- 1Departments of Biotherapy, Tianjin Medical University Cancer Institute and Hospital, National Clinical Research Center for Cancer, Tianjin’s Clinical Research Center for Cancer, State Key Laboratory of Druggability Evaluation and Systematic Translational Medicine, Key Laboratory of Cancer Immunology and Biotherapy, Tianjin, China
- 2Departments of Radiology, Tianjin Medical University Cancer Institute and Hospital, National Clinical Research Center for Cancer, Tianjin’s Clinical Research Center for Cancer, State Key Laboratory of Druggability Evaluation and Systematic Translational Medicine, Key Laboratory of Cancer Prevention and Therapy, Tianjin, China
- 3Department of Diagnostic Radiology, Tianjin Cancer Hospital Airport Hospital, Tianjin, China
Purpose: To identify radiomic features from preoperative computed tomography (CT) images and characteristics of the tumor immune microenvironment (TIME) associated with anaplastic lymphoma kinase (ALK) rearrangement in patients with lung adenocarcinomas and their prognostic value in predicting recurrence or metastases after surgery.
Materials and methods: This retrospective study included 66 ALK-positive and 66 ALK-negative patients who underwent surgical resected lung adenocarcinoma. The number of CD8+ T cells and Human leukocyte antigen class I (HLA-I)/programmed death ligand 1 (PD-L1) expression were determined using immunohistochemistry. Radiomic features were extracted from the preoperative CT images. Combined radiomic, clinicopathological, and clinicopathological-radiomic models were built to predict ALK rearrangements. The models’ prediction performance was analyzed using receiver operating characteristic (ROC) curves with five-fold cross-validation. Prediction models for determining disease-free survival (DFS) of ALK-rearranged patients were developed, and the C-index after internal cross-validation was calculated to evaluate the performance of the models.
Results: HLA-I and PD-L1 expression were negatively associated with ALK rearrangement (both P < 0.001). The ROC curve indicated areas under the curve of 0.763, 0.817, and 0.878 for the radiomics, clinicopathology, and combined models in predicting ALK rearrangement, respectively. The combined model showed significant improvement compared to the clinicopathological (P = 0.02) and radiomics (P < 0.001) models alone. The validation C-indices were 0.752, 0.712, and 0.808 for the radiomic, clinicopathological, and combined models in predicting the DFS of ALK-rearranged patients, respectively. The combined model showed a significant improvement (P < 0.001) compared to the clinicopathological model alone.
Conclusion: This study demonstrated the potential role of radiomics and TIME characteristics in identifying ALK rearrangements in lung adenocarcinomas and the prognostic value of radiomics in predicting DFS in patients with ALK rearrangements.
1 Introduction
Anaplastic lymphoma kinase (ALK) rearrangements are driver mutations that occur in approximately 3%–7% of non-small cell lung cancer (NSCLC) cases, primarily in the lung adenocarcinoma subtype (Qin and Gadgeel, 2017; Smolarz et al., 2025). Compared with other tumor genotypes, ALK-rearranged tumors exhibit more invasive histomorphological features and aggressive behaviors (Kim et al., 2013). ALK rearrangements are an important target for NSCLC treatment, and patients harboring ALK rearrangements receive significant clinical benefits from ALK tyrosine kinase inhibitors (TKIs) (Shaw et al., 2013).
Although targeted therapies are effective in patients with ALK rearrangements, drug resistance and tumor recurrence inevitably occur (Rothenstein and Chooback, 2018). Immune checkpoint inhibitors (ICIs) that block the programmed death-1 (PD-1)–programmed death ligand 1 (PD-L1) axis have demonstrated remarkable therapeutic effects against NSCLC (Borghaei et al., 2015; Brahmer et al., 2015; Reck et al., 2016). However, studies have shown that patients harboring ALK rearrangements do not benefit from ICIs (Gainor et al., 2016; Mazieres et al., 2019; Jahanzeb et al., 2021). This may be associated with the unique tumor immune microenvironment (TIME).
Human leukocyte antigen class I (HLA-I) plays a pivotal role in tumor neoantigen presentation and CD8+ T cell activation. Some studies have suggested that activation of oncogenes inhibits HLA-I expression, thus promoting immune escape and thereby contributing to the poor efficacy of immunotherapy (Brea et al., 2016; Watanabe et al., 2019).
Although previous studies have reported increased tumor PD-L1 expression to be an unfavorable prognostic factor for NSCLC, the characteristics of the TIME and their prognostic values in ALK-rearranged NSCLC remain unclear (Zhang et al., 2017; Zhang et al., 2022; Tian et al., 2023; Zhou et al., 2023).
Through the extraction of high-throughput quantitative characteristics from medical images acquired during clinical practice, radiomics can offer insights into unique phenotypes resulting from the underlying biological processes of a tumor (Tomaszewski and Gillies, 2021). These radiomic features can noninvasively provide comprehensive information about the microenvironmental heterogeneity of tumors, and radiomics-based biomarkers have been widely used to predict clinical outcomes and potential genomic patterns (Chen et al., 2017). However, few studies have been conducted on the radiomic features of NSCLC with ALK rearrangement, and none have examined the association between radiomic features and disease-free survival (DFS) after surgery in this specific population (Ninatti et al., 2020; Ma and Li, 2021; Chen et al., 2024).
Patients with ALK-rearranged lung adenocarcinoma exhibit low response rates to ICIs, potentially due to distinct TIME characteristics. Radiomics, by noninvasively quantifying tumor heterogeneity, may improve early detection and risk stratification, thus complementing conventional pathology. Thus, in this study, we aimed to (I) identify the preoperative computed tomography (CT) radiomic features and TIME characteristics associated with ALK rearrangement in lung adenocarcinomas, (II) determine their potential value in predicting recurrence or metastasis after surgery in patients with ALK rearrangement, and (III) examine whether a combination of radiomic features and TIME characteristics could improve the performance of the predictive model. This multimodal analysis may aid in the early identification of ALK rearrangement and risk stratification for ALK-positive patients and provide a rationale and guidance for tailored therapy in the early stages.
