- 1Department of Biology, San Diego State University, San Diego, CA, United States
- 2Marine Biology Research Division, Scripps Institution of Oceanography, University of California, San Diego, La Jolla, CA, United States
- 3Science Division, National Audubon Society, San Francisco, CA, United States
Conservation of marine megafauna is nested within an intricate tapestry of multiple ocean resource uses which are, in turn, embedded in a dynamic and complex ecological ocean system that varies and shifts across a wide range of spatial and temporal scales. Marine megafauna conservation is often further complicated by contemporaneous, and sometimes competing, social, economic, and ecological factors and related management objectives. Advances in emerging technologies and applications, such as remotely-sensed oceanographic data, animal-based telemetry, novel computational analyses, innovations in structured decision making, and stakeholder engagement and policy are supporting complex systems and complexity-aware approaches to megafauna conservation and research. Here we discuss several applications that focus on megafauna fisheries bycatch and exemplify how complex systems and complexity-aware approaches that inherently acknowledge and account for the complexity of ocean systems can advance megafauna conservation and research. Emerging technologies, applications and approaches that embrace, rather than ignore, complexity can drive innovation and success in megafauna conservation and research.
Introduction
“Stop trying to change reality by attempting to eliminate complexity”
-David Whyte
Marine megafauna, which we define as large-bodied, ocean dwellers like sea turtles, seabirds, marine mammals, and sharks, have experienced dramatic declines in many ocean regions (Davidson et al., 2012; Paleczny et al., 2015). The conservation of marine megafauna populations worldwide is challenged by a suite of pressures, many stemming directly from human activity, including incidental capture in fisheries or bycatch (Lewison et al., 2014), shipping strikes (Kraus et al., 2005; Panigada et al., 2006), direct harvest (Clapham, 2015; Fisher, 2016; Hofman, 2016), and contaminant exposure and accumulation (Law, 2014). In response to these pressures, research, policy, and education or conservation awareness campaigns regarding marine megafauna conservation issues have increased considerably (Authier et al., 2017). However, while megafauna research, conservation policies, and education efforts have produced important results (Taylor et al., 2000; Boyd et al., 2016; Morin et al., 2016), we suggest that many efforts have yet to adequately embrace the complexity of the systems in which marine megafauna reside. Given the mounting pressure on ocean resources and the growing concerns regarding marine megafauna conservation (National Academy of Sciences, 2017), failing to acknowledge and account for this inherent complexity may hinder much needed advances and success in marine megafauna research and conservation.
What are Complex Systems Analyses?
Complex systems approaches are not a single type of analyses, rather they are a diverse suite of conceptual, analytical, and computational methodologies that can be applied to “wicked” or unstructured problems (Jentoft and Chuenpagdee, 2009; Balint et al., 2011). To understand how complex systems approaches can support megafauna conservation and research, we must first define complex systems and complex system analyses. A complex system is one with a high number and diversity of interacting components or elements (Levin, 1999; Green et al., 2005). Complexity in natural systems arises when the system is influenced by multiple processes operating at disparate spatial and temporal scales—as is the case for ocean systems and many of the processes within them. Originating, in part, from general systems theory (Bertalanffy, 1968; Warren et al., 1998), complex system analysis focuses on capturing the linked and often reciprocal nature of a system's heterogeneous elements (Arthur, 1999; Manson, 2001; Strogatz, 2001; Levin et al., 2012). A complex systems approach contrasts to a reductionist scientific approach which assumes complex, dynamic, emergent phenomena can be described in terms of their individual, constituent parts and their interactions. Complexity can be measured in many forms, including non-linearity, multi-element feedback loops, path-dependence, self-organization, difficulty of prediction, and emergence of qualities not analytically tractable from system components and their attributes alone (Manson, 2001; Bankes, 2002; National Research Council, 2012). Methods and techniques of complex systems science include, but are not limited to, nonlinear dynamic analysis, cellular automata, agent-based modeling, information and network theory, and machine learning (Shalizi, 2006).
Technological and computational advances have increased the tractability of complex system approaches (Levin et al., 1997; Green et al., 2005) and in some ocean research domains, like fisheries science and fisheries policy, complex systems analyses have been adopted to some degree (Wilson et al., 1994; Knowlton, 2004; Anderson et al., 2008; Mahon et al., 2008; Glaser et al., 2013). Although less commonly applied to marine megafauna, studies that have embraced true complex system approaches highlight the utility of embracing complexity. For example, Agent Based Models (ABMs) that simulate the actions and interactions of autonomous agents have been used to successfully evaluate individual decision strategies of boat users in whale watching operations and how these collectively impact local whale populations in the St. Lawrence river, Canada (Anwar et al., 2007) and more recently to investigate optimal strategies for the monitoring of green turtle (Chelonia mydas) populations in Hawaii (Piacenza et al., 2017). Machine learning methods have been used to help devise complex models and algorithms for prediction to classify probable behaviors and to estimate habitat areas of importance in seabird populations (Guilford et al., 2009; Fox et al., 2017) whilst artificial neural networks that identify patterns through unguided simulations have been used to evaluate breeding habitat suitability for New Zealand fur seals (Arctocephalus forsteri) (Bradshaw et al., 2002). Finally, a number of recent advances have been made in understanding marine megafauna behavior by addressing the dynamic state space of behavior and by developing big-data approaches that require no “a priori” assumptions about the behaviors of study animals (Beyer et al., 2013). Other examples include the use of Stochastic Dynamic Programming (SDP) and state-dependent behavioral theory to investigate how disturbance affects pinniped pup recruitment (McHuron et al., 2017), a dynamic state model of blue whale migratory behavior and physiology to explore the effects of perturbations on reproductive success (Balaenoptera musculus) (Pirotta et al., 2018), and a study of tagged southern elephant seals (Mirounga leonina) that identifies intrinsic drivers of movement, to describe the migratory and foraging habitats (Rodríguez et al., 2017). State space models have also been used to characterize dynamic movement of sea turtles (Jonsen et al., 2007; Bailey et al., 2008), seabirds (Dean et al., 2013), other marine mammal species (Moore and Barlow, 2011), and sharks (Block et al., 2011).
One common feature of the examples of traditional complex system analyses is data richness, i.e., traditional complex systems analyses are data intensive. For this reason, a strictly defined complex systems approach may be challenging for many data limited ocean megafauna research and conservation efforts (Pott and Wiedenfeld, 2017). However, we suggest that even when data availability may limit the application of traditional complex system analyses, adopting complexity-aware approaches that acknowledge and strive to account for system complexity and adopt the fundamental precepts of complexity will be instrumental in advancing megafauna conservation and research. The term complexity-aware has been used in computing and computer science since the early 1990's (Mukherjee and van der Schaar, 2005). Outside of computer science, the concept of complexity-awareness has more recently been adopted in the context of social change, participatory research and project management by the non-governmental organization community (Paludan, 2015; US AID, 2016). Complexity-awareness acknowledges the prevalence and importance of non-linear, unpredictable interrelationships, non-linear causality and emergent properties, in essence, the tenets of a complex systems approach.
While the term complexity-awareness has not yet been widely adopted in marine megafauna conservation or the natural resource community, calls to increase and maintain complexity in ecological research and conservation efforts are growing (Crowder and Norse, 2008; Anand et al., 2010; Stirling, 2010; Parrott and Meyer, 2012; Howarth et al., 2013; Evans et al., 2017; Johnson and Lidstrom, 2018). Much of this growing body of literature articulates how complexity and complexity-aware frameworks and analyses can be adopted and applied in conservation science, affirming the need to incorporate complexity into the conservation science landscape, particularly in response to the growing threats and stressors on coupled ecological-human systems. Figure 1 captures this concept and illustrates the complexity landscape as a function of data availability (x axis), conceptual complexity (y axis), and analytical complexity (z axis). While traditional complex systems analyses will be data intensive and typically include a high level of analytical and conceptual complexity, even data-poor applications can adopt a high degree of conceptual complexity and be complexity-aware.
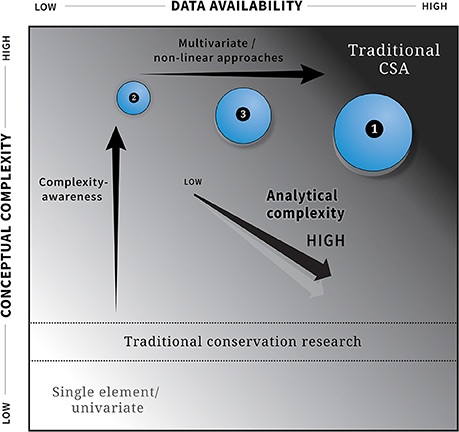
Figure 1. The three dimensional complexity landscape represented by three axes: (a) data availability (x-axis), (b) conceptual complexity (y-axis), (c) analytical complexity (z-axis), where circle size denotes analytical complexity (larger circles represent higher analytical complexity). Traditional Complex System Analyses (CSA) are possible when conceptual complexity, analytical complexity and data availability are high. As conceptual complexity increases, an analysis or application becomes complexity-aware even when data availability or analytical complexity are low. The three examples discussed in the text (1) dynamic ocean management, (2) spatially-explicit risk assessment and (3) the integration of complexity into economic and social domains are shown relative to these three axes.
