- 1Jet Propulsion Laboratory, California Institute of Technology, Pasadena, CA, United States
- 2Lamont Doherty Earth Observatory, Department of Earth and Environmental Science, Columbia University, Palisades, NY, United States
- 3Department of Earth and Environment, Boston University, Boston, MA, United States
- 4Department of Earth, Environment, and Physics, Worcester State University, Worcester, MA, United States
- 5Department of Biological Sciences, University of Southern California, Los Angeles, CA, United States
In this study, we examine the capabilities of the Landsat 8 Operational Land Imager (OLI), Thermal Infrared Sensor (TIRS), and Aqua Moderate resolution Imaging Spectroradiometer (MODIS) for monitoring the environmental impact of the 2015 Hyperion Treatment Plant (HTP) wastewater diversion in Santa Monica Bay, California. From 21 September−2 November 2015, the HTP discharged ~39 × 103 m3 h−1 of treated wastewater into Santa Monica Bay through their emergency 1-mile outfall pipe. Multi-sensor satellite remote sensing was employed to determine the biophysical impact of discharged wastewater in the shallow nearshore environment. Landsat 8 TIRS observed decreased sea surface temperatures (SST) associated with the surfacing wastewater plume. Chlorophyll-a (chl-a) concentrations derived from Landsat 8 OLI and Aqua MODIS satellite sensors were used to monitor the biological response to the addition of nutrient-rich wastewater. In situ chl-a and in situ remote sensing reflectance (Rrs) were measured before, during, and after the diversion event. These in situ data were paired with coincident OLI and MODIS satellite data to yield a more comprehensive view of the changing conditions in Santa Monica Bay due to the wastewater diversion. Two new local chl-a algorithms were empirically derived using in situ data for the OLI and MODIS sensors. These new local chl-a algorithms proved more accurate at measuring chl-a changes in Santa Monica Bay compared to the standard open ocean OC2 and OC3M algorithms, and the regional southern California CALFIT algorithm, as validated by in situ chl-a measurements. Additionally, the local OLI algorithm outperformed the local MODIS algorithm, especially in the nearshore region. A time series of chl-a, as detected by the local OLI chl-a algorithm, illustrated a very large increase in chl-a concentrations during the wastewater diversion, and a subsequent decrease in chl-a after the diversion. Our study demonstrates the capability of using Landsat 8 TIRS and OLI sensors for the monitoring of SST and surface chl-a concentrations at high spatial resolution in nearshore waters and highlights the value of these sensors for assessing the environmental effects of wastewater discharge in a coastal environment.
Introduction
The coastal waters of the Southern California Bight border one of the most densely populated regions in North America and are increasingly affected by pollution from both publicly owned treatment works (POTWs) and stormwater runoff due to increasing population growth and urbanization (McKinney, 2002; Bay et al., 2003; Creel, 2003; Schiff and Bay, 2003; Crossett et al., 2004; Lyon and Stein, 2009; Gierach et al., 2017; Holt et al., 2017). The Hyperion Treatment Plant (HTP) is the largest POTW in the Southern California Bight, discharging wastewater directly into Santa Monica Bay. Servicing the Los Angeles Metropolitan Area, HTP releases a daily average of 8.71 × 105 m3 of secondary treated effluent into Santa Monica Bay via a 5-mile (8.1 km) outfall pipe (Southern California Coastal Water Research Project, 1973; Steinberger and Stein, 2004; Lyon and Stein, 2009). However, during diversion events, when their main 5-mile outfall pipe is temporarily shut down, HTP diverts the wastewater to their 1-mile (1.2 km) outfall pipe, discharging the wastewater into the nearshore environment of Santa Monica Bay (Figure 1). Based on the findings from a 2006 inspection and diversion event (Reifel et al., 2013; Gierach et al., 2017), HTP scheduled another diversion event in Fall 2015 in order to conduct necessary infrastructure repairs. During this 6-week diversion event from 21 September−2 November 2015, HTP discharged an average of 39×103 m3 h−1 of treated wastewater through their backup 1-mile outfall pipe into the coastal environment.
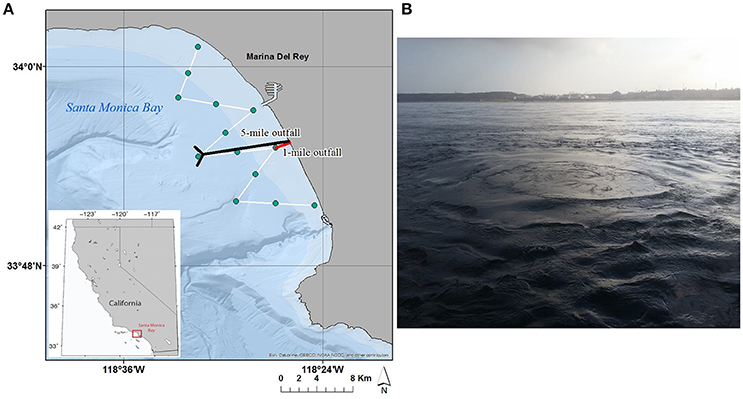
Figure 1. (A) Map of Santa Monica Bay, California, with the 5-mile and 1-mile outfall pipes denoted as black and red lines, respectively, and in situ sampling stations denoted as green circles. (B) Photo of the wastewater plume surfacing at the terminus of the 1-mile outfall pipe during the diversion.
Secondary treated effluent released in coastal waters not only contains contaminants (e.g., harmful chemicals, coliform bacteria, oils, and metals), but also organic matter and nutrients that can affect water quality (Steinberger and Stein, 2004). Wastewater effluent has been found to contribute as much nitrogen to coastal regions as wind-driven upwelling in several sub-regions of the Southern California Bight, including Santa Monica Bay, thereby doubling the nitrogen load (Howard et al., 2014; McLaughlin et al., 2017). A primary environmental concern of the release of nutrient-rich wastewater into the Southern California Bight is the stimulation of primary production, which can lead to eutrophication and the proliferation of toxic harmful algal blooms (Caron et al., 2017). Under typical density-stratification conditions for the region, the discharge of wastewater near the head of the Santa Monica Submarine Canyon (8.1 km offshore at 57 m depth), limits the likelihood that wastewater will surface and affect marine life and human health (Washburn et al., 1992; Uchiyama et al., 2014). In contrast, the 1-mile outfall pipe terminates within the shallow nearshore region of Santa Monica Bay, discharging the wastewater at 18 m depth. During diversion events, the discharge of wastewater through the 1-mile outfall pipe increases contaminant and nitrogen concentrations in the shallow and sunlit coastal waters of Santa Monica Bay, thereby increasing the probability of phytoplankton blooms and contaminant exposure to marine life and humans, as Santa Monica Bay is a major coastal recreation area (Caron et al., 2017; Gierach et al., 2017).
With the advent and continued advancement in satellite sensors, remote sensing has become an effective tool for monitoring the biophysical impacts of coastal pollution (e.g., DiGiacomo et al., 2004; Hu et al., 2004; Nezlin and DiGiacomo, 2005; Nezlin et al., 2005, 2008, 2012; Marmorino et al., 2010; Holt et al., 2017). Radar, thermal, and optical sensors have, respectively, been used to track the surface expression of wastewater plumes through reduced sea surface roughness, decreased temperature, and changes in surface-water reflectance due to increased organic matter (DiGiacomo et al., 2004; Marmorino et al., 2010; Nezlin et al., 2012). Most recently, Gierach et al. (2017) demonstrated the capabilities of the Moderate resolution Imaging Spectroradiometer (MODIS), on board NASA's Aqua satellite, launched in 2002, and the Advanced Spaceborne Thermal Emission and Reflection Radiometer (ASTER), on board NASA's Terra satellite, launched in 1999, in detecting changes in chlorophyll-a (chl-a) and sea surface temperature (SST) associated with the surfacing wastewater plume during the 2006 HTP diversion. Chl-a, a photosynthetic pigment present in phytoplankton, can be measured by optical satellite sensors, and is used as a proxy for phytoplankton biomass in the surface ocean.
Satellite remote sensing greatly expands the spatio-temporal coverage of the marine environment compared to in situ monitoring. However, the coarse spatial resolution (~750–1 km at nadir) of current optical satellite sensors used to monitor marine environments, and the uncertainties associated with instrument calibration and data processing algorithms in optically complex water (McClain, 2009), have limited the ability to monitor water quality in urban coastal waters like Santa Monica Bay. While improvements have been made in on-orbit assessment of sensor characteristics, atmospheric correction, and sensor calibration procedures over time (Franz et al., 2007), the resolution of current ocean color sensors still remains one of the most limiting factors for water quality applications in nearshore areas, as they are unable to accurately resolve coastal ocean characteristics (Mouw et al., 2015). The Thermal Infrared Sensor (TIRS) and the Operational Land Imager (OLI) on board Landsat 8, launched in 2013, provide two high-resolution sensors with high signal-to-noise ratios to more precisely monitor biophysical changes in the coastal Santa Monica Bay. With 100 m and 30 m spatial resolution, respectively, TIRS and OLI are better suited to resolve detailed features of SST and surface chl-a in the nearshore region compared to MODIS (1 km) and other similar satellite sensors, e.g., SeaWiFS (1.1 km), AVHRR (1.1 km), and VIIRS (750 m). Additionally, improved sensor signal-to-noise ratio and radiometric resolution of OLI and TIRS, compared to its predecessors, e.g., Landsat 7 ETM+, permits use of these land-designed sensors for marine applications. For example, the ability to monitor water quality in coastal regions, as coastal waters are generally dark targets, requires better signal-to-noise ratio and radiometric resolution to accurately resolve coastal features (Vanhellemont and Ruddick, 2014).
Though high-resolution sensors are likely to yield more detailed measurements than their coarser resolution counterparts, discrepancies in satellite chl-a values continue to be common in optically complex nearshore waters, such as the Southern California Bight (Kim et al., 2009). Therefore, we argue the necessity for the derivation and application of more locally derived empirical chl-a algorithms, as opposed to the standard open-ocean chl-a algorithms commonly used for each satellite sensor. In this study, we investigate the utility of high-resolution Landsat 8 TIRS and OLI satellite sensors, as compared to coarser resolution Aqua MODIS, in conjunction with corresponding in situ field measurements, to monitor the biophysical impacts of the Fall 2015 HTP wastewater diversion in Santa Monica Bay. Standard chl-a algorithms for each sensor are compared to newly derived empirical local chl-a algorithms, tuned specifically for the optically complex nearshore waters of Santa Monica Bay to accurately distinguish chl-a from other optical constituents in the water, such as suspended sediments and colored dissolved organic matter (CDOM). To the extent of our knowledge, this study is the first to develop, validate, and apply OLI chl-a retrievals for water quality monitoring in coastal waters.
The paper is organized as follows. Section Data and Methods describes the study region of Santa Monica Bay and the data utilized, including in situ, TIRS SST, satellite chl-a as detected by OLI and MODIS using standard and newly derived local chl-a algorithms. Section Data and Methods also describes the development of the local chl-a algorithms using in situ chl-a and in situ remote sensing reflectance (Rrs) measurements during the wastewater diversion. Results from the Fall 2015 HTP diversion are presented in section Results. Finally, in section Discussion we discuss the satellite findings and application of the newly derived local Landsat 8 OLI chl-a algorithm to more accurately monitor the evolution of the biological impact of the wastewater diversion.
Data and Methods
Study Area
Santa Monica Bay is a semi-enclosed nearshore bay in the Southern California Bight whose coastal waters are directly influenced by the densely-populated Los Angeles basin. The bay is characterized by a relatively wide continental shelf and features a mixture of submarine outcrops and canyons (Figure 1). The unique geography and coastal processes in the region create favorable conditions for a relatively productive coastal environment and diverse ecosystem (Hickey, 1992; Eganhouse and Venkatesan, 1993; Hickey et al., 2003; Corcoran and Shipe, 2011). Though the seasonal supply of nutrients from coastal upwelling is a major driver of phytoplankton biomass variability in Santa Monica Bay, there is increasing evidence that anthropogenic nutrient point sources also contribute significantly to the phytoplankton dynamics of the bay (Nezlin and Li, 2003; Corcoran and Shipe, 2011; Nezlin et al., 2012; Howard et al., 2014; Gierach et al., 2017).