2 Materials and methods
2.1 Study population
The institutional review board of Tianjin Medical University Cancer Institute and Hospital approved this retrospective study (Ethical approval No. EK20240091). Patients provided written informed consent prior to undergoing tests related to pathology, immune microenvironment, and ALK rearrangement status. This analysis included patients who underwent surgical resection for lung cancer in Tianjin Medical University Cancer Institute and Hospital between July 2016 and December 2019. Patients were consecutively included based on the following criteria: (I) Histologically confirmed lung adenocarcinoma with ALK rearrangement detected by Ventana D5F3 immunohistochemistry (IHC) in radical resection specimen, and (II) preoperative thin-slice CT images available on the Picture Archiving and Communication System conducted <1 month before surgery. Patients who had received chemotherapy or radiotherapy before surgery or those with other primary malignant tumors were excluded. Finally, 66 ALK-positive patients were included and 66 ALK-negative patients were randomly selected as controls (Supplementary Figure S1). Clinicopathological features, including sex, age, smoking history, and pathological TNM stage, were extracted from patients’ medical records. The tumors were histologically staged according to the eighth edition of the TNM classification system of the International Union Against Cancer and American Joint Committee on Cancer (Detterbeck et al., 2017). Patients were followed-up after surgery until December 2023. DFS was defined as the time from surgery to tumor recurrence or metastasis, which was confirmed by an investigator blinded to the predictor variables. Follow-up data were obtained from the medical records and telephone interviews. Among the ALK-positive patients, four received TKI treatment before tumor recurrence. Three patients without follow-up data were excluded from the prognostic analyses.
2.2 IHC
IHC staining was performed on paraffin-embedded sections of surgical samples using methods described previously (Mu et al., 2022). Primary antibodies included those against HLA-I (1:1,000; clone no. EMR8-5; cat. no. ab70328; Abcam, Cambridge, United Kingdom), PD-L1 (1:10,000; clone 2B11D11; cat. no. 66 248-1-Ig; Proteintech, Rosemont, IL, United States), and CD8 (1:10,000; clone no. 1G2B10; cat. no. 66 868-1-Ig; Proteintech).
Two pathologists who were blinded to the ALK status or clinical outcome independently evaluated all IHC images, and the final result was obtained by averaging the values from both pathologists. In this study, the H-score was used to evaluate the expression of HLA-I or PD-L1 on the cell membranes of tumor cells (Mu et al., 2022; Greeshma et al., 2023). The H-score was calculated as follows (Equation 1):
The number of CD8+ tumor-infiltrating lymphocytes (TILs), defined as CD8-positive cells regardless of the staining intensity, was recorded, and the density of TILs was determined by dividing the number of TILs by the total area of the observed fields (mm2) (Haratani et al., 2017). Five fields per section were randomly selected at ×200 magnification, avoiding necrotic and non-tumor regions, to calculate H-scores and CD8+ T cell density. The average values were then calculated.
2.3 CT imaging and radiomics feature extraction
Preoperative chest CT was performed using one of three multidetector CT systems: SOMOATOM Definition AS+ (Siemens Healthineers, Erlangen, Germany), LightSpeed 16 (GE Healthcare, Chicago, IL, United States), or Discovery CT750 HD (GE Healthcare). The scanning parameters were as follows: tube voltage, 120 kVp; tube current, 150–200 mA with automatic exposure control; reconstruction thickness and interval, 1.5 or 1.25 mm; mediastinal window reconstruction kernel, B30f/Standard; and lung window reconstruction kernel, B70f/lung.
Tumor segmentation was performed by a radiologist with 5 years of experience in thoracic CT diagnosis using a semiautomatic method, and reviewed by another radiologist with 16 years of experience. In addition to lung cancer diagnosis, the radiologists were unaware of clinical data and pathological information. 3D Slicer V5.1.0 (Fedorov et al., 2012) was used to segment tumors on unenhanced images using the B70f/lung reconstruction kernel. The B70f/lung kernel was selected because of its high resolution in capturing tumor edges and internal structures in the lung window, which is ideal for radiomic analysis. Three-dimensional (3D) radiomic features were extracted.
Finally, 851 features were extracted from the tumor CT images, as described in a previous study (Wang et al., 2022), including 14 shape features, 18 first-order features, 75 texture features [24 Gy-level co-occurrence matrix (GLCM), 14 Gy-level dependence matrix (GLDM), 16 Gy-level run-length matrix (GLRLM), 16 Gy-level size-zone matrix (GLSZM), and 5 neighboring gray-tone difference matrix (NGTDM)], and 744 wavelet-based features.
2.4 Feature selection and model development
2.4.1 Models for predicting ALK rearrangement
Three models (radiomics, clinicopathological, and clinicopathological-radiomics combined) were developed separately to predict ALK rearrangement.
For radiomic model development, preliminary screening was performed using univariate logistic regression, and statistically significant features were further screened using the least absolute shrinkage and selection operator (LASSO) regression methods. Ten-fold cross-validation was applied to select the penalty parameter (λ) of LASSO via minimum criteria to retain features with nonzero coefficients. Finally, multivariate logistic regression was performed using a forward stepwise strategy to select the most informative variables in a single parsimonious model. The radiomics score (Rad-score) for each patient was calculated as a linear combination of the selected features weighted by their regression coefficients (beta values).