How Can Complex System Analyses and Complexity-Awareness Support Megafauna Conservation in a Fisheries Bycatch Context?
We illustrate complex systems analyses and complexity-aware approaches in the context of fisheries bycatch, one of the most significant anthropogenic threats to marine megafauna (Lewison et al., 2014). Fisheries bycatch, the incidental capture of unwanted, unused, or unmanaged non-target species (Davies et al., 2009), is symptomatic of one of the central challenges to ocean fisheries—how to balance ecological sustainability with economic and social viability. Megafauna bycatch is a product of susceptibility driven by the distribution, type, and magnitude of fisheries effort, and vulnerability based on ecological characteristics such as life history and species distribution traits of the bycatch species (Lewison et al., 2014). For some megafauna species, such as Pacific leatherback turtle (Dermochelys coriacea), Amsterdam Albatross (Diomedea amsterdamensis), vaquita (Phocoena sinus), Atlantic humpbacked dolphin (Sousa teuszii), and Australian and New Zealand sea lion (Neophoca cinerea and Phocarctos hookeri), fisheries bycatch has been identified as the single largest threat to extant populations (Weimerskirch et al., 1987; Lewison et al., 2004; Chilvers, 2008; Weir et al., 2011; Hamer et al., 2013; Taylor et al., 2016).
In the past decade, research and development of gear and fishing practice modifications have advanced considerably and have made important progress in reducing megafauna bycatch. For some coastal drift and gillnet fisheries, deployment of visual or acoustic deterrents has been shown to substantially reduce seabird bycatch (Melvin et al., 1999; Maree et al., 2014), while acoustic alarms (pingers) have been demonstrated to decrease bycatch for multiple marine mammal species (Dawson et al., 1998; Barlow and Cameron, 2003; Carretta and Barlow, 2011; Mangel et al., 2013; Larsen and Eigaard, 2014), and buoyless nets have been found to reduce sea turtle bycatch (Peckham et al., 2016). The use of turtle exclusion devices (TEDs) can also be highly effective in reducing sea turtle bycatch in trawl fisheries (Crowder et al., 1994; Lewison et al., 2003) as can the simple use of net lights in small scale gillnet fisheries (Ortiz et al., 2016; Virgili et al., 2017). The implementation of circle hooks, alternate baits and bird scaring devices and improved setting practices in longline fisheries has been shown across multiple studies to reduce bycatch of sea turtles and seabirds as well as sharks and other non-target fishes (Gilman et al., 2005, 2007; Watson et al., 2005; Kerstetter and Graves, 2006). A number of these gear modifications have also increased survival rates for animals that are caught and released.
Despite bycatch mitigation innovations and advances, bycatch of megafauna remains a substantial challenge to population viability largely because addressing bycatch is an inherently complex problem. This complexity stems from the need to balance the benefits of bycatch reduction against the costs of altered fishing activity to fishers' livelihoods and culture. In addition, the diversity among the regulatory, logistical, and socio-cultural constraints that create complex context dependencies influencing bycatch reduction efficacy needs careful consideration. Further, complexity associated with data collection, integration, and analysis, and the complex nature of the dynamic ocean itself, with macro-, meso-, and micro-scale temporal and spatial variability in ocean structure, processes and species distributions all must be considered (Hazen et al., 2013). Here, we describe several approaches that address megafauna fisheries bycatch by embracing complex system and complexity-aware analyses or frameworks.
Dynamic Ocean Management
Dynamic ocean management (DOM) is an example of a complex systems approach that can support or supplement traditional management strategies to support sustainable fishery targets. DOM is an emerging management paradigm in which management responses change in space and time, at scales relevant for animal movement and human use. What differentiates DOM from static or traditional ocean management approaches is the use of real-time or near real-time data on the shifting physical, biological, socioeconomic, and other characteristics of the ocean and ocean resource users to generate responsive spatial management measures or strategies (Maxwell et al., 2012; Hobday and Hartog, 2014; Lewison et al., 2015). DOM holds promise for bycatch reduction, protected area design (Dunn et al., 2016) and management of populations of highly migratory and protected marine megafauna (Maxwell et al., 2015) because it integrates biological, ecological, environmental, and socioeconomic data collected over multiple spatiotemporal scales to provide information to managers and resource users in near real time (Hobday et al., 2014; Lewison et al., 2015).
While not all DOM approaches adopt traditional complex system approaches, many employ complex systems and complexity-aware ecological informatic or eco-informatics approaches (sensu Hobday et al., 2010; Scales et al., 2017; Brodie et al., 2018; Hazen et al., 2018). These cited examples use innovative digital approaches to the generation, sampling, processing, analysis, visualization, management, and dissemination of ecological, environmental, and socioeconomic data (Michener and Jones, 2012) and account for complexity at a number of levels. Central to these applications is the capacity to acknowledge complex, often non-linear and emergent, relationships between oceanographic and biological data using species distribution models (Elith and Leathwick, 2009; Žydelis et al., 2011; McGowan et al., 2013; Becker et al., 2014, 2016; Hobday et al., 2014), often using complex ocean circulation models. Rapid developments in ocean modeling have supported the integration of species distribution models with Regional Ocean Modeling Systems (ROMS), a family of models that use free-surface, hydrostatic, primitive equations over varying topography (Wang et al., 2016). Complex or complexity-aware DOM applications have been developed to reduce sea turtle bycatch in US Hawaiian fisheries (Howell et al., 2008, 2015), avoid sturgeon-fisheries interaction in the Atlantic (Breece et al., 2017), reduce bluefin tuna bycatch in Eastern Australia (Hobday et al., 2010, 2011), and limit megafauna bycatch in the US West Coast swordfish fishery (Scales et al., 2017; Hazen et al., 2018).
Spatially-Explicit Risk Assessment
In coastal fishing zones, resource managers, and planners have the challenging task of balancing ecological, conservation, socioeconomic, and cultural objectives. To protect marine megafauna while supporting fisheries, managers are often asked to map, measure, and monitor the relative and cumulative risks to megafauna, with fisheries bycatch as one of the primary risks. This is particularly challenging in developing countries where managers contend with the need to support local livelihoods, paucity of available data, incongruencies across spatial and temporal scales of available information and often meager monitoring budgets. Accurately characterizing risks to megafauna, and identifying opportunities to reduce bycatch, requires an approach that accounts for the complex relationship between humans and ocean systems. New methods to investigate cumulative impacts of human activities (Halpern et al., 2008; Worm et al., 2009) and drivers of ecosystem risk in marine systems (Patrick et al., 2010; Hobday et al., 2011; Williams et al., 2011) have led to the development of risk-based scenario assessment tools, a complexity-aware approach that uses existing data and knowledge to evaluate the direct effects of human activities, climate change, and other stressors on natural resource conservation and management.
Many applications of spatially-explicit risk assessment are driven and implemented by stakeholders, managers, and policy-makers needing a roadmap to understand the complexity of coastal and ocean systems, often in low capacity, data poor settings. Spatial risk assessment aims to synthesize and integrate primary data, literature reviews, expert opinion and other local knowledge in a transparent manner. Common examples of spatiotemporal risk assessment are bivariate analyses that include exposure of a habitat or species to a stressor and some metric for consequence and recovery potential, and the ability of a habitat or species to resist the stressor and recover following exposure. To account for uncertainty or missing data, these assessments can include variable weighting structure and data quality ratings, e.g., weighted averages, to acknowledge data limitations and account for uncertainty. Even in the face of data and capacity gaps, spatially-explicit risk assessments enable users to apply existing information to guide, inform, and identify appropriate survey methods, equipment and strata, establish baselines (Long et al., 2017), focus effort and resources in at-risk areas for the purpose of monitoring fisheries and marine megafauna, and evaluate alternative management scenarios that reduce risk to threatened populations (Henrichs et al., 2010).
Recent applications of tools for spatially-explicit risk assessment demonstrate the importance and utility of this complexity-aware approach in both data rich and data poor contexts (e.g., Guerry et al., 2012). Spatially-explicit risk assessments have been used to support conservation of habitats in coastal Belize (Arkema et al., 2014; Verutes et al., 2017), marine and terrestrial fauna in Washington state, USA (Samhouri and Levin, 2012; Duggan et al., 2017), freshwater lenses (aquifer) in The Bahamas (Holding and Allen, 2014), and dugongs (Dugong dugon) in Sabah, Malaysia (Briscoe et al., 2014). In Belize, the InVEST habitat risk assessment model (naturalcapitalproject.org) is a spatial risk assessment tool that was applied as part of a coastal zoning process where risk to habitats was used to alter inputs to ecological production functions in mechanistic complex, process-based models of spiny lobster catch and revenue, tourism visitation and expenditures, and natural protection provided by coastal habitats during storms (Arkema et al., 2015; Guannel et al., 2016).
