- Radiation and Cancer Biology Laboratory, Radiation Oncology Center, Chongqing Key Laboratory of Translational Research for Cancer Metastasis and Individualized Treatment, Chongqing Cancer Hospital, Chongqing University Cancer Hospital and Chongqing Cancer Institution, Chongqing, China
Purpose: Radiation therapy (RT) is one of the main treatments for cancer. The response to radiotherapy varies widely between individuals and some patients have poor response to RT treatment due to tumor radioresistance. Stratifying patients according to molecular signatures of individual tumor characteristics can improve clinical treatment. In here, we aimed to use clinical and genomic databases to develop miRNA signatures that can predict response to radiotherapy in various cancer types.
Methods: We analyzed the miRNAs profiles using tumor samples treated with RT across eight types of human cancers from TCGA database. These samples were divided into response group (S, n = 224) and progressive disease group (R, n = 134) based on RT response of tumors. To enhance the discrimination for S and R samples, the predictive models based on binary logistic regression were developed to identify the best combinations of multiple miRNAs.
Results: The miRNAs differentially expressed between the groups S and R in each caner type were identified. Total 47 miRNAs were identified in eight cancer types (p values <0.05, t-test), including several miRNAs previously reported to be associated with radiotherapy sensitivity. Functional enrichment analysis revealed that epithelial-to-mesenchymal transition (EMT), stem cell, NF-κB signal, immune response, cell death, cell cycle, and DNA damage response and DNA damage repair processes were significantly enriched. The cancer-type-specific miRNA signatures were identified, which consist of 2-13 of miRNAs in each caner type. Receiver operating characteristic (ROC) analyses showed that the most of individual miRNAs were effective in distinguishing responsive and non-responsive patients (the area under the curve (AUC) ranging from 0.606 to 0.889). The patient stratification was further improved by applying the combinatorial model of miRNA expression (AUC ranging from 0.711 to 0.992). Also, five miRNAs that were significantly associated with overall survival were identified as prognostic miRNAs.
Conclusion: These mRNA signatures could be used as potential biomarkers selecting patients who will benefit from radiotherapy. Our study identified a series of miRNA that were differentially expressed between RT good responders and poor responders, providing useful clues for further functional assays to demonstrate a possible regulatory role in radioresistance.
1 Introduction
Radiotherapy (RT) plays a crucial role in cancer treatment and more than half of all cancer patients receive RT during the course of disease (Baskar et al., 2012). In last 20 years, the outcomes of RT have been improved dramatically due to the developments of highly conformal RT techniques, such as intensity-modulated RT (IMRT), intensity-modulated arc therapy (IMAT) and stereotactic RT (SRT) (Minniti et al., 2021). However, unfortunately the outcomes of therapy are not fully satisfactory. The response to radiotherapy varies widely between individuals and some patients are resistant to RT treatment. Radioresistance is considered as a main factor impeding efficacy of radiotherapy. Although radioresistance has been implicated to be associated with several biological alterations of the tumor cells, such as tumor metabolism (Tang et al., 2018), cell cycle arrest (Chen et al., 2017), oncogene and tumor suppressor alterations (Pitroda et al., 2009), microenvironment (TME) change (Suwa et al., 2021), autophagic regulation (Chang et al., 2014), cancer stem cells (CSCs) generation (Ning et al., 2013), and DNA damage response (DDR) and repair (Huang and Zhou, 2020; Sun et al., 2020), the mechanisms underlying resistance to radiation are still largely obscure. Therefore, uncovering the processes and charactering the molecules associated with regulation of radioresistance may lead to improved, efficient treatment for cancer patients.
Previous studies revealed that the difference in the status of mutation and expression profile of gene including microRNAs (miRNAs), is associated with radioresistance (Mercatelli et al., 2008; Huang et al., 2013; Mueller et al., 2013; Hatano et al., 2015; Mao et al., 2016; Xu et al., 2016; Tao et al., 2018; Xu et al., 2018). MicroRNAs are small non-coding single-stranded RNAs of 19–23 nucleotides in length, which play a critical role in post-transcriptional regulation by degrading or preventing the translation of their target messenger RNA (mRNA). They play important roles in tumor development and metastasis. Emerging evidences demonstrated that miRNAs may be involved in regulation processes associated with response to radiation. For examples, previous studies revealed that some microRNAs, such as miR-95 (Huang et al., 2013), miR-221, miR-222 (Mercatelli et al., 2008), and miR-106b (Hatano et al., 2015) enhanced radioresistance in cancer cells, while miR-30a (Xu et al., 2016), miR-16 (Tao et al., 2018), miR-449 (Mao et al., 2016), miR-17 (Xu et al., 2018), and miR-100 (Mueller et al., 2013) enhanced the radiosensitivity. Several miRNAs regulate DNA damage response (DDR) pathway. For example, miR-101 can regulate the expression of ataxia-telangiectasia mutated (ATM) gene and DNA-PK. ATM is a central regulator of DNA damage response (Maréchal and Zou, 2013). Mutation and inactivation of ATM can lead to increased instability of genome and impaired repair ability for DNA double-strand breaks. It has been experimentally demonstrated that up-regulation of miR-101 reduced the protein levels of DNA-PK and ATM, rendering tumor cells more sensitive to radiation (Yan et al., 2010). MiR-223 is a regulator of maturation and differentiation of hematopoietic stem cells. Up-regulation of miR-223 will reduce the expression of ATM and make U87 cells sensitive to radiation in vitro (Liang et al., 2014). MiR-375 is a negative regulator of p53 which is a key tumor suppressor gene that inhibits cell growth by activating cell cycle arrest or apoptosis (Vousden and Prives, 2009). Increase of miR-375 was detected in recurrent gastric cancer (Zhang et al., 2011), and the increased miR-375 interacts with the 3′UTR of p53 gene that negatively regulates p53 and its downstream pathway genes, resulting in radioresistance of cells to radiation (Liu et al., 2013).
In the era of precision medicine, a biomarker of intrinsic radiosensitivity would be extremely valuable for selecting patients in whom will benefit from RT, adjusting individual dosing, and aiding decision making. Previous studies have demonstrated that several miRNAs were associated with radioresistance or radiosensitivity, implying they could serve as promising biomarkers for prediction of RT response. Besides, miRNAs serving as biomarkers have several advantages. Firstly, unlike mRNAs, miRNAs remain largely intact in routinely collected, formalin-fixed, paraffin-embedded (FFPE) clinical tissues. Therefore, the detection for miRNA levels could be conveniently performed in clinical practice. Secondly, miRNAs have been called ‘the master regulators’ of gene expression since a single miRNA can regulate several hundreds of mRNA targets. Researches have indicated that many cell phenotype or subtype is likely governed by a miRNA regulatory network (Yang D. et al., 2013), suggesting some miRNAs may sever as the possible major determinants of cellular phenotype (including radioresistant phenotype) (McDermott et al., 2017). In additional, cell lines are usually used as models for radioresistance research in previous reports. However, tumors from patients are heterogeneous. Thus, the factors related to RT response are more complex than in cell lines. On the other hand, the results from cellular experiments also need to be confirmed with clinical samples. Therefore, we proposed that miRNA profile analysis based on clinical samples could provide a direct assessment for their performances as biomarkers for prediction of RT response. In this study, we performed a large-scale analysis of miRNA expression data collected from The Cancer Genome Atlas (TCGA) from those who treated with RT across eight cancer types. By analyzing the expressional difference between RT response and progressive disease samples, the miRNA signatures for predicting RT response were obtained and their performance for prediction of RT response were estimated.
2 Materials and methods
2.1 Data collection
MiRNA expression data of cancer patients undergoing RT were downloaded from the website UCSC XENA - GDC TCGA (https://xenabrowser.net/hub/). The expression profiles were presented as RPM (reads of exon model per million mapped reads). Clinical information including patient’s response to RT and overall survival was downloaded from GDC TCGA website (https://portal.gdc.cancer.gov/). The tumor samples were categorized into complete response (S) and progressive disease (R) groups depending on their clinical response to RT treatment. Complete response and progressive disease was defined according to RECIST. Finally, we analyzed 358 clinical samples across eight different cancer types, including S group (n = 224) and R group (n = 134).