To build the clinicopathological model, univariate logistic regression analysis was performed, and statistically significant variables were included in a multivariate logistic regression model. A combined model was eventually developed by incorporating the independent predictive variables in the clinicopathological model and Rad-score into the multivariate logistic regression analysis.
The prediction performance of the models was analyzed using receiver operating characteristic (ROC) curves, and each model was cross-validated with five-fold cross-validation to ensure a robust area under the curve (AUC) estimate, given the limited sample size. Differences in the AUC between the models were compared using the DeLong test. Model stability was evaluated via the coefficient of variation (CV) of the AUCs derived from 500 bootstrap iterations (CV = standard deviation/mean).
2.4.2 Models for predicting the DFS of patients with ALK rearrangement
Three models (radiomics, clinicopathological, and clinicopathological-radiomics combined) were developed separately to predict the DFS of patients with ALK rearrangements.
For the radiomics model, univariate Cox proportional hazards regression analysis was performed, and statistically significant features were subjected to LASSO Cox regression. Features with nonzero coefficients selected by 10-fold cross-validation were included in the backward stepwise Cox regression analysis to identify independent prognostic variables and to build the final model. A radiomics risk score (RAD-risk score) was calculated for each patient via a linear combination of selected features weighted by their regression coefficients (beta values). Patients with ALK rearrangements were divided into two risk groups based on the median RAD-risk score, which served as the cutoff point.
Clinicopathological variables that were significantly associated with DFS were identified using univariate and multivariate Cox regression analyses to construct a clinicopathological model. The combined model was finally developed by adding the RAD-risk score to the clinicopathological model in the multivariate analysis.
The Harrel concordance index (C-index) was used to assess the model performance. Owing to the limited sample size, five-fold cross-validation was used to ensure a robust C-index estimate. The 95% confidence intervals (CIs) for the C-index were calculated by bootstrap resampling (500 replicates). Differences in the C-index between models were assessed using a likelihood ratio test. Model stability was assessed through the CV of the C-indices from 500 bootstrap iterations.
2.5 Statistical analyses
Differences between ALK-positive and ALK-negative patients in terms of HLA-I/PD-L1 H-score, CD8+ T-cell density, and other clinicopathological features were evaluated using the Chi-squared test for categorical variables and the Mann–Whitney U test for continuous variables.
To further elucidate the biological relevance of the radiomic signature, the association of the calculated Rad-score and RAD-risk score with clinicopathological features was analyzed using the Spearman correlation test for continuous variables and the Mann–Whitney U test for categorical variables.
Statistical analyses were performed using R version 4.3.2 (The R Foundation for Statistical Computing), Python version 3.12 (Python Software Foundation, Wilmington, DE, United States), and SPSS version 27.0 (IBM Corp., Armonk, NY, United States). Differences were considered statistically significant at P < 0.05.
3 Results
3.1 Clinicopathological features stratified by ALK rearrangement status
The distribution of clinicopathological features according to ALK rearrangement status is presented in Table 1. The HLA-I and PD-L1 H-scores were significantly lower (both P values < 0.001) in patients with ALK rearrangements than in those without ALK rearrangements. There were no significant differences in sex, age, smoking history, T stage, N stage, pathological stage, or CD8+ T cell density between the two groups.
3.2 Models for predicting ALK rearrangement
A total of 122 radiomic features associated with ALK rearrangements were initially identified using univariate logistic regression (Supplementary Table S1) and screened using LASSO. The optimal λ was calculated to be 0.013, corresponding to 17 features with nonzero coefficients (Supplementary Figure S2; Supplementary Table S2). Forward stepwise regression analysis identified four robust radiomic features as independent predictors of ALK rearrangement (Table 2). A prediction model based on the four radiomic features was constructed, and the Rad-score for each patient was calculated. The Rad-score calculation formula is as follows (Equation 2, Supplementary Material 1):

Table 2. Multivariate logistic regression analyses of radiomic features to predict ALK rearrangement.
Among the clinicopathological features, univariate logistic regression analysis revealed that HLA-I and PD-L1 were significantly associated with ALK rearrangements (Supplementary Table S3). These two features were included in a multivariate logistic regression analysis, which indicated that the independent predictive features were HLA-I [odds ratio (OR) = 0.99; 95% CI: 0.98–0.99; P < 0.001] and PD-L1 (OR = 0.99; 95% CI: 0.98–0.99; P < 0.001), and these were incorporated into the establishment of a clinicopathological model. After combination with the Rad-score, the multivariate analysis showed that the significant factors in the combined model were Rad-score (OR = 2.88; 95% CI: 1.82–4.88; P < 0.001), HLA-I (OR = 0.99; 95% CI: 0.98–0.99; P < 0.001), and PD-L1 (OR = 0.99; 95% CI: 0.98–0.99; P < 0.001) (Supplementary Table S4).
The ROC curves for the fivefold cross-validation of the models are presented in Figure 1. ROC curve analysis yielded an AUC of 0.763 (95% CI: 0.695–0.836) for the radiomics model and indicated no significant difference (P = 0.36) from the clinicopathological model (AUC = 0.817; 95% CI: 0.781–0.851). The combined model (AUC = 0.878; 95% CI: 0.825–0.973) showed significantly superior performance compared to the clinicopathological (P = 0.02) and radiomic (P < 0.001) models alone. Based on 500 bootstrap iterations, the combined model achieved a mean AUC of 0.879 (SD = 0.030), corresponding to a CV of 0.034, indicating high reproducibility.