Inspired by InVEST, a new spatial tool has been developed to evaluate fisheries bycatch risk and support marine megafauna conservation in developing countries, called ByRA (mmbycatchtoolbox.org). ByRA couples available information about the locations of megafauna with fishing effort categorized by gear type. ByRA outputs are spatially and temporally explicit, utilize existing data sources (e.g., Ponnampalam et al., 2014; Peter et al., 2016), community perspectives, and the human dimension of marine megafauna conservation (Hines et al., 2005; Teh et al., 2015). To date, the tool has been applied for the endangered Irrawaddy dolphins (Orcaella brevirostris) and dugongs (IUCN, 2017a,b) in Malaysia, Vietnam, and Thailand as the implementation of a new trade policy looms for these and other nations that currently export wild-caught seafood to the United States (Williams et al., 2016; Johnson et al., 2017).
Beyond Bycatch Ecology: Integrating Economics in Support of Complexity
As the spatially-explicit risk assessment and structured decision-making tools illustrate, the complexity of fisheries bycatch extends far beyond biological or ecological factors (Lotze et al., 2017). Bycatch of marine megafauna is also defined by their social, economic, and political contexts (Lewison et al., 2011; Bisack and Magnusson, 2016). Integrating the economic factors and dimensions of bycatch into ecology-focused studies illustrates one key example of supporting complexity-awareness, moving bycatch from a one-dimensional (ecological) to a two-dimensional domain (ecological-economic). While there has been some integration of ecological bycatch research and socioeconomic relevant factors, e.g., calculations of potential biological removal (PBR) are an obvious example of this (Lobo et al., 2010; Jin, 2012; Little et al., 2014; Abbott et al., 2015), socioeconomic considerations of bycatch are often overlooked in the marine megafauna conservation literature (Lent, 2015; Barnes et al., 2016; Alava et al., 2017; Lent and Squires, 2017; Lotze et al., 2017; van Beest et al., 2017).
Economic approaches to megafauna bycatch reductions are limited by data gaps, limited understanding of their effectiveness (Lent and Squires, 2017) and the difficulty of integrating economic valuation functions with ecological production functions (Tschirhart, 2011). One effort to integrate economics and endangered Stellar sea lion (Eumetopias jubatus) bycatch demonstrates the utility of integrated, complexity-aware approaches to bycatch (Finnoff and Tschirhart, 2008). Using economic and ecological dynamic general equilibrium models and applying economic principles such as rational behavior, efficiency, and equilibrium to ecosystem processes, integrated models were used to assess the impact of alternative quotas in a local pollock (Gadus chalcogrammus) fishery on eight bycatch species including otters (Enhydra lutris), killer whales (Orca orcinus), and Stellar sea lions. Related analyses also consider the effects of the pollock fishery on the non-consumptive use of these marine mammals (Finnoff and Tschirhart, 2003b). By embracing the linked economic-ecological complexity in fisheries bycatch, these integrated approaches demonstrate the ability of complexity-aware bycatch analyses to capture the key interactions and trade-offs between target catch and at-risk megafauna populations (Finnoff and Tschirhart, 2003a), and serve as a framework for how to also incorporate linked social or political factors that can strongly influence megafauna bycatch.
Complexity for Stakeholder Engagement, Consumer Awareness, and Policy
Embracing complexity is equally important outside the scientific community as a part of stakeholder engagement, education and awareness. Because stakeholder groups are the backbone of marine megafauna conservation and support for research (Fulton et al., 2015), stakeholder awareness of the interdependencies and inherent complexities of the megafauna conservation landscape is an essential ingredient to effective conservation (Prell et al., 2009). Although not specific to marine megafauna conservation, Q-methods, participatory mapping and collaborative learning methods are examples of approaches that have been used to help “unpack” complexity surrounding natural resource management and use (Davies et al., 2016; Hagan and Williams, 2016; Niedziałkowski et al., 2018). These methods can clarify and map viewpoints and perspectives of different stakeholder groups without bias, helping to articulate the potentially competing interests of the fishing industry and the marine megafauna conservation community (Prell et al., 2009; Forrester et al., 2015).
Complexity and complexity-awareness is also essential to consumer education and engagement particularly in the context of fisheries, and the co-creation of knowledge among stakeholder groups has been used to support complexity-aware approaches to education and outreach (Steyaert and Jiggins, 2007). Eco-labeling and certification standards, like SeaFood Watch (http://www.seafoodwatch.org/), RASS (http://www.seafish.org/rass/), or MSC (http://www.msc.org/) can help seafood consumers deal with complex market and supply chains and support effective megafauna conservation as well as reinforce corporate social responsibility commitments (Gutierrez and Thornton, 2014; Caveen et al., 2017; Lent and Squires, 2017). These complexity-aware education approaches allow consumers to move beyond a “not in my backyard” perspective to support meaningful megafauna conservation and sustainable fisheries across intricate, connected global market chains.
Understanding how complexity affects policies that govern bycatch will also be an important aspect to embracing complexity in marine megafauna conservation (Hirsch et al., 2010; Whitty, 2015). Policy approaches that explore policy pathways, such as the Pragmatic Enlightened Model (Edenhofer and Kowarsch, 2015), can help policy-makers understand what varying levels of complexity mean across the tradeoffs and practical consequences of each pathway. A better understanding and integration of complexity into a policy context will also help to strengthen policy development and implementation (Paul Cairney, 2017). Complexity-aware policies are ones that account for the diversity of resource stakeholders, and the reality of multiple resource uses across the seascape (Sayer et al., 2013). The conservation of marine megafauna, in particular, requires policy and governance structures that acknowledge the migratory nature of many megafauna species of conservation concern. Protection of important breeding or feeding grounds within one jurisdiction may prove to be necessary but not sufficient to conservation efforts if sensitive life stages move among unprotected waters, whether intra- or international. Policies that inherently recognize the complex relationships among ecological, social and economic systems and the influence these relationships can have on policy outcomes across jurisdictions can also ensure that well-intentioned megafauna conservation policies do not displace, and in some cases magnify, marine megafauna bycatch, or different risks in other jurisdictions (Lenzen et al., 2012; Lim et al., 2017).
Complexity and Uncertainty: a Tradeoff
Approaches that embrace complexity often improve the accuracy of how systems are represented and understood. Greater complexity is, however, also commonly associated with increased uncertainty that is borne from the addition of parameters which each have their own uncertainties associated with them (Fulton et al., 2003; Low-Décarie et al., 2014; Winkler, 2016). Conservation practitioners must therefore weigh the benefits of using simplified frameworks alongside more complex approaches. For all conservation applications and frameworks, there will be a fundamental and common challenge of how to balance system complexity while minimizing uncertainty (Collie et al., 2014). In fisheries science, attempts to strike this balance have led to the development of Models of Intermediate Complexity for Ecosystem assessments, or MICE (Plagányi et al., 2014). The MICE approach selects model complexity based on a specific problem statement and the data available, with temporal scales to match the questions being addressed (Essington and Plaganyi, 2013). The MICE approach for strategic complexity integration in fisheries research serves as a useful model for how to increase complexity while limiting sources of uncertainty in megafauna conservation and research efforts. Other fisheries-focused initiatives that attempt to recognize the inherently complex nature of ocean management include the concepts of Ecosystem Based Fisheries Management (EBFM) and Integrated Ecosystem Assessments (IEA), which aim to sustain healthy marine ecosystems and the fisheries they support by accounting for ecosystem complexity and the holistic impact of management decisions on those systems (Pickitch et al., 2004; Levin et al., 2009). Compared to more traditional, single species-based approaches to fisheries management, both EBFM and IEA represent how fisheries science has moved toward complexity and complexity-awareness (Marshak et al., 2017). However, the well-described shortcomings with the implementation of EBFM and IEA (Longhurst, 2006; Shelton, 2007; Borgström et al., 2015; Dolan et al., 2016) highlight the challenges that complex approaches in megafauna research and conservation will likely face in operationalizing and implementing complexity in a measurable and meaningful way.
Conclusion
Given the complex ecological, environmental, socioeconomic, and cultural dimensions that govern ocean systems, and thus megafauna conservation, the need for complex systems analyses or complexity-aware approaches will likely not come as a surprise to most readers of this special issue. While research and conservation methods that have approached megafauna conservation from a single element or domain perspective have yielded important insights and accomplishments, as the conservation status of many marine megafauna worsens (Davidson et al., 2012; Paleczny et al., 2015), there is a pressing need to embrace the complexity that governs marine megafauna and the systems in which they reside. Even in the face of limited data and uncertainties, adopting complex systems and complexity-aware approaches to resource management, marine spatial planning and resource and marine policy and education provides a more realistic lens for conservation and research efforts.
The need to view the conservation landscape as a complex system and calls for a “conceptual revolution” in how we approach marine megafauna conservation and research echo similar calls to infuse complexity into other conservation-related domains (Blaustein and Kiesecker, 2002; Parrott and Meyer, 2012; Lash-Marshall, 2013). By accepting that innovation and effective action in megafauna research and conservation means embracing complexity, and avoiding oversimplification sensu Stirling (2010), the marine megafauna research and conservation community is poised to focus on identifying levels of complexity that are needed to characterize and understand patterns of interest in time and space, and drive real world change. By embracing the inherent complexity of marine systems, conservation scientists and practitioners will be better equipped to provide actionable analyses, data, and information which can be used to protect and conserve megafauna.
