- 1Marine and Environmental Sciences Centre, Instituto do Mar, OKEANOS Research Unit, Universidade dos Açores, Horta, Portugal
- 2ICES Secretariat, Copenhagen, Denmark
- 3South Atlantic Environmental Research Institute, Stanley, Falkland Islands
- 4Ocean and Earth Science, National Oceanography Centre Southampton, University of Southampton, Southampton, United Kingdom
- 5Centro Oceanográfico de Vigo, Instituto Español de Oceanografía, Vigo, Spain
- 6Marine Scotland Science Marine Laboratory, Aberdeen, United Kingdom
In international fisheries management, scientific advice on the presence of “vulnerable marine ecosystems” (VMEs) per United Nations resolutions, has generally used qualitative assessments based on expert judgment of the occurrence of indicator taxa such as cold-water corals and sponges. Use of expert judgment alone can be criticized for inconsistency and sometimes a lack of transparency; therefore, development of robust and repeatable numeric methods to detect the presence of VMEs would be advantageous. Here, we present a multi-criteria assessment (MCA) method to evaluate how likely a given area of seafloor represents a VME. The MCA is a taxa-dependent spatial method that accounts for both the quantity and data quality available. This was applied to a database of records of VMEs built, held and compiled by the International Council for the Exploration of the Sea (ICES). A VME index was generated which ranged from 1.51 to 4.52, with 5.0 being reserved for confirmed VME habitats. An index of confidence was also computed that ranged from 0.0 to 0.75, with 1 being reserved for those confirmed VME habitats. Overall the MCA captured the important elements of the ICES VME database and provided a simplified, spatially aggregated, and weighted estimate of how likely a given area is to contain VMEs. The associated estimate of confidence gave an indication of how uncertain that assessment was for the same given area. This methodology provides a more systematic and standardized approach for assessing the likelihood of presence of VMEs in the North-East Atlantic.
Introduction
Recognizing the vulnerability of deep-sea biodiversity, the United Nations General Assembly (UNGA) called upon States and Regional Fisheries Management Organizations (RFMOs) to identify areas beyond national jurisdiction (ABNJ) where vulnerable marine ecosystems (VME) occur, or are likely to occur, and to prevent significant adverse impacts (UNGA, 2006). The Food and Agricultural Organization (FAO) of the United Nations subsequently developed guidelines for the management of deep-sea fisheries in the high seas (FAO, 2009). This included criteria for defining what constitutes a VME: (1) uniqueness or rarity; (2) functional significance of the habitat; (3) fragility; (4) life history traits of component species that make recovery difficult; and (5) structural complexity. These criteria may apply to a wide variety of habitats and ecosystems of the deep-sea (e.g., hydrothermal vents, seamounts, or cold seeps). Generally, VMEs have been identified based on the occurrence of indicator taxa such as stony or gorgonian corals, or sponges. However, these taxa can occur in varying spatial densities, and the FAO guidelines do not provide threshold values for defining what constitutes “significant concentrations” of VME indicator records that would constitute an actual VME (Auster et al., 2011).
Whilst significant steps have been made to map and protect VMEs in the high-seas areas in general (e.g., Portela et al., 2010) and in the high-seas areas regulated by RFMOs (e.g., Durán-Muñoz et al., 2012a), progress has been inconsistent or incomplete (Durán-Muñoz and Sayago-Gil, 2011; Wright et al., 2014; FAO, 2016). In part, limited knowledge about their spatial distribution has impeded the application of effective protective measures in many areas (Weaver et al., 2011). Although dedicated field surveys and species distribution models of some individual VME indicator taxa are increasingly being made available (Rengstorf et al., 2013, 2014; Vierod et al., 2014; Fabri et al., 2014; Anderson et al., 2016b), there is little information on the spatial distribution of species assemblages in concentrations that might constitute a VME and therefore what is needed to trigger management actions (Ardron et al., 2014).
Vulnerable marine ecosystems are best identified using high quality underwater imagery (Remotely Operated Vehicles – ROV, towed camera, etc.), allowing accurate and quantitative description of community composition and associated fauna (e.g., Fabri et al., 2014). Because of the high cost of operations associated with such imaging technologies, observations of VMEs are only available for a tiny fraction of the area of the deep seabed. However, with rapid advances in photo- and video-survey technology, much more of the seafloor will become known in the coming years. Techniques developed to identify VMEs could therefore inform what statistical power and effect size should be used to detect VMEs in future broad-scale surveys. Currently, larger amounts of data on VME indicator taxa occurrences across large spatial scales may be available from bycatch records from fisheries surveys (e.g., Murillo et al., 2011, 2012; Portela et al., 2012), cooperative surveys (Durán-Muñoz et al., 2011, 2012b) and commercial fishing operations. The problem in using bycatch data to inform on the presence of VMEs lies in the fact that bottom fishing gear are poor sampling tools for VME indicator organisms and that bycatch data may not represent the true benthic community composition and densities (Auster et al., 2011). Although better than no data, there is a large amount of uncertainty associated with bycatch data.
In the Northwest Atlantic Fisheries Organization (NAFO) Regulatory Area, a geospatial methodology was developed to identify VMEs using data from research trawl surveys (Kenchington et al., 2014). However, this approach requires comprehensive fisheries survey programs (Murillo et al., 2011, 2012) and standardized datasets that are generally not available for most areas beyond national jurisdiction. Generally, data on VME indicator presence are derived from a wide variety of sources (commercial trawl and longline operations, ROV surveys, towed camera surveys or research surveys), which are challenging to integrate and interpret as a whole. For example, some records are bona fide VME habitat types such as those identified through recent ROV video footage of large Lophelia reefs while others are scientific trawl survey bycatch records and anecdotal information from commercial fishing operations. As a result, VME related data are generally qualitatively assessed using expert judgment (ICES, 2013a). Expert judgment is a common component of resource management and conservation decision-making, but comes with known limitations and inconsistencies, many of which concern how disparate information is contextualized, and a poor characterization of uncertainty (Burgman et al., 2011; Martin et al., 2012). To aid decision-making concerning the protection of VMEs, we have developed methods that are capable of integrating the wide range of existing VME data, while taking into account some of the uncertainties associated with sampling methods and reported taxonomy.
Over the past 10 years the joint ICES/NAFO Working Group on Deep-water Ecology (WGDEC) has been compiling data on the distribution and abundance of VMEs and organisms considered to be indicators of VMEs across the North Atlantic (ICES, 2011). A database of these records was built to facilitate more scientifically robust advice on the distribution of VMEs and to aid the development of possible management recommendations such as bottom fishing closures within North East Atlantic Fisheries Commission (NEAFC) waters to protect VMEs. Thus there was a need to develop a system that would formalize expert opinion and utilize as much relevant information as possible from the ICES VME database. In this paper we present a multi-criteria assessment (MCA) method that was developed to evaluate how likely an area represents a VME, through integrating as much relevant information as possible from the ICES VME database.
Materials and Methods
The term VME is used here in the context of the FAO international guidelines (FAO, 2009) and reviewed by ICES (ICES, 2016b). The ICES VME database (ICES, 2016a) can be accessed online and is currently comprised of approximately 15,000 records (Figure 1).
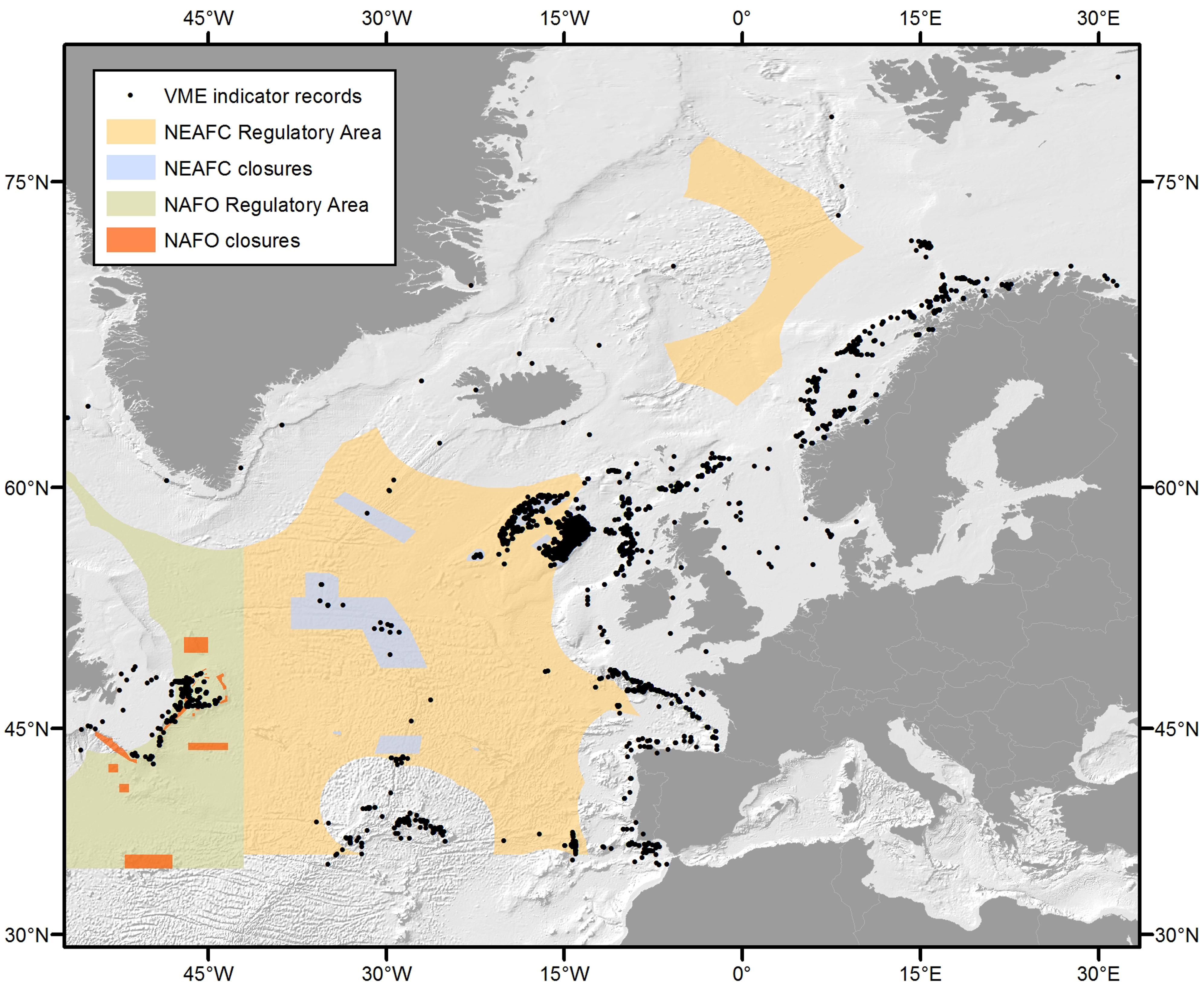
Figure 1. The distribution of VME indicator records throughout the North Atlantic contained within the ICES VME database (ICES, 2016a).