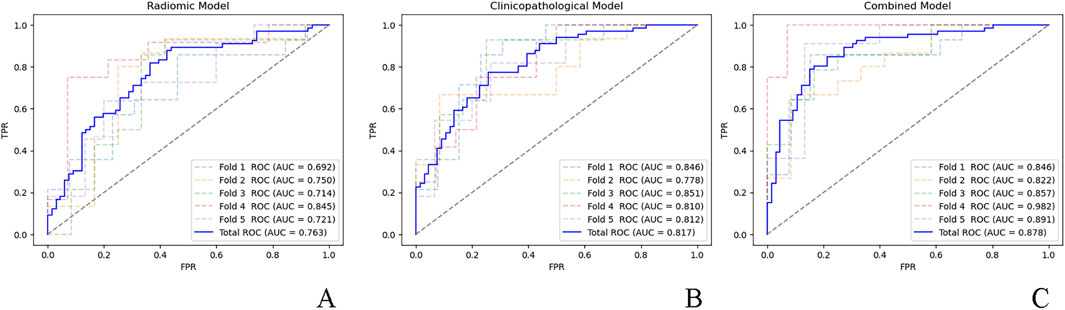
Figure 1. ROC curves for the five-fold cross-validation of the (A) radiomic model, (B) clinicopathological model, and (C) combined model. ROC, receiver operator characteristic; AUC, area under the curve; FPR, false positive rate; TPR, true positive rate.
As shown in Supplementary Figure S3, there was a weak negative correlation of Rad-score with HLA-I expression (r = −0.31; P < 0.001). No association was found between the Rad-score and other clinicopathological features.
3.3 Models for predicting the DFS of patients with ALK rearrangement
Among 63 patients with follow-up data, 26 experienced recurrence. The median follow-up period was 53 months, as determined using the reverse Kaplan-Meier method.
For the radiomic model, 400 features were selected by univariate analysis (Supplementary Table S5), and five features with nonzero coefficients remained after LASSO Cox regression (Supplementary Figure S4; Supplementary Table S6). Finally, two independent prognostic features were selected using Cox regression to build the RAD-risk score (Table 3), which was calculated as follows (Equation 3, Supplementary Material 1):

Table 3. Multivariate Cox regression analyses of radiomic features to predict disease-free survival.
Kaplan–Meier curves for the dichotomized RAD-risk score are illustrated in Figure 2, which shows that patients with ALK rearrangements can be divided into two risk groups. With the median RAD-risk score serving as the cut-off point, a higher RAD-risk score was significantly associated with a lower DFS probability (P = 0.03).
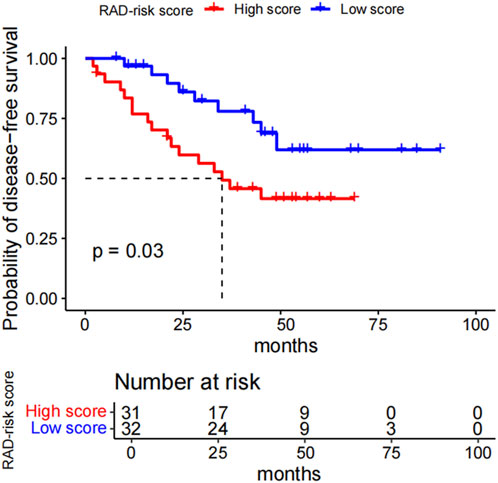
Figure 2. Kaplan-Meier survival curves for the RAD_risk score. With the median RAD_risk score value served as cut-off point, ALK-rearranged patients could be divided into two risk groups for disease-free survival (p = 0.03). RAD_risk score, radiomics risk score; ALK, anaplastic lymphoma kinase.
In the clinicopathological model, the univariate analysis showed that sex, T stage, N stage, and pathological stage were significantly associated with DFS (Supplementary Table S7). When these features were included in the multivariate analysis, N stage [hazard ratio (HR) = 5.31; 95% CI: 2.34–12.02; P < 0.001] was an independent predictor of DFS, resulting in a clinicopathological model. Finally, N stage and RAD-risk scores were incorporated into multivariate analysis to develop a combined model (Supplementary Table S8).
The C-indices for each model and 95% CIs are presented in Table 4. There was no significant difference between the radiomic model and the clinicopathological (P > 0.99) or combined models (P = 0.09), while the combined model showed significant improvement (P < 0.001) compared to the clinicopathological model alone. Based on 500 bootstrap iterations, the combined model achieved a mean C-index of 0.811 (SD = 0.042), corresponding to a CV of 0.052, indicating high reproducibility.
The RAD-risk score was statistically associated with T stage, N stage, and pathological stage (all P < 0.001) but not with other clinicopathological features (Supplementary Figure S5).
4 Discussion
In this study, we developed radiomics, clinicopathological, and combined models for predicting ALK rearrangements in lung adenocarcinomas and the DFS of patients with ALK rearrangements. The combined models outperformed the clinicopathological models in predicting ALK rearrangement status and DFS in patients with ALK rearrangement.
Immunosuppressive status is a characteristic of the TIME in patients with ALK-rearranged NSCLC (Zhang et al., 2022; Tian et al., 2023). CD8+ TILs are reduced in abundance (Zhang et al., 2022; Tian et al., 2023) or functionally impaired despite no decrease in their numbers (Zeng et al., 2020). Several studies have reported an association between PD-L1 expression and ALK rearrangements, but with inconsistent results. While Ota et al. found that ALK rearrangements can upregulate PD-L1 expression in NSCLC (Ota et al., 2015), Zeng et al. observed that PD-L1 was seldom expressed in ALK-positive tumor cells (Zeng et al., 2020). A meta-analysis concluded that PD-L1 expression did not correlate with ALK rearrangement (Zhang et al., 2017). The observed discrepancy in findings may be attributed to the limited sample size of ALK rearrangement cases in the studies and differences in ethnicity. We found that PD-L1 and HLA-I expression were adverse predictors of ALK rearrangement in lung adenocarcinomas, and that the abundance of CD8+ TILs in ALK-positive tumors was not different from that in ALK-negative tumors. PD-L1 downregulation indicates reduced reliance on the PD-1/PD-L1 axis for immune escape, whereas HLA-I downregulation impairs antigen presentation, rendering CD8+ T cells unable to recognize tumor cells. Preserved CD8+ T cell density may reflect functional impairment due to T cell exhaustion or antigen recognition impairment. These findings highlight the complexity of immune evasion in ALK-rearranged NSCLC and imply the potential involvement of other immune escape mechanisms that require further exploration.