Author Contributions
All authors listed have made a substantial, direct and intellectual contribution to the work, and approved it for publication.
Conflict of Interest Statement
The authors declare that the research was conducted in the absence of any commercial or financial relationships that could be construed as a potential conflict of interest.
Acknowledgments
RL was supported in part by NASA Earth Science Division/Applied Sciences Program's ROSES-2012 Ecological Forecasting grant (NNH12ZDA001N-COF). AJ was supported by National Science Foundation grant DEB-1632648 (2017/2018). Focus for this paper was conceived at the California Leatherback Day Celebration, Southwest Fisheries Science Center, October 2016.
References
Abbott, J. K., Haynie, A. C., and Reimer, M. N. (2015). Hidden flexibility: institutions, incentives, and the margins of selectivity in fishing. Land Econ. 91, 169–195. doi: 10.3368/le.91.1.169
Alava, J. J., Tatar, B., Barragán, M. J., Castro, C., Rosero, P., Denkinger, J., et al. (2017). Mitigating cetacean bycatch in coastal Ecuador_ Governance challenges for small-scale fisheries. Mar. Policy. doi: 10.1016/j.marpol.2017.05.025. [Epub ahead of print].
Anand, M., Gonzalez, A., Guichard, F., Kolasa, J., and Parrott, L. (2010). Ecological systems as complex systems: challenges for an emerging science. Diversity 2, 395–410. doi: 10.3390/d2030395
Anderson, C. N., Hsieh, C. H., Sandin, S. A., Hewitt, R., Hollowed, A., Beddington, J., et al. (2008). Why fishing magnifies fluctuations in fish abundance. Nature 452, 835–839. doi: 10.1038/nature06851
Anwar, S. M., Jeanneret, C. A., Parrott, L., and Marceau, D. J. (2007). Conceptualization and implementation of a multi-agent model to simulate whales-watching tours in the St. Lawrence Estuary in Quebec, Canada. Environ. Model. Softw. 22, 1775–1787. doi: 10.1016/j.envsoft.2007.02.007
Arkema, K. K., Verutes, G. M., Wood, S. A., Clarke-Samuels, C., Rosado, S., Canto, M., et al. (2015). Embedding ecosystem services in coastal planning leads to better outcomes for people and nature. Proc. Natl. Acad. Sci. U.S.A. 112, 7390–7395. doi: 10.1073/pnas.1406483112
Arkema, K. K., Verutes, G., Bernhardt, J. R., Clarke, C., Rosado, S., Canto, M., et al. (2014). Assessing habitat risk from human activities to inform coastal and marine spatial planning: a demonstration in Belize. Environ. Res. Lett. 9, 114016–114012. doi: 10.1088/1748-9326/9/11/114016
Arthur, W. B. (1999). Complexity and the economy. Science 284, 107–109. doi: 10.1126/science.284.5411.107
Authier, M., Spitz, J., Blanck, A., and Ridoux, V. (2017). Conservation science for marine megafauna in Europe - Historical perspectives and future directions. Deep Sea Res. Part II 141, 1–7. doi: 10.1016/j.dsr2.2017.05.002
Bailey, H., Shillinger, G., Palacios, D., Bograd, S., Spotila, J., Paladino, F., et al. (2008). Identifying and comparing phases of movement by leatherback turtles using state-space models. J. Exp. Mar. Biol. Ecol. 356, 128–135. doi: 10.1016/j.jembe.2007.12.020
Balint, P. J., Stewart, R. E., Desai, A., and Walters, L. (2011). Wicked Environmental Problems: Managing Uncertainty and Conflict. Washington, DC: Island Press.
Bankes, S. C. (2002). Tools and techniques for developing policies for complex and uncertain systems. Proc. Natl. Acad. Sci. U.S.A. 99, 7263–7266. doi: 10.1073/pnas.092081399
Barlow, J., and Cameron, G. A. (2003). Field experiments show that acoustic pingers reduce marine mammal bycatch in the California drift gill net fishery. Mar. Mamm. Sci. 19, 265–283. doi: 10.1111/j.1748-7692.2003.tb01108.x
Barnes, M. L., Lynham, J., Kalberg, K., and Leung, P. (2016). Social networks and environmental outcomes. Proc. Natl. Acad. Sci. U.S.A. 113, 6466–6471. doi: 10.1073/pnas.1523245113
Becker, E. A., Forney, K. A., Foley, D. G., Smith, R. C., Moore, T. J., and Barlow, J. (2014). Predicting seasonal density patterns of California cetaceans based on habitat models. Endang. Spec. Res. 23, 1–22. doi: 10.3354/esr00548
Becker, E., Forney, K., Fiedler, P., Barlow, J., Chivers, S., Edwards, C., et al. (2016). Moving towards dynamic ocean management: how well do modeled ocean products predict species distributions? Remote Sens. 8, 149–126. doi: 10.3390/rs8020149
Bertalanffy, L. V. (1968). General System Theory: Foundations, Development, Applications. New York, NY: George Braziller.
Beyer, H. L., Morales, J. M., Murray, D., and Fortin, M. J. (2013). The effectiveness of Bayesian state-space models for estimating behavioural states from movement paths. Methods Ecol. Evol. 4, 433–441. doi: 10.1111/2041-210X.12026
Bisack, K. D., and Magnusson, G. M. (2016). Measuring management success for protected species: looking beyond biological outcomes. Front. Mar. Sci. 3, 179–177. doi: 10.3389/fmars.2016.00061
Blaustein, A. R., and Kiesecker, J. M. (2002). Complexity in conservation: lessons from the global decline of amphibian populations. Ecol. Lett. 5, 597–608. doi: 10.1046/j.1461-0248.2002.00352.x
Block, B. A., Jonsen, I. D., Jorgensen, S. J., Winship, A. J., Shaffer, S. A., Bograd, S. J., et al. (2011). Tracking apex marine predator movements in a dynamic ocean. Nature 475:86. doi: 10.1038/nature10082
Borgström, S., Bodin, Ö., Sandström, A., and Crona, B. (2015). Developing an analytical framework for assessing progress toward ecosystem-based management. AMBIO 44, 357–369. doi: 10.1007/s13280-015-0655-7
Boyd, C., DeMaster, D. P., Waples, R. S., Ward, E. J., and Taylor, B. L. (2016). Consistent extinction risk ssessment under the U.S. Endangered Species Act. Conserv. Lett. 10, 328–336. doi: 10.1111/conl.12269
Bradshaw, C., Davis, L. S., Purvis, M., Zhou, Q. Q., and Benwell, G. L. (2002). Using artificial neural networks to model the suitability of coastline for breeding by New Zealand fur seals (Arctocephalus forsteri). Ecol. Model. 148, 111–131. doi: 10.1016/S0304-3800(01)00425-2
Breece, M. W., Dewayne, A. F., Haulsee, D. E., Wirgin, I. I., and Oliver, M. J. (2017). Satellite driven distribution models of endangered Atlantic sturgeon occurrence in the mid-Atlantic Bight. ICES J. Mar. Sci. 75, 1–10. doi: 10.1093/icesjms/fsx187
Briscoe, D. K., Hiatt, S., Lewison, R., and Hines, E. (2014). Modeling habitat and bycatch risk for dugongs in Sabah, Malaysia. Endang. Spec. Res. 24, 237–247. doi: 10.3354/esr00600
Brodie, S., Jacox, M. G., Bograd, S. J., Welch, H., Dewar, H., Scales, K. L., et al. (2018). Integrating dynamic subsurface habitat metrics into species distribution models. Front. Mar. Sci. 5:219. doi: 10.3389/fmars.2018.00219
Carretta, J. V., and Barlow, J. (2011). Long-term effectiveness, failure rates, and “dinner bell” properties of acoustic pingers in a gillnet fishery. Mar. Technol. Soc. J. 45, 7–19. doi: 10.4031/MTSJ.45.5.3
Caveen, A. J., Lart, W., Duggan, H., and Pickerell, T. (2017). The risk assessment for sourcing seafood (RASS)_ empowering businesses to buy responsibly. Mar. Policy 75, 1–10. doi: 10.1016/j.marpol.2016.10.005
Chilvers, B. L. (2008). New Zealand sea lions Phocarctos hookeri and squid trawl fisheries: bycatch problems and management options. Endang. Spec. Res. 5, 193–204. doi: 10.3354/esr00086
Clapham, P. J. (2015). Japan's whaling following the International Court of Justice ruling_ Brave New World – Or business as usual? Mar. Policy 51, 238–241. doi: 10.1016/j.marpol.2014.08.011
Collie, J. S., Botsford, L. W., Hastings, A., Kaplan, I. C., Largier, J. L., Livingston, P. A., et al. (2014). Ecosystem models for fisheries management: finding the sweet spot. Fish Fish. 17, 101–125. doi: 10.1111/faf.12093
Crowder, L. B., Crouse, D. T., Heppell, S. S., and Martin, T. H. (1994). Predicting the impact of turtle excluder devices on loggerhead sea-turtle populations. Ecol. Appl. 4, 437–445. doi: 10.2307/1941948
