- Australian Institute of Marine Science, Townsville, QLD, Australia
In a changing ocean, tropical waters can be instructive as to the potential effects of climate induced changes on marine ecosystem structure and function. We describe the relationships between planktonic community respiration (CR), net community production (NCP), gross primary production (GPP), and environmental variables in 14 regions and three ecosystem types (coastal, coral reef, and open sea) from Australia, Papua New Guinea, and Indonesia. The data are compiled from separate studies conducted between 2002 and 2014 with the goal of better parameterizing the metabolic balance in tropical marine waters. Overall, these regions were strongly autotrophic (average GPP:CR ratio: 2.14 ± 0.98), though our dataset of 783 paired measurements did include some oceanic stations where heterotrophy (GPP:CR < 1) was predominant, and some coastal stations that were intermittently heterotrophic. Our statistical analysis suggested that temperature was the most important determinant of CR in coral reef and ocean ecosystems but less so in coastal ecosystems, where chlorophyll concentration was more important. In contrast, chlorophyll and sampling depth were more important in regulating GPP than temperature. The relationships between temperatures and metabolic rates showed that these were ecosystem-dependent, with coastal ecosystems showing less response to temperature than coral reef and open sea sites. The threshold of GPP to achieve metabolic balance fell in a range between 0.715 mmol O2 m−3 d−1 in the Coral Sea to 10.052 mmol O2 m−3 d−1 in mangrove waterways of Hinchinbrook Channel. These data allow regions in and around northern Australia to be ranked in terms of trophic state, ranging from the oligotrophic Scott Reef (GPP:CR = 0.84 ± 0.08) to productive surface waters of the Kimberley coast (GPP:CR = 5.21 ± 0.62). The measurement of pelagic metabolism shows potential as a quantitative tool to monitor the trophic state of coastal waters.
Introduction
Sixty years ago the concept of net community production (NCP), the difference between gross primary production (GPP), and the community (autotrophic plus heterotrophic) respiration (CR) was framed by Odum (1956). Despite this, it is only within the last two decades that the oceanographic community has intensively studied the metabolic balance of the ocean (Ducklow and Doney, 2013). “Metabolic state” is defined as the ratio between GPP and CR at the scale of the ecosystem, with a system being autotrophic if it produces more organic matter than it consumes (GPP:CR > 1) and heterotrophic if organic matter consumption exceeds in situ production (GPP:CR < 1). This ratio therefore describes the balance between the flow of organic matter required to maintain community metabolic balance (GPP:CR = 1) and that available for growth or export away from the ecosystem (NCP) (Platt et al., 1992).
Globally, coastal waters account for 14–33% of total oceanic production and ~80% of organic matter burial, making them amongst the most productive and biogeochemically active zones of the biosphere (Gattuso et al., 1998). While some studies suggest that these systems are net heterotrophic due to the delivery of large amounts of continentally derived organic matter (Smith and Mackenzie, 1987; Smith and Hollibaugh, 1993), others have found that globally the coastal ocean is autotrophic, exporting 15% of its NCP to the open ocean (Ducklow and McAllister, 2005). These differences could be due to differences in methodology or to spatio-temporal variation in metabolic balance. Spatio-temporal variation has been attributed to factors such as (1) changes in environmental conditions (e.g., temperature: Regaudie-de-Gioux and Duarte, 2012); (2) differences in the input and composition of nutrients (Martínez-García et al., 2010); (3) changes in plankton abundance and productivity of primary producers (Gasol and Duarte, 2000); (4) variation in the phytoplankton size structure and growth rate (Marañón, 2015); and (5) the amount and reactivity of the locally produced (autochthonous) and imported (allochthonous) organic matter (e.g., Serret et al., 1999; Robinson et al., 2002; Lønborg and Álvarez-Salgado, 2012).
Tropical coastal waters receive approximately one order of magnitude more input of continental derived carbon, nitrogen, and phosphorus than temperate and arctic regions (Brunskill, 2010). The phytoplankton production rates in tropical coastal waters are high and can in some instances match some of the most productive areas of the global ocean (e.g., upwelling areas), with these high rates being sustained by high temperatures and a constant supply of nutrients and solar radiation (Nittrouer et al., 1995). In tropical coastal systems with a narrow shelf (e.g., coastal waters of the Bismarck Sea) most terrestrial material, together with the organic matter produced in situ, is exported to the open ocean (Burns et al., 2008). By contrast, in systems with a broad shelf (e.g., Great Barrier Reef), most organic matter is consumed within the continental shelf (Brunskill et al., 2010). The few published measurements of pelagic metabolism from coastal tropical waters suggest that these are mainly net autotrophic (GPP:CR > 1) but that the metabolic balance varies both temporally and spatially (McKinnon et al., 2007, 2013).
Robinson and Williams (2005) reviewed all the measurements of pelagic respiration available to that date, and identified a shortfall of data from tropical regions. Paired measurements of production and respiration are even fewer. Here, we present the results of metabolism (i.e., respiration and production) experiments conducted in 14 study regions of tropical Australia, Papua New Guinea, and Indonesia spanning latitudes from 5 to 23°S, collected in the course of numerous studies using similar experimental designs and methodologies. Collectively, these studies make a sizable contribution to the data deficit from tropical waters, but more importantly allow comparisons of production, respiration, and metabolic balance in tropical habitats ranging from turbid inshore waters to the oligotrophic waters of the tropical ocean.
Our objective is three-fold: (1) to compare tropical ecosystems by partitioning our data into different ecosystem types (coastal waters, coral reef waters, and open sea); (2) to rank regions in order of metabolic activity, an approach we consider useful for understanding and managing coastal waters increasingly threatened by anthropogenic influences on ecosystem productivity mediated by environmental variables such as nutrients and light, and; (3) to identify factors influencing metabolism that have the potential to shift the trophic state of these ecosystems as a result of environmental changes. All data has been made available as Supplementary Material. Measurements are in Supplementary Data Sheet 2, and station data in Supplementary Data Sheet 3.
Materials and Methods
Study Areas
This study is based on both published and unpublished data on NCP, dark Community Respiration (CR), and GPP collected between 2002 and 2014 from field campaigns conducted in tropical coastal and shelf waters of northern Australia, Indonesia, and Papua New Guinea (Table 1, Figure 1). The studies were undertaken in 14 regions characterized by high water temperatures (22–34°C), low dissolved nutrient concentrations and low plankton biomass (Furnas et al., 2011; Supplementary Material). The climate in these areas is typically monsoonal, with a wet summer season (December–March) and a dry winter season (April–November). Climatic events can result in elevations of nutrient and phytoplankton biomass during periods of strong vertical mixing (e.g. storms, cyclones) or from land runoff, particularly during the wet season (e.g., McKinnon et al., 2007; Furnas et al., 2011).
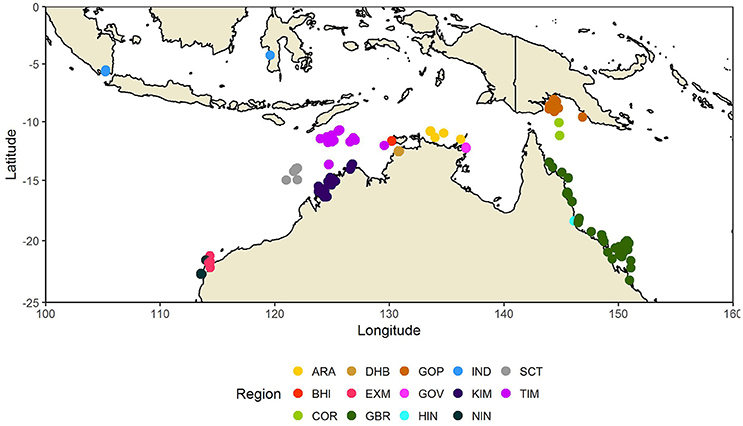
Figure 1. Map of Northern Australia, showing the regions where metabolism studies were conducted. Regional abbreviations are given in Table 1.
Sample Collection
Full-depth continuous conductivity-temperature-depth (CTD) profiles were recorded (Seabird SBE19Plus) at each sampling site to determine water depths for subsequent biological sampling. Following the CTD cast, water samples were taken with Niskin bottles at depths spanning the euphotic zone (defined here as extending to the 1% light level), with sampling depths determined as those best simulated by our on-deck incubators on the basis of the light profile either from CTD casts or from secchi depths. Therefore, the discrete sampling depths depended on the water depth and light profile at the particular study site, and ranged from the surface to a maximum depth of 100 m (Scott Reef; Supplementary Figure 1). In some studies an additional near-bottom sample was taken to identify potentially higher respiration rates associated with the presence of nepheloid layers generated by near-bottom resuspension. Immediately after retrieval on board, seawater from the Niskin bottles was used to fill calibrated acid-washed iodine flasks with a nominal volume of 125 mL for oxygen production and respiration experiments. For all studies a minimum of three replicate flasks were filled for measurement of initial oxygen concentration (zero-time samples), respiration (incubated in the dark for 24 h), and production (placed in deck incubators for 24 h under neutral density mesh to simulate light levels occurring at discrete depths throughout the euphotic zone). Surface seawater was continuously pumped through the incubators to approximate temperatures in situ. In the Arafura Sea the number of replicates was increased to 4, and at Scott Reef to 5.
We have compiled a total of 783 pairs of concurrent measurements of NCP, CR, and (GPP, as the sum of CR and NCP) based on changes in oxygen concentration in light and dark bottle incubations (Table 1). There were an additional 67 measurements of CR alone. In all, the data was derived from 12 regions in northern Australia, one in Papua New Guinea, and two in Indonesia. Data from the two Indonesian regions have been pooled because both originated from shallow bays containing sea cages for finfish aquaculture, and there was insufficient data density to establish any clear differences between them (for details see Alongi et al., 2009). The number of paired measurements varies greatly between regions: the smallest data set is from Gove (n = 6), and the largest from the waters of the Great Barrier Reef region (n = 300; Table 1).
Immediately after filling the iodine flasks, samples were taken from the same Niskin bottles for the analysis of total suspended solids (TSS), chlorophyll a (chl a), dissolved inorganic nutrients (, /, , and SiO4), particulate organic carbon (POC), nitrogen (PN) and phosphorus (PP), dissolved organic carbon (DOC), total dissolved nitrogen (TDN) and phosphorus (TDP) (Table 2). The filtration and processing of these water samples started immediately after collection. Sample water (1 L) for TSS analysis was collected on pre-weighed 0.4 μm polycarbonate filters (47 mm diameter). After filtration, filters were rinsed with 50 mL of deionized water to remove salt. TSS concentrations were determined gravimetrically from the mass difference between loaded and unloaded filters after drying overnight at 60°C. Chl a samples were collected by filtering between 100 and 200 mL of the sampled water through precombusted (450°C, 4 h) GF/F filters (pore size ~0.7 μm), which were frozen (−20°C) until analysis. Suspended matter was collected under low-vacuum on precombusted GF/F filters for particulate organic matter (250 mL) analysis. All filters were kept frozen (−20°C) until analysis. The samples for the dissolved phase (inorganic nutrients, DOC, TDN, and TDP) were immediately filtered through a 0.45 μm filter cartridge (Sartorius MiniSart) into acid-washed 10 mL HDPE plastic containers. Duplicate water samples (10 mL) for inorganic nutrients, TDN and TDP, were kept frozen (−20°C) until analysis. Ten milliliters sub-samples for DOC were collected in duplicate and preserved by adding 100 μL 32% AR-grade HCl and stored in the dark at 4°C until analysis.