With regard to the radiomic model, we identified four radiomic features as independent predictors of ALK rearrangement: one texture feature (Original.GLCM.Autocorrelation), two wavelet-transformed texture features (Wavelet_LLH.GLSZM.SmallAreaEmphasis and Wavelet_HLL.GLCM.Correlation), and one wavelet-transformed texture feature (Wavelet_LLL.Firstorder.Skewness). Skewness, which measures the asymmetry of the histogram from the mean, reflects intratumoral heterogeneity. Texture features are closely associated with tumor heterogeneity and prognosis, whereas wavelet-based features represent filtered transformations of intensity or texture features, capturing multiscale patterns within the tumor (Chen et al., 2017). Due to the low incidence of ALK-positive tumors and methodological bias, studies on the association between radiomic features and ALK rearrangements in NSCLC remain preliminary (Ninatti et al., 2020; Ma and Li, 2021; Chen et al., 2024). Correlation and skewness (original or filtered) are also found to be predictors of ALK rearrangements in previous studies (Agazzi et al., 2021; Choe et al., 2021; Aguloglu et al., 2022; Chen et al., 2025). Interestingly, Wavelet_LLH.GLSZM.SmallAreaEmphasis and Wavelet_LLL.Firstorder.Skewness have been observed to be predictors of brain metastases in patients with ALK-rearranged NSCLC (Wang et al., 2022), which may explain the high incidence of brain metastases in patients with ALK-rearranged NSCLC. Moreover, Wavelet_LLL.Firstorder.Skewness was also a predictor of DFS in the LASSO Cox regression model (Supplementary Table S5), demonstrating its prognostic value in ALK-positive patients.
Regarding prognostic value, PD-L1/HLA-I expression and the density of CD8+ TILs were not associated with DFS in patients with ALK rearrangement, while the radiomics signature was an independent prognostic factor in our study. Previous studies on the association between PD-L1 expression and progression-free survival (PFS) or overall survival (OS) in patients with ALK-rearranged advanced NSCLC treated with ALK TKIs found that high PD-L1 expression was associated with shorter PFS or OS (Zhang et al., 2022; Zhou et al., 2022; Tian et al., 2023). Similarly, studies regarding the association between radiomic features and the PFS of patients with ALK-rearranged NSCLC were limited to advanced-stage tumors treated with ALK-TKIs (Li et al., 2020; Hou et al., 2023; Sun et al., 2023), and also showed the significant prognostic performance of the radiomics signature.
To overcome the limitations of biopsy-related sampling artifacts and ensure robust pathological and molecular data, we focused on surgically resected lung adenocarcinomas. This approach allowed us to investigate the TIME and radiomic features of early stage tumors, offering new insights into their biological characteristics. Our findings may help identify and risk-stratify ALK-positive patients at an early stage, inform clinical decision-making, and guide adjuvant therapy or follow-up strategies for high-risk ALK-positive patients.
Our study showed that ALK-rearranged lung adenocarcinomas exhibit downregulation of PD-L1 and HLA-I, which may contribute to the limited efficacy of PD-1/PD-L1 inhibitors in this population. This observation aligns with emerging clinical evidence showing modest responses to ICIs in ALK-positive NSCLC (Mazieres et al., 2019; Jahanzeb et al., 2021). The lack of an association between TIME characteristics and DFS further underscores the need to explore alternative or combination immunotherapeutic strategies, such as targeting innate immune pathways or combining ICIs with ALK TKIs. Future studies should investigate these approaches to improve the outcomes for ALK-rearranged NSCLC patients.
The radiomics model, when combined with a clinicopathological model incorporating PD-L1 and HLA-I expression to predict ALK rearrangement and lymph node metastasis status to predict DFS in ALK-rearranged lung adenocarcinomas, demonstrated superior performance compared to the clinicopathological model alone. These findings suggest that radiomic features provide added value for the noninvasive identification of ALK rearrangement and prognostic prediction in patients with ALK-rearranged lung adenocarcinomas. Notably, we observed a significant correlation between the Rad-score and HLA-I expression as well as between the RAD-risk score and pathological TNM stage. These findings suggest that radiomic features may reflect underlying biological processes, such as immune microenvironment characteristics and tumor progression, thereby enhancing the interpretability of our data-driven models.
This study has several limitations. First, the sample size was relatively small owing to the rarity of ALK alterations, which may restrict feature diversity, and the random selection of controls may have introduced a selection bias. Future studies should employ matched designs to reduce the potential confounding factors. Second, as our study focused exclusively on surgically resected lung adenocarcinomas, further studies are needed to validate the applicability of our findings to non-resectable or advanced-stage tumors, which are the primary target population for TKIs or ICIs. Third, the inter-scanner and inter-vendor variability of features may have confounded the results. However, the fact that radiomic features were extracted from multiple scanners in our study may support the generalizability of our models. Moreover, we did not analyze the influence of treatment because only four patients in this early-stage disease cohort received TKI therapy. In future studies, we aim to include a broader patient population, including those receiving TKI therapy, to further evaluate the interplay between treatment, TIME, and outcomes. Finally, although we performed internal cross-validation, multicenter prospective studies with independent external validation are required to confirm our findings.