2.2 Differential expression analysis
The differential expression analysis of miRNAs was performed in each cancer type. The miRNA would not be analyzed further if the reads of these miRNAs were empty in more than 10% of the samples. Deseq2 package was used to normalize miRNA data and identify the difference of miRNA expression levels between S and R group (Love et al., 2014). Wald test and t-test was used to calculate the p-value. In this study, p < 0.05 and | logfc | > 1 were used as threshold criteria for screening DEMs between S and R group.
2.3 Identification of DEMs
The identification of differentially expressed miRNA (DEMs) was conducted using R language. The expression levels of DEMs were visualized using the ggpubr, ggplot2 and complexheatmap packages. The receiver operating characteristic (ROC) curve was drawn and the area under the curve (AUC) was calculated using the pROC package. Heatmap of miRNA expression levels for individual cancer types was drawn using pheatmap. Kaplan Meier curve of single gene was drawn by Survminer package. The samples were divided into high expression group and low expression group according to the median expression level of miRNA and p-value was calculated by log-rank test. Enrichment analysis of the 47 DEMs was performed by the online tool TAM 2.0 (http://www.lirmed.com/tam2/).
2.4 Combinatorial models of multiple miRNAs
A logistic regression model was developed by combining expressions of multiple miRNAs in each cancer type. In our study, the dependent variable of this model was the normalized expression level of miRNA; the independent variable was the RT response of samples (response or progressive disease). The method of combinatorial modelling was described in detail (Xu et al., 2022). The samples were randomly divided into a training set and a test set. The training set contained 80% of the total samples, and the test set contained 20% of the total samples. The K-fold cross-validation (K = 5) was used to fit the combinatorial model. We used different combinations of the data groups that were partitioned to train and test K different models, and then the performance was evaluated. In running the final model, we found that the independent variable were the most accurate predictors of the dependent variable. The formula of logistic regression is as follows:
Y is the predictive index of RT response. The optimal threshold for dividing S and R groups in the training set was calculated, and then the test set was tested using the threshold. If the predictive index is more than the threshold, it indicates that the sample is more likely to be radiosensitive; otherwise, the sample is more likely to be radioresistant. Finally, the area under the receiver operating characteristic curve was calculated to evaluate the predictive ability.
3 Results
3.1 Clinical information of cancer patients
To investigate the association between RT response and miRNA profiles, we analyzed miRNA expression data of patients undergoing RT from TCGA database. A total of 358 clinical samples across eight different types of human cancers were analyzed in this study, including bladder urothelial carcinoma (BLCA, n = 23), esophageal carcinoma (ESCA, n = 47), lung adenocarcinoma (LUAD, n = 61), lung squamous cell carcinoma (LUSC, n = 38), pancreatic cancer (PAAD, n = 35), sarcoma (SARC, n = 56), skin cutaneous melanoma (SKCM, n = 34) and stomach adenocarcinoma (STAD, n = 64). The samples of cancer patients were categorized into complete response (S) group and progressive disease (R) group depending on their clinical response to RT treatment. Finally, 358 clinical samples were analyzed, including S group (n = 224) and R group (n = 134). The cancer types and groups of samples were shown in Figure 1A.
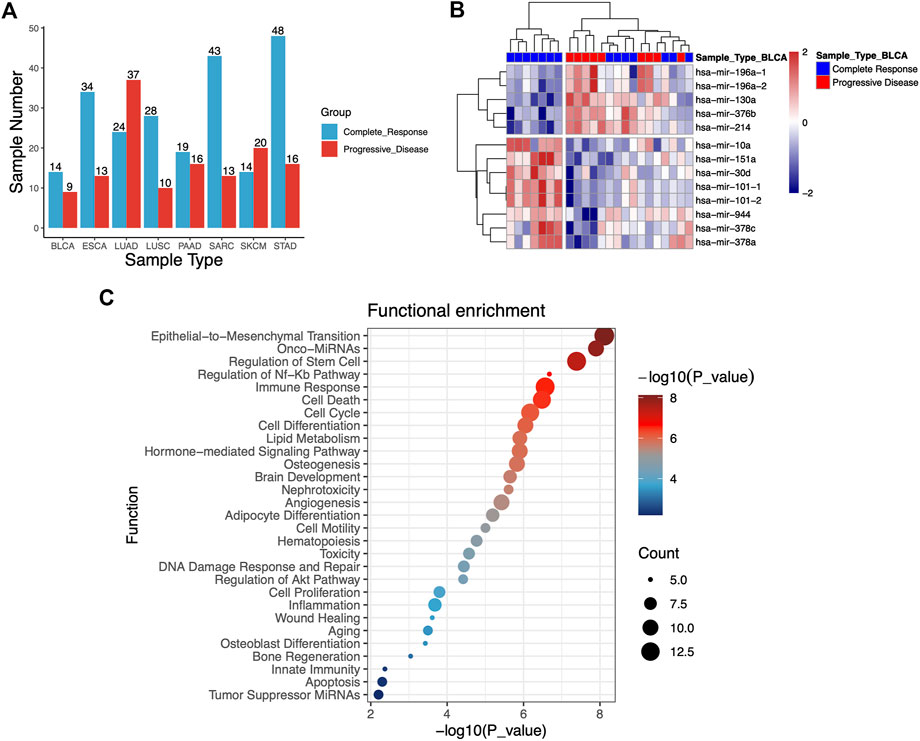
FIGURE 1. (A) Statistical histogram of cancer types of samples and grouping. The samples of cancer patients were categorized into complete response (S) group and progressive disease (R) group depending on their clinical response to RT treatment. A total of 358 tumor samples across eight different types of human cancers from TCGA database are analyzed. (B) Heatmap of 13 miRNAs that were differentially expressed from S and R samples in the bladder urothelial carcinoma (BLCA). (C) Functional enrichment analysis of 47 differentially expressed miRNAs.
3.2 Identification of DEMs in group S and R
To identify potential miRNA biomarkers, it is essential to compare miRNAs differentially expressed from RT response samples (S) and disease progression samples (R). According to the screening criteria of p-value <0.05 and | logfc | > 1, the miRNAs differentially expressed (DEMs) between the groups S and R in each caner type were identified. Each cancer type consisted of 2–13 of these miRNAs, and total 47 miRNAs were identified in eight cancer types (Table 1). Hierarchical unsupervised clustering analysis were performed to visualize the expression patterns of these 47 miRNAs. The results showed that these DEMs were cancer type-specific. For example, 13 miRNAs were identified in BLCA, five of them tended to be highly expressed in R group, including miR-196a-1 (p = 0.0048), miR-196a-2 (p = 0.005), miR-130a (p = 0.0163), miR-376b (p = 0.0078) and miR-30d (p = 0.0016), and eight miRNAs including miR-10a (p = 0.021), miR-151a (p = 0.013), miR-101-1 (p = 0.0007), miR-101-2 ((p = 0.0007), miR-944 (p = 0.049), miR-378c (p = 0.0153), miR-378a (p = 0.0158), and miR-214 (p = 0.0162) tended to be highly expressed in S (Figure 1B). Likewise, 4-miRNA signature was identified in LUSC. The high expression of miR-937 (p = 0.0154) was significantly enriched in R group, while the high expression of miR-592 (p = 0.0026), miR-3653 (p = 0.0214), miR-628 (p = 0.0222) and miR-106a (p = 0.0256) was significantly enriched in S group. The details were shown in Table 1.