Crowder, L., and Norse, E. (2008). Essential ecological insights for marine ecosystem-based management and marine spatial planning. Mar. Policy 32, 772–778. doi: 10.1016/j.marpol.2008.03.012
Davidson, A. D., Boyer, A. G., Kim, H., Pompa-Mansilla, S., Hamilton, M. J., Costa, D. P., et al. (2012). Drivers and hotspots of extinction risk in marine mammals. Proc. Natl. Acad. Sci. U.S.A. 109, 3395–3400. doi: 10.1073/pnas.1121469109
Davies, R. W. D., Cripps, S. J., Nickson, A., and Porter, G. (2009). Defining and estimating global marine fisheries bycatch. Mar. Policy 33, 661–672. doi: 10.1016/j.marpol.2009.01.003
Davies, W., Van Alstine, J., and Lovett, J. C. (2016). “Frame Conflicts” in natural resource use: exploring framings around arctic offshore petroleum using Q-methodology. Environ. Policy Govt. 26, 482–497. doi: 10.1002/eet.1729
Dawson, S. M., Read, A., and Slooten, E. (1998). Pingers, porpoises and power: uncertainties with using pingers to reduce bycatch of small cetaceans. Biol. Conserv. 84, 141–146. doi: 10.1016/S0006-3207(97)00127-4
Dean, B., Freeman, R., Kirk, H., Leonard, K., Phillips, R. A., Perrins, C. M., et al. (2013). Behavioural mapping of a pelagic seabird: combining multiple sensors and a hidden Markov model reveals the distribution of at-sea behaviour. J. R. Soc. Interface 10. doi: 10.1098/rsif.2012.0570. Available online at: http://rsif.royalsocietypublishing.org/content/early/2012/10/03/rsif.2012.0570.short
Dolan, T. E., Patrick, W. S., and Link, J. S. (2016). Delineating the continuum of marine ecosystem-based management: a US fisheries reference point perspective. ICES J. Mar. Sci. 73, 1042–1050. doi: 10.1093/icesjms/fsv242
Duggan, J. M., Eichelberger, B. A., Ma, S., Lawler, J. J., and Ziv, G. (2017). Informing management of rare species with an approach combining scenario modeling and spatially explicit risk assessment. Ecosyst. Health Sustain. 1, 1–18. doi: 10.1890/EHS14-0009.1
Dunn, D. C., Maxwell, S. M., Boustany, A. M., and Halpin, P. N. (2016). Dynamic ocean management increases the efficiency and efficacy of fisheries management. Proc. Natl. Acad. Sci. U.S.A. 113, 668–673. doi: 10.1073/pnas.1513626113
Edenhofer, O., and Kowarsch, M. (2015). Cartography of pathways: a new model for environmental policy assessments. Environ. Sci. Policy 51, 56–64. doi: 10.1016/j.envsci.2015.03.017
Elith, J., and Leathwick, J. R. (2009). Species distribution models: ecological explanation and prediction across space and time. Annu. Rev. Ecol. Evol. Syst. 40, 677–697. doi: 10.1146/annurev.ecolsys.110308.120159
Essington, T. E., and Plaganyi, E. E. (2013). Pitfalls and guidelines for “recycling” models for ecosystem-based fisheries management: evaluating model suitability for forage fish fisheries. ICES J. Mar. Sci. 71, 118–127. doi: 10.1093/icesjms/fst047
Evans, M. C., Davila, F., Toomey, A., and Wyborn, C. (2017). Embrace complexity to improve conservation decision making. Nat. Ecol. Evol. 1:1588. doi: 10.1038/s41559-017-0345-x
Finnoff, D., and Tschirhart, J. (2003a). Harvesting in an eight-species ecosystem. J. Environ. Econ. Manage. 45, 589–611. doi: 10.1016/S0095-0696(02)00025-6
Finnoff, D., and Tschirhart, J. (2003b). Protecting an endangered species while harvesting its prey in a general equilibrium ecosystem model. Land Econ. 79, 160–180. doi: 10.2307/3146865
Finnoff, D., and Tschirhart, J. (2008). Linking dynamic economic and ecological general equilibrium models. Resour. Energy Econ. 30, 91–114. doi: 10.1016/j.reseneeco.2007.08.005
Fisher, S. (2016). Japanese small type coastal whaling. Front. Mar. Sci. 3, 1191–1196. doi: 10.3389/fmars.2016.00121
Forrester, J., Cook, B., Bracken, L., Cinderby, S., and Donaldson, A. (2015). Combining participatory mapping with Q-methodology to map stakeholder perceptions of complex environmental problems. Appl. Geogr. 56, 199–208. doi: 10.1016/j.apgeog.2014.11.019
Fox, C. H., Huettmann, F. H., Harvey, G., Morgan, K. H., Robinson, J., Williams, R., et al. (2017). Predictions from machine learning ensembles: marine bird distribution and density on Canada's Pacific coast. Mar. Ecol. Prog. Ser. 566, 199–216. doi: 10.3354/meps12030
Fulton, E. A., Boschetti, F., Sporcic, M., Jones, T., Little, L. R., Dambacher, J. M., et al. (2015). A multi-model approach to engaging stakeholder and modellers in complex environmental problems. Environ. Sci. Policy 48, 44–56. doi: 10.1016/j.envsci.2014.12.006
Fulton, E. A., Smith, A., and Johnson, C. R. (2003). Effect of complexity on marine ecosystem models. Mar. Ecol. Prog. Ser. 253, 1–16. doi: 10.3354/meps253001
Gilman, E., Brothers, N., and Kobayashi, D. R. (2005). Principles and approaches to abate seabird bycatch in longline fisheries. Fish Fish. 6, 35–49. doi: 10.1111/j.1467-2679.2005.00175.x
Gilman, E., Kobayashi, D., Swenarton, T., Brothers, N., Dalzell, P., and Kinankelly, I. (2007). Reducing sea turtle interactions in the Hawaii-based longline swordfish fishery. Biol. Conserv. 139, 19–28. doi: 10.1016/j.biocon.2007.06.002
Glaser, S. M., Ye, H., and Sugihara, G. (2013). A nonlinear, low data requirement model for producing spatially explicit fishery forecasts. Fish. Oceanogr. 23, 45–53. doi: 10.1111/fog.12042
Green, J. L., Hastings, A., Arzberger, P., Ayala, F. J., Cottingham, K. L., and Cuddington, K. (2005). Complexity in ecology and conservation: mathematical, statistical, and computational challenges. Bioscience 55, 501–510. doi: 10.1641/0006-3568(2005)055[0501:CIEACM]2.0.CO;2
Guannel, G., Arkema, K., Ruggiero, P., and Verutes, G. (2016). The power of three: coral reefs, seagrasses and mangroves protect coastal regions and increase their resilience. PLoS ONE 11:e0158094. doi: 10.1371/journal.pone.0158094
Guerry, A. D., Ruckelshaus, M. H., Arkema, K. K., Bernhardt, J. R., Guannel, G., Kim, C. K., et al. (2012). Modeling benefits from nature: using ecosystem services to inform coastal and marine spatial planning. Int. J. Biodivers. Sci. Ecosyst. Serv. Manag. 8, 107–121. doi: 10.1080/21513732.2011.647835
Guilford, T., Meade, J., Willis, J., Phillips, R. A., Boyle, D., Roberts, S., et al. (2009). Migration and stopover in a small pelagic seabird, the Manx shearwater Puffinus puffinus: insights from machine learning. Proc. R. Soc. B: Biol. Sci. 276, 1215–1223. doi: 10.1098/rspb.2008.1577.
Gutierrez, A., and Thornton, T. (2014). Can consumers understand sustainability through seafood eco-labels? A U.S. and UK Case Study. Sustainability 6, 8195–8217. doi: 10.3390/su6118195
Hagan, K., and Williams, S. (2016). Oceans of discourses: utilizing Q methodology for analyzing perceptions on marine biodiversity conservation in the kogelberg biosphere reserve, South Africa. Front. Mar. Sci. 3:188. doi: 10.3389/fmars.2016.00188
Halpern, B. S., Walbridge, S., Selkoe, K. A., and Kappel, C. V. (2008). A global map of human impact on marine ecosystems. Science 319, 948–952. doi: 10.1126/science.1149345
Hamer, D. J., Goldsworthy, S. D., Costa, S. L., Fowler, B., and Sumner, M. D. (2013). The endangered Australian sea lion extensively overlaps with and regularly becomes by-catch in demersal shark gill-nets in South Australian shelf waters. Biol. Conserv. 157, 386–400. doi: 10.1016/j.biocon.2012.07.010
Hazen, E. L., Jorgensen, S., Rykaczewski, R. R., Bograd, S. J., Foley, D. G., Jonsen, I. D., et al. (2013). Predicted habitat shifts of Pacific top predators in a changing climate. Nat. Clim. Change 3, 234–238. doi: 10.1038/nclimate1686
Hazen, E. L., Scales, K. L., Maxwell, S. M., Briscoe, D. K., Benson, S. R., Eguchi, T., et al. (2018). A dynamic ocean management tool to reduce bycatch and support sustainable fisheries. Sci. Adv. 4:eaar3001. doi: 10.1126/sciadv.aar3001
Henrichs, T., Zurek, M., Eickhout, B., Kok, K., Raudsepp-Hearne, C., Ribeiro, T., et al. (2010). Ecosystems and Human Well-Being: A Manual for Assessment practitioners. Washington, DC: Island Press.