Multi-criteria assessment is a method of aggregating data based on different criteria or attributes that contain information relevant to the decision and weighting these to provide a single metric that captures all this information. The essential feature of MCA is the development of a matrix in which the performance of the data are weighted against each criterion (e.g., the survey method) and from which an aggregate value is derived. The MCA was based on spatially gridded data format, i.e., multiple individual points or records contributed to the assessment of a single grid cell containing a VME. A C-square spatial grid methodology (Rees, 2003) composed of grid cell size of 0.05 degrees by 0.05 degrees was adopted, similar to that used to analyze Vessel Monitoring System (VMS) data (Hintzen et al., 2012). As described below, the MCA captures the fact that not all VME indicators within the ICES VME database have the same vulnerability to human impacts, and thus should be weighted differently. Therefore, the MCA is a taxa-dependent spatial method. Additionally, to account for data quality issues a measure of the confidence associated with each VME record was developed.
For each c-square grid cell, two values were calculated:
• A “VME index” which combines how intrinsically vulnerable to human impacts the VME indicator is deemed to be, and how abundant the VME indicator is (for example, an aggregation representing a cold-water coral reef as opposed to a record of a single individual or taxon).
• A “Confidence index” associated with the “VME index.” This is a confidence (or uncertainty) estimate based upon (a) the numbers of samples available within the grid cell, (b) the provenance of the records in that cell (e.g., visual survey, fisheries data, or inferred from other methods), (c) the time span of the data (i.e., time between the first and last record), and (d) the age of the most recent survey.
“VME Index” Scoring Procedure
Because the ICES VME database includes both records of known VME habitats (ICES, 2016a) as well as VME indicators, these former bona fide records were treated separately from the latter. Bona fide records included high quality underwater imagery from ROV surveys of anemone aggregations, cold seeps, cold-water coral reefs, coral gardens, deep-sea sponge aggregations, hydrothermal vents, and sea-pen fields. Non-VME habitat grid cells (i.e., not containing bona fide records) were evaluated by assigning a VME indicator score to each VME indicators present, based on their taxonomy and their abundance as explained below.
Assigning a VME Indicator Score to VME Indicators
Twelve VME indicator types were agreed for inclusion in the ICES VME database (ICES, 2016b), reflecting the main taxonomic groups of VME indicators occurring in the NE Atlantic (Supplementary Table 1). Naturally, there are some interspecific inconsistencies, for example, not all species of “Gorgonian” will be equally vulnerable. However, these categories were considered to be a reasonable compromise between a manageable list and a range of vulnerabilities that was not excessive (ICES, 2016a). The category “sponges” was found to be particularly problematic because it includes all sponges, from small encrusting species to the massive, aggregation forming species (e.g., Geodiids). The reason a generic “sponges” category was created in the past was because there is often much uncertainty in species identification and many records in the VME database are simply identified to the Phylum level of “Porifera.” Nevertheless, the VME database contains sponge records identified to the lowest possible taxonomical level, which is of value for use in the MCA. When a sponge record was identified to the genus level, literature sources (e.g., Hogg et al., 2010; Murillo et al., 2012; McIntyre et al., 2016) and expert opinion was used to decide on those genera of sponges containing species that can be described as “massive” and forming aggregations. All species belonging to the following genera were classified as the type “Large Sponge” and as such would receive a different “VME” score than all other sponges: Asconema, Craniella, Chonelasma, Geodia, Pheronema, Polymastia, Stryphnus, Tetilla, Thenea, and Vazella. All other species of sponges and records for which no information of Genus were provided were ranked according to the scores in the type “Generic Sponge.” Following this assessment of sponge types, thirteen VME indicator types were considered in the next phase (Table 1).
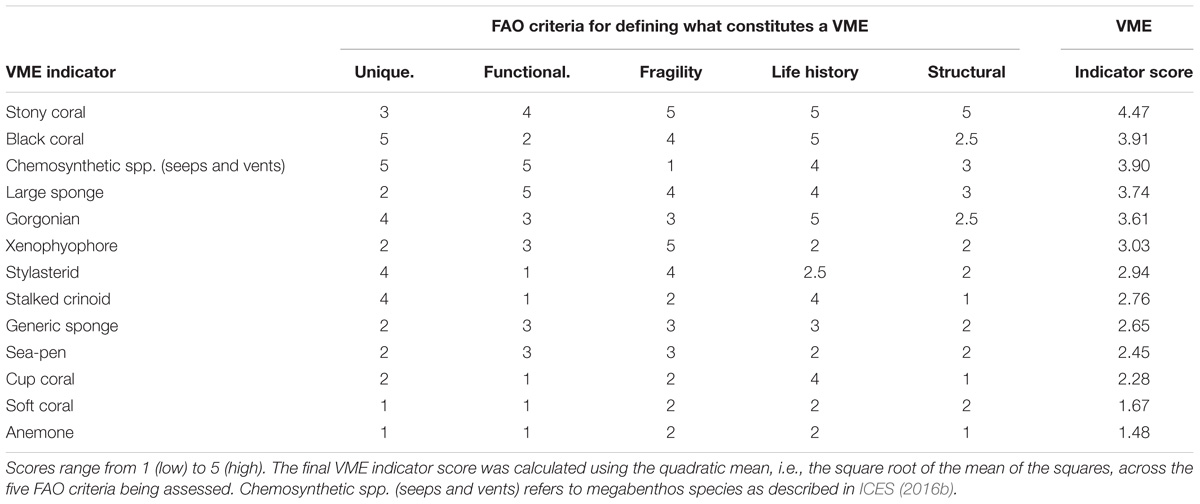
Table 1. Scores for VME indicators occurring in deep-water in the ICES/NAFO area based on the degree to which each VME indicator fit each of the five FAO criteria (FAO, 2009).
Vulnerable marine ecosystem indicator types included in the ICES VME database were assessed against each of the five FAO criteria for defining what constitute a VME. The FAO list of characteristics used as criteria in the identification of VMEs are (FAO, 2009):
• Uniqueness or rareness: an area or ecosystem that is unique or that contains rare species whose loss could not be compensated for by similar areas or ecosystems.
• Functional significance of the habitat: discrete areas or habitats that are necessary for the survival, function, spawning/reproduction, or recovery of fish stocks, particular life-history stages (e.g., nursery grounds or rearing areas), or of rare, threatened or endangered marine species.
• Fragility: an ecosystem that is highly susceptible to degradation by anthropogenic activities.
• Life-history of species make recovery difficult: ecosystems that are characterized by populations or assemblages of species with one or more of the following characteristics: slow growth rates, late age of maturity, low or unpredictable recruitment, or long-lived.
• Structural complexity: an ecosystem that is characterized by complex physical structures created by significant concentrations of biotic and abiotic features.
• The degree to which each VME indicator group (not the individual taxa contained) fit each of the five FAO criteria was scored from 1 (low) through 5 (high). The scoring procedure was discussed and agreed by a group of deep-sea scientists through an ICES Expert Group using existing informed expert judgment, and the following specific guidelines (Supplementary Table 2):
• Rarity: was scored according to presence on the IUCN red list, and if the indicator was known to be endemic, rare, threatened, or declining.
• Functionality: was scored by evaluating if the indicators were known to create nursery areas for other species, or known for having higher level ecosystem role, such as nutrient cycling and water filtration.
• Fragility: was scored according to the fragility of the indicator against physical contact, the height and complexity of its structure, and the capacity for retraction, retention or re-growth or if being naturally protected in some way.
• Life-history: was scored against the longevity, fecundity, age at maturity, growth rate, and known frequency of recruitment success.
• Structural complexity: was scored based on structural habitat created, frame-building, and presence of commensal or closely associated species.