In Situ Measurements
In situ samples and measurements in Santa Monica Bay were collected before, during, and after the wastewater diversion as part of a large collaborative sampling effort led by the City of Los Angeles' Environmental Monitoring Division (CLAEMD) to monitor the impacts of the HTP diversion on water quality (Figure 1 and Table 1). The samples and measurements were collected onboard the R/V La Mer and R/V Surveyor along a grid of 13 stations between 26 August and 11 November 2015 (Figure 1). Three main categories of samples/measurements were collected in situ: (1) hydrographic data from a Conductivity-Temperature-Depth (CTD) instrument, (2) surface water samples for lab-based chl-a concentration analyses, and (3) radiometric measurements to derive in situ hyperspectral remote sensing reflectance (Rrs). When possible, the in situ sampling was planned to coincide with satellite overpasses in order to facilitate comparison between in situ measurements and remote sensing retrievals.
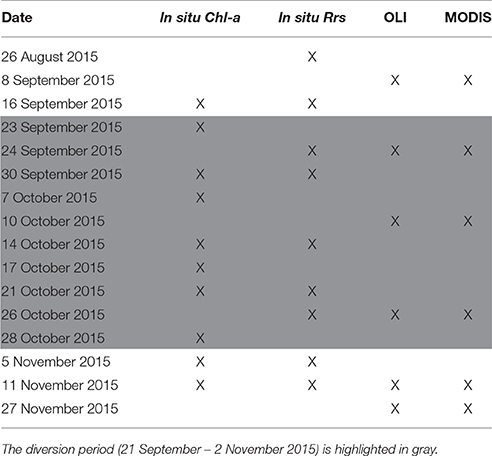
Table 1. In situ and remote sensing sampling dates before, during, and after the wastewater diversion event in Santa Monica Bay.
Hydrographic Profiles
Vertical hydrographic profiles were collected at all stations along the sample grid (Figure 1) using a SBE 19-plus Conductivity-Temperature-Depth (CTD) package (Sea-Bird Electronics, Inc. Bellevue WA), mounted on a rosette also equipped with a C-star transmissometer (WET Labs, Inc. Philomath, OR), and WETStar chl-a and CDOM fluorometers (WET Labs, Inc. Philomath, OR) (City of Los Angeles, 2009; Reifel et al., 2013) to determine temperature and chlorophyll fluorescence from 1 m below the surface to 1 m above the ocean floor.
In Situ Surface Chlorophyll-a Concentrations
Discrete surface water samples were collected at 1 meter for chl-a analysis at selected stations along the sample grid before, during, and after the wastewater diversion (Figure 1, Table 1). Duplicate water samples were collected from Niskin bottles and filtered immediately onboard the ship by gentle filtration of 5–100 mL of water onto 0.7-μm GF/F glass fiber filters. The filtration volume was reduced incrementally from 100 mL based on the biomass indicated by in situ chlorophyll fluorescence measured at 1 meter by the CTD profiler. Filters were then stored at −20°C until they were analyzed fluorometrically for chl-a in the laboratory using the non-acidification method (Welschmeyer, 1994). Filters were extracted in 100% acetone at −20°C in the dark for 24 h. Filter extracts were analyzed on a Turner Designs Trilogy fluorometer (Turner Designs, Sunnyvale, CA).
In Situ Remote Sensing Reflectance
In situ hyperspectral remote sensing reflectance, Rrs(λ,0+), was measured in the λ = 350–700 nm wavelength range at selected stations before, during, and after the diversion event (Table 1). Rrs(λ,0+) was derived from field measurements of spectral downwelling irradiance, Ed(λ), and upwelling radiance, Lu(λ), acquired using a Satlantic® HyperPRO free-falling optical profiler equipped with a surface irradiance reference. The measured Ed(λ) and Lu(λ) were used to calculate Rrs(λ,0+) following Equation (1):
where Ed(λ,0+) is the spectral downwelling irradiance just above the surface and Lw(λ) is the spectral water-leaving radiance, equivalent to Lu(λ) measured just above the surface: Lu(λ,0+).
About half of the Rrs(λ,0+) spectra were measured and derived using the HyperPRO in profiling mode. In this configuration, simultaneous profiles of Ed(λ,z) and Lu(λ,z) (where z is depth) were used to calculate Rrs(λ,0+). The maximum depth of the optical profiles ranged from a few meters in nearshore waters to 60 m in the more oligotrophic waters. Profiles were performed away from the influence of the ship, at a distance of at least 30 m and on the sunny side of the ship. Any data with an instrument tilt of >5° was discarded. At each sampled station, measurements of Ed(λ,z) and Lu(λ,z) were collected during three or four optical profiles in clear waters, and up to 10 optical profiles in more shallow and turbid waters. The data were processed to Level-2 with the Satlantic® Prosoft software. Our own MatLab® routine was then used to do a supervised processing of the Ed(λ,z) and Lu(λ,z) profiles and calculate Rrs(λ,0+). In this routine, Ed(λ,0−) was calculated as the exponential of the intercept (z = 0) of the least-square fit of the pooled profiles of ln[Ed(λ,z)] against depth z, and it was then converted to Ed(λ,0+) using the approximation: Ed(λ,0+) = 1.04 Ed(λ,0−) (Austin, 1974). Similarly, Lu(λ,0−) was calculated as the exponential of the intercept (z = 0) of the least-square fit of the pooled ln[Lu(λ,0−)] profiles against depth z, and it was then converted to Lw(λ) using the approximation Lw(λ) = 0.54 Lu(λ,0−) (Austin, 1974). Data from the multiple profiles were pooled together in order to provide sufficient data density to derive reliable linear fits of Ed(λ,z) and Lu(λ,z) against depth, and supervised processing with our MatLab® routine allowed to select adequate depth ranges (between 0.5 m to several meters depth) for reliable fits. At each station, profiles were collected within a short period of time (< 20–30 min) and, in most cases, the illumination conditions were very stable throughout the data collection. Any Ed(λ,z) and Lu(λ,z) profile that differed substantially was discarded in order to minimize errors in the fit. Note here that the spectral diffuse attenuation coefficient of upwelling radiance, KLu(λ), was also derived from these optical profiles as the slope of the least-square fit of the pooled ln[Lu(λ,0−)] profiles against depth z.
The remainder of the Rrs(λ,0+) were measured and derived by using the HyperPRO deployed in buoy mode. The use of the buoy-mode configuration was done for practical reasons and in the interest of saving time during the sampling cruises. In this configuration, Ed(λ,0+) and Lu(λ,0−) were measured directly for a short time period of ~2 min and used as in equation (1) to estimate Rrs(λ,0+) assuming Lw(λ) = 0.54 Lu(λ,0−). The Lu(λ,0−) measurements were corrected for the fact that upwelling radiance was measured ~ 0.2 m below the water surface rather than just below the surface. Estimates of KLu(λ) were thus used following the equation Lu(λ,0−) = Lu(λ,0.2)*exp(KLu(λ)*0.2) to correct for the diffuse attenuation of Lu(λ) occurring in the top 20 cm. However, KLu(λ) could not be measured using the HyperPRO configured in “buoy mode” and therefore had to be estimated from uncorrected Rrs(λ,0+) determined by assuming Lu(λ,0.2) = Lu(λ,0−). The estimation of KLu(λ) from the uncorrected Rrs(λ,0+) was made possible by using a partial-least-squares (PLS) regression model specifically developed for this study. The PLS model was developed using the other data set collected with the HyperPRO in “profiling mode”: First, the KLu(λ) and Rrs(λ,0+) derived from the profiles were used to simulate uncorrected Rrs(λ,0+). Then, a model of KLu(λ) applicable to uncorrected Rrs(λ,0+) was developed by doing a PLS regression of KLu(λ) on the simulated uncorrected Rrs(λ,0+). This PLS regression model, developed from the “profiling mode” data, was then applied to the “buoy mode” uncorrected Rrs(λ,0+) to provide a first-order approximation of KLu(λ) and derive corrected values of Rrs(λ,0+) for that data set. The approach presented above only provides an approximate correction. However, the differences between corrected and uncorrected Rrs(λ,0+) amounted to no more than a few percent for the stations sampled in this study, and the uncertainties linked to this correction therefore have a minimal impact on the measured Rrs(λ,0+) and especially on Rrs(λ,0+) ratios. For simplicity, in situ Rrs(λ,0+) will be further denoted in the paper as in situ Rrs.
Development of Local Chlorophyll-a Algorithms
The in situ hyperspectral Rrs spectra and simultaneously collected in situ chl-a concentrations were used to develop local blue-green band-ratio algorithms applicable to Landsat 8 OLI and Aqua MODIS. A total of 96 in situ chl-a concentrations and 49 in situ Rrs measurements were collected over the 14-week period, with 36 of these measurements coinciding in time and space for deriving the local algorithms (Table 1). Briefly, the natural log values of the measured surface chl-a concentrations were regressed on the natural log values of blue-green Rrs ratios following Equation (2):
The hyperspectral in situ Rrs facilitated the development of two different local algorithms using the respective blue and green wavebands of OLI and MODIS (Table 2). The highly correlated logarithmic relationship between in situ Rrs and in situ chl-a, including the regression coefficients (R2 = 0.90 and 0.89, respectively) and the coefficients of determination, are presented in Figure 2 and Table 2. These local algorithms were expected to perform better than the standard global, open ocean chl-a algorithms (e.g., OC2 and OC3M) for this study because of the unusual environmental conditions caused by the wastewater diversion.
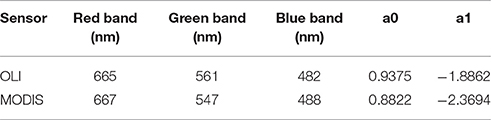
Table 2. Respective OLI and MODIS red, green, and blue band wavelengths, and derived local chl-a satellite algorithm coefficients.
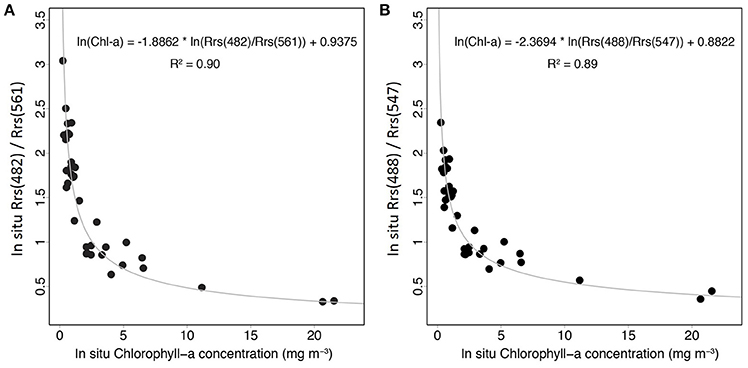
Figure 2. Local empirical relationships between in situ surface chl-a concentrations and in situ blue-green Rrs: (A) OLI (blue: 482 nm and green: 561 nm), (B) MODIS (blue: 488 nm and green: 547 nm).
Satellite Retrievals of Chlorophyll-a Concentrations
Satellite Rrs from Aqua MODIS and Landsat 8 OLI were used to monitor potential changes in chl-a concentration in Santa Monica Bay associated with the diversion (Table 1). Data from Aqua MODIS and Landsat 8 OLI were obtained for the region, before, during, and after the diversion from the Ocean Biology Distributed Active Archive Center (OB.DAAC; https://oceancolor.gsfc.nasa.gov) and the United States Geological Survey (USGS) EarthExplorer (https://earthexplorer.usgs.gov/), respectively. All satellite data were processed using the SeaWiFS Data Analysis System (SeaDAS) version 7.4 (https://oceancolor.gsfc.nasa.gov/seadas).
Aqua MODIS
Daily Aqua MODIS Level 1 radiance data were obtained from the OB.DAAC. SeaDAS was used to atmospherically correct MODIS optical radiance data, using a standard multi-scattering and iterative near infrared (NIR) model (Bailey et al., 2010) with bidirectional reflectance correction (Morel and Gentili, 1996), and generate a standard 1-km OC3M chl-a product. The 1-km chl-a product was then interpolated to a 250-m product (Franz et al., 2006) for a more detailed evaluation of the wastewater plume in the nearshore environment. The standard open-ocean OC3M model (Maritorena et al., 2002; Hu et al., 2012) was used to obtain chl-a measurements using the MODIS specific Rrs wavelengths in Table 2. Additionally, a more regional chl-a algorithm (CALFIT) developed by Kahru et al. (2012) for MODIS was used to potentially improve chl-a accuracy in Santa Monica Bay. The CALFIT algorithm was empirically derived from a large archive of in situ chl-a data for the coastal waters of the greater southern California Current system.