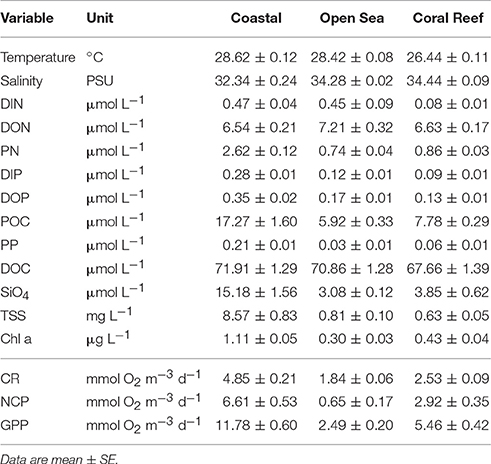
Table 2. Environmental properties of water used in metabolism experiments (see Materials and Methods for definitions) and summary of volumetric CR, NCP, and GPP by ecosystem type.
Sample Analysis
Dissolved oxygen concentrations were determined with an automated high-precision Winkler titration system which uses the absorption of 365 nm ultraviolet light for endpoint detection. The entire set of flasks from each experiment was titrated as a single batch within 24 h of completion of the experiments. NCP was calculated as the difference in dissolved oxygen concentration between light-incubated samples and zero time samples; dark CR was defined as the change in oxygen concentration between dark-incubated samples and zero samples. GPP was calculated as the sum of NCP and CR, and the P:R ratio as the GPP:CR ratio. Where near-bottom samples were taken, these were not included in production calculations since only CR was measured. Coefficients of variation of the replicate O2 concentration measurements in the zero, dark, and light bottles were typically 0.09, 0.20, and 0.28%, respectively. In this manuscript we report area-specific metabolic rates by trapezoidal integration of volumetric values of NCP and CR.
Chl a was determined with a Turner Designs 10 AU fluorometer after 90% acetone extraction (Yentsch and Menzel, 1963). Inorganic nutrients (, /, , and SiO4) were determined using standard segmented flow analysis (Hansen and Koroleff, 1999). The precisions of replicate samples were ±0.01 μmol L−1 for , ±0.1 μmol L−1 for /, ±0.02 μmol L−1 for , and ±0.05 μmol L−1 for SiO4.
Particulate organic carbon and nitrogen content (POC and PN) were measured by high temperature combustion (950°C) using a Shimadzu TOC-V carbon analyser fitted with a SSM-5000A solid sample module, after the inorganic carbon on the filters (e.g., CaCO3) had been removed by acidification of the sample with 2M HCl (Nieuwenhuize et al., 1994). The analyser was calibrated using AR Grade EDTA for the 5 point standard curve. Particulate phosphorus (PP) was determined spectrophotometrically as inorganic P after digesting the particulate matter in 5% potassium persulphate. The method was standardized using orthophosphoric acid as the standard for the 4 point calibration. We compared peak areas of the filter blanks and standard solutions to ensure consistency between runs with no major deviations found.
Dissolved organic carbon (DOC) concentrations were measured by high temperature combustion (680°C) using a Shimadzu TOC-5000A carbon analyser. Concentrations were determined by subtracting a Milli-Q blank and dividing by the slope of a daily 4 points standard curve made from potassium hydrogen phthalate and glycine. The consistency between runs was verified by comparing peak areas of standard solutions with no major deviations found. Analyses of total dissolved nitrogen (TDN) and phosphorus (TDP) were determined by oxidation (121°C, 70 min) in alkaline conditions by persulphate digestion of water samples (Valderrama, 1981), which were then analyzed for inorganic nutrients, as described above. Dissolved organic nitrogen (DON) concentrations were calculated as the difference between TDN and dissolved inorganic nitrogen (DIN; /) (DON = TDN–DIN) and dissolved organic phosphorus (DOP) as the difference between TDP and dissolved inorganic phosphorus (DIP; ) (DOP = TDP–DIP).
Statistical Analysis
We first explored the distribution of the data using Cleveland dotplots of all variables (Table 2) individually by sampling location, alongside box, and whisker plots categorized by season, since in previous studies there were strong contrasts between wet (December–March) and dry seasons (April–November).
Our dataset includes a range of ecosystem types. In addition to conducting statistical analyses on the entire dataset, we also conducted analyses grouped by ecosystem type. Regional data were classified into three ecosystem types: Coral Reef (GBR, NIN); Open sea (ARA, COR, SCT, TIM); and Coastal (BHI, DHB, EXM, GOP, GOV, HIN, IND, KIM). Of these, the coral reef ecosystems are most clearly defined as the waters surrounding the two largest coral reef systems in Australia. The open sea ecosystems were characterized as being primarily in open ocean water out of sight of land. The Scott Reef region was classified as oceanic because most sampling took place in Indian Ocean waters on the shelf edge. The coastal ecosystem includes regions that were all estuarine to some degree, though there is a gradation in the extent of fresh water influence. For example, the Hinchinbrook Channel (HIN) was strongly influenced by freshwater in the wet season, and the innermost stations of the Gulf of Papua (GOP) were influenced by the Fly River. At the other extreme, Exmouth Gulf is a tropical embayment in which inshore waters can be hypersaline, and is best described as a negative estuary. Moreover, the coastal regions were all characterized by the presence of mangrove forests on their shores.
Physical, chemical, and biological variables potentially influencing CR and GPP were explored via Gradient Boosted Regression Tree (BRT) Models (Ridgeway, 2017). We chose to use BRT's because of their ability to accommodate missing data by imputation, their suitability to independently estimate the effects of predictors that are themselves correlated, and their ability to estimate the relative importance of predictors in complex systems. BRT models combine two machine learning methodologies; regression trees and boosting. Regression trees are hierarchical models that recursively partition the data on the basis of minimizing prediction errors and in so doing provide a powerful framework that is insensitive to outliers and missing data, unaffected by monotone transformations and scales of covariates and naturally accommodate non-linearity and complex interactions (Hastie et al., 2011). Boosting in a regression tree context is a numerical optimization technique for minimizing deviance (a measure of the difference between observed and predicted) at each step of a sequential series of trees, each of which focuses on the most poorly predicted components of the preceding tree (De'ath, 2007; Elith et al., 2008). BRT models are typically encouraged to “learn” slowly and thoroughly by constructing very large numbers of small regression trees, training each tree on a random subset of the residuals from the proceeding tree and “shrinking” the contribution of each tree. Finally, over-fitting can be countered through one of a number of regularization techniques (such as cross-validation) that serve to strike a balance between predictive performance and model fit (Hastie et al., 2011). Collectively, this stochasticity and sequential slow learning through large numbers of small regression trees typically yield predictions that are superior to regular linear modeling approaches.
BRT models for log-transformed CR and GPP included the covariates listed in Table 2. A total of 5,000 trees were fit to an interaction depth of 5, bag fraction of 0.5 and shrinkage rate of 0.001. The optimal number of boosting iterations was determined by the “out-of-bag” method. The relative importance of each predictor was calculated as the frequency of splits involving each variable weighted by the associated square improvement in the model averaged over all trees and scaled out of 100 such that larger values signify stronger influence. Uncertainty in partial effects and relative importance estimates was incorporated by bootstrapping (sampling with replacement) each BRT 100 times. All BRT models were fit via the gbm package (Ridgeway, 2017) within the R Statistical and Graphical Environment (R Core Team, 2016).
To examine the role of temperature in regulating metabolic rates it is necessary to account for the co-variance between temperature and plankton biomass. We, therefore, standardized CR and GPP to Chl a concentration, the only index of plankton biomass available in our dataset. The data was then averaged within 1°C data bins to provide an average response to a given temperature. This procedure also forces that all bins have the same weight in the subsequent statistical analyses, independent of the amount of data that they initially contain.
The relationship between temperature and biological activity is normally modeled using an Arrhenius-type relationship, assuming that chemical kinetics control the observed rates (Ahlgren, 1987). The Arrhenius equation is defined as:
where k is the enzyme activity; A is the theoretical enzyme activity in the absence of activation energy (Ea): Ea is the energy barrier to be surpassed in order for the reaction to take place (in J mol−1); R is the universal gas constant (8.314 J mol−1 K−1); and T is the temperature in Kelvin (K). The factor is known as the Boltzmann expression, which shows the fraction of the reactive molecules having energy in excess of Ea (Arrhenius, 1889). An estimate of Ea can be derived from the slope of an Arrhenius plot of natural logarithm of activity (CR and GPP) vs. the reciprocal of absolute temperature (T). The Ea, can thereafter be calculated by multiplying the regression slope by R, the gas constant.
Regression analyses of CR, NCP, GPP, and Chl a (total dataset and by ecosystem type) were performed using the best–fit between the two variables X and Y obtained by Model II regression as described in Sokal and Rohlf (1995) using the package lmodel2 within R (R Core Team, 2016).
Results
Environmental Conditions
Salinity varied overall from a minimum of 18.5 to a maximum of 36.6, with the lowest values taken from waters close to shore influenced by wet season runoff (Supplementary Figure 2). Temperature varied between 22 and 34°C, with highest levels found in surface waters during the wet season (Supplementary Figure 3). Overall, there was little difference in temperature and salinity between ecosystems (Table 2). TSS concentrations varied between 0.01 and 67.92 mg L−1 with highest levels in the Gulf of Papua and at nearshore stations in Darwin Harbour and on the Kimberley Coast (Supplementary Figure 4). The coastal ecosystems had an order of magnitude higher TSS than the open sea or coral reef ecosystems. Chlorophyll a (Chl a) concentrations ranged between 0.02 and 8.26 μg L−1, with highest levels in the mangrove waterways of Bathurst Island during the wet season (Supplementary Figure 5), with coastal ecosystems having ~3-fold higher concentrations than the other ecosystem types. The dissolved inorganic nitrogen (DIN) and phosphorus (DIP) concentrations varied between levels below detection limit up to 8.2 and 1.50 μmol L−1, respectively (Supplementary Figures 6, 7). Generally, DIN and DIP concentrations of surface waters were close to the detection limits of standard methods, but occasionally higher levels were found at stations closer to shore directly affected by river runoff. DIN concentrations were similar in coastal and open sea ecosystems, but lower in the coral reef ecosystem, whereas DIP was highest in the coastal ecosystems (Table 2). Dissolved silicate (SiO4) concentrations were highest in the coastal ecosystems (Table 2), but was always detectable in all ecosystems with levels between 0.2 and 121.4 μmol l−1 (Supplementary Figure 8), suggesting that in all regions there was mostly sufficient silicate present to support growth of diatom populations.
Concentrations of particulate organic matter (POM) were elevated in surface waters during the wet season and at stations closer to shore, as indicated by POC (Supplementary Figure 9), PN (Supplementary Figure 10), and PP (Supplementary Figure 11), and all of which were highest in the coastal ecosystems (Table 2). The average C: N: P stoichiometry of the suspended particulate fraction was 150:10:1, which is slightly higher but not different to the Redfield ratio and ratios found for recently produced organic matter (106:16:1, Redfield et al., 1963), suggesting a predominantly planktonic origin of this material.