In conclusion, our results support the potential role of radiomics and TIME in identifying ALK rearrangements in lung adenocarcinomas and the prognostic value of radiomics in predicting the DFS of patients with ALK rearrangements. We believe that radiomics may improve the risk stratification of patients with ALK rearrangements, thereby facilitating personalized treatment. Future studies should validate these models in large multicenter cohorts and integrate multi-omics data (e.g., genomic, transcriptomic, and proteomic) and additional TIME markers to elucidate the biological mechanisms of radiomic features, improve prognostic accuracy, and guide personalized therapy for ALK-positive patients.
Data availability statement
The original contributions presented in the study are included in the article/Supplementary Material, further inquiries can be directed to the corresponding author.
Ethics statement
The studies involving humans were approved by the institutional review board of Tianjin Medical University Cancer Institute and Hospital. The studies were conducted in accordance with the local legislation and institutional requirements. The participants provided their written informed consent to participate in this study.
Author contributions
YH: Conceptualization, Data curation, Funding acquisition, Investigation, Resources, Writing – review and editing. WF: Data curation, Investigation, Writing – review and editing. HL: Formal Analysis, Writing – original draft. HW: Conceptualization, Funding acquisition, Investigation, Methodology, Project administration, Writing – original draft, Writing – review and editing. ZY: Funding acquisition, Supervision, Writing – review and editing.
Funding
The author(s) declare that financial support was received for the research and/or publication of this article. This work was supported by the National Natural Science Foundation of China (grant Nos 81601492 and 81702268), Ministry of Science and Technology of China (No. 2024ZD0520002), the Tianjin Municipal Health Commission Science and Technology project (grant No. TJWJ2022MS052), and the Tianjin Key Medical Discipline (Specialty) Construction Project (No. TJYXZDXK-009A).
Acknowledgments
We would like to thank Huan Zhang (Cancer Prevention Center, Tianjin Medical University Cancer Institute and Hospital) for kindly providing statistical advice for this study. We would like to thank Editage (www.editage.cn) for English language editing.
Conflict of interest
The authors declare that the research was conducted in the absence of any commercial or financial relationships that could be construed as a potential conflict of interest.
Generative AI statement
The author(s) declare that no Generative AI was used in the creation of this manuscript.
Publisher’s note
All claims expressed in this article are solely those of the authors and do not necessarily represent those of their affiliated organizations, or those of the publisher, the editors and the reviewers. Any product that may be evaluated in this article, or claim that may be made by its manufacturer, is not guaranteed or endorsed by the publisher.
Supplementary material
The Supplementary Material for this article can be found online at: https://www.frontiersin.org/articles/10.3389/fgene.2025.1581937/full#supplementary-material
Abbreviations
ALK, anaplastic lymphoma kinase; AUC, area under the curve; CI, confidence interval; CV, coefficient of variation; DFS, disease-free survival; GLCM, gray-level cooccurrence matrix; GLDM, gray-level dependence matrix; GLRLM, gray-level run-length matrix; GLSZM, gray-level size-zone matrix; HLA-I, human leukocyte antigen class I; HR, hazard ratio; ICI, immune checkpoint inhibitor; IHC, immunohistochemistry; LASSO, least absolute shrinkage and selection operator; NGTDM, neighborhood gray-tone difference matrix; NSCLC, non–small cell lung cancer; OR, odds ratio; OS, overall survival; PD-L1, programmed death ligand 1; PFS, progression-free survivall; OC, receiver operator characteristic; TIME, tumor immune microenvironment; TKI, tyrosine kinase inhibitor.
References
Agazzi, G. M., Ravanelli, M., Roca, E., Medicina, D., Balzarini, P., Pessina, C., et al. (2021). CT texture analysis for prediction of EGFR mutational status and ALK rearrangement in patients with non-small cell lung cancer. Radiol. Med. 126 (6), 786–794. doi:10.1007/s11547-020-01323-7
Aguloglu, N., Aksu, A., Akyol, M., Katgi, N., and Doksoz, T. C. (2022). IMPORTANCE of PRETREATMENT 18F-FDG PET/CT TEXTURE ANALYSIS in PREDICTING EGFR and ALK MUTATION in PATIENTS with NON-SMALL CELL LUNG CANCER. Nuklearmedizin 61 (6), 433–439. doi:10.1055/a-1868-4918
Borghaei, H., Paz-Ares, L., Horn, L., Spigel, D. R., Steins, M., Ready, N. E., et al. (2015). Nivolumab versus docetaxel in advanced nonsquamous non-small-cell lung cancer. N. Engl. J. Med. 373 (17), 1627–1639. doi:10.1056/NEJMoa1507643
Brahmer, J., Reckamp, K. L., Baas, P., Crino, L., Eberhardt, W. E., Poddubskaya, E., et al. (2015). Nivolumab versus docetaxel in advanced squamous-cell non-small-cell lung cancer. N. Engl. J. Med. 373 (2), 123–135. doi:10.1056/NEJMoa1504627