The local chl-a algorithm for MODIS (Table 2, Figure 2B), as well as the standard OC3M algorithm, and regional CALFIT algorithm were then applied to the MODIS-specific blue-green in situ Rrs retrievals to estimate chl-a concentrations. These values were compared to corresponding in situ chl-a measurements to determine the accuracy of each algorithm in Santa Monica Bay during the wastewater diversion. Additionally, each local and standard chl-a algorithm was then applied directly to MODIS satellite Rrs data. These remotely-sensed chl-a values were then directly compared to in situ chl-a measurements in order to determine the capability of MODIS under each algorithm in accurately detecting chl-a concentrations during the diversion. Due to its relatively coarse spatial resolution of 1 km, a single MODIS chl-a pixel value was compared to surface in situ chl-a from the corresponding station.
Landsat 8 OLI
Data from Landsat 8 OLI were obtained from the USGS EarthExplorer. The OLI radiance at the top of atmosphere data were atmospherically corrected, using the same methods described for MODIS, and processed to generate a standard OC2 chl-a product using SeaDAS (Werdell and Bailey, 2005). The local chl-a algorithm for OLI (Table 2, Figure 2A), as well as the standard OC2 OLI algorithm, were then applied to OLI-specific blue-green (Table 2) in situ Rrs retrievals to estimate chl-a concentrations. These values were compared to corresponding in situ chl-a measurements to determine the accuracy of each algorithm in Santa Monica Bay during the wastewater diversion. Additionally, each local and standard chl-a algorithm was then applied directly to OLI satellite Rrs data. These remotely-sensed chl-a values were then directly compared to in situ chl-a measurements in order to determine the capability of OLI under each algorithm in accurately detecting chl-a concentrations during the diversion. With its high spatial resolution of 30 m, a 3 × 3 pixel mean chl-a value around the sample station was compared to surface in situ chl-a from the corresponding station. A time series analysis was completed using the local chl-a algorithm for OLI in which maximum chl-a concentrations for each OLI scene were found within in Santa Monica Bay.
Landsat 8 TIRS Retrievals of Sea Surface Temperature
The effect of the surfacing wastewater plume on SST was monitored using Landsat 8 TIRS data obtained from the USGS EarthExplorer. TIRS has two longwave thermal bands, band 10 and 11 (10.3–11.3 μm and 11.5–12.5 μm, respectively), that can facilitate the retrieval of SSTs with a spatial resolution of 100 m, resampled to 30 m resolution. Stray light issues limit the applicability of the split-window algorithm traditionally used to derive SSTs from thermal imagery (Barsi et al., 2014; Montanaro et al., 2014). Therefore, we applied a single channel method developed by Malakar et al. (in preparation). The method uses physical emissivity data from ASTER GEDv3 (Hulley et al., 2015) and a radiative transfer model for atmospheric correction (Berk et al., 2005). Specifically, to estimate SST from observed thermal radiance data the measured radiance was (1) atmospherically corrected using a radiative transfer model (Berk et al., 2005), (2) the ASTER GEDv3 was spectrally adjusted to the TIRS wavebands, and (3) the temperature was retrieved by inverting the atmospherically and emissivity corrected TIRS radiances using a look-up table (Alley and Jentoft-Nilsen, 1999).
The TIRS band 10 data was used because it is less affected by the stray light issue than band 11 (Barsi et al., 2014). The correction algorithm of Montanaro et al. (2015), refined by Gerace and Montanaro (2017), was applied to the TIRS band 10 data to further reduce the effects of stray light. This stray light correction algorithm has since been implemented operationally into the Landsat Product Generation System in early 2017 by the USGS (Gerace and Montanaro, 2017), and methods are currently being developed to continually improve the accuracy of SST retrievals from the Landsat 8 TIRS. Herein, TIRS data are used solely to detect relative SST differences between plume and non-plume waters due to current limitations in TIRS-derived SST accuracy and lack of in situ skin temperature data collection during the diversion.
Additionally, MODIS Level 1 thermal infrared (TIR) radiance data were atmospherically corrected and processed to generate a standard 250-m product in SeaDAS, as described above in the Aqua MODIS section. However, given the spatially confined nature of the SST plume during the Fall 2015 diversion (Figure 3B), and the comparatively coarse spatial resolution of MODIS, MODIS TIR data were not able to detect changes in SST during the 2015 HTP wastewater diversion related to the wastewater plume (Supplemental Figure 1).
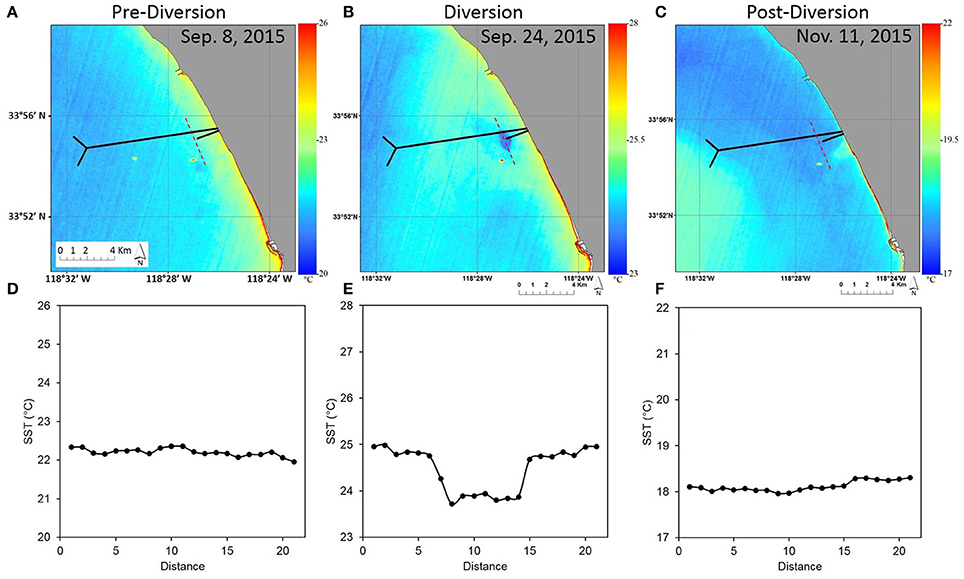
Figure 3. (A–C) Landsat 8 TIRS-derived SST in Santa Monica Bay with the 5-mile and 1-mile outfall pipes shown as black lines and a 4 km alongshore transect at the terminus of the 1-mile outfall pipe denoted by red dashed lines. (D–F) SSTs across the 4 km alongshore transect, from north to south. (A,D) SST on 8 September 2015, pre-diversion; (B,E) SST on 24 September 2015, during the diversion; (C,F) SST on 11 November 2015, after the diversion. Cooler SSTs were detected at the terminus of the 1-mile outfall pipe during the diversion, due to entrainment of cold bottom water as the wastewater plume surfaced. Pre- and post-diversion, no SST anomalies were observed at the terminus of the 1-mile outfall pipe. The warm signature of an oil tanker to the southwest of the 1-mile outfall pipe is clearly visible. SSTs uniformly decreased from September to November due to seasonal cooling, therefore each image is shown on different temperature scales.
Results
Wastewater Plume Detection via Sea Surface Temperature
The SST response to the wastewater plume was clearly detected by Landsat 8 TIRS (Figure 3). Before the diversion, on 8 September 2015, wastewater was being discharged from the 5-mile outfall pipe and no SST anomalies were detected in the vicinity of either the 5-mile or 1-mile outfall pipes (Figure 3A). Figure 3D shows no significant change in SST across an alongshore transect, shown as a dashed red line, in the vicinity of the 1-mile outfall pipe. During the diversion, on 24 September 2015, the wastewater was diverted from the 5-mile outfall pipe to the shallow (18 m depth) 1-mile outfall pipe and decreased SSTs were clearly detected at the terminus of the 1-mile outfall pipe in comparison to ambient waters (Figures 3B,E). The wastewater plume was ~1°C colder than the surrounding water (Figure 3B). The depressed SSTs at the terminus of the 1-mile outfall pipe resulted from the entrainment of colder bottom water to the surface as the buoyant wastewater plume rose (Washburn et al., 1992; Marmorino et al., 2010; Rogowski et al., 2014). This cold SST signal was absent in MODIS TIR data from the same day, due to the coarse resolution of MODIS (Supplemental Figure 1). The wastewater was diverted back to the 5-mile outfall pipe on 2 November 2015, and by 11 November 2015, no SST anomalies were observed near either outfall pipe (Figure 3C) and no significant change in SST was detected across the alongshore transect (Figure 3F). By late fall, SSTs had uniformly decreased compared to September due to seasonal cooling.
TIRS SST data clearly show the relative temperature difference between the wastewater plume and ambient waters. The observed difference was similar to those detected by MODIS Aqua and ASTER during the 2006 HTP and 2012 Orange County Sanitation District diversions (Gierach et al., 2017). In situ CTD temperature data also showed colder water temperatures in the plume compared to ambient waters. Direct comparisons to in situ data are not provided herein as TIRS SST data provide skin temperature measurements of the top few millimeters of the ocean surface, whereas in situ CTD temperature measurements were taken at depths greater than 1 m below the surface.
Wastewater Impact on chl-a in Santa Monica Bay
Satellite and In Situ Rrs Comparison
To validate satellite retrievals for use in ocean color monitoring of the wastewater diversion, OLI and MODIS Rrs at the red, green, and blue wavelengths were compared to corresponding in situ Rrs measurements (Supplemental Figures 2, 3). Respective OLI and MODIS blue-green Rrs wavelength ratios (Table 2) were compared to corresponding in situ Rrs as an initial measure of suitability for chl-a measurements in Santa Monica Bay during the diversion. Figure 4A shows OLI Rrs(482)/Rrs(561) retrievals compared to in situ measured Rrs(482)/Rrs(561). OLI and in situ Rrs values corresponded well (R2 = 0.78), with most of the satellite retrievals falling within ±30% of in situ values. The mean percent error between OLI Rrs and in situ Rrs blue-green ratio was ±19%. Figure 4B shows MODIS Rrs(488)/Rrs(547) retrievals compared to in situ measured Rrs(488)/Rrs(547). MODIS greatly overestimated the Rrs blue-green ratio by over 30%, as seen in the inset showing the full range of MODIS Rrs ratio values compared to corresponding in situ values (R2 = 0.05). The mean percent error between MODIS Rrs and in situ Rrs blue-green ratio was ±271%. OLI Rrs retrievals for each red, blue, green, wavelength (Supplemental Figure 2), as well as the blue-green ratio (Figure 4A), corresponded much more closely to in situ Rrs values than MODIS Rrs retrievals (Supplemental Figure 3 and Figure 4B), demonstrating the suitability of the higher resolution OLI sensor for the monitoring of surface chl-a in the nearshore coastal waters of Santa Monica Bay.
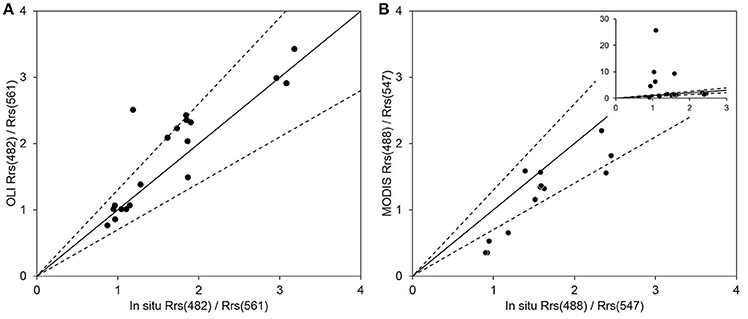
Figure 4. Comparison between in situ and satellite-derived Rrs blue-green ratios. (A) OLI Rrs(482)/Rrs(561) vs. in situ Rrs(482)/Rrs(561). (B) MODIS Rrs(488)/Rrs(547) vs. in situ Rrs(488)/Rrs(547). The inset graph in panel (B) shows the full extent of MODIS data, indicating that several nearshore reflectance ratio values are significantly overestimated, a problem that is much less pronounced for the OLI data. The solid black line is the 1:1 line. Dashed lines are ± 30% from the 1:1 line.
Local and Standard chl-a Algorithm Comparison
To demonstrate the performance capabilities of the standard OLI and MODIS chl-a algorithms (OC2 and OC3M, respectively), in comparison to the newly derived local OLI and MODIS chl-a algorithms (Figure 2), we applied the algorithms to in situ derived Rrs using respective OLI and MODIS Rrs blue-green wavelengths (Table 2) and compared the resulting estimated satellite chl-a values to corresponding surface in situ chl-a measurements (Figure 5).