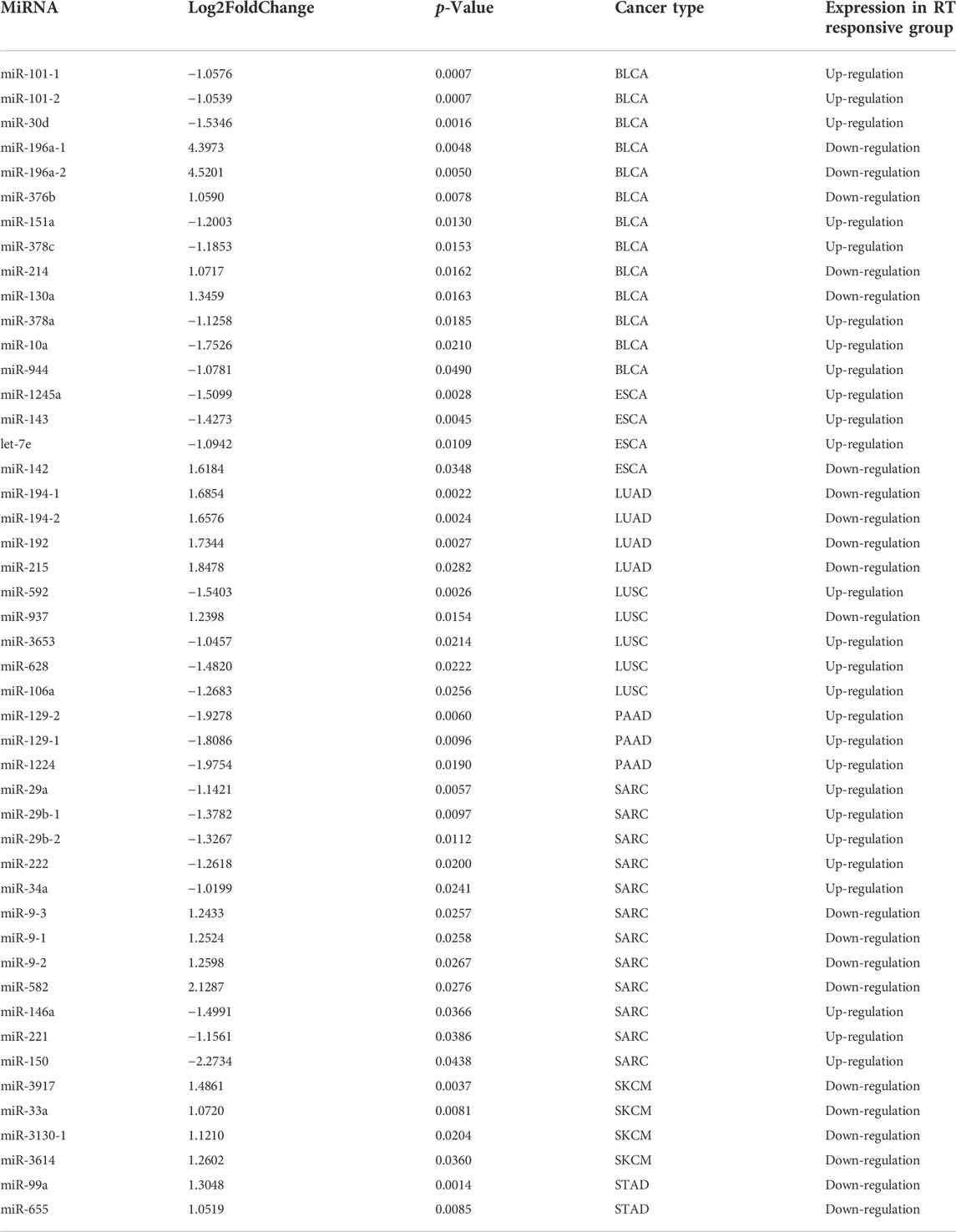
TABLE 1. The differentially expressed miRNAs (DEMs) between RT responsive group (S) and the progressive disease group (R).
Mapping the differentially expressed miRNAs to signal transduction pathways is important toward understanding their significance in radioresistance. Therefore, we performed enrichment analysis to identify significantly enriched terms. As shown in Figure 1C, the identified pathways were primarily involved in regulation of epithelial-to-mesenchymal transition and stem cell, NF-κB pathway, immune response, cell death and cell cycle. EMT plays a critical role not only in tumor metastasis but also in tumor radioresistance. Epithelial–mesenchymal transition could increase radioresistance (Zhang et al., 2014). We found that EMT signalling pathway to be among the top enriched pathways, implying that the radioresistant phenotype of tumour could be largely explained by the enhancement of EMT pathway. Activation of DNA damage response and DNA damage repair pathway has been demonstrated to be involved in radioresistance (Vousden and Prives, 2009; Yan et al., 2010; Liu et al., 2013; Liang et al., 2014). Enrichment analysis revealed that several DEMs including miR-34a, miR-146a, miR-130a, miR-196a, miR-143, and miR-101 were involved in DNA damage response and DNA damage repair. We also found that several miRNAs (miR-1224, miR-33a, and miR-142) were involved in inflammatory process which was implicated to be a critical radio-response in radioresistant lung cancer cells (Yang H. J. et al., 2013).
3.3 Distinguishing S and R group with a single miRNA
To evaluate the performance for distinguishing between S and R samples, we analyzed the specificity of each miRNA identified in DEMs list with the area under the curve (AUC). The expressed difference of individual miRNA for two groups was exhibited by boxplots. As shown in Figure 2, the expressed difference of individual miRNA was significant between RT responsive and non-responsive patients (p values <0.05, t-test). Particularly, miR-101-1 had the highest discrimination for the two groups in BLCA (p = 0.0007). Receiver operating characteristics (ROC) analyses were conducted to further assess the prediction performance of individual miRNA. As shown in Figure 3 several miRNAs had relatively high specificity for distinguish S and R samples, e.g., miR-101-1 (BLCA AUC = 0.889), miR-101-2 (BLCA, AUC = 0.889), miR-30d (BLCA, AUC = 0.889), miR-196a-1 (BLCA, AUC = 0.865), miR-196a-2 (BLCA, AUC = 0.865), and miR-592 (LUSC, AUC = 0.832). MiR-101 was known to be involved in the regulation of DNA damage repair and radiosensitivity. In our study, expression of miR-101 was up-regulated in RT responsive samples, hinting the high expression of miR-101 may be associated with radiosensitive phenotype. This is consistent with previous studies showing that up-regulation of miR-101 will enhance radiosensitivity (Yan et al., 2010). Our results implied that these miRNAs could be used as potential biomarkers for stratify patients treated with RT.
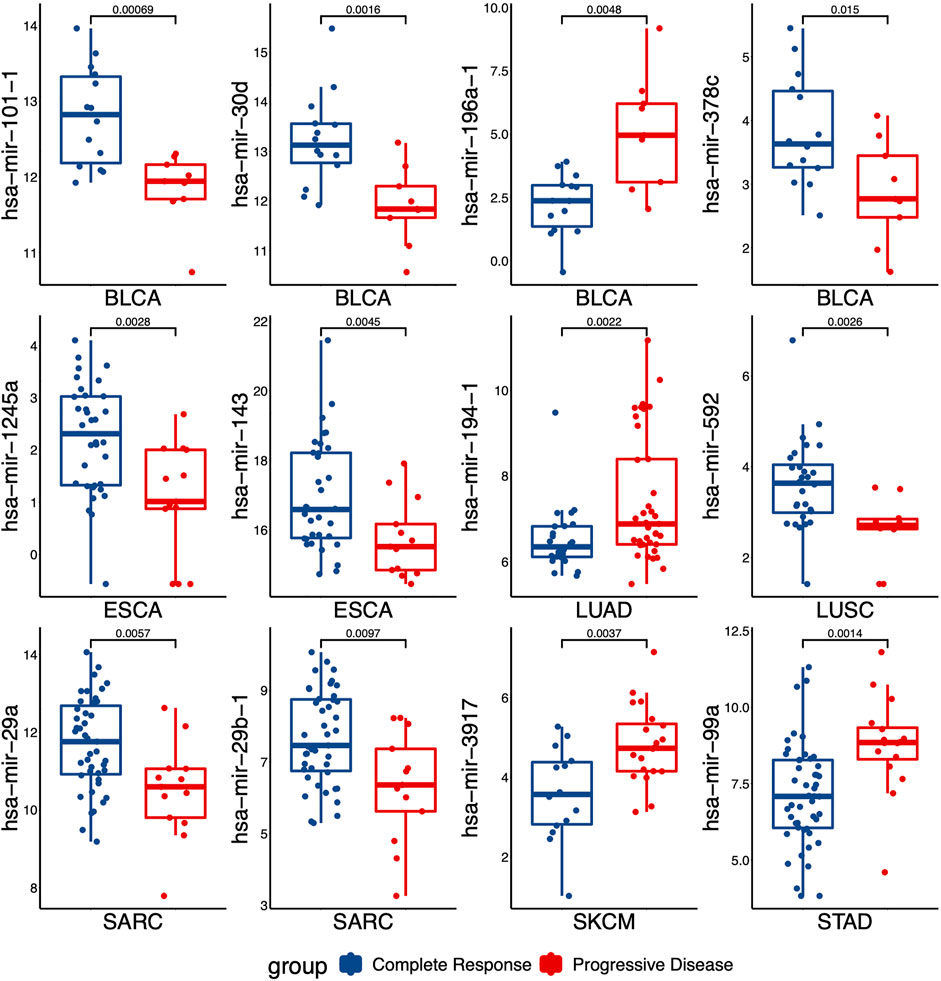
FIGURE 2. The levels of miRNAs expression that were differentially expressed between the responding (complete response) versus non-responding (progressive disease) tumors. p values were calculated by t-test.