Higher levels of dissolved organic matter (DOM) were observed in surface waters during the wet season and closer to shore. Dissolved organic carbon (DOC) was similar in all ecosystems, with a tendency to be higher in the wet season (Supplementary Figure 12). Dissolved organic nitrogen (DON) was similar in all ecosystems (Table 2), and slightly higher in the wet season in some regions (Supplementary Figure 13). DOP was highest in the coastal ecosystems (Table 2), with seasonal differences in some regions (Supplementary Figure 14). The average C:N:P stoichiometry of the DOM pool was 367:49:1, which was higher than the Redfield ratio but comparable with ratios reported for coastal waters worldwide (Lønborg and Álvarez-Salgado, 2012).
Oxygen Fluxes
CR ranged from 0.12 to 16.67 mmol O2 m−3 d−1 and was highest in the coastal ecosystems [mean 4.22 ± 0.17(SE) mmol O2 m−3 d−1]; for the coral reef ecosystems it was 2.53 ± 0.09 mmol O2 m−3 d−1 and for the open sea 1.77 ± 0.90 mmol O2 m−3 d−1. Ranking regions by median CR (Figure 2) illustrated the variation within the coastal ecosystem group, with lowest CR in the Gulf of Papua (2.36 ± 0.24 mmol O2 m−3 d−1) and highest in Bathurst Island (6.70 ± 0.84 mmol O2 m−3 d−1). Overall, CR was lowest in the oceanic waters of the Coral Sea (0.76 ± 0.07 mmol O2 m−3 d−1) (Figure 2, Supplementary Figure 15).
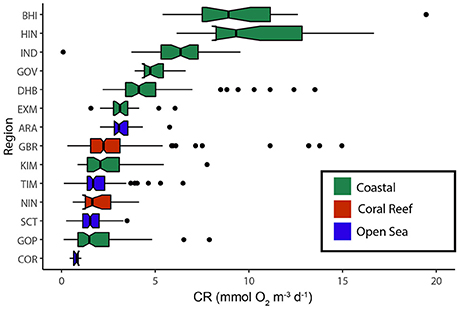
Figure 2. Box plot of the volume-specific plankton community respiration (mmol O2 m−3 d−1) by location (see Table 1). The data is ranked by the median and 50% of the data are included within the limit of the boxes, and the error bars represent the 10 and 90th percentiles. Ecosystem type is indicated by the color of the boxes.
Most experiments were conducted in shallow waters (Supplementary Figure 1): 79% of measurements were made in 25 m or less. Because the water column was generally well mixed over this depth range there were negligible vertical differences in CR, and where near-bottom samples were taken these did not differ from rates higher in the water column. In the oceanic waters adjacent to Scott Reef, however, experiments were conducted at six depths through the euphotic zone down to 100 m in both the wet and dry seasons of 2008 and 2009 (Figure 3). In the dry season there was a well-defined mixed layer to about 90 m; in the wet season the mixed layer was less well-defined and confined to the top 35 m. Even over the 100 m depth range of the Scott Reef experiments there was no indication of vertical differences in CR in either season (Figure 3).
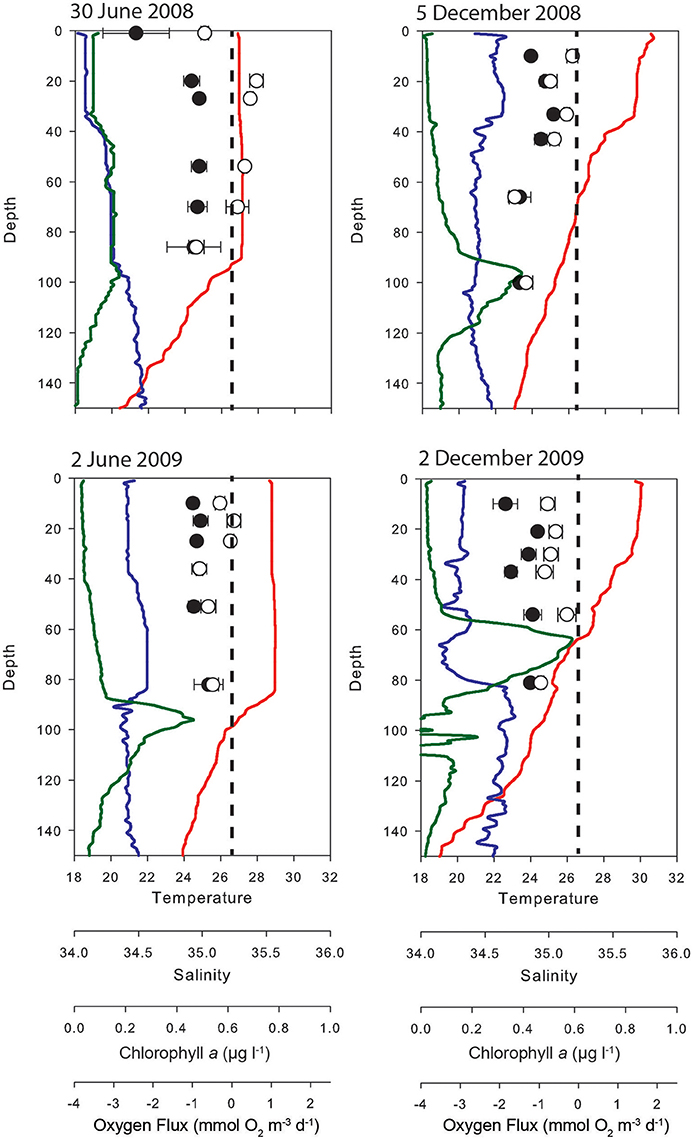
Figure 3. Vertical patterns of CR (filled symbols) and NCP (open symbols) as measured in dark and light bottles respectively, at an oceanic station SW of Scott Reef. Oxygen flux are reported as mmol O2 m−3 d−1. The vertical dashed line is the compensation point (i.e., NCP = CR). Vertical profiles of temperature (red), salinity (blue), and chlorophyll fluorescence μg L−1 (green) are derived from the CTD data.
In our dataset the volume-specific NCP varied between −9.34 to 76.99 mmol O2 m−3 d−1. NCP was highest in the coastal ecosystems (6.61 ± 0.53 mmol O2 m−3 d−1) followed by coral reef waters (2.92 ± 0.35 mmol O2 m−3 d−1) and the open sea (0.65 ± 0.17 mmol O2 m−3 d−1; Table 2). On average, Darwin Harbour had the highest volume-specific NCP (10.71 ± 0.88 mmol O2 m−3 d−1). Scott Reef was the only dataset to be heterotrophic on average (NCP of −0.25 ± 0.09 mmol O2 m−3 d−1).
GPP was highest in the coastal ecosystems (11.89 ± 0.86 mmol O2 m−3 d−1), followed by reef ecosystems (5.46 ± 0.68 mmol O2 m−3 d−1) and the open sea (2.57 ± 0.28 mmol O2 m−3 d−1). The maximum volume-specific GPP of 91.95 mmol O2 m−3 d−1 occurred in flood waters in the inshore waters of the Great Barrier Reef, but otherwise GPP was highest in the Hinchinbrook Channel (19.71 ± 1.21 mmol O2 m−3 d−1), Darwin Harbour (15.85 ± 0.88 mmol O2 m−3 d−1) and Bathurst Island (15.03 ± 0.65 mmol O2 m−3 d−1) (Supplementary Figure 16).
Relationships between Production and Respiration
On a volumetric basis, all regions were mean autotrophic except for Scott Reef (GPP:CR = 0.84 ± 0.08; Table 3), though heterotrophy was also observed in some experiments in the Hinchinbrook Channel and in the Timor Sea. Darwin Harbour and the Kimberley coast had disproportionally high GPP:CR ratios (3.51 ± 0.34 and 5.21 ± 0.62 respectively; Table 3). Overall, volume-specific CR scaled with GPP in our dataset (Figure 4). Model II regression (RMA) between CR and GPP for the entire dataset results in a slope of 0.42 ± 0.06 (95% C.I.), with an R2 of 0.43.
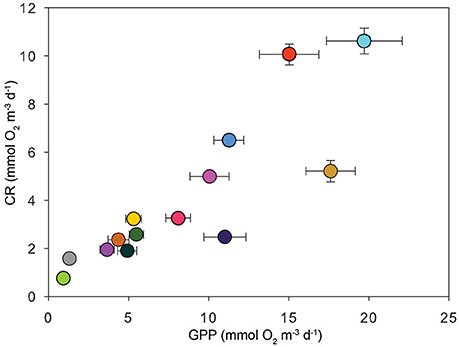
Figure 4. The relationship between the means of volume-specific GPP and CR (both mmol O2 m−3 d−1) for each location (Table 1). Error bars correspond to the SE and are within the symbol size in some cases.
Examination of the relationship between NCP and GPP using Model 2 Regression allows the estimation of the threshold values of GPP necessary to achieve metabolic balance (GPP:CR = 1) (Table 4). The resulting metabolic thresholds rank all regions in order of trophic state, within the allocated ecosystem type. The threshold of GPP to achieve metabolic balance fell in a range between 0.715 mmol O2 m−3 d−1 in the Coral Sea to 10.052 mmol O2 m−3 d−1 in mangrove waterways of Hinchinbrook Channel (Table 4). There was a considerable range of threshold values within the coastal group. The mean threshold of GPP over the whole dataset was 2.38 mmol O2 m−3 d−1 and 5.56, 1.87, and 1.71 mmol O2 m−3 d−1 for the coastal, and coral reef open sea ecosystems, respectively.
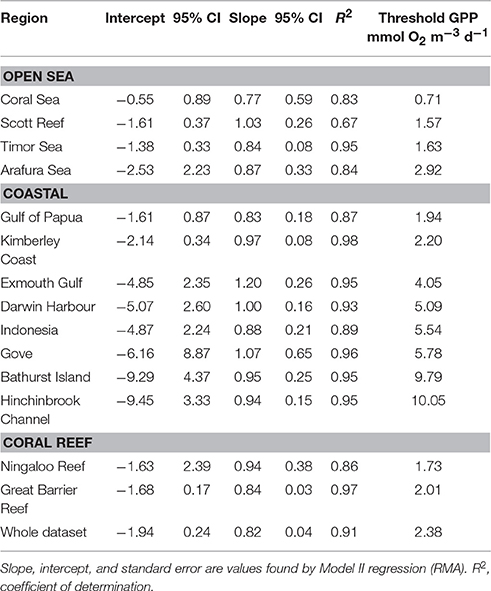
Table 4. Significant regressions of the relationship between net community production (NCP) and gross primary production (GPP) to determine the threshold necessary for metabolic balance for regions reported in Table 1.
CR scaled with both GPP and CR, with CR scaling at the 0.77 power of GPP, and the 0.47 power of NCP (Figure 5; Table 5). In the open sea, CR scaled at the 0.22 power of NCP, lower than the other ecosystems (Table 5).
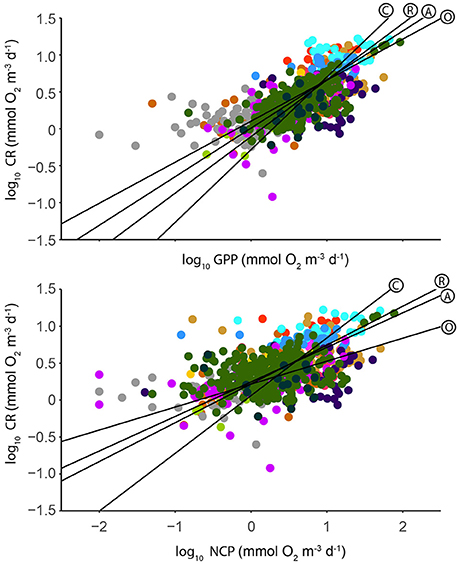
Figure 5. Log-log relationships of GPP and CR (Top) and NCP and CR (Bottom), as mmol O2 m−3 d−1. Symbol colors correspond to the location of the measurement as indicated in Figure 1, and the regression line for each group is plotted as: A, entire data set; C, coastal ecosystems; O, open sea ecosystems; R, coral reef ecosystems. Model 2 regression statistics are given in Table 5.