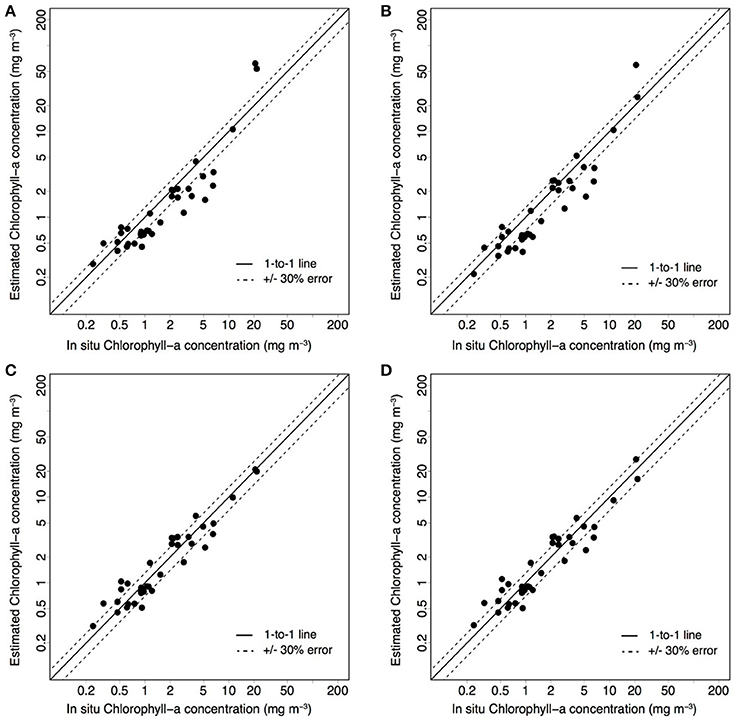
Figure 5. Algorithm performance comparison using in situ data. Comparison of estimated chl-a derived from standard and local algorithms to in situ chl-a on a log-log scale. (A) Standard OC2 chl-a algorithm applied to OLI Rrs. (B) Standard OC3M chl-a algorithm applied to MODIS Rrs. (C) Local OLI-derived chl-a algorithm applied to OLI Rrs. (D) Local MODIS-derived chl-a algorithm applied to MODIS Rrs. Standard algorithms (A,B) tend to overestimate chl-a values in respect to measured values. The local OLI chl-a algorithm (C) estimates chl-a values more accurately than the standard OC2, standard OC3M, and local MODIS algorithms. The solid black line is the 1:1 line. Dashed lines are ± 30% from the 1:1 line.
When the standard OC2 chl-a algorithm was applied to OLI in situ Rrs wavelengths, the mean percent error between the estimated chl-a values and in situ chl-a values was ±40% (Figure 5A). The standard OC3M chl-a algorithm applied to MODIS in situ Rrs wavelengths yielded a mean percent error of ±35% (Figure 5B). Applying our local OLI-derived chl-a algorithm to OLI in situ Rrs wavelengths yielded a mean percent error between the estimated chl-a values and in situ chl-a values of ±30% (Figure 5C). When our local MODIS-derived chl-a algorithm was applied to MODIS in situ Rrs wavelengths, the mean percent error between the estimated chl-a values and in situ chl-a values was ±32%. The standard algorithms tended to underestimate midlevel chl-a values and overestimate high chl-a values in respect to measured values, with the highest values falling well above the +30% error dashed line (Figures 5A,B). The local OLI chl-a algorithm (Figure 5C), with the lowest mean percent error, estimated chl-a values more accurately than the standard OC2, standard OC3M, and local MODIS algorithms.
In addition to the standard open ocean algorithms (OC2 and OC3M), we applied the Kahru et al. (2012) Aqua MODIS CALFIT chl-a algorithm, empirically derived for the regional southern California Current system, to MODIS in situ Rrs wavelengths and compared it to corresponding in situ chl-a measurements (Supplemental Figure 4). This regional chl-a algorithm more accurately estimated chl-a values than the standard OC3M algorithm, but still greatly overestimated high chl-a values with respect to measured values, and performed less accurately than our local MODIS chl-a algorithm (Figure 5).
The local and standard chl-a algorithms were applied directly to OLI and MODIS scenes from 11 November 2015, which is the only date in our time series for which there were coincident in situ chl-a and in situ Rrs measurements with Landsat 8 OLI and Aqua MODIS overpasses (Table 1). Figure 6 illustrates the differences between the local and standard chl-a algorithms for OLI and MODIS in comparison to in situ chl-a measurements at each sampling station. Comparison of in situ chl-a to remotely-sensed chl-a as detected by our local OLI chl-a algorithm (Figure 6A), standard OC2 chl-a algorithm (Figure 6B), our local MODIS chl-a algorithm (Figure 6C), and standard OC3M chl-a algorithm (Figure 6D) highlight the discrepancies in chl-a conditions depending on which sensors and algorithms are used to monitor Santa Monica Bay.
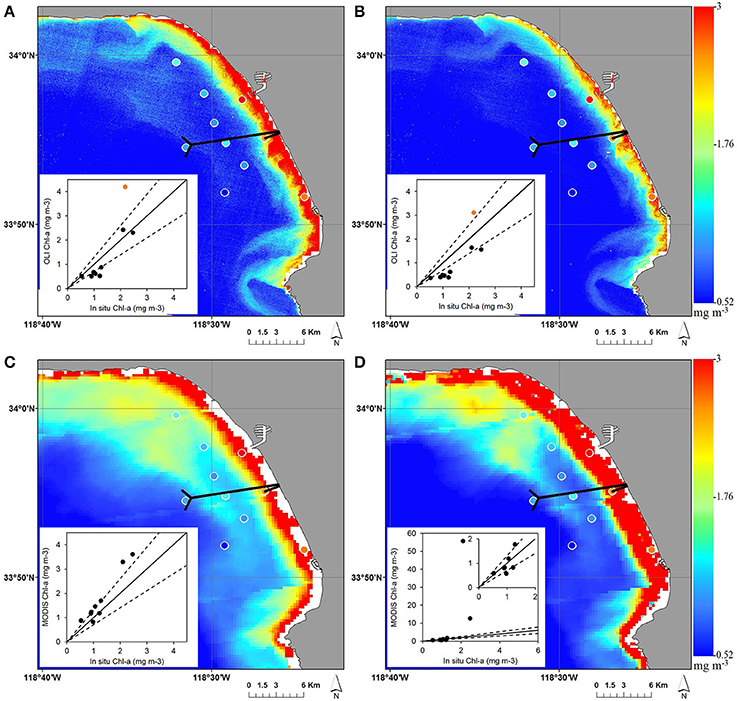
Figure 6. Maps of chl-a concentrations on 11 November 2015, overlaid with 10 corresponding in situ chl-a measurements from sampling stations (denoted as circles using the same color scale as the satellite chl-a concentrations). (A) Local OLI chl-a algorithm. (B) Standard OC2 OLI chl-a algorithm. (C) Local MODIS chl-a algorithm. (D) Standard OC3M MODIS chl-a algorithm. Inset graphs show comparison between and in situ chl-a values. Orange data points in the insets of (A,B) denote the southernmost nearshore in situ sampling location, where there is no corresponding MODIS chl-a retrieval.
On 11 November 2015, 9 days after the end of the diversion, there was no evidence of any chl-a response at either outfall pipe in association with excess nutrient availability from surfaced wastewater (Figure 6). Elevated chl-a levels were observed along the coastline, decreasing in concentration in the offshore direction. This trend is seen in both OLI and MODIS data, as well as in situ chl-a data. However, MODIS greatly overestimated chl-a concentrations, both inshore and offshore, with OC3M doing the worst job reconciling in situ chl-a concentrations. More fine scale details in chl-a distribution are visible in OLI data compared to MODIS. As seen in Figure 6, in situ chl-a samples most closely matched concentrations detected by OLI using our local chl-a algorithm. Table 3 illustrates the statistical differences between the four chl-a algorithms analyzed for OLI and MODIS and in situ chl-a concentrations from Figure 6. Nine out of 10 corresponding observations present in both OLI and MODIS retrievals were analyzed as MODIS was unable to detect the southernmost nearshore in situ sampling location due to its coarser spatial resolution and inability to fully resolve the shoreline. Overall, OLI chl-a retrievals were more accurate than MODIS. The local OLI chl-a algorithm outperformed MODIS (local and OC3M) and OC2 algorithms with a mean percent error of ~29% and the smallest RMSE of ~0.36. The standard OC2 algorithm underestimated chl-a values and had a mean percent error of ~26% and RMSE of ~0.58. Our local MODIS chl-a algorithm performed better than the OC3M algorithm, but still overestimated chl-a concentrations with a mean percent error of ~37% and RMSE of ~0.67. The standard OC3M algorithm had a mean percent error of ~349% and RMSE of ~18.2, performing an order of magnitude poorer than our local MODIS chl-a algorithm and either OLI algorithm (local or OC2).
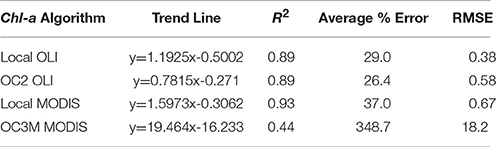
Table 3. Comparison of local and standard OLI and MODIS algorithm chl-a retrievals vs. in situ chl-a on 11 November 2015 for nine observations (see Figure 6 for observation locations).
Chlorophyll-a Evolution in Response to the Wastewater Diversion
A time series of chl-a from before, during, and after the diversion using the local OLI algorithm is shown in Figure 7. The white box denotes a coastal region of interest (ROI) most affected by changes in chl-a, analyzed in Figure 9. Pre-diversion, on 8 September 2015, elevated chl-a levels were confined along the shore of Santa Monica Bay (Figure 7A). On 24 September 2015 (3 days into the diversion) elevated chl-a levels were detected in the vicinity of the 1-mile outfall pipe and extend northward along the coastline (Figure 7B). The area of enhanced chl-a concentration near the 1-mile outfall pipe increased in spatial extent on 10 October 2015 (19 days into the wastewater diversion), extending well offshore and alongshore (Figure 7C). Elevated chl-a concentrations remained visible alongshore on 26 October 2015 (35 days into the wastewater diversion) (Figure 7D). Figure 8 shows an enlarged area view of chl-a data on 26 October 2015, in which the full extent of the chl-a bloom could be seen along the Santa Monica Bay coast wrapping around the peninsula to the south, then moving offshore and to the east. Post-diversion, chl-a concentrations returned to nominal levels along the shore of Santa Monica Bay (Figures 7E,F). Similar trends in chl-a were observed using the local MODIS algorithm (Supplemental Figures 5, 6); however, MODIS tended to overestimate chl-a concentrations and did not capture the fine scale structure shown by OLI. Additionally, MODIS was unable to resolve the shoreline as well as OLI, leaving gaps in the observed response to the diversion.
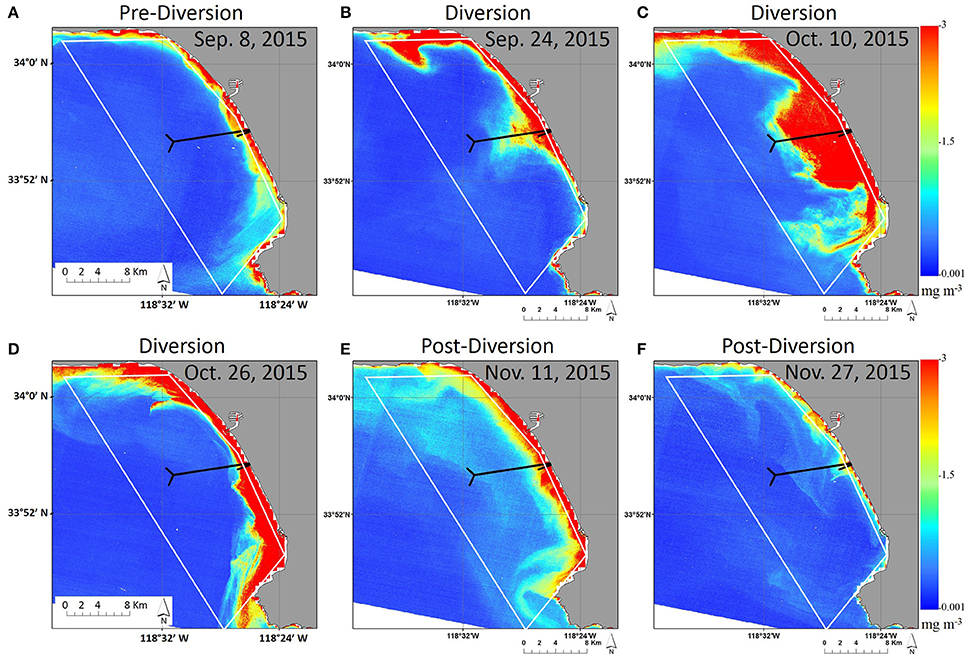
Figure 7. Time series of chl-a in Santa Monica Bay using the local OLI algorithm. The white box denotes a coastal region of interest most affected by changes in chl-a, analyzed in Figure 9. (A) 8 September 2015, pre-diversion; (B) 24 September 2015, 3 days into the diversion; (C) 10 October 2015, 19 days into the diversion; (D) 26 October 2015, 35 days into the diversion; (E) 11 November 2015, 9 days post-diversion; (F) 27 November 2015, 25 days post-diversion. During the diversion, elevated chl-a levels were detected in the vicinity of the 1-mile outfall pipe, extending offshore, and along the coastline. Values returned to nominal levels post-diversion.
