pbi.12690
Tester, M., and Langridge, P. (2010). Breeding technologies to increase crop production in a changing world. Science 327, 818–822.
Valluru, R., Reynolds, M. P., Davies, W. J., and Sukumaran, S. (2017). Phenotypic and genome-wide association analysis of spike ethylene in diverse wheat genotypes under heat stress. New Phytol. 214, 271–283.
Wang, J., Wang, R., Mao, X., Zhang, J., Liu, Y., Xie, Q., et al. (2020). RING finger ubiquitin E3 ligase gene TaSDIR1-4A contributes to determination of grain size in common wheat. J. Exp. Bot. 71, 5377–5388.
Wang, J. S., Liu, W. H., Wang, H., Li, L. H., Wu, J., Yang, X. M., et al. (2011). QTL mapping of yield-related traits in the wheat germplasm. Euphytica 177, 277–292. doi: 10.1007/s10681-010-0267-z
Wang, S. C., Wong, D., Forrest, K., Allen, A., Chao, S., Huang, B. E., et al. (2014). Characterization of polyploid wheat genomic diversity using a high-density 90000 single nucleotide polymorphism array. Plant Biotechnol. J. 12, 787–796.
Wang, Y., Hou, J., Liu, H., Li, T., Wang, K., Hao, C., et al. (2019). TaBT1, affecting starch synthesis and thousand kernel weight, underwent strong selection during wheat improvement. J. Exp. Bot. 70, 1497–1511. doi: 10.1093/jxb/erz032
Würschum, T., Langer, S. M., Longin, C. F. H., Tucker, M. R., and Leiser, W. L. (2017). A modern Green Revolution gene for reduced height in wheat. Plant J. 92, 892–903. doi: 10.1111/tpj.13726
Yamburenko, M. V., Kieber, J. J., and Schaller, G. E. (2017). Dynamic patterns of expression for genes regulating cytokinin metabolism and signaling during rice inflorescence development. PLoS One 12:e0176060. doi: 10.1371/journal.pone.0176060
Zhou, K. Q., Wang, G. D., Li, Y. H., Liu, X. B., Herbert, S. J., and Hashemi, M. (2014). Assessing variety mixture of continuous spring wheat (Triticum aestivum L.) on grain yield and flour quality in Northeast China. Int. J. Plant Prod. 8, 91–105.
Zhou, Y., He, Z. H., Sui, X. X., Xia, X. C., Zhang, X. K., and Zhang, G. S. (2007). Genetic improvement of grain yield and associated traits in the northern China winter wheat region from 1960 to 2000. Crop Sci. 47, 245–253. doi: 10.2135/cropsci2006.03.0175
Keywords: GWAS, marker-assisted selection, single nucleotide polymorphism, Triticum aestivum, grain yield
Citation: Li Y, Tang J, Liu W, Yan W, Sun Y, Che J, Tian C, Zhang H and Yu L (2021) The Genetic Architecture of Grain Yield in Spring Wheat Based on Genome-Wide Association Study. Front. Genet. 12:728472. doi: 10.3389/fgene.2021.728472
Received: 21 June 2021; Accepted: 30 August 2021;
Published: 15 November 2021.
Edited by:
Ahmed Sallam, Assiut University, EgyptReviewed by:
Karansher Singh Sandhu, Washington State University, United StatesMohamed El-Soda, Cairo University, Egypt
Copyright © 2021 Li, Tang, Liu, Yan, Sun, Che, Tian, Zhang and Yu. This is an open-access article distributed under the terms of the Creative Commons Attribution License (CC BY). The use, distribution or reproduction in other forums is permitted, provided the original author(s) and the copyright owner(s) are credited and that the original publication in this journal is cited, in accordance with accepted academic practice. No use, distribution or reproduction is permitted which does not comply with these terms.
*Correspondence: Hongji Zhang, ZnVtYWlAMTYzLmNvbQ==; Lihe Yu, eXVsaWhlMjAwMkAxMjYuY29t
†These authors have contributed equally to this work