These ratings resulted in VME indicator scores for each VME indicator type (Table 1) where, for example, stony corals were considered the most susceptible to degradation by anthropogenic activities and with life-history characteristics that make recovery more difficult. It should, however, be noted that these scores could change if new data were to become available and when the taxonomic resolution of the indicators present in the database is improved. As the five FAO criteria were seen as being approximately orthogonal, the final VME indicator score for each of the thirteen VME indicators was calculated using the quadratic mean, i.e., the square root of the mean of the squares.
Assigning Abundance Score to VME Indicator Records
For each record with weight data in the database, the abundance recorded was evaluated against the NEAFC (Recommendation 19 2014: Protection of VMEs in NEAFC Regulatory Areas as Amended by Recommendation 09:2015) and EU (Regulation 2016/2336) VME encounter thresholds for live corals (30 kg) or live sponges (400 kg). If the abundance was over the encounter threshold, a value of 5 was assigned. If the abundance was below the encounter threshold we used Jenks natural breaks classification method (Jenks, 1967) to identify an intermediate encounter threshold. Therefore, a value of 3 was assigned if abundance was above 1 kg (for corals) or 60 kg (for sponges) and otherwise a value of 1 was assigned. If no data for abundance were available, a score of 0 was allocated to the “abundance score” and thus had no effect on the final “VME index.” As there are no agreed thresholds for VME indicators that are not “corals” or “sponges” (e.g., anemones) we used the encounter thresholds values defined for corals. Although the NEAFC thresholds are considered to be too high and not based on robust scientific data (Ardron et al., 2014), without agreed thresholds this was considered the most appropriate option, so as to be relevant for current (albeit imperfect) management practices.
Defining the Final “VME Index”
Vulnerable marine ecosystem bona fide habitats identified in the ICES VME database received the maximum “VME index” of 5. The final “VME index” for each remaining records was calculated based on the VME indicator score and the abundance score. In the current version of the MCA we gave 90% weight to the “VME indicator score” and 10% weight to the abundance score. A low weighting was assigned to the abundance score because of the limited number of records where such information is available, and because there is much uncertainty regarding encounter thresholds when little is known about how VMEs abundances and vulnerabilities have been estimated (ICES, 2012).
After assigning a VME index to each VME indicator record, the results were then aggregated to a grid cell of 0.05 degrees × 0.05 degrees. For each cell, the maximum VME index value was retained as the overall value for that cell. This was to prevent down-weighting of important records by less important records as would happen if, for example, the median or the mean value of a cell was used. It was therefore acknowledged that some cells would have high scores due to a single high scoring record even when other records in that cell may have a low score. This approach was viewed as consistent with the precautionary approach.
The final outcome was presented as VME habitat for these grid cells containing bona fide records and as three nominal categories of “VME index” scores, indicating the likelihood of encountering a VME in the assessed grid cells. Thresholds were computed using the Jenks natural breaks classification method (Jenks, 1967). The categories were: low “VME index,” for total scores <2.64; medium, for total scores between 2.64 and 3.74; and high, for total scores >3.74. The breaks produced suggested that the high “VME index” scores would pick out stony corals at any abundance, and black corals, large sponges and chemosynthetic species when above the NEAFC VME threshold. The medium “VME index” scores would pick out black corals, sponges and chemosynthetic species when below the NEAFC VME threshold, and gorgonians, stylasterids, sea-pens, sponges, xenophyophores, and stalked crinoids when above the threshold.
“Confidence Index” Scoring Procedure
To account for data uncertainty such as data quality issues and the varying degree of knowledge regarding each cell (i.e., how well it has been surveyed), we developed a data confidence index similar to the ones elaborated by Wallace et al. (2011). This index served as a measure of confidence in the “VME index” scores assigned to individual grid cells and was calculated independently of the “VME index.” The “Confidence index” was not calculated for bona fide VME habitats identified in the ICES VME database where a confidence index of one was allocated.
Two measures are usually incorporated in such indices (Wallace et al., 2011; Taranto et al., 2012): data quality and data deficiency. We considered using a measure of data deficiency for each grid cell but did not implement that measure as data deficiency is being partially covered in the data quality measure. Therefore, data quality here reflects the origin and nature of the collected data and was divided into three categories: low (scored as 0), medium (scored as 0.5), and high (scored as 1) data quality. The high data quality category highlights cells with information derived from scientific visual surveys, sampled by many independent surveys (>5 surveys), over a long time period (>10 years), and where the most recent record is recent (<10 years) and thus giving an idea if the VME is still present. Low quality data refers to a VME index derived from a poorly sampled grid cell (<3 surveys), where the presence of a VME had been somehow inferred, sampled for only a short period (<5 years) and a long time ago (>30 years). Consequently, four distinct criteria were used for estimating the data “Confidence index” (Table 2).
The resulting data “Confidence index” for each grid cell was calculated as the quadratic mean (i.e., the square root of the mean of the squares) of the scores associated with the records producing the highest “VME index,” and had a minimum value of 0 (few factors scored or low data quality) and a maximum value approaching 1 (all factors scored with high data quality). As in the “VME index,” the final outcome was presented by grid cell containing no uncertainty (i.e., confidence index of 1) or VME bona fide habitat, and as three nominal categories of “Confidence index” computed using the Jenks natural breaks classification method (Jenks, 1967): low confidence, for scores smaller than 0.35; medium confidence, for score between 0.35 and 0.63; and high confidence for scores greater than 0.63.
Implementation of the MCA to the ICES VME Database
The implementation of the MCA can be illustrated schematically by generating maps of the VME and Confidence indices (Figure 2). By combining these maps, cells with high “VME index” scores and different confidences can be highlighted. In the top panel of Figure 2, VME bona fide habitats with total confidence are highlighted. In the second panel of Figure 2, we highlighted those grid cells scoring high in the “VME index” with all confidence categories. In the third panel, grid cells scoring high in the “VME index” but excluding those cells with a low confidence index were highlighted. In the bottom panel of Figure 2, we highlighted only those grid cells scoring high in the VME index and high confidence. It should be noted that cells with low confidence are not unimportant, but rather the degree of uncertainty means that additional sampling is required to produce a more reliable “VME index” value. Implementation of the framework was done through the development of an R script that accessed the tables in the ICES VME database.
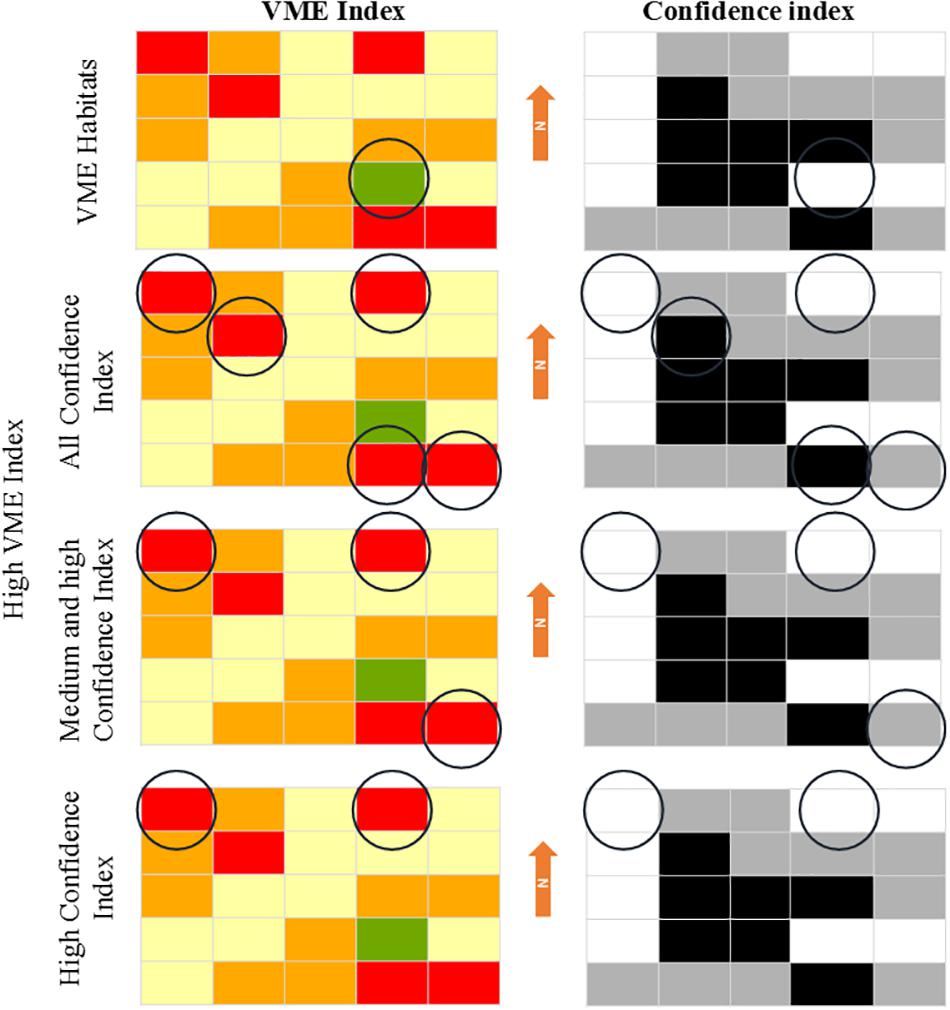
Figure 2. Representation of the usefulness of the VME and Confidence indices maps. The “VME index” hypothetical map contains VME habitats in green, cells with high “VME index” scores in red, medium in orange, and low in yellow. The “Confidence index” map contained cells with high confidence score in white, medium in gray, and low confidence in black. Circles indicate those cells in the hypothetical map that meet the criteria described on the left of the panel.