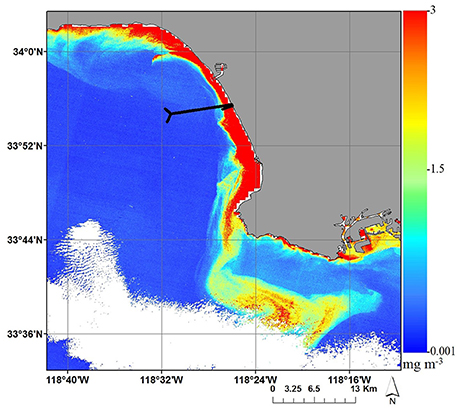
Figure 8. Larger spatial extent of chl-a distribution on 26 October 2015, using the local OLI chl-a algorithm. Elevated chl-a values were detected along the coast that continue to wrap around the peninsula to the south. A large cloud region has been masked out in the bottom of the OLI frame.
Figure 9 shows a boxplot distribution of chl-a concentration within the ROI denoted by the white box on each date of the time series shown in Figure 7.The period during the wastewater diversion is highlighted in gray. The ROI selected focuses on the coastal area most impacted by the wastewater diversion and chl-a blooms. Prior to the diversion, the maximum chl-a concentration detected by OLI in the ROI was 2.90 mg/m3. There was a low range of variability in chl-a concentration prior to the diversion. During the diversion, the maximum chl-a concentrations increased to 4.33 mg/m3 on 24 September 2015 and peaked at 9.99 mg/m3 on 10 October 2015. The small range of chl-a values in terms of the first quartile and third quartiles on 24 September 2015 can be attributed to the small spatial extent of the bloom and the high presence of low chl-a pixels. The greatest range of chl-a values occurred in the large chl-a patch in the ROI on 10 October 2015 during the diversion. The ROI does not fully encompass the chl-a bloom of 26 October 2015 (Figure 7D), in which the majority of the bloom was advected to the south, wrapping around the peninsula, as seen in Figure 8. Nevertheless, the part of the bloom captured in the ROI (Figure 7D) yielded high chl-a concentrations, with a maximum of 8.89 mg/m3. After the diversion, chl-a concentration in Santa Monica Bay declined. On 11 November 2015, the maximum chl-a concentration in the ROI was 3.90 mg/m3. By 27 November 2015, chl-a concentrations in the ROI declined to a maximum of 1.20 mg/m3, and exhibited a very low chl-a range, as illustrated by the first quartile and third quartiles, similar to 8 September 2015, prior to the diversion.
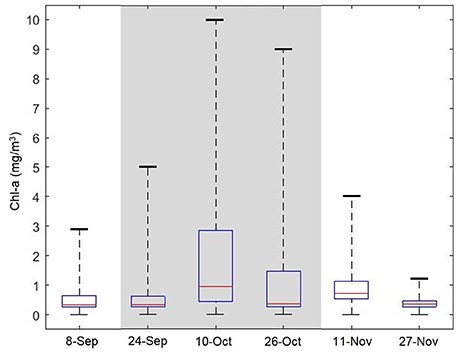
Figure 9. OLI-derived chl-a concentration range in the region-of-interest for each date of the time series shown in Figure 7. Prior to the diversion, chl-a levels were relatively low with low variability within the ROI. During the diversion, gray region, chl-a levels increased well above nominal values. Post-diversion, chl-a values returned to nominal levels.
Discussion
Utility of Landsat 8 TIRS and OLI for Coastal Water Quality Monitoring
The scheduled maintenance work by HTP, as a result of the findings from their 2006 wastewater diversion event, allowed for foresight into planning monitoring efforts for the Fall 2015 diversion. This allowed for a large collaborative effort in which the pairing of satellite remote sensing and in situ sampling created a unique opportunity, not only for monitoring crucial changes in the environment in response to the shallow discharged wastewater, but also for creating and validating new and existing satellite remote sensing chl-a algorithms with coincident, high precision in situ data. Additionally, the Fall 2015 timeframe for the diversion minimized influences of upwelling nutrients, as well as incidences of cloud cover for the Southern California Bight region, which allowed for optimal satellite acquisitions with no sunglint issues.
Our results show that high-resolution thermal and optical sensors, such as Landsat 8 TIRS and OLI, with improved high signal-to-noise ratio, can facilitate the monitoring of SST and chl-a in complex nearshore coastal environments. Like many coastal environments, the nearshore waters of Santa Monica Bay are heterogeneous, dynamic, and optically complex due to high primary production and proximity to varied and multiple terrestrial inputs. The southward flowing California Current delivers relatively cold waters into Santa Monica Bay through the Santa Barbara Channel, with flow intensifying in spring in response to large-scale upwelling (e.g., Lynn and Simpson, 1987; Bray et al., 1999; DiGiacomo and Holt, 2001). The Southern California Countercurrent (Sverdrup and Fleming, 1941) penetrates the basin alongshore, bringing relatively warm waters from the southeast. SST gradients near the coast are enhanced by the effects of local wind-driven upwelling. This upwelling of cold, nutrient-rich waters injects nitrogen into surface waters along the coast and drives increased phytoplankton production (Cullen and Eppley, 1981; Hickey, 1992; Jones et al., 2002; Nezlin and Li, 2003; Kim et al., 2009; Nezlin et al., 2012). As a result, the bay is characterized by dynamic temperature gradients and relatively high primary production year-round compared to offshore oligotrophic waters. Furthermore, nutrient inputs associated with the wastewater diversion represent an additional source of biogeochemical and optical variability to this already complex system. Therefore, incorporating high-resolution satellite sensors into the monitoring of coastal Santa Monica Bay greatly increases the ability to detect environmental change, especially during pollution-related events.
In this study, we demonstrate the utility of TIRS in resolving small scale cold water plumes associated with surfacing wastewater. The buoyant wastewater, discharged at a temperature of about 25–29°C, entrains colder deep ocean water as it rises through the water column, resulting in a cold SST anomaly at the surface. Satellite SST has been available from a number of operational and experimental satellites for over 30 years (Guan and Kawamura, 2003). Sensors such as AVHRR (1.1 km) and MODIS (1 km) are widely used in global SST retrievals. However, these sensors, with comparatively coarser spatial resolution, are inadequate for detecting thermal plumes in complex coastal waters as they are unable to resolve features finer than a few kilometers (Thomas et al., 2002; Tang et al., 2003; Ahn et al., 2006). Radiometers optimized for terrestrial applications, such as the Landsat satellite series, deliver considerably higher spatial resolution (Thomas et al., 2002). The thermal infrared bands of Landsat-5 Thematic Mapper (TM), Landsat-7 Enhanced Thematic Mapper Plus (ETM+), and Terra ASTER, have high spatial resolutions of 120, 60, and 90 m, respectively, and have been adapted for use in coastal SST retrievals (Gibbons et al., 1989; Mustard et al., 1999; Chen et al., 2003; Suga et al., 2003; Gierach et al., 2017). Landsat 8 TIRS is the latest high-resolution thermal sensor for use in coastal monitoring. In this study, we found that the spatial resolution of TIRS was fine enough to detect not only the cold surfacing wastewater plume, but also the thermal signature of an oil tanker moored just offshore from the 1-mile outfall pipe (Figure 3). TIRS has great promise for application to coastal SST studies; however, at present further refinement to TIRS SST accuracy is necessary and is an ongoing effort.
Landsat 8 OLI has been shown to outperform its predecessors (e.g., TM and ETM+) in terms of waterbody classification (Ko et al., 2015; Kim et al., 2016). Before its launch, the suitability of OLI for coastal water monitoring was demonstrated using simulated data (Gerace et al., 2013; Pahlevan and Schott, 2013), as the suite of relatively narrow spectral bands and high spatial resolution in the visible to shortwave infrared made OLI a potential tool for ocean color radiometry (Franz et al., 2015). OLI ocean color monitoring in terms of total suspended solids was first analyzed by Vanhellemont and Ruddick (2014), in which they demonstrated the enhanced accuracy of OLI compared to dedicated wide-swath ocean color instruments such as MODIS, as well as TM and ETM+. Compared to its predecessors, OLI offers higher signal-to-noise ratios, due mainly to longer integration times on the push-broom scanner, better quantization due to 12-bit radiometric resolution, and the addition of a band centered at 443 nm (Knight and Kvaran, 2014; Pahlevan et al., 2014). The presence of the high-quality SWIR bands on Landsat 8 facilitates more accurate quantification of aerosol contribution to the top of atmosphere radiance over very turbid waters, enabling more accurate remote sensing of ocean color and water quality monitoring (Franz et al., 2015; Garaba and Zielinski, 2015; Vanhellemont and Ruddick, 2015). These enhanced features of OLI allow for a more lucid characterization of chl-a and CDOM in open ocean and coastal waters (Franz et al., 2015). In Franz et al. (2015), standard chl-a algorithm coefficients were tuned for OLI using the NASA Bio-Optical Marine Algorithm Dataset (NOMAD) but no OLI data or coincident in situ measurements were used in the algorithm development. Here, we expand on the demonstrated applications of Landsat imagery in marine environments by validating the utility of OLI for coastal chl-a measurements as an indicator of changing water quality due to anthropogenic pollution with coincident in situ measurements of both Rrs and surface chl-a.
This study demonstrates the improved accuracy of chl-a from high-resolution OLI in comparison to coarser resolution MODIS for coastal chl-a detection, wherein the high-resolution of OLI enables detection of a large range of chl-a values found for a single MODIS pixel (Figure 4). Moreover, we show that the application of our local chl-a algorithms, developed using in situ chl-a and in situ Rrs measurements, improves chl-a retrievals in Santa Monica Bay during the 2015 HTP wastewater diversion in comparison to the standard open ocean algorithms (i.e., OC2 and OC3M) (Figure 5), and the regional CALFIT algorithm (Kahru et al., 2012; Supplemental Figure 4). The OC3M and OC2 algorithms are empirical algorithms developed for global applications and have been shown to overestimate chl-a in coastal environments, in part due to increased concentrations of other optical constituents (e.g., Muller-Karger et al., 2005). Within optically complex coastal waters such as Santa Monica Bay, CDOM, sediments, bottom reflectance (Maritorena et al., 1994; Cannizzaro and Carder, 2006), land adjacency effects (Santer and Schmechtig, 2000), and urban and absorbing aerosols (Moulin et al., 2001; Claustre et al., 2002; Ransibrahmanakul and Stumpf, 2006) can all interfere with the accurate detection of chl-a. This challenge is potentially exacerbated during the unusual conditions created by the wastewater diversion. The wastewater diversion increased the concentration of optical constituents in the water. Prior to the diversion, the beam attenuation coefficient at 650 nm was 0.89 m−1 at 1 meter depth at the sampling station at the terminus of the 1-mile outfall pipe. At the same sampling station, this increased to 1.78 m−1 during the wastewater diversion event. After the diversion ended, the beam attenuation coefficient decreased to 1.08 m−1. Additionally, the absorption coefficient of CDOM at 400 nm prior to the diversion at the same sampling station was 0.29 m−1. During the diversion, it increased to 0.57 m−1. After the diversion, it decreased to 0.12 m−1. Here, the development and application of our local OLI chl-a algorithm clearly improved chl-a retrievals relative to the standard OC2 and OC3M retrievals. Overall, our local OLI chl-a algorithm provided the best assessment of change in surface chl-a concentrations related to the 2015 HTP wastewater diversion.