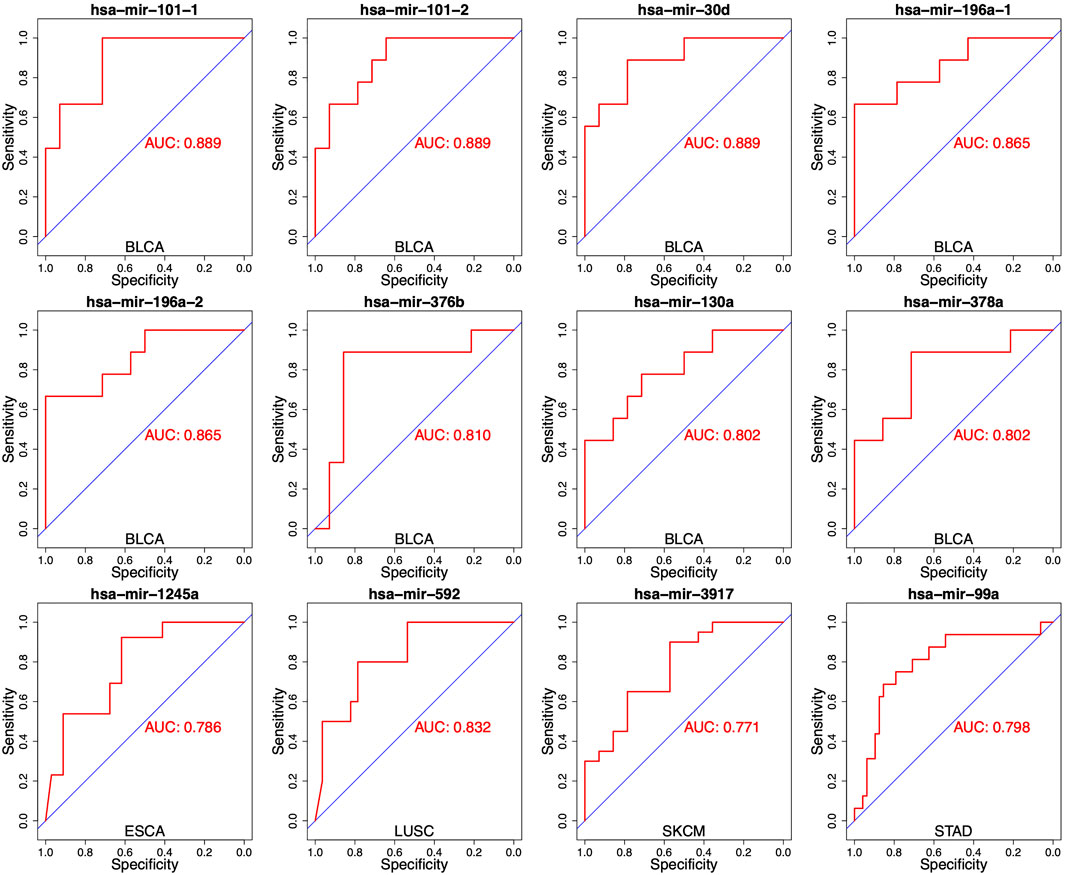
FIGURE 3. Receiver operating characteristics (ROC) analyses of the performance for the predictions for RT response using one single miRNA in different cancer types. The representative results were presented.
3.4 Using combinatorial model to predict RT response
Despite the most of differentially expressed miRNAs could be used to distinguish patients according to RT response (e.g., AUC ranging from 0.667 to 0.786 in ESCA), further improvement on discriminatory ability is still needed. Therefore, we developed the combinatorial models based on a binary linear regression model. The combinatorial models for each cancer type consisted of 2-13 miRNAs (Supplementary Table S1). By applying this model, the best combination of multiple miRNAs for RT response prediction in each cancer type was identified (Supplementary Table S1). As showed in Figure 4, the optimal cut-off value for distinguishing S and R samples was indicated by a dotted line, and the predictive index of each sample was calculated for classification. The results showed that the most of S samples were above the threshold line, while the R samples were below the threshold line, implying that the most samples could be correctly classified in both training set and test set. Receiver operating characteristic (ROC) analysis revealed that the predictive reliability was significantly increased in all caner types by using these combinatorial models (Figure 5). For example, the AUC of single miRNA in LUSC was between 0.664 and 0.832. The AUC value was improved to 0.879 in of LUSC by applying the combinatorial models. Likewise, the combinatorial model improved the AUC to 0.900 in ESCA. These results indicated that the combinatorial model could significantly improv the AUC relative to the single miRNA.
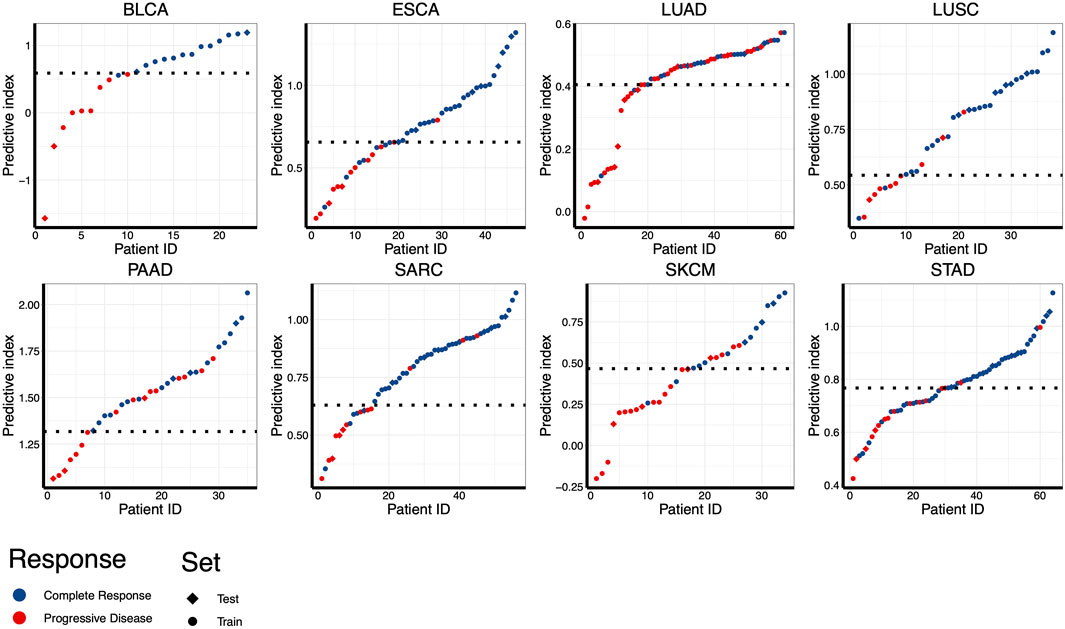
FIGURE 4. Assessing the performance for distinguishing RT responsive samples in eight different cancer types using combinatorial models. Predictive indexes were calculated to classify samples. Patients are shown in columns, and predictive index was showed in rows. The optimal cut-off value for distinguishing S and R samples was indicated by a dotted line. Samples of training set were indicated by circles, and samples of test set were indicated by diamonds.