Hines, E., Adulyanukosol, K., Duffus, D., and Dearden, P. (2005). Community perspectives and conservation needs for dugongs (dugong dugon) along the Andaman coast of Thailand. Environ. Manage. 36, 654–664. doi: 10.1007/s00267-003-0287-8
Hirsch, P. D., Adams, W. M., Brosius, J. P., Zia, A., Bariola, N., and Dammert, J. L. (2010). Acknowledging conservation trade-offs and embracing complexity. Conserv. Biol. 25, 259–264. doi: 10.1111/j.1523-1739.2010.01608.x
Hobday, A. J., and Hartog, J. R. (2014). Derived ocean features for dynamic ocean management. Oceanography 27, 134–145. doi: 10.5670/oceanog.2014.92
Hobday, A. J., Hartog, J. R., Timmiss, T., and Fielding, J. (2010). Dynamic spatial zoning to manage southern bluefin tuna (Thunnus maccoyii) capture in a multi-species longline fishery. Fish. Oceanogr. 19, 243–253. doi: 10.1111/j.1365-2419.2010.00540.x
Hobday, A. J., Maxwell, S. M., Forgie, J., McDonald, J., Darby, M., Poulos, G., et al. (2014). Dynamic ocean management: integrating scientific and technological capacity with law, policy, and management. Stanford Environ. Law J. 33, 125–165. Available online at: https://www.scopus.com/record/display.uri?eid=2-s2.0-84908378600&origin=inward&txGid=892d96155ab118815d6ee56c41e171ab
Hobday, A. J., Smith, A. D. M., Stobutzki, I. C., Bulman, C., Daley, R., Dambacher, J. M., et al. (2011). Ecological risk assessment for the effects of fishing. Fish. Res. 108, 372–384. doi: 10.1016/j.fishres.2011.01.013
Hofman, R. J. (2016). Sealing, whaling and krill fishing in the Southern Ocean: past and possible future effects on catch regulations. Polar Rec. 53, 88–99. doi: 10.1017/S0032247416000644
Holding, S., and Allen, D. M. (2014). From days to decades: numerical modeling of freshwater lens response to climate change stressors on small islands. Hydrol. Earth Syst. Sci. Discuss. 11, 11439–11487. doi: 10.5194/hessd-11-11439-2014
Howarth, L. M., Roberts, C. M., Thurstan, R. H., and Stewart, B. D. (2013). The unintended consequences of simplifying the sea: making the case for complexity. Fish Fish. 15, 690–711. doi: 10.1111/faf.12041
Howell, E. A., Hoover, A., Benson, S. R., Bailey, H., Polovina, J. J., Seminoff, J. A., et al. (2015). Enhancing the TurtleWatch product for leatherback sea turtles, a dynamic habitat model for ecosystem-based management. Fish. Oceanogr. 24, 57–68. doi: 10.1111/fog.12092
Howell, E. A., Kobayashi, D. R., Parker, D. M., Balazs, G. H., and Polovina, A. (2008). TurtleWatch: a tool to aid in the bycatch reduction of loggerhead turtles Caretta caretta in the Hawaii-based pelagic longline fishery. Endang. Spec. Res. 5, 267–278. doi: 10.3354/esr00096
IUCN (2017b). Orcaella brevirostris: Minton, G., Smith, B.D., Braulik, G.T., Kreb, D., Sutaria, D. and Reeves, R.
Jentoft, S., and Chuenpagdee, R. (2009). Fisheries and coastal governance as a wicked problem. Mar. Policy 33, 553–560. doi: 10.1016/j.marpol.2008.12.002
Jin, D. (2012). Aquaculture and capture fisheries: a conceptual approach toward an integrated economic-ecological analysis. Aquacult. Econ. Manag. 16, 167–181. doi: 10.1080/13657305.2012.678551
Johnson, A. F., Caillat, M., Verutes, G. M., Peter, C., Junchompoo, C., Long, V., et al. (2017). Poor fisheries struggle with U.S. import rule. Science 355, 1031–1032. doi: 10.1126/science.aam9153
Johnson, A.F., and Lidström, S. (2018). The balance between concepts and complexity in ecology. Nat. Ecol. Evol. 2, 585–587.
Jonsen, I. D., Myers, R. A., and James, M. C. (2007). Identifying leatherback turtle foraging behaviour from satellite telemetry using a switching state-space model. Mar. Ecol. Prog. Ser. 337, 255–264. doi: 10.3354/meps337255
Kerstetter, D. W., and Graves, J. E. (2006). Effects of circle versus J-style hooks on target and non-target species in a pelagic longline fishery. Fish. Res. 80, 239–250. doi: 10.1016/j.fishres.2006.03.032
Knowlton, N. (2004). Multiple “stable” states and the conservation of marine ecosystems. Prog. Oceanogr. 60, 387–396. doi: 10.1016/j.pocean.2004.02.011
Kraus, S. D., Brown, M. W., Caswell, H., Clark, C. W., Fujiwara, M., Hamilton, P. K., et al. (2005). North Atlantic right whales in crisis. Science 309, 561–562. doi: 10.1126/science.1111200
Larsen, F., and Eigaard, O. R. (2014). Acoustic alarms reduce bycatch of harbour porpoises in Danish North Sea gillnet fisheries. Fish. Res. 153, 108–112. doi: 10.1016/j.fishres.2014.01.010
Lash-Marshall, W. G. (2013). Embracing Complexity in Conservation Theory and Practice. State University of New York.
Law, R. J. (2014). An overview of time trends in organic contaminant concentrations in marine mammals: going up or down? Mar. Pollut. Bull. 82, 7–10. doi: 10.1016/j.marpolbul.2014.03.024
Lent, R. J. (2015). Conservation benefits of an interdisciplinary approach to marine mammal science. Front. Mar. Sci. 2:67. doi: 10.3389/fmars.2015.00067
Lent, R., and Squires, D. (2017). Reducing marine mammal bycatch in global fisheries_ an economics approach. Deep Sea Res. Part II 140, 268–277. doi: 10.1016/j.dsr2.2017.03.005
Lenzen, M., Moran, D., Kanemoto, K., Foran, B., Lobefaro, L., and Geschke, A. (2012). International trade drives biodiversity threats in developing nations. Nature 486, 109–112. doi: 10.1038/nature11145
Levin, P. S., Fogarty, M. J., Murawski, S. A., and Fluharty, D. (2009). Integrated ecosystem assessments: developing the scientific basis for ecosystem-based management of the ocean. PLoS Biol. 7:e14 doi: 10.1371/journal.pbio.1000014
Levin, S. A., Grenfell, B., Hastings, A., and Perelson, A. S. (1997). Mathematical and computational challenges in population biology and ecosystems science. Science 275, 334–343. doi: 10.1126/science.275.5298.334
Levin, S., Xepapadeas, T., Crépin, A. S., Norberg, J., Zeeuw, A. D., Folke, C., et al. (2012). Social-ecological systems as complex adaptive systems: modeling and policy implications. Environ. Dev. Econ. 18, 111–132. doi: 10.1017/S1355770X12000460
Lewison, R. L., Crowder, L. B., and Shaver, D. J. (2003). The impact of turtle excluder devices and fisheries closures on loggerhead and Kemp's ridley strandings in the western Gulf of Mexico. Conserv. Biol. 17, 1089–1097. doi: 10.1046/j.1523-1739.2003.02057.x
Lewison, R. L., Crowder, L. B., Wallace, B. P., Moore, J. E., Cox, T., Zydelis, R., et al. (2014). Global patterns of marine mammal, seabird, and sea turtle bycatch reveal taxa-specific and cumulative megafauna hotspots. Proc. Natl. Acad. Sci. U.S.A. 111, 5271–5276. doi: 10.1073/pnas.1318960111
Lewison, R. L., Freeman, S. A., and Crowder, L. B. (2004). Quantifying the effects of fisheries on threatened species: the impact of pelagic longlines on loggerhead and leatherback sea turtles. Ecol. Lett. 7, 221–231. doi: 10.1111/j.1461-0248.2004.00573.x
Lewison, R. L., Hobday, A. J., Maxwell, S., Hazen, E., Hartog, J. R., Dunn, D. C., et al. (2015). Dynamic ocean management: identifying the critical ingredients of dynamic approaches to ocean resource management. Bioscience 65, 486–498. doi: 10.1093/biosci/biv018
Lewison, R. L., Soykan, C. U., Cox, T., Peckham, H., Pilcher, N., LeBoeuf, N., et al. (2011). Ingredients for addressing the challenges of fisheries bycatch. Bull. Mar. 87, 235–250. doi: 10.5343/bms.2010.1062