Brea, E. J., Oh, C. Y., Manchado, E., Budhu, S., Gejman, R. S., Mo, G., et al. (2016). Kinase regulation of human MHC class I molecule expression on cancer cells. Cancer Immunol. Res. 4 (11), 936–947. doi:10.1158/2326-6066.CIR-16-0177
Chen, B., Zhang, R., Gan, Y., Yang, L., and Li, W. (2017). Development and clinical application of radiomics in lung cancer. Radiat. Oncol. 12 (1), 154. doi:10.1186/s13014-017-0885-x
Chen, J., Chen, A., Yang, S., Liu, J., Xie, C., and Jiang, H. (2024). Accuracy of machine learning in preoperative identification of genetic mutation status in lung cancer: a systematic review and meta-analysis. Radiother. Oncol. 196, 110325. doi:10.1016/j.radonc.2024.110325
Chen, W., Lin, G., Feng, Y., Chen, Y., Li, Y., Li, J., et al. (2025). Intratumoral and peritumoral CT radiomics in predicting anaplastic lymphoma kinase mutations and survival in patients with lung adenocarcinoma: a multicenter study. Cancer Imaging 25 (1), 35. doi:10.1186/s40644-025-00856-2
Choe, J., Lee, S. M., Kim, W., Do, K. H., Kim, S., Choi, S., et al. (2021). CT radiomics-based prediction of anaplastic lymphoma kinase and epidermal growth factor receptor mutations in lung adenocarcinoma. Eur. J. Radiol. 139, 109710. doi:10.1016/j.ejrad.2021.109710
Detterbeck, F. C., Boffa, D. J., Kim, A. W., and Tanoue, L. T. (2017). The eighth edition lung cancer stage classification. Chest 151 (1), 193–203. doi:10.1016/j.chest.2016.10.010
Fedorov, A., Beichel, R., Kalpathy-Cramer, J., Finet, J., Fillion-Robin, J. C., Pujol, S., et al. (2012). 3D slicer as an image computing platform for the quantitative imaging network. Magn. Reson Imaging 30 (9), 1323–1341. doi:10.1016/j.mri.2012.05.001
Gainor, J. F., Shaw, A. T., Sequist, L. V., Fu, X., Azzoli, C. G., Piotrowska, Z., et al. (2016). EGFR mutations and ALK rearrangements are associated with low response rates to PD-1 pathway blockade in non-small cell lung cancer: a retrospective analysis. Clin. Cancer Res. 22 (18), 4585–4593. doi:10.1158/1078-0432.CCR-15-3101
Greeshma, L. R., Joseph, A. P., Sivakumar, T. T., Raghavan Pillai, V., and Vijayakumar, G. (2023). Correlation of PD-1 and PD-L1 expression in oral leukoplakia and oral squamous cell carcinoma: an immunohistochemical study. Sci. Rep. 13 (1), 21698. doi:10.1038/s41598-023-48572-w
Haratani, K., Hayashi, H., Tanaka, T., Kaneda, H., Togashi, Y., Sakai, K., et al. (2017). Tumor immune microenvironment and nivolumab efficacy in EGFR mutation-positive non-small-cell lung cancer based on T790M status after disease progression during EGFR-TKI treatment. Ann. Oncol. 28 (7), 1532–1539. doi:10.1093/annonc/mdx183
Hou, D., Zheng, X., Song, W., Liu, X., Wang, S., Zhou, L., et al. (2023). Radiomic-signature changes after early treatment improve the prediction of progression-free survival in patients with advanced anaplastic lymphoma kinase-positive non-small cell lung cancer. Acta Radiol. 64 (3), 1194–1204. doi:10.1177/02841851221119621
Jahanzeb, M., Lin, H. M., Pan, X., Yin, Y., Baumann, P., and Langer, C. J. (2021). Immunotherapy treatment patterns and outcomes among ALK-positive patients with non-small-cell lung cancer. Clin. Lung Cancer 22 (1), 49–57. doi:10.1016/j.cllc.2020.08.003
Kim, H., Jang, S. J., Chung, D. H., Yoo, S. B., Sun, P., Jin, Y., et al. (2013). A comprehensive comparative analysis of the histomorphological features of ALK-rearranged lung adenocarcinoma based on driver oncogene mutations: frequent expression of epithelial-mesenchymal transition markers than other genotype. PLoS One 8 (10), e76999. doi:10.1371/journal.pone.0076999
Li, H., Zhang, R., Wang, S., Fang, M., Zhu, Y., Hu, Z., et al. (2020). CT-based radiomic signature as a prognostic factor in stage IV ALK-positive non-small-cell lung cancer treated with TKI crizotinib: a proof-of-concept study. Front. Oncol. 10, 57. doi:10.3389/fonc.2020.00057
Ma, J. W., and Li, M. (2021). Molecular typing of lung adenocarcinoma with computed tomography and CT image-based radiomics: a narrative review of research progress and prospects. Transl. Cancer Res. 10 (9), 4217–4231. doi:10.21037/tcr-21-1037
Mazieres, J., Drilon, A., Lusque, A., Mhanna, L., Cortot, A. B., Mezquita, L., et al. (2019). Immune checkpoint inhibitors for patients with advanced lung cancer and oncogenic driver alterations: results from the IMMUNOTARGET registry. Ann. Oncol. 30 (8), 1321–1328. doi:10.1093/annonc/mdz167
Mu, D., Guo, J., Yu, W., Zhang, J., Ren, X., and Han, Y. (2022). Downregulation of PD-L1 and HLA-I in non-small cell lung cancer with ALK fusion. Thorac. Cancer 13 (8), 1153–1163. doi:10.1111/1759-7714.14372
Ninatti, G., Kirienko, M., Neri, E., Sollini, M., and Chiti, A. (2020). Imaging-based prediction of molecular therapy targets in NSCLC by radiogenomics and AI approaches: a systematic review. Diagn. (Basel) 10 (6), 359. doi:10.3390/diagnostics10060359