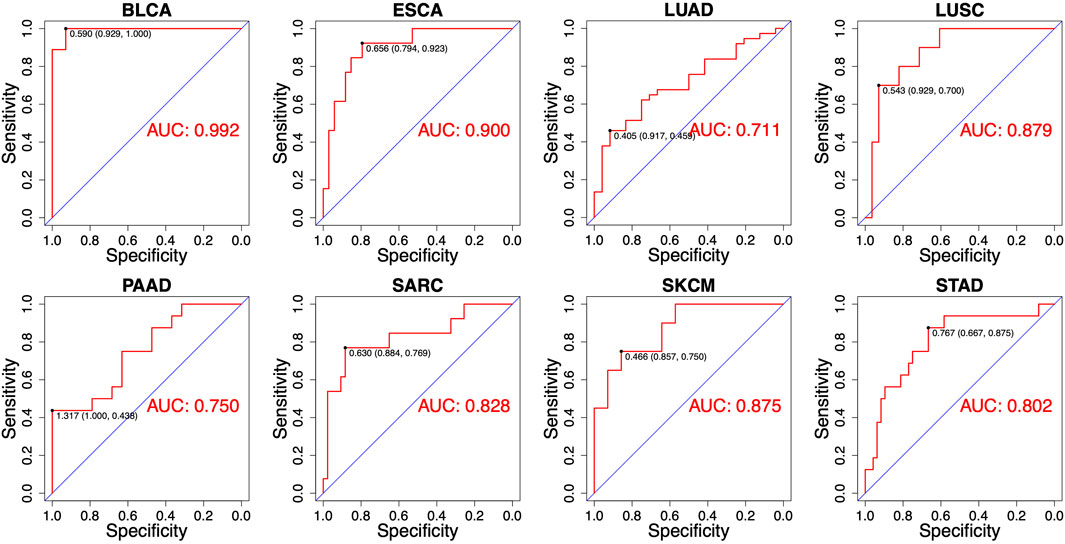
FIGURE 5. Receiver operating characteristics (ROC) analyses of the performance for the predictions for RT response using combinatorial models in eight different cancer types.
3.5 Evaluation of survival rate with single miRNA signature
We also explored the potential link between these differentially expressed miRNAs and the overall survival of cancer patients. We found that the expression level of five miRNAs, including miR-378a (p = 0.017, in BLCA), miR-142 (p = 0.018, ESCA), miR-655 (p = 0.03, in STAD), miR-29a (p = 0.025, in SARC), and miR-150 (p = 0.019, SARC), were significantly associated with the overall survival time (Figure 6). Among them, miR-378a, miR-29a, and miR-150 tended to highly expressed in S group. The patients with high expression of these miRNAs showed a trend of better survival. In contrast, miR-142 (p = 0.018, ESCA) and miR-655 (p = 0.03, STAD) tended to be highly expressed in R group, and the worse survival was observed in these high expression samples. The results showed that only five miRNAs of 47 miRNAs were predictive for patient survival. It is not surprised since the differentially expressed miRNAs were identified based on the response to RT treatment rather than the overall survival of cancer patients.
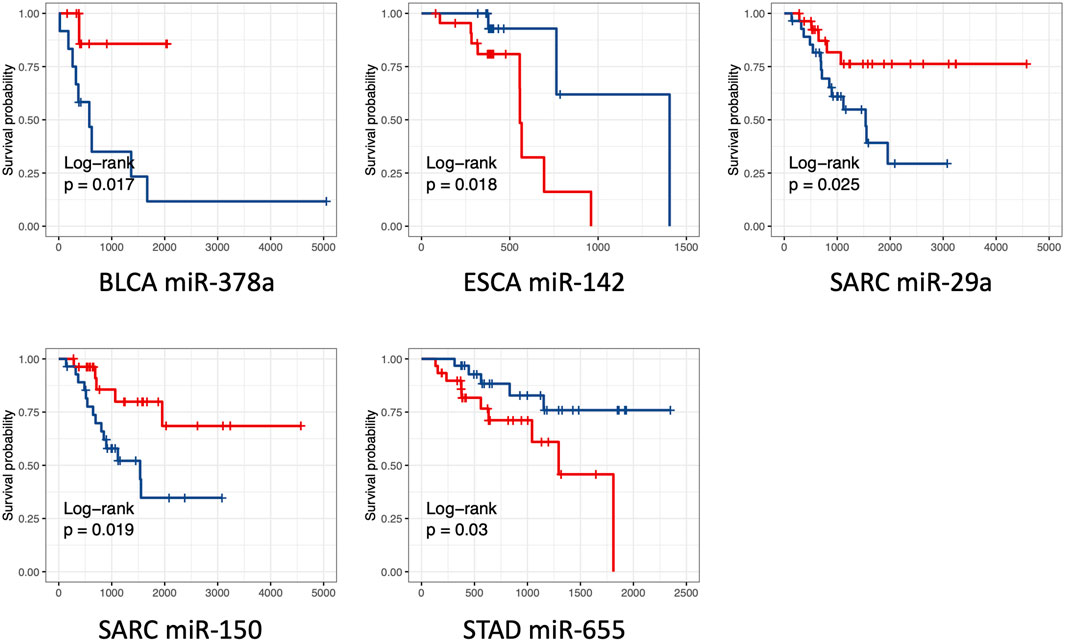
FIGURE 6. Kaplan Meier overall survival curves for patients with one single miRNA stratified by high versus low miRNA expression. Results from five miRNAs with low p values (p values <0.05) were shown. The high and low expression was indicated with red and blue color, respectively. p values were derived from log-rank test.
4 Discussion
Currently, only few biomarkers have been evaluated for their radiotherapy-specific predictive value. Several research groups have reported that miRNAs were involved in regulation of radioresistance, hinting they have the potential serving as biomarkers for prediction of RT response. However, most of them was identified based on cellular experiments in previous studies. The evaluation based on clinical samples should be performed. In this study, we evaluated the predictive value of miRNA signatures for predicting RT response by using data of clinical samples. Comparison of miRNA expression of radioresistant and radiosensitive tumors led to the identification of 47 miRNAs. Most of them showed to be predictive for RT response (AUC ranging from 0.606 to 0.889). Some of them showed the high specificity for the prediction. For instance, miR-101, miR-30d, and miR-196s has AUC of 0.889, 0.889, and 0.865, respectively. To further improve the prediction performance, we developed a combinatorial model in each cancer type. In these models, the best combinations of multiple miRNAs could be obtained, which leads to an improved discriminatory power. The results showed that the most of AUC in each cancer type was greater than 0.8 when the combinatorial models were applied. Particular, the AUC value reach to 0.992 by applying the combinatorial model in BLCA. The prediction models and miRNAs identified here have the potential clinical application. As these combinatorial models contained 2-13 miRNAs, it is convenient to develop digital PCR and real-time qPCR based clinical test in routine practice (Hindson et al., 2013).