Lim, F. K. S., Carrasco, L. R., McHardy, J., and Edwards, D. P. (2017). Perverse market outcomes from biodiversity conservation interventions. Conserv. Lett. 10, 506–516. doi: 10.1111/conl.12332
Little, A. S., Needle, C. L., Hilborn, R., Holland, D. S., and Marshall, C. T. (2014). Real-time spatial management approaches to reduce bycatch and discards: experiences from Europe and the United States. Fish Fish. 16, 576–602. doi: 10.1111/faf.12080
Lobo, A. S., Balmford, A., Arthur, R., and Manica, A. (2010). Commercializing bycatch can push a fishery beyond economic extinction. Conserv. Lett. 3, 277–285. doi: 10.1111/j.1755-263X.2010.00117.x
Long, V., Tho, T. A., Hung, N. N., and Duy, L. (2017). Conservation of cetaceans in Kien Giang Biosphere Reserve, Vietnam. Conservation Leadership Program: 03234115 Available oline at: http://www.conservationleadershipprogramme.org/media/2017/12/03234115-Conservation-of-Cetacean-in-Kien-Giang-Biosphere-Reserve-Vietnam.pdf
Longhurst, A. (2006). The sustainability myth. Fish. Res. 81, 107–112 doi: 10.1016/j.fishres.2006.06.022
Lotze, H. K., Flemming, J. M., and Magera, A. M. (2017). Critical factors for the recovery of marine mammals. Conserv. Biol. 31, 1301–1311. doi: 10.1111/cobi.12957
Low-Décarie, E., Chivers, C., and Granados, M. (2014). Rising complexity and falling explanatory power in ecology. Front. Ecol. Environ. 12, 412–418. doi: 10.1890/130230
Mahon, R., McConney, P., and Roy, R. N. (2008). Governing fisheries as complex adaptive systems. Mar. Policy 32, 104–112. doi: 10.1016/j.marpol.2007.04.011
Mangel, J. C., Alfaro-Shigueto, J., Witt, M. J., Hodgson, D. J., and Godley, B. J. (2013). Using pingers to reduce bycatch of small cetaceans in Peru's small-scale driftnet fishery. Oryx 47, 595–606. doi: 10.1017/S0030605312000658
Manson, S. M. (2001). Simplifying complexity: a review of complexity theory. Geoforum 32, 405–414. doi: 10.1016/S0016-7185(00)00035-X
Maree, B. A., Wanless, R. M., Fairweather, T. P., Sullivan, B. J., and Yates, O. (2014). Significant reductions in mortality of threatened seabirds in a South African trawl fishery. Anim. Conserv. 17, 520–529. doi: 10.1111/acv.12126
Marshak, A. R., Link, J. S., Shuford, R., Mocano, M. E., Johannesen, E., Bianchi, G., et al. (2017). International perceptions of an integrated, multi-sectoral, ecosystem approach to management. ICES J. Mar. Sci. 74, 414–420. doi: 10.1093/icesjms/fsw214
Maxwell, S. M., Hazen, E. L., Lewison, R. L., Dunn, D. C., Bailey, H., Bograd, S. J., et al. (2015). Dynamic ocean management_ defining and conceptualizing real-time management of the ocean. Mar. Policy 58, 42–50. doi: 10.1016/j.marpol.2015.03.014
Maxwell, S. M., Hazen, E. L., Morgan, L. E., Bailey, H., and Lewison, R. (2012). Finding balance in fisheries management. Science 336, 413–413. doi: 10.1126/science.336.6080.413-a
McGowan, J., Hines, E., Elliott, M., Howar, J., Dransfield, A., Nur, N., et al. (2013). Using seabird habitat modeling to inform marine spatial planning in central California's National Marine Sanctuaries. PLoS ONE 8:e71406. doi: 10.1371/journal.pone.0071406
McHuron, E. A., Costa, D. P., Schwarz, L., and Mangel, M. (2017). State-dependent behavioural theory for assessing the fitness consequences of anthropogenic disturbance on capital and income breeders. Methods Ecol. Evol. 8, 552–560. doi: 10.1111/2041-210X.12701
Melvin, E. F., Parrish, J. K., and Conquest, L. L. (1999). Novel tools to reduce seabird bycatch in coastal gillnet fisheries. Conserv. Biol. 13, 1386–1397. doi: 10.1046/j.1523-1739.1999.98426.x
Michener, W. K., and Jones, M. B. (2012). Ecoinformatics: supporting ecology as a data-intensive science. Trends Ecol. Evol. 27, 85–93. doi: 10.1016/j.tree.2011.11.016
Moore, J. E., and Barlow, J. (2011). Bayesian state space model of fin whale abundance trends from a 1991-2008 time series of line transect surveys in the California Current. J. Appl. Ecol. 48, 1195–1205. doi: 10.1111/j.1365-2664.2011.02018.x
Morin, P. A., Scott Baker, C., Brewer, R. S., Burdin, A. M., Dalebout, M. L., Dines, J. P., et al. (2016). Genetic structure of the beaked whale genus Berardiusin the North Pacific, with genetic evidence for a new species. Mar. Mamm. Sci. 33, 96–111. doi: 10.1111/mms.12345
Mukherjee, D., and van der Schaar, M. (2005). “Compact dependent key generation methods for encryption-based subscription differentiation for scalable bit-streams” in IEEE (Genova), II–1114.
National Academy of Sciences, Engineering and Medicine. (2017). Approaches to Understanding the Cumulative Effects of Stressors on Marine Mammals. Washington, DC:The National Academies Press.
National Research Council (2012). Assessing the Reliability of Complex Models: Mathematical and Statistical Foundations of Verification, Validation, and Uncertainty Quantification. National Academies of sciences, engineering and medicine.
Niedziałkowski, K., Komar, E., Pietrzyk-Kaszynska, A., Olszanska, A., and Grodzinska-Jurczak, M. (2018). Discourses on public participation in protected areas governance - application of Q methodology in Poland. Ecol. Econ. 145, 401–409. doi: 10.1016/j.ecolecon.2017.11.018
Ortiz, N., Mangel, J. C., Wang, J., Alfaro-Shigueto, J., Pingo, S., Jimenez, A., et al. (2016). Reducing green turtle bycatch in small-scale fisheries using illuminated gillnets: the cost of saving a sea turtle. Mar. Ecol. Prog. Ser. 545, 251–259. doi: 10.3354/meps11610
Paleczny, M., Hammill, E., Karpouzi, V., and Pauly, D. (2015). Population trend of the world's monitored seabirds, 1950–2010. PLoS ONE 10:e0129342. doi: 10.1371/journal.pone.0129342
Paludan, M. B. (2015). Monitoring of Advocacy - When the Change Process is Complex. Available online at: http://www.globaltfokus.dk/images/Pulje/Arkiv/Fagligt_Fokus/Complexity_aware_approach_to_monitoring_advocacy.pdf
Panigada, S., Pesante, G., Zanardelli, M., Capoulade, F., Gannier, A., and Weinrich, M. T. (2006). Mediterranean fin whales at risk from fatal ship strikes. Mar. Pollut. Bull. 52, 1287–1298. doi: 10.1016/j.marpolbul.2006.03.014
Parrott, L., and Meyer, W. S. (2012). Future landscapes: managing within complexity. Front. Ecol. Environ. 10, 382–389. doi: 10.1890/110082
Patrick, W. S., Spencer, P., LinK, J., Cope, J., and Fishery, J. F. (2010). Using productivity and susceptibility indices to assess the vulnerability of United States fish stocks to overfishing. Fish. Bull. 108, 305–322. Available online at: http://aquaticcommons.org/id/eprint/8747
Paul Cairney, R. G. (2017). A critical discussion of complexity theory: how does “complexity thinking” improve our understanding of politics and policymaking? Complex. Govern. Netw. 3, 1–11. doi: 10.20377/cgn-56
Peckham, S. H., Lucero-Romero, J., Maldonado-Díaz, D., Rodríguez-Sánchez, A., Senko, J., Wojakowski, M., et al. (2016). Buoyless nets reduce sea turtle bycatch in coastal net fisheries. Conserv. Lett. 9, 114–121. doi: 10.1111/conl.12176
Peter, C., Zulkifli, A. N., Ngeian, J., Tuen, A. A., and Minton, G. (2016). Identifying Habitat Characteristics and Critical Areas for Irrawaddy Dolphin, Orcaella brevirostris: Implications for Conservation, eds. Das, I., and A. A. Tuen.