Prior to the application of the procedure, a detailed quality check of the ICES VME database was performed. This included: (1) standardization and correction of the information contained in all fields; (2) filling missing mandatory fields or correcting detected errors by contacting original data providers, by thorough searches, or ultimately deleting records for which validation was not possible; (3) correction and validation of all species identifications using World Register of Marine Species database (WoRMS Editorial Board, 2017); and (4) deleting duplicated records.
VME and Fishing Effort Portfolio Categories
One of the main advantages of deriving a gridded “VME index” is that is can be directly compared to other gridded data with the same grid size, as for example fishing effort. Fishing intensity data can therefore be used to account for the anthropogenic activities occurring within each cell. We suggest an approach that combines the “VME index” and the level of fishing activity, measured through VMS data. This methodology allows the classification of individual cells into four main categories, which can help in optimizing management efforts toward spatial management: Low VME index-Low fishing; Low VME index-High fishing; High VME index-Low fishing; High VME index-High fishing. “VME index” and fishing intensity for individual cells can therefore be easily summarized and graphically compared (Figure 3).
The application of the VME and fishing effort portfolio categories to the ICES VME and VMS databases was tested. Here the VME index used was calculated as described above. For estimating the fishing effort for each grid cell, the cumulative number of records of trawling activity (vessel speed from 1 to 4 knots) from VMS data for the past 10 years was used (ICES, 2015). Due to the non-normal range of values, including the presence of few cells with very large records of trawling activity, the cumulative number of records was log transformed. Fishing effort was then re-scaled from 1 where no fishing activity is present to 5 where the maximum value of fishing effort was reached.
Results and Discussion
The FAO guidelines provide a comprehensive list of species and habitats that form VMEs. Intuitively all types of VMEs are deemed vulnerable, however, the current scientific information on function, fragility and life-history of various types of VME indicator species suggest that some VMEs should be considered more vulnerable to anthropogenic impacts than others. For example, deep-sea stony corals aggregations create structural diverse habitats, are relatively long lived and slow growing and are very sensitive to bottom fishing impact (Clark et al., 2016 and references therein). On the other hand, sea pens, while they are much less well understood, do not appear to as slow growing or long lived (de Moura Neves et al., 2015). We sought to capture this variation in developing our methodology by developing a means of weighting the vulnerability of different types of VMEs.
Implementation of the MCA to the ICES VME Database
When applying the MCA to the ICES VME database, the VME index ranged from 1.51 to 4.52. A score of 5.0 was reserved for records of bona fide VME habitats. The observed frequency of the VME index showed a unimodal distribution with most cells being between 2 and 4 (Figure 4). The output appears to capture the main features of the database despite the paucity of life-history data for many of the VME indicator taxa (e.g., Ramirez-Llodra et al., 2010). The results remain to some extent dependent on the expert judgment on scoring how each VME indicator fit the FAO VME criteria. This source of variability, however, was minimal as scores were thoroughly discussed and agreed by a group of approximately 20 deep-sea experts. We recognize that knowledge gaps for some taxon are a major limitation for appropriately scoring some VME indicators (e.g., anemones or sponges). As more scientific information is gathered, this “inter-VME” weighting may be revised by WGDEC.
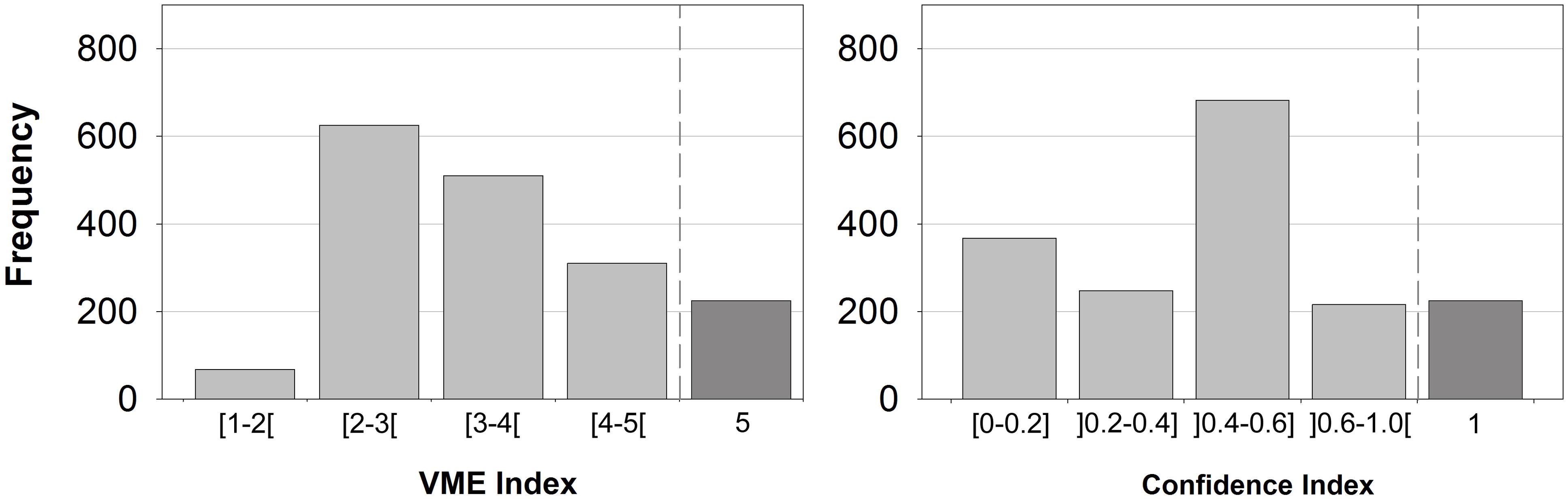
Figure 4. Resulting distribution of the VME index (left) and the confidence index (right) when applied to the ICES VME database, including certain VME habitats (VME index of 5 and confidence index of 1; n = 225). Each value represents one grid cell of approximately 0.05 degrees by 0.05 degrees.
The VME index is also dependent on the encounter thresholds adopted by NEAFC. There is currently a recommendation to reduce these to 15 kg of live corals and 200 kg of live sponges (ICES, 2012). If new encounter thresholds are adopted, the MCA methodology should be updated accordingly.
The confidence index ranged from 0.0 to 0.75. Again a score of 1 was reserved for those records of bona fide VME habitats (Figure 4). Most of the cells showed a confidence index lower than 0.6 highlighting a reduced sampling effort, with records often falling into the lowest category.
Hatton-Rockall Bank
The Hatton-Rockall Bank area in the NE Atlantic provides a model case study to illustrate the application of the MCA approach. Scientific data on the presence of coral at Rockall dates back to the 1970s (Wilson, 1979) and a spate of recent surveys have revealed the occurrence of coral mounds (Wienberg et al., 2008; Durán Muñoz et al., 2009), cold seep ecosystems (Neat et al., 2018), and other important geomorphological features throughout the area (Roberts et al., 2008; Sayago-Gil et al., 2010). In addition, several VME indicator species including cold-water corals and sponges have been identified in recent years through collaboration with commercial trawl and longline fisheries (Durán-Muñoz et al., 2011, Durán-Muñoz et al., 2012b). Thus the variable amount of information for this area and the range in quality of that information (e.g., fishing records vs. scientific observations), serves as a good case study to illustrate the approach.
The data outputs for the Hatton-Rockall Bank area illustrate several important aspects of the MCA (Figure 5). First, there were some bona fide VME habitats identified in the Rockall Bank which are clearly highlighted by the method (Figure 5a). Second, the areas identified by the MCA as high values of “VME index” often fell within existing NEAFC closures for example NW Rockall and SW Rockall. This suggests the method is useful for identifying areas that need protection. Third, it identified areas of medium and high value of “VME index” situated outside closed areas thereby suggesting such areas should be carefully assessed and possibly protected. Finally, in Figure 5b, it is apparent that the intensive sampling on the Rockall plateau yields high confidence, whereas the less-well sampled Hatton Bank yields lower overall confidence. This can then be seen particularly clearly where only the cells with medium and high confidence are plotted (Figures 5c,d). This does not mean Rockall Bank is more important from a VME perspective; rather, only that we are more confident that Rockall Bank is an important VME area. There is good evidence that VMEs are present at Hatton Bank, but the confidence of these records is not as high as it is at the Rockall Bank.