Identifying genetic clues to the molecular basis of radioresistance is a major challenge. Our study identified a series of miRNA that were differentially expressed between RT good responders and poor responders, providing useful clues for further functional assays to demonstrate a possible regulatory role in radioresistance. Among DEMs, several miRNAs were known to be involved in the regulation of DNA damage repair or radiosensitivity. For example, our results showed that miR-34a was upregulated in S group and was downregulated in R group in SARC. MiR-34a is one of the family members of miR-34 (Misso et al., 2014), and is a key regulator of tumor suppression. The overexpression of miR-34a makes cells sensitive to radiation by inhibiting several targets of DNA damage repair pathway (Lacombe and Zenhausern, 2017), such as Bcl-2 (Liu et al., 2011), LyGDI (Duan et al., 2013), Notch-1 (Kang et al., 2013), Rad51 (Cortez et al., 2015). MiR-29a is a member of the miR-29 family (Patel and Noureddine, 2012), and miR-29 is a tumor suppressor that can promote cell senescence and differentiation (Martinez et al., 2011; Chuang et al., 2022). The studies found that overexpression of miR-29a enhanced radiosensitivity, and promoted apoptosis in radiation resistant CaSki and c33a cells (Martinez et al., 2011). Overexpression of miR-29a induces a significant decrease in cell migration speed through K-ras/c-raf/p38 signal pathway, and may reduce metastasis of lung cancer (Chuang et al., 2022). In this study, we found that miR-29a in SARC tended to be highly expressed in S group. MiR-214 involved in radioresistance have been demonstrated in multiple cancer types (Zhang and Zhang, 2017; Hu et al., 2018; Li et al., 2019). In ovarian cancer, the expression level of miR-214 rises after ionizing radiation, which activates P13K/Akt pathway by targeting PTEN, resulting in the increased radioresistance of cell lines (Zhang and Zhang, 2017). In colorectal cancer, miR-214 is significantly down-regulated in cells after ionizing radiation, which resulting in the increased sensitivity of cells to radiation (Hu et al., 2018). MiR-214 is overexpressed in osteosarcoma tissues and is a negative regulator of phlda2, maintaining radioresistance of osteosarcoma cells to apoptosis (Li et al., 2019). We found that miR-214 tended to be highly expressed in the R group, implying it may play a role in radioresistance in BLCA. Besides, miR-101, miR-146a, miR-196a, miR-143, miR-222 (Shi et al., 2019), and mir-130a (Ha Thi et al., 2019), has been reported to be involved in the regulation of DNA damage repair or radiosensitivity in previous studies.
As several miRNAs previously reported to be associated with radiotherapy sensitivity (e.g., miR-34, miR-101, miR-29a, miR-214, miR-146a, miR-196a, miR-143, miR-222, and mir-130a), we hypothesized that the signatures identified in here would identify additional radiotherapy sensitivity-related miRNAs. Our gene function enrichment analysis showed the many miRNAs were involved in EMT pathway. EMT is associated with characteristics of cancer stem cells, including radioresistance and chemoresistance. Several miRNAs were reported to directly target multiple key components of EMT pathway. For example, the miR-200 family inhibits EMT and tumor metastasis, inhibits self-renewal of cancer stem cells, and enhances radiosensitivity of several types of cancer (Baumann et al., 2008). Overexpression of miR-200c regulates oxidative stress response genes and increases radiosensitivity of lung cancer cells (Magenta et al., 2011). Zheng et al. found that the down-regulation of miR-200c was related to radiation tolerance in esophageal squamous cell carcinoma (Zheng et al., 2017). Zhang et al. found miR-205 can suppress EMT by targeting the EMT-inducing transcription factor ZEB1 (Zhang et al., 2014). Function enrichment analysis indicated that 14 miRNAs, including miR-34a, miR-215, miR-221, miR-30d, miR-194-2, miR-let-7, miR-194-1, miR-129-1, miR-29b-2, miR-29b-1, miR-29a, miR-192, miR-150, and miR-143, were associated with EMT pathway in our study. This enrichment implied that these miRNAs might be involved in radioresistant phenotype through EMT pathway. Previous studies reported that miR192 regulated the EMT pathway (Khella et al., 2013). Song et al. confirmed that miR-192 promoted EMT of gastric cancer, migration and invasion by targeting RB1 (Song et al., 2022). In additional, the previous study has demonstrated that miR-192 was significantly upregulated in cisplatin-resistant lung cancer cells, and miR-192 induced cisplatin resistance through activating the NF-κB pathway (Li et al., 2022). Moreover, miR-192 could influence 5-fluorouracil resistance (Boni et al., 2010). Cisplatin and 5-fluorouracil were genotoxic and their cytotoxic mode of action prominently involves the generation of DNA lesions followed by the activation of the DNA damage response and the induction of mitochondrial apoptosis. Zhai et al. found miR-143 suppressed epithelial–mesenchymal transition and inhibited tumor growth of breast cancer through down-regulation of ERK5 (Zhai et al., 2016). Up-regulating miR-143 enhances E-cadherin-mediated cell-cell adhesion ability, reduces mesenchymal markers, and decreases cell proliferation, migration, and invasion in vitro (Zhai et al., 2016). Yang et al. found that up-regulated miR-143 represses EMT in esophageal cancer cells (Yang et al., 2019). Our results showed that miR-143 was upregulated in S group and was downregulated in R group in esophageal cancer samples. We suggested that miR-143 might play a regulatory role in radiosensitivity through influencing EMT pathway in esophageal cancer. The further experiments will be required for function assay. We also noticed that miR-150 tended to be highly expressed in the RT response samples, implying it may play a role in radiosensitivity. Previous report showed that the expression of miR-150 decreased significantly in serum after irradiation in animal studies (Jia and Wang, 2022). Recently, miR-150-5p were confirmed to target ZEB1 and caused mRNA degradation, thus blocking EMT (Lu et al., 2017). Together, these multiple clues suggested that these miRNAs (miR-192, miR-143, and miR150) might serve as putative regulators of radiosensitivity through EMT pathway.
In conclusion, this work showed the miRNA signatures could serves as biomarkers to classify the RT response patients, and further investigations with larger numbers of patient samples are currently underway to validate the utility of using these biomarkers. Finally, additional work will be required to determine the role of these miRNAs in radioresistance of tumor.
Data availability statement
Publicly available datasets were analyzed in this study and are included in the article/Supplementary Material, further inquiries can be directed to the corresponding authors.
Author contributions
All authors listed have made a substantial, direct, and intellectual contribution to the work and approved it for publication.
Funding
This work was supported in part by projects (cstc2020jxjl130015, cstc2021jcyj-msxmX0767, cstc2019jcyj-msxmX0671, and cstc2020jcyj-msxmX1093), project (no. 82073347) from the National Natural Science Foundation of China, cstc2022ycjh-bgzxm0208, and the Integrated Innovation and Application of Key Technologies for Precise Prevention and Treatment of Primary Lung Cancer (no. 2019ZX002) from the Chongqing Municipal Health Committee.
Conflict of interest
The authors declare that the research was conducted in the absence of any commercial or financial relationships that could be construed as a potential conflict of interest.
Publisher’s note
All claims expressed in this article are solely those of the authors and do not necessarily represent those of their affiliated organizations, or those of the publisher, the editors and the reviewers. Any product that may be evaluated in this article, or claim that may be made by its manufacturer, is not guaranteed or endorsed by the publisher.