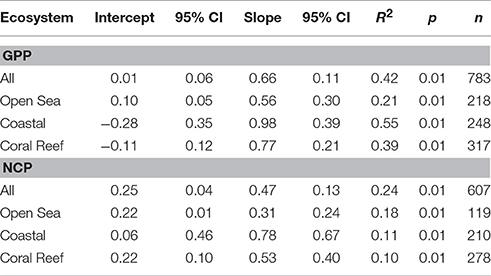
Table 5. Model 2 Regression statistics (RMA) for the relationship between log10 transformed volumetric CR, and GPP, and NCP respectively (see Figure 5).
Environmental Predictors of Metabolism
We used Boosted Regression Trees to compare the relative importance of individual physico-chemical and biological variables as well as Region, Sampling depth, and Season as predictors of both volumetric CR and GPP. For CR in the whole dataset, regional differences were the most important determinant, closely followed by Chl a with minor effects of temperature and depth (Supplementary Figure 17). In the case of Chl a there is a threshold at ~1.0 μg L−1 above which CR increases markedly (Supplementary Figure 17E). In the case of GPP (Supplementary Figure 18), Chl a was the most important predictor (Supplementary Figure 18Q), with region and depth the only other variables exerting any influence.
The importance of region in the analysis of the whole dataset suggested differences in trophic functioning across the different ecosystem types. Accordingly, we repeated our analysis for the three ecosystem types (coastal, coral reef and open sea) as shown in Table 1. The coral reef dataset comprised 317 paired measurements of CR and GPP, and the open sea and coastal ecosystems 218 and 248 paired measurements, respectively (Table 1).
Considering the environmental variables that best predicted CR in coral reef ecosystems, temperature and POC were selected as most important, with a lesser effect of season and PP (Figures 6A–E). There was a pronounced increase in CR in waters with temperatures between 25 and 28°C, and POC levels between 8 and 15 μmol L−1. There was also a slight tendency for CR to be higher in the wet season, and a gradual increase in CR above PP concentrations of 0.07 μmol L−1. In the open sea, temperature, Chl a, salinity and POC were the best predictors of CR, with a minor effect of sampling depth (Figures 6F–J). CR increased above temperatures of 28°C and Chl a concentrations of 0.3 μg L−1. The effects of salinity and depth are more subtle, with a slight decrease in CR with depths between 10 and 50 m and salinity >33.8. Within the coastal ecosystems, regional differences in CR outweighed other environmental predictors (Figures 6K–O) with Chl a concentration of secondary importance, showing a marked increase in CR above 0.7 μg L−1. Conversely, there was a decrease in CR at POC concentrations between 6 and 12 μmol L−1, while a slight increase was found for temperatures above ~28.5°C.
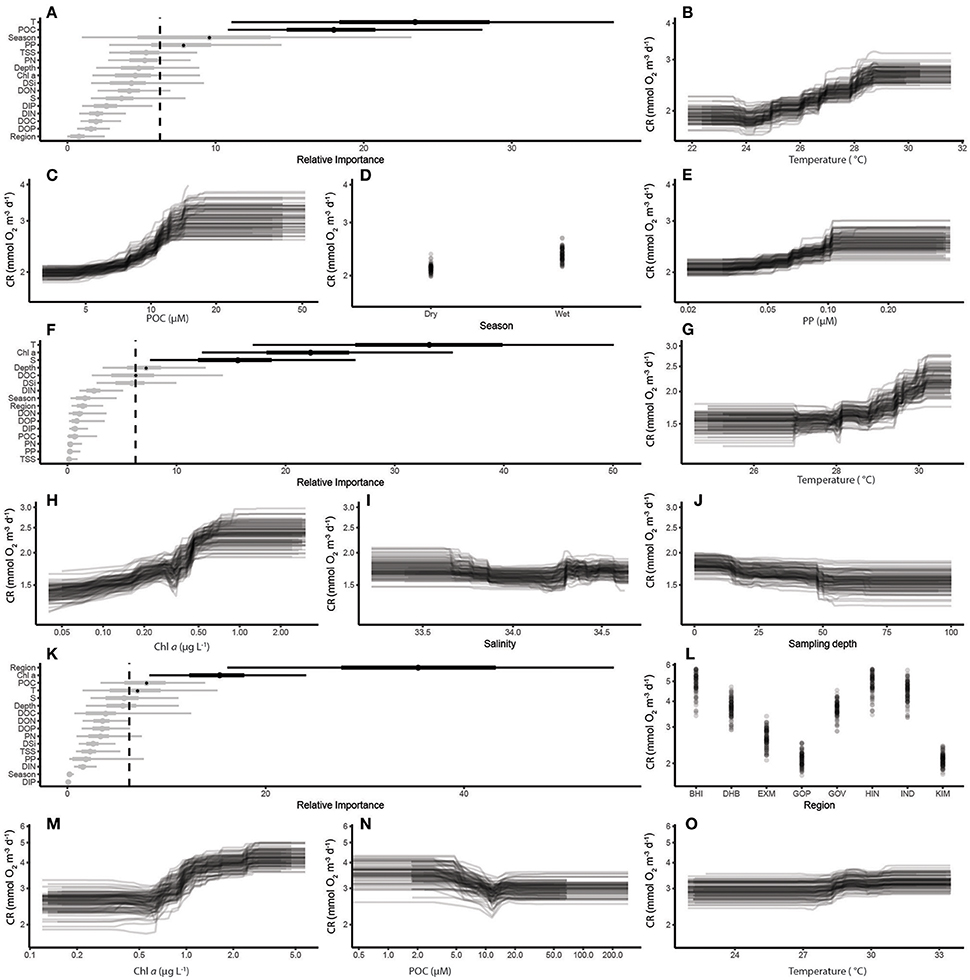
Figure 6. Partial-dependency plots (based on Boosted Regression Tree analysis – see Methods) of the response of CR to the most important predictors. Coral Reef Ecosystems, (A) relative importance of predictors for coral reef ecosystems; (B) temperature; (C) POC; (D) season; (E) PP; Open Sea Ecosystems (F) relative importance of predictors for open sea ecosystems; (G) temperature; (H) Chl a; (I) salinity; (J) depth; Coastal Ecosystems, (K) the relative importance of predictors for coastal ecosystems; (L) Region; (M) Chl a; (N) POC; and (O) temperature. Regional abbreviations are defined in Table 1, and Covariate labels are defined in the Materials and Methods section. Each graph represents the modeled effect of each covariate holding all other covariates constant. Since the relative importance of a covariate is the percentage of all node splits involving that covariate, a simple critical threshold (indicated by the vertical dashed line) can be established as 100/n (where n is the number of covariates) since if all covariates where equally important, they would each have a relative importance of 100/n. Therefore, the relative importance of covariates above this threshold can be interpreted as more important than would be expected by pure chance alone.
For GPP, Chl a was the most important predictor in coral reef ecosystems, with smaller effects of TSS, PN and depth (Figures 7A–E). There was a pronounced increase in GPP at Chl a concentrations between 0.3 and 0.5 μg L−1, and moderate increases at TSS > 1.0 mg L−1 and PN above 1.3 μmol L−1. GPP declined at depths between 10 and 35 m. In the open sea, Chl a was again the best predictor, with depth second. DOP had a smaller impact, while there was no difference between the four open sea regions (Figures 7F–J). GPP increased with Chl a concentrations between 0.07 and 0.40 μg L−1 and slightly decreased at depths >30 m. Regional differences remained the most important predictor of GPP in the coastal ecosystems, with Chl a and depth also being important (Figures 7K–O). GPP increased at Chl a concentrations >0.4 μg L−1 and stabilized at >1.2 μg L−1. The GPP was highest in surface waters, and stabilized at depths >15 m. Dissolved silicate, an index of terrestrial influence, had little effect on GPP.
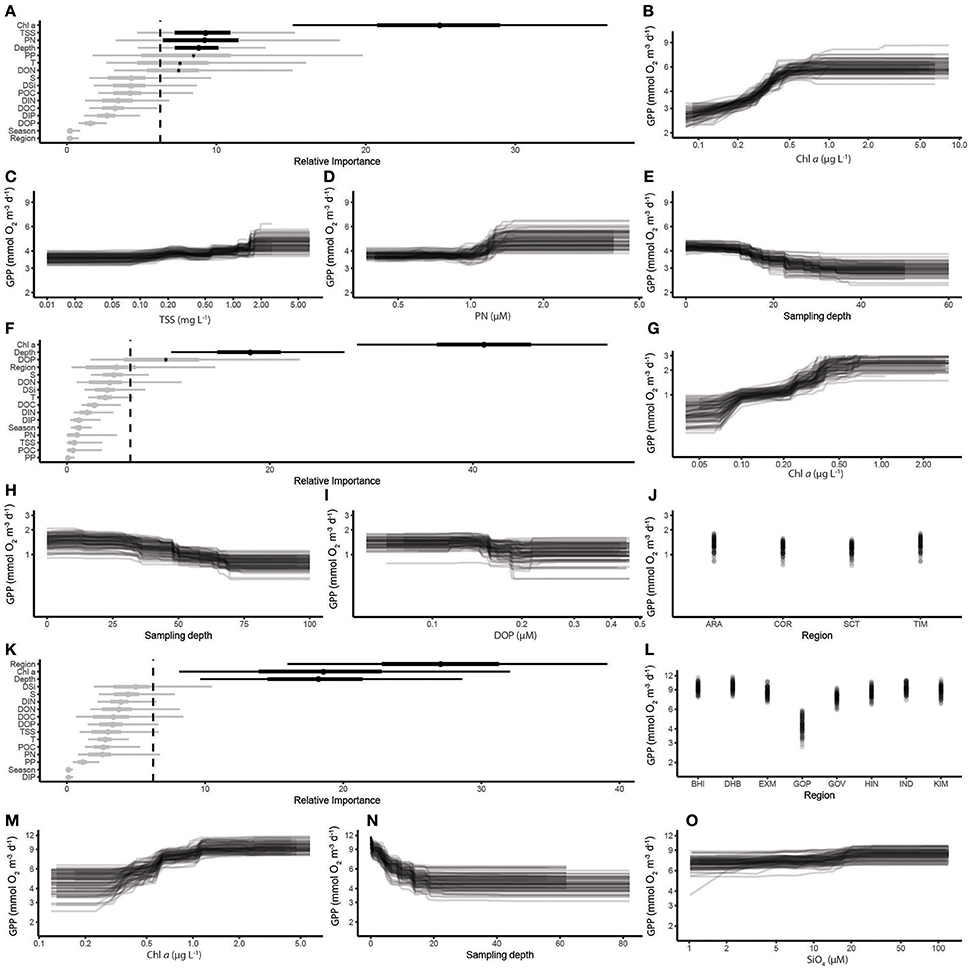
Figure 7. Partial-dependency plots of the response of GPP to the most important predictors based on BRT statistics, as for Figure 6. Coral Reef Ecosystems, (A) relative importance of predictors for coral reef ecosystems; (B) Chl a; (C) TSS; (D) PN; (E) depth; Open Sea Ecosystems (F) relative importance of predictors for open sea ecosystems; (G) Chl a; (H) depth; (I) DOP; (J) region; Coastal Ecosystems (K) relative importance of predictors for coastal ecosystems; (L) region; (M) Chl a; (N) depth; (O) SiO4.