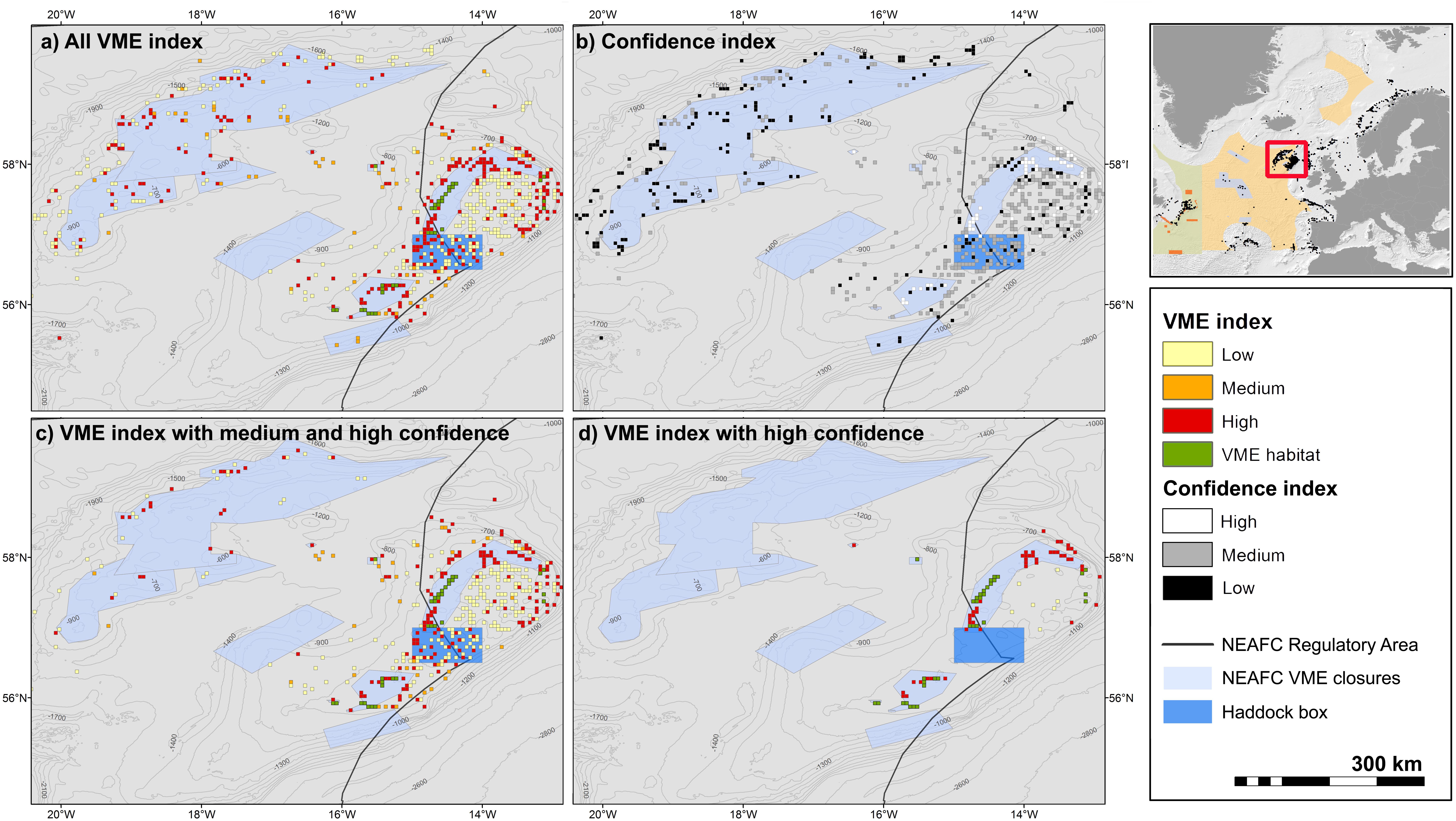
Figure 5. Applying the VME index and confidence index to the VME database: Rockall and Hatton Bank. (a) VME index for all cells with data in the ICES VME database; (b) confidence index for all cells with data in the ICES VME database; (c) VME index for cells with medium and high confidence; and (d) VME index for cells with high confidence.
Charlie-Gibbs Fracture Zone
The Charlie-Gibbs Fracture Zone (CGFZ) area in the Mid-Atlantic Ridge between Iceland and the Azores, provides a model case study to illustrate the limitations of the application of the MCA approach to data-poor areas. A portion of the CGFZ was nominated as an OSPAR MPA and adopted by NEAFC to protect the unique natural features associated with the Mid-Atlantic Ridge. Due to its remoteness, very limited information on the benthic communities is available. The main exceptions were the MAR-ECO and ECOMAR expeditions that recorded cold-water corals belonging to 34 different taxa in areas close to the CGFZ (Molodtsova et al., 2008; Mortensen et al., 2008) along with different species of hexactinellid sponges and echinoderms (Gebruk and Krylova, 2013). During the MAR-ECO expedition Lophelia pertusa was frequently observed but no large live reef structures have been detected (Mortensen et al., 2008). Thus the limited amount of information with limited spatial coverage for this area, serves as an illustrative data-poor case study.
The data outputs for the CGFZ area illustrate several important aspects of the ICES VME database and the MCA (Figure 6). Although we found literature sources describing the occurrence of stony corals in the area (e.g., Mortensen et al., 2008), those have not been transposed to the ICES VME database which highlights the need for an improved data reporting to ICES. Also, the records in the database lack important information such the survey method or the observation date preventing a proper estimation of the confidence index, highlighting the need for strictly following of the ICES VME data reporting guidelines. The application of the MCA to the CGFZ reveals some areas with medium values of “VME index” with very low confidence scores (Figure 6), demonstrating the limitations of the MCA applications to data-poor areas.
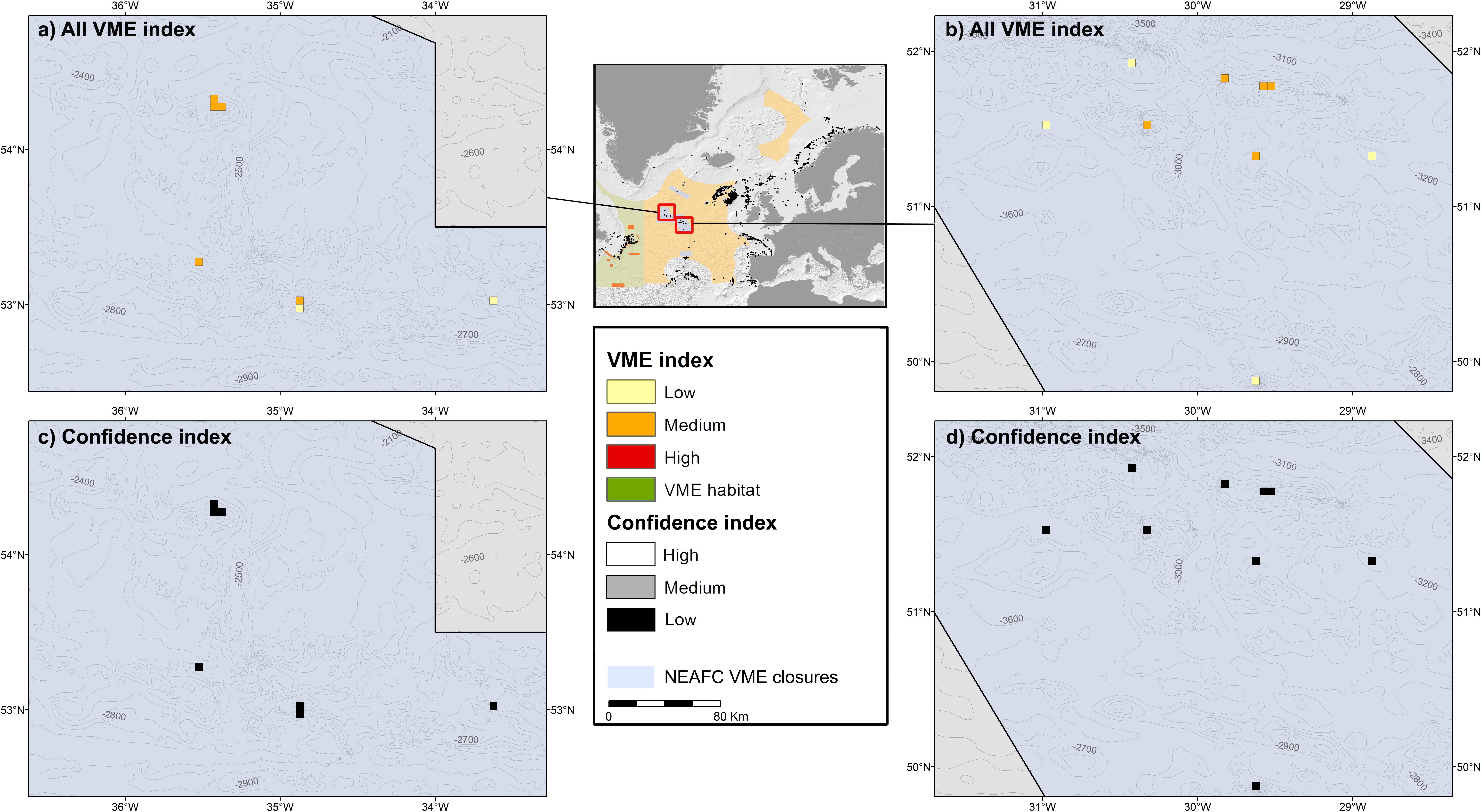
Figure 6. Applying the VME index and confidence index to the VME database: Charlie-Gibbs Fracture Zone. (a,b) VME index for all cells with data in the ICES VME database; (c,d) confidence index for all cells with data in the ICES VME database.
VME and Fishing Effort Portfolio Categories
The ICES data was assigned to all four portfolio categories (Figure 7). The outcomes of the framework can be visualized for comparing different areas, allowing managers to prioritize their choices or policies in terms of closing pristine VME areas, closing disturbed areas for recovery of VMEs, or both (Figure 7). A large portion of the cells were revealed as high VME and low VMS (59%) or low VME and low VMS (35%) (Figure 7). Only a small portion of the cells fell in the category high VME and high VMS (2%) (Figure 7). However, the high numbers of cells with low fishing effort should be considered with caution because: (1) many cells fall within national jurisdiction for where we had limited access to VMS data; (2) a large portion of the fishing vessels do not have a fishing license assigned and therefore are not considered as trawlers and were excluded from the analyses; and (3) some areas were closed to fishing in recent years but may have experienced higher impacts previously.