Environmental Impacts of the 2015 HTP Wastewater Diversion
High-resolution TIRS and OLI were used to monitor two major environmental impacts of the Fall 2015 HTP wastewater diversion, SST and chl-a. Changes in SST were spatially limited to cold plumes near the terminus of the 1-mile outfall pipe, before mixing with warmer ambient surface water. The cold SST anomaly provides a physical indicator of whether or not the discharged wastewater has risen to the surface and provides some indication of its transport direction. The surfacing wastewater introduces excess nutrients, mainly ammonium, to the euphotic zone, where it has been shown to stimulate phytoplankton growth and production in the region (Reifel et al., 2013; Howard et al., 2014; Caron et al., 2017; Gierach et al., 2017).
During the diversion event, cold water plumes were detected by TIRS (Figure 3) and in situ measurements (not shown) at the terminus of the 1-mile outfall pipe, indicating that, throughout the diversion, the fresh wastewater plume consistently reached the surface, entraining cold bottom water with it as it rose through the water column. Pre- and post-diversion, when wastewater was discharged through the 5-mile outfall pipe, no SST anomalies were seen in the vicinity of either the 1-mile or 5-mile outfall pipes. The 5-mile outfall pipe terminates at 57 m depth, allowing the discharged wastewater to mix more thoroughly with ambient seawater and largely remain at depth in association with density stratification (Washburn et al., 1992; Uchiyama et al., 2014). TIRS data additionally shows warmer SSTs along the coast, cooling in the offshore direction, and an overall decrease in SST from September to November (Figure 3). These trends are consistent with the inshore-offshore temperature gradient and seasonally driven temperature decrease characteristic of the coastal Santa Monica Bay region (Corcoran and Shipe, 2011).
In the Southern California Bight, like many other coastal waters dominated by seasonal upwelling, nitrogen is generally the limiting nutrient for phytoplankton growth (Capone and Hutchins, 2013). Nitrate is the dominant form of nitrogen brought to surface waters via upwelling. Ammonium is overwhelmingly the dominant form of nitrogen (92%) in secondarily-treated wastewater discharged into the Southern California Bight (Howard et al., 2014). In Santa Monica Bay, the flux of nitrogen from upwelling and treated wastewater are about equal (102 × 102 kg N km−2 y−1 and 99 × 102 kg N km−2 y−1, respectively), comprising over 95% of the total nitrogen flux for the region (Howard et al., 2017). During normal operations, treated wastewater is discharged from the 5-mile outfall pipe at the head of a submarine canyon that quickly drops off to over 100 m depth (Figure 1), allowing for dilution and mixing and limiting the impact on the near-surface phytoplankton community. During the diversion, there was major concern not only in regards to the magnitude of nitrogen being released into surface waters, but also in regards to the species of the nitrogen being released (Howarth and Marino, 2006) as it may cause shifts in phytoplankton community structure due to different nutrient preferences among algal taxa (Howard et al., 2007; Ryan et al., 2017). Ammonium is the most biologically available form of nitrogen and is usually present in the euphotic zone in limited concentrations because it is assimilated so quickly. Therefore, such a large release of ammonium, specifically in the fall, a time in which nutrients tend to be limited, can rapidly stimulate phytoplankton blooms in this area. The preferential stimulation of harmful algal blooms was a particular concern as anthropogenic nutrients have been shown to be a factor in the increased occurrence of these outbreaks (Anderson et al., 2002; Heisler et al., 2008; Kudela et al., 2008). The increased frequency and duration of phytoplankton blooms related to anthropogenic nutrient loading have also coincided with increased incidents of eutrophication of coastal waters over the last several decades (Howarth, 2008; Paerl and Piehler, 2008).
During the 6-week 2015 HTP wastewater diversion, ~1.61 × 106 kg of ammonium was released into the shallow coastal waters of Santa Monica Bay. This amount of nitrogen loading was likely to have greatly altered the composition of the nitrogen pool available to phytoplankton populations. With these concerns in mind, the main goal of this study was to use high-resolution satellite remote sensing to monitor changes in the biological response through chl-a concentrations in surface waters during the wastewater diversion. Given the immense amount of ammonium introduced into surface waters, the observed increase in chl-a during the diversion in Santa Monica Bay was not surprising (Figure 7). The maximum chl-a level during the diversion in the ROI, using our local OLI chl-a algorithm, was 9.99 mg/m3 on 10 October 2015 (Figure 9), far exceeding the nominal chl-a value of 1.7 ± 0.33 mg/m3 measured in situ (Corcoran and Shipe, 2011) and 0.61 mg/m3 remotely-sensed by SeaWiFS (Nezlin and Li, 2003) for the same region and time of year. The chl-a enhancement seen during the 2015 HTP wastewater diversion was larger than that seen during the 2006 HTP diversion (Reifel et al., 2013; Gierach et al., 2017), not only in terms of concentration but also in terms of spatial extent. This can most likely be attributed to the much longer duration of the 2015 diversion (6 weeks as opposed to 3 days in 2006).
Prior to the diversion, the maximum chl-a concentration measured by OLI, was 2.90 mg/m3 (Figure 9). After the diversion event, on 27 November 2015, the maximum chl-a concentration measured by OLI was 1.20 mg/m3. These maximum chl-a values were found in the most nearshore region of Santa Monica Bay. The general inshore-offshore gradient in chl-a detected before and after the diversion is characteristic of the Southern California Bight, with higher values of chl-a observed at shallower depths (Kim et al., 2009; Corcoran and Shipe, 2011; Gierach et al., 2017). However, during the diversion, high chl-a values, were found further offshore and away from the terminus of the 1-mile outfall pipe (Figures 7B–D). As exemplified in Figure 8 and Supplemental Figure 6, the effects of the wastewater diversion were not confined to the nearshore region. Given the complex nature of currents and wind in the region, as demonstrated by models of current velocity and direction, as well as by tracks of experimental drifters placed in the water near the 1-mile outfall pipe (City of Los Angeles, Environmental Monitoring Division, 2017), and the volume of wastewater being discharged, the nutrient- and organic matter-rich wastewater can be exported offshore into the more oligotrophic region of Santa Monica Bay where it can fuel primary production and heterotrophic processes in areas that may not commonly experience such conditions during the fall (Boehm et al., 2002; Landry et al., 2009). Fortunately, testing in areas with enhanced chl-a as identified by OLI found that no algal blooms produced harmful toxins during the wastewater diversion (City of Los Angeles, Environmental Monitoring Division, 2017).
Satellite chl-a data clearly show the spatial contrast between productive coastal waters and oligotrophic offshore waters along the coast of California (Eppley, 1992; Thomas et al., 1994; Legaard and Thomas, 2006). However, as noted in previous studies (Kahru and Mitchell, 1999; Darecki and Stramski, 2004; Kim et al., 2009; Kahru et al., 2012; Gierach et al., 2017), satellite chl-a data in very nearshore waters have systematic and variable errors due to the proximity of land (e.g., adjacency effects, breaking waves) and due to the optical complexity of the waters caused by varying concentrations of CDOM and inorganic suspended particles, and bottom reflectance. Errors due to proximity of land appeared to be less pronounced in high-resolution Landsat 8 OLI (30 m) compared to MODIS. The newly derived local chl-a algorithm, tuned for the optically complex nearshore environment of Santa Monica Bay, helped reduce the discrepancy between satellite chl-a and in situ chl-a.
In this study, we demonstrated the enhanced performance of TIRS and OLI for detecting mesoscale nearshore features, in terms of SST and surface chl-a concentrations. Although TIRS and OLI show great promise for monitoring water quality indicators in coastal environments, Landsat 8 has a repeat cycle of 16 days. This infrequent coverage yielded a limited number of satellite overpasses during a diversion event and did not capture the complete biophysical response to the wastewater diversion event. Phytoplankton blooms and die-offs were captured by in situ monitoring on timescales less than the 16-day repeat cycle. In contrast to Landsat 8, MODIS has a coarser spatial resolution but daily repeat cycle. In the future, coastal water quality monitoring efforts can employ additional high-resolution sensors with improved temporal resolutions, such as the two Sentinel-2 MultiSpectral Instruments (MSI) with a 5-day repeat cycle and Landsat-9, predicted to launch in December 2020, with an 8-day offset from Landsat 8 repeat cycle, to capture the environmental response over greater temporal resolution. This study was the first to use high-resolution Landsat 8 TIRS and OLI for coastal water quality monitoring of wastewater diversions. By employing satellite monitoring in concert with in situ sampling, we were able to develop local empirical chl-a algorithms for both OLI and MODIS to gain an improved understanding of the biophysical dynamics occurring within Santa Monica Bay during a wastewater diversion event.
Author Contributions
BH and MG were the principal investigators for the study, and along with RT and CF, helped conceive, plan, and implement the study. RT and CF collected in situ remote sensing reflectance data each week. CF processed the in situ remote sensing reflectance data. RT obtained and processed satellite ocean color data and wrote the bulk of the manuscript with edits from co-authors. NM and GH helped process Landsat sea surface temperature data. JS collected and processed in situ chlorophyll samples used in this study.
Conflict of Interest Statement
The authors declare that the research was conducted in the absence of any commercial or financial relationships that could be construed as a potential conflict of interest.
Acknowledgments
This work was performed at the Jet Propulsion Laboratory, California Institute of Technology, under contract with the National Aeronautics and Space Administration. The NASA DEVELOP program (http://develop.larc.nasa.gov/) provided the primary support for this project with additional support provided by the Physical Oceanography Distributed Active Archive Center. The authors would like to acknowledge the earlier contributions of Mark Barker and Lindsay Amaleh, who performed preliminary analysis of the satellite data collected during the 2015 HTP diversion. We would also like to acknowledge Matt Montanaro for his assistance in applying the stray-light correction to our TIRS data. Finally, we would like to acknowledge our project partners for assistance in the field sample collection, instrument deployment, and inclusion in the 2015 HTP diversion monitoring program: Curtis Cash and Mas Dojiri of the City of Los Angeles Environmental Monitoring Division, as well as the very helpful captain and crew of the R/V La Mer and R/V Surveyor.
Supplementary Material
The Supplementary Material for this article can be found online at: https://www.frontiersin.org/articles/10.3389/fmars.2017.00329/full#supplementary-material
References
Ahn, Y. H., Shanmugam, P., Lee, J. H., and Kang, Y. Q. (2006). Application of satellite infrared data for mapping of thermal plume contamination in coastal ecosystem of Korea. Mar. Environ. Res. 61, 186–201. doi: 10.1016/j.marenvres.2005.09.001
Alley, R., and Jentoft-Nilsen, M. (1999). Algorithm Theoretical Basis Document for: Brightness Temperature. Pasadena, CA: Jet Propulsion Lab., California Inst. of Tech.
Anderson, D. M., Glibert, P. M., and Burkholder, J. M. (2002). Harmful algal blooms and eutrophication: nutrient sources, composition, and consequences. Estuaries 25, 704–726. doi: 10.1007/BF02804901
Austin, R. W. (1974). “The remote sensing of spectral radiance from below the ocean surface,” in Optical Aspects of Oceanography, eds N. G. Jerlov and E. S. Nielsen (London: Academic Press), 317–344.
Bailey, S. W., Franz, B. A., and Werdell, P. J. (2010). Estimation of near-infrared water-leaving reflectance for satellite ocean color data processing. Opt. Express 18, 7521–7527. doi: 10.1364/OE.18.007521
Barsi, J. A., Schott, J. R., Hook, S. J., Raqueno, N. G., Markham, B. L., and Radocinski, R. G. (2014). Landsat-8 Thermal Infrared Sensor (TIRS) vicarious radiometric calibration. Remote Sens. 6, 11607–11626. doi: 10.3390/rs61111607
Bay, S., Jones, B. H., Schiff, K., and Washburn, L. (2003). Water quality impacts of stormwater discharges to Santa Monica Bay. Mar. Environ. Res. 56, 205–223. doi: 10.1016/S0141-1136(02)00331-8
Berk, A., Anderson, G. P., Acharya, P. K., Bernstein, L. S., Muratov, L., Lee, J., et al. (2005). “MODTRANTM 5, a reformulated atmospheric band model with auxiliary species and practical multiple scattering options: update,” in Algorithms and Technologies for Multispectral, Hyperspectral, and Ultraspectral Imagery XI, eds S. S. Sylvia and P. E. Lewis (Bellingham, WA: Proceedings of SPIE).