The relationship between CR and GPP with temperature, described by the Arrhenius equation, showed only significant impacts of temperature for CR in the coral reef ecosystems and for GPP in the open sea ecosystems. This yielded apparent activation energies (Ea) of 127 ± 45 kj mol−1 for CR in the coral reef and 266 ± 56 kj mol−1 for GPP in the open sea ecosystems, respectively.
GPP scaled with Chl a to the power of 1.017, and CR scaled with Chl a to the power of 0.645 (Figure 8).
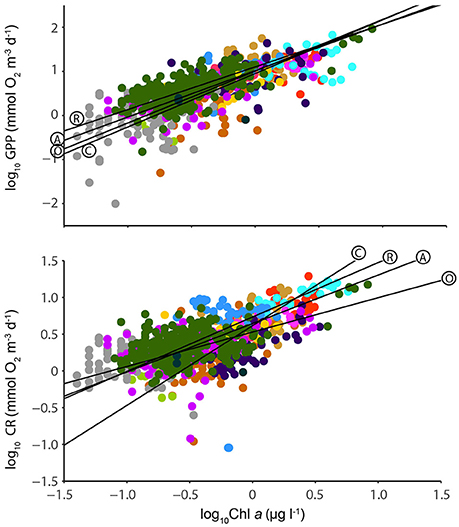
Figure 8. Log-log relationships of GPP (mmol O2 m−3 d−1) and chlorophyll a (μg L-1) (Top) and CR (mmol O2 m−3 d−1) and chlorophyll a (μg L−1) (Bottom). Symbol colors correspond to the location of the measurement as indicated on Figure 1, and regression lines indicated as for Figure 5. Model 2 regression statistics are given in Table 6.
Productivity of Waters Adjacent to Northern Australia
On an area-specific basis, all locations were predominately autotrophic (Figure 9), though some stations in mangrove waterways were heterotrophic in the wet season. In the case of Scott Reef, however, there was positive NCP in only one season (June 2008), and the system was heterotrophic on all other occasions.
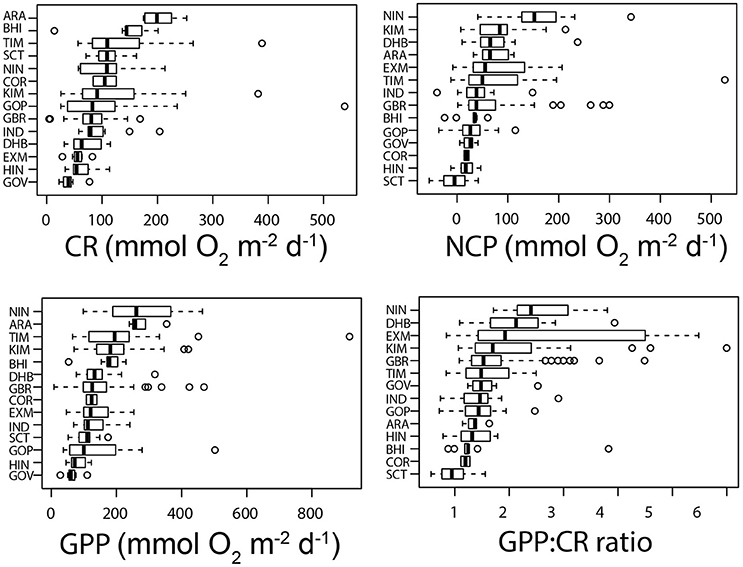
Figure 9. Box plots of area-specific community respiration (CR), area-specific net community production (NCP), area-specific gross primary production (GPP)—all as and the area-specific GPP:CR ratio. The data are ranked by the median. Fifty percent of the data are included within the limit of the boxes, and the error bars represent the 10 and 90th percentiles. Solid lines represent the median and dots represent outlying values.
Comparison of the regional area-specific data reveals a quite different ranking in the scale of metabolic rates to that derived from the volume-specific data (Figure 9), in large part due to the effects of depth (i.e., a deep location with low volume-specific production may have higher area-specific production than a shallow location with very high volume-specific production). Moreover, the ranking of regions varied depending on the variable; for instance, the Arafura Sea ranked highest in terms of area-specific CR whereas Ningaloo ranked highest in NCP, GPP, and GPP:CR. The highest area-specific CR occurred in the Arafura Sea (206 mmol O2 m−2 d−1; Figure 9), where high volume specific CR and a comparatively large depth range (up to 45 m) combined to yield a high area-specific rate.
The locations with the highest values of area-specific NCP include blue-water locations such as Ningaloo (169 mmol O2 m−2 d−1, equivalent to about 2 g Cm−2 d−1), which is fueled by virtue of upwelling jets of nutrient-rich water, and turbid inshore waters such as Darwin Harbour and the Kimberley coast where very high rates of production in a very shallow euphotic zone serve to subsidize respiration throughout the water column. The trophic state of these regions, as indicated by the GPP:CR ratio, reflecting very high rates of autotrophy at Ningaloo, Darwin Harbour and Exmouth Gulf (average ratio ~1.5), and heterotrophy at Scott Reef (average ratio ~0.79) (Figure 9). Heterotrophic stations (ratio below 1) were intermittently observed at Exmouth Gulf, the Timor Sea, the Gulf of Papua, and Hinchinbrook Channel.
Discussion
Plankton CR, NCP, and GPP are key factors in controlling the response of the marine carbon cycle to global ocean warming and eutrophication. Few measurements of plankton CR are available for the tropical ocean and from the southern hemisphere in general (Robinson and Williams, 2005; Regaudie-de-Gioux and Duarte, 2013). Our data greatly expand the information available for shelf seas but include only a few oceanic data points. The mean CR for surface marine waters globally is 3.5 ± 0.13 (SE) mmol O2 m−3 d−1, 4.9 ± 0.23 mmol O2 m−3 d−1 for depths <10 m, 7.4 ± 0.74 mmol O2 m−3 d−1 for coastal waters and 3.3 ± 0.15 mmol O2 m−3 d−1 for the open ocean (Robinson and Williams, 2005). By comparison, our data have regional means ranging from 0.76 mmol O2 m−3 d−1 in the Coral Sea to 10.62 mmol O2 m−3 d−1 in the mangrove waterways of Hinchinbrook Island (Figure 2).
Our data are derived from experiments from a potpourri of seasons and oceanographic conditions; for example our Coral Sea measurements comprise only 2 experiments conducted on an opportunistic basis, whereas other studies include seasonal and spatial contrasts. The larger studies have been published separately, and the reader is referred to those publications for full discussion of pelagic metabolism and the environmental correlates of metabolism in each system (Great Barrier Reef; McKinnon et al., 2013), Gulf of Papua (McKinnon et al., 2007), Timor Sea (McKinnon et al., 2011). The oceanographic setting of the Scott Reef stations (on the margin of the Indian Ocean) places these measurements of metabolism in the same context as those used to support the theory that the open ocean is heterotrophic (Duarte et al., 2013).
Unfortunately, we have very little idea of the organisms responsible for the metabolic activity in our study areas. That said, in pelagic systems generally photosynthetic bacteria and algae are primarily responsible for the formation of organic material (i.e., GPP), but both autotrophic and heterotrophic organisms contribute to CR. Robinson and Williams (2005) noted that organisms <10 μm in size are responsible for at least 75% of CR, and that 99% occurs in organisms <50 μm in size. Respiration by >73 μm zooplankton from the Kimberley coast and the GBR accounted for only 1% of the seasonally averaged whole water respiration (McKinnon et al., 2015). In the tropical coastal waters described in our study picoplankton contributed the overwhelming majority of primary production (Furnas et al., 2005; Furnas and Carpenter, 2016) and there is a rich community of heterotrophic microbes (Alongi et al., 2015). However, coastal waters have a greater proportion of larger cells such as diatoms (e.g., Revelante et al., 1982), though the sporadic occurrence of the diazotrophic cyanobacterium Trichodesmium is common to all regions.
The GPP:CR ratio ranged between 0.84 ± 0.08 and 5.21 ± 0.62 depending on region (Table 3) with an average GPP:CR ratio of 2.09 ± 0.08, which is greater than on most other continental margins (Mackenzie et al., 2005; Jahnke, 2010; Liu et al., 2010; Bauer et al., 2013) (Table 3; Figure 4). Differences in GPP:CR ratios can be caused by differences in (1) light and nutrient availability; (2) food web organization; (3) changes in phytoplankton community structure and cell sizes; and/or (4) bioavailability of the organic matter pool which varies over both seasonal and spatial scales (Mallin and Paerl, 1992; Lønborg and Álvarez-Salgado, 2012; Marañón, 2015). Due to our current limited understanding of plankton community composition and the supply mechanisms of nutrients and organic matter in these waters means it is difficult to account for the main driving force of the variable GPP: CR ratio. The water clarity is high in most of the investigated areas and the shelves are on average shallower than in other systems (Liu et al., 2010), so these factors would tend to foster naturally high rates of autotrophic production in relation to CR. Accordingly, we suggest that the tropical estuarine and coastal regions considered here are mainly autotrophic and do not support the widely held premise that the coastal ocean is heterotrophic (GPP:CR < 1). However, we acknowledge that as this is based on the measurement of dark respiration only, and it is likely that light respiration is higher than in the dark, our values of GPP may be underestimated (Pringault et al., 2007).
In this study we have measured the organic matter fraction that is produced and degraded within a 24 h cycle, but in reality the organic matter pool contains labile compounds with short turnover times (from hours to days), a semi-labile pool with longer turnover times (from weeks to months) and a recalcitrant background pool (Boudreau and Ruddick, 1991; Hansell, 2013). Therefore, estimates of both organic matter degradation rates and water residence time are needed to determine if the organic matter in a water sample is degraded within the shelf or exported to the open ocean. In one of the regions included in our study (the Great Barrier Reef), a geochemical estimate of the carbon balance using radioisotopes suggested a near balance between production and respiration with only minor export of labile organic matter (Brunskill et al., 2010). Our data suggest that tropical coastal waters are indeed exporting organic matter (potentially both particulate and dissolved) to adjacent marine ecosystems.
Environmental Determinants of Metabolism
Our BRT analysis illustrates the relative importance of different environmental variables as predictors of metabolism in the three ecosystem types. For CR, temperature was selected as the most important in coral reef and open ocean ecosystems, while it was less important in coastal ecosystems. Chl a was an important predictor of CR in coastal and open sea ecosystems, but not in coral reef ecosystems, where other variables associated with particulate material (POC, PP) were more important. Regional differences remained the most important predictor of both CR and GPP in the coastal ecosystems, indicating that some communities were more productive than others. The only ecosystem where depth had an important effect on CR was in the open sea ecosystem. This may be related to the differences in temperature through the water column, which was most pronounced in these regions because of the greater depth range. In contrast, Chl a and depth were among the most important determinants of GPP in all three ecosystem types, which is unsurprising given the importance of biomass and light availability in regulating primary production.