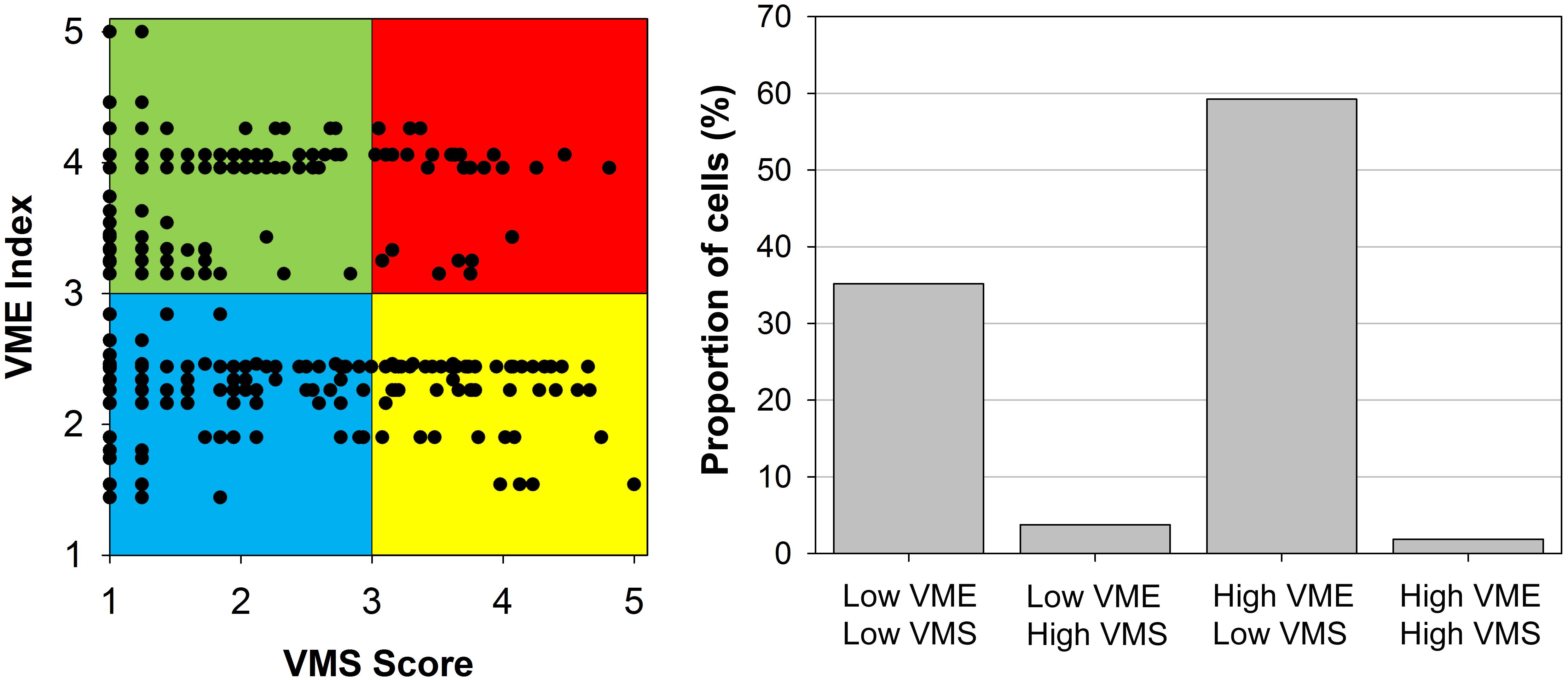
Figure 7. Application of the portfolio categories concept to the ICES database. In the left panel, the different colors represent four portfolio categories: blue is low VME – low VMS, yellow is low VME – high VMS, green is high VME – low VMS, and red is high VME – high VMS. In the right panel, proportions of cells falling into those categories are shown.
Mapping the outcomes of the framework can be another way to visualize areas falling in different portfolio categories. For example, in the Hatton-Rockall area (Figure 8), despite the shortcomings of the VMS data described above, most areas of high VME score lie inside closed areas and have low fishing effort. Only few cells with high VME index and high fishing effort are observed mostly, around the closed areas.
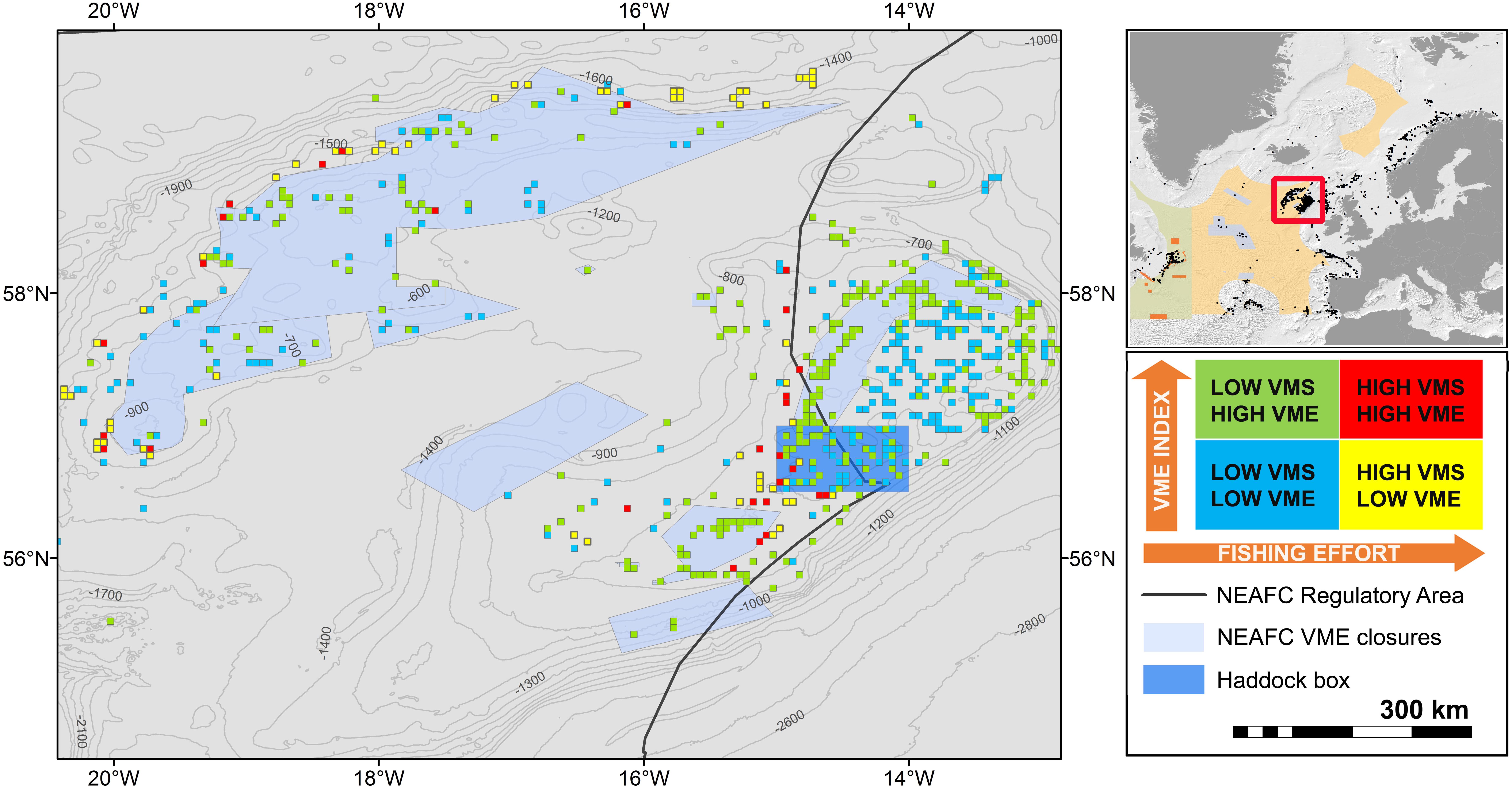
Figure 8. Application of the portfolio categories concept to the Hatton-Rockall area. Blue cells are low VME – low VMS, yellow cells are low VME – high VMS, green cells are high VME – low VMS, and red cells are high VME – high VMS.
Conclusion
In general, the MCA provides a simplified, spatially aggregated and weighted estimate of the degree an area could be considered to contain VMEs under the FAO definition. The VME index clearly highlights areas where a VME is more likely to occur while the associated estimate of confidence gives an indication of how (un)certain that assessment is. The methodology is transparent and the aggregate cells can be explored in greater detail to reveal the individual data points that have contributed to the assessment. It integrates far more of the information in the ICES VME database than previous methods and as such, better captures the underlying reasoning behind much of WGDEC’s past advice (e.g., ICES, 2013a). The MCA can be expected to be updated each year as new data are submitted and thus provide an up to date, repeatable and defensible source upon which to base advice as new information is received.
The MCA approach achieved three main aims. (1) To develop a system which could provide a measure of the likelihood of a cell constituting a VME and the associated level of fishing activity. The choice of the VME scoring criteria and the definition of the most relevant fishing activities were based on the existing information but should be revisited when more information is made available; as for example on the life history of VME indicator taxa. Using quadratic means to estimate the VME indicator score was chosen to avoid several low scores adding up to a misleadingly high score; but it could still underestimate the value of abundant VME indicators that rank high in only few criteria as compared to less abundant indicators with several high scores. This problem may be overcome by increasing the weight assigned to the abundance score; however, this should only be done when more abundance data in weight are reported in the ICES VME database. (2) To design a system compatible with, and making use of all the data currently available. The major constraint faced by this analysis is the general scarcity of information, mostly related to the lack of abundance data (weight or numbers) for each VME indicator record present in the ICES VME database. Additionally, some work has to be done to develop a methodology to standardize information that has originated from very different sampling methods, as for example fisheries trawls and ROV transects. (3) To deliver an output that is simple to visualize and understand, in order to facilitate its implementation in management deliberations.