Boehm, A. B., Sanders, B. F., and Winant, C. D. (2002). Cross-shelf transport at Huntington Beach. Implications for the fate of sewage discharged through an offshore ocean outfall. Environ. Sci. Technol. 36, 1899–1906. doi: 10.1021/es0111986
Bray, N. A., Keyes, A., and Morawitz, W. M. L. (1999). The California current system in the southern California bight and the Santa Barbara Channel. J. Geophys. Res. Oceans 104, 7695–7714. doi: 10.1029/1998JC900038
Cannizzaro, J. P., and Carder, K. L. (2006). Estimating chlorophyll a concentrations from remote-sensing reflectance in optically shallow waters. Remote Sens. Environ. 101, 13–24. doi: 10.1016/j.rse.2005.12.002
Capone, D. G., and Hutchins, D. A. (2013). Microbial biogeochemistry of coastal upwelling regimes in a changing ocean. Nat. Geosci. 6, 711–717. doi: 10.1038/ngeo1916
Caron, D. A., Gellene, A. G., Smith, J., Seubert, E. L., Campbell, V., Sukhatme, G. S., et al. (2017). Response of phytoplankton and bacterial biomass during a wastewater effluent diversion into nearshore coastal waters. Estuar. Coast. Shelf Sci. 186, 223–236. doi: 10.1016/j.ecss.2015.09.013
Chen, C., Shi, P., and Mao, Q. (2003). Application of remote sensing techniques for monitoring the thermal pollution of cooling-water discharge from nuclear power plant. J. Environ. Sci. Heal. A, A38, 1659–1668. doi: 10.1081/ESE-120021487
City of Los Angeles, Environmental Monitoring Division. (2009). 2006 Hyperion Receiving Water Monitoring for Diversion of 5-Mile Discharge to 1-Mile Pipe during the 5-Mile Pipe Inspection. California, CA: Department of Public Works, Bureau of Sanitation, Hyperion Treatment Plant, Playa del Rey.
City of Los Angeles, Environmental Monitoring Division. (2017). Fall 2015 Hyperion Treatment Plant Effluent Diversion to the 1-Mile Outfall Comprehensive Monitoring Program Final Report. Environmental Monitoring Division, Bureau of Sanitation, Department of Public Works, City of Los Angeles, pp. 160 + appendicies.
Claustre, H., Morel, A., Hooker, S. B., Babin, M., Antoine, D., Oubelkheir, K., et al. (2002). Is desert dust making oligotrophic waters greener? Geophys. Res. Lett. 29, 107-1–107-4. doi: 10.1029/2001GL014056
Corcoran, A. A., and Shipe, R. F. (2011). Inshore–offshore and vertical patterns of phytoplankton biomass and community composition in Santa Monica Bay, CA (USA). Estuar. Coast. Shelf Sci. 94, 24–35. doi: 10.1016/j.ecss.2011.05.007
Creel, L. (2003). Ripple Effects: Population and Coastal Regions. Washington, DC: Population Reference Bureau.
Crossett, K. M., Culliton, T. J., Wiley, P. C., and Goodspeed, T. R. (2004). Population Trends Along the Coastal United States: 1980−2008. Coastal Trends Report Series. NOAA, National Oceanic Service Management Budget Off-Spec Project, Silver Spring, MD.
Cullen, J. J., and Eppley, R. W. (1981). Chlorophyll maximum layers of the Southern-California Bight and possible mechanisms of their formation and maintenance. Oceanol. Acta. 4, 23–32.
Darecki, M., and Stramski, D. (2004). An evaluation of MODIS and SeaWiFS bio-optical algorithms in the Baltic Sea. Remote Sens. Environ. 89, 326–350. doi: 10.1016/j.rse.2003.10.012
DiGiacomo, P. M., and Holt, B. (2001). Satellite observations of small coastal ocean eddies in the Southern California Bight. J. Geophys. Res. 106, 22521–22543. doi: 10.1029/2000JC000728
DiGiacomo, P. M., Washburn, L., Holt, B., and Jones, B. H. (2004). Coastal pollution hazards in southern California observed by SAR imagery: stormwater plumes, wastewater plumes, and natural hydrocarbon seeps. Mar. Pollut. Bull. 49, 1013–1024. doi: 10.1016/j.marpolbul.2004.07.016
Eganhouse, R. P., and Venkatesan, M. I. (1993). “Chemical oceanography and geochemistry,” in Ecology of the Southern California Bight: A Synthesis and Interpretation, eds M. D. Dailey, D. J. Reish, and J. W. Anderson (Berkeley, CA: University of California Press), 71–189.
Eppley, R. W. (1992). Chlorophyll, photosynthesis and new production in the Southern California Bight. Prog. Oceanogr. 30, 117–150. doi: 10.1016/0079-6611(92)90010-W
Franz, B. A., Bailey, S. W., Kuring, N., and Werdell, P. J. (2015). Ocean color measurements with the Operational Land Imager on Landsat-8: implementation and evaluation in SeaDAS. J. Appl. Remote Sens. 9:096070. doi: 10.1117/1.JRS.9.096070
Franz, B. A., Bailey, S. W., Werdell, P. J., and McClain, C. R. (2007). Sensor-independent approach to the vicarious calibration of satellite ocean color radiometry. Appl. Opt. 46, 5068–5082. doi: 10.1364/AO.46.005068
Franz, B. A., Werdell, P. J., Meister, G., Kwiatkowska, E. J., Bailey, S. W., Ahmad, Z., et al. (2006). “MODIS land bands for Ocean Remote sensing applications,” in Proceedings of Ocean Optics XVIII (Montreal, QC), 9–13.
Garaba, S. P., and Zielinski, O. (2015). An assessment of water quality monitoring tools in an estuarine system. Remote Sens. Appl. Soc. Environ. 2, 1–10. doi: 10.1016/j.rsase.2015.09.001
Gerace, A. D., Schott, J. R., and Nevins, R. (2013). Increased potential to monitor water quality in the near-shore environment with Landsat's next-generation satellite. J. Appl. Remote Sens. 7, 73558–73558. doi: 10.1117/1.JRS.7.073558
Gerace, A., and Montanaro, M. (2017). Derivation and validation of the stray light correction algorithm for the thermal infrared sensor onboard Landsat 8. Remote Sens. Environ. 191, 246–257. doi: 10.1016/j.rse.2017.01.029
Gibbons, D. E., Wukelic, G. E., Leighton, J. P., and Doyle, M. J. (1989). Application of Landsat thematic mapper data for coastal thermal plume analysis at Diablo Canyon. Photogr. Eng. Rem. Sens. 55, 903–909.
Gierach, M., Holt, B., Trinh, R., Pan, B., and Rains, C. (2017). Satellite detection of wastewater diversion plumes in Southern California. Estuar. Coast. Shelf Sci. 186, 171–182. doi: 10.1016/j.ecss.2016.10.012
Guan, L., and Kawamura, H. (2003). SST availabilities of satellite infrared and microwave measurements. J. Oceanogr. 59, 201–209. doi: 10.1023/A:1025543305658
Heisler, J., Glibert, P., Burkholder, J., Anderson, D., Cochlan, W., Dennison, W., et al. (2008). Eutrophication and harmful algal blooms: a scientific consensus. Harmful Algae 8, 3–13. doi: 10.1016/j.hal.2008.08.006
Hickey, B. M. (1992). Circulation over the Santa Monica-San Pedro basin and shelf. Prog. Oceanogr. 30, 37–115. doi: 10.1016/0079-6611(92)90009-O
Hickey, B. M., Dobbins, E. L., and Allen, S. E. (2003). Local and remote forcing of currents and temperature in the central Southern California Bight. J. Geophys. Res. 108:3081. doi: 10.1029/2000JC000313
Holt, B., Trinh, R., and Gierach, M. M. (2017). Stormwater runoff plumes in the Southern California Bight: a comparison study with SAR and MODIS imagery. Mar. Pollut. Bull. 118, 141–154. doi: 10.1016/j.marpolbul.2017.02.040
Howard, M. D. A., Cochlan, W. P., Ladizinsky, N., and Kudela, R. M. (2007). Nitrogenous preference of toxigenic Pseudo-nitzschia australis (Bacillariophyceae) from field and laboratory experiments. Harmful Algae 6, 206–217. doi: 10.1016/j.hal.2006.06.003
Howard, M. D. A., Kudela, R. M., and McLaughlin, K. (2017). New insights into impacts of anthropogenic nutrients on urban ecosystem processes on the Southern California coastal shelf: introduction and synthesis. Estuarine Coast. Shelf Sci. 186, 163–170. doi: 10.1016/j.ecss.2016.06.028
Howard, M. D. A., Sutula, M., Caron, D. A., Chao, Y., Farrara, J. D., Frenzel, H., et al. (2014). Anthropogenic nutrient sources rival natural sources on small scales in the coastal waters of the Southern California Bight. Limnol. Oceanogr. 59, 285–297. doi: 10.4319/lo.2014.59.1.0285
Howarth, R. W. (2008). Coastal nitrogen pollution: a review of sources and trends globally and regionally. Harmful Algae 8, 14–20. doi: 10.1016/j.hal.2008.08.015
Howarth, R. W., and Marino, R. (2006). Nitrogen as the limiting nutrient for eutrophication in coastal marine ecosystems: evolving views over three decades. Limnol. Oceanogr. 51, 364–376. doi: 10.4319/lo.2006.51.1_part_2.0364
Hu, C., Chen, Z., Clayton, T. D., Swarzenski, P., Brock, J. C., and Muller-Karger, F. E. (2004). Assessment of estuarine water-quality indicators using MODIS medium-resolution bands: initial results from Tampa Bay, FL. Remote Sens. Environ. 93, 423–441. doi: 10.1016/j.rse.2004.08.007
Hu, C., Lee, Z., and Franz, B. (2012). Chlorophyll a algorithms for oligotrophic oceans: a novel approach based on three-band reflectance difference. J. Geophys. Res. 117, C01011. doi: 10.1029/2011JC007395
Hulley, G. C., Hook, S. J., Abbott, E., Malakar, N., Islam, T., and Abrams, M. (2015). The ASTER Global Emissivity Dataset (ASTER GED): mapping earth's emissivity at 100 meter spatial scale. Geophys. Res. Lett. 42, 7966–7976. doi: 10.1002/2015GL065564
Jones, B. H., Noble, M. A., and Dickey, T. D. (2002). Hydrographic and particle distributions over the Palos Verdes Continental Shelf: spatial, seasonal and daily variability. Cont. Shelf Res. 22, 945–965. doi: 10.1016/S0278-4343(01)00114-5
Kahru, M., Kudela, R. M., Manzano-Sarabia, M., and Mitchell, B. G. (2012). Trends in the surface chlorophyll of the California Current: merging data from multiple ocean color satellites. Deep Sea Res. II Top. Stud. Oceanog. 77, 89–98. doi: 10.1016/j.dsr2.2012.04.007
Kahru, M., and Mitchell, B. G. (1999). Empirical chlorophyll algorithm and preliminary SeaWiFS validation for the California Current. Int. J. Remote Sens. 20, 3423–3429. doi: 10.1080/014311699211453
Kim, H. H., Ko, B. C., and Nam, J. Y. (2016). Predicting chlorophyll-a using Landsat 8 OLI sensor data and the non-linear RANSAC method–a case study of Nakdong River, South Korea. Int. J. Remote Sens. 37, 3255–3271. doi: 10.1080/01431161.2016.1196839
Kim, H. J., Miller, A. J., McGowan, J., and Carter, M. L. (2009). Coastal phytoplankton blooms in the Southern California Bight. Prog. Oceanogr. 82, 137–147. doi: 10.1016/j.pocean.2009.05.002
Knight, E. J., and Kvaran, G. (2014). Landsat-8 operational land imager design, characterization and performance. Remote Sens. 6, 10286–10305. doi: 10.3390/rs61110286
Ko, B. C., Kim, H. H., and Nam, J. Y. (2015). Classification of potential water bodies using Landsat 8 OLI and a combination of two boosted random forest classifiers. Sensors 15, 13763–13777. doi: 10.3390/s150613763
Kudela, R. M., Ryan, J. P., Blakely, M. D., Lane, J. Q., and Peterson, T. D. (2008). Linking the physiology and ecology of Cochlodinium to better understand harmful algal bloom events: a comparative approach. Harmful Algae 7, 278–292. doi: 10.1016/j.hal.2007.12.016