In our dataset, volumetric CR scaled as the 0.66 power of GPP overall (0.56, 0.77, and 0.98 powers in open sea, coral reef, and coastal ecosystems respectively; Table 5), in good agreement with previous estimates: 0.79 reported by Regaudie-de-Gioux and Duarte (2013), 0.50 by Duarte and Agustí (1998), and 0.62 by Robinson and Williams (2005). For the relationship between CR and NCP the scaling factors were all lower, confirming the pattern that CR increases more slowly with overall productivity than NCP (Duarte and Agustí, 1998). The scaling factors between CR and both GPP and NCP were highest in coastal ecosystems, and lowest in the open sea (Table 5; Figure 5). Overall, in our dataset the regression of GPP on Chl a explained 50% of the variance across all study regions (Table 6), in contrast to the study of Regaudie-de-Gioux and Duarte (2013) where only 30% of the variance (from mostly oceanic stations) was explained.
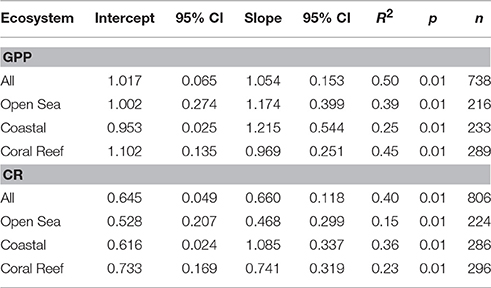
Table 6. Model 2 Regression statistics (RMA) for the relationship between log10 transformed volumetric GPP and CR with log10 transformed chlorophyll respectively (see Figure 8).
Our studies did not show large differences in CR through the water column since depth was only found as a minor influence in open sea ecosystems (Figure 6F) and had no impact in the other ecosystem types. This was not the case for GPP or NCP since these are light dependent and the light climate varied enormously between regions. Some of our regions (Hinchinbrook Channel, Bathurst Island, and some on the Kimberley coast) have highly turbid water where light is virtually extinguished in the top meter of the water column. In these conditions, phytoplankton are distributed throughout the water column by turbulence and adapt to low light conditions by increasing the Chl a concentration per cell (Demers et al., 1986). Under our experimental protocol these cells were maintained in constant light rather than continuously circulated through the range of light climates in the water column, which may inflate the productivity values by holding light-adapted cells in a high light environment, or alternatively deflate them by photoinhibition. Mallin and Paerl (1992) simulated the effects of a variable light climate in a shallow estuary and demonstrated that phytoplankton cells moved rapidly through light-limited conditions as a result of mixing which allowed them sufficient light to maintain high productivity.
The temperature dependence of the ocean's metabolism has been explained using the Arrhenius law within the framework of the metabolic theory of ecology (MTE) (López-Urrutia et al., 2006). This theory scales the metabolic rates by combining the effects of body size and temperature, suggesting that autotrophic and heterotrophic processes in marine systems will react differently to ocean warming, thus possibly changing the metabolic balance and leading to a more heterotrophic ocean (Yvon-Durocher et al., 2010). In accordance with the MTE, Regaudie-de-Gioux and Duarte (2012) found that temperature exerted a strong influence on Chl a-specific metabolic rates. In a similar fashion, we examined the relationship between temperature and Chl a-specific metabolic rates assuming an Arrhenius-type relationship (linearity of the natural logarithm of a rate process vs. inverse absolute temperature). Our analysis showed temperature dependence of CR in the coral reef and GPP in the open sea ecosystems. These disparate responses to temperature may be related to differences in the supply of nutrients, availability of organic matter, and/or food web community composition and structure (Sarmento et al., 2010; López-Urrutia and Morán, 2015; Lønborg et al., 2016). These results are also slightly at odds with our BRT analysis, where temperature was an important predictor of CR in both coral reef and open sea ecosystems, and was not selected as a predictor of GPP in any. These disparities occur because the BRT analysis considered the effects of multiple predictors in parallel. For example, depth was selected as an important predictor of GPP, in large part because of the covariance of temperature (and indeed other variables, e.g., light in the case of GPP) with depth. In combination, our analyses suggest differences in how the metabolic rates in the three ecosystems types will be impacted by increasing ocean temperatures, with least impact found in coastal ecosystems.
Productivity of Coastal Waters Adjacent to Northern Australia
On an area-specific basis, GPP was highest off Ningaloo Reef and in the Arafura Sea. Furnas (2007) showed sporadically very high production rates as a result of cryptic upwelling off Australia's NW Cape, just north of Ningaloo Reef. Furnas and Carpenter (2016) found that the highest primary production in the shelf seas of northern Australia occurred in the Arafura Sea. The water column in the Arafura Sea has a depth of about 65 m and was strongly stratified during the wet season conditions at the time of sampling, with a mixed layer of about 30 m. The bottom layer is sourced from sub-thermocline water in the Timor Trough and the widespread undercurrent-driven upwelling and tidal stirring persistently pump nutrient-rich sub-surface water into the region (Rochford, 1966; Condie, 2011; Kampf, 2015a,b), in turn contributing to rapid phytoplankton growth in the well-insolated surface layers. The high standing stocks of diazotrophs including blooms of Trichodesmium (Montoya et al., 2004) hint at the regional importance of N2 fixation in driving phytoplankton growth and productivity. Very high rates of productivity (up to 7 g C m−2 d−1) are also known to occur in the nearby Banda Sea (Gieskes et al., 1990). At the other end of the productivity spectrum, Scott Reef, on the outer edge of the continental shelf of NW Australia, was the only region that was consistently heterotrophic, though heterotrophy was also observed in the Timor Sea (McKinnon et al., 2011), Hinchinbrook Channel at low tide during the wet season (McKinnon et al., 2010), and in the Coral Sea. Stations at Bathurst Island, Hinchinbrook, and in Indonesia were part of studies of the environmental effects of sea cage aquaculture, and were to some extent enriched by organic wastes from the farms. Despite this, these locations were mainly autotrophic. Our finding of net heterotrophy at the most oceanic stations (Coral Sea, Scott Reef) is in alignment with other studies of the tropical ocean (e.g., Viviani et al., 2011), and fuel the wider debate on the heterotrophy of the global ocean (Duarte et al., 2013). These results furthermore support the idea that the net heterotrophic conditions in more offshore regions could be driven by the export of excess autotrophic production from inshore waters.
Productivity data are usually expressed in units of carbon, since the 14C method has by far the largest number of measurements. Our oxygen-based measurements can be converted to carbon equivalents assuming respiratory and photosynthetic quotients of 1.2 and 1.4 respectively (Bender et al., 1999). On this basis, the locations with the highest GPP (Ningaloo Reef, Arafura Sea) have C-specific CR of 1.3 and 2.5 g C m−2 d−1, and C-specific NCP of 2.0 and 4.3 g C m−2 d−1 respectively. Summing these gives GPP values for Ningaloo Reef of 3.3 g C m−2 d−1 and Arafura Sea of 6.8 g C m−2 d−1. Assuming 14C measurements are 45% of GPP (Bender et al., 1999), then these approximate primary production rates of 1.5 and 3.1 g C m−2 d−1, which are comparable to the 14C measurements at shelf stations near NW Cape (the northern extent of Ningaloo Reef) but about 50% higher than those from the Arafura Sea (Furnas, 2007; Furnas and Carpenter, 2016).
Furnas and Carpenter (2016) reviewed 14C measurements of primary production taken in northern Australian waters over a period of 23 years. Their study domain is very similar to that of the present study, but includes a greater proportion of shelf as opposed to coastal stations. Two of the stations sampled by Furnas and Carpenter (2016) are also included in our data set (Scott Reef and the Arafura Sea), and in fact the water used for both productivity measurements was taken from the same bottle casts. Estimates of total areal production based on 14C from those experiments (Furnas and Carpenter, 2016) account for 41% (Ordinary Least Squares) or 52% (Model 2 regression, Ranged Major Axis) of our estimated GPP (R2 = 0.63), which is in good agreement with the conclusion of Bender et al. (1999) that 14C measurements account for 45% of GPP.
Ranking the Trophic State of Coastal and Shelf Pelagic Ecosystems
Inorganic nutrients turn over very quickly in the tropical coastal waters adjacent to northern Australia. For instance, in the Great Barrier Reef DIN are so rapidly acquired by microbiota as to be fully depleted within hours (Furnas et al., 2005) resulting in standing stocks of nutrients being close to the detection levels of the analytical methods. Despite this, a great deal of research effort has gone into monitoring of “water quality,” especially in the coastal waters of the Great Barrier Reef, primarily by measuring inorganic and organic nutrient species as well as Chl a. The nutrient data have overall proved of very limited use in understanding human impacts because nutrients are so rapidly transformed and have low and invariant standing stocks. In partial recognition of this, increased emphasis has been placed upon the use of Chl a as an index to detect spatial and temporal changes in water quality resulting from increased nutrient loads (e.g., Brodie et al., 2007).
We believe indices of the formation and decay of organic matter are crucial to measure ecosystem health and make informed management decisions. Strictly speaking, the “trophic status” of a water body is defined by the GPP:CR ratio (“P:R ratio”), with a ratio greater than unity denoted autotrophic because there is net production of organic matter, and those less than unity said to be heterotrophic, in which case there is net degradation of organic matter. Therefore, in theory the degree of “eutrophication” of a water body can be quantified by measurement of the GPP:CR ratio, and regions ranked in order of trophic state.
An even better way to rank regions by trophic state may be to use the thresholds necessary to achieve metabolic balance. The threshold GPP below which plankton communities tend to be heterotrophic scales from 0.71 mmol O2 m−3 d−1 in the Coral Sea to 10.05 mmol O2 m−3 d−1 in Hinchinbrook, thus ranking each region on a scale from oligotrophic to eutrophic (Table 4). For comparison, Duarte and Regaudie-de-Gioux (2009) found a global ocean average of 1.07, 1.33 for the Pacific Ocean, and 4.16 mmol O2 m−3 d−1 for the Mediterranean Sea. Comparing our thresholds it is clear that mangroves have a high threshold GPP because of the contribution of labile organic matter from the mangrove forests themselves, but also from tidal resuspension of fine sediments, settled detritus and microphytobenthos (for instance, Bathurst Island has a tidal range of 8 m, some parts of Kimberley coast over 10 m). By contrast, the waters of the central Coral Sea are some of the most oligotrophic in the world ocean, and have a comparatively low threshold GPP. It is also noteworthy that our ranking of regions by trophic state bears little resemblance to that derived on the basis of Chl a alone (Supplementary Table 1).
Phytoplankton growth depends on light availability, which is influenced by cloud cover and turbidity arising from turbulence as a result of wind and tides, all of which can vary on time scales of hours to days. For example, consecutive cloudy and cloud-free days and the resulting changes in irradiance may change the scale of production measured, resulting in high variability in the GPP:CR ratio. Also, if incubation techniques are employed the light climate in experimental containers needs to reflect that in the field, which can be challenging. For example, it has recently been demonstrated that borosilicate flasks widely used for experimental incubations filter out UV light resulting in overestimation of production (Agustí et al., 2014). Moreover, the use of incubators allowing the passage of UV light may also mistakenly include the UV photo-oxidation (oxygen sink) in their plankton productivity term, which can lead to an underestimation of NCP (Kitidis et al., 2014; Gieskes et al., 2015). For a routine and repeatable index of trophic state, we therefore conclude that including production measurements that are light dependent is adding errors that are difficult to control.