The UN General Assembly resolution that committed to protecting VMEs from destructive fishing practices also calls upon States to do so, “consistent with the precautionary approach” (UNGA, 2006, paras. 80 and 83). The inclusion of the confidence index in the MCA allows for decision-making concerning possible fisheries closures (and other management measures) to explicitly determine what level of uncertainty may still warrant precautionary actions.
Overall the MCA appears to capture most of the important elements of the ICES VME database used by WGDEC to assess VMEs. This methodology may be considered as a first step toward a systematic approach for the identification and protection of VME in the NE Atlantic. Our methodology clearly considered several of the steps proposed by Ardron et al. (2014), namely step 1 on assessing potential VME indicator taxa and habitats in a region, step 3 on considering areas already known for their ecological importance, step 4 on compiling information on the distributions of likely VME indicator species and habitats, step 6 on considering fishing impacts, and step 8 on identify ecologically important areas. However, at least one important aspect of the Ardron et al. (2014) framework is missing in the current MCA which refers to understanding the natural distribution of VMEs before significant impacts occurred. This aspect could be considered in future improvements of the MCA to encompass predicted distribution of VME as discussed in Vierod et al. (2014) and Anderson et al. (2016a).
By providing an indication of uncertainty alongside predicted occurrence, the MCA allows for management decisions to be openly discussed, logically weighed, and documented. In the future, with better recognition technology becoming available, these methods could be automated and applied to survey data as they are collected, or soon thereafter, thus avoiding delays that could leave newly identified VME areas at risk. The ability to readily incorporate new data also makes the MCA approach appropriate for adaptive management frameworks.
Author Contributions
FN, TM, NG, JA, and CP designed the study. CP, NG, FN, and PDM compiled the data. CP, TM, FN, NG, and JA analyzed the data. All authors wrote the paper.
Funding
TM was supported by Program Investigador FCT (IF/01194/2013), IFCT Exploratory Project (IF/01194/2013/CP1199/CT0002) from the Fundação para a Ciência e Tecnologia (POPH and QREN), PO2020 MapGes (Acores-01-0145-FEDER-000056), and also acknowledge funds provided by the Fundação para a Ciência e Tecnologia (FCT) through the strategic project (FCT/UID/MAR/04292/2013) granted to MARE. PDM was supported by IEO project ECOPESLE-2, FN was supported by Scottish Government Project ST0150 (MOREDEEP). This work contributes to the European Union’s Horizon 2020 research and innovation program under grant agreement no. 678760 (ATLAS) and no. 679849 (SponGES). This output reflects only the author’s view and the European Union cannot be held responsible for any use that may be made of the information contained therein.
Conflict of Interest Statement
The authors declare that the research was conducted in the absence of any commercial or financial relationships that could be construed as a potential conflict of interest.
Acknowledgments
The authors would like to thank all WGDEC members for their input.
Supplementary Material
The Supplementary Material for this article can be found online at: https://www.frontiersin.org/articles/10.3389/fmars.2018.00460/full#supplementary-material
References
Anderson, O. F., Guinotte, J. M., Rowden, A. A., Clark, M. R., Mormede, S., Davies, A. J., et al. (2016a). Field validation of habitat suitability models for vulnerable marine ecosystems in the South Pacific Ocean: implications for the use of broad-scale models in fisheries management. Ocean Coast. Manag. 120, 110–126. doi: 10.1016/j.ocecoaman.2015.11.025
Anderson, O. F., Guinotte, J. M., Rowden, A. A., Tracey, D. M., Mackay, K. A., and Clark, M. R. (2016b). Habitat suitability models for predicting the occurrence of vulnerable marine ecosystems in the seas around New Zealand. Deep Sea Res. Part I 115, 265–292. doi: 10.1016/j.dsr.2016.07.006
Ardron, J. A., Clark, M. R., Penney, A. J., Hourigan, T. F., Rowden, A. A., Dunstan, P. K., et al. (2014). A systematic approach towards the identification and protection of vulnerable marine ecosystems. Mar. Policy 49, 146–154. doi: 10.1016/j.marpol.2013.11.017
Auster, P. J., Gjerde, K., Heupel, E., Watling, L., Grehan, A., and Rogers, A. D. (2011). Definition and detection of vulnerable marine ecosystems on the high seas: problems with the “move-on” rule. ICES J. Mar. Sci. 68, 254–264. doi: 10.1093/icesjms/fsq074
Burgman, M., Carr, A., Godden, L., Gregory, R., McBride, M., Flander, L., et al. (2011). Redefining expertise and improving ecological judgment. Conserv. Lett. 4, 81–87. doi: 10.1111/j.1755-263X.2011.00165.x
Clark, M. R., Althaus, F., Schlacher, T. A., Williams, A., Bowden, D. A., and Rowden, A. A. (2016). The impacts of deep-sea fisheries on benthic communities: a review. ICES J. Mar. Sci. 73, i51–i69. doi: 10.1093/icesjms/fsv123
de Moura Neves, B., Edinger, E., Layne, G. D., and Wareham, V. E. (2015). Decadal longevity and slow growth rates in the deep-water sea pen Halipteris finmarchica (Sars, 1851) (Octocorallia: Pennatulacea): implications for vulnerability and recovery from anthropogenic disturbance. Hydrobiologia 759, 147–170. doi: 10.1007/s10750-015-2229-x
Durán-Muñoz, P., Murillo, F. J., Sayago-Gil, M., Serrano, A., Laporta, M., Otero, I., et al. (2011). Effects of deep-sea bottom longlining on the Hatton Bank fish communities and benthic ecosystem, north-east Atlantic. J. Mar. Biol. Assoc. U.K. 91, 939–952. doi: 10.1017/S0025315410001773
Durán Muñoz, P., Sayago-Gil, M., Cristobo, J., Parra, S., Serrano, A., Díaz del Rio, V., et al.. (2009). Seabed mapping for selecting cold-water coral protection areas on Hatton Bank, Northeast Atlantic. ICES J. Mar. Sci. 66, 2013–2025. doi: 10.1093/icesjms/fsp170
Durán-Muñoz, P., and Sayago-Gil, M. (2011). An overview of cold-water coral protection on the high seas: the Hatton Bank (NE Atlantic)—a case study. Mar. Policy 35, 615–622. doi: 10.1016/j.marpol.2011.01.023
Durán-Muñoz, P., Sayago-Gil, M., Murillo, F. J., Del Rio, J. L., López-Abellán, L. J., Sacau, M., et al. (2012a). Actions taken by fishing Nations towards identification and protection of vulnerable marine ecosystems in the high seas: the Spanish case (Atlantic Ocean). Mar. Policy 36, 536–543. doi: 10.1016/j.marpol.2011.09.005
Durán-Muñoz, P., Sayago-Gil, M., Patrocinio, T., Gonzalez-Porto, M., Murillo, F. J., Sacau, M., et al. (2012b). Distribution patterns of deep-sea fish and benthic invertebrates from trawlable grounds of the Hatton Bank, north-east Atlantic: effects of deep-sea bottom trawling. J. Mar. Biol. Assoc. U.K. 92, 1509–1524. doi: 10.1017/S002531541200015X
Fabri, M. C., Pedel, L., Beuck, L., Galgani, F., Hebbeln, D., and Freiwald, A. (2014). Megafauna of vulnerable marine ecosystems in French mediterranean submarine canyons: spatial distribution and anthropogenic impacts. Deep Sea Res. Part II 104, 184–207. doi: 10.1016/j.dsr2.2013.06.016
FAO (2009). Report of the technical consultation on international guidelines for the management of deep-sea fisheries in the high seas, Rome. 4–8 February and 25–29 August 2008. Food Agric. Organ. U.N. Fish. Aquac. Rep. 881:86.
FAO (2016). Vulnerable marine ecosystems. Processes and practices in the high seas. Food Agric. Organ. U.N. Fish. Aquac. Techn. Pap. 595:185.
Gebruk, A. V., and Krylova, E. M. (2013). Megafauna of the Charlie–Gibbs fracture zone (northern Mid-Atlantic Ridge) based on video observations. J. Mar. Biol. Assoc. U.K. 93, 1143–1150. doi: 10.1017/S0025315412001890
Hintzen, N. T., Bastardie, F., Beare, D., Piet, G. J., Ulrich, C., Deporte, N., et al. (2012). VMStools: open-source software for the processing, analysis and visualisation of fisheries logbook and VMS data. Fish. Res. 115, 31–43. doi: 10.1016/j.fishres.2011.11.007
Hogg, M. M., Tendall, O. S., Conway, K. W., Pomponi, S. A., Van Soest, R. W. M., Gutt, J., et al. (2010). Deep-Sea Sponge Grounds: Reservoirs of Biodiversity. Amsterdam: University of Amsterdam.
ICES (2011). Report of the ICES/NAFO Joint Working Group on Deep-water Ecology (WGDEC), 28 February–4 March. Copenhagen: ICES.
ICES (2012). Report of the ICES∖NAFO Joint Working Group on Deep-water Ecology (WGDEC), 26-30 March 2012. Copenhagen: ICES.