Supplementary material
The Supplementary Material for this article can be found online at: https://www.frontiersin.org/articles/10.3389/fgene.2022.1069112/full#supplementary-material
References
Baskar, R., Lee, K. A., Yeo, R., and Yeoh, K. W. (2012). Cancer and radiation therapy: current advances and future directions. Int. J. Med. Sci. 9 (3), 193–199. doi:10.7150/ijms.3635
Baumann, M., Krause, M., and Hill, R. (2008). Exploring the role of cancer stem cells in radioresistance. Nat. Rev. Cancer 8 (7), 545–554. doi:10.1038/nrc2419
Boni, V., Bitarte, N., Cristobal, I., Zarate, R., Rodriguez, J., Maiello, E., et al. (2010). miR-192/miR-215 influence 5-fluorouracil resistance through cell cycle-mediated mechanisms complementary to its post-transcriptional thymidilate synthase regulation. Mol. Cancer Ther. 9 (8), 2265–2275. doi:10.1158/1535-7163.MCT-10-0061
Chang, L., Graham, P. H., Hao, J., Ni, J., Bucci, J., Cozzi, P. J., et al. (2014). PI3K/Akt/mTOR pathway inhibitors enhance radiosensitivity in radioresistant prostate cancer cells through inducing apoptosis, reducing autophagy, suppressing NHEJ and HR repair pathways. Cell Death Dis. 5 (10), e1437. doi:10.1038/cddis.2014.415
Chen, Y., Li, Z., Dong, Z., Beebe, J., Yang, K., Fu, L., et al. (2017). 14-3-3σ contributes to radioresistance by regulating DNA repair and cell cycle via PARP1 and CHK2. Mol. Cancer Res. 15 (4), 418–428. doi:10.1158/1541-7786.MCR-16-0366
Chuang, P. C., Chen, P. T., Wang, C. C., Su, W. H., Chen, Y. H., and Huang, E. Y. (2022). MicroRNA-29a manifests multifaceted features to intensify radiosensitivity, escalate apoptosis, and revoke cell migration for palliating radioresistance-enhanced cervical cancer progression. Int. J. Mol. Sci. 23 (10), 5524. doi:10.3390/ijms23105524
Cortez, M. A., Valdecanas, D., Niknam, S., Peltier, H. J., Diao, L., Giri, U., et al. (2015). In vivo delivery of miR-34a sensitizes lung tumors to radiation through RAD51 regulation. Mol. Ther. Nucleic Acids 4 (12), e270. doi:10.1038/mtna.2015.47
Duan, W., Xu, Y., Dong, Y., Cao, L., Tong, J., and Zhou, X. (2013). Ectopic expression of miR-34a enhances radiosensitivity of non-small cell lung cancer cells, partly by suppressing the LyGDI signaling pathway. J. Radiat. Res. 54 (4), 611–619. doi:10.1093/jrr/rrs136
Ha Thi, H. T., Kim, H. Y., Kim, Y. M., and Hong, S. (2019). MicroRNA-130a modulates a radiosensitivity of rectal cancer by targeting SOX4. Neoplasia (New York, N.Y.) 21 (9), 882–892. doi:10.1016/j.neo.2019.07.005
Hatano, K., Kumar, B., Zhang, Y., Coulter, J. B., Hedayati, M., Mears, B., et al. (2015). A functional screen identifies miRNAs that inhibit DNA repair and sensitize prostate cancer cells to ionizing radiation. Nucleic Acids Res. 43 (8), 4075–4086. doi:10.1093/nar/gkv273
Hindson, C. M., Chevillet, J. R., Briggs, H. A., Gallichotte, E. N., Ruf, I. K., Hindson, B. J., et al. (2013). Absolute quantification by droplet digital PCR versus analog real-time PCR. Nat. Methods 10 (10), 1003–1005. doi:10.1038/nmeth.2633
Hu, J. L., He, G. Y., Lan, X. L., Zeng, Z. C., Guan, J., Ding, Y., et al. (2018). Inhibition of ATG12-mediated autophagy by miR-214 enhances radiosensitivity in colorectal cancer. Oncogenesis 7 (2), 16. doi:10.1038/s41389-018-0028-8
Huang, R. X., and Zhou, P. K. (2020). DNA damage response signaling pathways and targets for radiotherapy sensitization in cancer. Signal Transduct. Target. Ther. 5 (1), 60. doi:10.1038/s41392-020-0150-x
Huang, X., Taeb, S., Jahangiri, S., Emmenegger, U., Tran, E., Bruce, J., et al. (2013). miRNA-95 mediates radioresistance in tumors by targeting the sphingolipid phosphatase SGPP1. Cancer Res. 73 (23), 6972–6986. doi:10.1158/0008-5472.CAN-13-1657
Jia, M., and Wang, Z. (2022). MicroRNAs as biomarkers for ionizing radiation injury. Front. Cell Dev. Biol. 10, 861451. doi:10.3389/fcell.2022.861451
Kang, J., Kim, E., Kim, W., Seong, K. M., Youn, H., Kim, J. W., et al. (2013). Rhamnetin and cirsiliol induce radiosensitization and inhibition of epithelial-mesenchymal transition (EMT) by miR-34a-mediated suppression of Notch-1 expression in non-small cell lung cancer cell lines. J. Biol. Chem. 288 (38), 27343–27357. doi:10.1074/jbc.M113.490482
Khella, H. W., Bakhet, M., Allo, G., Jewett, M. A., Girgis, A. H., Latif, A., et al. (2013). miR-192, miR-194 and miR-215: a convergent microRNA network suppressing tumor progression in renal cell carcinoma. Carcinogenesis 34 (10), 2231–2239. doi:10.1093/carcin/bgt184
Lacombe, J., and Zenhausern, F. (2017). Emergence of miR-34a in radiation therapy. Crit. Rev. Oncol. Hematol. 109, 69–78. doi:10.1016/j.critrevonc.2016.11.017
Li, Y., Song, X., Liu, Z., Li, Q., Huang, M., Su, B., et al. (2019). Upregulation of miR-214 induced radioresistance of osteosarcoma by targeting PHLDA2 via PI3K/akt signaling. Front. Oncol. 9, 298. doi:10.3389/fonc.2019.00298
Li, Y., Zu, L., Wu, H., Zhang, F., Fan, Y., Pan, H., et al. (2022). MiR-192/NKRF axis confers lung cancer cell chemoresistance to cisplatin via the NF-κB pathway. Thorac. Cancer 13 (3), 430–441. doi:10.1111/1759-7714.14278
Liang, L., Zhu, J., Zaorsky, N. G., Deng, Y., Wu, X., Liu, Y., et al. (2014). MicroRNA-223 enhances radiation sensitivity of U87MG cells in vitro and in vivo by targeting ataxia telangiectasia mutated. Int. J. Radiat. Oncol. Biol. Phys. 88 (4), 955–960. doi:10.1016/j.ijrobp.2013.12.036
Liu, C., Zhou, C., Gao, F., Cai, S., Zhang, C., Zhao, L., et al. (2011). MiR-34a in age and tissue related radio-sensitivity and serum miR-34a as a novel indicator of radiation injury. Int. J. Biol. Sci. 7 (2), 221–233. doi:10.7150/ijbs.7.221
Liu, Y., Xing, R., Zhang, X., Dong, W., Zhang, J., Yan, Z., et al. (2013). miR-375 targets the p53 gene to regulate cellular response to ionizing radiation and etoposide in gastric cancer cells. DNA repair 12 (9), 741–750. doi:10.1016/j.dnarep.2013.06.002
Love, M. I., Huber, W., and Anders, S. (2014). Moderated estimation of fold change and dispersion for RNA-seq data with DESeq2. Genome Biol. 15 (12), 550. doi:10.1186/s13059-014-0550-8
Lu, W., Zhang, H., Niu, Y., Wu, Y., Sun, W., Li, H., et al. (2017). Long non-coding RNA linc00673 regulated non-small cell lung cancer proliferation, migration, invasion and epithelial mesenchymal transition by sponging miR-150-5p. Mol. Cancer 16 (1), 118. doi:10.1186/s12943-017-0685-9
Magenta, A., Cencioni, C., Fasanaro, P., Zaccagnini, G., Greco, S., Sarra-Ferraris, G., et al. (2011). miR-200c is upregulated by oxidative stress and induces endothelial cell apoptosis and senescence via ZEB1 inhibition. Cell Death Differ. 18 (10), 1628–1639. doi:10.1038/cdd.2011.42
Mao, A., Liu, Y., Wang, Y., Zhao, Q., Zhou, X., Sun, C., et al. (2016). miR-449a enhances radiosensitivity through modulating pRb/E2F1 in prostate cancer cells. Tumour Biol. 37 (4), 4831–4840. doi:10.1007/s13277-015-4336-8
Maréchal, A., and Zou, L. (2013). DNA damage sensing by the ATM and ATR kinases. Cold Spring Harb. Perspect. Biol. 5 (9), a012716. doi:10.1101/cshperspect.a012716
Martinez, I., Cazalla, D., Almstead, L. L., Steitz, J. A., and DiMaio, D. (2011). miR-29 and miR-30 regulate B-Myb expression during cellular senescence. Proc. Natl. Acad. Sci. U. S. A. 108 (2), 522–527. doi:10.1073/pnas.1017346108
McDermott, N., Meunier, A., Wong, S., Buchete, V., and Marignol, L. (2017). Profiling of a panel of radioresistant prostate cancer cells identifies deregulation of key miRNAs. Clin. Transl. Radiat. Oncol. 2, 63–68. doi:10.1016/j.ctro.2017.01.005
Mercatelli, N., Coppola, V., Bonci, D., Miele, F., Costantini, A., Guadagnoli, M., et al. (2008). The inhibition of the highly expressed miR-221 and miR-222 impairs the growth of prostate carcinoma xenografts in mice. PloS one 3 (12), e4029. doi:10.1371/journal.pone.0004029
Minniti, G., Niyazi, M., Alongi, F., Navarria, P., and Belka, C. (2021). Current status and recent advances in reirradiation of glioblastoma. Radiat. Oncol. 16 (1), 36. doi:10.1186/s13014-021-01767-9
Misso, G., Di Martino, M. T., De Rosa, G., Farooqi, A. A., Lombardi, A., Campani, V., et al. (2014). Mir-34: A new weapon against cancer? Mol. Ther. Nucleic Acids 3 (9), e194. doi:10.1038/mtna.2014.47
Mueller, A. C., Sun, D., and Dutta, A. (2013). The miR-99 family regulates the DNA damage response through its target SNF2H. Oncogene 32 (9), 1164–1172. doi:10.1038/onc.2012.131
Ning, X., Shu, J., Du, Y., Ben, Q., and Li, Z. (2013). Therapeutic strategies targeting cancer stem cells. Cancer Biol. Ther. 14 (4), 295–303. doi:10.4161/cbt.23622
Patel, V., and Noureddine, L. (2012). MicroRNAs and fibrosis. Curr. Opin. Nephrol. Hypertens. 21 (4), 410–416. doi:10.1097/MNH.0b013e328354e559
Pitroda, S. P., Wakim, B. T., Sood, R. F., Beveridge, M. G., Beckett, M. A., MacDermed, D. M., et al. (2009). STAT1-dependent expression of energy metabolic pathways links tumour growth and radioresistance to the Warburg effect. BMC Med. 7, 68. doi:10.1186/1741-7015-7-68
Shi, L., Wang, X., Hu, B., Wang, D., and Ren, Z. (2019). miR-222 enhances radiosensitivity of cancer cells by inhibiting the expression of CD47. Int. J. Clin. Exp. Pathol. 12 (11), 4204–4213.