Ota, K., Azuma, K., Kawahara, A., Hattori, S., Iwama, E., Tanizaki, J., et al. (2015). Induction of PD-L1 expression by the EML4-ALK oncoprotein and downstream signaling pathways in non-small cell lung cancer. Clin. Cancer Res. 21 (17), 4014–4021. doi:10.1158/1078-0432.CCR-15-0016
Qin, A., and Gadgeel, S. (2017). The current landscape of anaplastic lymphoma kinase (ALK) in non-small cell lung cancer: emerging treatment paradigms and future directions. Target Oncol. 12 (6), 709–718. doi:10.1007/s11523-017-0526-1
Reck, M., Rodriguez-Abreu, D., Robinson, A. G., Hui, R., Csoszi, T., Fulop, A., et al. (2016). Pembrolizumab versus chemotherapy for PD-L1-positive non-small-cell lung cancer. N. Engl. J. Med. 375 (19), 1823–1833. doi:10.1056/NEJMoa1606774
Rothenstein, J. M., and Chooback, N. (2018). ALK inhibitors, resistance development, clinical trials. Curr. Oncol. 25 (Suppl. 1), S59–S67. doi:10.3747/co.25.3760
Shaw, A. T., Kim, D. W., Nakagawa, K., Seto, T., Crino, L., Ahn, M. J., et al. (2013). Crizotinib versus chemotherapy in advanced ALK-positive lung cancer. N. Engl. J. Med. 368 (25), 2385–2394. doi:10.1056/NEJMoa1214886
Smolarz, B., Lukasiewicz, H., Samulak, D., Piekarska, E., Kolacinski, R., and Romanowicz, H. (2025). Lung cancer-epidemiology, pathogenesis, treatment and molecular aspect (review of literature). Int. J. Mol. Sci. 26 (5), 2049. doi:10.3390/ijms26052049
Sun, J., Li, F., Yang, J., Lin, C., Zhou, X., Liu, N., et al. (2023). Pretherapy investigations using highly robust visualized biomarkers from CT imaging by multiple machine-learning techniques toward its prognosis prediction for ALK-inhibitor therapy in NSCLC: a feasibility study. J. Cancer Res. Clin. Oncol. 149 (10), 7341–7353. doi:10.1007/s00432-023-04615-3
Tian, X., Li, Y., Huang, Q., Zeng, H., Wei, Q., and Tian, P. (2023). High PD-L1 expression correlates with an immunosuppressive tumour immune microenvironment and worse prognosis in ALK-rearranged non-small cell lung cancer. Biomolecules 13 (6), 991. doi:10.3390/biom13060991
Tomaszewski, M. R., and Gillies, R. J. (2021). The biological meaning of radiomic features. Radiology 298 (3), 505–516. doi:10.1148/radiol.2021202553
Wang, H., Chen, Y. Z., Li, W. H., Han, Y., Li, Q., and Ye, Z. (2022). Pretreatment thoracic CT radiomic features to predict brain metastases in patients with ALK-rearranged non-small cell lung cancer. Front. Genet. 13, 772090. doi:10.3389/fgene.2022.772090
Watanabe, S., Hayashi, H., Haratani, K., Shimizu, S., Tanizaki, J., Sakai, K., et al. (2019). Mutational activation of the epidermal growth factor receptor down-regulates major histocompatibility complex class I expression via the extracellular signal-regulated kinase in non-small cell lung cancer. Cancer Sci. 110 (1), 52–60. doi:10.1111/cas.13860
Zeng, C., Gao, Y., Xiong, J., Lu, J., Yang, J., Wang, X., et al. (2020). Tumor-infiltrating CD8(+) T cells in ALK-positive lung cancer are functionally impaired despite the absence of PD-L1 on tumor cells. Lung Cancer 150, 139–144. doi:10.1016/j.lungcan.2020.10.009
Zhang, B., Zeng, J., Zhang, H., Zhu, S., Wang, H., He, J., et al. (2022). Characteristics of the immune microenvironment and their clinical significance in non-small cell lung cancer patients with ALK-rearranged mutation. Front. Immunol. 13, 974581. doi:10.3389/fimmu.2022.974581
Zhang, M., Li, G., Wang, Y., Wang, Y., Zhao, S., Haihong, P., et al. (2017). PD-L1 expression in lung cancer and its correlation with driver mutations: a meta-analysis. Sci. Rep. 7 (1), 10255. doi:10.1038/s41598-017-10925-7
Zhou, J., Lin, H., Ni, Z., Luo, R., Yang, D., Feng, M., et al. (2023). Expression of PD-L1 through evolution phase from pre-invasive to invasive lung adenocarcinoma. BMC Pulm. Med. 23 (1), 18. doi:10.1186/s12890-023-02310-0
Keywords: radiomics, tumor microenvironment, lung adenocarcinoma, anaplastic lymphoma kinase, computed tomography
Citation: Han Y, Feng W, Li H, Wang H and Ye Z (2025) Radiomic features and tumor immune microenvironment associated with anaplastic lymphoma kinase-rearranged lung adenocarcinoma and their prognostic value. Front. Genet. 16:1581937. doi: 10.3389/fgene.2025.1581937
Received: 23 February 2025; Accepted: 14 April 2025;
Published: 01 May 2025.
Edited by:
Yiming Meng, China Medical University, ChinaReviewed by:
Shaohui Wang, Chengdu University of Traditional Chinese Medicine, ChinaJunmeng Li, Henan Provincial People’s Hospital, China
Chunji Chen, Shanghai Jiao Tong University, China
Wang Fang, University of Chinese Academy of Sciences, China
Shen Lao, First Affiliated Hospital of Guangzhou Medical University, China
Copyright © 2025 Han, Feng, Li, Wang and Ye. This is an open-access article distributed under the terms of the Creative Commons Attribution License (CC BY). The use, distribution or reproduction in other forums is permitted, provided the original author(s) and the copyright owner(s) are credited and that the original publication in this journal is cited, in accordance with accepted academic practice. No use, distribution or reproduction is permitted which does not comply with these terms.
*Correspondence: Hua Wang, d2FuZ2h1YTA3MThAMTYzLmNvbQ==
†These authors share first authorship