ICES (2013a). Report of the ICES∖NAFO Joint Working Group on Deep-water Ecology (WGDEC), 11–15 March 2013. Copenhagen: ICES.
ICES (2013b). “Vulnerable deep-water habitats in the NEAFC regulatory area,” in Proceedings of the ICES Advisory Committee, 2013 (Copenhagen: ICES).
ICES (2015). Report of the ICES∖NAFO Joint Working Group on Deep-water Ecology (WGDEC), 16–20 February 2015. Copenhagen: ICES.
ICES (2016a). Report of the Joint ICES/NAFO Working Group on Deep-water Ecology (WGDEC), 15–19 February 2016. Copenhagen: ICES.
ICES (2016b). Report of the Workshop on the Vulnerable Marine Systems Database (WKVME), 10–11 December 2015, Peterborough: ICES.
Jenks, G. F. (1967). The data model concept in statistical mapping. Int. Yearb. Cartogr. 7, 186–190.
Kenchington, E., Murillo, F. J., Lirette, C., Sacau, M., Koen-Alonso, M., Kenny, A., et al. (2014). Kernel density surface modelling as a means to identify significant concentrations of vulnerable marine ecosystem indicators. PLoS One 9:e109365. doi: 10.1371/journal.pone.0109365
Martin, T. G., Burgman, M. A., Fidler, F., Kuhnert, P. M., Low-Choy, S., Mcbride, M., et al. (2012). Eliciting expert knowledge in conservation science. Conserv. Biol. 26, 29–38. doi: 10.1111/j.1523-1739.2011.01806.x
McIntyre, F. D., Drewery, J., Eerkes-Medrano, D., and Neat, F. C. (2016). Distribution and diversity of deep-sea sponge grounds on the rosemary bank Seamount. NE Atlantic. Mar. Biol. 163:143. doi: 10.1007/s00227-016-2913-z
Molodtsova, T. N., Sanamyan, N. P., and Keller, N. B. (2008). Anthozoa from the northern Mid-Atlantic Ridge and charlie-gibbs fracture zone. Mar. Biol. Res. 4, 112–130. doi: 10.1080/17451000701821744
Mortensen, P. B., Buhl-Mortensen, L., Gebruk, A. V., and Krylova, E. M. (2008). Occurrence of deep-water corals on the Mid-Atlantic ridge based on MAR-ECO data. Deep Sea Res. Part II 55, 142–152. doi: 10.1016/j.dsr2.2007.09.018
Murillo, F. J., Durán-Muñoz, P., Altuna, A., and Serrano, A. (2011). Distribution of deep-water corals of the Flemish Cap, Flemish Pass and the Grand Banks of Newfoundland (Northwest Atlantic Ocean): interaction with fishing activities. ICES J. Mar. Sci. 68, 319–332. doi: 10.1093/icesjms/fsq071
Murillo, F. J., Durán-Munoz, P., Cristobo, F. J., Rios, P., Gonzalez, C., Kenchington, E., et al. (2012). Deep-sea sponge grounds of the Flemish Cap, Flemish Pass and the Grand Banks of Newfoundland (Northwest Atlantic Ocean): distribution and species composition. Mar. Biol. Res. 8, 842–854. doi: 10.1080/17451000.2012.682583
Neat, F. C., Jamieson, A. J., Stewart, H. A., Narayanaswamy, B. E., Collie, N., Stewart, M., et al. (2018). Visual evidence of reduced seafloor conditions and indications of a cold-seep ecosystem from the Hatton–Rockall basin (NE Atlantic). J. Mar. Biol. Assoc. U.K. 1–7. doi: 10.1017/S0025315418000115
Portela, J., Acosta, J., Cristobo, J., Muñoz, A., Parra, S., Ibarrola, T., et al. (2012). “Management strategies to limit the impact of bottom trawling on VMEs in the high seas of the SW Atlantic,” in Marine Ecosystems, ed. A. Cruzado (Rijeka: InTech), 199–228. doi: 10.5772/34610
Portela, J. M., Pierce, G. J., del Río, J. L., Sacau, M., Patrocinio, T., and Vilela, R. (2010). Preliminary description of the overlap between squid fisheries and VMEs on the high seas of the Patagonian Shelf. Fish. Res. 106, 229–238. doi: 10.1016/j.fishres.2010.06.009
Ramirez-Llodra, E., Brandt, A., Danovaro, R., De Mol, B., Escobar, E., German, C., et al. (2010). Deep, diverse and definitely different: unique attributes of the world’s largest ecosystem. Biogeosciences 7, 2851–2899. doi: 10.5194/bg-7-2851-2010
Rees, T. (2003). “C-squares”, a new spatial indexing system and its applicability to the description of oceanographic datasets. Oceanography 16, 11–19. doi: 10.5670/oceanog.2003.52
Rengstorf, A. M., Mohn, C., Brown, C., Wisz, M. S., and Grehan, A. J. (2014). Predicting the distribution of deep-sea vulnerable marine ecosystems using high-resolution data: considerations and novel approaches. Deep Sea Res. Part I 93, 72–82. doi: 10.1016/j.dsr.2014.07.007
Rengstorf, A. M., Yesson, C., Brown, C., and Grehan, A. J. (2013). High-resolution habitat suitability modelling can improve conservation of vulnerable marine ecosystems in the deep sea. J. Biogeogr. 40, 1702–1714. doi: 10.1111/jbi.12123
Roberts, J. M., Henry, L. A., Long, D., and Hartley, J. P. (2008). Cold-water coral reef frameworks, megafaunal communities and evidence for coral carbonate mounds on the Hatton Bank, north east Atlantic. Facies 54, 297–316. doi: 10.1007/s10347-008-0140-x
Sayago-Gil, M., Long, D., Hitchen, K., Díaz-del-Río, V., Fernández-Salas, L. M., and Durán-Muñoz, P. (2010). Evidence for current-controlled morphology along the western slope of Hatton Bank (Rockall Plateau, NE Atlantic Ocean). Geo Mar. Lett. 30, 99–111. doi: 10.1007/s00367-009-0163-5
Taranto, G. H., Kvile, K. Ø, Pitcher, T. J., and Morato, T. (2012). An ecosystem evaluation framework for global seamount conservation and management. PLoS One 7:e42950. doi: 10.1371/journal.pone.0042950
UNGA (2006). Resolution 61/105. Sustainable Fisheries, Including Through the 1995 Agreement for the Implementation of the Provisions of the United Nations Convention on the Law of the Sea of 10 December 1982 Relating to the Conservation and Management of Straddling Fish Stocks and Highly Migratory Fish Stocks, and Related Instruments. Available at: http://www.un.org/Depts/los/general_assembly/general_assembly_reports.htm
Vierod, A. D. T., Guinotte, J. M., and Davies, A. J. (2014). Predicting the distribution of vulnerable marine ecosystems in the deep sea using presence-background models. Deep Sea Res. Part II 99, 6–18. doi: 10.1016/j.dsr2.2013.06.010
Wallace, B. P., DiMatteo, A. D., Bolten, A. B., Chaloupka, M. Y., Hutchinson, B. J., Abreu-Grobois, F. A., et al. (2011). Global conservation priorities for marine turtles. PLoS One 6:e24510. doi: 10.1371/journal.pone.0024510
Weaver, P. P. E., Benn, A., Arana, P. M., Ardron, J. A., Bailey, D. M., Baker, K., et al. (2011). The Impact of Deep-Sea Fisheries and Implementation of the UNGA Resolutions 61/105 and 64/72. Report of an international scientific workshop. Southampton: National Oceanography Centre.
Wienberg, C., Beuck, L., Heidkamp, S., Hebbeln, D., Freiwald, A., Pfannkuche, O., et al. (2008). Franken Mound: facies and biocoenoses on a newly-discovered “carbonate mound” on the western Rockall Bank, NE Atlantic. Facies 54, 1–24. doi: 10.1007/s10347-007-0118-0
Wilson, J. B. (1979). Patch’ development of the deep-water coral Lophelia pertusa (L.) on Rockall Bank. J. Mar. Biol. Assoc. U.K. 59, 165–177. doi: 10.1017/S0025315400043459
WoRMS Editorial Board (2017). World Register of Marine Species. Available at: http://www.marinespecies.org at VLIZ.
Keywords: vulnerable marine ecosystems, deep-sea, multi-criteria assessment, cold-water corals, deep-sea sponges
Citation: Morato T, Pham CK, Pinto C, Golding N, Ardron JA, Durán Muñoz P and Neat F (2018) A Multi Criteria Assessment Method for Identifying Vulnerable Marine Ecosystems in the North-East Atlantic. Front. Mar. Sci. 5:460. doi: 10.3389/fmars.2018.00460
Received: 11 May 2018; Accepted: 14 November 2018;
Published: 13 December 2018.
Edited by:
Anthony Grehan, National University of Ireland Galway, IrelandReviewed by:
Lorenzo Angeletti, Istituto di Scienze Marine (ISMAR), ItalyLenaick Menot, Institut Français de Recherche pour l’Exploitation de la Mer (IFREMER), France
Copyright © 2018 Morato, Pham, Pinto, Golding, Ardron, Durán Muñoz and Neat. This is an open-access article distributed under the terms of the Creative Commons Attribution License (CC BY). The use, distribution or reproduction in other forums is permitted, provided the original author(s) and the copyright owner(s) are credited and that the original publication in this journal is cited, in accordance with accepted academic practice. No use, distribution or reproduction is permitted which does not comply with these terms.
*Correspondence: Telmo Morato, dC5tb3JhdG9AZ21haWwuY29t