Landry, M. R., Ohman, M. D., Goericke, R., Stukel, M. R., and Tsyrklevich, K. (2009). Lagrangian studies of phytoplankton growth and grazing relationships in a coastal upwelling ecosystem off Southern California. Prog. Oceanogr. 83, 208–216. doi: 10.1016/j.pocean.2009.07.026
Legaard, K. R., and Thomas, A. C. (2006). Spatial patterns in seasonal and interannual variability of chlorophyll and sea surface temperature in the California Current. J. Geophys. Res. 111, C06032. doi: 10.1029/2005JC003282
Lynn, R. J., and Simpson, J. J. (1987). The California Current System: the seasonal variability of its physical characteristics. J. Geophys. Res. Oceans 92, 12947–12966. doi: 10.1029/JC092iC12p12947
Lyon, G. S., and Stein, E. D. (2009). How effective has the Clean Water Act been at reducing pollutant mass emissions to the Southern California Bight over the past 35 years? Environ. Monit. Assess. 154, 413–426. doi: 10.1007/s10661-008-0408-1
Maritorena, S., Morel, A., and Gentili, B. (1994). Diffuse reflectance of oceanic shallow waters: influence of water depth and bottom albedo. Limnol. Oceanogr. 39, 1689–1703. doi: 10.4319/lo.1994.39.7.1689
Maritorena, S., Siegel, D. A., and Peterson, A. R. (2002). Optimization of a semi analytical ocean color model for global-scale applications. Appl. Opt. 41, 2705–2714. doi: 10.1364/AO.41.002705
Marmorino, G. O., Smith, G. B., Miller, W. D., and Bowles, J. H. (2010). Detection of a buoyant coastal wastewater discharge using airborne hyperspectral and infrared imagery. J. Appl. Remote Sens. 4:043502. doi: 10.1117/1.3302630
McClain, C. R. (2009). A decade of satellite ocean color observations. Ann. Rev. Mar. Sci. 1, 19–42. doi: 10.1146/annurev.marine.010908.163650
McKinney, M. L. (2002). Urbanization, biodiversity, and conservation. Bioscience 52, 883–890. doi: 10.1641/0006-3568(2002)052[0883:UBAC]2.0.CO;2
McLaughlin, K., Nezlin, N. P., Howard, M. D., Beck, C. D., Kudela, R. M., Mengel, M. J., et al. (2017). Rapid nitrification of wastewater ammonium near coastal ocean outfalls, Southern California, USA. Estuar. Coast. Shelf Sci. 186, 263–275. doi: 10.1016/j.ecss.2016.05.013
Montanaro, M., Gerace, A., Lunsford, A., and Reuter, D. (2014). Stray light artifacts in imagery from the landsat 8 thermal infrared sensor. Remote Sens. 6, 10435–10456. doi: 10.3390/rs61110435
Montanaro, M., Gerace, A., and Rohrbach, S. (2015). Toward an operational stray light correction for the Landsat 8 thermal infrared sensor. Appl. Opt. 54, 3963–3978. doi: 10.1364/AO.54.003963
Morel, A., and Gentili, B. (1996). Diffuse reflectance of oceanic waters. III. Implication of bidirectionality for the remote-sensing problem. Appl. Opt. 35, 4850–4862. doi: 10.1364/AO.35.004850
Moulin, C., Gordon, H. R., Chomko, R. M., Banzon, V. F., and Evans, R. H. (2001). Atmospheric correction of ocean color imagery through thick layers of Saharan dust. Geophys. Res. Lett. 28, 5–8. doi: 10.1029/2000GL011803
Mouw, C. B., Greb, S., Aurin, D., DiGiacomo, P. M., Lee, Z., Twardowski, M., et al. (2015). Aquatic color radiometry remote sensing of coastal and inland waters: challenges and recommendations for future satellite missions. Remote Sens. Environ. 160, 15–30. doi: 10.1016/j.rse.2015.02.001
Muller-Karger, F. E., Hu, C., Andréfouët, S., Varela, R., and Thunell, R. (2005). “The color of the coastal ocean and applications in the solution of research and management problems,” in Remote Sensing of Coastal Aquatic Environments, eds R. L. Miller, C. E. Del Castillo, and B. A. McKee (Dordrecht: Springer), 101–127.
Mustard, J. F., Carney, M. A., and Sen, A. (1999). The use of satellite data to quantify thermal effluent impacts. Estur. Coast. Shelf S. 49, 509–524. doi: 10.1006/ecss.1999.0517
Nezlin, N. P., and DiGiacomo, P. M. (2005). Satellite ocean color observations of stormwater runoff plumes along the San Pedro Shelf (southern California) during 1997–2003. Cont. Shelf Res. 25, 1692–1711. doi: 10.1016/j.csr.2005.05.001
Nezlin, N. P., DiGiacomo, P. M., Diehl, D. W., Jones, B. H., Johnson, S. C., Mengel, M. J., et al. (2008). Stormwater plume detection by MODIS imagery in the southern California coastal ocean. Estuar. Coast. Shelf Sci. 80, 141–152. doi: 10.1016/j.ecss.2008.07.012
Nezlin, N. P., DiGiacomo, P. M., Stein, E. D., and Ackerman, D. (2005). Stormwater runoff plumes observed by SeaWiFS radiometer in the Southern California Bight. Remote Sens. Environ. 98, 494–510. doi: 10.1016/j.rse.2005.08.008
Nezlin, N. P., and Li, B. L. (2003). Time-series analysis of remote-sensed chlorophyll and environmental factors in the Santa Monica–San Pedro Basin off Southern California. J. Mar. Syst. 39, 185–202. doi: 10.1016/S0924-7963(03)00030-7
Nezlin, N. P., Sutula, M. A., Stumpf, R. P., and Sengupta, A. (2012). Phytoplankton blooms detected by SeaWiFS along the central and southern California coasts. J. Geophys. Res. 117, C07004. doi: 10.1029/2011JC007773
Paerl, H. W., and Piehler, M. F. (2008). Nitrogen and marine eutrophication. Nitrog. Mar. Environ. 2, 529–567. doi: 10.1016/B978-0-12-372522-6.00011-6
Pahlevan, N., Lee, Z., Wei, J., Schaaf, C. B., Schott, J. R., and Berk, A. (2014). On-orbit radiometric characterization of OLI (Landsat-8) for applications in aquatic remote sensing. Remote Sens. Environ. 154, 272–284. doi: 10.1016/j.rse.2014.08.001
Pahlevan, N., and Schott, J. R. (2013). Leveraging EO-1 to evaluate capability of new generation of Landsat sensors for coastal/inland water studies. Sel. Top. Appl. Earth Obs. Remote Sens. IEEE J. 6. 360–374. doi: 10.1109/JSTARS.2012.2235174
Ransibrahmanakul, V., and Stumpf, R. P. (2006). Correcting ocean colour reflectance for absorbing aerosols. Int. J. Remote Sens. 27, 1759–1774. doi: 10.1080/01431160500380604
Reifel, K. M., Corcoran, A. A., Cash, C., Shipe, R., and Jones, B. H. (2013). Effects of a surfacing effluent plume on a coastal phytoplankton community. Cont. Shelf Res. 60, 38–50. doi: 10.1016/j.csr.2013.04.012
Southern California Coastal Water Research Project (1973). The Ecology of the Southern California Bight: Implications for Water Quality Management. Three-year Report of the Southern California Coastal Water Research Project, El Segundo, CA.
Rogowski, P., Terrill, E., Thomas, J., Rosenfeld, L., and Largier, J. (2014). 2012 Orange County Sanitation District (OCSD) Outfall Diversion – Summary Report. Final Report Prepared for the Orange County Sanitation District, 92 pp, plus appendices.
Ryan, J. P., Kudela, R. M., Birch, J. M., Blum, M., Bowers, H. A., Chavez, F. P., et al. (2017). Causality of an extreme harmful algal bloom in Monterey Bay, California, during the 2014–2016 northeast Pacific warm anomaly. Geophys. Res. Lett. 44, 5571–5579. doi: 10.1002/2017GL072637
Santer, R., and Schmechtig, C. (2000). Adjacency effects on water surfaces: primary scattering approximation and sensitivity study. Appl. Opt. 39, 361–375. doi: 10.1364/AO.39.000361
Schiff, K., and Bay, S. (2003). Impacts of stormwater discharges on the nearshore benthic environment of Santa Monica Bay. Mar. Environ. Res. 56, 225–243. doi: 10.1016/S0141-1136(02)00332-X
Steinberger, A., and Stein, E. D. (2004). “Effluent discharges to the Southern California Bight from large municipal wastewater treatment facilities in 2001 and 2002,” in Southern California Coastal Water Research Project Biennial Report 2003-2004, eds D. Elmore and S. B. Weisberg (Westminster, CA), 2–15.
Suga, Y., Ogawa, H., Ohno, K., and Yamada, K. (2003). Detection of surface temperature from Landsat-7/ETM+. Adv. Space Res. 32, 2235–2240. doi: 10.1016/S0273-1177(03)90548-5
Sverdrup, H. U., and Fleming, R. H. (1941). The waters off southern California March to July 1937. Bull. Scripps Inst. Oceanogr. 4, 261–378.
Tang, D. L., Kester, D. R., Wang, Z., Lian, J., and Kawamura, H. (2003). AVHRR satellite remote sensing and shipboard measurements of the thermal plume from the Daya Bay, nuclear power station, China. Remote Sens. Environ. 84, 506–515. doi: 10.1016/S0034-4257(02)00149-9
Thomas, A. C., Huang, F., Strub, P. T., and James, C. (1994). Comparison of the seasonal and interannual variability of phytoplankton pigment concentrations in the Peru and California Current systems. J. Geophys. Res. Oceans 99, 7355–7370. doi: 10.1029/93JC02146
Thomas, A., Byrne, D., and Weatherbee, R. (2002). Coastal sea surface temperature variability from Landsat infrared data. Remote Sens. Environ. 81, 262–272. doi: 10.1016/S0034-4257(02)00004-4
Uchiyama, Y., Idica, E. Y., McWilliams, J. C., and Stolzenbach, K. D. (2014). Wastewater effluent dispersal in Southern California Bays. Cont. Shelf Res. 76, 36–52. doi: 10.1016/j.csr.2014.01.002
Vanhellemont, Q., and Ruddick, K. (2014). Turbid wakes associated with offshore wind turbines observed with Landsat 8. Remote Sens. Environ. 145, 105–115. doi: 10.1016/j.rse.2014.01.009
Vanhellemont, Q., and Ruddick, K. (2015). Advantages of high quality SWIR bands for ocean colour processing: examples from Landsat-8. Remote Sens. Environ. 161, 89–106. doi: 10.1016/j.rse.2015.02.007
Washburn, L., Jones, B. H., Bratkovich, A., Dickey, T. D., and Chen, M. S. (1992). Mixing, dispersion, and resuspension in vicinity of ocean wastewater plume. J. Hydraul. Eng. 118, 38–58. doi: 10.1061/(ASCE)0733-9429(1992)118:1(38)
Welschmeyer, N. A. (1994). Fluorometric analysis of chlorophyll a in the presence of chlorophyll b and pheopigments. Limnol. Oceanogr. 39, 1985–1992. doi: 10.4319/lo.1994.39.8.1985
Keywords: Landsat 8, Chlorophyll, coastal water quality, sea surface temperature (SST), wastewater diversion, satellite remote sensing
Citation: Trinh RC, Fichot CG, Gierach MM, Holt B, Malakar NK, Hulley G and Smith J (2017) Application of Landsat 8 for Monitoring Impacts of Wastewater Discharge on Coastal Water Quality. Front. Mar. Sci. 4:329. doi: 10.3389/fmars.2017.00329
Received: 03 August 2017; Accepted: 04 October 2017;
Published: 26 October 2017.
Edited by:
Kevin Ross Turpie, University of Maryland, Baltimore County, United StatesReviewed by:
Saumitra Mukherjee, Jawaharlal Nehru University, IndiaOliver Zielinski, University of Oldenburg, Germany
Copyright © 2017 Trinh, Fichot, Gierach, Holt, Malakar, Hulley and Smith. This is an open-access article distributed under the terms of the Creative Commons Attribution License (CC BY). The use, distribution or reproduction in other forums is permitted, provided the original author(s) or licensor are credited and that the original publication in this journal is cited, in accordance with accepted academic practice. No use, distribution or reproduction is permitted which does not comply with these terms.
*Correspondence: Rebecca C. Trinh, cnRyaW5oQGxkZW8uY29sdW1iaWEuZWR1