Piacenza, S. E., Richards, P. M., and Heppell, S. S. (2017). An agent-based model to evaluate recovery times and monitoring strategies to increase accuracy of sea turtle population assessments. Ecol. Modell. 358, 25–39. doi: 10.1016/j.ecolmodel.2017.05.013
Pickitch, E. K., Santora, C., Babcock, E. A., Bakun, A., Bonfil, R., Conover, D. O., et al. (2004). Ecosystem-based fishery management. Science 305, 346–347. doi: 10.1126/science.1098222
Pirotta, E., Mangel, M., Costa, D. P., Mate, B., Goldbogen, J. A., Palacios, D. M., et al. (2018). A dynamic state model of migratory behavior and physiology to assess the consequences of environmental variation and anthropogenic disturbance on marine vertebrates. Am. Nat. 191, E40–E56. doi: 10.1086/695135
Plagányi, É. E., Punt, A. E., Hillary, R., Morello, E. B., Thébaud, O., Hutton, T., et al. (2014). Multispecies fisheries management and conservation: tactical applications using models of intermediate complexity. Fish Fish. 15, 1–22. doi: 10.1111/j.1467-2979.2012.00488.x
Ponnampalam, L. S., Fairul Izmal, J. H., Adulyanukosol, K., Ooi, J. L. S., and Reynolds, J. E. (2014). Aligning conservation and research priorities for proactive species and habitat management: the case of dugongs Dugong dugon in Johor, Malaysia. Oryx 49, 743–749. doi: 10.1017/S0030605313001580
Pott, C., and Wiedenfeld, D. A. (2017). Information gaps limit our understanding of seabird bycatch in global fisheries. Biol. Conserv. 210, 192–204. doi: 10.1016/j.biocon.2017.04.002
Prell, C., Hubacek, K., and Reed, M. (2009). Stakeholder analysis and social network analysis in natural resource management. Soc. Nat. Resour. 22, 501–518. doi: 10.1080/08941920802199202
Rodríguez, J. P., Fernández-Gracia, J., Thums, M., Hindell, M. A., Sequeira, A. M., Meekan, M.G., et al. (2017). Big data analyses reveal patterns and drivers of the movements of southern elephant seals. Sci. Rep. 7:112. doi: 10.1038/s41598-017-00165-0
Samhouri, J. F., and Levin, P. S. (2012). Linking land- and sea-based activities to risk in coastal ecosystems. Biol. Conserv. 145, 118–129. doi: 10.1016/j.biocon.2011.10.021
Sayer, J., Sunderland, T., Ghazoul, J., Pfund, J. L., Sheilb, D., Meijaard, E., et al. (2013). Ten principles for a landscape approach to reconciling agriculture, conservation, and other competing land uses. Proc. Natl. Acad. Sci. U.S.A.110, 8349–8356. doi: 10.1073/pnas.1210595110
Scales, K. L., Hazen, E. L., Maxwell, S. M., Dewar, H., Kohin, S., Jacox, M. G., et al. (2017). Fit to predict? Eco-informatics for predicting the catchability of a pelagic fish in near real time. Ecol. Appl. 27, 2313–2329. doi: 10.1002/eap.1610
Shalizi, C. R. (2006). “Methods and techniques of complex systems science: an overview,” in Complex Systems Science in Biomedicine, eds T. S. Deisboeck and J. Y. Kresh (New York, NY: Springer), 33–114.
Shelton, P. A. (2007). The weakening role of science in the management of groundfish off the east coast of Canada. ICES J. Mar. Sci. 64, 723–729. doi: 10.1093/icesjms/fsm008
Steyaert, P., and Jiggins, J. (2007). Governance of complex environmental situations through social learning: a synthesis of SLIM's lessons for research, policy and practice. Environ. Sci. Policy 10, 575–586. doi: 10.1016/j.envsci.2007.01.011
Taylor, B. L., Rojas-Bracho, L., Moore, J., Jaramillo-Legorreta, A., Ver Hoef, J. M., Cardenas-Hinojosa, G., et al. (2016). Extinction is imminent for Mexico's endemic porpoise unless fishery bycatch is eliminated. Conserv. Lett. 10, 588–595. doi: 10.1111/conl.12331
Taylor, B. L., Wade, P. R., De Master, D. P., and Barlow, J. (2000). Incorporating uncertainty into management models for marine mammals. Conserv. Biol. 14, 1243–1252. doi: 10.1046/j.1523-1739.2000.99409.x
Teh, L. S., Teh, L. C., Hines, E., Junchompoo, C., and Lewison, R. L. (2015). Contextualising the coupled socio-ecological conditions of marine megafauna bycatch. Ocean Coastal Manage. 116, 449–465. doi: 10.1016/j.ocecoaman.2015.08.019
Tschirhart, J. (2011). Biology as a source of non-convexities in ecological production functions. Environ. Resour. Econ. 51, 189–213. doi: 10.1007/s10640-011-9494-6
US AID (2016). Complexity-Aware Monitoring. Available online at: https://usaidlearninglab.org/sites/default/files/resource/files/201sad_complexity_aware_monitoring_discussion_note.pdf
van Beest, F. M., Kindt-Larsen, L., Bastardie, F., Bartolino, V., and Nabe-Nielsen, J. (2017). Predicting the population-level impact of mitigating harbor porpoise bycatch with pingers and time-area fishing closures. Ecosphere 8, e01785–e01717. doi: 10.1002/ecs2.1785
Verutes, G. M., Arkema, K. K., Clarke-Samuels, C., Wood, S. A., Rosenthal, A., Rosado, S., et al. (2017). Integrated planning that safeguards ecosystems and balances multiple objectives in coastal Belize. Int. J. Biodiver. Sci. Ecosyst. Serv. Manage. 13, 1–17. doi: 10.1080/21513732.2017.1345979
Virgili, M., Vasapollo, C., and Lucchetti, A. (2017). Can ultraviolet illumination reduce sea turtle bycatch in Mediterranean set net fisheries? Fish. Res. 199, 1–7. doi: 10.1016/j.fishres.2017.11.012
Wang, P., Song, Y. T., Chao, Y., and Zhang, H. (2016). Parallel computation of the regional ocean modeling system. Int. J. High Perform. Comput. Appl. 19, 375–385. doi: 10.1177/1094342005059115
Warren, K., Franklin, C., and Streeter, C. L. (1998). New directions in systems theory: chaos and complexity. Soc. Work 43, 357–372. doi: 10.1093/sw/43.4.357
Watson, J. W., Epperly, S. P., Shah, A. K., and Foster, D. G. (2005). Fishing methods to reduce sea turtle mortality associated with pelagic longlines. Canadian J. Fish. Aquat. Sci. 62, 965–981. doi: 10.1139/f05-004
Weimerskirch, H., Brothers, N., and Jouvntin, P. (1987). Population-dynamics of the wandering albatross, diomedea-exulans, of the crozet islands - causes and consequences of the population decline. Oikos 49, 315–322. doi: 10.2307/3565767
Weir, C. R., Waerebeek, K. V., Jefferson, T. A., and Collins, T. (2011). West Africa's Atlantic Humpback Dolphin (Sousa teuszii): endemic, enigmatic and soon endangered? Afr. Zool. 46, 1–17. doi: 10.3377/004.046.0101
Whitty, T. S. (2015). Governance potential for cetacean bycatch mitigation in small-scale fisheries: a comparative assessment of four sites in Southeast Asia. Appl. Geography. 59, 131–141. doi: 10.1016/j.apgeog.2015.01.003
Williams, A., Dowdney, J., Smith, A. D. M., Hobday, A. J., and Fuller, M. (2011). Evaluating impacts of fishing on benthic habitats: a risk assessment framework applied to Australian fisheries. Fish. Res. 112, 154–167. doi: 10.1016/j.fishres.2011.01.028
Williams, R., Burgess, M. G., Ashe, E., Gaines, S. D., and Reeves, R. R. (2016). U.S. seafood import restriction presents opportunity and risk. Science 354, 1372–1374. doi: 10.1126/science.aai8222
Wilson, J. A., Acheson, J. M., Metcalfe, M., and Kleban, P. (1994). Chaos, complexity and community management of fisheries. Mar. Policy 18, 291–305. doi: 10.1016/0308-597X(94)90044-2
Winkler, J. A. (2016). Embracing complexity and uncertainty. Ann. Am. Assoc.Geogr. 106, 1418–1433. doi: 10.1080/24694452.2016.1207973
Worm, B., Hilborn, R., Baum, J. K., Branch, T. A., Collie, J. S., Costello, C., et al. (2009). Rebuilding global fisheries. Science 325, 578–585. doi: 10.1126/science.1173146
Keywords: complexity, megafauna, bycatch, fisheries management, complexity-awareness
Citation: Lewison RL, Johnson AF and Verutes GM (2018) Embracing Complexity and Complexity-Awareness in Marine Megafauna Conservation and Research. Front. Mar. Sci. 5:207. doi: 10.3389/fmars.2018.00207
Received: 05 January 2018; Accepted: 28 May 2018;
Published: 26 June 2018.
Edited by:
Lisa Marie Komoroske, National Oceanic and Atmospheric Administration (NOAA), United StatesReviewed by:
Graham Pierce, Instituto de Investigaciones Marinas (IIM), SpainDaniel Paul Costa, University of California, Santa Cruz, United States
Beatriz Dos Santos Dias, University of Massachusetts Amherst, United States
Copyright © 2018 Lewison, Johnson and Verutes. This is an open-access article distributed under the terms of the Creative Commons Attribution License (CC BY). The use, distribution or reproduction in other forums is permitted, provided the original author(s) and the copyright owner are credited and that the original publication in this journal is cited, in accordance with accepted academic practice. No use, distribution or reproduction is permitted which does not comply with these terms.
*Correspondence: Rebecca L. Lewison, cmxld2lzb25Ac2RzdS5lZHU=