The measurement of dark CR is comparatively straightforward and does not have the problems associated with variation in light climate. CR ranks in accordance with what might reasonably be expected of the trophic status of marine ecosystems in northern Australia (Figure 2) and closely resembles the ranking of the threshold necessary to achieve metabolic balance (Table 4). Highest rates are found in the “ultratrophic” (sensu; Burford et al., 2003) mangroves such as those in creeks running into Hinchinbrook Channel, lowest rates in the oligotrophic Coral Sea. Our regression analyses indicate that the measurement of CR accounts for 42% of the variance in GPP, reinforcing the point made by Robinson and Williams (2005) that respiration, rather than primary production, is a both practical and reliable measurement of trophic state. On this basis, we advocate the adoption of measurements of CR as a quantitative tool to monitor the trophic state of coastal waters (Dodds and Cole, 2007).
In conclusion, this study demonstrates that: (1) the tropical coastal waters studied are overall strongly autotrophic (average GPP:CR ratio 2.14 ± 0.98); (2) CR scaled according to the 2/3 power of GPP, and accounted for 42% of the variance in GPP; (3) temperature, Chl a (phytoplankton biomass) and regional differences were the main factors influencing both CR and GPP; (4) the impact of temperature on metabolic rates is ecosystem-dependent, with coastal ecosystems showing least response; and (5) measurements of pelagic metabolism could be used as a quantitative tool to monitor the trophic state of coastal waters.
Author Contributions
AM designed the study. AM and SD collected and processed samples, performed experiments and laboratory assays, and analyzed and interpreted the data. ML conducted statistical analyses. AM and CL wrote the manuscript, with input from all authors. All authors approved the manuscript.
Conflict of Interest Statement
The authors declare that the research was conducted in the absence of any commercial or financial relationships that could be construed as a potential conflict of interest.
Acknowledgments
Financial support was provided by the Australian Institute of Marine Science, with co-investment from other agencies on specific projects. Measurements at Bathurst Island and in Indonesia were supported by Australian Centre for International Agricultural Research (ACIAR) grant FIS/2003/027. The Kimberley component was enabled by collaboration and opportunities developed by AIMS' partnership in the Western Australian Marine Science Institution. The 2011 Kimberley cruises were partially supported by Australian Research Council grant DP1095294 to Greg Ivey (University of Western Australia). We thank Woodside Energy Ltd., as operator of the Browse LNG Development for financial support for work at Scott Reef. Funding was provided by Rio Tinto Alcan Pty. Ltd., to work at Nhulunbuy (Gove). We thank the crews of the RV Cape Ferguson, RV Solander, Southern Surveyor, and Melville for their invaluable support in the field. We thank the two reviewers for their valuable comments on the manuscript.
Supplementary Material
The Supplementary Material for this article can be found online at: https://www.frontiersin.org/articles/10.3389/fmars.2017.00346/full#supplementary-material
References
Agustí, S., Regaudie-de-Gioux, A., Arrieta, J. M., and Duarte, C. M. (2014). Consequences of UV-enhanced community respiration for plankton metabolic balance. Limnol. Oceanogr. 59, 223–232. doi: 10.4319/lo.2014.59.1.0223
Ahlgren, G. (1987). Temperature functions in biology and their application to algal growth constants. Oikos 49, 177–190.
Alongi, D. M., and McKinnon, A. D. (2011). Impact of hydrotalcite deposition on biogeochemical processes in a shallow tropical bay. Mar. Environ. Res. 71, 111–121. doi: 10.1016/j.marenvres.2010.11.006
Alongi, D. M., McKinnon, A. D., Brinkman, R., Trott, L. A., Undu, M. C., Muawanah, et al. (2009). The fate of organic matter derived from small-scale fish cage aquaculture in coastal waters of Sulawesi and Sumatra, Indonesia. Aquaculture 295, 60–75. doi: 10.1016/j.aquaculture.2009.06.025
Alongi, D. M., Patten, N. L., McKinnon, A. D., Köstner, N., Bourne, D. G., and Brinkman, R. (2015). Phytoplankton, bacterioplankton and virioplankton structure and function across the southern Great Barrier Reef shelf. J. Mar. Syst. 142, 25–39. doi: 10.1016/j.jmarsys.2014.09.010
Arrhenius, S. Z. (1889). Über die Reaktionsgeschwindigkeit bei der Inversion von Rohrzucker durch Säuren. Phys. Chem. 4, 226–248.
Bauer, J. E., Cai, W.-J., Raymond, P. A., Bianchi, T. S., Hopkinson, C. S., and Regnier, P. A. G. (2013). The changing carbon cycle of the coastal ocean. Nature 504, 61–70. doi: 10.1038/nature12857
Bender, M., Orchardo, J., Dickson, M., Barber, R., and Lindley, S. (1999). In vitro O2 fluxes compared with 14C production and other rate terms during the JGOFS Equatorial Pacific experiment. Deep Sea Res. I 46, 637–654.
Boudreau, B. P., and Ruddick, B. R. (1991). On a reactive continuum representation of organic matter diagenesis. Am. J. Sci. 291, 507–538.
Brodie, J., De'ath, G., Devlin, M., Furnas, M., and Wright, M. (2007). Spatial and temporal patterns of near-surface chlorophyll a in the Great Barrier Reef lagoon. Mar. Freshw. Res. 58, 342–353. doi: 10.1071/MF06236
Brunskill, G. J. (2010). “An overview of tropical margins,” in Carbon and Nutrient Fluxes in Continental Margins, eds K.-K. Liu, L. Atkinson, R. Quinones, and L. Talaue-McManus (Berlin: Springer), 423–426.
Brunskill, G. J., Zagorskis, I., and Pfitzner, J. (2010). “Tropical coastal seas of Australia and Papua New Guinea,” in Carbon and Nutrient Fluxes in Continental Margins, eds K.-K. Liu, L. Atkinson, R. Quinones, and L. Talaue-McManus (Berlin: Springer), 482–493.
Burford, M. A., Alongi, D. M., McKinnon, A. D., and Trott, L. A. (2008). Primary production and nutrients in a tropical macrotidal estuary, Darwin Harbour, Australia. Estuar. Coast. Shelf Sci. 79, 440–448. doi: 10.1016/j.ecss.2008.04.018
Burford, M. A., Costanzo, S. D., Dennison, W. C., Jackson, C., Jones, A., McKinnon, A. D., et al. (2003). A synthesis of dominant ecological processes in intensive shrimp ponds and adjacent coastal environments in NE Australia. Mar. Pollut. Bull. 46, 1456–1469. doi: 10.1016/S0025-326X(03)00282-0
Burns, K. A., Brunskill, G. J., Brinkman, D., and Zagorskis, I. (2008). Organic carbon and nutrient fluxes to the coastal zone from the Sepik River outflow. Cont. Shelf Res. 28, 283–301. doi: 10.1016/j.csr.2007.08.004
Condie, S. A. (2011). Modeling seasonal circulation, upwelling and tidal mixing in the Arafura and Timor Seas. Cont. Shelf Res. 31, 1427–1436. doi: 10.1016/j.csr.2011.06.005
De'ath, G. (2007). Boosted trees for ecological modeling and prediction. Ecology 88, 243–251. doi: 10.1890/0012-9658(2007)88[243:BTFEMA]2.0.CO;2
Demers, S. L., Legendre, L., and Therriault, J. C. (1986). “Phytoplankton responses to vertical tidal mixing,” in Tidal Mixing and Plankton Dynamics, eds M. J. Bowman, C. M. Yentsch, and W. T. Peterson (Berlin: Springer), 1–40.
Dodds, W. K., and Cole, J. J. (2007). Expanding the concept of trophic state in aquatic ecosystems: it's not just the autotrophs. Aquat. Sci. 69, 427–439. doi: 10.1007/s00027-007-0922-1
Duarte, C. M., and Agustí, S. (1998). The CO2 balance of unproductive aquatic ecosystems. Science 281, 234–236.
Duarte, C. M., and Regaudie-de-Gioux, A. (2009). Thresholds of gross primary production for the metabolic balance of marine planktonic communities. Limnol. Oceanogr. 54, 1015–1022. doi: 10.4319/lo.2009.54.3.1015
Duarte, C. M., Regaudie-de-Gioux, A., Arrieta, J. M., Delgado-Huertas, A., and Agustí, S. (2013). The oligotrophic ocean is heterotrophic. Ann. Rev. Mar. Sci. 5, 1–19. doi: 10.1146/annurev-marine-121211-172337
Ducklow, H. W., and Doney, S. C. (2013). What is the metabolic state of the oligotrophic ocean? A debate. Annu. Rev. Mar. Sci. 5, 525–533. doi: 10.1146/annurev-marine-121211-172331
Ducklow, H. W., and McAllister, S. L. (2005). “The biogeochemistry of carbon dioxide in the coastal oceans,” in The Sea, Vol. 13, eds A. R. Robinson and K. H. Brink (Cambridge, MA: Harvard University Press), 269–315.
Elith, J., Leathwick, J. R., and Hastie, T. (2008). A working guide to boosted regression trees. J. Anim. Ecol. 77, 802–813. doi: 10.1111/j.1365-2656.2008.01390.x
Furnas, M. (2007). Intra-seasonal and inter-annual variations in phytoplankton biomass, primary production and bacterial production at North West Cape, Western Australia: Links to the 1997–1998 El Ni-o event. Cont. Shelf Res. 27, 958–980. doi: 10.1016/j.csr.2007.01.002
Furnas, M. J., Alongi, D. M., McKinnon, D., Trott, L., and Skuza, M. (2011). Regional-scale nitrogen and phosphorus budgets for the northern (14°S) and central (17°S) Great Barrier Reef shelf ecosystem. Cont. Shelf Res. 31, 1967–1990. doi: 10.1016/j.csr.2011.09.007
Furnas, M. J., and Carpenter, E. J. (2016). Primary production in the tropical continental shelf seas bordering northern Australia. Cont. Shelf Res. 129, 33–48. doi: 10.1016/j.csr.2016.06.006
Furnas, M., Mitchell, A., Skuza, M., and Brodie, J. (2005). In the other 90%: phytoplankton responses to enhanced nutrient availability in the Great Barrier Reef Lagoon. Mar. Pollut. Bull. 51, 253–265. doi: 10.1016/j.marpolbul.2004.11.010
Gasol, J., and Duarte, C. M. (2000). Comparative analyses in aquatic microbial ecology: how far do they go? FEMS Microb. Ecol. 31, 99–106. doi: 10.1111/j.1574-6941.2000.tb00675.x
Gattuso, J.-P., Frankignoulle, M., and Wollast, R. (1998). Carbon and carbonate metabolism in coastal aquatic ecosystems. Annu. Rev. Ecol. Syst. 29, 405–433.
Gieskes, W. W. C., Laane, R. W. P. M., and Ruardij, P. (2015). Photo-oxidation: major sink of oxygen in the ocean surface layer. Mar. Chem. 177, 472–475. doi: 10.1016/j.marchem.2015.06.003
Gieskes, W. W., Kraay, G. W., Nontji, A., Setiapermana, D., and Sutomo (1990). Monsoonal differences in primary production in the eastern Banda Sea (Indonesia). Netherl. J. Sea Res. 25, 473–483.