Song, J., Lin, Z., Liu, Q., Huang, S., Han, L., Fang, Y., et al. (2022). MiR-192-5p/RB1/NF-κBp65 signaling axis promotes IL-10 secretion during gastric cancer EMT to induce Treg cell differentiation in the tumour microenvironment. Clin. Transl. Med. 12 (8), e992. doi:10.1002/ctm2.992
Sun, J., Zhu, Z., Li, W., Shen, M., Cao, C., Sun, Q., et al. (2020). UBE2T-regulated H2AX monoubiquitination induces hepatocellular carcinoma radioresistance by facilitating CHK1 activation. J. Exp. Clin. Cancer Res. 39 (1), 222. doi:10.1186/s13046-020-01734-4
Suwa, T., Kobayashi, M., Nam, J. M., and Harada, H. (2021). Tumor microenvironment and radioresistance. Exp. Mol. Med. 53 (6), 1029–1035. doi:10.1038/s12276-021-00640-9
Tang, L., Wei, F., Wu, Y., He, Y., Shi, L., Xiong, F., et al. (2018). Role of metabolism in cancer cell radioresistance and radiosensitization methods. J. Exp. Clin. Cancer Res. 37 (1), 87. doi:10.1186/s13046-018-0758-7
Tao, Z., Xu, S., Ruan, H., Wang, T., Song, W., Qian, L., et al. (2018). MiR-195/-16 family enhances radiotherapy via T cell activation in the tumor microenvironment by blocking the PD-L1 immune checkpoint. Cell. Physiol. biochem. 48 (2), 801–814. doi:10.1159/000491909
Vousden, K. H., and Prives, C. (2009). Blinded by the light: The growing complexity of p53. Cell 137 (3), 413–431. doi:10.1016/j.cell.2009.04.037
Xu, C. G., Yang, M. F., Fan, J. X., and Wang, W. (2016). MiR-30a and miR-205 are downregulated in hypoxia and modulate radiosensitivity of prostate cancer cells by inhibiting autophagy via TP53INP1. Eur. Rev. Med. Pharmacol. Sci. 20 (8), 1501–1508.
Xu, Z., Zhang, Y., Ding, J., Hu, W., Tan, C., Wang, M., et al. (2018). miR-17-3p downregulates mitochondrial antioxidant enzymes and enhances the radiosensitivity of prostate cancer cells. Mol. Ther. Nucleic Acids 13, 64–77. doi:10.1016/j.omtn.2018.08.009
Xu, Y., Tang, C., Wu, Y., Luo, L., Wang, Y., Wu, Y., et al. (2022). Prediction of response to radiotherapy by characterizing the transcriptomic features in clinical tumor samples across 15 cancer types. Comput. Intell. Neurosci. 2022, 5443709. doi:10.1155/2022/5443709
Yan, D., Ng, W. L., Zhang, X., Wang, P., Zhang, Z., Mo, Y. Y., et al. (2010). Targeting DNA-PKcs and ATM with miR-101 sensitizes tumors to radiation. PloS one 5 (7), e11397. doi:10.1371/journal.pone.0011397
Yang, D., Sun, Y., Hu, L., Zheng, H., Ji, P., Pecot, C. V., et al. (2013). Integrated analyses identify a master microRNA regulatory network for the mesenchymal subtype in serous ovarian cancer. Cancer Cell 23 (2), 186–199. doi:10.1016/j.ccr.2012.12.020
Yang, H. J., Kim, N., Seong, K. M., Youn, H., and Youn, B. (2013). Investigation of radiation-induced transcriptome profile of radioresistant non-small cell lung cancer A549 cells using RNA-seq. PloS one 8 (3), e59319. doi:10.1371/journal.pone.0059319
Yang, C., Shen, S., Zheng, X., Ye, K., Sun, Y., Lu, Y., et al. (2019). Long noncoding RNA HAGLR acts as a microRNA-143-5p sponge to regulate epithelial-mesenchymal transition and metastatic potential in esophageal cancer by regulating LAMP3. FASEB J. 33 (9), 10490–10504. doi:10.1096/fj.201802543RR
Zhai, L., Ma, C., Li, W., Yang, S., and Liu, Z. (2016). miR-143 suppresses epithelial-mesenchymal transition and inhibits tumor growth of breast cancer through down-regulation of ERK5. Mol. Carcinog. 55 (12), 1990–2000. doi:10.1002/mc.22445
Zhang, Q., and Zhang, S. (2017). miR-214 promotes radioresistance in human ovarian cancer cells by targeting PETN. Biosci. Rep. 37 (4), BSR20170327. doi:10.1042/BSR20170327
Zhang, X., Yan, Z., Zhang, J., Gong, L., Li, W., Cui, J., et al. (2011). Combination of hsa-miR-375 and hsa-miR-142-5p as a predictor for recurrence risk in gastric cancer patients following surgical resection. Ann. Oncol. 22 (10), 2257–2266. doi:10.1093/annonc/mdq758
Zhang, P., Wang, L., Rodriguez-Aguayo, C., Yuan, Y., Debeb, B. G., Chen, D., et al. (2014). miR-205 acts as a tumour radiosensitizer by targeting ZEB1 and Ubc13. Nat. Commun. 5, 5671. doi:10.1038/ncomms6671
Keywords: radiotherapy, radioresistance, miRNA expression, combinatorial model, TCGA databases, overall survival
Citation: Tang C, Qi J, Wu Y, Luo L, Wang Y, Wu Y and Shi X (2022) Improving the prediction for the response to radiotherapy of clinical tumor samples by using combinatorial model of MicroRNA expression. Front. Genet. 13:1069112. doi: 10.3389/fgene.2022.1069112
Received: 13 October 2022; Accepted: 11 November 2022;
Published: 22 November 2022.
Edited by:
Yin Yin, Nanjing Drum Tower Hospital, ChinaCopyright © 2022 Tang, Qi, Wu, Luo, Wang, Wu and Shi. This is an open-access article distributed under the terms of the Creative Commons Attribution License (CC BY). The use, distribution or reproduction in other forums is permitted, provided the original author(s) and the copyright owner(s) are credited and that the original publication in this journal is cited, in accordance with accepted academic practice. No use, distribution or reproduction is permitted which does not comply with these terms.
*Correspondence: Yongzhong Wu, Y3FtZHd5ekAxNjMuY29t; Xiaolong Shi, eHNoaS5iZWFyQGZveG1haWwuY29t
†These authors have contributed equally to this work