Hansell, D. A. (2013). Recalcitrant dissolved organic carbon fractions. Ann. Rev. Mar. Sci. 5, 421–445. doi: 10.1146/annurev-marine-120710-100757
Hansen, H. P., and Koroleff, F. (1999). “Determination of nutrients,” in Methods of Seawater Analysis, 3rd Edn., eds K. Grasshoff, K. Kremling, and M. Ehrhardt (Weinheim: Wiley-VCH Verlag GmbH). doi: 10.1002/9783527613984.ch10
Hanson, C. E., and McKinnon, A. D. (2009). Pelagic ecology of the Ningaloo region, Western Australia: influence of the Leeuwin Current. J. R. Soc. W A 92, 129–137.
Hastie, T., Tibshirani, R., and Friedman, J. (2011). The Elements of Statistical Learning: Data Mining, Inference, and Prediction, 2nd Edn. Berlin: Springer, 698.
Jahnke, R. A. (2010). “Global synthesis”, in Carbon and Nutrient Fluxes in Continental Margins, eds K.-K. Liu, L. Atkinson, R. Quinones, and L. Talaue-McManus (Berlin: Springer), 597–615.
Kampf, J. (2015a). Undercurrent-driven upwelling in the northwestern Arafura Sea. Geophys. Res. Lett. 42, 9362–9368. doi: 10.1002/2015GL066163
Kampf, J. (2015b). On the majestic seasonal upwelling system of the Arafura Sea. J. Geophys. Res. Ocean 121, 1218–1228. doi: 10.1002/2015JC011197
Kitidis, V., Tilstone, G. H., Serret, P., Smyth, T. J., Torres, R., and Robinson, C. (2014). Oxygen photolysis in the Mauritanian upwelling: implications for net community production. Limnol. Oceanogr. 59, 299–310. doi: 10.4319/lo.2014.59.2.0299
Liu, K.-K., Atkinson, L., Qui-ones, R. A., and Talaue-McManus, L. (2010). “Biogeochemistry of continental margins in a global context,” in Carbon and Nutrient Fluxes in Continental Margins, eds K.-K. Liu, L. Atkinson, R. A. Qui-ones, and L. Talaue-McManus (Berlin: Springer), 3–24.
Lønborg, C., and Álvarez-Salgado, X. A. (2012). Recycling versus export of bioavailable dissolved organic matter in the coastal ocean and efficiency of the continental shelf pump. Global Biogeochem. Cycles 26. doi: 10.1029/2012GB004353
Lønborg, C., Cuevas, A., Reinthaler, T., Herndl, G., Gasol, J. M., Morán, X. A. G., et al. (2016). Depth dependent relationships between temperature and ocean heterotrophic prokaryotic production. Front. Mar. Sci. 3:90. doi: 10.3389/fmars.2016.00090
López-Urrutia, Á., and Morán, X. A. G. (2015). Temperature affects the size-structure of phytoplankton communities in the ocean. Limnol. Oceanogr. 60, 733–738. doi: 10.1002/lno.10049
López-Urrutia, Á., San Martin, E., Harris, R. P., and Irigoien, X. (2006). Scaling the metabolic balance of the oceans. Proc. Natl. Acad. Sci. U.S.A. 103, 8739–8744. doi: 10.1073/pnas.0601137103
Mackenzie, F. T., Andersson, A., Lerman, A., and Ver, L. M. (2005). “Boundary exchanges in the global coastal margin: implications for the organic and inorganic carbon cycles,” in The Sea, The Global Coastal Ocean: Multiscale Interdisciplinary Processes, Vol. 13, eds A. R. Robinson and K. H. Brink (Cambridge, MA: Harvard University Press), 193–225.
Mallin, M. A., and Paerl, H. W. (1992). Effects of variable irradiance on phytoplankton productivity in shallow estuaries. Limnol. Oceanogr. 37, 54–62.
Marañón, E. (2015). Cell size as a key determinant of phytoplankton metabolism and community structure. Annu. Rev. Mar. Sci. 7, 241–264. doi: 10.1146/annurev-marine-010814-015955
Martínez-García, S., Fernández, E., Álvarez-Salgado, X. A., González, J., Lønborg, C., Maranon, E., et al. (2010). Differential responses of phytoplankton versus heterotrophic bacteria after organic and inorganic nutrient additions in coastal waters off NW Iberian Peninsula. Mar. Ecol. Prog. Ser. 416, 17–33. doi: 10.3354/meps08776
McKinnon, A. D., Carleton, J. H., and Duggan, S. (2007). Pelagic production and respiration in the Gulf of Papua during May 2004. Cont. Shelf Res. 27, 1643–1655. doi: 10.1016/j.csr.2007.01.026
McKinnon, A. D., Carleton, J. H., and Duggan, S. (2011). Determinants of pelagic metabolism in the Timor Sea during the inter-monsoon period. Mar. Freshw. Res. 62, 130–140. doi: 10.1071/MF10170
McKinnon, A. D., Doyle, J., Duggan, S., Logan, M., and Lønborg, C. (2015). Zooplankton growth, respiration and grazing on the Australian margins of the tropical Indian and Pacific oceans. PLoS ONE 10:e0140012. doi: 10.1371/journal.pone.0140012
McKinnon, A. D., Logan, M., Castine, S., and Duggan, S. (2013). Pelagic metabolism in the waters of the Great Barrier Reef. Limnol. Oceanogr. 58, 1227–1242. doi: 10.4319/lo.2013.58.4.1227
McKinnon, A. D., Trott, L. A., Brinkman, R., Duggan, S., Castine, S., O'Leary, R., et al. (2010). Seacage aquaculture in a World Heritage Area: the environmental footprint of a Barramundi farm in tropical Australia. Mar. Pollut. Bull. 60, 1489–1501. doi: 10.1016/j.marpolbul.2010.04.021
Montoya, J. P., Holl, C. M., Zehr, J. P., Hansen, A., and Villareal TA Capone, D. G. (2004). High rates of N2 fixation by unicellular diazotrophs in the oligotrphic Pacific Ocean. Nature 430, 1027–1031. doi: 10.1038/nature02824
Nieuwenhuize, J., Maas, Y. E. M., and Middelburg, J. J. (1994). Rapid analysis of organic carbon and nitrogen in particulate materials. Mar. Chem. 5, 217–224.
Nittrouer, C. A., Brunskill, G. J., and Figueiredo, A. G. (1995). Importance of tropical coastal environments. Geo Mar. Lett. 15, 121–126.
Platt, T., Jauhari, P., and Sathyendranath, S. (1992). “The importance and measurement of new production,” in Primary Productivity and Biogeochemical Cycles in the Sea, eds P. G. Falkowski and A. D. Woodhead (New York, NY: Plenum Press), 273–284.
Pringault, O., Tassas, V., and Rochelle-Newall, E. (2007). Consequences of respiration in the light on the determination of production in pelagic systems. Biogeosciences 4, 105–114. doi: 10.5194/bg-4-105-2007
R Core Team (2016). R: A Language and Environment for Statistical Computing. Vienna: R Foundation for Statistical Computing. Available online at: https://www.R-project.org/.
Redfield, A. C., Ketchum, B. K., and Richards, F. A. (1963). “The influence of organisms on the composition of sea–water,” in The Sea, Vol. 2, The Composition of Sea Water: Comparative and Descriptive Oceanography, ed M. N. Hill (New York, NY: Wiley–Interscience), 26–77.
Regaudie-de-Gioux, A., and Duarte, C. M. (2012). Temperature dependence of planktonic metabolism in the ocean. Global Biogeochem. Cycles 26:GB1015. doi: 10.1029/2010GB003907
Regaudie-de-Gioux, A., and Duarte, C. M. (2013). Global patterns in oceanic planktonic metabolism. Limnol. Oceanogr. 58, 977–986. doi: 10.4319/lo.2013.58.3.0977
Revelante, N., Williams, W. T., and Bunt, J. S. (1982). Temporal and spatial distribution of diatoms, dinoflagellates and Trichodesmium in waters of the Great Barrier Reef. J. Exp. Mar. Biol. Ecol. 63, 27–45.
Ridgeway, G. (2017). Gbm: Generalized Boosted Regression Models. Available online at: https://CRAN.R-project.org/package=gbm.
Robinson, C., Serret, P., Tilstone, G., Teira, E., Zubkov, M. V., Rees, A. P., et al. (2002). Plankton respiration in the Eastern Atlantic Ocean. Deep Sea Res. I 49, 787–813. doi: 10.1016/S0967-0637(01)00083-8
Robinson, C., and Williams, P. J. L. B. (2005). “Respiration and its measurement in surface marine waters,” in Respiration in Aquatic Ecosystems, eds P. A. del Giorgio and P. J. L. B. Williams (Oxford: Oxford University Press), 147–180.
Rochford, D. J. (1966). Some hydrological features of the eastern Arafura Sea and the Gulf of Carpentaria in August 1964. Aust. J. Mar. Freshw. Res. 17, 31–60.
Sarmento, H., Montoya, J. M., Vázquez-Domínguez, E., Vaqué, D., and Gasol, J. M. (2010). Warming effects on marine microbial food web processes: how far can we go when it comes to predictions? Philos. Trans. R. Soc. B Biol. Sci. 365, 2137–2149. doi: 10.1098/rstb.2010.0045
Serret, P., Fernandez, E., Sostres, J. A., and Anadon, R. (1999). Seasonal compensation of plankton production and respiration in a temperate sea. Mar. Ecol. Prog. Ser. 187, 43–57.
Smith, S. V., and Hollibaugh, J. T. (1993). Coastal metabolism and the oceanic organic carbon balance. Rev. Geophys. 31, 75–89.
Smith, S. V., and Mackenzie, F. T. (1987). The ocean as a net heterotrophic system: implications from the carbon biogeochemical cycle. Global Biogeochem. Cycles 1, 187–198.
Valderrama, J. C. (1981). The simultaneous analysis of total nitrogen and total phosphorus in natural waters. Mar. Chem. 10, 109–122.
Viviani, D., Björkman, K., Karl, D., and Church, M. (2011). Plankton metabolism in surface waters of the tropical and subtropical Pacific Ocean. Aquat. Microb. Ecol. 62, 1–12. doi: 10.3354/ame01451
Yentsch, C. S., and Menzel, D. W. (1963). A method for the determination of phytoplankton chlorophyll and phaeophytin by fluorescence. Deep Sea Res. Oceanogr. Abstr. 10, 221–231.
Keywords: plankton respiration, primary production, metabolic balance, trophic state, tropical coastal waters
Citation: McKinnon AD, Duggan S, Logan M and Lønborg C (2017) Plankton Respiration, Production, and Trophic State in Tropical Coastal and Shelf Waters Adjacent to Northern Australia. Front. Mar. Sci. 4:346. doi: 10.3389/fmars.2017.00346
Received: 23 June 2017; Accepted: 16 October 2017;
Published: 31 October 2017.
Edited by:
Gordon T. Taylor, Stony Brook University, United StatesReviewed by:
Pablo Serret, University of Vigo, SpainGotzon Basterretxea, Consejo Superior de Investigaciones Científicas (CSIC), Spain
Copyright © 2017 McKinnon, Duggan, Logan and Lønborg. This is an open-access article distributed under the terms of the Creative Commons Attribution License (CC BY). The use, distribution or reproduction in other forums is permitted, provided the original author(s) or licensor are credited and that the original publication in this journal is cited, in accordance with accepted academic practice. No use, distribution or reproduction is permitted which does not comply with these terms.
*Correspondence: A. David McKinnon, ZC5tY2tpbm5vbkBhaW1zLmdvdi5hdQ==; ZGF2b21ja0BnbWFpbC5jb20=